- 1Institute for Personalized Medicine, Pennsylvania State University College of Medicine-Milton S. Hershey Medical Center, Hershey, PA, United States
- 2Department of Industrial and Manufacturing Engineering, Pennsylvania State University, University Park, PA, United States
- 3Department of Public Health Sciences, Pennsylvania State University College of Medicine-Milton S. Hershey Medical Center, Hershey, PA, United States
- 4Department of Neurology, Pennsylvania State University College of Medicine-Milton S. Hershey Medical Center, Hershey, PA, United States
- 5Department of Pharmacology, Pennsylvania State University College of Medicine-Milton S. Hershey Medical Center, Hershey, PA, United States
- 6Department of Statistics, Pennsylvania State University, University Park, PA, United States
- 7Department of Radiology, Pennsylvania State University College of Medicine-Milton S. Hershey Medical Center, Hershey, PA, United States
- 8Department of Neurosurgery, Pennsylvania State University College of Medicine-Milton S. Hershey Medical Center, Hershey, PA, United States
- 9Department of Kinesiology, Pennsylvania State University College of Medicine-Milton S. Hershey Medical Center, Hershey, PA, United States
Objectives: A growing literature suggests that circulating cholesterol levels have been associated with Parkinson’s disease (PD). In this study, we investigated a possible causal basis for the cholesterol-PD link.
Methods: Fasting plasma cholesterol levels were obtained from 91 PD and 70 age- and gender-matched controls from an NINDS PD Biomarkers Program cohort at the Pennsylvania State University College of Medicine. Based on the literature, genetic polymorphisms in selected cholesterol management genes (APOE, LDLR, LRP1, and LRPAP1) were chosen as confounding variables because they may influence both cholesterol levels and PD risk. First, the marginal structure model was applied, where the associations of total- and LDL-cholesterol levels with genetic polymorphisms, statin usage, and smoking history were estimated using linear regression. Then, potential causal influences of total- and LDL-cholesterol on PD occurrence were investigated using a generalized propensity score approach in the second step.
Results: Both statins (p < 0.001) and LRP1 (p < 0.03) influenced total- and LDL-cholesterol levels. There also was a trend for APOE to affect total- and LDL-cholesterol (p = 0.08 for both), and for LRPAR1 to affect LDL-cholesterol (p = 0.05). Conversely, LDLR did not influence plasma cholesterol levels (p > 0.19). Based on propensity score methods, lower total- and LDL-cholesterol were significantly linked to PD (p < 0.001 and p = 0.04, respectively).
Conclusion: The current study suggests that circulating total- and LDL-cholesterol levels potentially may be linked to the factor(s) influencing PD risk. Further studies to validate these results would impact our understanding of the role of cholesterol as a risk factor in PD, and its relationship to recent public health controversies.
Introduction
Although the exact cause for Parkinson’s disease (PD) is unknown, behavioral and environmental factors have a strong effect on PD risk. A growing literature has provided evidence that circulating cholesterol is related to PD. First, case–control studies have found that higher plasma cholesterol is associated with reduced PD prevalence (1–4). Second, several prospective studies have indicated that low cholesterol predates the diagnosis of PD (5–9). Third, higher baseline cholesterol may be linked to slower PD progression (10), better cognitive and motor performance (11), and delayed age of PD onset (12).
Although these studies show clear and consistent associations, the observed cholesterol–PD relationship may not be causal. For example, PD diagnosis may prime for adoption of a “healthier” lifestyle, thereby leading to lower cholesterol, or indeed, there could be an unknown behavioral (e.g., smoking) or medical (e.g., use of statin) confounder. Alternatively, lower plasma cholesterol simply may reflect metabolic or non-motor changes that are associated with PD. Thus, the current study was designed to investigate a potential causal relationship between the cholesterol–PD link by studying a number of factors that may affect both circulating cholesterol levels and PD risk.
Materials and Methods
Cohort Subjects
Parkinson’s disease (n = 91) and control (n = 70) subjects (hereafter controls) with a Mini-Mental State Exam (MMSE) score ≥26 were selected from an ongoing cohort study that is part of the NINDS PD Biomarkers Program (13) at the Penn State site. PD patients were recruited from the Penn State Hershey Medical Center movement disorders clinic, and Controls free of any major neurological disorders were recruited from the spouse population and local community. PD and Controls were age-, gender-, and education-matched based on overall distribution. PD diagnosis was confirmed using published criteria (14). Subjects with signs of dementia were excluded using the MMSE score cutoff described above. All PD and control subjects also were free of neurological disorders other than PD, and free of major and acute medical issues such as liver, kidney, or thyroid abnormalities, and deficiencies of B12 or folate. All brain images were deemed free of any major structural abnormalities. Blood was collected after an 8–12 h overnight fast at the baseline visit. Although many metabolic factors may influence cholesterol or PD, the role of diabetes in PD is still in debate. In the current study, we collected fasting lipid profiles and statin usage as main factors of interest for this study. We did not assess either diagnosis of diabetes or drugs to treat diabetes, nor did we measure blood glucose or hemoglobin A1c levels. Written informed consent was obtained for all subjects in accordance with the Declaration of Helsinki. The Penn State Hershey Institutional Review Board approved the research study protocol.
