- 1Laboratoire d’Electroniques et Micro-électroniques (LR99ES30), Faculty of Sciences Of Monastir, University of Monastir, Monastir, Tunisia
- 2Center for Microsystems Technology and IMEC, University of Ghent, Ghent, Belgium
- 3National Institute of Technologies and Sciences of Elkef, University of Elkef, Elkef, Tunisia
In recent years, the interest of science in big data sensing, storage and processing has been growing fast. Nano-materials have been widely used in resistive switching devices thanks to their distinguished properties. Furthermore, they provide nano-scale dimensions and compatibility with fabrication procedures and complementary metal oxide semiconductor (CMOS) technology. Nano-materials can also enhance the performance of memristive structures. The operation of a memristor, which enables efficient resistive switching characterized by fast response, increased storage density, and low power requirements, depends largely on nano-materials and deposition techniques. Herein, a comprehensive brief review of nano-material RRAM arrays and their application in biomedical is discussed. First, we introduce planar and array resistive switching structures. Second, we report the different nanomaterial categories that can be used in resistive random-access memories (RRAMs). Then, we focus on the integration of 3D nano-material-based memristive crossbars for in-memory computing and biosensing arrays and discuss representative applications. The exploration of nano-materials enables the development of enhanced resistive switching architectures with increased signal integrity, great speed, and ultra-high sensitivity towards thermally and electrically stable memristive biomedical platforms.
1 Introduction
Since their breakthrough in 2008, HP memristors have been broadly used in various applications (Strukov et al., 2008), including analogue and digital circuits, biosensors, artificial neurons and synapses, as well as RRAMs for memory storage (Homsi et al., 2023; Li et al., 2018; Barraj et al., 2024). Resistive switching devices, often referred to as non-volatile memories, consist of sandwiched structures evolving metal-insulator-metal materials. They store data by alternating the insulator material’s resistive level between high and low states. RRAMs are characterised by rapid switching speed and ON/OFF ratio, efficient endurance, and long retention time (Bouzouita et al., 2024). These memristive operating standards are often affected by different properties of nanomaterials (Zahoor et al., 2020). In other words, memristive benchmarks interconnect the choice of nanomaterials with memristor performance (Wang Miao et al., 2018) through the diversity of intrinsic physical and chemical reactions of materials, as well as fabrication techniques and defect engineering that benefit the development and destruction of the conductive filament within the insulator layer. Various review papers on nanomaterials for memristive architectures exist in this context. In 2018, Ahn et al. explored
2 Introduction to RRAM
2.1 RRAM structure
Various RRAM structures have recently been investigated to enhance performance and address emerging computational and storage challenges. This paper discusses three primary RRAM architectures: (a) metal-insulator-metal (MIM) or planar, (b) Crossbar arrays, and (c) three-dimensional (3D) RRAM.
2.1.1 Planar structure
In 1967, Simmons et al. introduced the first proposed MIM structure (Figure 1a) in which resistive switching is attributed to the formation and rupture of conductive filaments in the insulating layer (Simmons and Verderber, 1967; Sawa, 2008). The MIM structure has shown high endurance
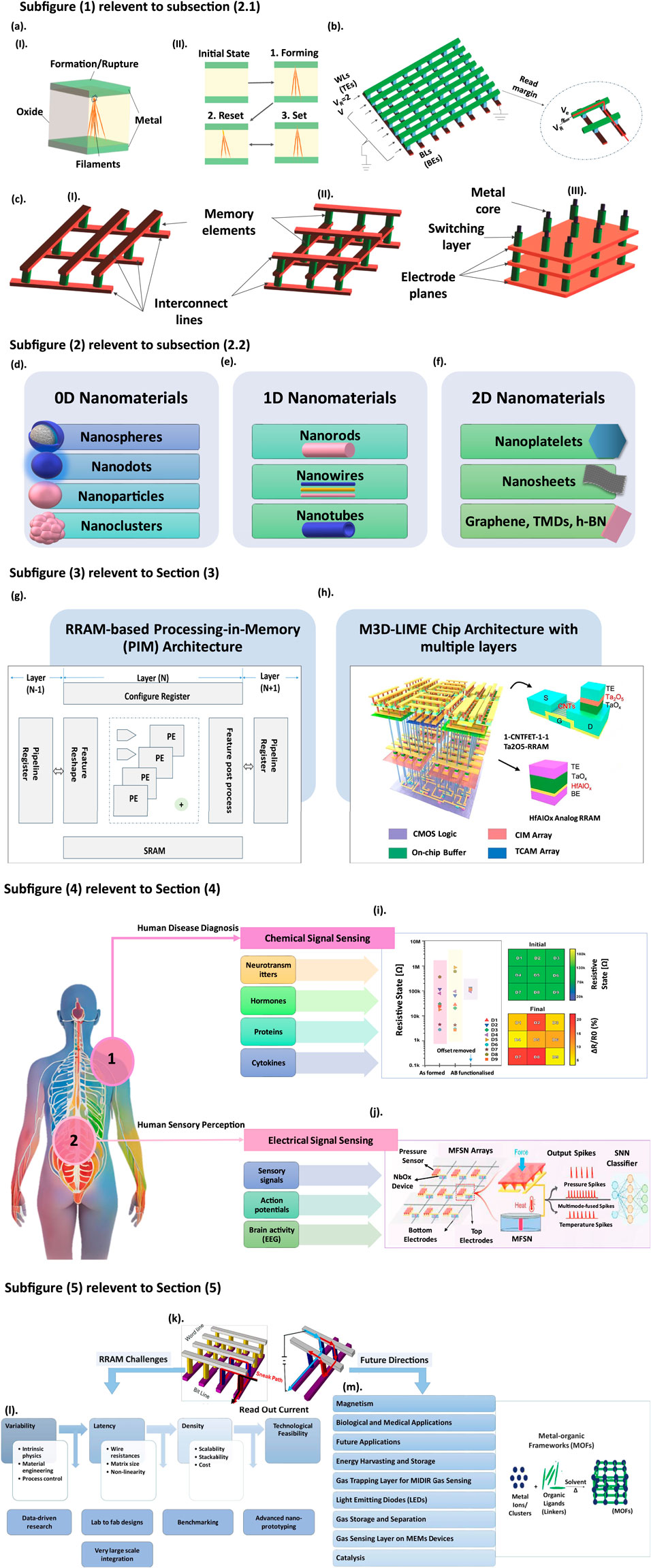
Figure 1. Comprehensive overview of RRAM structures, nano-materials, and characteristics. (a) Conductive filament dynamics and resistive switching processes. (a.I): Atomic-scale filament formation. (a.II): Operational phases. (b) Crossbar topology with word lines (WLs) and bit lines (BLs) and voltage distribution in the reading scheme. (c) RRAM crossbar array architectures and scaling approaches. (c.I): Planar crossbar array: Single-layer configuration with memory cells at word-line bit-line intersections. (c.II): Horizontal 3D stacking (HRRAM). (c.III): Vertical 3D architecture (VRRAM). (d) 0D nano-materials used in RRAMs. (e) 1D nano-materials used in RRAMs. (f) 2D nano-materials used in RRAMs. (g) Proposed M3D-LIME Chip Architecture with multiple layers. (h) RRAM-based Processing-in-Memory (PIM) Architecture. (i) RRAM array for multisensory perception. (j) Device-level calibration of the PSA sensing array baseline. (k) Sneak path effect that corrupts the stored data and lead to erroneous readouts. (l) examples of RRAM challenges. (m) Proposed solution: synthesis methods and applications of metal-organic frameworks (MOFs). (a) Reprinted from Sawa (2008). Copyright (2008), with permission from Elsevier. (b, c, g, h, j, k, l, m) reprinted with modification and permission. “Creative Commons Attribution” from (Chen et al., 2024; Wang Chenyu et al., 2023; Wang Hongzhe et al., 2023; Li Yijun et al., 2023; Tzouvadaki et al., 2020a; Shi et al., 2020; Adam et al., 2018; Li et al., 2024). (i) Reprinted from Zhu et al. (2022). Copyright (2022), with permission from John Wiley and Sons.
