- 1Section of Neurosurgery, Department of Surgery, Rady Faculty of Health Sciences, University of Manitoba, Winnipeg, MB, Canada
- 2Department of Human Anatomy and Cell Science, Rady Faculty of Health Sciences, University of Manitoba, Winnipeg, MB, Canada
- 3Biomedical Engineering, Faculty of Engineering, University of Manitoba, Winnipeg, MB, Canada
- 4Centre on Aging, University of Manitoba, Winnipeg, MB, Canada
- 5Division of Anaesthesia, Department of Medicine, Addenbrooke's Hospital, University of Cambridge, Cambridge, United Kingdom
- 6Department of Neurology and Neurosurgery, Faculty of Medicine, McGill University, Montreal, QC, Canada
- 7McConnell Brain Imaging Centre, Montreal Neurological Institute, Montreal, QC, Canada
- 8Ludmer Centre for Neuroinformatics and Mental Health, Montreal, QC, Canada
- 9Department of Clinical Neuroscience, Karolinska Institutet, Stockholm, Sweden
- 10Department of Neurology, Karolinska University Hospital, Stockholm, Sweden
- 11Department of Radiology, Rady Faculty of Health Sciences, University of Manitoba, Winnipeg, MB, Canada
- 12Neuroscience Research Program, Kleysen Institute for Advanced Medicine, Winnipeg, MB, Canada
- 13Department of Pharmacology and Therapeutics, Rady Faculty of Health Sciences, University of Manitoba, Winnipeg, MB, Canada
Despite changes in guideline-based management of moderate/severe traumatic brain injury (TBI) over the preceding decades, little impact on mortality and morbidity have been seen. This argues against the “one-treatment fits all” approach to such management strategies. With this, some preliminary advances in the area of personalized medicine in TBI care have displayed promising results. However, to continue transitioning toward individually-tailored care, we require integration of complex “-omics” data sets. The past few decades have seen dramatic increases in the volume of complex multi-modal data in moderate and severe TBI care. Such data includes serial high-fidelity multi-modal characterization of the cerebral physiome, serum/cerebrospinal fluid proteomics, admission genetic profiles, and serial advanced neuroimaging modalities. Integrating these complex and serially obtained data sets, with patient baseline demographics, treatment information and clinical outcomes over time, can be a daunting task for the treating clinician. Within this review, we highlight the current status of such multi-modal omics data sets in moderate/severe TBI, current limitations to the utilization of such data, and a potential path forward through employing integrative neuroinformatic approaches, which are applied in other neuropathologies. Such advances are positioned to facilitate the transition to precision prognostication and inform a top-down approach to the development of personalized therapeutics in moderate/severe TBI.
Background and Current State
Traumatic brain injury (TBI) is one of the leading causes of death and disability globally, carrying significant societal costs (1, 2). Though across the severity spectrum mild TBI is the most prevalent, moderate and severe TBI carry the highest mortality and morbidity burden per case (1, 3). The cornerstone of the clinical management of moderate/severe TBI relies on rapid access to tertiary neurosurgical and neurocritical care services, with the goal of reducing injury insult burden. The primary injury in TBI refers to the structural damage incurred at the time of injury and relies on public health level changes aimed at prevention and risk reduction. Thus, the primary injury burden cannot be changed by the treating clinician, other than policy-level engagements aimed at population-level incidence reduction strategies (1). However, secondary injury refers to the cascade of host cellular responses to the primary injury, which exposes the brain to ongoing insult over the acute and subacute phases of care. The secondary injury cascade is theoretically amenable to therapeutic intervention, with the goal of tissue preservation leading to improved clinical outcomes in the long term (2).
The existing paradigm of clinical care provision in moderate/severe TBI focuses on the “one-treatment fits all” approach, with consensus-based physiologic targets applied to all-comers, regardless of demographics and injury pattern (2). Not surprisingly, recent retrospective evaluation of these guideline-based therapeutic strategies has demonstrated little impact on morbidity and mortality over the last 25-years, despite adherence to such treatment targets (3). This is echoed by additional studies highlighting the individual heterogeneity of cerebral physiome responses in moderate/severe TBI, using high-fidelity advanced multi-modal cerebral physiologic monitoring (4–7). Such work has gone further to demonstrate that much of the cerebral physiologic insult burden seen in moderate/severe TBI is resistant to current therapeutic interventions, calling for more personalized and directed approaches (8–12). Furthermore, aside from the lack of efficacy of guideline-based approaches, the ability to prognosticate in this population has been limited as well, with current population-based standard models accounting for less than half of the variance seen in outcomes (1, 13, 14).
