- 1Department of Neurology, McGovern Medical School, University of Texas Health Science Center at Houston, Houston, TX, United States
- 2Department of Diagnostic and Interventional Radiology, McGovern Medical School, University of Texas Health Science Center at Houston, Houston, TX, United States
- 3Department of Psychiatry, McGovern Medical School, University of Texas Health Science Center at Houston, Houston, TX, United States
Background and objective: Cognitive impairment (CI) is common in multiple sclerosis (MS), but underlying mechanisms and their imaging correlates are not completely understood. The gray and white matter structures of the limbic system (LS) play crucial roles in different aspects of cognition. To investigate their role in MS related CI, and since a detailed evaluations are lacking in the literature, we used a comprehensive neuroimaging approach to evaluate CI’s correlations with the main components of the LS.
Methods: Ten non-cognitively impaired MS patients and 30 MS patients with diagnosed CI, who underwent a comprehensive neuropsychological evaluation were included in the analysis. Microstructural integrity, volumetry of main limbic gray and white matter structures and cortical thickness were assessed for associations with CI.
Results: Fornix and cingulum/cingulate cortices were found to be the strongest correlates of CI in MS. As expected, LS’ gray and white matter structures were involved in various cognitive functions. Uncinate fasciculi showed significant correlation with verbal and visuospatial learning and memory, phonemic and semantic fluency; hippocampi with visuospatial skills, phonemic and semantic fluency, executive functions, and processing speed; thalami with verbal learning, visuospatial skills, semantic fluency; and amygdala with verbal recognition discrimination.
Conclusion: This comprehensive neuroimaging approach elucidated the role of the main limbic structures in cognitive functions associated with MS-related CI.
Introduction
Cognitive impairment (CI) occurs in 40–65% of patients with multiple sclerosis (MS) (1), mainly encompassing disturbances in memory, attention, verbal fluency (VF), information processing speed, conceptual reasoning, and visuospatial perception (2). Damage to white matter structures from demyelination or axonal loss leading to disconnection between the cortical and subcortical regions responsible for cognition, underlies the cognitive symptomatology in MS (3, 4). Although MS was originally thought to be mainly a white matter disease, gray matter structures are also known to be involved in the disease pathogenesis and shown to correlate with impairment (5, 6).
The limbic system (LS) includes gray matter and white matter structures that along with paralimbic structures, play a crucial role in different aspects of cognition (7). Hippocampus, thalamus, and the ventral cingulum connected by the fornix, are responsible for memory and spatial orientation, whereas the amygdala, orbitofrontal cortex (OFC), entorhinal cortex (EC), and parahippocampal (PH) cortex connected by uncinate fasciculus, are involved in multimodal sensory integration, behavioral inhibition, the reward-pleasure system, and memory for visual information (8). On the other hand, the cingulate gyrus and cingulum are associated with attention, response selection/action monitoring, self-knowledge, and reasoning (9) (see Figure 1).
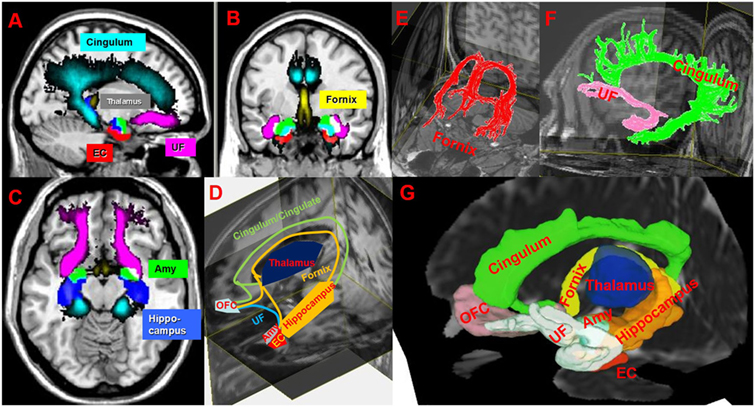
Figure 1. (A–C) Illustration of spatial-normalized MNI summary of cingulum, fornix, uncinated fasciculus (UF), thalamus, entorhinal cortex (EC), amygdala (Amy) in sagittal, coronal and axial T1w slices in our multiple sclerosis (MS) cohort. (D,G) Schematic (D) and atlas-based (G) representation (in one MS patient) of limbic connections between limbic gray matters on 3D T1w and mean diffusivity images. (E,F) Illustrates cingulum (green), UF (pink), and fornix (red) on T1w. Abbreviation: OFC, orbitofrontal cortex. MRIcron (http://people.cas.sc.edu/rorden/mricron/index.html), Diffusion tensor imaging (http://cmrm.med.jhmi.edu/), and DSI (http://dsi-studio.labsolver.org/) studios are used to generate this figure.
The LS recently received more attention in the pursuit of understanding the neural correlates of abnormal cognitive symptomatology in MS (4, 10). The LS’s white (11, 12) and gray matter structures (13, 14) are found to be implicated in various cognitive symptoms in MS. Of note, compared with healthy controls, reduced cerebral blood flow was present in limbic regions of patients with CI (15). Although most studies focus on individual parts of LS, a comprehensive evaluation that included all components of the LS is lacking (see Table 1 for a summary of key MRI studies investigating limbic structures in MS).
