- 1School of Electronics and Information Engineering, Guangdong Ocean University, Zhanjiang, China
- 2Guangdong Provincial Key Laboratory of Inteligent Equipment for South China Sea Marine Ranching, Zhanjiang, China
With the growing global population and economic development, the demand for sashimi has increased, presenting both new opportunities and challenges for aquaculture. As a key species for sashimi, Greater Amberjack faces significant potential in aquaculture but is also vulnerable to temperature fluctuations, particularly during its juvenile stage, which can lead to abnormal behaviors. These behavioral anomalies, if undetected, can impede growth and result in substantial economic losses. Traditional methods for detecting abnormal behavior rely heavily on manual inspection, a process that is time-consuming and labor-intensive. Meanwhile, existing automated detection algorithms often struggle with a trade-off between detection accuracy and model size. To address this issue, we propose a precise and lightweight model for detecting Greater Amberjack’s abnormal behaviors, based on the YOLOv8n architecture (named GAB-YOLO). First, we introduce the SobelMaxDS module, designed to enhance the network’s ability to extract edge and spatial features, thereby enabling more effective capture of the fish’s behavioral contours and preserving rich target information. This enhancement improves the model’s robustness against challenges such as image blurring, occlusion, and false detections in complex environments. Additionally, the PMSRNet module is integrated into the backbone network to replace C2f, improving the model’s feature extraction capabilities through multi-scale feature fusion and enhanced spatial information capture, which aids in the accurate localization of the fish target.Furthermore, by incorporating shared decoupled heads for classification and regression features, alongside GroupConv and DBB(Diverse Branch Block) modules in the detection head, we significantly reduce the model’s parameter count while simultaneously improving its accuracy and robustness. Finally, the introduction of the Wise-ShapeIoU loss function further accelerates the model’s convergence and optimization process. Experimental results demonstrate that, compared to the original model, the number of parameters and FLOPs are reduced by 36.7% and 28.4%, respectively, while the Precision is increased by 5.1%. The model achieves a detection speed of 172 frames per second, outperforming other mainstream detection models. This study addresses the real-time detection requirements for Greater Amberjack’s abnormal behaviors in aquaculture and offers considerable practical value for fish farming operations.
1 Introduction
Greater Amberjack (Seriola dumerili) is a species of fish primarily found in the upper layers of the ocean, distributed mainly in tropical and subtropical waters (Shi et al., 2024). Due to its excellent meat quality and nutritional value, particularly as sushi and sashimi, Greater Amberjack is highly valued by consumers, and its market demand has significantly increased in recent years (Pinheiro et al., 2024). This species is also known for its rapid growth and strong adaptability to various environmental conditions, making it a prime candidate for deep-sea cage farming (Tone et al., 2022). However, before being transferred to cage farming systems, juvenile fish must be raised in recirculating aquaculture systems until they reach a certain size, which requires precise monitoring of their health status (Barany et al., 2021).In industrial aquaculture, high-density fish farming, combined with the complex and uncontrollable underwater environment, can expose juvenile fish to a variety of abnormal conditions such as diseases, hypoxia, pollution, parasites, and temperature fluctuations, all of which can lead to abnormal behaviors. These abnormal behaviors may result in a decline in both the quantity and quality of the fish, causing substantial economic losses to the aquaculture industry (Zhao et al., 2021). Therefore, it is essential to detect abnormal behaviors in fish quickly and accurately to mitigate these risks and ensure the sustainable development of aquaculture.
For aquaculture operators, timely health monitoring of fish populations is critical (Mandal and Ghosh, 2024). However, traditional farming methods often rely on manual observation, which is not only inefficient but also difficult to maintain on a 24/7 basis. This results in missed detections of abnormal behavior, potentially leading to large-scale fish mortality and significant financial losses (Li et al., 2024b). In recent years, with the advancement of computer vision technology and the rise of intelligent aquaculture, many researchers have applied computer vision techniques to aquaculture (Liu et al., 2023). By utilizing computer analysis and processing of images or videos, some of the drawbacks of traditional methods can be overcome, ultimately reducing economic losses. For example, Yu et al. (2021) employed Harris corner detection to extract specific behavioral features, followed by the Lucas-Kanade optical flow method to determine the swimming speed of carp in sub-images, allowing them to assess whether the swimming speed of the fish school was abnormal. However, this approach relies on traditional computer vision techniques that require manual feature extraction algorithms and have certain limitations. These methods often struggle with large, diverse datasets and complex recognition tasks, leading to suboptimal performance and accuracy in practical applications. Moreover, in high-density aquaculture, issues such as overlapping individuals, partial occlusions, and image blurring caused by rapid fish movement frequently arise. Consequently, it is necessary to explore more advanced and effective techniques.
Deep learning, a machine learning technique based on neural networks, has proven to be highly effective in extracting high-level features for recognition and classification. It demonstrates strong performance and robustness, making it widely applicable for detecting abnormal fish behavior (Kaur et al., 2023; Yang et al., 2021). The rapid development of deep learning has opened new possibilities for fish behavior detection. In recent years, numerous visual models have emerged that help address many challenges in aquaculture (Zhao et al., 2025). For example, Chen et al. (2022) introduced a two-stage, ImageNet-pretrained deep learning model to identify abnormal appearances in three types of grouper fish. Their model achieved high accuracy in classifying abnormal and normal fish. However, this study focused primarily on detecting specific external appearance features of the fish and neglected the health-related information conveyed by their behavior. Although the two-stage algorithm is effective, it is difficult to achieve real-time performance on devices with limited computational resources. In contrast, single-stage algorithms, such as those in the YOLO series (Jiang et al., 2022), predict directly on images, eliminating the candidate region generation phase. This results in higher speeds and gradually superior accuracy compared to two-stage algorithms. To detect dead fish based on belly-up behavior, Zhou et al. (2025) employed an improved lightweight CSPDarknet53 as the backbone network to reduce the number of parameters. By integrating a ReLUMemristor-like activation function into the YOLOv4 model, they improved the accuracy of dead fish detection. However, a limitation of this approach is that it can only detect dead fish and cannot predict the health condition of fish before they die.
In aquaculture, the high density of fish often leads to overlapping and partial occlusion among individuals. Wang et al. (2022b) proposed an improved YOLOv5 model for detecting and tracking abnormal fish behavior. By integrating diverse features and adding feature maps, this model demonstrated enhanced detection of small targets within fish schools, significantly improving its effectiveness in ideal environments. However, further improvements are required to boost detection accuracy in complex industrial aquaculture settings. Subsequently, to address issues such as image blurring induced by the swift movement of fish in dynamic environments, Wang et al. (2022a) proposed an enhanced architecture based on YOLOv5. This approach integrates a multi-head attention mechanism into the backbone network, combines BiFPN (Bidirectional Feature Pyramid Network) for weighted feature fusion, and employs CARAFE (ContentAware ReAssembly of FEatures) upsampling operators to replace conventional upsampling methods. These improvements enhanced the model’s feature extraction and fusion, enabling it to better handle challenges such as small target sizes, severe occlusion, and blurring in real-world fish school images; however, its ability to capture the edges and fine details of fish remains insufficient. Li et al. (2024a) and colleagues designed a multi-task learning network, YOLO-FD, for detecting fish infected with Nocardiosis through segmentation and detection. However, this approach has limited applicability since it is specifically designed for detecting Nocardiosis infections and lacks generalization for other types of fish health conditions. On the other hand, Wang et al. (2023) applied an improved YOLOX-S algorithm to detect abnormal fish behavior in complex aquatic environments. They enhanced detection accuracy by integrating coordinate attention, but this increase in network weight and parameters reduced the detection speed, making it unsuitable for real-time applications (Zhao et al., 2024). proposed a fish behavior recognition and visualization framework based on the Slowfast network and spatiotemporal graph convolution networks (ST-GCN), which improved the ability to detect abnormal behaviors from spatial information. However, the technology proposed in these studies is limited because it only addresses the extraction of spatiotemporal network features while neglecting the extraction of image contour edge information. Furthermore, all these studies are designed to distinguish between normal and abnormal fish schools, lacking the capability to specifically detect and analyze various types of abnormal behaviors.
Based on the above analysis, in practical aquaculture, fish schools often exhibit high-density aggregation with significant overlap among individuals, resulting in severe occlusion of some fish. Moreover, due to water current interference, camera shake, and rapid fish movement in underwater environments, images frequently suffer from motion blur and dynamic scene changes, which greatly increase the difficulty of object detection. Existing object detection methods—such as the traditional YOLO series and Faster R-CNN—generally struggle with insufficient edge information extraction (Lin et al., 2025), blurred local features, and difficulties in handling multi-scale targets under these conditions, leading to a significant decline in both detection precision and recall in high-density, dynamic, and heavily occluded scenarios. Furthermore, achieving a balance between precision and model size remains challenging, as current models often fail to maintain high accuracy while delivering rapid detection. To address these issues, this study focuses on Greater Amberjack fish and proposes an improved YOLOv8 model—GAB-YOLO—for real-time detection of abnormal behaviors in juvenile Greater Amberjack under different temperature conditions. The main contributions of this study are as follows: (1) To address the boundary and motion blur caused by fish swimming in water, we design the SobelMax module to enhance the network’s ability to extract edge and spatial features, enabling the model to better capture the contours of fish behavior and retain rich target information, thereby improving its ability to detect boundary and motion blur in images.