Based on the above, it is readily apparent that the future in moderate/severe TBI care provision calls for two main advances: (1) Precision prognostication and (2) Development of personalized therapeutics directed at secondary injury mechanisms driving morbidity and mortality. Precision prognostication of long-term outcomes would facilitate improved communication between healthcare professionals involved in care provision, and between the care team and families, hopefully minimizing the uncertainty of the future. Further, such prognostication can be applied to higher temporal physiology/cellular states over the course of the patient's acute and subacute hospital stay, facilitating prediction of upcoming events and hopefully early mitigation/prevention. Personalized therapeutics directed at patient-specific secondary injury mechanisms, targeting molecular pathways driving such physiology or cellular dysfunction, would reduce insult burden, alter tissue-fate, and hopefully improving morbidity and mortality for moderate/severe TBI patients.
Though precision prognostication and personalized therapeutics concepts are simply stated, in TBI the practical steps involved in developments within these two areas is complex. Both require integration of complex omics data sets (1), consisting of comprehensive cerebral physiome (4–6), advanced neuroimaging modalities (15–19), proteome (20–24), and genome/epigenome (25–28). Such omics data would be ideally sampled serially, and combined with detailed patient demographics, treatment information, and clinical outcomes. With proper integrative analyses, these complex data sets could inform precise prognostication, tailored to the individual patient. Similarly, these data sets could inform a “top-down” approach to the development of personalized precision therapeutic regimens in moderate/severe TBI care.
Over the past decade, there has been a rapid expansion of omics data reported in the moderate/severe TBI literature. The complexity of such data limits the ability of the bedside clinician to interpret and translate this information into improved prognostication or individualized management. As such, there is a need for advanced integrative neuroinformatics approaches, harnessing techniques in data science and machine learning/artificial intelligence, so that precision prognostication and personalized therapeutics development may become a reality (29–31). Within this review, we begin with an overview of the current state of omics data in moderate/severe TBI, highlighting physiome, advanced neuroimaging, proteome, and genome applications. Next, we outline the current limitation of such data sets in clinical care provision. Finally, we outline a potential path forward to achieve precision medicine in moderate/severe TBI, using advanced integrative neuroinformatics, drawing from recent advances in other neuropathologies.
Current Status of OMICS Data in Moderate/Severe TBI
Within the subsections below, we will highlight some of the pertinent recent literature with relation to the various omics data categories in human moderate/severe TBI. We outline recent discoveries in the physiome, advanced neuroimaging, proteome, and genome research. These sections are not meant to be exhaustive collections of advances, so we direct interested readers to the references and related publications in the field for more details if additional information is desired. The figures providing examples of high-frequency cerebral physiology data were obtained from our existing, approved (University of Manitoba REB: H2017:181, H2017:188, H2020:118) and previously published database work in moderate/severe TBI (9, 10, 32–35).
High-Frequency Multi-Modal Cerebral Physiome
Over recent decades there has been a dramatic expansion of continuous bedside multi-modal cerebral physiologic monitoring, beyond the classic intra-cranial pressure (ICP) and cerebral perfusion pressure (CPP) (4, 6). Such devices include continuous multi-channel near infrared spectroscopy (NIRS) based cerebral oximetry, (36) invasive parenchymal extracellular oxygen partial pressure monitoring (PbtO2) (6, 37, 38), invasive parenchymal thermal diffusion based cerebral blood flow (CBF) monitoring (39), transcranial Doppler (TCD) based cerebral blood flow velocity (CBFV) monitoring (40–42), and continuous electroencephalogram (EEG) (4, 43–45). Many of these devices output full-waveform level data, in high-frequency, with varying levels of literature supporting their association with patient's long-term outcomes (3, 4, 6, 46–49) and variable support from multi-disciplinary groups (4, 5, 50, 51). Some devices, such as PbtO2 monitoring, have shown such robust relationships between low PbtO2 and poor outcome, that randomized trials into ICP vs. ICP and PbtO2 directed therapies are now being undertaken (6). Thus, expanding the characterization of the physiome at the bedside improves our understanding and detection of secondary insult burden. Figure 1 provides an example of a continuous, raw, multi-modal cerebral monitoring data stream in moderate/severe TBI, and demonstrates both the variety and complexity of currently utilized modalities.
