- 1Navigation College, Dalian Maritime University, Dalian, Liaoning, China
- 2Key Laboratory of Navigation Safety Guarantee of Liaoning Province, Dalian, China
Introduction: Accurate identification of ship behavioral patterns is essential for maritime management, contributing to improved regulatory efficiency, accident prevention, navigation safety, and scheduling. However, traditional methods often struggle with the complexity of high-dimensional, time-series trajectory data.
Methods: To overcome these challenges, this study proposes the following optimized graph neural network (GNN) models: an optimized adjacency matrix graph convolutional network, a hybrid model combining a graph convolutional network with a graph attention network (GAT), and an integrated model of GAT and long short-term memory. These models leverage standardized automatic identification system data to improve feature extraction and recognition accuracy.
Results: Experimental results demonstrate that the proposed models achieve over 98% accuracy in ship behavioral pattern recognition, with fast convergence and superior performance compared to conventional GNN-based methods.
Discussion: The models provide robust and efficient solutions for maritime traffic analysis, offering significant potential for real-world applications in ship monitoring, intelligent navigation, and maritime safety management.
1 Introduction
The shipping industry is vital to the global economy, handling over 90% of global trade and significantly influencing economic patterns, international cooperation, and environmental protection. Navigation safety is a top priority, and modern ship behavior pattern recognition technology ensures safe and efficient navigation. By monitoring real-time data such as position, speed, and heading, ship behavior pattern recognition systems can prevent collisions and optimize traffic flow, thereby enhancing the operational efficiency of maritime transport (Wu et al., 2021). As highlighted by the International Maritime Organization (IMO), most of maritime accidents are attributable to human error, many of which stem from misjudged or uncoordinated ship maneuvers. Ship behavior recognition thus serves as a critical foundation for proactive safety monitoring and early warning systems (Zhang et al., 2022a). Automatic identification system (AIS) data, which includes both static and dynamic information, are crucial for ship behavior analysis (Zhou et al., 2019). In port waters and congested sea lanes, real-time recognition of ship behavior patterns aids in vessel traffic service (VTS), congestion prediction, and illegal anchoring detection. It also underpins intelligent maritime surveillance systems by enabling dynamic risk assessment and anomaly detection (Ribeiro et al., 2023). Beyond ensuring navigation safety, AIS data play a fundamental role in maritime traffic systems by supporting traffic optimization, intelligent monitoring, and environmental management. As such, they have become a core data resource driving the digitalization and intelligent transformation of modern maritime governance (Almunia et al., 2021; Guan et al., 2022). Previous studies have explored various methods for behavior pattern recognition (Gao et al., 2018; Li et al., 2024; Wei et al., 2024; Xin et al., 2024; Han et al., 2025). Most existing methods depend on parameter settings and thresholds, which limits their generalizability and requires extensive tuning. Deep learning, particularly GNN, has the potential to improve spatial and temporal relationship modeling, feature extraction, and handling of irregular data (Xin et al., 2024). However, the application of GNN in marine ship motion pattern recognition remains limited. This suggests that optimizing GNN improves ship behavior pattern recognition. Existing research has explored various behavioral pattern recognition methods; however, these methods face many challenges when handling high-dimensional, time-series ship trajectory data (Gao et al., 2018; Yan et al., 2020; Rong et al., 2022). For example, many methods rely on parameter settings and thresholds, which results in poor model generalizability and requires extensive manual tuning. In addition, conventional methods typically handle ships independently; thus, the complex interactions between ships are difficult to capture, along with generalization across regions and under different conditions. To solve these problems, this study aims to achieve high-accuracy ship behavior pattern recognition using hybrid GNN models trained on AIS data.
In this study, we define three primary ship behavior patterns: underway, anchoring, and mooring, based on their fundamental significance in maritime management and monitoring. These patterns represent the most common operational states of ships and are widely regarded as essential categories in ship behavior recognition. The underway state denotes a vessel actively navigating, a crucial aspect of maritime traffic control, route optimization, and voyage planning. The anchoring state characterizes a ship remaining stationary in designated sea areas or ports, necessitating continuous monitoring to ensure compliance with anchorage regulations and maritime safety protocols. The mooring state describes the process of vessels docking at ports or berthing along the shore, a behavior closely associated with port operations, berth management, and cargo handling. Given their prevalence and operational significance, these three patterns comprehensively encompass the majority of typical ship activities, making them highly representative for maritime surveillance, decision-making, and intelligent management applications.
The structure of this paper is as follows. Section 2 reviews related research on ship behavior pattern recognition. In Section 3, we present a ship behavior pattern recognition model based on optimized GNN. Section 4 describes the experimental process and discusses the experimental results. Section 5 concludes the study and recommends future research directions.
2 Literature review
2.1 Ship behavior pattern recognition methods
In recent years, various ship behavior pattern recognition methods have been developed based on AIS data (Chen et al., 2020; Zhang et al., 2022b). Researchers have analyzed rich motion features in AIS data to identify ship behavior patterns. Li et al. proposed a multi-attribute trajectory similarity method by analyzing static, dynamic, and port geospatial features and their semantic relationships (Li et al., 2024). Data mining techniques have been used to extract key behavioral features, explore motion characteristics in AIS data, and propose efficient traffic network extraction frameworks (Liu et al., 2023). Suo et al. introduced a complex ship behavior pattern mining model to classify sailing behaviors (Suo et al., 2022), and Yan et al. extracted ship docking information using fused trajectory features and geographic scene semantics (Filipiak et al., 2020). Clustering methods are also popular for detecting abnormal behaviors and recognizing loading and unloading patterns. Wei et al. used the DBSCAN algorithm to classify maritime traffic behavior patterns (Wei et al., 2024). Gao et al. proposed a method to recognize loading and unloading behaviors using sub-trajectory clustering (Gao and Shi, 2020). Zhou et al. developed a new method for clustering ship paths and ground speeds to understand port behavioral patterns (Zhou et al., 2019). However, conventional methods have limitations. For example, they are sensitive to trajectory noise, sensor errors, and environmental factors; they typically treat ships independently, thereby missing complex interactions; they struggle to generalize across regions and conditions, thereby requiring extensive manual tuning. Consequently, these methods may perform poorly with new or uncommon ship states. To address these challenges, graph neural networks offer a more advanced approach by modeling ship movements as a graph, where nodes represent ships and edges capture their interactions. Unlike traditional methods, GNN automatically learn spatial and temporal dependencies, reducing reliance on manual feature extraction and improving adaptability to varying maritime scenarios. By incorporating optimized GNN architectures, this study demonstrates enhanced recognition accuracy, robustness, and efficiency in ship behavior pattern analysis, paving the way for more intelligent maritime traffic management.
2.2 Graph neural network
GNN excel at modeling and analyzing graph-structured data, achieving good results in social networks, bioinformatics, and recommender systems (Shaw and Wu, 2023; Ünal and Akkaş, 2023). By combining graph topology and node characteristics, GNN effectively model complex relationships. GNN offer new opportunities for ship behavior pattern recognition, improving accuracy and robustness by comprehensively understanding ship relationships. These models synthesize node connections, extract state information from AIS data, and consider all data connections to optimize model parameters based on feature correlations. Zhang et al. explored semi-supervised learning with Graph Convolutional Networks (GCN) (Zhang et al., 2021). Liu et al. introduced a spatiotemporal multi-GCN for ship trajectory prediction (Liu et al., 2022). Zhao et al. developed a framework combining k-GCNs and long short-term memory (LSTM) for predicting ship speeds (Zhao et al., 2022). A deep attention awareness spatiotemporal GCN has been proposed to predict the future trajectories of ships (Wang et al., 2024). Zhang et al. developed G-STGAN, which aggregates spatial and temporal features for ship trajectory prediction, demonstrating competitive performance (Zhang et al., 2023). Gao et al. applied GCNs to multi-ship encounter identification (Gao et al., 2024). Conventional GCNs have fixed weights; thus, they treat all neighboring nodes equally, which limits their ability to differentiate important nodes and capture the influence of various AIS data features. Although GAT models incorporate attention mechanisms, they have high computational complexity and scalability problems. This study addresses these challenges by optimizing GNN models to improve the performance and efficiency of ship behavior pattern recognition.
Section 2.1 highlights the shortcomings of conventional ship behavior pattern recognition methods. These methods typically encounter several challenges in handling high-dimensional and sequential AIS data. For example, the presence of data noise, sensor inaccuracies, and potential data loss complicates the precise extraction of track features; The intricate spatiotemporal interactions inherent in ship behavior patterns are typically overlooked by conventional approaches; the fixed parameter settings and reliance on thresholds restrict the adaptability and generalization capabilities of these methods.