(2) The proposed PMSRNet module enhances the model’s detection capability in complex environments by incorporating multi-scale feature fusion and capturing rich spatial information, which helps tackle challenges such as fish body deformation, occlusion, and false detections.
(3) To balance the trade-off between accuracy and model size, we introduce the RLSHead detection head, which not only reduces the parameter count in the decoupled heads but also enhances the model’s global perception of target information, allowing for more accurate detection of small targets without introducing additional inference delay.
The structure of this paper is as follows: Section 2 introduces the model used in this experiment. Section 3 details the experimental setup and dataset construction. Section 4 presents the experimental results and discusses the findings. Finally, Section 5 outlines the conclusions and future directions for this research.
2 Methods
This study proposes a lightweight GAB-YOLO model for real-time monitoring of abnormal behaviors in Greater Amberjack fish in response to temperature changes, as illustrated in Figure 1. Initially, the SobelMax feature extraction module is designed to replace certain convolution (Conv) modules. This module uses the Sobel operator to extract edge features, which are then integrated with the broader spatial information to provide richer semantic context.Next, the PMSRNet module reduces the number of channels in the feature map by half, splitting the feature map into two branches. One branch performs complex multi-scale convolutions, while the other passes the features directly through. These two branches are then combined to integrate all features, thereby enhancing the model’s performance while maintaining its lightweight structure. Additionally, shared parameter techniques are employed, introducing GroupNorm convolution and the reparameterizable DBB(Diverse Branch Block) module. These innovations help reduce the number of parameters in the detection head, lower the model’s storage and computation requirements, and improve detection accuracy without adding significant computational cost. Finally, the Wise-ShapeIoU loss function is introduced to replace CIoU, improving the accuracy of bounding box regression. This adjustment allows the model to capture target location information more effectively and improves its bounding box prediction accuracy, enhancing both performance and robustness. A detailed explanation of these modules will be provided in the following subsection.
2.1 SobelMax
In actual fish aquaculture, due to insufficient underwater illumination or turbid water conditions, highspeed fish movement often causes significant boundary and motion blur in the captured images, which greatly impedes timely detection and accurate assessment of fish behavior. Traditional object detection models generally struggle to precisely capture fish contours under such conditions. Moreover, the first two layers of YOLOv8, which primarily extract low-level features such as edges, textures, and perform downsampling, exhibit poor performance when processing blurred images. To address this problem, we designed a structure named SobelMax to replace two convolution modules in the backbone, as shown in Figure 2.
When fish swim in water, boundary and motion blurring between the foreground and background can occur, affecting the capture of behavioral features. By using the SobelConv branch to extract edge information (Hu et al., 2024; Gao et al., 2010), we enhance the edge details in the image, making the fish behavior contours more distinct and improving feature extraction (Vincent and Folorunso, 2009). The SobelConv branch effectively compensates for the detail loss that often occurs when the original convolution modules process image edges. Additionally, this method provides some resistance to noise. We apply the Sobel operator to two 3D convolution layers, which highlight points with significant brightness changes along the horizontal and vertical edges of the image. This significantly reduces the boundary and motion blurring caused by the fish swimming in complex environments, while preserving essential structural features in the image. The SobelConv structure is shown in Figure 3.
In addition to edge information, spatial information within the image is equally crucial. To address this, we employ a max-pooling convolutional method in a parallel branch to preserve critical information in fish behavior feature maps. This approach enables the detector to learn richer image feature representations, thereby preventing false detection issues caused by positional shifts of fish in video sequences. Finally, the features extracted from both branches are fused to enrich the target feature representation.Through this feature fusion mechanism, the model’s robustness and detection accuracy are significantly enhanced, effectively mitigating edge blur and motion blur artifacts arising during image acquisition. This advancement thereby achieves efficient object detection in complex underwater environments.
2.2 PMSRNet
In high-density aquaculture environments, fish schools are arranged extremely densely, resulting in severe occlusion that poses a significant challenge for object detection. To enhance the extraction of fish features, YOLOv8n incorporates the C2f module, which reinforces feature extraction by increasing the number of bottleneck structures. However, this design inevitably introduces redundancy in feature maps and channel information, thereby impacting the overall efficiency and accuracy of the model. Therefore, drawing inspiration from the design philosophy of GhostNet, we propose a new structure called the multi-scale feature PMSRNet, whose architecture is shown in Figure 4.
Han et al. (2020) proposed GhostNet, a lightweight backbone network that utilizes traditional convolutions to extract rich feature information and incorporates efficient linear transformations to generate redundant features. Its architecture is illustrated in Figure 5.
However, the Ghost module mainly relies on linear transformations to generate “ghost” features, which may result in insufficient feature diversity when processing complex images, particularly limiting its ability to capture fine details of multi-scale targets. To address this limitation, we incorporated the efficient feature generation concept of GhostNet into PMSRNet and further optimized the learning strategy for multi-scale features. By deeply coupling and fusing features from different scales, PMSRNet significantly enhances the model’s ability to detect fish targets in complex underwater environments. Specifically, to address issues such as morphological deformation, occlusion, and misdetections that frequently occur during fish movement, we divide the input feature maps into two parts during the feature processing stage. One part undergoes multi-scale convolution operations, focusing on extracting local details to strengthen the feature representation (Lin et al., 2024), thereby enabling the model to capture subtle yet critical textures and edge information in fish behavior (Wang et al., 2016; Xiao et al., 2025). The other part directly retains the original features to ensure that key information is preserved, preventing the loss of small target details during the downsampling process. Finally, a 1x1 convolution layer fuses the features from different scales, while a residual connection adds the input features back to the processed features. This mechanism effectively retains the original information and introduces new multi-scale information, improving the model’s ability to represent complex features. By optimizing the use of computational resources, this approach reduces unnecessary computations and memory access, while lowering the overall model complexity. Moreover, the enhanced feature fusion allows the model to more accurately detect abnormal behavior, improving its ability to handle challenges like fish body deformation, occlusion, and false detection in complex environments.
2.3 RLSHead
YOLOv8 introduces a decoupled Head architecture that separates the classification and detection processes, which enhances its detection performance. However, this design results in a substantial increase in the number of parameters, with the head section alone consisting of 12 layers. Each of the three detection heads includes two 3x3 convolutions and one 1x1 convolution, which could make it challenging to fully leverage YOLOv8’s performance advantages in resource-constrained environments. Furthermore, this separation limits information flow between the two components. To address this challenge, we propose a redesigned, reparameterized lightweight shared convolution detection head, called RLSHead (Reparameterized Shared Lightweight Head), which reduces the number of parameters and computational load, thus improving the model’s speed while maintaining high detection accuracy. The structure of the RLSHead detection head is shown in Figure 6.
After the three feature layers from the neck are passed into the detection head, each branch first undergoes a 1x1 CGS convolution. The CGS module replaces the Batch Normalization (BN) layer in the original CBS convolution module with a Group Normalization (GN) layer (Wu and He, 2018). While BN normalizes by calculating statistics based on the batch size, this can become unstable for small batches, leading to higher error rates. GN overcomes this issue by grouping feature channels and calculating the mean and variance within each group, making normalization independent of the batch size. This ensures stable accuracy across varying batch sizes.
The CGS module standardizes the number of channels, and then all the feature layers are merged into the shared convolution DBB (Ding et al., 2021) for feature extraction. Shared convolutions significantly reduce the parameter count, making the model more lightweight. However, using shared parameters could limit the model’s ability to capture complex patterns, as different features might require different convolution kernels. To mitigate this limitation, we incorporate reparameterized convolution DBB, as shown in Figure 7.
Introducing more learnable parameters allows the network to extract features more effectively, improving its global understanding of target information. This makes the model more accurate when dealing with small targets, compensating for any potential accuracy loss due to the lightweight design. Furthermore, reparameterized convolutions improve parameter utilization significantly and, during inference, perform similarly to standard convolutions, offering a lossless optimization solution. Additionally, to address the issue of varying target scales detected by each detection head, we use a scale layer on the regression branch’s output to rescale features (Tan and Le, 2019), allowing the model to locate fish targets of different sizes. This reparameterized shared convolution structure reduces the model’s parameter count and computational cost while improving performance.
2.4 Wise-ShapeIoU
In industrial aquaculture environments, residual feed and fish waste often cause water turbidity, resulting in low-quality images with unavoidable poor samples. This decreases the model’s ability to generalize. Therefore, a well-designed loss function is crucial for improving detection accuracy and avoiding false or missed detections. YOLOv8 utilizes Distribution Focal Loss (DFL) (Li et al., 2020) and CIoU loss (Zheng et al., 2020) for bounding box regression. While the CIoU loss function considers the overlap area, centroid distance, and aspect ratio in bounding box regression, it exacerbates the penalty for low-quality samples, especially when there are low-quality samples in the training set. This results in the penalty not reflecting the true difference between the ground truth and predicted bounding boxes. The formula for CIoU is shown in Equations (1–3).