Plasma Cholesterol
Plasma triglyceride, total-, and HDL-cholesterol levels were measured by enzymatic methods as described previously (11). LDL-cholesterol was calculated using the Friedwald equation:
Behavioral and Medical Information
Age, gender, statin usage, and history of smoking were obtained as part of a comprehensive demographic survey. Subjects were considered smokers if they had smoked one cigarette per day for 6 months or more at some point in the past (15). Subjects were considered statin users if they were using statins at the time of blood sampling.
NeuroX Genotyping Data Acquisition and Analysis
Single-nucleotide polymorphism (SNP) genotyping was performed on whole blood DNA samples extracted by the NINDS PDBP using the Illumina NeuroX array. A total 269,476 variants were genotyped, and the Genotyping Analysis Module within Genome Studio version 1.9.4 was used to call participant genotypes. The threshold call rate for sample inclusion was 95%. Concordance between reported sex and determined sex estimated from X chromosome heterogeneity was required for enrollment in the current study. X chromosome heterogeneity calculations were based on common SNPs from the International HapMap Project that had genotypes with missingness <5% and Hardy–Weinberg equilibrium p values > 10–5. Samples also were excluded if they were six SDs from the population mean rate of heterozygosity based on common polymorphisms (16).
The Klemann et al. (17) study of 13,094 PD and 47,148 controls showed that lipids and lipoproteins are involved functionally in, and affect, dopaminergic neuron-specific signaling cascades, providing insight into how lipids and lipoproteins may play a key role in PD. The APOE and amyloid-β (Aβ) pathways in the brain show APOE, LDLR, LRP1, and LRPAP1 are involved in the cycling of cholesterol and the delivery of lipids (18). Several other studies show that cholesterol recycling may be linked to PD (19–21). APOE and LRPAP1 SNPs are associated significantly with increased PD risk (22), and both APOE and its receptors (LDLR and LRP1) have been shown to be increased in striatal and hippocampal tissue after MPTP lesions (23). Wilhelmus et al. (24) also observed increased APOE and LRP1 expression in PD and Lewy body disease. Together, the literature provided a strong biological rationale to choose these genes for this study.
We extracted the APOE, LDLR, LRP1, and LRPAP1 SNPs from the NINDS NeuroX genotyping data. Within the NeuroX genotyping data, there are 40 allele loci for the LDLR that contain two major mutation SNPs (rs6511720 and rs2228671), 72 allele loci for LRP1 with three mutation SNPs (rs11172113, rs1466535 and rs1800127), and 17 allele loci for LRPAP1 that contain one major mutation SNP (rs11549516). GWAS studies with these SNPs show they have an association with lipids at p < 0.0001 (25, 26).
Statistical Analysis
For comparison of demographic data between PD and controls, Pearson chi-square tests or Fisher’s exact tests were applied for categorical variables (e.g., gender) and two-sample t-tests or Wilcoxon rank-sum tests were used for continuous variables (e.g., age), as appropriate in Table 1. The effects of genetic polymorphisms (LDLR, LRP1, LRPAP1, and APOE), age, gender, statin usage, and behavioral factors (smoking) on total- and LDL-cholesterol levels first were analyzed using linear regression analyses for estimating the propensity scores. To determine whether total- and LDL-cholesterol (exposures of interests) had a causal influence on PD occurrence, a marginal structure model with estimated propensity score (27, 28) was used next. Namely, the propensity score of being assigned to the exposure group given a set of observed potential confounders (e.g., genetic polymorphisms, age, gender, and behavioral factors) controls for differences in confounders between the exposure and non-exposure groups (29–31). Recently, Zhu et al. (28) described a boosting algorithm for estimating generalized propensity scores. The generalized propensity score was assumed to depend on a linear combination of the confounders, and the ignorability assumption was imposed such that it was sufficient to condition on the generalized propensity scores in order to adjust for confounding instead of conditioning on the vector of covariates (28).
If we let Y denote PD status (Yes = 1; No = 0), M be the continuous measurement (such as LDL- or total-cholesterol), and X be a p-dimensional vector of covariates (including genetic markers and environmental factors), then the observed data including n random samples can be represented as (Yi, Mi, Xi), i = 1, 2, …, n. For any value of the M from a continuous domain noted by m, the corresponding outcome is defined as Yi(m). Given the ignorability assumption described by Zhu et al. (28), the following marginal structural model (MSM) for the binary outcome is
where the parameters with standard errors (SE) can be estimated by inverse probability weights (IPW). Thus, the estimates of odds ratios with 95% confidence intervals (CIs) can be calculated without conditioning on any covariates. Of note, the weights can be obtained by the generalized propensity score that is the conditional density of observing m given the covariates denoted by r(m, X) = fm|X(m, X). Here, we consider a multiple regression model for the generalized propensity score, which is traditional and written by
where the considered covariates include age, gender, statin usage, smoking, APOE, LDLR, LRP1, and LRPAP1 gene SNPs. Following the procedures of Ji et al. (32), the weight for the ith subject is calculated by , where is the estimation of the propensity score. The analyses were conducted using R software (version 3.3.3) and the “Survey” package was used for parameter estimation.