To address these issues, this study proposes three GNN-based optimization models to improve both the accuracy and robustness of ship behavior pattern recognition by effectively modeling the spatiotemporal relationships in AIS data, these models leverage advanced GNN techniques to improve the accuracy of ship behavior pattern recognition.
3 Optimization model framework
In this section, several ship behavior pattern recognition models using optimized GNN and hybrid models are presented. In the first part, the AIS data are initially cleaned and processed to obtain effective and reliable ship trajectory segments. In the second part, the structure and content of conventional GNN are introduced, and the optimization and improvement of the models are performed by combining the features of different GNN for the special structure and complex information of AIS data. The main flow of the model is illustrated in Figure 1.
3.1 AIS data processing
AIS data are typically affected by GPS interference, sensor errors, and transmission delays, which reduce data quality. To address this, we employed data cleaning and correction methods to improve the accuracy and reliability of the AIS data. For missing data, abnormal position and speed data were detected by calculating the mean and standard deviation, setting three standard deviations as thresholds, outliers that significantly deviated from normal trajectory patterns were first removed and then replaced using linear interpolation. For repeated latitude and longitude values caused by signal noise or transmission errors, only the first occurrence was retained to preserve trajectory continuity. Regarding missing values, records with missing timestamps were directly discarded, while missing values in critical fields such as position (longitude and latitude), speed over ground (SOG), and course over ground (COG) were filled using interpolation methods, depending on the location and pattern of the missing data. This approach effectively reduces the impact of AIS data errors.
The MMSI number was used to uniquely identify each ship. LAT and LON represent the latitude and longitude of ships. These coordinates provide the spatial foundation for analyzing behavioral patterns such as anchoring, drifting, or navigation within constrained waterways. Speed over ground (SOG), heading (HEA), and course over ground (COG) are dynamic AIS attributes that describe the vessel’s motion characteristics. Specifically, SOG reflects the ship’s actual velocity over the Earth’s surface, while COG and HEA together help capture directional stability and maneuvering behavior. The difference between heading and COG may indicate lateral drift or external influences such as wind or current, which are informative for detecting anomalies or behavior transitions. Due to sensor errors, data loss, or communication failures, some of the AIS data are inaccurate (Xin et al., 2024). Speed over ground (SOG) values of 102.3 were considered invalid and deleted. Course over ground (COG) and heading values of 511 were also considered invalid and were removed. Other data were deemed irrelevant and excluded from the study. Shorter time intervals help capture subtle changes in a ship’s behavior without losing information due to long sampling intervals.
As shown in Figure 2, to ensure the accuracy and validity of the data during model training, we grouped every 50 consecutive AIS data points for each ship, ensuring that the state remained consistent and the time interval did not exceed 10 min. Each state contained an equal number of data points to ensure balance in the data. Each group was labeled based on the ship’s state. We retained the five-dimensional features longitude, latitude, SOG, heading, and COG and calculated the time difference between adjacent data points to capture both static and motion features. After processing, the data were grouped into sets with 50 data points each, with each point formatted as A1 = (LAT1, LON1, SOG1, COG1, HEA1, T1), where HEA denotes the heading and T denotes the time interval. Each data group was labeled with the corresponding vessel behavior pattern. Each data sample obtained after processing has 50 sets of data points, each with multidimensional features. The results of data processing and grouping are shown in Figure 2D.
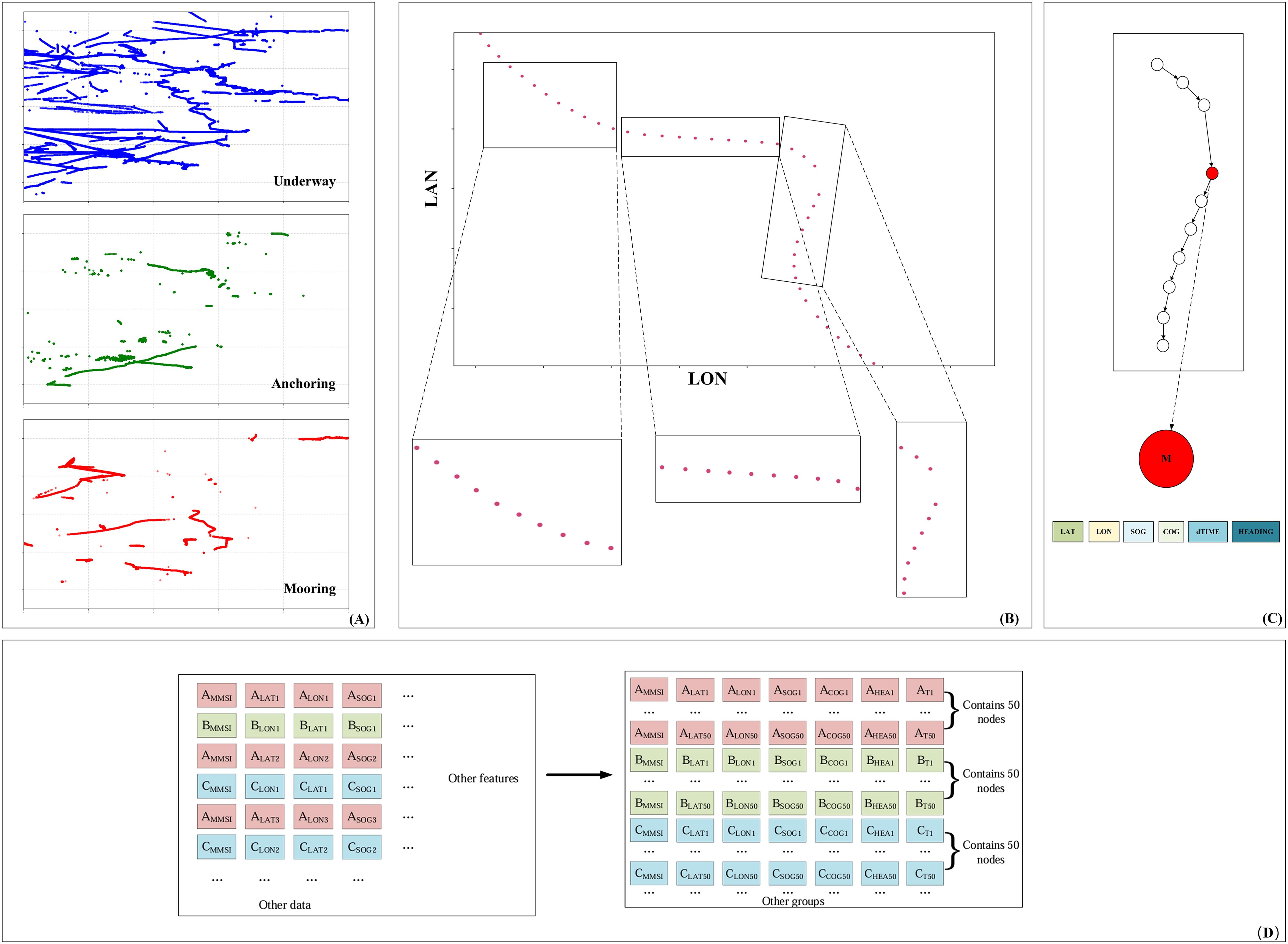
Figure 2. Schematic of AIS data processing flow. (A) Visualization of AIS data of selected ships with different behavioral models (the horizontal and vertical axes represent latitude and longitude coordinates). (B) Every tenth consecutive node is taken as a group (for schematic purposes only, 50 nodes in the model). (C) Each node has six features. (D) Data processing and grouping.
3.2 Graph neural network model construction
After processing the AIS data, as described in Section 3.1, the cluttered AIS data were organized into a standardized dataset. Then, a model was developed based on the data characteristics and the GNN structure. In this study, we developed three hybrid GNN models and conducted experimental analyses to compare their respective strengths and weaknesses:
AMO-GCN (Adjacency matrix Optimized GCN): This model addresses the issue of fixed weights in conventional GCNs, which fail to distinguish important neighboring nodes. By optimizing the adjacency matrix, AMO-GCN effectively captures the dependency relationships and structural information between nodes.
Hybrid GAT–GCN model: To tackle the high computational complexity of GAT models, this hybrid model uses GCNs for initial feature extraction and then uses GATs for fine-grained feature aggregation, thereby improving performance and efficiency.