In the formula,b and bgt represent the centroids of the anchor box and the target box, respectively. ρ2(b,bgt) represents the Euclidean distance between the centroids of the anchor box and the target box. c is the diagonal length of the minimum bounding box enclosing both the anchor and target. α is the weight parameter, and v is the consistency of the aspect ratio between the two boxes. wgt and hgt represent the width and height of the anchor box, respectively.
To accelerate convergence and improve detection accuracy, this study introduces the WIoU loss function (Tong et al., 2023) to replace CIoU. Unlike traditional loss functions with fixed focusing mechanisms, WIoU considers aspect ratio, centroid distance, and overlap area, and incorporates a dynamic, nonmonotonic focusing mechanism. This helps balance the weights between high and low-quality samples during bounding box regression. In the current version, we use WIoUv3, which adds a non-monotonic focusing coefficient to WIoUv1. The formulas are as follows (Equations 4–6):
x and y are the coordinates of the center of the anchor box, and xgt and ygt represent the coordinates of the center of the target box. Wg and Hg are the width and height of the minimum bounding rectangle that encloses both the anchor and target boxes. RWIoU primarily focuses on the center distance between the anchor and target boxes. For anchor boxes with lower overlap with the target, RWIoU becomes larger.
To better reduce the gradient loss from low-quality samples, dynamic non-monotonic modulation is introduced to create WIoUv3. This method improves the focus on correct samples and prevents large harmful gradients from low-quality samples. The non-monotonic modulation factor is derived from the anchor box’s outlier degree and adjusted using hyperparameters, which enhances the model’s accuracy. As shown in Equations (7–8), represents the monotonic focus factor, β is the outlier degree of the anchor box, α and δ are hyperparameters, and r is the non-monotonic focus factor.
In fish behavior detection, fish close to the camera appear larger in the image, while those farther away appear smaller. In the images or sequences for detection, fish often exhibit variations in scale. The challenge of bounding box scale variation is one of the primary difficulties in fish behavior detection. To adjust the inherent attributes of bounding boxes, such as shape and size, and to enhance the model’s generalization capability across different scenes and its effective deployment in various environments, this study incorporates Shape-IoU (Zhang and Zhang, 2023) into WIoUv3. The Shape-IoU loss function integrates geometric constraints to further refine the accuracy of bounding box regression. The specific formulas are presented in Equations 9–12.
In the formula, B represents the predicted bounding box, and Bgt represents the ground truth bounding box. Scale is the scaling factor, which is related to the size of the targets in the dataset. ww and hh represent the weight coefficients in the horizontal and vertical directions, respectively, and their values depend on the shape of the ground truth box. distanceshape represents the distance between the center of the predicted bounding box and the center of the actual ground truth box. wgt and hgt are the width and height of the ground truth bounding box. When estimating the distance loss, the horizontal and vertical weight constraints of the ground truth bounding box are incorporated. Ωshape denotes the shape loss, which is also derived from SIoU (Gevorgyan, 2022). The bounding box regression loss function is expressed as Equation 13.
Finally, we combine Wise-IoU and Shape-IoU to propose a new loss function, Wise-ShapeIoU. Wise-IoU reduces the impact of low-quality samples on bounding box regression, and Shape-IoU accounts for the effect of shape and aspect ratio on localization accuracy. The Wise-ShapeIoU loss function improves the detection of fish behavior, achieving more accurate localization by addressing the diversity of scales, shapes, and features. Its complete mathematical formulation is formally defined in Equation 14.
3 Materials
3.1 The aquaculture management of greater amberjack
The experiments in this study were conducted at the Zhanjiang Bay Laboratory in Zhanjiang, Guangdong Province, using a total of 200 greater amberjack fish as experimental subjects. These fish were temporarily housed in several recirculating water tanks (50 cm × 50 cm × 40 cm), each with a water capacity of approximately 100 liters. Prior to the experiment, the fish were acclimated to the experimental environment for one week to adjust to the new conditions. During the experimental period, the researchers fed the fish twice a day, once in the morning and once in the evening, to ensure their health and promote normal growth.
To maintain optimal water quality, each tank was equipped with an automated recirculation system that efficiently removed fish excrement. After being processed by a biological filter, the water was returned to the tank. The dissolved oxygen level in the water was maintained above 8.0 mg/L using an oxygen pump to ensure the fish school grew in an ideal water quality environment. The initial water temperature was set to 20°C and kept stable by a control system. Feeding was discontinued 24 hours before the experiment to ensure the fish were in optimal health.
3.2 Experimental design and operation
The experimental setup included 20 recirculating water tanks, a computer, a Tplink network camera, and a camera mount. Each camera had a resolution of 2560×1440 and was capable of capturing video at 25 frames per second. The researchers designed five sets of temperature experiments, each consisting of four water tanks, with 10 fish in each tank, ensuring a moderate fish school density that would not interfere with the experimental results. The initial water temperature was set to 20°C and gradually increased via the heating system, with target temperatures set to 21°C, 24°C, 27°C, 30°C, and 33°C to observe the behavioral changes of the fish under different temperature conditions (Wiles et al., 2020).
The water temperature for each experimental group was controlled by the system, with the temperature increasing by 1°C every 4 hours until the target temperature was reached. Experimental data were recorded using Tplink’s TL-IPC44B series network cameras, and all video footage was stored on a Hikvision DVR. The cameras were positioned directly in front of the tanks and carefully adjusted to ensure full coverage of the tank’s activity area. After the experiment, the video footage was reviewed to analyze the behavior of the fish and further investigate the effects of temperature variations on their activity.The experimental setup is shown in Figure 8.
3.3 Observation of anomalous behavior
As ectothermic animals, fish experience body temperature fluctuations in response to changes in water temperature, which directly impacts their physiological activities and behavioral patterns (Abram et al., 2017). Juvenile fish, in particular, exhibit lower vitality and are highly sensitive to water temperature changes, often manifesting distinct abnormal behaviors (Li et al., 2022; Trippel, 1995). This experiment aims to observe behavioral changes in fish under varying temperature conditions, facilitating the early detection of potential health risks and preventing large-scale mortality events.
In low-temperature environments, Greater Amberjack exhibit a marked reduction in activity levels. Many fish remain stationary at the bottom of the tank for prolonged periods, with minimal movement, and some display irregular swimming patterns. Conversely, in high-temperature environments, certain fish exhibit upward swimming behavior, tilting their heads upward, likely in an attempt to find more comfortable positions in response to excessive water temperatures. These observed abnormal behaviors not only provide critical training data for object detection algorithms but also offer practical insights for temperature regulation in aquaculture management.
3.4 DataSets
The image dataset for object detection in this study was derived from videos recorded during experiments. A TP-Link TL-IPC44B camera (resolution: 2560×1440, frame rate: 25 FPS) was positioned vertically to cover the entire activity area of the aquarium. Continuous 30-day recordings encompassed fish behaviors during morning and evening periods, including hypoxia-associated swimming patterns (characterized by upward orientation with head toward the tank surface) and benthic resting behavior (defined as stationary positioning at the tank bottom exceeding 30 seconds). Videos were captured at 20 frames per second (FPS). Key frames were extracted at 2-second intervals from each video, followed by manual screening and image normalization to 640×640 resolution, ultimately yielding 1,312 images for model training.Given the homogeneous experimental background, multiple data augmentation techniques were implemented to enhance dataset diversity. These included affine transformations, random cropping, horizontal flipping, Gaussian noise injection, and random adjustments to brightness and contrast. Through these methods, the dataset was augmented to 2,893 images for subsequent analysis.To ensure the effectiveness of training our deep learning model, the collected dataset of fish abnormal behavior images was randomly divided into training, validation, and testing sets using a 7:2:1 ratio. The training set is used to continuously update and optimize model parameters, the validation set serves to evaluate performance and adjust hyperparameters during training, and the testing set is employed to assess the model’s generalization ability upon completion of training. All image data were annotated using the LabelImg tool, which included the target locations of abnormal fish behavior. After the annotations were completed, XML files were generated. These annotation data provided the necessary foundation for subsequent deep learning model training, aiding in improving the model’s accuracy and robustness.
3.5 Experimental environment and parameter settings
All experiments were conducted on the same computer, with hardware configuration including a 13th Gen Intel(R) Core(TM) i7-13700KF processor and NVIDIA GeForce RTX 4070 Ti SUPER GPU, and the operating system was Windows 10. To thoroughly evaluate the model’s performance, the experiment ran for a total of 300 training epochs, with a batch size of 32 and a learning rate set to 0.01. The SGD optimizer was used to optimize the training process, ensuring the model meets the fast detection requirements while maintaining high accuracy.
By training the fish behavior data under different temperature conditions, the experiment aimed to achieve high detection accuracy to enable real-time monitoring of abnormal fish behavior and provide alerts. This training process is of great significance for validating the application of object detection algorithms in real-world aquaculture environments.
4 Results and analysis
4.1 Evaluation metrics
In this study, the proposed model is comprehensively evaluated using six established metrics: Precision
(P), Recall (R), mean Average Precision (mAP), Giga Floating-point Operations (GFLOPs), parameter count, and Frames Per Second (FPS). Specifically, P quantifies the classification accuracy of positive samples, while R measures their retrieval effectiveness. The mAP metric provides a holistic assessment of detection precision across all object categories. Computational complexity is characterized by GFLOPs, and model compactness is reflected in the parameter count. Additionally, FPS serves as a critical indicator of real-time processing capability. These metrics collectively align with standard evaluation protocols for object detection tasks. The formulas are as follows: Equations 15–18.