2.1.2 Crossbar array structure
In crossbar array structures (Figure 1b), RRAM cells are placed at the intersections of perpendicular electrode lines, allowing a higher integration density than the planar configuration. Crossbar arrays have the potential for cell sizes as small as 4F2, where F is the minimum feature size, resulting in storage densities exceeding 100 Gb/cm2 (Ali et al., 2016). However, crossbar arrays are plagued by sneak path currents that corrupt stored data and can lead to erroneous readouts (Huang et al., 2011; Son and Min, 2014). To overcome this problem, selector devices, including diodes or transistors, are added to RRAM cells to control sneak currents and enhance the reliability of the array (Linn et al., 2010; Yin Chee et al., 2022).
2.1.3 3D RRAM structure
3D RRAM structures (Figure 1c) utilize vertical space in the three dimensions to construct multilayer memory arrays and increase storage capacity with a reduced footprint (Deng et al., 2013). Its implementations can be broadly categorized into (i) Horizontal Stacked 3D RRAM (HRRAM) and (ii) vertical (VRRAM) schemes, which are distinguished to scalability and fabrication complexity (Kim and Li, 2020).
HRRAM is a memory architecture composed of multiple planar 2D RRAM layers superimposed as shown in Figure 1cII. (Chen et al., 2021; Wang et al., 2015). Wang et al. fabricated a 3D RRAM cube by strategically stacking a 2D
The conventional horizontal crossbar array structure is vertically extended for 3D VRRAM (Figure 1cIII; Chen et al., 2022). It is organized in two configurations: The plane word line (WL) structure and the even/odd WL structure (Kim and Li, 2020). It shows increased storage capacity with a small footprint (Kim and Li, 2020; Yue et al., 2014). In particular, compared to 2D crossbar array, 3D VRRAM with an even/odd WL structure occupies only
Structural innovations introduced in these devices give rise to next-generation memory solutions in diverse computing applications (Ielmini and Wong, 2018).
2.2 Nano materials in RRAM structures
Nanomaterials thanks to their properties at the nanoscale, have been revolutionizing modern science and technology. Unlike their rival bulks, Nano-materials’s size and shape determine their unique optical, chemical, thermal, electrical, mechanical, and catalytic properties (Mekuye and Abera, 2023). Nanomaterials are generally known for their dimensional configuration diversification ranging between 1 and 100 nm (Baig et al., 2021). These invaluable features pave the way for emergent fields of applications citing, energy storage and conversion (Yu et al., 2024), environment pollutants degradation (Saroha et al., 2023), disease diagnosis and drug delivery (Karnwal et al., 2024; Singh et al., 2022; Singh et al., 2023), as well as memory architectures like RRAMs (Xu and Liu, 2024). In general, nanomaterials are important for RRAMs’ operational characteristics (Xiao et al., 2023). Indicatively, various memristive metrics such as resistive switching behaviour, ON/OFF ratio, set/reset voltages, retention time, endurance and power consumption (Zayer Fakhreddine et al., 2019) are directly linked to the RRAM nanomaterial properties, namely, the electrical, physical, thermal and mechanical properties (Dongale et al., 2016; Shen et al., 2020; Das et al., 2023). Hence, it is essential to study the different memristive materials dimensional configuration categories (0D, 1D, 2D) (Panisilvam et al., 2024). Numerous nano-materials can be employed for the fabrication of RRAMs, the most common ones are metal oxides, perovskites, and organic materials. Furthermore, other nanomaterials contribute to RRAM modelling, for instance, transition metal oxides (TMOs), and transition metal dichalcogenides (TMDs), etc. Table A1 of the appendix and Figures 1d–f outline the categories of nanomaterials used in RRAMs according to their dimensions.