Hybrid GAT–LSTM model: This model combines GAT and LSTM to better understand complex spatiotemporal relationships. GAT mines potential relationships in the spatial dimension, whereas LSTM models long-term dependencies in the temporal dimension, thereby improving accuracy and robustness.
The structure of a conventional graph convolutional neural network (GCNN) is shown in Figure 3A. Following hidden layer computation to extract features according to the features and data processing, as described in Section 3.1, the graph structure data are divided into two categories, Y1 and Y2. The convolutional structure diagram of our model is shown in Figure 3B. The model inputs the processed data, uses a GNN to extract data features, and weights them into three states. When extracting feature information, each node is associated with other nodes by default, and all associations are combined into an adjacency matrix. In Figure 3C, node M is used as an example. A, B, M, C, and D represent several neighboring nodes, and we briefly explain the structure of the model by analyzing the associations between these nodes. The maximum influence distance between nodes is 2, and nodes A, B, M, C, and D have six features. In the model, for node M feature extraction, the five node features are weighted and summed. When initializing the GCN model, the adjacency matrix (Adj or A) is used to define the topology of the graph-structured data to ensure that the model can accurately perform graph convolution operations. During model initialization, Adj is passed to the model to allow it to understand the connection relationships between nodes. In a GCNN, layers are propagated from layer to layer as Equation 1:
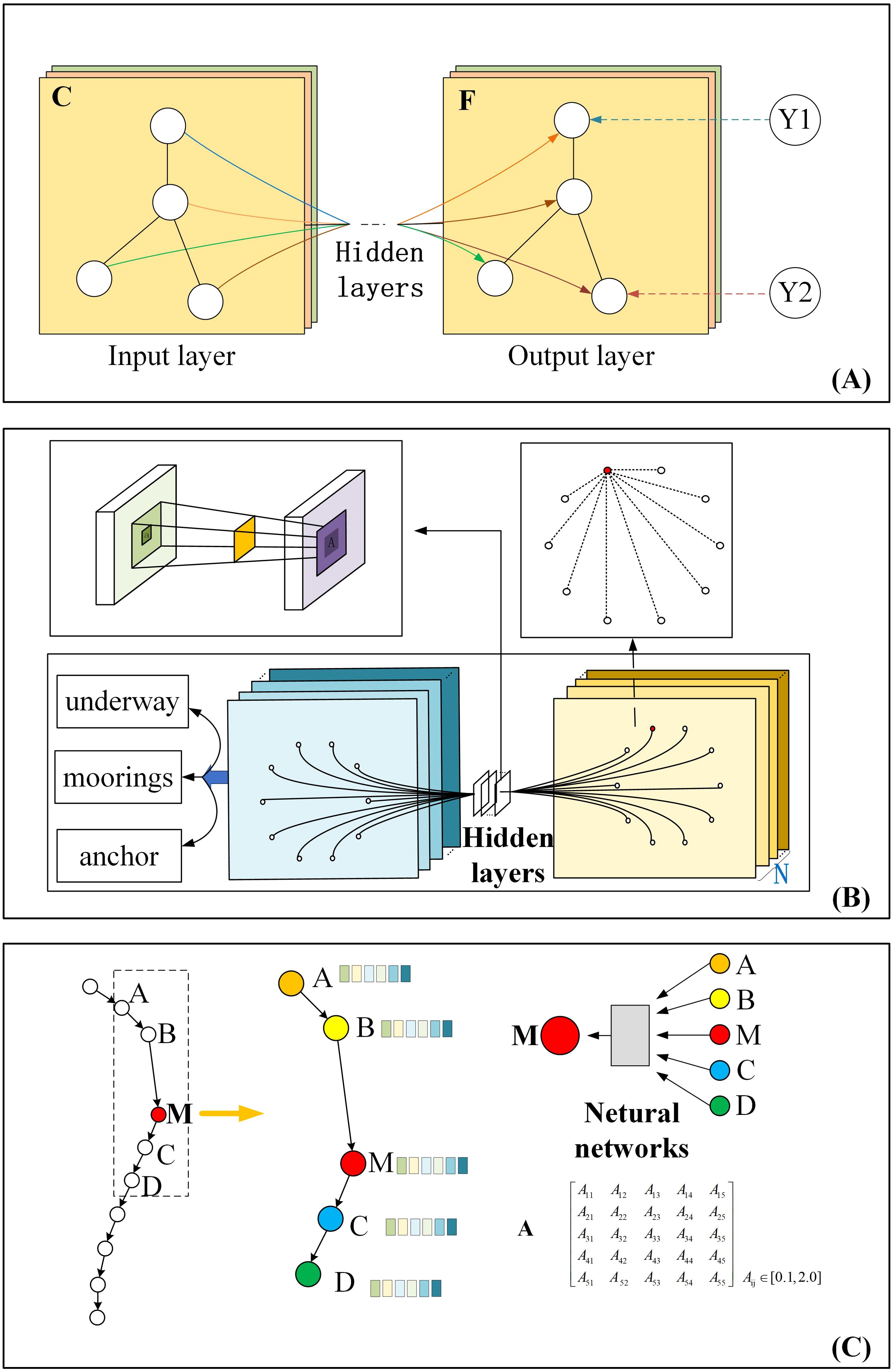
Figure 3. Model structure association diagram. (A) Conventional GCNN structure. (B) Convolutional structure diagram of our model. (C) Data feature calculation diagram (take node M as an example).
In this equation, denotes the node features at layer , representing the updated node representations after graph convolution. is the node feature matrix from the previous layer. Here, . is the initial adjacency matrix, and I represents the unit matrix. An increase in the unit matrix allows the model to consider the node relationship and its influence. D is the degree matrix, a diagonal matrix used for normalization based on node degrees. is the learnable weight matrix at layer l, which transforms the node features and captures structural relationships. δ is the activation function, which introduces non-linearity to enhance the model’s representational capacity. In summary, the proposed model integrates a Graph Neural Network structure to perform modeling and classification of ship trajectory data. Based on the preprocessed AIS data, a graph structure is constructed by defining the connections between nodes, ensures the graph convolution operations accurately reflect potential interactions among vessels. The model then applies a two-layer graph convolution architecture combined with feature fusion, enabling effective extraction of spatial neighborhood features for each node. These features are aggregated using weighted adjacency information. The incorporation of graph-based structure significantly enhances the model’s capability in capturing spatiotemporal behavior patterns of ships and improves recognition performance for complex navigational states. Through this propagation rule, the GCNN updates node features layer by layer, effectively aggregating information from neighbors and learning hierarchical feature representations.
In this study, optimizing the adjacency matrix and other hyperparameter combinations of GNN requires extensive computations. The optimization of model parameters is critical to enhancing the performance and generalization capability of the proposed GNN-based architectures. Different hyperparameters play distinct roles during training and directly affect the model’s learning behavior. Key hyperparameters such as the learning rate, dropout rate, weight decay, number of graph convolutional layers, and the structure of attention mechanisms substantially influence the model’s convergence speed, resistance to overfitting, and its ability to capture spatial-temporal dependencies inherent in AIS data. For instance, the learning rate determines how rapidly the model updates its weights during training. An excessively high rate may lead to instability or divergence, while a rate that is too low can slow convergence and increase the risk of getting trapped in local minima. Dropout acts as a regularization technique by randomly deactivating neurons during training, thereby reducing overfitting and improving model robustness. Weight decay helps prevent the model from becoming overly complex by penalizing large weights, promoting simpler and more generalizable representations. The number of graph convolutional layers controls the extent of neighborhood information aggregation—too few layers may limit the model’s expressive power, while too many may lead to over-smoothing, making node representations indistinguishable and degrading classification performance.
Considering the complex interplay among these hyperparameters and the high computational cost of exhaustive search strategies, traditional grid search or random search approaches are inefficient for identifying optimal configurations. To address this, we adopted Bayesian optimization via the fmin function from the Hyperopt library. Bayesian optimization constructs a probabilistic surrogate model of the objective function here, the model’s validation error and iteratively selects hyperparameter combinations by balancing exploration and exploitation. By leveraging prior evaluations to inform future trials, this method efficiently navigates the hyperparameter space and significantly reduces the number of evaluations required. In this study, we applied Bayesian optimization not only to refine key hyperparameters but also to adjust the structure of the adjacency matrix, thereby enhancing the model’s ability to capture meaningful graph topology. The resulting optimized parameter set collectively improves model accuracy, training efficiency, and robustness in the ship behavior pattern recognition task.