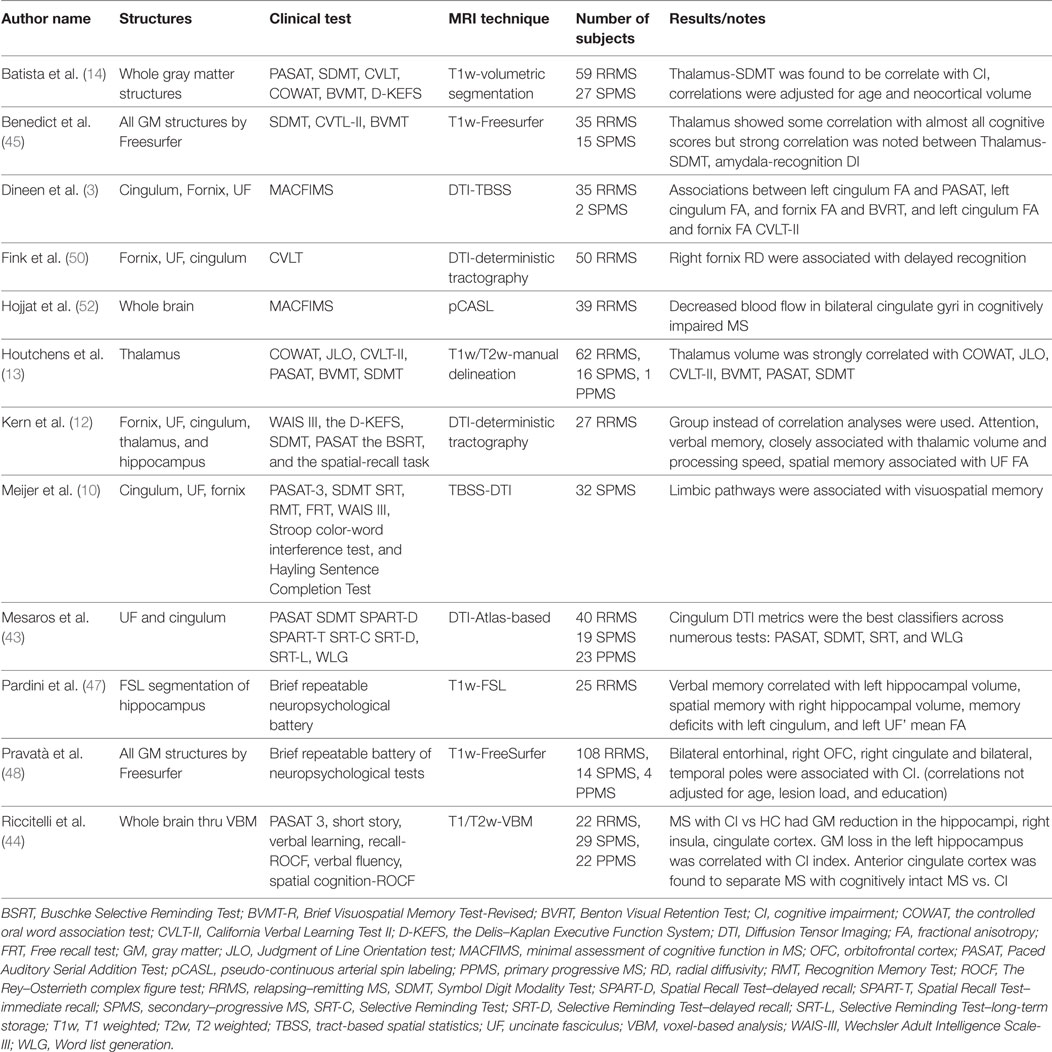
Table 1. Tabulated summary of key MRI studies investigating limbic structures in multiple sclerosis (MS).
Several studies have shown correlation between various MRI measure and histopathology (16, 17, 18). Diffusion tensor imaging (DTI) metrics of white matter tracts were found to correlate with a histologically derived measure of tract myelination (17, 18). It is suggested that fractional anisotropy (FA) and mean diffusivity (MD) of white matter tracts are affected by axonal count in post mortem analyses in MS (18). Additionally, cortical thickness measures derived from MRI and histological measurements showed significant correlations in MS (17). While no specific postmortem DTI studies have been detailed for limbic structure damage in MS, cortical thickness (19) and diffusion measurements are a reliable in vivo technique to quantify gray and white matter injury in MS (18).
In this study, we attempted to provide a comprehensive analysis of the roles of the LS’s gray and white matter structures on the main cognitive functions affected by MS. We used quantitative MRI (qMRI) methods such as cortical thickness and volumetric analysis of deep and cortical gray matter structures derived from T1-weighted (T1w) images. In addition, we used microstructural measures such as FA and MD derived from DTI. Cognitive scores were obtained from subsets of the Minimal Assessment of Cognitive Function in MS (MACFIMS), a comprehensive cognitive battery assessing various cognitive domains specifically related to MS (20).
Materials and Methods
Ten non-cognitively impaired MS patients (MSNI) and 30 patients with diagnosed CI (MSCI) were included (36 relapsing–remitting, and 4 secondary-progressive) age 40.53 ± 11.53 (range 18–58) years, education 14.65 ± 2.33 years, disease duration 13.71 ± 8.82 years, Expanded disability status scale 3.21 ± 1.89 (0–7), left handedness 3/40 (Table 2). Written informed consent was obtained from each subject following University of Texas Health Science Center Institutional Review Board approval of the protocol. All patients underwent the comprehensive cognitive testing as detailed below. Cognitive testing was performed in the morning to avoid fatigue, prior to and within 2 weeks of the imaging session. Inclusion criteria specified meeting 2010 McDonald Criteria for MS. Exclusion criteria included history of psychiatric disorders, recent history of drug or alcohol abuse, history of depression or relapse within 3 months of enrollment, history of allergy to gadolinium, history of other brain pathology, claustrophobia, or positive urine pregnancy test prior to MRI.
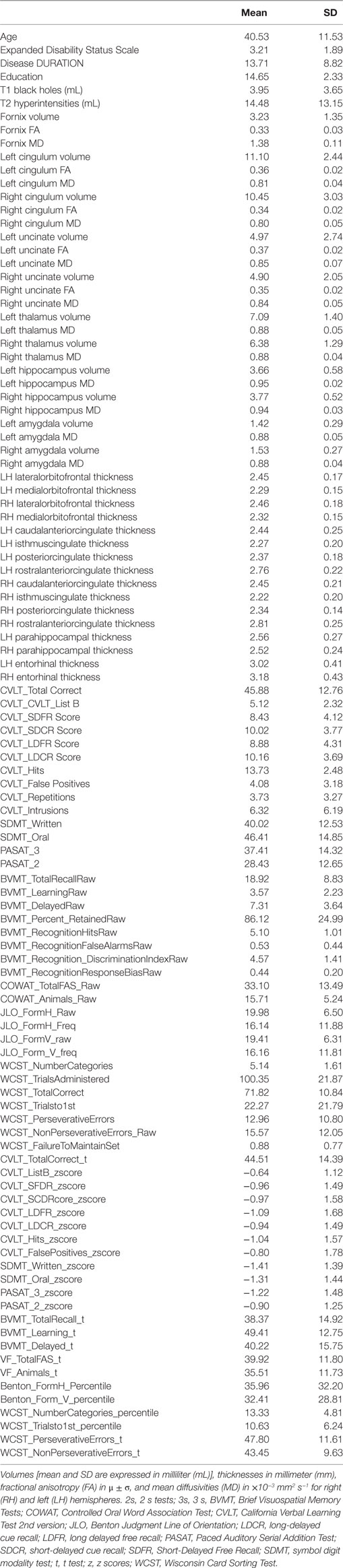
Table 2. Subject characteristics, MRI derived values of the brain structures, and cognitive scores investigated in this study.
Behavioral Laboratory Measures
In order to determine CI in our cohort, an impairment index methodology was applied to the behavioral scores. Based on previously validated methodology (20), 20 MACFIMS parameters were identified as most pertinent in the measurement of MS-related cognitive deficits and a CI index was derived. The processing speed and working memory was evaluated by using Paced Auditory Serial Addition Test (PASAT) (21) and symbol digit modality test (SDMT) (22), memory and learning evaluated by using California Verbal Learning Test Second Edition (CVLT-II) (23, 24) and Brief Visuospatial Memory Test-Revised (25), executive function using Wisconsin Card Sorting (26), visual perception/spatial processing using Judgment of Line Orientation test (JLO) (27), and VF measured by the controlled oral word association test (COWAT) (27). Patients were classified as cognitive impaired if their performance was more than one standard deviation below the mean on at least 40% of the pre-identified parameters and classified as cognitively intact if performance was less than 40% impaired.