2.2.1 0D nano-materials in RRAMs
0D carbon nano-materials like carbon nano-dots, graphene quantum dots, and fullerenes assist in memristive functional layer growth through the generation of directional conductive filament formation (Yang Fan et al., 2024). Furthermore, metallic nanoparticles (MNPs) like copper
2.2.2 1D nano-materials in RRAMs
1D nano-materials, thanks to their nanoscale size, unique transport and high electric conductivity at low voltage, are widely used in RRAMs (Zhang et al., 2023). For instance, 1D carbon nano-materials are proven to be good candidates for elaborating highly performing RRAMs because of device stability and weight tunability (Patil et al., 2024). Moreover, metal oxide nanowires and nanorods, such as zinc oxide
2.2.3 2D nano-materials in RRAMs
2D nano-materials are known for ultra-thin, flexible, and layered structures (Muqeet Rehman et al., 2020). 2D TMDs semiconductors such as tungsten disulfide
3 3D RRAM for in-memory computing for biomedical applications
3D RRAM-based systems provide better performance and energy efficiency than traditional computing architectures by computing directly within the memory array (Yan et al., 2019). Several 3D RRAM-based in-memory computing architectures have been proposed and demonstrated in recent years. Wang et al. propose a 3D RRAM-based processing-in-memory (PIM) architecture (Figure 1g) that integrates 3D RRAM with CMOS logic, enabling in situ computation with reduced data movement and energy (Wang Hongzhe et al., 2023). For heavy-load convolutional neural network (CNN) algorithms, the RRAM PIM generally outperforms other architectures in terms of identification rate, speed, and image size (Burr et al., 2017). Building on this, Yao et al. developed a 3D RRAM-based neuromorphic computing system for more efficient processing and analyzing of large-scale datasets, such as images and time series. This system achieved a classification accuracy of 97.8% on the Modified National Institute of Standards and Technology (MNIST) dataset (Peng et al., 2020). Hao et al. presented a study of computing-in-memory macro based on 3D RRAM in 2022. Their work was based on a 3D VRRAM in which they introduced a two-kilobit non-volatile computing-in-memory (nvCIM) macro. They demonstrated an energy efficiency of 8.32 tera-operations per second per watt on 3D vector-matrix multiplication operations. This advancement can facilitate more efficient management of medical imaging data, improving diagnostic accuracy (Wang Tian-Yu et al., 2021). It was found that the brain Magnetic Resonance Imaging (MRI) edge detection performance and the inference accuracy on the Canadian Institute for Advanced Research (CIFAR-10) dataset were improved compared to conventional approaches (Wang Tian-Yu et al., 2021). In 2023, Li et al. recently introduced the monolithic three-dimensional integration of hybrid memory architecture based on RRAM, named M3D-LIME. This M3D-LIME chip (Figure 1h) demonstrated approximately 96% accuracy on the Omniglot dataset with 18.3
4 3D RRAM for multi-sensing arrays for biomedical applications
RRAM architectures can be integrated as chemical signal sensors for disease biomarker detection (Bouzouita et al., 2024) and drug delivery and electrical signal sensors for human multi-sensory perception (Tzouvadaki et al., 2023). Indeed, thanks to their efficient ON/OFF ratio, fast response, low power requirements, low cost, etc. RRAMs can be implemented as highly performing bio/chemical sensing arrays (Tzouvadaki et al., 2020b). In this case, each RRAM layer or section is transformed into a single sensing device, leveraging advancements in memristive nano-materials and biomedical technologies, like lab-on-chip perspectives to simultaneously harvesting multiple responses. In 2018, Adeyemo et al. simulated the detection of three different gases with an 8 × 8 memristive crossbar array. The simulation results highlighted a ten-times increase in accuracy compared to single biosensing devices (Adeyemo et al., 2018). In 2020, Ioulia Tzouvadaki et al. demonstrated a chemical biosensor array (Figure 1i) directly transducing Prostate Specific Antigen (PSA) cancer biomarker concentration levels to discrete memory states, expressing a device-level calibration of the sensing array baseline (Tzouvadaki et al., 2020a). Moreover, in 2023, Doowon Lee et al. developed a highly accurate zirconium nitride
5 Challenges and future directions
5.1 Challenges of 3D RRAM technologies
While memristive crossbar arrays offer considerable potential in the biomedical field, they face numerous obstacles that could impact their development and implementation. While advancing RRAMs, certain critical barriers have emerged in both vertical and horizontal directions. In their work, Gina C. Adam et al. reported potential challenges of memristive matrix integration (Figures 1k, l) citing variability, density, latency, and technology feasibility (Adam et al., 2018). For instance, as RRAM arrays simulate processing operations, errors may appear due to large device variability. Indeed, memristive crossbar arrays often exhibit vast variability due to stoichiometric inconsistency of inharmonious temporal memristive dimensions. Specifically, the variability of selected cross-points within the RRAM causes signal decay (Chen and Lin, 2011). A.P. James et al. emphasized that the variability problem originates either from device-to-device variations, or the nonlinearity of latency and programming. In memristive crossbars, the current flowing through the metal wires (Figure 1k) causes the reduction of voltage drop through the structure. This problem induces the damage of metal wires. The elevation of resistance is a further challenge causing thermal crosstalk and energy loss. All these circumstances initiate signal integrity obstacles (Li et al., 2021; Xu et al., 2015). In general, RRAM challenges reflect on the correct functioning of memristive biosensing devices, therapeutic systems and neural prosthetics since these applications necessitate specific performance metrics citing the response time, sensitivity, stability, reliability, etc., which are linked originally to used memristive device and nanomaterials (Yang Yulong et al., 2024; Zhu et al., 2024).
Various works have been addressing RRAM challenges and proposing solutions. Several nanomaterials have shown a potential to solve some remaining RRAM bottlenecks. For example, it is proved that titanium nitride, while deposited as an atomic layer (ALD-TiN), reduces the penetration of titanium (Ti) atoms to hafnium oxide
5.2 Future directions
There are several significant research opportunities in the future for the 3D RRAM nanomaterial memristive architectures for biomedical applications. Among these, the interconnecting and evolving of new nanomaterials and 2D materials with CIM sensing, and computing properties are paramount. Materials like TMDs, graphene and metal-organic frameworks (MOFs) (Figure 1m) bear the pre-scientific potential to improve the device performance in high-density, multifunctional biomedical implants, diagnostics and real-time health monitoring devices (Zahoor et al., 2023). Specifically, MOFs, with their high surface area and tunable porosity, improve the switching behaviour of RRAM devices through increased ion mobility and low energy consumption. This makes them suitable for neuromorphic systems in implantation devices thus making them potential implant sources (Zhang et al., 2022; Li et al., 2024). Improving these materials to get the best switching characteristics is very important for protracted implantable devices (Shen et al., 2020). However, reducing device variability is an issue that has not yet been solved successfully. More investigations should be dedicated to the reliable suppression of device-to-device and cycle-to-cycle fluctuations to promote the stability of neuromorphic designs for neural computing: sensory signal processing in real-time, control of prosthetic devices, etc., (Shen et al., 2020; Donati and Valle, 2024; Buccelli et al., 2019). The bio-electronic interface is another area with considerable potential for advancement. Therefore, it is desirable that future studies must further extend efforts in stable and long-lasting operation of these interfaces in physiological conditions for the further effectiveness of implantable devices (Zahoor et al., 2023). Recent research on combining RRAM with CMOS chips has shown that on-chip processing is possible at an energy density close to 10 fJ/operation - a critical feature for implantable biomedical applications (Shen et al., 2020). Prospective work should focus on how to continue driving energy consumption down while, at the same time, enhancing the computational abilities of the end-devices, so that in the future it can be possible to perform local analysis and decision making of the data (Li Yijun et al., 2023; D’Agostino et al., 2024; Huo et al., 2022; Aziza et al., 2021). Furthermore, identifying ways in which 3D RRAM devices may be applied for closed-loop therapeutic systems, personalized healthcare, and AI-based diagnosis is another interesting research direction. Additionally, when combined with other new technologies like flexible electronics and biodegradable materials the 3D RRAM may revolutionize the wireless implantable and wearable medicine (Zahoor et al., 2023). Overall, these aspects can open new paths for the use of 3D RRAM nanomaterial memristive architectures in biomedical applications, significantly benefiting healthcare.