In GAT, the attentional layer is the only layer that constitutes the entire GAT. The input to this layer is the original features of the node, and the output is the new features of the node. For example, the input feature is, and the output feature is , ( denotes a multidimensional real vector space). The GAT network is realized based on the attention mechanism, and the core of the attention mechanism is to calculate the degree of the contribution of each part to the final goal. The degree of contribution is represented by the attention coefficient. First, the self-attention mechanism is executed by linearly varying the input features; second, the above-calculated coefficients are normalized by softmax, and the attention coefficients are then calculated by the nonlinear layer; finally, the normalized attention coefficients are linearly combined with their corresponding features to be the final output features of each node.
To synthesize the local feature aggregation capability of GCNs and the attention mechanism of GAT for robust graph feature representation, we developed a hybrid GCN–GAT model for recognizing ship sailing patterns. Node features enter the input layer, and the initial feature representation of each node is generated by first performing global feature extraction of the features of the nodes in the graph through the GCN layer. The weights are then dynamically assigned among the neighbors of each node using the GAT layer to re-aggregate the features according to the importance of the neighboring nodes. The final feature representation of a node is generated by combining the output features of both parts. Finally, the output layer is used for classification. This hybrid network leverages the strengths of both GCNs and GAT, thereby more effectively handling complex relationships in graph-structured data. The GAT–LSTM hybrid model combines a GAT and a LSTM network to process data with both graph structures and sequence information. The GAT layer processes the input features to generate new node representations that serve as input to the LSTM layer. The LSTM layer models temporal sequence information, and its output is fed to a final GAT layer, resulting in node classification (Figure 4). This hybrid model effectively utilizes the advantages of GAT for graph data and LSTM for sequence data, thereby addressing more complex tasks.
The data used by the three proposed models were AIS datasets in the same format, as shown in Figure 1. Although data processing was consistent across all models, the neural networks and algorithms differed, leading to distinct ship behavior recognition results. Each method has unique characteristics: AMO-GCN improves accuracy and robustness in processing graph-structured data by optimizing the adjacency matrix; the GCN–GAT hybrid model combines graph convolution and attention mechanisms for strong comprehensive performance, effectively learning both graph structures and node features; the GAT–LSTM hybrid model merges graph structure learning with time-series modeling, making it suitable for dynamic behavior pattern recognition with flexible and comprehensive performance.
4 Results analysis and discussion
This section describes the entire experimental process, starting with the processing of the experimental data, followed by the ship behavior pattern recognition using each model, and finally the analysis of the experimental results.
4.1 Experimental data
Publicly available AIS data collected from January 2021 to December 2022 collected from AccessAIS-MarineCadastre.gov were used in the experiment.
As shown in Figure 5, we selected the coastal waters of Texas as the study area, specifically encompassing the region between 29°18′N–29°33′N latitude and 94°45′W–95°06′W longitude. This area includes major ports such as Galveston, Texas City, and the surrounding bay areas. It represents a highly active maritime region with frequent ship movements, making it suitable for behavior pattern recognition research. The AIS data visualized in the Figure are plotted as red dots, indicating ship trajectories collected during the observation period.
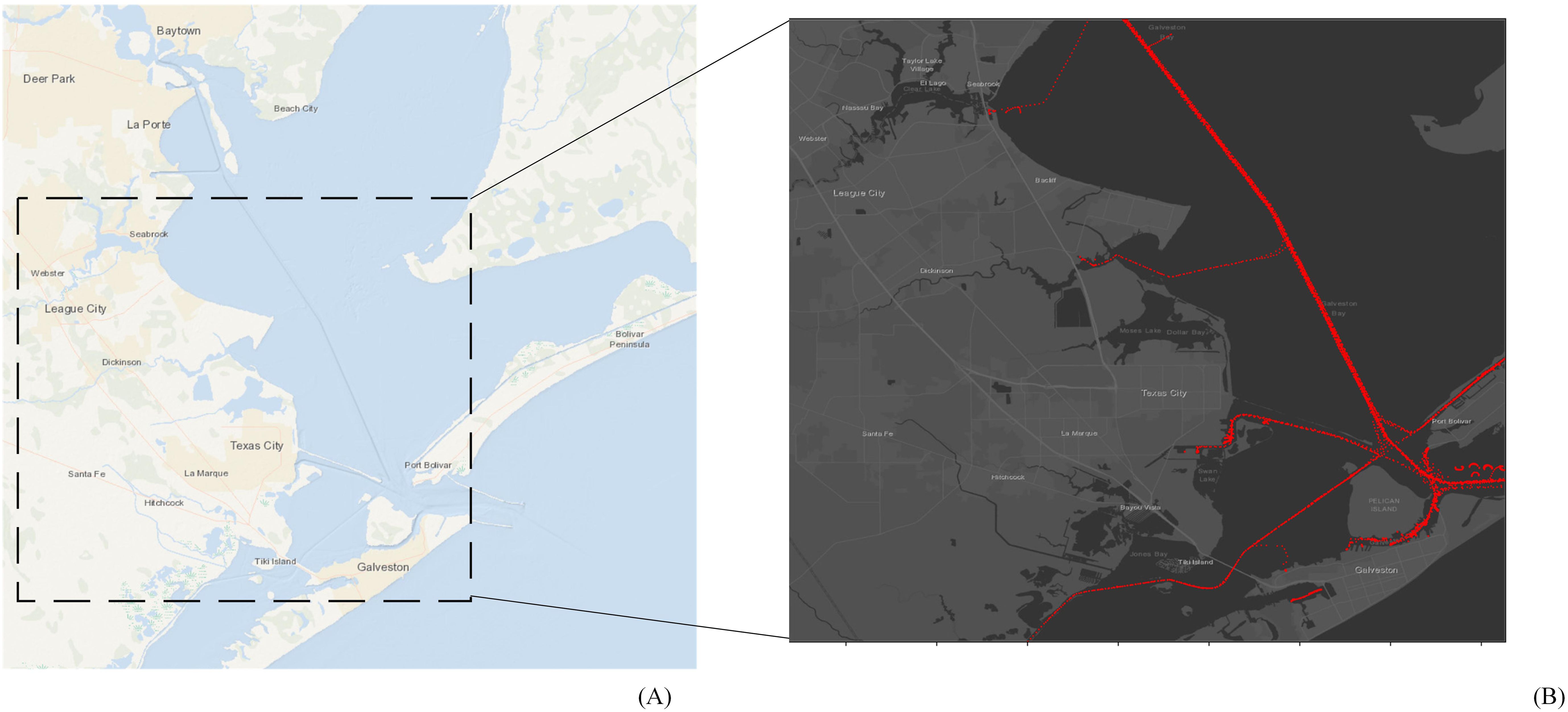
Figure 5. Research area. (A) Geographical map of the study area. (B) Ship trajectories in the study area, visualized using AIS data as red dots.
To ensure the diversity and reliability of the experimental data, various types of ships—including passenger ships, cargo ships, and oil tankers—were selected, with lengths ranging from 15 m (e.g., RHONDA E) to 338 m (e.g., LIBERTY OF THE SEAS). After cleaning and organizing the AIS data, a total of 750,000 high-quality data points were obtained from 1,174,918 raw trajectory records. These cleaned and filtered trajectory points, indicated as red dots in the corresponding figure, represent consistent and valid motion patterns. To facilitate controlled evaluation and reduce confounding factors during model comparison, this study employed a balanced sampling strategy, wherein an equal number of trajectory groups (5,000) were constructed for each of the three ship behavior categories: underway, mooring, and anchored. Each group contains 50 consecutive data nodes, with each node described by six key features: latitude, longitude, speed over ground (SOG), course over ground (COG), heading, and timestamp interval. This balanced design allows for fair performance comparison across different models and clearer attribution of effectiveness to architectural components, rather than to class distribution biases. It also ensures that the behavior recognition model can be evaluated under uniform data conditions before being extended to naturally imbalanced datasets in future work.
The AIS data for each state were divided into training, validation, and test sets at a 3:1:1 ratio. Specifically, the training, validation, and test sets contained 3,000, 1,000, and 1,000 data groups, respectively. The training set was used for model training, where the model learned the features and patterns of the data. The validation set was used to evaluate model performance on unseen data to prevent overfitting and to adjust hyperparameters (e.g., learning rate, regularization factor, and number of network layers) to find the optimal configuration. The test set, which was completely unseen during training, was used to evaluate the final trained model to obtain performance metrics and objectively reflect the generalizability of the model.