Magnetic Resonance Imaging Data Acquisition
Whole brain MRI data were acquired on a Philips 3.0T Intera scanner using a SENSE receive head coil. The MRI protocol included conventional and non-conventional MRI sequences [dual echo turbo spin echo, fluid attenuation by inversion recovery (FLAIR) and 3D T1-weighted magnetization prepared rapid acquisition with gradient echo (MPRAGE)]. The T1-weighted sequence spatial resolution was 1 mm × 1 mm × 1 mm and field-of-view was 256 mm × 256 mm. Diffusion-weighted image (DWI) data were acquired axially from the same graphically prescribed conventional MRI volumes using a single-shot multi-slice 2-D spin-echo diffusion sensitized and fat-suppressed echo planar imaging (EPI) sequence, with the balanced Icosa21 tensor encoding scheme (28, 29). The b-factor = 1,000 s mm−2, TR/TE = 7,100/65 ms, FOV = 256 mm × 256 mm, and slice thickness/gap/#slices = 3 mm/0 mm/44. The EPI phase encoding used a SENSE k-space undersampling factor of two, with an effective k-space matrix of 128 × 128, and an image matrix after zero-filling of 256 × 256. The constructed image spatial resolution for the DWI data was = 1 mm × 1 mm × 3 mm.
Lesion Load (LL) Segmentation using Conventional MRI
Whole brain LL was quantified in all patients using the co-registered multispectral dual FSE and the FLAIR volumes. The lesion probability masks were computed in MRIcron (http://www.nitrc.org/projects/mricron/) (30, 31). The lesion volumes were saved as binary masks to enable fusion with other multimodal volumes acquired from the same subject. We obtained both T1 and T2 LL to adjust for in the correlation analyses.
Diffusion Tensor White Matter Tractography
We used a brute force and multiple regions-of-interest (ROI) tracking method and the fiber assignment with continuous tractography (FACT) algorithm (32, 33) (DTI Studio, Johns Hopkins University, Baltimore, MD, USA) to reconstruct fornix, cingulum, and uncinated fasciculus with a FA threshold of 0.15 and an angle threshold of 70°. Reproducibility of the fiber construction in both hemispheres was tested on all subjects by two experienced raters (Zafer Keser and Khader M. Hasan). We used color-coded principal eigenvector red–green–blue (RGB) map derived from DTI to seed ROIs. Multiple ROI-based deterministic tractography was used for fornix, cingulum, and uncinate fasciculus as described in Figure 2 (4, 34–37) (Figure 2).
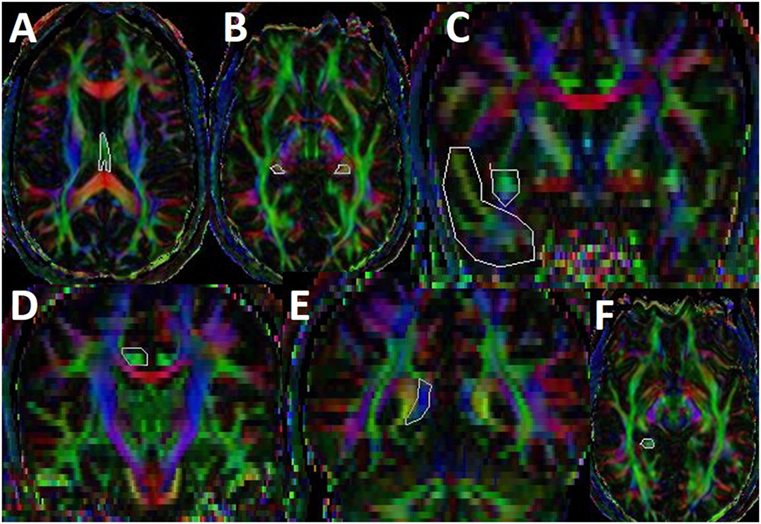
Figure 2. Illustration of fiber tractography in color-coded diffusion tensor imaging map. For fornix, we have seeded first ROI in subcallosal green fibers seen in axial slice where body and crus of fornix can be observed (A) and second and third ROIs are seeded in the mid and distal parts of crus (B) (35). For uncinate fasciculus (UF), we have seeded first ROI in the coronal section of temporal lobe and second ROI in the superomedial green projections fibers at the anterior commissure level (C) (34). For cingulum, we have seeded first ROI and second ROI in supracallosal green projection fibers anteriorly (D) and posteriorly (E). Third ROI was seeded in the green hippocampal cingulum fibers seen in the medial temporal lobe (F) (4). Once a fiber tract was reconstructed, its entire trajectory was verified on a slice-by-slice basis to compare with established anatomical landmarks described in the human brain neuroanatomy atlases (33).
Tissue Segmentation and Parcelation Using T1-Weighted and DTI Data
Using FreeSurfer software library (version 5.3) (38), the T1-weighted brain data were automatically segmented into cerebellar, brainstem, brain, and cerebrospinal fluid, which also included thalamus, amygdale, and hippocampus (39). DTI-derived data volumes (FA, mean, axial, and radial diffusivities) were coregistered to the T1-weighted volume to obtain diffusivity values for gray matter limbic structures. In brief, all the T1-weighted data were visually inspected to rule out artifacts and input to FreeSurfer’s “recon-all” routine for segmentation and extraction of morphometric measurements. FreeSurfer provided average cortical thickness using the cortical atlas labels described elsewhere (40).
Statistical Analyses
We used raw scores for cognitive scores. We tested normality using the Kolmogorov–Smirnov test for all reported measures. As our data couldn’t assure normal distribution for all the variables likely due to small sample size, we used non-parametric Spearman correlation analyses. For the whole cohort (n = 40), individual behavioral scores from each component of the MACFIMS were adjusted for age, years of education, and total brain lesion volume (T1 hypointensities + T2 hyper intensities without double counting), but not for handedness. They were then, computed with all qMRI measures using the partial Spearman rank correlations. We used normalized volumetric measures scaled for each subject by the individual estimated intracranial volume. All analyses and generation of scatter plots were performed in SPSS Statistics 24.0-IBM software. Significance defined if p < 0.05. We used Bonferroni corrections for multiple comparisons. After multiple comparisons, significance defined as p < 0.00003.
Results
Figures 3 and 4 highlight scattered plots with the best fit line curves for the some of the most notable correlations. Table 3 shows all the significant correlations with r and p-values. Most of the significant correlations did not survive multiple comparisons except the correlations of fornix FA with long delayed free recall in CVLT-II, SDMT oral test, and COWAT animals; left cingulum FA-PASAT 2 s, left hemisphere rostral anterior cingulate-form H in JLO and left hippocampus MD-form H in JLO.