4.2 Comparison of model improvements
The complex underwater environment and high fish density are critical factors affecting aquatic target detection. When the density of the fish school is too high, occlusion between the fish and motion blur caused by rapid swimming can make the target difficult to identify, reducing the performance of detection algorithms. Moreover, unclear images of fish schools and the varying sizes of the targets further complicate fish school detection. In this study, we performed a detailed comparison of the Precision and mAP@50 for detecting two abnormal behaviors using the GAB-YOLO and YOLOv8n models. The experimental results are presented in Figure 9. Compared to the original model, GAB-YOLO significantly improved the detection accuracy, with Precision for the two abnormal behaviors increasing by 2.9% and 7.3%, respectively. For mAP@50, our model showed an improvement in detecting “hypoxic behavior” (increased from 91.1% to 91.9%, a 0.8% increase) and in detecting Bottom-lying behavior (from 87.7% to 88.1%, a 0.4% increase). These improvements demonstrate that our model offers higher accuracy in detecting these two abnormal behaviors in Greater Amberjack (Seriola dumerili). Additionally, the overall accuracy has been enhanced, indicating that our efforts in optimizing the model for the aquaculture environment of Greater Amberjack have been successful.
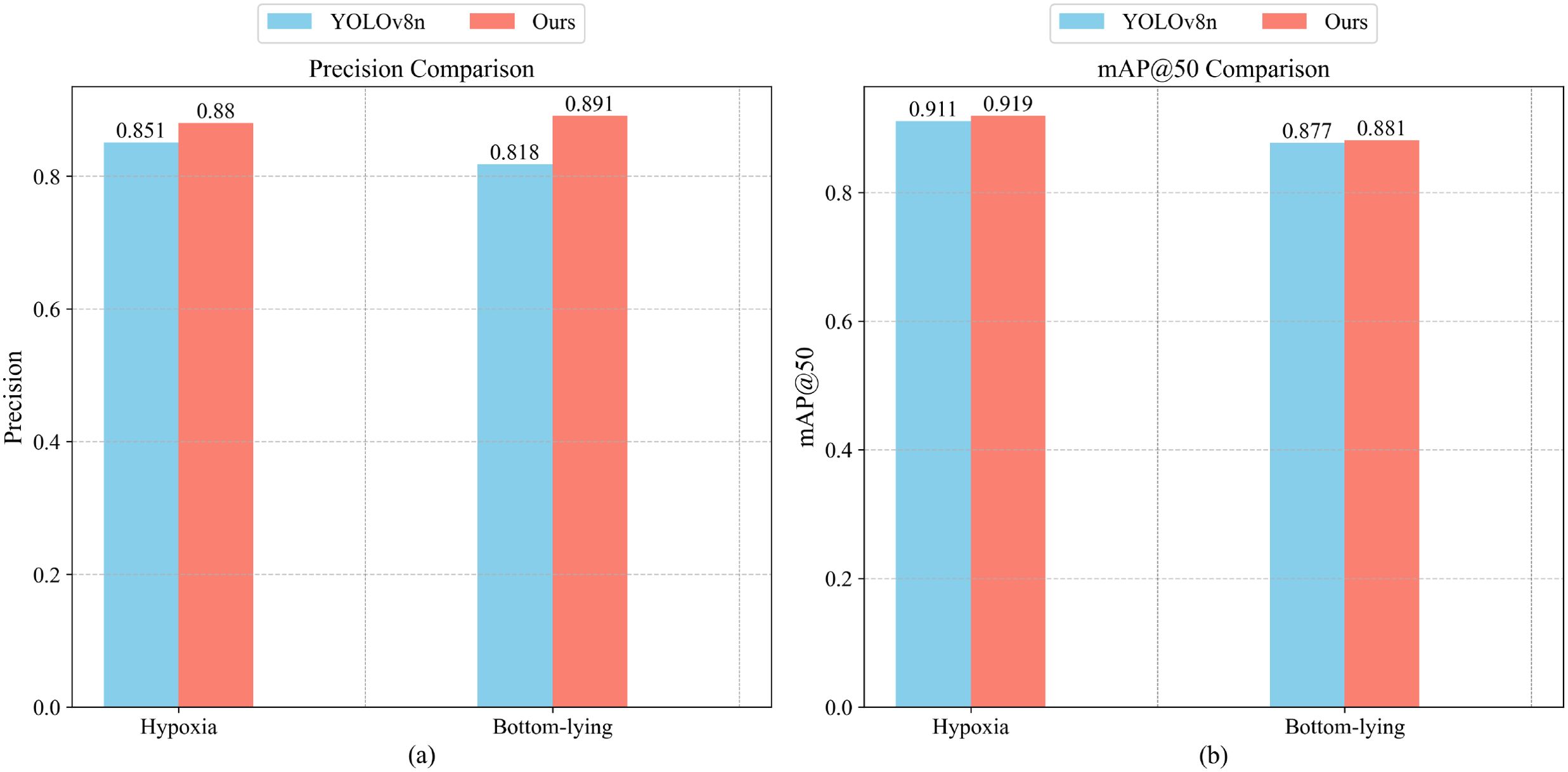
Figure 9. (a, b) respectively illustrate the precision and mAP@50 comparisons between Ours and YOLOv8n.
4.3 Ablation experiments
GAB-YOLO is based on an improved YOLOv8 model. To evaluate the accuracy of our proposed abnormal behavior detection model, we performed validation in seven different ways while maintaining consistent initial hyperparameters throughout each training stage. The impact of adding different modules to YOLOv8n’s performance is shown in Table 1.
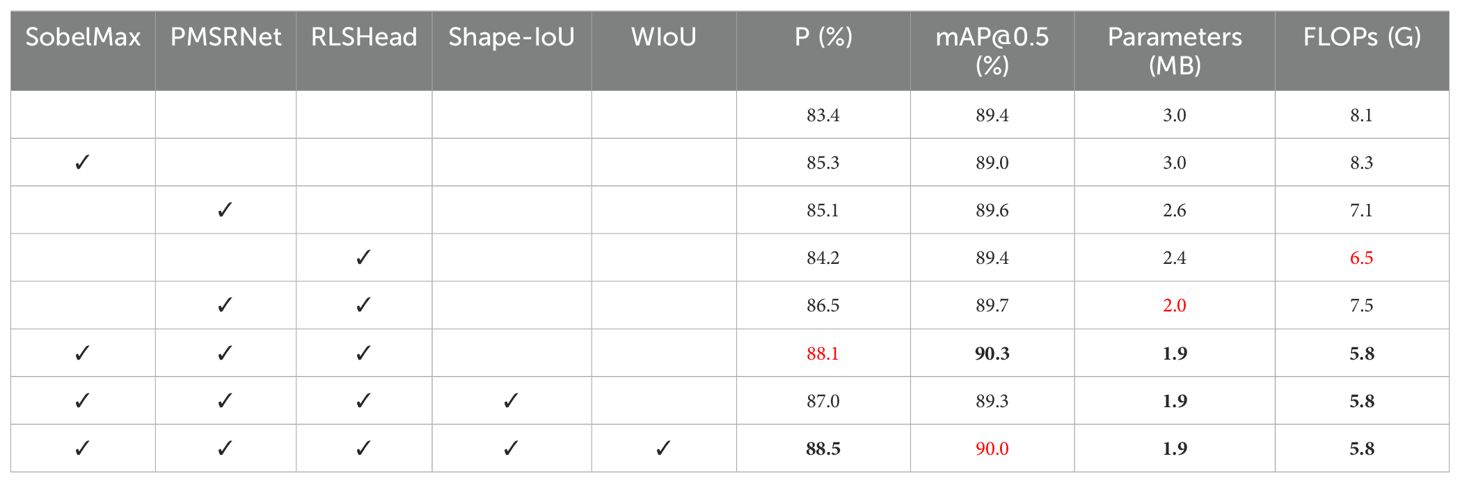
Table 1. Ablation study on detection performance and complexity, with the best results highlighted in bold, and the second-best results highlighted in red.
We first introduced the SobelMax, PMSRNet, and RLSHead modules into the model and compared the enhanced version with the original model. The results showed an increase in detection accuracy by 1.9%, 1.7%, and 0.8%, respectively, indicating significant improvements from each module. The SobelMax and PMSRNet modules contributed the most to the performance gains. The SobelMax module extracts edge information from images and integrates it with spatial data, allowing the model to better capture fish behavior contours and retain rich target information. This improvement enhances the model’s ability to handle boundary and motion blur, as well as to detect occluded and overlapping targets in complex underwater environments. The PMSRNet module, based on partial convolution, applies multiscale convolutions to part of the information, enriching the model’s ability to extract fine details. The remaining information is passed directly, retaining the richness of the original features, and then fused together. This approach enhances the model’s ability to detect various abnormal behaviors in fish schools and reduces redundancy.