Results
Demographic Characteristics of Study Subjects
Parkinson’s disease and controls were similar in age, gender, history of smoking, and statin usage (Table 1). Total- and LDL-cholesterol levels were significantly lower in the PD group (p = 0.03 and 0.04, respectively), whereas HDL and triglycerides were similar in the two groups. The majority of both control (80%) and PD (86%) subjects had the ε3ε3 phenotype, with the remaining control and PD subjects having the ε3ε2 phenotype. One PD subject had the ε2ε2 phenotype, and no subjects had an ε4 allele. PD and controls had similar proportions of the two LDLR SNPs investigated in our study (p > 0.10). In addition, there was no difference in the proportion of the three LRP1 SNPs between PDs and controls (p > 0.19), or the LRPAP1 SNP (p = 0.24).
The Effects of Age, Gender, Smoking, Statin Usage, and Genes on Blood Cholesterol Levels
Age and history of smoking did not influence significantly cholesterol levels in our cohort, whereas PD subjects had lower total- and LDL-cholesterol (Table 1). The histogram in Figure 1A and the QQ plot in Figure 1B show that LDL is approximately normally distributed (total-cholesterol also has an approximately normal distribution, not shown). We conducted multiple regression using our initial model (Table 2, Model I) that included APOE and six SNPs in the LDLR, LRPI, and LRPAP1 genes, and adjusted for several potential confounding variables, including smoking history, statin use, age, and gender. We also performed model selection based on the Akaike information criterion (AIC, stepAIC function in R) for total- and LDL-cholesterol, referred to as Model II in Table 2. Figure 1C shows that the residuals from fitted values for LDL in Model II from Eq. 3 have approximately constant variance and are normally distributed (Shapiro–Wilk test has p = 0.1).
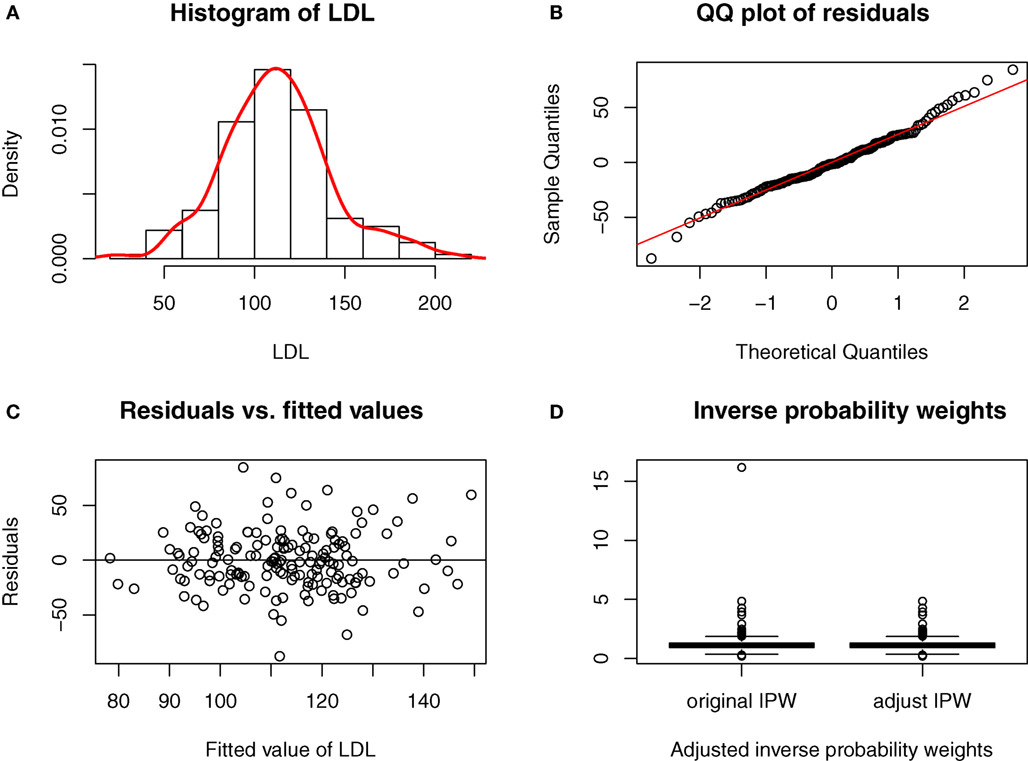
Figure 1. Model diagnostic for fitted model in the causal link of LDL-cholesterol to PD risk. (A) Histogram of LDL. (B) QQ plot of residuals. (C) Residuals vs. fitted values. (D) Inverse probability weights.
As expected, statin usage was associated significantly with lower total- and LDL-cholesterol levels (Table 2). Among the genes we investigated, before model selection only LRP1 demonstrated a statistically significant influence on total- and LDL-cholesterol levels (p = 0.04 for both in Model I, and p = 0.0027 and p = 0.024 separately in Model II). LDLR SNPs did not significantly impact cholesterol levels and were not selected in Model II after step-wise model selection. There was a trend for APOE polymorphisms to influence both total- and LDL-cholesterol (p = 0.08 and p = 0.08, respectively), and also a trend of LRPAP1 influencing LDL-cholesterol (p = 0.05).