Author contributions
MB: Writing – original draft, Writing – review and editing. SP: Writing – original draft, Writing – review and editing. FZ: Supervision, Validation, Writing – review and editing. HB: Supervision, Validation, Writing – review and editing. IT: Conceptualization, Investigation, Methodology, Project administration, Supervision, Validation, Writing – review and editing.
Funding
The author(s) declare that financial support was received for the research and/or publication of this article. This work was supported by the Bijzonder Onderzoeksfonds under the Grant No. BOF.STG. 2023.0008.01 and the Tunisian Ministry of Higher Education and Scientific Research scholarship “bourse d’alternance” under the reference “2023-BALT-378”.
Conflict of interest
The authors declare that the research was conducted in the absence of any commercial or financial relationships that could be construed as a potential conflict of interest.
Generative AI statement
The author(s) declare that Generative AI was used in the creation of this manuscript. Generative AI was used to rewrite some sentences more academically.
Publisher’s note
All claims expressed in this article are solely those of the authors and do not necessarily represent those of their affiliated organizations, or those of the publisher, the editors and the reviewers. Any product that may be evaluated in this article, or claim that may be made by its manufacturer, is not guaranteed or endorsed by the publisher.
References
Adam, G. C., Ali, K., and Prodromakis, T. (2018). Challenges hindering memristive neuromorphic hardware from going mainstream. Nat. Commun. 9 (1), 5267. doi:10.1038/s41467-018-07565-4
Adeyemo, A., Mathew, J., Jabir, A., Di Natale, C., Martinelli, E., and Ottavi, M. (2018). Efficient sensing approaches for high-density memristor sensor array. J. Comput. Electron. 17, 1285–1296. doi:10.1007/s10825-018-1176-y
Ahn, C., Jiang, Z., Lee, C.-S., Chen, H.-Y., Liang, J., Liyanage, L. S., et al. (2015). 1d selection device using carbon nanotube fets for high-density cross-point memory arrays. IEEE Trans. Electron Devices 62 (7), 2197–2204. doi:10.1109/ted.2015.2433956
Ahn, E. C., Wong, H.-S. P., and Pop, E. (2018). Carbon nanomaterials for non-volatile memories. Nat. Rev. Mater. 3 (3), 18009–18015. doi:10.1038/natrevmats.2018.9
Ali, K., Ayliffe, P., and Prodromakis, T. (2016). High density crossbar arrays with sub-15 nm single cells via liftoff process only. Sci. Rep. 6 (1), 32614. doi:10.1038/srep32614
Aziza, H., Hamdioui, S., Fieback, M., Taouil, M., Moreau, M., Girard, P., et al. (2021). Multi-level control of resistive ram (rram) using a write termination to achieve 4 bits/cell in high resistance state. Electronics 10 (18), 2222. doi:10.3390/electronics10182222
Baig, N., Kammakakam, I., and Falath, W. (2021). Nanomaterials: a review of synthesis methods, properties, recent progress, and challenges. Mater. Adv. 2 (6), 1821–1871. doi:10.1039/d0ma00807a
Bankman, D., Messner, J., Gural, A., and Murmann, B. (2019). “Rram-based in-memory computing for embedded deep neural networks,” in 2019 53rd asilomar conference on signals, systems, and computers (IEEE), 1511–1515.
Barraj, I., Mestiri, H., and Masmoudi, M. (2024). Overview of memristor-based design for analog applications. Micromachines 15 (4), 505. doi:10.3390/mi15040505
Betal, A., Bera, J., Sharma, A., Rath, A. K., and Sahu, S. (2023). Charge trapped cds quantum dot embedded polymer matrix for a high speed and low power memristor. Phys. Chem. Chem. Phys. 25 (5), 3737–3744. doi:10.1039/d2cp05014e
Bouzouita, M., Zayer, F., and Belgacem, H. (2024). “Simulation of chemical engineering memristive biosensor,” in 2024 IEEE 7th international conference on advanced technologies, signal and image processing (ATSIP) (IEEE), 159–164.
Buccelli, S., Bornat, Y., Colombi, I., Ambroise, M., Martines, L., Pasquale, V., et al. (2019). A neuromorphic prosthesis to restore communication in neuronal networks. IScience 19, 402–414. doi:10.1016/j.isci.2019.07.046
Burr, G. W., Shelby, R. M., Sebastian, A., Kim, S., Kim, S., Sidler, S., et al. (2017). Neuromorphic computing using non-volatile memory. Adv. Phys. X 2 (1), 89–124. doi:10.1080/23746149.2016.1259585
Chen, A., and Lin, M.-R. (2011). “Variability of resistive switching memories and its impact on crossbar array performance,” in 2011 international reliability physics symposium (IEEE), MY–7.
Chen, Q., Wang, Z., Lin, M., Qi, X., Yu, Z., Wu, L., et al. (2021). Homogeneous 3d vertical integration of parylene-c based organic flexible resistive memory on standard cmos platform. Adv. Electron. Mater. 7 (2), 2000864. doi:10.1002/aelm.202000864
Chen, Y.-C., Sarkar, S., Gibbs, J. G., Huang, Y., Lee, J. C., Lin, C.-C., et al. (2022). Nano helical-shaped dual-functional resistive memory for low-power crossbar array application. ACS Appl. Eng. Mater. 1 (1), 252–257. doi:10.1021/acsaenm.2c00050
Chen, Z., Zhao, X., Bengel, C., Liu, F., Li, K., Menzel, S., et al. (2024). Assessment of functional performance in self-rectifying passive crossbar arrays utilizing sneak path current. Sci. Rep. 14 (1), 24682. doi:10.1038/s41598-024-74667-z
D’Agostino, S., Moro, F., Torchet, T., Demirağ, Y., Grenouillet, L., Castellani, N., et al. (2024). Denram: neuromorphic dendritic architecture with rram for efficient temporal processing with delays. Nat. Commun. 15 (1), 3446. doi:10.1038/s41467-024-47764-w
Das, N. C., Kim, Y.-P., Hong, S.-M., and Jang, J.-H. (2023). Effects of top and bottom electrodes materials and operating ambiance on the characteristics of mgfx based bipolar rrams. Nanomaterials 13 (6), 1127. doi:10.3390/nano13061127
Deng, Y., Chen, H.-Y., Gao, B., Yu, S., Wu, S.-C., Zhao, L., et al. (2013). “Design and optimization methodology for 3d rram arrays,” in 2013 IEEE international electron devices meeting (IEEE), 25–27.