The RLSHead module not only improved detection accuracy but also minimized model parameters, thanks to the use of shared convolution and the introduction of the DBB module. The shared convolution allows the model to interactively regress classification information while making the model more lightweight. Meanwhile, the DBB module extracts both low-level and high-level feature maps from the input image and fuses them, utilizing the image’s fine details and semantic data to prevent accuracy loss and improve small target detection.
Building on the advantages of the lightweight RLSHead module, we incorporated the PMSRNet and SobelMax modules. Experiments show that these three modules are highly complementary, significantly improving the model’s accuracy, parameter size, and FLOPs.
Lastly, loss functions play an essential role in enhancing model performance without introducing additional complexity. We introduced Shape-IoU and WIoU, and the results showed that their combination can better handle the diversity of scales, shapes, and features in fish behavior, leading to more accurate localization without increasing complexity. Ultimately, the GAB-YOLO model was developed, reducing the model’s parameters and FLOPs by 36.7% and 28.4%, respectively, while improving Precision by 5.1%. This demonstrates the significant impact of the proposed improvements on model optimization.
4.4 Model comparison
To further evaluate the detection performance of the proposed model, we compared it with several mainstream object detection algorithms, including SSD (Liu et al., 2016), RetinaNet (Lin, 2017), Mobilenet_v3-YOLO (Howard et al., 2019; Bochkovskiy et al., 2020), YOLOv5n, YOLOX-tiny, YOLOv7tiny, YOLOv9t (Wang et al., 2025), YOLOv10n (Wang et al., 2024), and YOLOv11n (Khanam and Hussain, 2024). The evaluation metrics included Precision, Recall, mAP@50, Parameters, and FLOPs. The experimental results are summarized in Table 2. All benchmark models were retrained and fine-tuned under identical training protocols (SGD optimizer, learning rate of 0.01, 300-epoch duration).
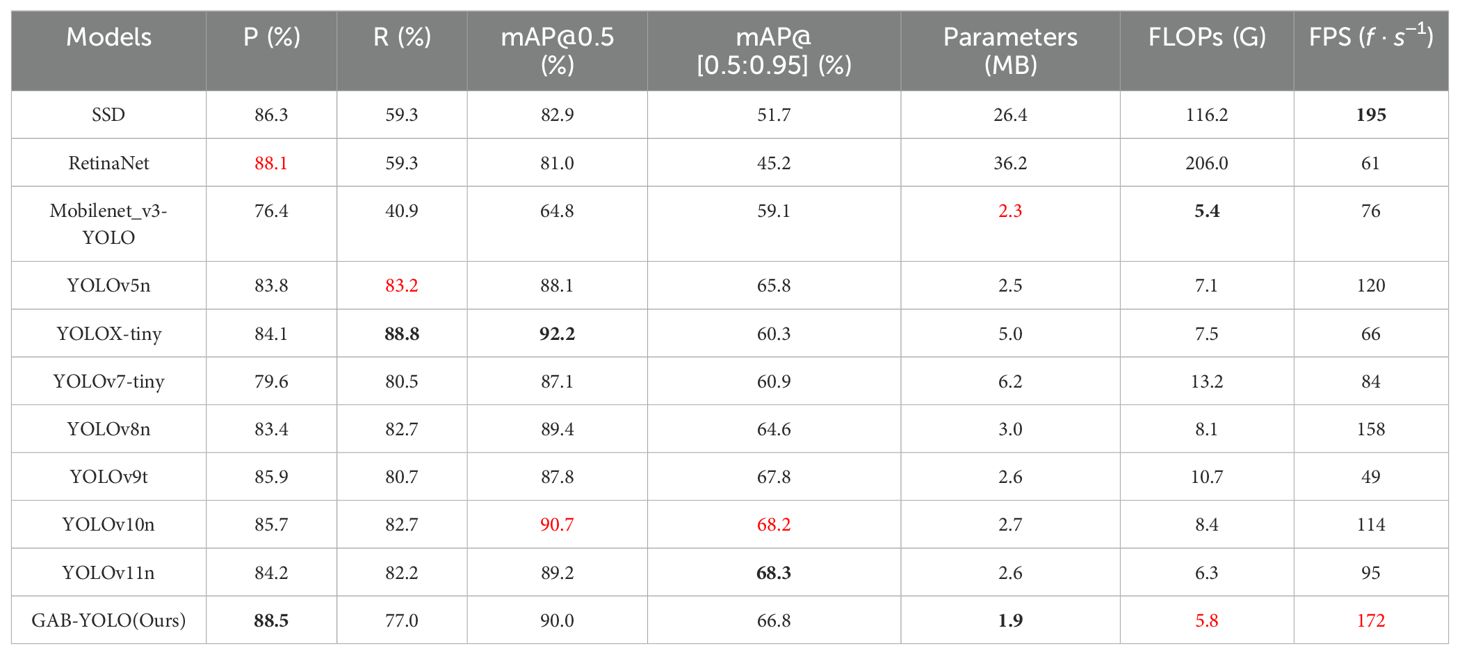
Table 2. Experimental results of Ours and other object detection models on the dataset presented in this paper, with the best results highlighted in bold, and the second-best results highlighted in red.
Compared to single-stage models like SSD and other YOLO variants, our model showed clear improvements across all metrics. Specifically, in terms of Precision, our model outperformed Mobilenet_v3-YOLO by 12.1%, and exceeded YOLOv8n, YOLOv5n, YOLOX-tiny, YOLOv7-tiny, YOLOv9t, YOLOv10n, and YOLOv11n by 5.1%, 4.7%, 4.4%, 8.9%, 2.6%, 2.8%, and 4.3%, respectively. In terms of Parameters, our model is smaller than all other models, with a 36.7% reduction compared to the original YOLOv8n. Regarding FLOPs, our model achieves 5.8G, a 28.4% reduction from the original model. Overall, our model has significant advantages in Precision, Parameters, and FLOPs.This study primarily focuses on achieving a balance between detection accuracy and model lightweight design. GAB-YOLO demonstrates remarkable efficiency advantages: compared with the lightweight models YOLOX-tiny and YOLOv7-tiny, it reduces computational load by 20.5% and 56.1%, respectively, and decreases the parameter count by 62.0% and 69.4%. Additionally, its frame rate is 157.6% higher than that of YOLOX-tiny and 104% higher than that of YOLOv7-tiny. These improvements significantly enhance detection speed and resource utilization, making GAB-YOLO highly competitive for real-time monitoring and edge device deployment, with overall performance that fully meets practical application requirements.
To further evaluate the model’s detection capabilities across different scenarios, we tested it under conditions such as occlusion, varying target scales, and motion blur. As shown in Figure 10, the detection performance comparison of different models reveals that, in occlusion scenarios, YOLOv8n suffered from false negatives, while GAB-YOLO showed better detection accuracy. In the varying target scale scenario, GAB-YOLO demonstrated superior accuracy. For motion blur, YOLOv8n and YOLOv10n showed varying levels of false negatives, while GAB-YOLO performed well. Figure 11 displays the loss curves for the training and validation sets of YOLOv8n, YOLOv9t, YOLOv10n, YOLOv11n, and GAB-YOLO. GAB-YOLO exhibits smaller losses across all aspects compared to YOLOv8n, YOLOv9t, YOLOv10n, and YOLOv11n, which indicates that it effectively reduces false positives and false negatives, resulting in improved recognition accuracy and regression performance. Figure 12 compares Precision, Recall, mAP@0.5, and mAP@[0.5:0.95] for YOLOv8n, YOLOv9t, YOLOv10n, YOLOv11n, and GAB-YOLO, showing that GAB-YOLO significantly outperforms the other models.