The Causal Effect Analyses of Total- and LDL-Cholesterol Levels on PD Using the Generalized Propensity Score Approach
To assess the causal relationship between cholesterol and PD, a marginal structure model with generalized propensity score approach was adopted. This model can be employed for more general settings than structural equation modeling (SEM) (33), and more importantly, it involves fewer assumptions than SEM because it targets a particular set of effects of interest, and only makes assumptions needed for those effects (28, 34–37).
Figure 1D shows the boxplot of the IPW for LDL-cholesterol, and there is one extreme weight with a value greater than 10. This extreme value can affect the variance of causal estimates (38), and thus we shrank it to the 95th quantile as an adjustment for the IPW. Total-cholesterol had two extreme weights greater than 10, and we shrank these two values to the 95th quantile as well.
Both total- and LDL-cholesterol levels were significantly linked to PD occurrence through the generalized propensity score method. The estimates of effects are −0.032 (SE: 0.009) with p < 0.001 for total-cholesterol, and −0.016 (SE: 0.008) with p = 0.04 for LDL-cholesterol, respectively. The odds ratios are 0.97 [95% CI: 0.95, 0.99] and 0.98 [95% CI: 0.97, 0.99] for total- and LDL-cholesterol, respectively.
Discussion
Despite a growing literature implying that serum/plasma total- and LDL-cholesterol may be linked to PD (1–12), the cause of the association is not known. In the current study, we applied the generalized propensity score approach to investigate a potential causal relationship between circulating cholesterol levels and PD. SEM is an alternative tool for causal inference, but generalized propensity score with inverse probability of weights is a new class of causal model that can handle more challenges (e.g., time-dependent confounding) with fewer assumptions than SEM. Following the convention in the literature of causal inference (28), the method accounts for confounding effects through a linear combination of genetic, biological, behavioral, and environmental factors. Due to the small set of genes for consideration, there is no need to conduct a boosting algorithm for propensity score estimation in our case, and we assumed a normal distribution for the multiple linear regressions shown in Figure 1A.
We took into consideration age; gender; APOE polymorphisms; LDLR, LRP1, and LRPAP1 SNPs; smoking history; and statin usage in our initial model (Model I) because they are suspected to influence both circulating cholesterol levels and the occurrence of PD. After step-wise model selection, SNPs from LDLR were removed in Model II. The results of these analyses suggest that circulating total- and LDL-cholesterol levels may be linked to the factors influencing PD risk.
The Effect of Smoking, Statins, and Cholesterol-Related Genes on Cholesterol Levels
Our analyses, as expected, found that statins lower cholesterol and lower cholesterol (after controlling for statin use) could be a risk factor for PD, in agreement with the large majority of the literature (1–12). Diabetes may affect lipid levels, and statins have been suggested to be of use in diabetes (39), but because this is not clearly established, diabetes was not included in our analyses. The literature related to the role of smoking on cholesterol levels has been mixed, with some studies reporting an association, but others not (40, 41). In our current cohort, history of smoking did not influence cholesterol profiles significantly, although this may have been affected by our categorization of smokers as those who had smoked consistently for six months at any time in their life. The lack of an association of age and cholesterol levels probably resulted from the very narrow age range of the subjects in the study (i.e., PD is a disorder of later life and the controls were age-matched).
The most common allele in APOE, ε3, is present in ~77% of the population, whereas the least common ε2 is represented in only 8%. Individuals with an ε2 allele have been known to have a propensity for lower plasma LDL-cholesterol levels, whereas ε4 is linked to higher LDL-cholesterol levels (42). Although ε2 is linked to a number of beneficial outcomes in terms of cardiovascular disease and lower risk of AD, some studies (19, 20), but not all in others (43), have associated it with higher risk of PD. Conversely, the ε4 allele is associated with poorer cardiovascular disease outcomes and a significantly increased risk of AD (44, 45), but may be protective and/or associated with lower PD risk (21). Although there was a trend for APOE to influence cholesterol levels in the current study, the analysis did not reach statistical significance, probably due to the small sample size and the lack of ε4 alleles in this cohort. Since the ε4 allele occurs in ~14% of the general population (46), it is somewhat remarkable that no subject in the study had an ε4 allele. This may be due to the lower occurrence of ε4 in PD (21), and the fact that the Controls, by being “healthy” (i.e., no cardiovascular disease or AD), would tend not to be ε4 carriers.
A Potential Causal Cholesterol–PD Link
The current study suggests for the first time that circulating total- and LDL-cholesterol may be linked to the factors influencing PD risk, but it is not informative about the specific mechanism or factor(s) that mediate such a link. Statins have been suggested to be neuroprotective for PD (47–49). The current study, however, suggested that statins, by lowering cholesterol, actually may be linked to PD risk. Consistent with this latter hypothesis, a prospective study in the Atherosclerosis Risk in Community cohort found that statin usage was associated with increased future risk of PD (9). Most recently, an analysis of the large MarketScan national claims database found that statins facilitated PD diagnosis (50). Together, these data suggest caution to proposing statins as being neuroprotective for PD.