Donati, E., and Valle, G. (2024). Neuromorphic hardware for somatosensory neuroprostheses. Nat. Commun. 15 (1), 556. doi:10.1038/s41467-024-44723-3
Dongale, T. D., Khot, K. V., Mohite, S. V., Khandagale, S. S., Shinde, S. S., Moholkar, A. V., et al. (2016). Investigating the temperature effects on resistive random access memory (rram) devices. arXiv Prepr. doi:10.48550/arXiv.1602.08262
Fang, Y., Yu, Z., Wang, Z., Zhang, T., Yang, Y., Cai, Y., et al. (2018). Improvement of hfo x-based rram device variation by inserting ald tin buffer layer. IEEE Electron Device Lett. 39 (6), 819–822. doi:10.1109/led.2018.2831698
Fang Lu, X., Zhang, Y., Wang, N., Luo, S., Peng, K., Wang, L., et al. (2021). Exploring low power and ultrafast memristor on p-type van der waals sns. Nano Lett. 21 (20), 8800–8807. doi:10.1021/acs.nanolett.1c03169
Homsi, R., Al-Azzam, N., Baker, M., and Alazzam, A. (2023). Memristive biosensors for cancer biomarkers detection: a review. Ieee Access 11, 19347–19361. doi:10.1109/access.2023.3248683
Huang, C.-Y., Ho, Y.-T., Hung, C.-J., and Tseng, T.-Y. (2014). Compact ga-doped zno nanorod thin film for making high-performance transparent resistive switching memory. IEEE Trans. Electron Devices 61 (10), 3435–3441. doi:10.1109/ted.2014.2343631
Huang, J.-J., Tseng, Y.-M., Hsu, C.-W., and Hou, T.-H. (2011). Bipolar nonlinear $\hbox{Ni/TiO}_{2}\hbox{/}\hbox{Ni}$ selector for 1S1R crossbar array applications. IEEE Electron Device Lett. 32 (10), 1427–1429. doi:10.1109/led.2011.2161601
Huang, T.-K., Chen, J.-Y., Ting, Y.-H., and Wu, W.-W. (2018). Ni/nio/hfo2 core/multishell nanowire reram devices with excellent resistive switching properties. Adv. Electron. Mater. 4 (11), 1800256. doi:10.1002/aelm.201800256
Huh, W., Lee, D., and Lee, C.-H. (2020). Memristors based on 2d materials as an artificial synapse for neuromorphic electronics. Adv. Mater. 32 (51), 2002092. doi:10.1002/adma.202002092
Huo, Q., Yang, Y., Wang, Y., Lei, D., Fu, X., Ren, Q., et al. (2022). A computing-in-memory macro based on three-dimensional resistive random-access memory. Nat. Electron. 5 (7), 469–477. doi:10.1038/s41928-022-00795-x
Ielmini, D., and Wong, H.-S. P. (2018). In-memory computing with resistive switching devices. Nat. Electron. 1 (6), 333–343. doi:10.1038/s41928-018-0092-2
Jean Yoon, K., Han, J.-W., Moon, D.-I., Seol, M. L., Meyyappan, M., Kim, H. J., et al. (2019). Electrically-generated memristor based on inkjet printed silver nanoparticles. Nanoscale Adv. 1 (8), 2990–2998. doi:10.1039/c9na00329k
Karnwal, A., Yasser Jassim, A., Mohammed, A. A., Sharma, V., Sivanesan, I., and Sivanesan, I. (2024). Nanotechnology for healthcare: plant-derived nanoparticles in disease treatment and regenerative medicine. Pharmaceuticals 17 (12), 1711. doi:10.3390/ph17121711
Kim, B., and Li, H. (2020). “Leveraging 3d vertical rram to developing neuromorphic architecture for pattern classification,” in 2020 IEEE computer society annual symposium on VLSI (ISVLSI) (IEEE), 258–263.
Kim, J. P., Kim, S. K., Park, S., Kuk, S.-H., Kim, T., Kim, B.H., et al. (2023). Dielectric-engineered high-speed, low-power, highly reliable charge trap flash-based synaptic device for neuromorphic computing beyond inference. Nano Lett. 23 (2), 451–461. doi:10.1021/acs.nanolett.2c03453
Kim, Y. N., Lee, N. H., Yun, D. Y., and Kim, T. W. (2015). Multilevel characteristics and operating mechanisms of nonvolatile memory devices based on a floating gate of graphene oxide sheets sandwiched between two polystyrene layers. Org. Electron. 25, 165–169. doi:10.1016/j.orgel.2015.06.028
Kwon, J. Y., Kim, J. E., Kim, J. S., Chun, S. Y., Soh, K., and Yoon, J. H. (2024). Artificial sensory system based on memristive devices. Exploration 4, 20220162. doi:10.1002/exp.20220162
Lee, D., Chae, M., Jung, J., and Kim, H.-D. (2023). Correlation between sensing accuracy and read margin of a memristor-based no gas sensor array estimated by neural network analysis. ACS sensors 8 (5), 2105–2114. doi:10.1021/acssensors.3c00541
Lee, M.-J., Lee, C. B., Lee, D., Lee, S. R., Chang, M., Hur, Ji H., et al. (2011). A fast, high-endurance and scalable non-volatile memory device made from asymmetric ta2o5-x/tao2-x bilayer structures. Nat. Mater. 10 (8), 625–630. doi:10.1038/nmat3070
Li, D., Yadav, A., Zhou, H., Roy, K., Thanasekaran, P., and Lee, C. (2024). Advances and applications of metal-organic frameworks (mofs) in emerging technologies: a comprehensive review. Glob. Challenges 8 (2), 2300244. doi:10.1002/gch2.202300244
Li, H., Wang, S., Zhang, X., Wang, W., Yang, R., Zhong, S., et al. (2021). Memristive crossbar arrays for storage and computing applications. Adv. Intell. Syst. 3 (9), 2100017. doi:10.1002/aisy.202100017
Li, Q., Liu, M., Zhang, Y., and Liu, Z. (2016). Hexagonal boron nitride–graphene heterostructures: synthesis and interfacial properties. Small 12 (1), 32–50. doi:10.1002/smll.201501766
Li, W., Wan, J., Tu, Z., Li, H., Wu, H., and Liu, C. (2022). Optimizing endurance performance of ga2o3 random resistive access memories by altering oxygen vacancy content. Ceram. Int. 48 (3), 3185–3191. doi:10.1016/j.ceramint.2021.10.091