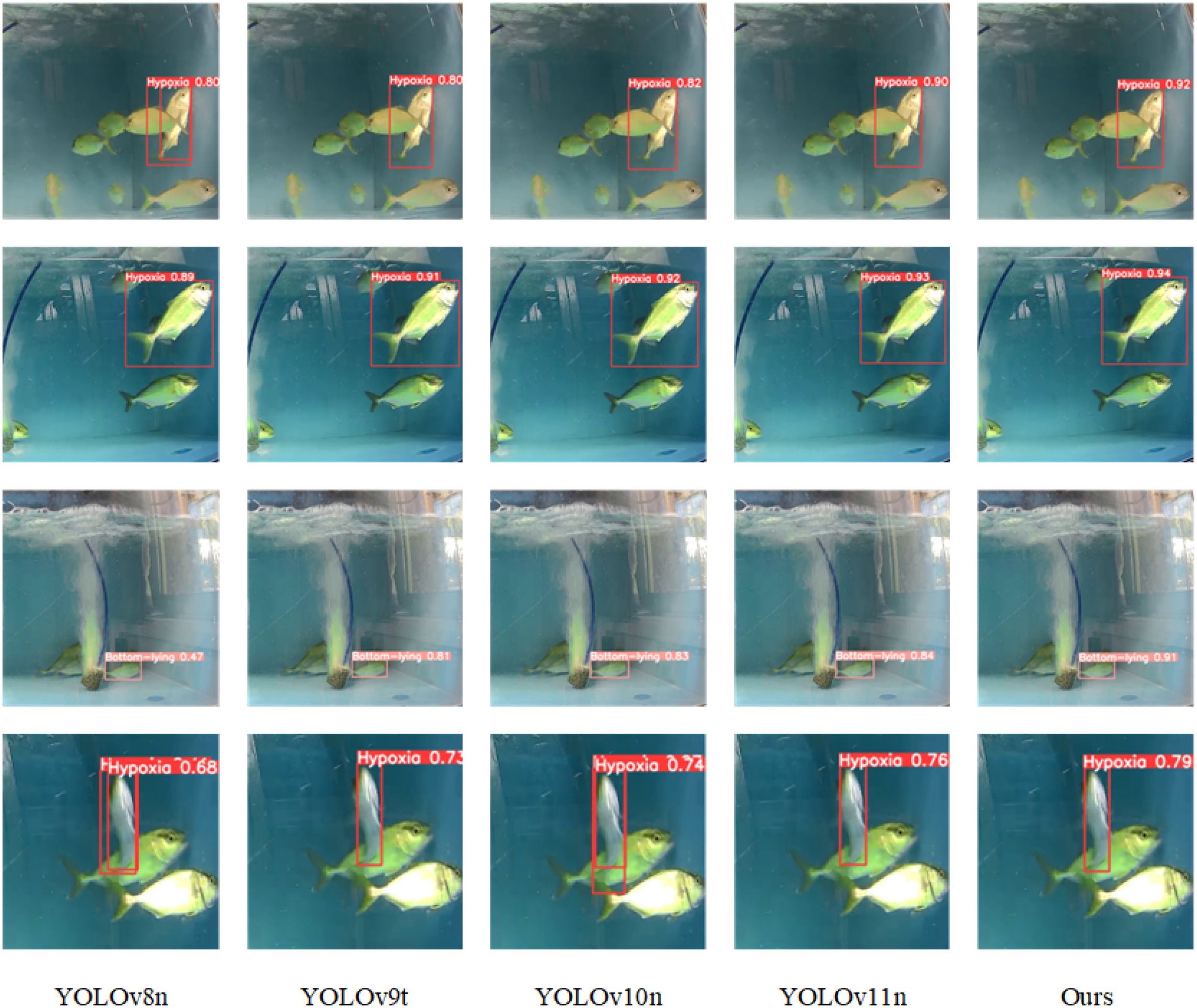
Figure 10. Comparison of detection results of YOLOv8n, YOLOv9t, YOLOv10n, YOLOv11n, and ours in various scenarios.
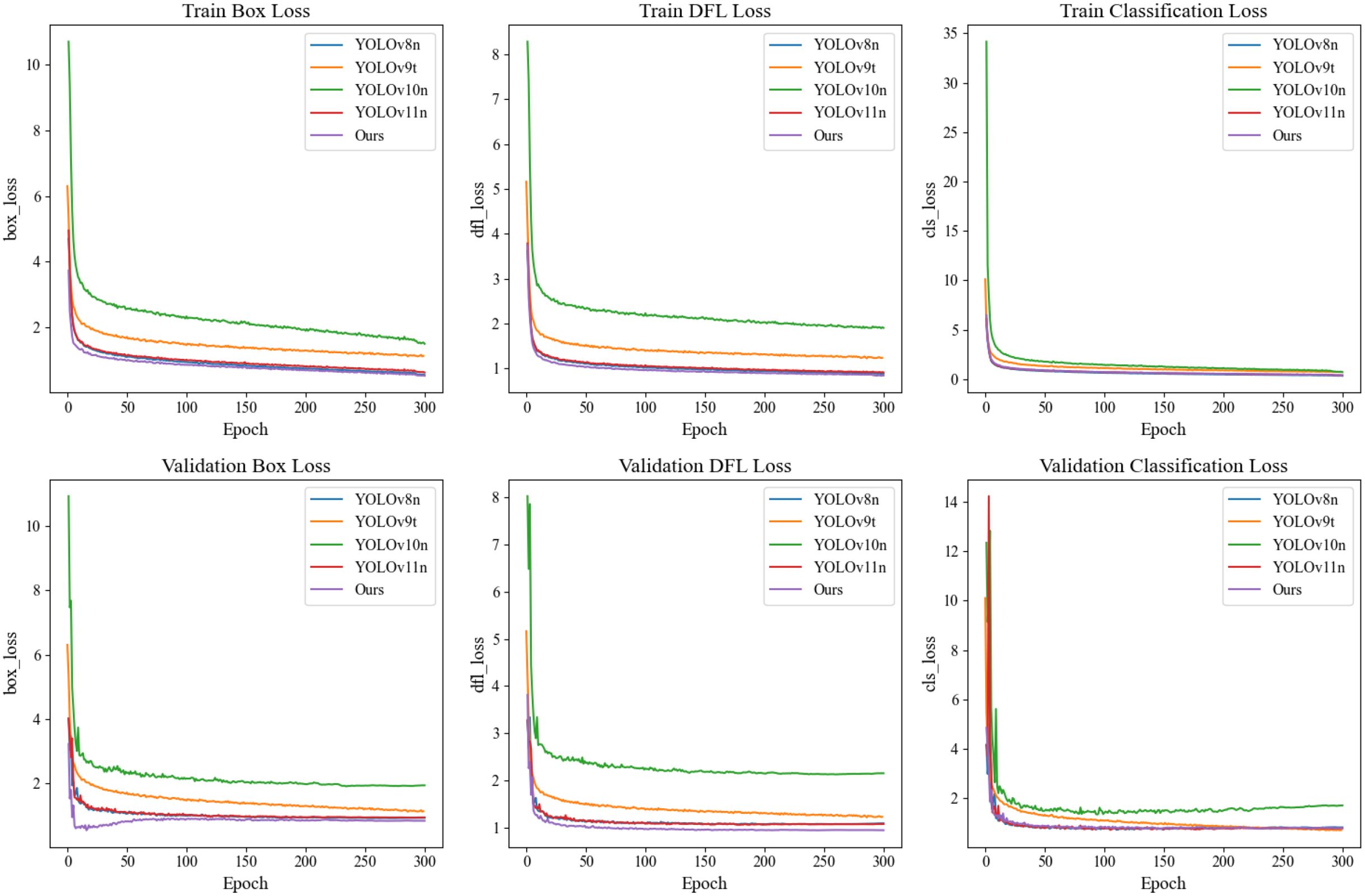
Figure 11. Comparison of bounding box loss, classification loss, and DFL loss values for different models on training and validation sets.
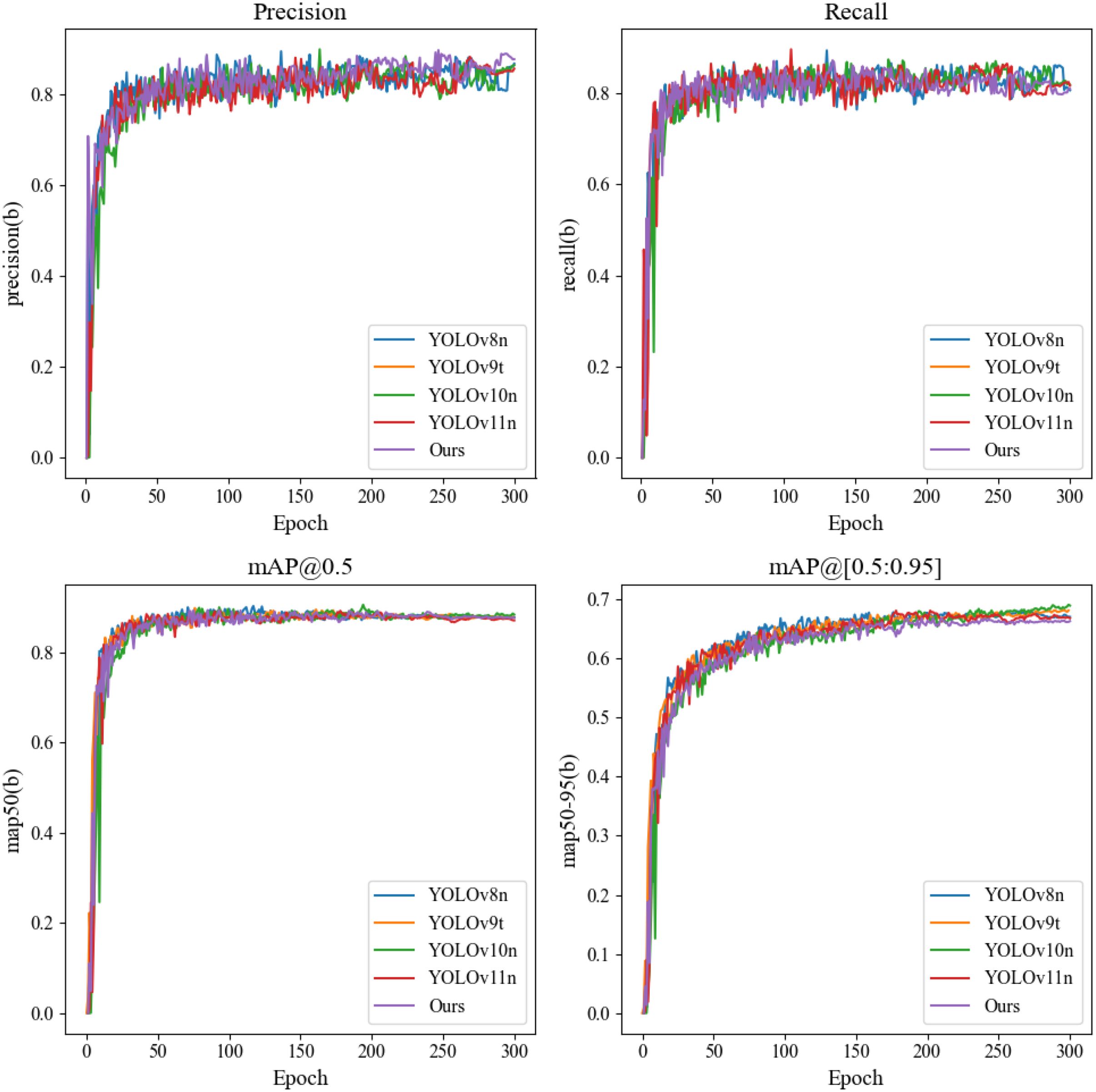
Figure 12. Comparison of fit curves for precision, recall, mAP@0.5, and mAP@[0.5:0.95] for different models.