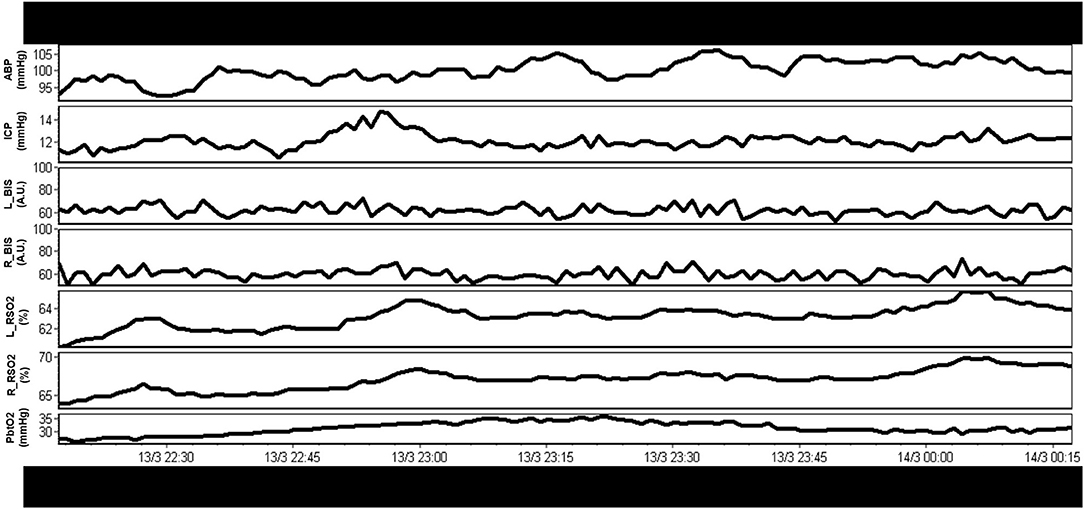
Figure 1. Example of raw continuous multi-modal cerebral physiologic monitoring in moderate/severe TBI. A rich variety of continuous physiologic parameters are currently monitored, however, interpretation of these raw parameters in concert is complex. A.U., arbitrary units; ABP, arterial blood pressure; BIS_L, bispectral index on left hemisphere; BIS_R, bispectral index on right hemisphere; ICP, intracranial pressure; mmHg, millimeters of Mercury; rSO2_L, regional oxygen saturations on left frontal lobe (using near infrared spectroscopy); rSO2_R, regional oxygen saturations on right frontal lobe (using near infrared spectroscopy); PbtO2, extracellular partial pressure of oxygen in the brain tissue; TBI, traumatic brain injury; %, percent. Data taken from previously published and approved studies (University of Manitoba REB: H2017:181, H2017:188 and H2020:118) (9, 10, 32–35).
Aside from the raw physiologic parameters provided from these multi-modal devices, biomedical engineering principles applied to signal analysis has led to additional derived metrics of cerebral physiologic function. Such measures include continuous assessments of autonomic function (52), cerebrovascular reactivity/autoregulation (46, 47, 53), cerebral compensatory reserve (54, 55), and entropy indices (56–58). All such measures have demonstrated associations with patient outcome, many showing strong independent associations and an increased account of outcome variance beyond standard ICP/CPP monitoring, when adjusting for baseline patient characteristics (48, 49, 59). In addition, many of these measures remain independent to current guideline-based therapeutic interventions in moderate/severe TBI care (11, 12), highlighting the need for additional precision therapeutics aimed at the physiologic insult burden/dysfunction that such metrics are monitoring. This ever-growing literature body is further bolstered by the recent acceptance of these parameters by multi-disciplinary consensus groups as important adjunct monitoring variables for TBI care (4, 5, 51). Furthermore, in the spirit of transitioning to more personalized treatment regimens, there has also been recent work describing individualized physiologic targets/thresholds. Such personalized thresholds include continuously updating optimal CPP (CPPopt) (60–64), individualized ICP thresholds (65, 66), and individualized depth of sedation targeting (34), all based on cerebrovascular reactivity monitoring and its relationships with CPP, ICP, and EEG entropy index, respectively. These individualized physiologic targets have shown stronger associations with patient outcome, compared to current guideline “one target fits all” thresholds for CPP and ICP (66, 67). Figure 2 provides an example of continuous derived cerebral physiologic metrics in TBI and demonstrates their ability to be computed in real time, in concert with raw physiologic parameters, enabling them to guide bedside management. Figure 3 provides an example of personalized CPP targeting using cerebrovascular reactivity metrics.