The brain is the most cholesterol-rich organ in the body (accounting for ~25% of the total amount). In mature brain, cholesterol is synthesized primarily by astrocytes and then transported to neurons via endocytosis and interaction with the LDL receptor and apolipoprotein E (51). There is very limited ability for circulating cholesterol to traverse the blood–brain barrier (BBB) (52). Thus, according to current thinking (53), brain cholesterol is made mainly de novo, and central nervous system (CNS) and plasma compartments generally do not communicate (54). There is, however, evidence for the uptake of LDL particles and other apolipoproteins across the BBB, possibly via the LDLR and/or LDLR-related proteins, and oxysterols also may mediate peripheral-central cholesterol communication (55).
Genetic defects in cholesterol metabolism (i.e., lipoprotein receptors, cholesterol biosynthesis, lipid transport and assembly, or signaling molecules) may lead to structural and functional CNS diseases (53), and many cholesterol metabolites are centrally active (56). Cholesterol synthesis in CNS can be regulated by the APOE-mediated uptake of lipoproteins via the LDLR family of proteins. After synthesized cholesterol is delivered to neurons, it is bound by cell surface lipoprotein receptors such as the LDLR receptor and the LDLR-related protein (LRP1) that recognize APOE prior to endocytosis.
It is also possible that LRP1 mediates a compensatory response to neural stress and elicits a parallel change in LDL particles and other apolipoproteins across the BBB via the LRP receptor. MPTP lesions increase both APOE and its receptors (LDLR and LRP) in brain (23). APOE and LRPAP1 SNPs are associated significantly with increased PD risk, and high levels of LDL-cholesterol appear to have a protective role against PD (22). Consistent with this notion, our results showed that the LDLR-related gene LRP1 had a significant influence on circulating total- and LDL-cholesterol levels. These lines of evidence may seem paradoxical, but can be reconciled. The synthesis of cholesterol, its oxidation products, and APOE are increased in response to neural cell injury, thus an increase in brain cholesterol and CSF levels of LRP1 could be compensatory responses to neural stress.
Limitations and Future Directions
There are 152 cholesterol-related genes and more than 3,000 SNPs covered in the NeuroX array based on plasma lipid studies in coronary artery disease (26). In the current study, we investigated only four genetic polymorphisms potentially linked to both cholesterol profiles and neurodegenerative processes (18). As such, further studies in larger samples are needed to investigate other potentially important cholesterol-related genes and SNPs that also may be involved in neurodegenerative processes. Although our study suggested a potential causal link between circulating cholesterol and PD, there are no clear mechanistic explanations for this finding. Future studies are needed to provide mechanistic understanding and shall include a revisit of the presumed segregation of peripheral and central cholesterol, especially in pathological states and/or aging.
Ethics Statement
This study was carried out in accordance with the recommendations of XH’s NIH PD Biomarkers Program at the Penn State site, with written informed consent from all subjects. All subjects gave written informed consent in accordance with the Declaration of Helsinki. The protocol was approved by the Penn State Hershey Institutional Review Board.
Author Contributions
LZ: organization, execution of the project; statistical design and execution; writing of the first draft; and review and critique of the manuscript. XW: statistical design and execution, and review and critique of the manuscript; MW: review of statistical design and review and critique of the manuscript. NS: organization of the project and review and critique of the manuscript. GD: organization of the project; review and critique of the statistical design; and critique of the manuscript. ML: organization of the project and extensive review and critique of the manuscript. TY: execution of the project; statistical design and execution; and review and critique of the manuscript. RM: review of statistical design and extensive review and critique of the manuscript. RL: conception, statistical design and execution, and review and critique of the manuscript; XH: conception, organization, execution, obtaining funding of the project; review of the statistical design; writing of the first draft; and review and critique of the manuscript.
Conflict of Interest Statement
The authors declare that the research was conducted in the absence of any commercial or financial relationships that could be construed as a potential conflict of interest.
The reviewer PV-C and handling editor declared their shared affiliation.
Acknowledgments
We express gratitude to all of the participants who volunteered for this study and study personnel who contributed to its success. This work was supported in part by the National Institute of Neurological Disease and Stroke (NS060722 and NS082151 to XH), NIDA (P50 DA039838, and P50 DA036107 to RL), NSF (DMS1512422 to RL), the Hershey Medical Center General Clinical Research Center (C06 RR016499, UL1 TR000127 to XH), the PA Department of Health Tobacco CURE Funds, and the Penn State University CTSI Big Grant RFA (UL TR000127 to LZ). All analyses, interpretations, and conclusions are those of the authors and not the research sponsors.
Abbreviations
APOE, apolipoprotein E gene; CNS, central nervous system; LDLR, low-density lipoprotein receptor gene; LDLR1, low-density lipoprotein receptor related protein 1 gene; LRPAP1, low-density lipoprotein-related protein-associated protein 1 gene; BBB, blood–brain barrier.