4.2 Experimental procedure
The three proposed innovative models, namely, AMO-GCN, a hybrid GCN–GAT model, and a hybrid GAT–LSTM model, were evaluated and compared with the conventional GCN and GAT models. Uniform hyperparameters and graph convolutional layers were set for consistency: two graph convolutional layers with 64 kernels each, a dropout rate of 60%, a learning rate of 0.01, a weight decay of 0.0001, and 200 runs. All models used the same AIS dataset format. The GCN model uses multiple graph convolutional layers to perform convolution operations on nodes (ship locations) via the adjacency matrix to extract local and global features. The GAT model employs graph attention layers, which extract features through the weighted summation of node features, consisting of embedding and output layers. The embedding layer has multiple parallel graph attention layers, stitching features together, and the output layer maps these features to the final category labels. The hybrid GAT–LSTM model integrates an LSTM layer to receive input from the graph attention layer of the GAT. The feature matrix was reshaped into sequence data to better capture the temporal relationships and dependencies in the graph data. This setup allows for a thorough comparison of the ability of the models to recognize ship behavior patterns from AIS data.
4.3 Analysis and comparison of results
4.3.1 Analysis of model results
The models were coded in Python using PyTorch, a library for developing and training deep-learning models. To evaluate the performance and effectiveness of the hybrid model, conventional GCN and GAT were used as control groups, each processing data differently and efficiently over time. Performance was assessed by tracking loss and accuracy. Loss, derived from the negative log-likelihood function, measures prediction discrepancies. Minimizing this loss optimizes the model parameters, which improves performance. Loss on the validation set helps visualize the prediction accuracy of the model, whereas accuracy provides insight into the classification performance and generalizability of the model. To visualize the training process, we plotted the loss and accuracy curves over the training rounds for each model (Figure 6). The total time for each model run was recorded, along with the final accuracy on the test set. The results are shown in Table 1 and Figure 7.
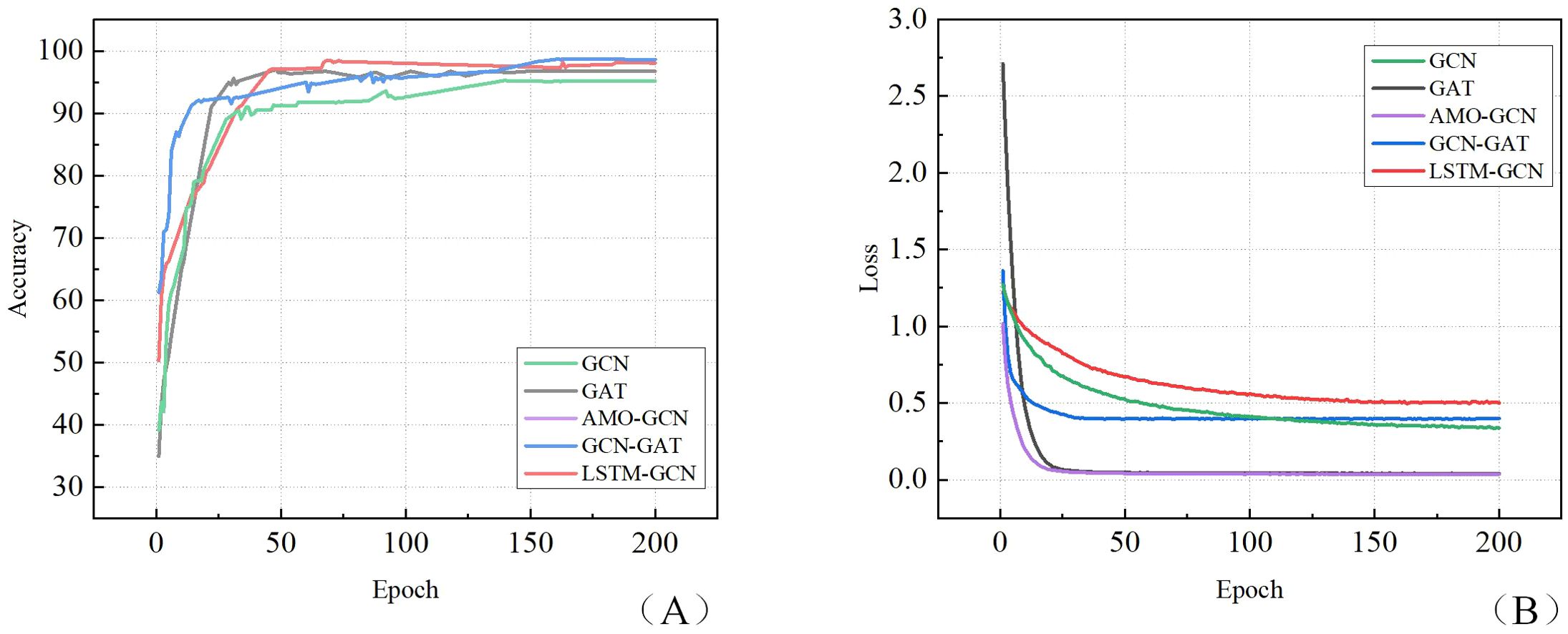
Figure 6. Model performance curve on the validation set. (A) Accuracy curves for the five models. (B) Loss curves for the five models.
The experimental results demonstrate that the traditional GCN model has the fastest processing speed (a running time of 241.91 s) due to its low computational complexity and direct convolution on graph nodes. Although the GCN model effectively captures local node information, it cannot finely model relationships between neighboring nodes, which leads to its slightly lower accuracy. Its simple weighted summation encounters difficulties in handling complex graph structures and long-range dependencies; thus, it is suitable for high-speed scenarios despite its higher loss values.
The GAT model, which uses a multi-head attention mechanism to weight and sum node relationships, exhibits increased computational complexity and longer processing times. Although GAT first optimizes rapidly, its performance subsequently decreases, which requires further parameter tuning. However, the attention mechanism improves accuracy by finely capturing node relationships and weight distributions. The AMO-GCN model improves accuracy by optimizing the adjacency matrix, thereby capturing node relationships more effectively. This increases computational time and resource consumption but improves feature extraction and reduces errors during training. Despite having the longest running time, the higher accuracy of AMO-GCN makes it suitable for precision-demanding tasks with ample computational resources. The GCN–GAT hybrid model combines the rapid local information processing of the GCN with the attention mechanism of GAT, thereby improving efficiency and achieving the highest final accuracy. It balances accuracy and running time, making it ideal for high-accuracy tasks with moderate time constraints. Although the GAT–LSTM hybrid model exhibits longer computational times due to the complexity of LSTM when handling sequence data, it effectively captures complex relationships and dynamic features over time. Its accuracy is second only to that of GCN–GAT, which makes it suitable for tasks requiring high accuracy despite longer running times. In summary, all models achieved greater than 95% accuracy, with the basic GCN achieving 95.16%, demonstrating the effectiveness of GNN in capturing the relational structure of AIS data. The hybrid GCN–GAT and GAT–LSTM models achieved the highest and second-highest accuracies (98.86% and 98.67%, respectively) by combining the strengths of the different approaches. Base models such as GCNs offer shorter running times, which makes them ideal for time-sensitive or resource-constrained tasks. This comparison highlights the strengths of hybrid models in terms of accuracy and feature fusion for complex tasks, whereas base models excel in terms of efficiency for fast-paced, resource-limited scenarios. The selection and optimization of an appropriate model ensures the best performance and efficiency across different applications.
To visually assess the classification results, we presented confusion matrices for each model, which clearly display the true positives, false positives, and misclassifications for each behavioral pattern, as illustrated in Figure 8. Based on the confusion matrix, we observe that the different models exhibit slight differences in recognizing the three behavioral patterns; however, similarly, each model exhibits high accuracy in distinguishing mooring patterns from the other behavioral patterns.