Li, Y., Tang, J., Gao, B., Yao, J., Fan, A., Yan, B., et al. (2023a). Monolithic three-dimensional integration of rram-based hybrid memory architecture for one-shot learning. Nat. Commun. 14 (1), 7140. doi:10.1038/s41467-023-42981-1
Li, Y., Wang, Z., Midya, R., Xia, Q., and Yang, J. J. (2018). Review of memristor devices in neuromorphic computing: materials sciences and device challenges. J. Phys. D Appl. Phys. 51 (50), 503002. doi:10.1088/1361-6463/aade3f
Li, Z., Tang, W., Zhang, B., Yang, R., and Miao, X. (2023b). Emerging memristive neurons for neuromorphic computing and sensing. Sci. Technol. Adv. Mater. 24 (1), 2188878. doi:10.1080/14686996.2023.2188878
Linn, E., Rosezin, R., Kügeler, C., and Waser, R. (2010). Complementary resistive switches for passive nanocrossbar memories. Nat. Mater. 9 (5), 403–406. doi:10.1038/nmat2748
Liu, P., Hui, F., Aguirre, F., Saiz, F., Tian, L., Han, T., et al. (2022). Nano-memristors with 4 mv switching voltage based on surface-modified copper nanoparticles. Adv. Mater. 34 (20), 2201197. doi:10.1002/adma.202201197
Lu, W., and Lieber, C. M. (2007). Nanoelectronics from the bottom up. Nat. Mater. 6 (11), 841–850. doi:10.1038/nmat2028
Mekuye, B., and Abera, B. (2023). Nanomaterials: an overview of synthesis, classification, characterization, and applications. Nano Sel. 4 (8), 486–501. doi:10.1002/nano.202300038
Milano, G., Porro, S., Valov, I., and Ricciardi, C. (2019). Recent developments and perspectives for memristive devices based on metal oxide nanowires. Adv. Electron. Mater. 5 (9), 1800909. doi:10.1002/aelm.201800909
Milozzi, A., Ricci, S., and Ielmini, D. (2024). Memristive tonotopic mapping with volatile resistive switching memory devices. Nat. Commun. 15 (1), 2812. doi:10.1038/s41467-024-47228-1
Moon, K., Lim, S., Park, J., Sung, C., Oh, S., Woo, J., et al. (2019). Rram-based synapse devices for neuromorphic systems. Faraday Discuss. 213, 421–451. doi:10.1039/c8fd00127h
Muqeet Rehman, M., Rehman, H. M. M.U., Gul, J. Z., Kim, W. Y., Karimov, K. S., and Ahmed, N. (2020). Decade of 2d-materials-based rram devices: a review. Sci. Technol. Adv. Mater. 21 (1), 147–186. doi:10.1080/14686996.2020.1730236
Panisilvam, J., Lee, Ha Y., Byun, S., Fan, D., and Kim, S. (2024). Two-dimensional material-based memristive devices for alternative computing. Nano Converg. 11 (1), 25. doi:10.1186/s40580-024-00432-7
Pappachen James, A., and Chua, L. O. (2022). Variability-aware memristive crossbars—a tutorial. IEEE Trans. Circuits Syst. II Express Briefs 69 (6), 2570–2574. doi:10.1109/tcsii.2022.3169416
Park, J., Kim, T.-H., Kwon, O., Ismail, M., Mahata, C., Kim, Y., et al. (2022). Implementation of convolutional neural network and 8-bit reservoir computing in cmos compatible vrram. Nano Energy 104, 107886. doi:10.1016/j.nanoen.2022.107886
Patil, S. L., Pawar, O. Y., Dongale, T. D., Chang, S., Lim, S., and Song, Y. M. (2024). Recent advancements in carbon-based materials for resistive switching applications. Carbon 228, 119320. doi:10.1016/j.carbon.2024.119320
Peng, Y., Wu, H., Gao, B., Tang, J., Zhang, Q., Zhang, W., et al. (2020). Fully hardware-implemented memristor convolutional neural network. Nature 577 (7792), 641–646. doi:10.1038/s41586-020-1942-4
Qian, K., Tay, R. Y., Nguyen, V. C., Wang, J., Cai, G., Chen, T., et al. (2016). Hexagonal boron nitride thin film for flexible resistive memory applications. Adv. Funct. Mater. 26 (13), 2176–2184. doi:10.1002/adfm.201504771
Saroha, J., Rani, E., Devi, M., Pathi, P., Kumar, M., and Sharma, S. N. (2023). Plasmon-assisted photocatalysis of organic pollutants by au/ag–tio2 nanocomposites: a comparative study. Mater. Today Sustain. 23, 100466. doi:10.1016/j.mtsust.2023.100466
Sawa, A. (2008). Resistive switching in transition metal oxides. Mater. today 11 (6), 28–36. doi:10.1016/s1369-7021(08)70119-6
Shen, Z., Zhao, C., Qi, Y., Xu, W., Liu, Y., Mitrovic, I. Z., et al. (2020). Advances of rram devices: resistive switching mechanisms, materials and bionic synaptic application. Nanomaterials 10 (8), 1437. doi:10.3390/nano10081437
Shi, L., Zheng, G., Tian, B., Dkhil, B., and Duan, C. (2020). Research progress on solutions to the sneak path issue in memristor crossbar arrays. Nanoscale Adv. 2 (5), 1811–1827. doi:10.1039/d0na00100g
Simmons, J. G., and Verderber, R. R. (1967). New conduction and reversible memory phenomena in thin insulating films. Proc. R. Soc. Lond. Ser. A. Math. Phys. Sci. 301 (1464), 77–102. doi:10.1098/rspa.1967.0191
Singh, D. K., and Gupta, G. (2024). Brain-inspired computing: can 2d materials bridge the gap between biological and artificial neural networks? Mater. Adv. 5 (8), 3158–3172. doi:10.1039/d4ma00133h
Singh, D. K., Pant, R. K., Nanda, K. K., and Krupanidhi, S. B. (2022). Pulsed laser deposition for conformal growth of mos 2 on gan nanorods for highly efficient self-powered photodetection. Mater. Adv. 3 (15), 6343–6351. doi:10.1039/d2ma00577h
Singh, D. K., Prajapat, P., Saroha, J., Pant, R. K., Sharma, S. N., Kar Nanda, K., et al. (2023). Photocurrent polarity switching and enhanced photoresponse in silver nanoparticles decorated a-gan-based photodetector. ACS Appl. Electron. Mater. 5 (3), 1394–1400. doi:10.1021/acsaelm.2c01549