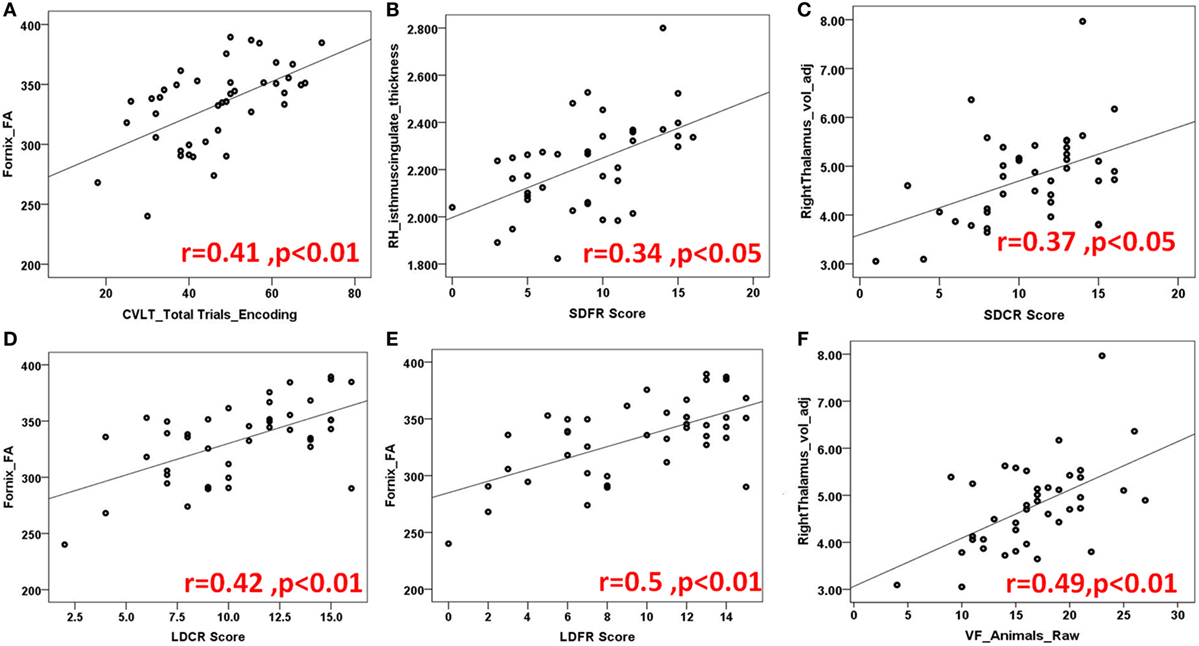
Figure 3. Demonstration of several scatter plots of limbic structures and California verbal learning (CVLT) (A–E) and controlled word association test [semantic verbal fluency (VF)] (F). Abbreviations: FA, fractional anisotropy; RH, right hemisphere; SDFR, Short-Delayed Free Recall; SDCR, short-delayed cue recall; LDCR, Long-delayed cue recall; LDFR, Long Delayed Free Recall; voladj, volume adjusted for intracranial volume.
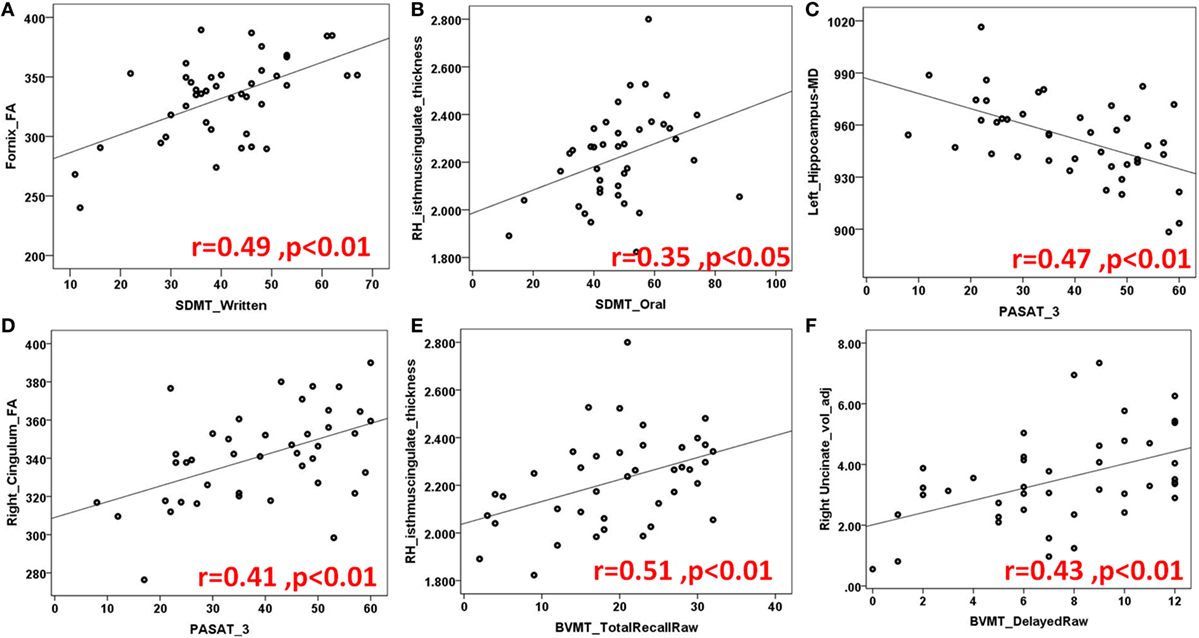
Figure 4. Another demonstration of several scatter plots of limbic structures and Symbol Digit Modality Test (SDMT) (A,B) written and oral versions, Paced auditory serial addition test (PASAT) (C,D), Brief visuospatial memory test (BVMT) total (E) and delayed recall (F) sets. Abbreviations: FA, fractional anisotropy; MD, mean diffusivity; RH, right hemisphere; voladj, volume adjusted for intracranial volume.