References
1. Huang X, Miller WC, Mailman RB, Woodard JL, Chen PC, Xiang D, et al. Cardiovascularly “desirable” cholesterol levels associated with Parkinson’s disease. Ann Neurol (2005) 58:S23. doi:10.1002/ana.11300
2. Scigliano G, Musicco M, Soliveri P, Piccolo I, Ronchetti G, Girotti F. Reduced risk factors for vascular disorders in Parkinson disease patients: a case-control study. Stroke (2006) 37:1184–8. doi:10.1161/01.STR.0000217384.03237.9c
3. Huang X, Chen H, Miller WC, Mailman RB, Woodard JL, Chen PC, et al. Lower low-density lipoprotein cholesterol levels are associated with Parkinson’s disease. Mov Disord (2007) 22:377–81. doi:10.1002/mds.21290
4. Miyake Y, Tanaka K, Fukushima W, Sasaki S, Kiyohara C, Tsuboi Y, et al. Case-control study of risk of Parkinson’s disease in relation to hypertension, hypercholesterolemia, and diabetes in Japan. J Neurol Sci (2010) 293:82–6. doi:10.1016/j.jns.2010.03.002
5. de Lau LM, Koudstaal PJ, Hofman A, Breteler MM. Serum cholesterol levels and the risk of Parkinson’s disease. Am J Epidemiol (2006) 164:998–1002. doi:10.1093/aje/kwj283
6. de Lau LM, Stricker BH, Breteler MM. Serum cholesterol, use of lipid-lowering drugs, and risk of Parkinson disease. Mov Disord (2007) 22:1985. doi:10.1002/mds.21582
7. Simon KC, Chen H, Schwarzschild M, Ascherio A. Hypertension, hypercholesterolemia, diabetes, and risk of Parkinson disease. Neurology (2007) 69:1688–95. doi:10.1212/01.wnl.0000271883.45010.8a
8. Huang X, Abbott RD, Petrovitch H, Mailman RB, Ross GW. Low LDL cholesterol and increased risk of Parkinson’s disease: prospective results from Honolulu-Asia Aging Study. Mov Disord (2008) 23:1013–8. doi:10.1002/mds.22013
9. Huang X, Alonso A, Guo X, Umbach DM, Lichtenstein ML, Ballantyne CM, et al. Statins, plasma cholesterol, and risk of Parkinson’s disease: a prospective study. Mov Disord (2015) 30:552–9. doi:10.1002/mds.26152
10. Huang X, Auinger P, Eberly S, Oakes D, Schwarzschild M, Ascherio A, et al. Serum cholesterol and the progression of Parkinson’s disease: results from DATATOP. PLoS One (2011) 6:e22854. doi:10.1371/journal.pone.0022854
11. Sterling NW, Lichtenstein M, Lee EY, Lewis MM, Evans A, Eslinger PJ, et al. Higher plasma LDL-cholesterol is associated with preserved executive and fine motor functions in Parkinson’s disease. Aging Dis (2016) 7:237–45. doi:10.14336/AD.2015.1030
12. Mahlknecht P, Sprenger F, Seppi K, Poewe W. Plasma fasting cholesterol profiles and age at onset in Parkinson’s disease. Mov Disord (2015) 30:1974–5. doi:10.1002/mds.26452
13. Rosenthal LS, Drake D, Alcalay RN, Babcock D, Bowman FD, Chen-Plotkin A, et al. The NINDS Parkinson’s disease biomarkers program. Mov Disord (2016) 31:915–23. doi:10.1002/mds.26438
14. Litvan I, Bhatia KP, Burn DJ, Goetz CG, Lang AE, Mckeith I, et al. Movement Disorders Society Scientific Issues Committee report: SIC Task Force appraisal of clinical diagnostic criteria for Parkinsonian disorders. Mov Disord (2003) 18:467–86. doi:10.1002/mds.10459
15. Lucassen EB, Sterling NW, Lee EY, Chen H, Lewis MM, Kong L, et al. History of smoking and olfaction in Parkinson’s disease. Mov Disord (2014) 29:1069–74. doi:10.1002/mds.25912
16. Nalls MA, Bras J, Hernandez DG, Keller MF, Majounie E, Renton AE, et al. NeuroX, a fast and efficient genotyping platform for investigation of neurodegenerative diseases. Neurobiol Aging (2015) 36:1605.e7–12. doi:10.1016/j.neurobiolaging.2014.07.028
17. Klemann C, Martens GJM, Sharma M, Martens MB, Isacson O, Gasser T, et al. Integrated molecular landscape of Parkinson’s disease. NPJ Parkinsons Dis (2017) 3:14. doi:10.1038/s41531-017-0015-3
18. Holtzman DM, Herz J, Bu G. Apolipoprotein E and apolipoprotein E receptors: normal biology and roles in Alzheimer disease. Cold Spring Harb Perspect Med (2012) 2:a006312. doi:10.1101/cshperspect.a006312