5 Conclusions
To address challenges such as occlusion, varying target scales, motion blur, and the trade-off between accuracy and model size in detecting abnormal behaviors of juvenile Greater Amberjack fish in aquaculture, we propose a novel network structure, GAB-YOLO, to detect hypoxic and bottom-lying behaviors caused by temperature changes. By incorporating the SobelMax and PMSRNet modules, along with WiseShapeIoU, into YOLOv8n, we enhanced the model’s ability to extract edge and spatial features, capture detailed information, and improve the accuracy of bounding box regression. Additionally, the lightweight RLSHead detection head was introduced, reducing the model’s parameters and FLOPs by 36.7% and 28.4%, respectively, while increasing Precision by 5.1%. Experimental results show that the proposed algorithm can detect hypoxic and bottom-lying behaviors of juvenile Greater Amberjack fish in real-time. The lightweight design makes the algorithm suitable for practical use in aquaculture environments. The model can be deployed on a monitoring platform to identify and assess the severity of abnormal behaviors in juvenile Greater Amberjack fish, enabling aquaculture operators to take timely actions to reduce such behaviors, thus improving yield and reducing losses. Future research will focus on three major directions to further expand and optimize the application potential of the GAB-YOLO model. First, our current work primarily targets abnormal behavior detection in juvenile Greater Amberjack, whose excellent performance is partly due to the uniformity in body shape and behavior within this species. However, significant differences exist among fish species in terms of morphology, swimming patterns, and background conditions, which may limit the direct applicability of the model to other species (e.g., tuna, grouper) or to large-scale aquaculture scenarios (such as offshore cages or recirculating water systems). To address this, we plan to pursue cross-species detection by optimizing the SobelMaxDS module to better adapt to the morphological characteristics of different fish, incorporating dynamic deblurring convolution techniques to alleviate motion blur caused by high-speed swimming, and integrating domain adaptation methods to enhance the model’s generalization ability in diverse aquaculture environments. Second, considering the practical requirements for real-time monitoring with low latency, we propose to adopt hierarchical quantization and knowledge distillation strategies. In combination with operator fusion optimization techniques (e.g., TensorRT) and a co-design approach for hardware and software, our goal is to achieve efficient, low-latency real-time detection on embedded platforms such as Jetson Nano, thus meeting the high-speed response demands of on-site applications.Finally, in the area of multimodal behavior analysis, we plan to integrate environmental sensor data—such as water temperature and dissolved oxygen levels—and develop a fusion model based on spatiotemporal attention mechanisms to establish dynamic correlations between environmental parameters and behavioral abnormalities. For instance, when dissolved oxygen levels drop below a certain threshold, the model can autonomously increase the detection weight for hypoxic behavior, while a joint confidence calibration mechanism will be employed to reduce false alarm rates, thereby further enhancing detection accuracy and robustness.
Data availability statement
The raw data supporting the conclusions of this article will be made available by the authors, without undue reservation.
Ethics statement
The Animal Experiment Ethics Committee of Guangdong Ocean University has conducted a review of this project’s experimental protocols in accordance with relevant laws, regulations, and ethical standards. The committee confirms that the experimental animals used in this project meet ethical requirements. As all experimental procedures and sterilization protocols comply with the relevant regulations for animal experimentation research, the committee has approved the continuation of project-related experiments (Approval Number: No. 8203).
Author contributions
ML: Conceptualization, Funding acquisition, Methodology, Software, Writing – original draft, Writing – review & editing. CZ: Methodology, Software, Writing – original draft, Writing – review & editing. CL: Conceptualization, Project administration, Writing – original draft, Writing – review & editing.
Funding
The author(s) declare that financial support was received for the research and/or publication of this article. This work was partly supported by the National Natural Science Foundation of China (62171143), Guangdong Provincial University Innovation Team (2023KCXTD016), special projects in key fields of ordinary universities in Guangdong, Province (2021ZDZX1060), the Stable Supporting Fund of Acoustic Science and Technology Laboratory (JCKYS2024604SSJS00301), the Undergraduate Innovation Team Project of Guangdong Ocean University under Grant CXTD2024011, the Open Fund of Guangdong Provincial Key Laboratory of Intelligent Equipment for South China Sea Marine Ranching (Grant NO. 2023B1212030003), Guangdong Ocean University Technology Achievement Transformation Project Team (JDTD2024003).
Conflict of interest
The authors declare that the research was conducted in the absence of any commercial or financial relationships that could be construed as a potential conflict of interest.
Generative AI statement
The author(s) declare that no Generative AI was used in the creation of this manuscript.
Publisher’s note
All claims expressed in this article are solely those of the authors and do not necessarily represent those of their affiliated organizations, or those of the publisher, the editors and the reviewers. Any product that may be evaluated in this article, or claim that may be made by its manufacturer, is not guaranteed or endorsed by the publisher.
References
Abram P. K., Boivin G., Moiroux J., Brodeur J. (2017). Behavioural effects of temperature on ectothermic animals: unifying thermal physiology and behavioural plasticity. Biol. Rev. 92, 1859–1876. doi: 10.1111/brv.2017.92.issue-4
Barany A., Gilannejad N., Alameda-López M., Rodríguez-Velásquez L., Astola A., Martínez-Rodríguez G., et al. (2021). Osmoregulatory plasticity of juvenile greater amberjack (seriola dumerili) to environmental salinity. Animals 11, 2607. doi: 10.3390/ani11092607
Bochkovskiy A., Wang C.-Y., Liao H.-Y. M. (2020). Yolov4: Optimal speed and accuracy of object detection. arXiv. doi: 10.48550/arXiv.2004.10934
Chen J. C., Chen T.-L., Wang H.-L., Chang P.-C. (2022). Underwater abnormal classification system based on deep learning: A case study on aquaculture fish farm in Taiwan. Aquacultural Eng. 99, 102290. doi: 10.1016/j.aquaeng.2022.102290
Ding X., Zhang X., Han J., Ding G. (2021). “Diverse branch block: Building a convolution as an inception-like unit,” in Proceedings of the IEEE/CVF conference on computer vision and pattern recognition (Piscataway, New Jersey, USA: Institute of Electrical and Electronics Engineers (IEEE)), 10886–10895.
Gao W., Zhang X., Yang L., Liu H. (2010). “An improved sobel edge detection,” in 2010 3rd International conference on computer science and information technology, vol. 5. (Piscataway, New Jersey, USA: IEEE), 67–71.
Gevorgyan Z. (2022). SIoU loss: more powerful learning for bounding box regression. arXiv:2205.12740. doi: 10.48550/arXiv.2205.12740
Han K., Wang Y., Tian Q., Guo J., Xu C., Xu C. (2020). “Ghostnet: More features from cheap operations,” in Proceedings of the IEEE/CVF conference on computer vision and pattern recognition. (Silver Spring, Maryland, USA: IEEE Computer Society), 1580–1589.
Howard A., Sandler M., Chu G., Chen L.-C., Chen B., Tan M., et al. (2019). “Searching for mobilenetv3,” in Proceedings of the IEEE/CVF international conference on computer vision (Los Alamitos, California, USA: IEEE Computer Society), 1314–1324.