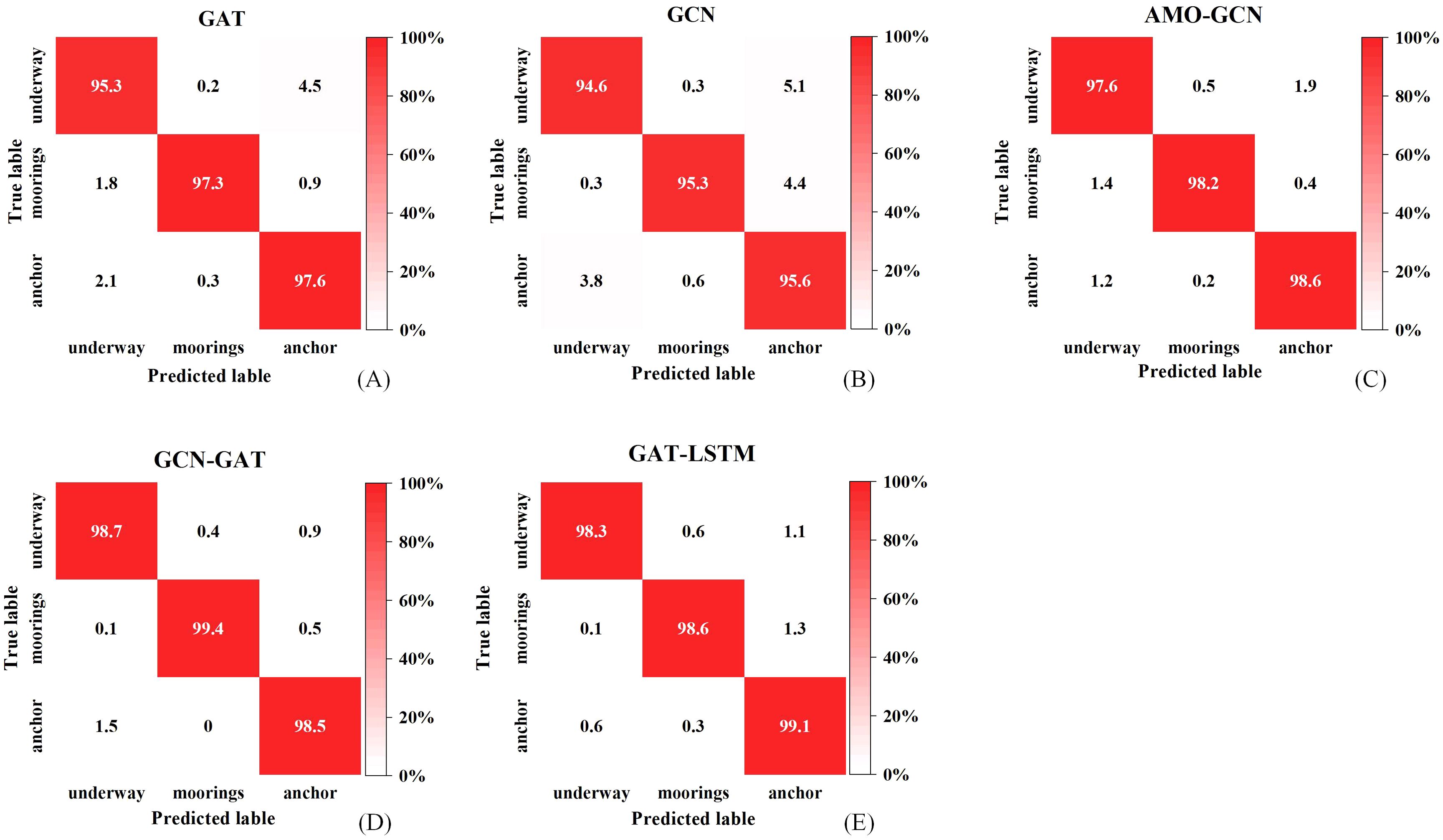
Figure 8. Confusion matrixes of results on five training model test sets: (A) GAT model, (B) GCN model, (C) AMO-GCN model, (D) GCN-GAT model, (E) GAT-LSTM model.
Precision measures the accuracy of a model in predicting a specific class, whereas recall evaluates the ability of the model to identify all samples of that class. Both metrics are critical in assessing the performance of classification models on imbalanced datasets or for multiclass problems. To obtain a more complete view of the ability of the model to accurately categorize samples in each category, we computed the precision and recall for each category to evaluate classification performance. The results are shown in Figure 9.
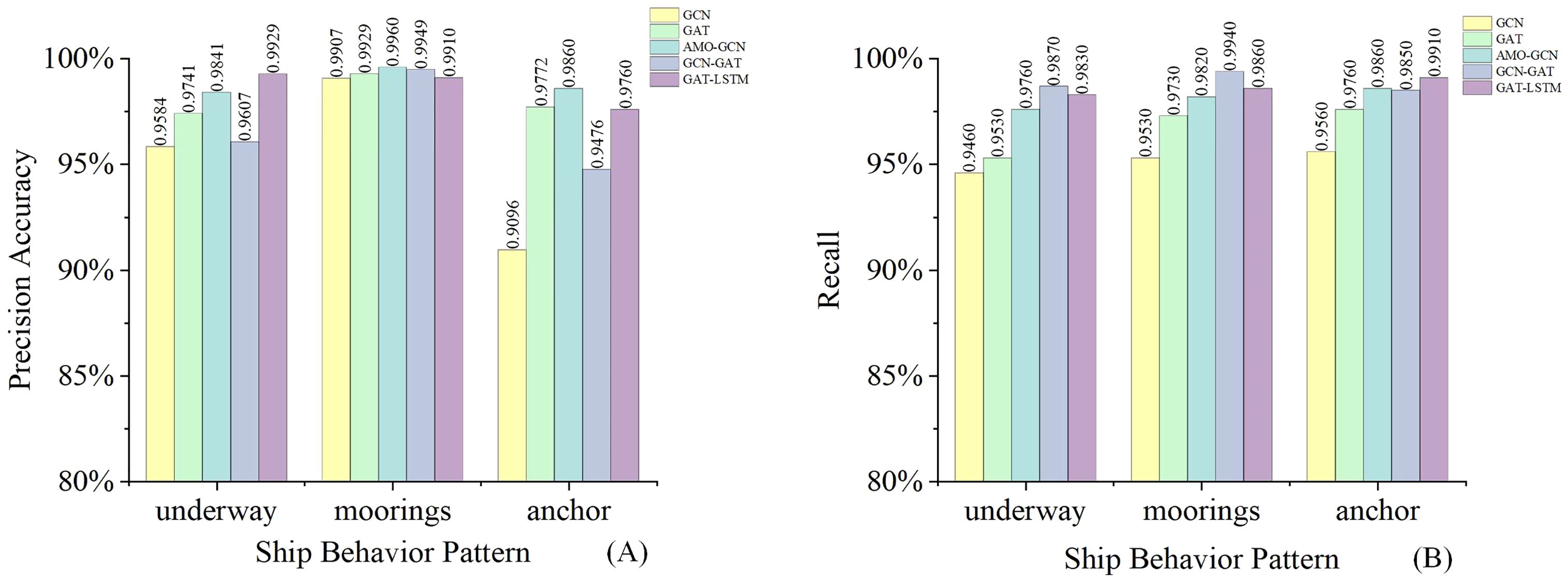
Figure 9. Histogram of accuracy vs. recall for various categories for each model: (A) Precision accuracy for each ship behavior pattern, (B) Recall for each ship behavior pattern.
As shown in the Figure 9, the precision and recall values of the GCN and GAT models are low, particularly for the “underway” and “anchor” states. In contrast, the precision and recall values of the GCN–GAT hybrid model reached over 98% for most of the categories, indicating a significant improvement in the classification performance. For “underway,” precision and recall were slightly lower than for “mooring” and “anchor” patterns in all experiments. There may be feature ambiguities or overlaps with other states (e.g., “anchor”), making them susceptible to misclassification. “Mooring” states may have more defined characteristics and are thus easier for classifiers to distinguish. There may be some overlap of features between the “mooring” and “underway” states, such as certain low-speed moving behaviors, leading to model confusion.
To better understand the contribution of each key component in our hybrid GNN models, we conducted a comprehensive ablation study. Specifically, we assessed the impact of three core architectural modules: adjacency matrix optimization, attention mechanism and temporal modeling via LSTM. The performance of each model was evaluated using three metrics: validation loss, classification accuracy, and inference time. The results are summarized in Table 2.
The ablation study demonstrates the importance of each architectural component in the proposed hybrid models. The AMO-GCN model, which integrates graph convolution, attention mechanisms, and adjacency matrix optimization via an actor module, achieved a high recognition accuracy of 98.16% with moderate inference time. When adjacency optimization and attention were removed, resulting in a GCN-only version, the accuracy dropped to 94.96%. This performance gap underscores the value of adaptive structure learning in enhancing behavior recognition. Similarly, the GCN-GAT model, which fuses convolutional and attention-based spatial learning, achieved an accuracy of 98.70%. In comparison, the GCN-only and GAT-only variants achieved 95.03% and 96.33%, respectively, suggesting that their combination yields a more comprehensive representation than either component alone. The GAT-LSTM model, designed to jointly capture spatial attention and temporal dependencies, achieved 98.53% accuracy. Its GAT-only variant showed a modest decline to 97.01%, while the LSTM-only version performed significantly worse at 35.54%, highlighting that spatial contextual information is essential for ship behavior recognition and cannot be replaced by temporal modeling alone.