Singh, P., Simanjuntak, F. M., Kumar, A., and Tseng, T.-Y. (2018). Resistive switching behavior of ga doped zno-nanorods film conductive bridge random access memory. Thin Solid Films 660, 828–833. doi:10.1016/j.tsf.2018.03.027
Son, N. T., and Min, K.-S. (2014). New memristor-based crossbar array architecture with 50-% area reduction and 48-% power saving for matrix-vector multiplication of analog neuromorphic computing. JSTS J. Semicond. Technol. Sci. 14 (3), 356–363. doi:10.5573/jsts.2014.14.3.356
Stelling, C., and Retsch, M. (2018). Nanomeshes at liquid interfaces: from free-standing hole arrays toward metal–insulator–metal architectures. Adv. Mater. Interfaces 5 (10), 1800154. doi:10.1002/admi.201800154
Strukov, D. B., Snider, G. S., Stewart, D. R., and Williams, R. S. (2008). The missing memristor found. nature 453 (7191), 80–83. doi:10.1038/nature06932
Tang, Y., Lei, P., Liao, K., Jiang, T., Chen, S., Xie, Q., et al. (2021). Observation of nonvolatile resistive switching behaviors in 2d layered inse nanosheets through controllable oxidation. Appl. Phys. Lett. 119 (13). doi:10.1063/5.0061792
Tzouvadaki, I., De Micheli, G., and Carrara, S. (2020b). Memristive biosensors for ultrasensitive diagnostics and therapeutics. Appl. Emerg. Mem. Technol. Beyond Storage, 133–157. doi:10.1007/978-981-13-8379-3_5
Tzouvadaki, I., Gkoupidenis, P., Vassanelli, S., Wang, S., and Prodromakis, T. (2023). Interfacing biology and electronics with memristive materials. Adv. Mater. 35 (32), 2210035. doi:10.1002/adma.202210035
Tzouvadaki, I., Stathopoulos, S., Abbey, T., Michalas, L., and Prodromakis, T. (2020a). Monitoring psa levels as chemical state-variables in metal-oxide memristors. Sci. Rep. 10 (1), 15281. doi:10.1038/s41598-020-71962-3
Wang, C., Shi, G., Qiao, F., Lin, R., Wu, S., and Hu, Z. (2023a). Research progress in architecture and application of rram with computing-in-memory. Nanoscale Adv. 5 (6), 1559–1573. doi:10.1039/d3na00025g
Wang, C.-C., Chiou, Y.-K., Chang, C.-H., Tseng, J.-Y., Wu, L.-J., Chen, C.-Y., et al. (2007). Memory characteristics of au nanocrystals embedded in metal–oxide–semiconductor structure by using atomic-layer-deposited al2o3 as control oxide. J. Phys. D Appl. Phys. 40 (6), 1673–1677. doi:10.1088/0022-3727/40/6/016
Wang, H., Wang, J., Hu, H., Guo, L., Hu, S., Qi, Y., et al. (2023b). Ultra-high-speed accelerator architecture for convolutional neural network based on processing-in-memory using resistive random access memory. Sensors 23 (5), 2401. doi:10.3390/s23052401
Wang, M., Cai, S., Chen, P., Wang, C., Lian, X., Zhuo, Y., et al. (2018a). Robust memristors based on layered two-dimensional materials. Nat. Electron. 1 (2), 130–136. doi:10.1038/s41928-018-0021-4
Wang, M., Zhou, J., Yang, Y., Gaba, S., Liu, M., and Lu, W. D. (2015). Conduction mechanism of a tao x-based selector and its application in crossbar memory arrays. Nanoscale 7 (11), 4964–4970. doi:10.1039/c4nr06922f
Wang, S., Ning, X., Aize, H., and Chen, R. (2022). Metal nanoparticles layer boosted resistive switching property in nife2o4-based memory devices. J. Alloys Compd. 908, 164569. doi:10.1016/j.jallcom.2022.164569
Wang, T., Huang, H.-M., Wang, X.-X., and Guo, X. (2021b). An artificial olfactory inference system based on memristive devices. InfoMat 3 (7), 804–813. doi:10.1002/inf2.12196
Wang, T.-Y., Jia-Lin, M., Lin, C., Zhu, H., Sun, Q.-Q., Ding, S.-J., et al. (2021a). Flexible 3d memristor array for binary storage and multi-states neuromorphic computing applications. InfoMat 3 (2), 212–221. doi:10.1002/inf2.12158
Wang, X.-F., Tian, H., Zhao, H.-M., Zhang, T.-Y., Mao, W.-Q., Qiao, Y.-C., et al. (2018b). Interface engineering with mos2–pd nanoparticles hybrid structure for a low voltage resistive switching memory. Small 14 (2), 1702525. doi:10.1002/smll.201702525
Wen, X., Zhu, L. Q., Ye, C., Ren, Z.Y., Yu, F., Xiao, H., et al. (2020). Flexible poly (vinyl alcohol)–graphene oxide hybrid nanocomposite based cognitive memristor with pavlovian-conditioned reflex activities. Adv. Electron. Mater. 6 (5), 1901402. doi:10.1002/aelm.201901402
Xiang, H., Yan, X., Liu, C., Ding, S., Zhang, D. W., and Zhou, P. (2018). Operation mode switchable charge-trap memory based on few-layer mos2. Semicond. Sci. Technol. 33 (3), 034001. doi:10.1088/1361-6641/aaa79e
Xiao, Y., Jiang, B., Zhang, Z., Ke, S., Jin, Y., Wen, X., et al. (2023). A review of memristor: material and structure design, device performance, applications and prospects. Sci. Technol. Adv. Mater. 24 (1), 2162323. doi:10.1080/14686996.2022.2162323
Xu, C., Niu, D., Muralimanohar, N., Balasubramonian, R., Zhang, T., Yu, S., et al. (2015). “Overcoming the challenges of crossbar resistive memory architectures,” in 2015 IEEE 21st international symposium on high performance computer architecture (HPCA) (IEEE), 476–488.
Xu, W., and Liu, J. (2024). “Nanomaterials in nonvolatile resistive memory devices,” in Handbook of nanomaterials (Elsevier), 57–79.