Hu K., Zhang E., Xia M., Wang H., Ye X., Lin H. (2024). Cross-dimensional feature attention aggregation network for cloud and snow recognition of high satellite images. Neural Computing Appl. 36, 7779–7798. doi: 10.1007/s00521-024-09477-5
Jiang P., Ergu D., Liu F., Cai Y., Ma B. (2022). A review of yolo algorithm developments. Proc. Comput. Sci. 199, 1066–1073. doi: 10.1016/j.procs.2022.01.135
Kaur G., Adhikari N., Krishnapriya S., Wawale S. G., Malik R., Zamani A. S., et al. (2023). Recent advancements in deep learning frameworks for precision fish farming opportunities, challenges, and applications. J. Food Qual. 2023, 4399512. doi: 10.1155/2023/4399512
Khanam R., Hussain M. (2024). Yolov11: An overview of the key architectural enhancements. arXiv. doi: 10.48550/arXiv.2410.17725
Li D., Wang G., Du L., Zheng Y., Wang Z. (2022). Recent advances in intelligent recognition methods for fish stress behavior. Aquacultural Eng. 96, 102222. doi: 10.1016/j.aquaeng.2021.102222
Li X., Wang W., Wu L., Chen S., Hu X., Li J., et al. (2020). Generalized focal loss: Learning qualified and distributed bounding boxes for dense object detection. Adv. Neural Inf. Process. Syst. 33, 21002–21012. doi: 10.48550/arXiv.2006.04388
Li X., Zhao S., Chen C., Cui H., Li D., Zhao R. (2024a). Yolo-fd: An accurate fish disease detection method based on multi-task learning. Expert Syst. Appl. 258, 125085. doi: 10.1016/j.eswa.2024.125085
Li Y., Hu Z., Zhang Y., Liu J., Tu W., Yu H. (2024b). Ddeyolov9: network for detecting and counting abnormal fish behaviors in complex water environments. Fishes 9, 242. doi: 10.3390/fishes9060242
Lin C., Jiang Z., Cong J., Zou L. (2025). Rnn with high precision and noise immunity: A robust and learning-free method for beamforming. IEEE Internet Things J. doi: 10.1109/JIOT.2025.3529532
Lin C., Mao X., Qiu C., Zou L. (2024). Dtcnet: Transformer-cnn distillation for super-resolution of remote sensing image. IEEE J. Selected Topics Appl. Earth Observations Remote Sens. 17, 11117–11133. doi: 10.1109/JSTARS.2024.3409808
Liu W., Anguelov D., Erhan D., Szegedy C., Reed S., Fu C.-Y., et al. (2016). “Ssd: Single shot multibox detector,” in Computer Vision – ECCV 2016: 14th European Conference, Amsterdam, The Netherlands, October 11–14, 2016, Proceedings. 21–37 (Berlin/Heidelberg, Germany: Springer).
Liu C., Wang Z., Li Y., Zhang Z., Li J., Xu C., et al. (2023). Research progress of computer vision technology in abnormal fish detection. Aquacultural Eng. 103, 102350. doi: 10.1016/j.aquaeng.2023.102350
Mandal A., Ghosh A. R. (2024). Role of artificial intelligence (ai) in fish growth and health status monitoring: A review on sustainable aquaculture. Aquaculture Int. 32, 2791–2820. doi: 10.1007/s10499-023-01297-z
Pinheiro A. C. D. A. S., Schouten M. A., Tappi S., Gottardi D., Barbieri F., Ciccone M., et al. (2024). Strategies for the valorization of fish by-products: Fish balls formulated with mechanically separated amberjack flesh and mullet hydrolysate. LWT 209, 116724. doi: 10.1016/j.lwt.2024.116724
Shi H., Li J., Li X., Ru X., Huang Y., Zhu C., et al. (2024). Survival pressure and tolerance of juvenile greater amberjack (seriola dumerili) under acute hypo-and hyper-salinity stress. Aquaculture Rep. 36, 102150. doi: 10.1016/j.aqrep.2024.102150
Tan M., Le Q. (2019). “Efficientnet: Rethinking model scaling for convolutional neural networks,” in International conference on machine learning (Cambridge, Massachusetts, USA: PMLR), 6105–6114.
Tone K., Nakamura Y., Chiang W.-C., Yeh H.-M., Hsiao S.-T., Li C.-H., et al. (2022). Migration and spawning behavior of the greater amberjack seriola dumerili in eastern Taiwan. Fisheries Oceanography 31, 1–18. doi: 10.1111/fog.12559
Tong Z., Chen Y., Xu Z., Yu R. (2023). Wise-iou: bounding box regression loss with dynamic focusing mechanism. arXiv. doi: 10.48550/arXiv.2301.10051
Trippel E. A. (1995). Age at maturity as a stress indicator in fisheries. Bioscience 45, 759–771. doi: 10.2307/1312628
Vincent O. R., Folorunso O. (2009). “A descriptive algorithm for sobel image edge detection,” in Proceedings of informing science & IT education conference (InSITE). (Santa Rosa, California, USA: Informing Science Institute), 40, 97–107.
Wang A., Chen H., Liu L., Chen K., Lin Z., Han J., et al. (2024). Yolov10: Real-time end-to-end object detection. arXiv. doi: 10.48550/arXiv.2405.14458
Wang C.-Y., Yeh I.-H., Mark Liao H.-Y. (2025). “Yolov9: Learning what you want to learn using programmable gradient information,” in European conference on computer vision (Berlin/Heidelberg, Germany: Springer), 1–21.
Wang Z., Zhang X., Su Y., Li W., Yin X., Li Z., et al. (2023). Abnormal behavior monitoring method of larimichthys crocea in recirculating aquaculture system based on computer vision. Sensors 23, 2835. doi: 10.3390/s23052835
Wang H., Zhang S., Zhao S., Lu J., Wang Y., Li D., et al. (2022a). Fast detection of cannibalism behavior of juvenile fish based on deep learning. Comput. Electron. Agric. 198, 107033. doi: 10.1016/j.compag.2022.107033
Wang H., Zhang S., Zhao S., Wang Q., Li D., Zhao R. (2022b). Real-time detection and tracking of fish abnormal behavior based on improved yolov5 and siamrpn++. Comput. Electron. Agric. 192, 106512. doi: 10.1016/j.compag.2021.106512
Wang J., Zhuang J., Duan L., Cheng W. (2016). “A multi-scale convolution neural network for featureless fault diagnosis,” in 2016 international symposium on flexible automation (isfa) (Piscataway, New Jersey, USA: IEEE), 65–70.
Wiles S. C., Bertram M. G., Martin J. M., Tan H., Lehtonen T. K., Wong B. B. (2020). Long-term pharmaceutical contamination and temperature stress disrupt fish behavior. Environ. Sci. Technol. 54, 8072–8082. doi: 10.1021/acs.est.0c01625
Wu Y., He K. (2018). “Group normalization,” in Proceedings of the European conference on computer vision (Berlin/Heidelberg, Germany: ECCV), 3–19.
Xiao H., Chen X., Luo L., Lin C. (2025). A dual-path feature reuse multi-scale network for remote sensing image super-resolution. J. Supercomputing 81, 1–28. doi: 10.1007/s11227-024-06569-w
Yang L., Liu Y., Yu H., Fang X., Song L., Li D., et al. (2021). Computer vision models in intelligent aquaculture with emphasis on fish detection and behavior analysis: a review. Arch. Comput. Methods Eng. 28, 2785–2816. doi: 10.1007/s11831-020-09486-2
Yu X., Wang Y., An D., Wei Y. (2021). Identification methodology of special behaviors for fish school based on spatial behavior characteristics. Comput. Electron. Agric. 185, 106169. doi: 10.1016/j.compag.2021.106169
Zhang H., Zhang S. (2023). Shape-iou: More accurate metric considering bounding box shape and scale. arXiv. doi: 10.48550/arXiv.2312.17663
Zhao Y., Qin H., Xu L., Yu H., Chen Y. (2025). A review of deep learning-based stereo vision techniques for phenotype feature and behavioral analysis of fish in aquaculture. Artif. Intell. Rev. 58, 1–61. doi: 10.1007/s10462-024-10960-7
Zhao H., Wu Y., Qu K., Cui Z., Zhu J., Li H., et al. (2024). Vision-based dual network using spatial-temporal geometric features for effective resolution of fish behavior recognition with fish overlap. Aquacultural Eng. 105, 102409. doi: 10.1016/j.aquaeng.2024.102409
Zhao S., Zhang S., Liu J., Wang H., Zhu J., Li D., et al. (2021). Application of machine learning in intelligent fish aquaculture: A review. Aquaculture 540, 736724. doi: 10.1016/j.aquaculture.2021.736724
Zheng Z., Wang P., Liu W., Li J., Ye R., Ren D. (2020). “Distance-iou loss: Faster and better learning for bounding box regression,” in Proceedings of the AAAI conference on artificial intelligence. (Washington, DC, USA: Association for the Advancement of Artificial Intelligence (AAAI Press)), vol. 34, 12993–13000.
Keywords: deep learning, abnormal behavior detection, greater amberjack, aquaculture, YOLOv8, real-time monitoring
Citation: Liu M, Zhang C and Lin C (2025) GAB-YOLO: a lightweight deep learning model for real-time detection of abnormal behaviors in juvenile greater amberjack fish. Front. Mar. Sci. 12:1574580. doi: 10.3389/fmars.2025.1574580
Received: 11 February 2025; Accepted: 07 April 2025;
Published: 08 May 2025.
Edited by:
Zhibin Yu, Ocean University of China, ChinaReviewed by:
Yun Li, Guangxi University of Finance and Economics, ChinaYinke Dou, Taiyuan University of Technology, China
Copyright © 2025 Liu, Zhang and Lin. This is an open-access article distributed under the terms of the Creative Commons Attribution License (CC BY). The use, distribution or reproduction in other forums is permitted, provided the original author(s) and the copyright owner(s) are credited and that the original publication in this journal is cited, in accordance with accepted academic practice. No use, distribution or reproduction is permitted which does not comply with these terms.
*Correspondence: Cong Lin, bGluY29uZ0BnZG91LmVkdS5jbg==