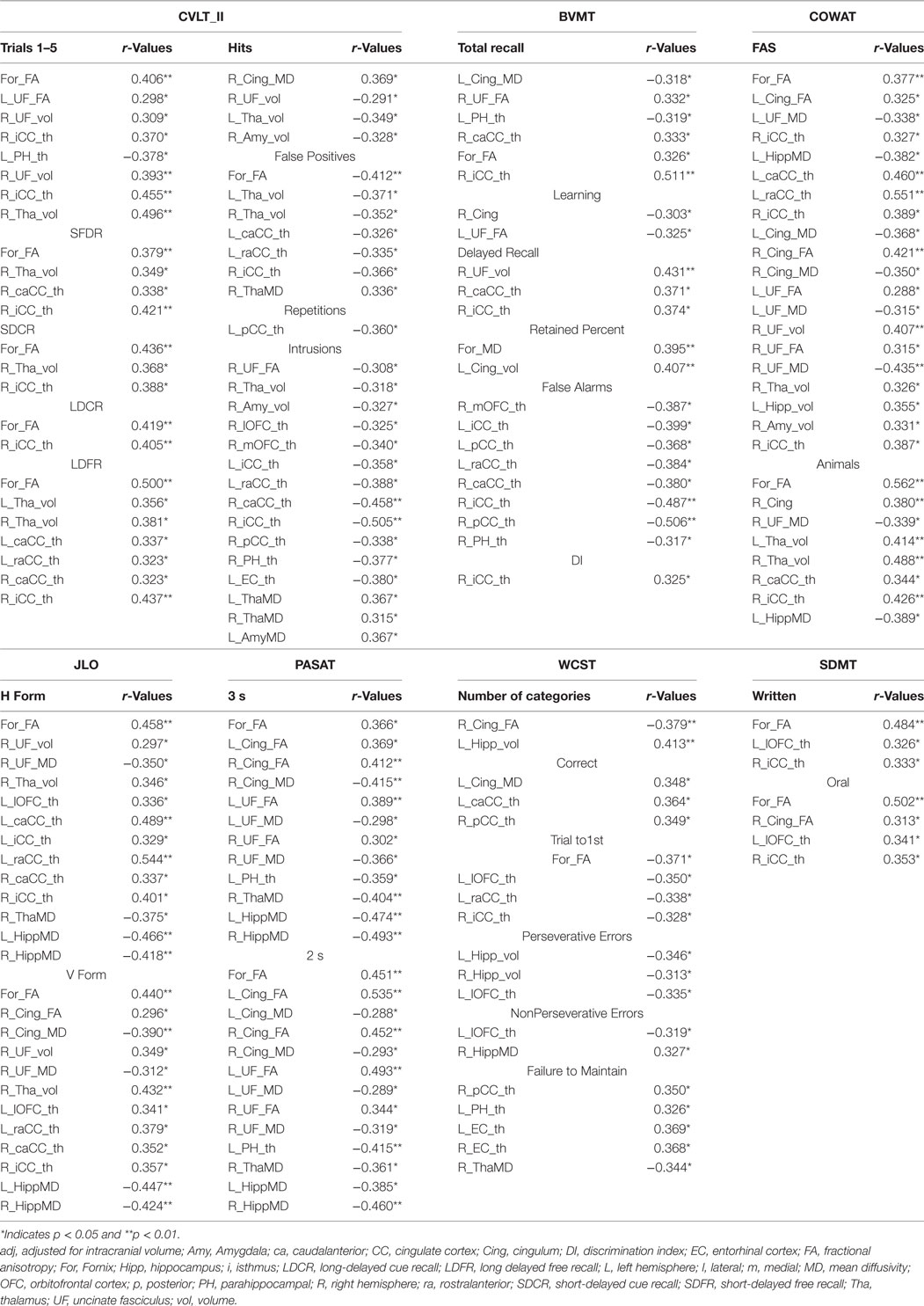
Table 3. Significant correlations are shown for California Verbal Learning Test 2nd version (CVLT_II), Brief Visuospatial Memory Tests (BVMT), symbol digit modality test (SDMT) and Paced Auditory Serial Addition Test (PASAT) 3 seconds (3 s) and 2 second (2 s) tests, Controlled Oral Word Association Test (COWAT), Benton Judgment Line of Orientation (JLO), Wisconsin Card Sorting Test (WCST).
California Verbal Learning Test II (CVLT II)
California Verbal Learning Test II evaluates episodic verbal learning and memory (24). In our study, the fornix, bilateral uncinated fasciculus (UF) bilateral thalami, left parahippocampus, and right posterior cingulate cortex were found to be critical regions for encoding function. For short term recall, our analyses showed significant correlations for right anterior and posterior cingulate cortices and thalamus whereas for long-term recall; bilateral thalami, and bilateral anterior and posterior cingulate cortices. Fornix was found to be crucial for both short and long term recall.
Recall discriminability measures, which we recently included in CVLT version II for better recall accuracy, were shown to be related with most of the limbic gray and white matter structures bilaterally; frontal limbic cortices, bilateral anterior and posterior cingulate cortices, bilateral thalami, fornix and right uncinate and cingulum tracts. Please see Table 3 for the numeric results and Figures 3A–E for the prominent scatter plots.
The Brief Visuospatial Memory Test—Revised
Brief Visuospatial Memory Test—Revised has been commonly used to evaluate visuospatial memory abilities in neuropsychological populations (25). In our MS population, visuospatial recall is found to be related to fornix, right UF, right anterior, and posterior cingulate cortices whereas learning to right cingulum, left UF, left EC. Delayed recall scores showed correlations with right UF, right anterior, and posterior cingulate cortices.
Although verbal recall discrimination is found to be carried out by more structures in LS, visuospatial recall discrimination is found to be related to bilateral anterior and posterior cingulate cortices bilaterally and right PH cortex. Table 3 and Figures 4E,F illustrates numeric results and notable scatter plots, respectively.
Controlled Oral Word Association Test
Controlled oral word association test evaluates phonemic fluency with FAS and semantic fluency with animal’s test (27). Previously (41), phonemic and semantic fluency are related to temporal regions. In our study, main limbic pathways connecting frontal and temporal lobes are shown to correlate with verbal phonemic and semantic fluency as well as right anterior and posterior cingulate cortices. Interestingly, bilateral thalami showed more association to semantic VF than phonemic VF (Table 3; Figure 3F).
Symbol Digit Modality Test
Symbol Digit Modality Test is a useful screening test for CI in MS and assesses attention, visual scanning, and motor speed (22), in our cohort; fornix, right cingulum, left OFC, and right posterior cingulate cortex, we found to be associated with the SDMT score (Table 3; Figures 4A,B).
Paced Auditory Serial Addition Test
Paced Auditory Serial Addition Test measures auditory information processing speed and flexibility, as well as calculation ability (21). All the main limbic white matter pathways, bilateral hippocampi, left PH cortex, and right thalamus were found to be associated with PASAT (Table 3; Figures 4C,D).
Benton Judgment of Line Orientation (JLO)
Judgment of Line Orientation is a standardized test of visuospatial skills (27) commonly associated with functioning right parietal and occipital activation, as well as bilateral frontal activation (42). In our MS population, although it was found to be lateralized to right cingulum and UF, and to left OFC, overall it showed bilateral associations to gray and white matter limbic structures. Fornix, bilateral cingulate cortices, bilateral hippocampi, and thalami were the most prominent structures related to the scores (Table 3).
Wisconsin Card Sorting Test
Wisconsin Card Sorting Test scores test attention, working memory, and visual processing. These scores measure frontal lobe functions; strategic planning, organized searching, utilizing environmental feedback to shift cognitive sets, directing behavior toward achieving a goal, and modulating impulsive responding (26). As expected, OFC showed correlation but lateralized to left. Temporal lobe structures were also found to be involved; bilateral hippocampi and entorrhinal cortices as well as left PH cortex. Bilateral cinguli and fornix as well as right thalamus showed significant correlations with the functions tested (Table 3).
Fornix
Our data indicate forniceal white matter to be essential for multimodal cognitive functions. It might be due to its central location connecting important limbic centers.
Cinguli/Cingulate Cortices (CC)
Left cingulum was found to be associated with visual spatial memory, phonemic fluency, executive functions, and processing speed whereas right cingulum similar to fornix was associated with broader cognitive functions such as executive functions, attention motor and processing speed, phonemic and semantic fluency, visuospatial learning and skills, and verbal learning. When it comes to parts of cingulate cortices, the isthmus of right CC showed correlation with all cognitive modalities; right anterior caudal CC with visuospatial memory and skills, verbal learning, semantic fluency; isthmus of left CC with visuospatial skills and verbal memory, and left anterior caudal CC with executive functions, visuospatial skills, and verbal learning. Posterior CC was associated with verbal learning bilaterally and executive functions on the right side.