19. Huang X, Chen PC, Poole C. APOE-[epsilon]2 allele associated with higher prevalence of sporadic Parkinson disease. Neurology (2004) 62:2198–202. doi:10.1212/01.WNL.0000130159.28215.6A
20. Williams-Gray CH, Goris A, Saiki M, Foltynie T, Compston DA, Sawcer SJ, et al. Apolipoprotein E genotype as a risk factor for susceptibility to and dementia in Parkinson’s disease. J Neurol (2009) 256:493–8. doi:10.1007/s00415-009-0119-8
21. Gao J, Huang X, Park Y, Liu R, Hollenbeck A, Schatzkin A, et al. Apolipoprotein E genotypes and the risk of Parkinson disease. Neurobiol Aging (2011) 32:2106.e1–6. doi:10.1016/j.neurobiolaging.2011.05.016
22. Singh NK, Banerjee BD, Bala K, Mitrabasu, Dung Dung AA, Chhillar N. APOE and LRPAP1 gene polymorphism and risk of Parkinson’s disease. Neurol Sci (2014) 35:1075–81. doi:10.1007/s10072-014-1651-6
23. Domenger D, Dea D, Theroux L, Moquin L, Gratton A, Poirier J. The MPTP neurotoxic lesion model of Parkinson’s disease activates the apolipoprotein E cascade in the mouse brain. Exp Neurol (2012) 233:513–22. doi:10.1016/j.expneurol.2011.11.031
24. Wilhelmus MMM, Bol JG, Van Haastert ES, Rozemuller AJ, Bu G, Drukarch B, et al. Apolipoprotein E and LRP1 increase early in Parkinson’s disease pathogenesis. Am J Pathol (2011) 179:2152–6. doi:10.1016/j.ajpath.2011.07.021
25. Kathiresan S, Melander O, Guiducci C, Surti A, Burtt NP, Rieder MJ, et al. Six new loci associated with blood low-density lipoprotein cholesterol, high-density lipoprotein cholesterol or triglycerides in humans. Nat Genet (2008) 40:189–97. doi:10.1038/ng.75
26. Global Lipids Genetics Consortium, Willer CJ, Schmidt EM, Sengupta S, Peloso GM, Gustafsson S, et al. Discovery and refinement of loci associated with lipid levels. Nat Genet (2013) 45:1274–83. doi:10.1038/ng.2797
27. Imbers GW. The role of the propensity score in estimating dose-response functions. Biometrika (2000) 87:706–10. doi:10.1093/biomet/87.3.706
28. Zhu Y, Coffman DL, Ghosh D. A boosting algorithm for estimating generalized propensity scores with continuous treatments. J Causal Inference (2015) 3:25–40. doi:10.1515/jci-2014-0022
29. Rosenbaum PR, Rubin DB. The central role of the propensity score in observational studies for causal effects. Biometrika (1983) 70:41–55. doi:10.1093/biomet/70.1.41
30. Robins JM. Association, causation, and marginal structural model. Synthese (1999) 121:151–79. doi:10.1023/A:1005285815569
31. Robins JM, Hernan MA, Brumback B. Marginal structural models and causal inference in epidemiology. Epidemiology (2000) 11:550–60. doi:10.1097/00001648-200009000-00011
32. Ji L, Wang Y, Zhu D, Liu W, Shi J. White matter differences between multiple system atrophy (parkinsonian type) and Parkinson’s disease: a diffusion tensor image study. Neuroscience (2015) 305:109–16. doi:10.1016/j.neuroscience.2015.07.060
33. VanderWeele TJ. Invited commentary: structural equation models and epidemiologic analysis. Am J Epidemiol (2012) 176:608–12. doi:10.1093/aje/kws213
34. Bray BC, Almirall D, Zimmerman RS, Lynam D, Murphy SA. Assessing the total effect of time-varying predictors in prevention research. Prev Sci (2006) 7:1–17. doi:10.1007/s11121-005-0023-0
35. Drton M, Foygel R, Sullivant S. Global identifiability of linear structural equation models. Ann Stat (2011) 39:865–86. doi:10.1214/10-AOS859
36. VanderWeele TJ, Hawkley LC, Thisted RA, Cacioppo JT. A marginal structural model analysis for loneliness: implications for intervention trials and clinical practice. J Consult Clin Psychol (2011) 79:225–35. doi:10.1037/a0022610
37. VanderWeele TJ. Explanation in Causal Inference: Methods for Mediation and Interaction. New York: Oxford Press (2015).