From a computational perspective, GCN-only models demonstrated the lowest inference latency, making them suitable for resource-constrained or real-time applications. However, the hybrid models, while incurring higher computational costs, consistently delivered superior recognition performance. Overall, the ablation results confirm that incorporating attention mechanisms, temporal dynamics, and adaptive graph structures significantly enhances the model’s ability to capture complex maritime behavior patterns from AIS data.
4.3.2 Discussion
This study optimized GNN algorithms for ship behavior pattern recognition by leveraging the ability of GNN to handle connections between data points. We developed hybrid models based on the characteristics of different GNN and the AIS data structure, achieving high recognition accuracy. After cleaning and organizing the AIS data, we retained six key features: LON, LAT, SOG, HEA, COG and T. Each of the 50 consecutive nodes formed a complete data unit, resulting in 5,000 samples for each behavior category: underway, anchor, and mooring.
Considering the spatial nature of ship trajectory data and the ability of GNN to learn spatial patterns, we developed three hybrid models: AMO-GCN, GCN–GAT, and GAT–LSTM. These models combined the strengths of various approaches, thereby significantly improving the accuracy of ship behavior pattern recognition. Although the control GCN model was less accurate, it had the shortest running time, making it suitable for tasks with limited computational resources or time constraints. The models can extract specific ship state information from AIS data, thereby providing reliable navigation advice and enhancing maritime traffic safety. In practical applications of ship behavior pattern recognition, for real-time requirements, it is recommended to use GCN models with slightly lower accuracy but faster processing speed. For higher-accuracy requirements, the AMO-GCN and GCN–GAT models with higher accuracy are more suitable, and the GAT–LSTM model is suitable for tasks with high-accuracy requirements and is sensitive to temporal relationships. In summary, this study achieved high-accuracy behavior pattern recognition by optimizing GNN to yield hybrid models. The GNN models demonstrated strong data mining capabilities and high accuracy and were particularly useful in waters with rich historical AIS data.
However, the proposed models still have certain limitations. For instance, the current hybrid architectures that combine GCN, GAT, or LSTM operate in a multi-stage manner, where spatial and temporal features are modeled separately. While this modular design provides flexibility and interpretability, it also introduces challenges such as increased training complexity and potential inconsistencies across stages. To address these issues, future research will explore the integration of temporal GNN, which are capable of simultaneously capturing both spatial dependencies and temporal dynamics in an end-to-end fashion. These models leverage time-aware graph structures or time-encoding mechanisms to enhance the representation of evolving ship behaviors over time. By adopting such unified frameworks, we expect to improve both the training efficiency and the coherence of spatiotemporal feature extraction.
This study employed a balanced sampling strategy to enable controlled evaluation; however, this approach does not fully reflect the naturally imbalanced distribution of AIS data, where the “underway” state occurs more frequently than “anchored” or “moored” states. Future work will consider techniques such as resampling or cost-sensitive learning to improve model robustness under real-world class imbalance.
In addition, future work will aim to enhance the real-time monitoring capabilities of the proposed models, enabling timely and intelligent responses to complex maritime traffic scenarios. This will support the development of a more flexible, scalable, and intelligent maritime transportation system. Although the hybrid models proposed in this study achieve high recognition accuracy, their multi-stage architecture increases computational complexity. To facilitate practical deployment in time-sensitive and resource-limited environments, future research will explore lightweight and adaptive GNN designs that reduce inference latency while maintaining performance.
Furthermore, to enhance the operational applicability and practical value of the proposed framework, future research will investigate the extension of behavior categories beyond the current classification of underway, moored, and anchored. In particular, nuanced and operationally significant behaviors such as loitering, drifting, illegal anchoring, and search-and-rescue standby will be considered. These patterns are increasingly relevant in real-world maritime security, anomaly detection, and environmental protection scenarios. For instance, loitering behavior, often characterized by low-speed circular or meandering paths in non-designated zones, may indicate suspicious or unauthorized activity. Similarly, illegal anchoring can be inferred from stationary behavior in environmentally protected or restricted areas, violating port regulations or navigational laws. Detecting such complex behaviors requires the model to capture finer-grained temporal-spatial variations and contextual cues from AIS trajectories. While this introduces new challenges such as class imbalance, data annotation difficulty, and inter-behavior similarity, it also presents an opportunity to strengthen the model’s generalization ability and broaden its utility in intelligent maritime surveillance systems. Therefore, future work will focus on refining trajectory segmentation, incorporating context-aware features (e.g., restricted area maps, VTS zones), and developing adaptive thresholds to support the identification of these subtle yet critical patterns. By expanding the behavioral taxonomy, the proposed framework can better assist in monitoring vessel compliance, detecting maritime anomalies, and supporting domain-specific applications such as marine protected area enforcement, illegal fishing prevention, and dynamic anchorage management.
5 Conclusions
In this study, we propose ship behavior pattern recognition methods based on AIS data and GNN. We developed hybrid models based on conventional GNN, achieving over 98% accuracy on the test set. These hybrid models improve the accuracy and efficiency of ship movement behavior pattern recognition, providing powerful tools for maritime traffic management. They have broad applications in maritime supervision, navigation safety, and ship management, offering reliable technical support and improving the overall effectiveness of maritime traffic networks.
The innovative aspect of this study is the use of GNN and hybrid models to transform error-prone AIS data into valuable ship behavior pattern information. This transformation helps decision makers and planners improve navigation paths and maritime safety. Promoting international AIS data sharing will enhance global maritime traffic management and safety, thereby supporting the development of more efficient maritime traffic systems. Continuous model optimization and expanded research areas will further promote global maritime traffic management, protect navigators, and lay the foundation for a comprehensive maritime transportation network. Future research should focus on systems capable of processing and analyzing real-time data to facilitate real-time decision-making and response. This will enhance the flexibility and responsiveness of maritime traffic management, ensure navigation safety and efficiency, optimize navigation routes and schedules, strengthen emergency response capabilities, and support long-term analysis and strategic planning. To further improve performance and generalization, future research will explore the incorporation of adaptive graph construction mechanisms, enabling the adjacency matrix to be learned dynamically rather than predefined. Such adaptive graph neural networks (AGNN) have shown significant potential in handling heterogeneous and evolving topologies in maritime scenarios. Moreover, we will investigate ensemble-based learning strategies and end-to-end architectures such as T-GCN, TGAT, or ST-GCN to unify spatial and temporal modeling in a more efficient and scalable manner. These improvements are expected to address current limitations in trajectory segmentation, feature interaction, and ship-type generalization.
With the increasing availability of reliable external data sources and the continuous advancement of model capabilities, future work may explore multimodal data fusion techniques. By incorporating additional contextual features—such as weather conditions, vessel types, and destinations—these methods have the potential to enhance model performance and generalization, improve classification robustness, and support broader maritime monitoring tasks under diverse operational scenarios. To support real-time maritime surveillance, future research will also focus on reducing inference latency and improving model scalability. This includes the development of lightweight and adaptive GNN architectures, as well as the integration of early-exit strategies and stream-based data processing frameworks. These enhancements aim to maintain recognition accuracy while ensuring timely responses in resource-constrained and dynamic environments.
We also plan to expand behavior categories, incorporate vessel-specific profiles, and deploy real-time behavior recognition systems. Additionally, ablation studies will be conducted to quantitatively evaluate the contribution of each architectural module to model performance. This comprehensive research roadmap supports long-term development of intelligent maritime management systems. Expanding the model’s capabilities will allow for a more nuanced understanding of vessel activities, which is crucial for improving intelligent maritime management. Through persistent research and innovation, we can comprehensively elevate maritime traffic management.
Data availability statement
The raw data supporting the conclusions of this article will be made available by the authors, without undue reservation.
Author contributions
LM: Conceptualization, Visualization, Validation, Writing – original draft, Supervision, Writing – review & editing, Data curation. HC: Validation, Writing – original draft, Conceptualization. G-YS: Funding acquisition, Formal Analysis, Writing – review & editing.