Uncinate Fasciculus
Left UF showed significant correlation with verbal and visuospatial learning, phonemic fluency, and processing speed whereas right UF with visuospatial memory and skills, verbal learning, phonemic and semantic fluency, and processing speed.
Orbitofrontal Cortex
Left lateral OFC correlated with written and oral attention, executive functions, whereas right lateral OFC with verbal learning and right medial OFC with visuospatial learning and memory.
Hippocampus/Parahippocampus
Right hippocampus was microstructurally related to visuospatial skills, executive functions and processing speed, left hippocampus related to phonemic and semantic fluency, executive functions and processing speed. Bilateral parahipppocampi related to visual and verbal learning, and left parahippocampus with processing speed and executive functions.
Thalamus
Left thalamus was found to be correlated with verbal learning, visuospatial skills, semantic fluency whereas right thalamus with verbal learning, visuospatial skills, processing speed and executive functions, phonemic and semantic fluency.
Amygdala
Bilateral amygdala were associated with verbal recognition discrimination.
Entorhinal Cortex
Bilateral entorhinal cortices were correlated with executive functions and left entorhinal with verbal recognition discrimination.
Discussion
This study provided associations between LS structures including volumes and microstructure measures with various cognitive functions, both evaluated in a comprehensive manner for the purpose of a detailed cognitive mapping of limbic regions in MS patients with and without CI. Our findings are mostly in line with previous less comprehensive MS studies as can be seen in Table 1. The novelty of this study is the adoption of a comprehensive approach to the role of all major limbic structures in different cognitive functions rather than studying one or some limbic structures and their cognitive functions. Results show that many limbic gray and white matter structures are involved in various cognitive functions rather than one specific center being responsible of a specific function. We adjusted our correlations for age, LL and education, which play independent roles in cognitive functioning of patients. Overall, fornix and cingulum/cingulate cortices were found to be the strongest correlates of CI in MS.
Highlights of our findings are that cingulate cortex and its main white matter pathway the cingulum, which have multiple complex functions that make its exact behavioral significance very elusive but are known to mainly serve as a modulator among different cognitive networks (7). In our cohort, similar to the literature, the cingulate cortex especially its anterior part, showed correlation with multi cognitive modalities evaluated by the MACFIMS. Interestingly, although posterior cingulate is known to be involved with visuospatial awareness and memory, our MS cohort showed association to verbal learning and executive functions. Like fornix, cingulum was associated with broader cognitive functions. A previous study revealed that cingulum DTI metrics were the best classifiers across numerous cognitive tests measuring CI in MS [(43); see also Table 1].
Although classically hippocampal formation is responsible for semantic memory formation and spatial mapping, it also plays a critical role in modulating attention, reactivity to external cues, behavioral flexibility. Previously, gray matter loss in hippocampus was shown to be related with overall CI in MS (44, 45). Our analyses showed that injury to hippocampal formation led to more cognitive slowing more than verbal and visual learning. Also other studies have shown hippocampal associations with verbal memory on the left (46, 47), and spatial memory on the right in MS (47). We have not observed any lateralization in our cohort except for verbal recognition discrimination lateralized to the left EC also shown in a previous study (48). Our findings for lateralization should be interpreted cautiously as we had several left handed (3) subjects in our cohort.
In contrast to limited functions of hippocampus in our MS cohort, its main output the fornix, was a predictor of multimodal CI. It is not surprising that injury to fornix leads to such widespread effect on cognition as it is one of the more prominent pathways in the CNS and serves as bridge between most limbic structures (7). Previous MS studies support our findings showing fornix to be related to various cognitive functions (4) such as visuospatial memory (10, 49), verbal memory (11, 49), and delayed recognition (50).
Thalamus showed widespread associations with multimodal CI in our cohort similar to previous studies (13, 14). Some other studies in MS patients showed association between thalamus and attention/executive functions (12, 14, 45) verbal memory (12).
Lesions affecting amygdala (i.e., Kluver–Bucy syndrome) are known to cause loss of insight and inability of recognizing one’s self socially inappropriate behaviors (51). In parallel, in our study, degeneration in bilateral amygdala were associated with failure verbal recognition discrimination. OFC is classically known as top–down execution modulator of the limbic networks (8). It was previously shown to be related with overall CI in MS (48). In our cohort, language-related attention and executive functions mainly lateralized to left whereas visuospatial learning and memory lateralized to right. This can be explained by the fact that the right hemisphere is more involved with visuospatial awareness, whereas the left with language related functions. As the main white matter pathway connecting OFC to LS, the correlation profile of uncinate fasciculus and CI was similar to OFC without lateralization though. Our findings are somewhat in parallel with previous studies showing the role of UF in visuospatial memory (10), processing speed (11) in MS patients.
Conclusion
A more detailed cognitive mapping of the LS is important in understanding functional neuroanatomy of common cognitive domains affected by MS. It also provides a non-invasive, in vivo marker for possibly assessing cognitive recovery and designing more targeted treatment approaches for CI. To the best of our knowledge, our study is the first to have comprehensive cognitive mapping of the LS in an MS cohort, previous studies have limited correlations between specific structures and cognitive domains. Our cross-sectional study is limited by a relatively small sample size, lack of healthy subjects, and heterogeneity of the MS groups. Also most of the significant correlations in our study did not survive multiple comparisons possibly due to small sample size; however, false positivity cannot be ruled out. Longitudinal prospective studies with MS patients enrolled soon after diagnosis and monitored by MRI and cognitive testing for progression would more clearly reveal the role of LS in the MS related CI. I Larger clinical studies with adequate sample size will provide further exploration in the role of LS and other brain structures in MS, such studies are in the planning phase.
Ethics Statement
Written informed consent was obtained from each subject following University of Texas Health Science Center Institutional Review Board approval of the protocol.
Author Contributions
ZK—writing and preparation of the manuscript and MRI and statistical analyses; KH and BM—MRI and statistical analyses and critical revision of the manuscript for important intellectual content; KY, MK-K, AK, JL, FN—critical revision of the manuscript for important intellectual content.
Conflict of Interest Statement
The authors declare that the research was conducted in the absence of any commercial or financial relationships that could be construed as a potential conflict of interest.
Acknowledgments
We wish to thank Vipul Kumar Patel for helping in data acquisition.
Funding
This study was funded by a K-23 (the project # K23 NS072134) training award to FN. DUNN research foundation to KH.