38. Kang JDY, Schafer JL. Demystifying double robustness: a comparison of alternative strategies for estimating a population mean from incomplete data. Stat Sci (2007) 22:523–39. doi:10.1214/07-STS227REJ
39. Millan Nunez-Cortes J, Cases Amenos A, Ascaso Gimilio JF, Barrios Alonso V, Pascual Fuster V, Pedro-Botet Montoya JC, et al. Consensus on the statin of choice in patients with impaired glucose metabolism: results of the DIANA Study. Am J Cardiovasc Drugs (2017) 17:135–42. doi:10.1007/s40256-016-0197-9
40. Freeman JS, Cody FW, Schady W. The influence of external timing cues upon the rhythm of voluntary movements in Parkinson’s disease. J Neurol Neurosurg Psychiatry (1993) 56:1078–84. doi:10.1136/jnnp.56.10.1078
41. Gepner AD, Piper ME, Johnson HM, Fiore MC, Baker TB, Stein JH. Effects of smoking and smoking cessation on lipids and lipoproteins: outcomes from a randomized clinical trial. Am Heart J (2011) 161:145–51. doi:10.1016/j.ahj.2010.09.023
42. Davies NM, Windmeijer F, Martin RM, Abdollahi MR, Smith GD, Lawlor DA, et al. Use of genotype frequencies in medicated groups to investigate prescribing practice: APOE and statins as a proof of principle. Clin Chem (2011) 57:502–10. doi:10.1373/clinchem.2010.156356
43. Federoff M, Jimenez-Rolando B, Nalls MA, Singleton AB. A large study reveals no association between APOE and Parkinson’s disease. Neurobiol Dis (2012) 46:389–92. doi:10.1016/j.nbd.2012.02.002
44. Corder EH, Saunders AM, Strittmatter WJ, Schmechel DE, Gaskell PC, Small GW, et al. Gene dose of apolipoprotein E type 4 allele and the risk of Alzheimer’s disease in late onset families. Science (1993) 261:921–3. doi:10.1126/science.8346443
45. Liu CC, Kanekiyo T, Xu H, Bu G. Apolipoprotein E and Alzheimer disease: risk, mechanisms and therapy. Nat Rev Neurol (2013) 9:106–18. doi:10.1038/nrneurol.2012.263
46. van Duijn CM, De Knijff P, Cruts M, Wehnert A, Havekes LM, Hofman A, et al. Apolipoprotein E4 allele in a population-based study of early-onset Alzheimer’s disease. Nat Genet (1994) 7:74–8. doi:10.1038/ng0594-74
47. Tan EK, Tan LC. Holding on to statins in Parkinson disease. Neurology (2013) 81:406–7. doi:10.1212/WNL.0b013e31829d87bb
48. University of Plymouth. Could a Cholesterol-Lowering Drug be a Potential Treatment for Parkinson’s? Cholesterol-Lowering Drug Simvastatin Trialled as a Potential Neuroprotective Treatment for Parkinson’s. (2016). Available from: www.sciencedaily.com/releases/2016/01/160111092404.htm
49. Venosa A. Cholesterol medication simvastatin tested as potential treatment for Parkinson’s disease in new clinical trial. Medical Daily. (2016). Available from: http://www.medicaldaily.com/cholesterol-medication-simvastatin-tested-potential-treatment-parkinsons-disease-new-369008
50. Liu G, Sterling NW, Kong L, Lewis MM, Mailman RB, Chen H, et al. Statins may facilitate Parkinson’s disease: insight gained from a large, national claims database. Mov Disord (2017) 32(6):913–7. doi:10.1002/mds.27006
51. Mauch DH, Nagler K, Schumacher S, Goritz C, Muller EC, Otto A, et al. CNS synaptogenesis promoted by glia-derived cholesterol. Science (2001) 294:1354–7. doi:10.1126/science.294.5545.1354
52. Bjorkhem I, Meaney S. Brain cholesterol: long secret life behind a barrier. Arterioscler Thromb Vasc Biol (2004) 24:806–15. doi:10.1161/01.ATV.0000120374.59826.1b
53. Orth M, Bellosta S. Cholesterol: its regulation and role in central nervous system disorders. Cholesterol (2012) 2012:292598. doi:10.1155/2012/292598
54. Vance JE, Karten B, Hayashi H. Lipid dynamics in neurons. Biochem Soc Trans (2006) 34:399–403. doi:10.1042/BST0340399
55. Bjorkhem I. Crossing the barrier: oxysterols as cholesterol transporters and metabolic modulators in the brain. J Intern Med (2006) 260:493–508. doi:10.1111/j.1365-2796.2006.01725.x
Keywords: Parkinson’s disease, cholesterol, genetics, statins, propensity score
Citation: Zhang L, Wang X, Wang M, Sterling NW, Du G, Lewis MM, Yao T, Mailman RB, Li R and Huang X (2017) Circulating Cholesterol Levels May Link to the Factors Influencing Parkinson’s Risk. Front. Neurol. 8:501. doi: 10.3389/fneur.2017.00501
Received: 08 May 2017; Accepted: 07 September 2017;
Published: 27 September 2017
Edited by:
Oscar Arias-Carrión, Hospital General Dr. Manuel Gea Gonzalez, MexicoReviewed by:
Paola Vázquez-Cárdenas, Hospital General Dr. Manuel Gea Gonzalez, MexicoGraziella Madeo, National Institutes of Health (NIH), United States
Copyright: © 2017 Zhang, Wang, Wang, Sterling, Du, Lewis, Yao, Mailman, Li and Huang. This is an open-access article distributed under the terms of the Creative Commons Attribution License (CC BY). The use, distribution or reproduction in other forums is permitted, provided the original author(s) or licensor are credited and that the original publication in this journal is cited, in accordance with accepted academic practice. No use, distribution or reproduction is permitted which does not comply with these terms.
*Correspondence: Lijun Zhang, bHpoYW5nNkBwZW5uc3RhdGVoZWFsdGgucHN1LmVkdQ==;
Xuemei Huang, eHVlbWVpQHBzdS5lZHU=