Yan, B., Li, B., Qiao, X., Xue, C.-X., Chang, M.-F., Chen, Y., et al. (2019). Resistive memory-based in-memory computing: from device and large-scale integration system perspectives. Adv. Intell. Syst. 1 (7), 1900068. doi:10.1002/aisy.201900068
Yan, X., Han, X., Fang, Z., Zhao, Z., Zhang, Z., Sun, J., et al. (2023a). Reconfigurable memristor based on srtio3 thin-film for neuromorphic computing. Front. Phys. 18 (6), 63301. doi:10.1007/s11467-023-1308-0
Yan, X., Zhang, Y., Fang, Z., Sun, Y., Liu, P., Sun, J., et al. (2023b). A multimode-fused sensory memory system based on a robust self-assembly nanoscaffolded BaTiO3:Eu2O3 memristor. InfoMat 5 (9), e12429. doi:10.1002/inf2.12429
Yang, F., Liu, Z., Ding, X., Li, Y., Wang, C., and Shen, G. (2024a). Carbon-based memristors for resistive random access memory and neuromorphic applications. Chip 3 (2), 100086. doi:10.1016/j.chip.2024.100086
Yang, Y., Sun, B., Suo Mao, S., Qin, J., Yang, Y., Liu, M., et al. (2024b). Biomedical applications of sensing devices with memristive behaviors. J. Mater. Chem. C 12, 13762–13783. doi:10.1039/d4tc02289k
Yin Chee, M., Dananjaya, P. A., Lim, G. J., Du, Y., and Wen, S. L. (2022). Frequency-dependent synapse weight tuning in 1S1R with a short-term plasticity TiOx-based exponential selector. ACS Appl. Mater. and Interfaces 14 (31), 35959–35968. doi:10.1021/acsami.2c11016
Yoon, J. H., Song, Y.-W., Ham, W., Park, J.-M., and Kwon, J.-Y. (2023). A review on device requirements of resistive random access memory (rram)-based neuromorphic computing. Apl. Mater. 11 (9). doi:10.1063/5.0149393
Yu, G., Huang, J., Bai, X., Li, T., Song, S., Zhou, Y., et al. (2024). Engineering of cerium modified tinb2o7 nanoparticles for low-temperature lithium-ion battery. Small 20 (34), 2308858. doi:10.1002/smll.202308858
Yu, M., Cai, Y., Wang, Z., Fang, Y., Liu, Y., Yu, Z., et al. (2016). Novel vertical 3d structure of taox-based rram with self-localized switching region by sidewall electrode oxidation. Sci. Rep. 6 (1), 21020. doi:10.1038/srep21020
Yue, B., Wu, H., Wang, K., Wu, R., Lin, S., Li, T., et al. (2015). Stacked 3d rram array with graphene/cnt as edge electrodes. Sci. Rep. 5 (1), 13785. doi:10.1038/srep13785
Yue, B., Wu, H., Wu, R., Zhang, Y., Deng, N., Yu, Z., et al. (2014). Study of multi-level characteristics for 3d vertical resistive switching memory. Sci. Rep. 4 (1), 1–7. doi:10.1038/srep05780
Zahoor, F., Hussin, F. A., Isyaku, U. B., Gupta, S., Ahmad Khanday, F., Chattopadhyay, A., et al. (2023). Resistive random access memory: introduction to device mechanism, materials and application to neuromorphic computing. Discov. Nano 18 (1), 36. doi:10.1186/s11671-023-03775-y
Zahoor, F., Zulkifli, T. Z. A., and Khanday, F. A. (2020). Resistive random access memory (rram): an overview of materials, switching mechanism, performance, multilevel cell (mlc) storage, modeling, and applications. Nanoscale Res. Lett. 15, 1–26. doi:10.1186/s11671-020-03299-9
Zayer, F., Dghais, W., and Belgacem, H. (2019a). Modeling framework and comparison of memristive devices and associated stdp learning windows for neuromorphic applications. J. Phys. D Appl. Phys. 52 (39), 393002. doi:10.1088/1361-6463/ab24a7
Zayer, F., Lahbacha, K., Dghais, W., Belgacem, H., de Magistris, M., Melnikov, A. V., et al. (2019b). “Electrothermal analysis of 3d memristive 1d-1rram crossbar with carbon nanotube electrodes,” in 2019 IEEE international conference on design and test of integrated micro and nano-systems (DTS) (IEEE), 1–6.
Zhang, F., Zhang, H., Krylyuk, S., Milligan, C. A., Zhu, Y., Zemlyanov, D. Y., et al. (2019). Electric-field induced structural transition in vertical mote2-and mo1–x w x te2-based resistive memories. Nat. Mater. 18 (1), 55–61. doi:10.1038/s41563-018-0234-y
Zhang, L., Xu, K., and Wei, F. (2023). Fabrication of electronic switches based on low-dimensional nanomaterials: a review. J. Mater. Sci. 58 (5), 2087–2110. doi:10.1007/s10853-023-08177-0
Zhang, W., Taheri-Ledari, R., Saeidirad, M., Qazi, F. S., Kashtiaray, A., Ganjali, F., et al. (2022). Regulation of porosity in mofs: a review on tunable scaffolds and related effects and advances in different applications. J. Environ. Chem. Eng. 10 (6), 108836. doi:10.1016/j.jece.2022.108836
Zhou, F., Zhou, Z., Chen, J., Choy, T. H., Wang, J., Zhang, N., et al. (2019). Optoelectronic resistive random access memory for neuromorphic vision sensors. Nat. Nanotechnol. 14 (8), 776–782. doi:10.1038/s41565-019-0501-3
Zhu, J., Zhang, X., Wang, R., Wang, M., Chen, P., Cheng, L., et al. (2022). A heterogeneously integrated spiking neuron array for multimode-fused perception and object classification. Adv. Mater. 34 (24), 2200481. doi:10.1002/adma.202200481
Zhu, S., Cao, Z., Zhou, G., Tong, G., Ma, Y., Yang, W., et al. (2024). An implantable memristor towards biomedical applications. Appl. Mater. Today 38, 102214. doi:10.1016/j.apmt.2024.102214
Appendix
Keywords: nano-materials, memristor, resistive switching, crossbar, in-memory computing, biosensing arrays
Citation: Bouzouita M, Pathak S, Zayer F, Belgacem H and Tzouvadaki I (2025) Advanced memristive architectures based on nanomaterials for biomedical applications: a mini review. Front. Nanotechnol. 7:1558743. doi: 10.3389/fnano.2025.1558743
Received: 10 January 2025; Accepted: 27 March 2025;
Published: 23 April 2025.
Edited by:
Dimitra G. Georgiadou, University of Southampton, United KingdomReviewed by:
Pan Hu, University of Southern California, Los Angeles, United StatesDeependra Kumar Singh, National Institute of Technology Raipur, India
Copyright © 2025 Bouzouita, Pathak, Zayer, Belgacem and Tzouvadaki. This is an open-access article distributed under the terms of the Creative Commons Attribution License (CC BY). The use, distribution or reproduction in other forums is permitted, provided the original author(s) and the copyright owner(s) are credited and that the original publication in this journal is cited, in accordance with accepted academic practice. No use, distribution or reproduction is permitted which does not comply with these terms.
*Correspondence: Ioulia Tzouvadaki , aW91bGlhLnR6b3V2YWRha2lAdWdlbnQuYmU=
†These authors have contributed equally to this work