References
1. Jongen PJ, Ter Horst AT, Brands AM. Cognitive impairment in multiple sclerosis. Minerva Med (2012) 103(2):73–96.
2. Ron MA, Callanan MM, Warrington EK. Cognitive abnormalities in multiple sclerosis: a psychometric and MRI study. Psychol Med (1991) 21:59–68. doi:10.1017/S0033291700014653
3. Dineen RA, Vilisaar J, Hlinka J, Bradshaw CM, Morgan PS, Constantinescu CS, et al. Disconnection as a mechanism for cognitive dysfunction in multiple sclerosis. Brain (2009) 132(Pt 1):239–49. doi:10.1093/brain/awn275
4. Keser Z, Hasan KM, Mwangi B, Gabr RE, Steinberg JL, Wilken J, et al. Limbic pathway correlates of cognitive impairment in multiple sclerosis. J Neuroimaging (2017) 27(1):37–42. doi:10.1111/jon.12381
5. Geisseler O, Pflugshaupt T, Buchmann A, Bezzola L, Reuter K, Schuknecht B, et al. Random number generation deficits in patients with multiple sclerosis: characteristics and neural correlates. Cortex (2016) 82:237–43. doi:10.1016/j.cortex.2016.05.007
6. Nelson F, Datta S, Garcia N, Rozario NL, Perez F, Cutter G, et al. Intracortical lesions by 3T magnetic resonance imaging and correlation with cognitive impairment in multiple sclerosis. Mult Scler (2011) 17:1122–9. doi:10.1177/1352458511405561
7. Mendoza J, Foundas A. Clinical Neuroanatomy: A Neurobehavioral Approach. New Orleans, LA: Springer (2007). p. 213–71.
8. Blumenfeld H. Neuroanatomy through Clinical Cases. Sunderland, MA: Sinauer Associates (2010). p. 132–7.
9. Catani M, de Schotten T. Atlas of Human Brain Connections. 1st ed. New York: Oxford University Press (2012). p. 49–76.
10. Meijer KA, Cercignani M, Muhlert N, Sethi V, Chard D, Geurts JJ, et al. Patterns of white matter damage are non-random and associated with cognitive function in secondary progressive multiple sclerosis. Neuroimage Clin (2016) 12:123–31. doi:10.1016/j.nicl.2016.06.009
11. Kern KC, Ekstrom AD, Suthana NA, Giesser BS, Montag M, Arshanapalli A, et al. Fornix damage limits verbal memory functional compensation in multiple sclerosis. Neuroimage (2012) 59(3):2932–40. doi:10.1016/j.neuroimage.2011.09.071
12. Kern KC, Gold SM, Lee B, Montag M, Horsfall J, O’Connor MF, et al. Thalamic-hippocampal-prefrontal disruption in relapsing-remitting multiple sclerosis. Neuroimage Clin (2014) 8:440–7. doi:10.1016/j.nicl.2014.12.015
13. Houtchens MK, Benedict RH, Killiany R, Sharma J, Jaisani Z, Singh B, et al. Thalamic atrophy and cognition in multiple sclerosis. Neurology (2007) 69(12):1213–23. doi:10.1212/01.wnl.0000276992.17011.b5
14. Batista S, Zivadinov R, Hoogs M, Bergsland N, Heininen-Brown M, Dwyer MG, et al. Basal ganglia, thalamus and neocortical atrophy predicting slowed cognitive processing in multiple sclerosis. J Neurol (2012) 259(1):139–46. doi:10.1007/s00415-011-6147-1
15. Vitorino R, Hojjat SP, Cantrell CG, Feinstein A, Zhang L, Lee L, et al. Regional frontal perfusion deficits in relapsing-remitting multiple sclerosis with cognitive decline. AJNR Am J Neuroradiol (2016) 37(10):1800–7. doi:10.3174/ajnr.A4824
16. Mori S, Kageyama Y, Hou Z, Aggarwal M, Patel J, Brown T, et al. Elucidation of white matter tracts of the human amygdala by detailed comparison between high-resolution postmortem magnetic resonance imaging and histology. Front Neuroanat (2017) 11:16. doi:10.3389/fnana.2017.00016
17. Kolasinski J, Stagg CJ, Chance SA, Deluca GC, Esiri MM, Chang EH, et al. A combined post-mortem magnetic resonance imaging and quantitative histological study of multiple sclerosis pathology. Brain (2012) 135(Pt 10):2938–51. doi:10.1093/brain/aws242
18. Schmierer K, Wheeler-Kingshott CA, Boulby PA, Scaravilli F, Altmann DR, Barker GJ, et al. Diffusion tensor imaging of post mortem multiple sclerosis brain. Neuroimage (2007) 35(2):467–77.
19. Popescu V, Klaver R, Versteeg A, Voorn P, Twisk JW, Barkhof F, et al. Postmortem validation of MRI cortical volume measurements in MS. Hum Brain Mapp (2016) 37(6):2223–33. doi:10.1002/hbm.23168
20. Reitan RM, Wolfson D. Theoretical, methodological, and validational bases of the Halstead-Reitan neuropsychological test battery. In: Goldstein G, Beers GS, Hersen M, editors. Comprehensive Handbook of Psychological Assessment: Intellectual and Neuropsycological Assessment. Hoboken, NJ: John Wiley & Sons (2003). p. 105–33.
21. Gronwall DM. Paced auditory serial addition task: a measure of recovery from concussion. Percept Mot Skills (1977) 44:367–73. doi:10.2466/pms.1977.44.2.367
22. Smith A. Symbol Digit Modalities Test: Manual. Los Angeles: Western Psychological Services (1982).
23. Delis DC, Kramer JH, Kaplan E. California Verbal Learning Test Manual. 2nd ed. Adult version. San Antonio, TX: Psychological Corp (2000).
24. Delis DC, Wetter SR, Jacobson MW, Peavy G, Hamilton J, Gongvatana A, et al. Recall discriminability: utility of a new CVLT-II measure in the differential diagnosis of dementia. J Int Neuropsychol Soc (2005) 11(6):708–15. doi:10.1017/S1355617705050812
25. Benedict RH. Brief Visuospatial Memory Test–Revised: Professional Manual. Odessa, FL: Psychological Assessment Resources, Inc (1997).
26. Berg EA. A simple objective technique for measuring flexibility in thinking. J Gen Psychol (1948) 39:15–22. doi:10.1080/00221309.1948.9918159
27. Benton AL, Sivan AB, Hamsher KS. Contributions to Neuropsychological Assessment: A Clinical Manual 2nd ed. New York: Oxford University Press (1994).