Funding
The author(s) declare that financial support was received for the research and/or publication of this article. This research was funded by China Environment and Zoology Protection for Offshore Oil and Ocean Foundation (no.CF-MEEC-TR-2023-8).
Conflict of interest
The authors declare that the research was conducted in the absence of any commercial or financial relationships that could be construed as a potential conflict of interest.
Generative AI statement
The author(s) declare that no Generative AI was used in the creation of this manuscript.
Publisher’s note
All claims expressed in this article are solely those of the authors and do not necessarily represent those of their affiliated organizations, or those of the publisher, the editors and the reviewers. Any product that may be evaluated in this article, or claim that may be made by its manufacturer, is not guaranteed or endorsed by the publisher.
References
Almunia J., Delponti P., and Rosa F. (2021). Using automatic identification system (AIS) data to estimate whale watching effort. Front. Marine Sci. 8. doi: 10.3389/fmars.2021.635568
Chen X., Liu Y., Achuthan K., and Zhang X. (2020). A ship movement classification based on Automatic Identification System (AIS) data using Convolutional Neural Network. Ocean Eng. 218, 108182. doi: 10.1016/j.oceaneng.2020.108182
Filipiak D., Węcel K., Stróżyna M., Michalak M., and Abramowicz W. (2020). Extracting maritime traffic networks from AIS data using evolutionary algorithm. Business Inf. Syst. Eng. 62, 435–450. doi: 10.1007/s12599-020-00661-0
Gao M., Liang M., Zhang A., Hu Y., and Zhu J. (2024). Multi-ship encounter situation graph structure learning for ship collision avoidance based on AIS big data with spatio-temporal edge and node attention graph convolutional networks. Ocean Eng. 301, 117605. doi: 10.1016/j.oceaneng.2024.117605
Gao M. and Shi G.-Y. (2020). Ship-handling behavior pattern recognition using AIS sub-trajectory clustering analysis based on the T-SNE and spectral clustering algorithms. Ocean Eng. 205, 106919. doi: 10.1016/j.oceaneng.2020.106919
Gao M., Shi G., and Li S. (2018). Online prediction of ship behavior with automatic identification system sensor data using bidirectional long short-term memory recurrent neural network. Sensors 18, 4211. doi: 10.3390/s18124211
Guan Y. A., Zhang J., Zhang X., Li Z. W., Meng J. M., Liu G. W., et al. (2022). Study on the activity laws of fishing vessels in China's sea areas in winter and spring and the effects of the COVID-19 pandemic based on AIS data. Front. Marine Sci. 9. doi: 10.3389/fmars.2022.861395
Han X., Zhou Y., Weng J. J., Chen L. J., and Liu K. (2025). Research on fishing vessel recognition based on vessel behavior characteristics from AIS data. Front. Marine Sci. 12. doi: 10.3389/fmars.2025.1547658
Li G., Zhang X., Jiang L., Wang C., Huang R., and Liu Z. (2024). An approach for traffic pattern recognition integration of ship AIS data and port geospatial features. Geo-spatial Inf. Sci. 27, 1–28. doi: 10.1080/10095020.2024.2308715
Liu Z., Gao H., Zhang M., Yan R., and Liu J. (2023). A data mining method to extract traffic network for maritime transport management. Ocean Coastal Manage. 239, 106622. doi: 10.1016/j.ocecoaman.2023.106622
Liu R. W., Liang M., Nie J., Yuan Y., Xiong Z., Yu H., et al. (2022). STMGCN: mobile edge computing-empowered vessel trajectory prediction using spatio-temporal multigraph convolutional network. IEEE Trans. Industrial Inf. 18, 7977–7987. doi: 10.1109/tii.2022.3165886
Ribeiro C. V., Paes A., and Oliveira D. D. (2023). AIS-based maritime anomaly traffic detection: A review. Expert Syst. Appl. 231, 120561. doi: 10.1016/j.eswa.2023.120561
Rong H., Teixeira A. P., and Guedes Soares C. (2022). Maritime traffic probabilistic prediction based on ship motion pattern extraction. Reliability Eng. System Saf. 217, 4211. doi: 10.1016/j.ress.2021.108061
Shaw J. and Wu B. J. (2023). Artificial intelligence in prediction of the remaining useful life of wind turbine shaft bearings. J. Marine Sci. Technol. 31(4), 12. doi: 10.51400/2709-6998.2719
Suo Y., Ji Y., Zhang Z., Chen J., and Claramunt C. (2022). A formal and visual data-mining model for complex ship behaviors and patterns. Sensors (Basel) 22, 5281. doi: 10.3390/s22145281
Ünal O. and Akkaş N. (2023). Smart sounding table using adaptive neuro-fuzzy inference system. J. Marine Sci. Technol. 31(3), 8. doi: 10.51400/2709-6998.2703
Wang S., Li Y., Xing H., and Zhang Z. (2024). Vessel trajectory prediction based on spatio-temporal graph convolutional network for complex and crowded sea areas. Ocean Eng. 298, 117232. doi: 10.1016/j.oceaneng.2024.117232
Wei Z., Gao Y., Zhang X., Li X., and Han Z. (2024). Adaptive marine traffic behaviour pattern recognition based on multidimensional dynamic time warping and DBSCAN algorithm. Expert Syst. Appl. 238, 122229. doi: 10.1016/j.eswa.2023.122229
Wu Z., Pan S., Chen F., Long G., Zhang C., and Yu P. S. (2021). A comprehensive survey on graph neural networks. IEEE Trans. Neural Netw Learn Syst. 32, 4–24. doi: 10.1109/TNNLS.2020.2978386
Xin R., Pan J., Yang F., Yan X., Ai B., and Zhang Q. (2024). Graph deep learning recognition of port ship behavior patterns from a network approach. Ocean Eng. 305, 117921. doi: 10.1016/j.oceaneng.2024.117921
Yan Z., Xiao Y., Cheng L., He R., Ruan X., Zhou X., et al. (2020). Exploring AIS data for intelligent maritime routes extraction. Appl. Ocean Res. 101, 102271. doi: 10.1016/j.apor.2020.102271
Zhang Z., Huang L., Peng X., Wen Y., and Song L. (2022b). Loitering behavior detection and classification of vessel movements based on trajectory shape and Convolutional Neural Networks. Ocean Eng. 258, 111852. doi: 10.1016/j.oceaneng.2022.111852
Zhang X., Liu J., Gong P., Chen C., Han B., and Wu Z. (2023). Trajectory prediction of seagoing ships in dynamic traffic scenes via a gated spatio-temporal graph aggregation network. Ocean Eng. 287, 115886. doi: 10.1016/j.oceaneng.2023.115886
Zhang H., Lu G., Zhan M., and Zhang B. (2021). Semi-supervised classification of graph convolutional networks with laplacian rank constraints. Neural Process. Lett. 54, 2645–2656. doi: 10.1007/s11063-020-10404-7
Zhang C., Zou X., and Lin C. (2022a). Fusing XGBoost and SHAP models for maritime accident prediction and causality interpretability analysis. J. Marine Sci. Eng. 10, 1154. doi: 10.3390/jmse10081154
Zhao J., Yan Z., Chen X., Han B., Wu S., and Ke R. (2022). k-GCN-LSTM: A k-hop Graph Convolutional Network and Long–Short-Term Memory for ship speed prediction. Physica A: Stat. Mechanics its Appl. 606, 128107. doi: 10.1016/j.physa.2022.128107
Keywords: maritime traffic networks, graph neural networks, AIS data, behavioral pattern recognition, ship motion analysis
Citation: Ma L, Cao H and Shi G-Y (2025) Ship behavior pattern recognition method based on hybrid graph neural networks. Front. Mar. Sci. 12:1605216. doi: 10.3389/fmars.2025.1605216
Received: 04 April 2025; Accepted: 12 May 2025;
Published: 29 May 2025.
Edited by:
Jose M. Molina, Universidad Carlos III de Madrid, SpainReviewed by:
Chengbo Wang, University of Science and Technology of China, ChinaJie Wu, Tianjin University, China
Copyright © 2025 Ma, Cao and Shi. This is an open-access article distributed under the terms of the Creative Commons Attribution License (CC BY). The use, distribution or reproduction in other forums is permitted, provided the original author(s) and the copyright owner(s) are credited and that the original publication in this journal is cited, in accordance with accepted academic practice. No use, distribution or reproduction is permitted which does not comply with these terms.
*Correspondence: Lin Ma, MTg5MDk4NTIzNTlAMTYzLmNvbQ==