28. Hasan KM, Narayana PA. Computation of the fractional anisotropy and mean diffusivity maps without tensor decoding and diagonalization: theoretical analysis and validation. Magn Reson Med (2003) 50:589–98. doi:10.1002/mrm.10552
29. Hasan KM, Halphen C, Sankar A, Eluvathingal TJ, Kramer L, Stuebing KK, et al. Diffusion tensor imaging-based tissue segmentation: validation and application to the developing child and adolescent brain. Neuroimage (2007) 34:1497–505. doi:10.1016/j.neuroimage.2006.10.029
30. Hasan KM, Walimuni IS, Abid H, Hahn KR. A review of diffusion tensor magnetic resonance imaging computational methods and software tools. Comput Biol Med (2011) 41:1062–72. doi:10.1016/j.compbiomed.2010.10.008
31. Hasan KM, Walimuni IS, Abid H, Wolinsky JS, Narayana PA. Multi-modal quantitative MRI investigation of brain tissue neurodegeneration in multiple sclerosis. J Magn Reson Imaging (2012) 35:1300–11. doi:10.1002/jmri.23539
32. Mori S, Kaufmann WE, Davatzikos C, Stieltjes B, Amodei L, Fredericksen K, et al. Imaging cortical association tracts in the human brain using diffusion-tensor-based axonal tracking. Magn Reson Med (2002) 47:215–23. doi:10.1002/mrm.10074
33. Wakana S, Caprihan A, Panzenboeck MM, Fallon JH, Perry M, Gollub RL, et al. Reproducibility of quantitative tractography methods applied to cerebral white matter. Neuroimage (2007) 36:630–44. doi:10.1016/j.neuroimage.2007.02.049
34. Hasan KM, Iftikhar A, Kamali A, Kramer LA, Ashtari M, Cirino PT, et al. Development and aging of the healthy human brain uncinate fasciculus across the lifespan using diffusion tensor tractography. Brain Res (2009) 1276:67–76. doi:10.1016/j.brainres.2009.04.025
35. Kamali A, Yousem DM, Lin DD, Sair HI, Jasti SP, Keser Z, et al. Mapping the trajectory of the stria terminalis of the human limbic system using high spatial resolution diffusion tensor tractography. Neurosci Lett (2015) 608:45–50. doi:10.1016/j.neulet.2015.09.035
36. Lebel C, Gee M, Camicioli R, Wieler M, Martin W, Beaulieu C. Diffusion tensor imaging of white matter tract evolution over the lifespan. Neuroimage (2012) 60(1):340–52. doi:10.1016/j.neuroimage.2011.11.094
37. Beaulieu C. The basis of anisotropic water diffusion in the nervous system – a technical review. NMR Biomed (2002) 15(7–8):435–55. doi:10.1002/nbm.782
38. Fischl B, Salat DH, Busa E, Albert M, Dieterich M, Haselgrove C, et al. Whole brain segmentation: automated labeling of neuroanatomical structures in the human brain. Neuron (2002) 33:341–55. doi:10.1016/S0896-6273(02)00569-X
39. Hasan KM, Walimuni IS, Abid H, Frye RE, Ewing-Cobbs L, Wolinsky JS, et al. Multimodal quantitative magnetic resonance imaging of thalamic development and aging across the human lifespan: implications to neurodegeneration in multiple sclerosis. J Neurosci (2011) 31:16826–32. doi:10.1523/JNEUROSCI.4184-11.2011
40. Desikan RS, Ségonne F, Fischl B, Quinn BT, Dickerson BC, Blacker D, et al. An automated labeling system for subdividing the human cerebral cortex on MRI scans into gyral based regions of interest. Neuroimage (2006) 31:968–80. doi:10.1016/j.neuroimage.2006.01.021
41. Baldo JV, Schwartz S, Wilkins D, Dronkers NF. Role of frontal versus temporal cortex in verbal fluency as revealed by voxel-based lesion symptom mapping. J Int Neuropsychol Soc (2006) 12(6):896–900. doi:10.1017/S1355617706061078
42. Kesler SR, Haberecht MF, Menon V, Warsofsky IS, Dyer-Friedman J, Neely EK, et al. functional neuroanatomy of spatial orientation in processing in Turner syndrome. Cereb Cortex (2004) 14(2):174–80. doi:10.1093/cercor/bhg116
43. Mesaros S, Rocca MA, Kacar K, Kostic J, Copetti M, Stosic-Opincal T, et al. Diffusion tensor MRI tractography and cognitive impairment in multiple sclerosis. Neurology (2012) 78(13):969–75. doi:10.1212/WNL.0b013e31824d5859
44. Riccitelli G, Rocca MA, Pagani E, Rodegher ME, Rossi P, Falini A, et al. Cognitive impairment in multiple sclerosis is associated to different patterns of gray matter atrophy according to clinical phenotype. Hum Brain Mapp (2011) 32(10):1535–43. doi:10.1002/hbm.21125
45. Benedict RH, Ramasamy D, Munschauer F, Weinstock-Guttman B, Zivadinov R. Memory impairment in multiple sclerosis: correlation with deep grey matter and mesial temporal atrophy. J Neurol Neurosurg Psychiatry (2009) 80:201–6. doi:10.1136/jnnp.2008.148403
46. Koenig KA, Sakaie KE, Lowe MJ, Lin J, Stone L, Bermel RA, et al. Hippocampal volume is related to cognitive decline and fornicial diffusion measures in multiple sclerosis. Magn Reson Imaging (2014) 32:354–8. doi:10.1016/j.mri.2013.12.012
47. Pardini M, Bergamino M, Bommarito G, Bonzano L, Luigi Mancardi G, Roccatagliata L. Structural correlates of subjective and objective memory performance in multiple sclerosis. Hippocampus (2014) 24(4):436–45. doi:10.1002/hipo.22237
48. Pravatà E, Rocca MA, Valsasina P, Riccitelli GC, Gobbi C, Comi G, et al. Gray matter trophism, cognitive impairment, and depression in patients with multiple sclerosis. Mult Scler (2017) 23(14):1864–74. doi:10.1177/1352458517692886
49. Dineen RA, Bradshaw CM, Constantinescu CS, Auer DP. Extra-hippocampal subcortical limbic involvement predicts episodic recall performance in multiple sclerosis. PLOS One (2012) 7(10):e44942. doi:10.1371/journal.pone.0044942
50. Fink F, Eling P, Rischkau E, Beyer N, Tomandl B, Klein J, et al. The association between California verbal learning test performance and fibre impairment in multiple sclerosis: evidence from diffusion tensor imaging. Mult Scler (2010) 16(3):332–41. doi:10.1177/1352458509356367
51. Kluver H, Bucy PC. Preliminary analysis of functions of the temporal lobes in monkeys. Arch Neurol Psychiatry (1939) 42:979–1000. doi:10.1001/archneurpsyc.1939.02270240017001
Keywords: limbic system, cognition, multiple sclerosis, diffusion tensor imaging, cortical thickness
Citation: Keser Z, Hasan KM, Mwangi B, Younes K, Khayat-Khoei M, Kamali A, Lincoln JA and Nelson FM (2018) Quantitative Limbic System Mapping of Main Cognitive Domains in Multiple Sclerosis. Front. Neurol. 9:132. doi: 10.3389/fneur.2018.00132
Received: 29 December 2017; Accepted: 22 February 2018;
Published: 12 March 2018
Edited by:
Fabienne Brilot, University of Sydney, AustraliaReviewed by:
Maria José Sá, Centro Hospitalar São João, PortugalRalf Lürding, University of Regensburg, Germany
Copyright: © 2018 Keser, Hasan, Mwangi, Younes, Khayat-Khoei, Kamali, Lincoln and Nelson. This is an open-access article distributed under the terms of the Creative Commons Attribution License (CC BY). The use, distribution or reproduction in other forums is permitted, provided the original author(s) and the copyright owner are credited and that the original publication in this journal is cited, in accordance with accepted academic practice. No use, distribution or reproduction is permitted which does not comply with these terms.
*Correspondence: Zafer Keser, emFmZXIua2VzZXJAdXRoLnRtYy5lZHU=