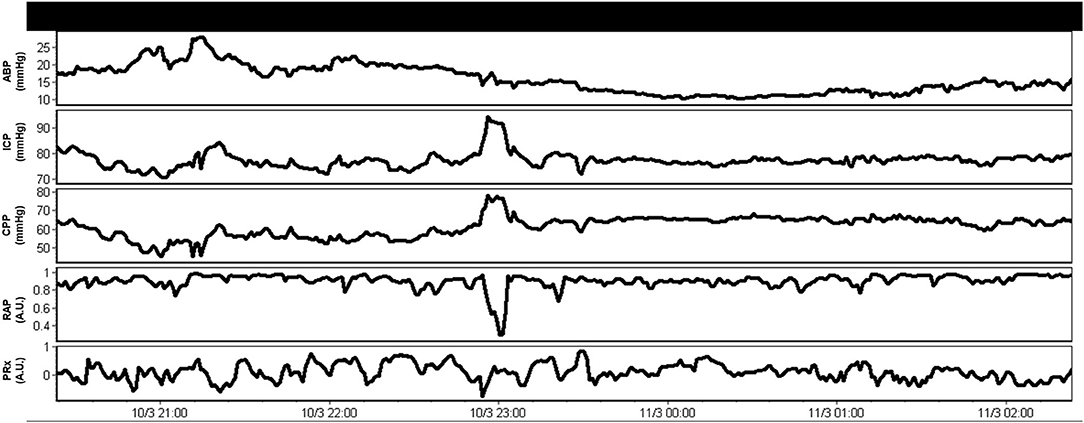
Figure 2. Example of combined raw and derived continuous multi-modal monitoring metrics in moderate/severe TBI. These derived continuous parameters can be computed in real time and made available to the treating clinician to help tailor management. A.U., arbitrary units; ABP, arterial blood pressure; CPP, cerebral perfusion pressure; ICP, intracranial pressure; mmHg, millimeters of Mercury; PRx, pressure reactivity index for cerebrovascular reactivity monitoring (moving correlation between ICP and ABP); RAP, compensatory reserve index (moving correlation between ICP and pulse amplitude of ICP). Data taken from previously published and approved studies (University of Manitoba REB: H2017:181, H2017:188 and H2020:118) (9, 10, 32–35).
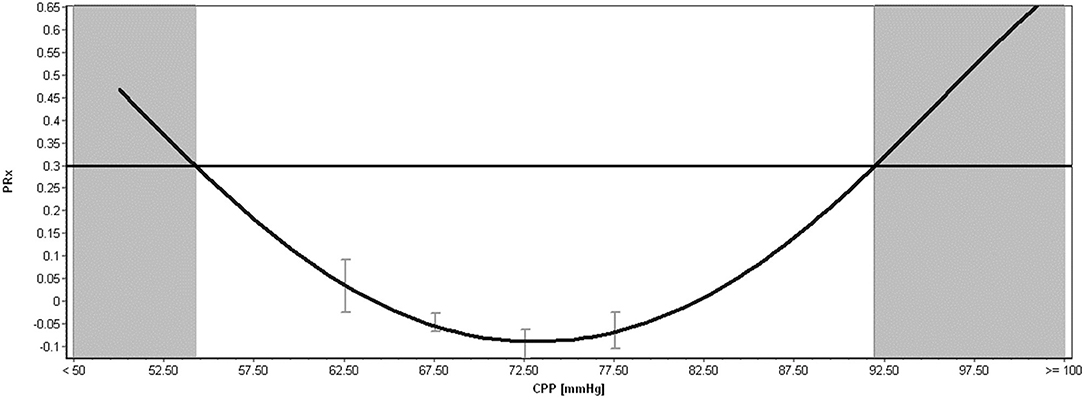
Figure 3. Example of Individualized Optimal CPP, Upper and Lower Limits of Regulation Calculation in Moderate/Severe TBI. CPP, cerebral perfusion pressure; mmHg, millimeters of Mercury; PRx, pressure reactivity index for cerebrovascular reactivity monitoring. The above plot shows an example of individualized optimal CPP estimation, calculated through error-bar plots of PRx vs. CPP and the fitting of a parabolic curve to the relationship. The minimum of this parabolic distribution represents the optimal CPP, based on the past 4-h of data. The shaded areas represent the lower limit of regulation (LLR; on the far left of the figure) and the upper limit of regulation (ULR; on the far right of the figure). The area in-between these two shaded zones represent a theoretical safe CPP range where autoregulation is mostly preserved (i.e., < +0.30), which in this example is a wide CPP range. Both the optimal CPP and LLR/ULR is continuously calculated over time using sliding windows of data, leading to continuously updating individualized bedside targets. Data taken from previously published and approved studies (University of Manitoba REB: H2017:181, H2017:188 and H2020:118) (9, 10, 32–35).
However, despite the promising nature of multi-modal monitoring of the cerebral physiome, the sheer volume of data presented to the clinician often leads to information overload, clouding one's ability to make informed changes in therapeutic intervention for TBI patients. In attempts to improve understanding of such complex high-fidelity data sets, machine learning techniques have been applied (49, 68, 69). Such work has preliminarily led to improved understanding of the multi-variate relationships between multi-model data sets, aided in deriving prognostic models based on patient demographics and cerebral physiology, and set the stage for potential forecasting of cerebral physiologic events into the future using either invasive and non-invasive techniques. Yet, such efforts remain in their infancy, often requiring advanced data science and engineering skill sets for appropriate analysis. Further, integration with other omics data streams has been limited to only a few small biomarker (21, 70) and neuroimaging studies (71–73). As such, there is a desperate need for uniform and comprehensive integration of these complex physiome data sets with other omics data, to facilitate improved prognostication across different time scales, and highlight potential molecular pathways of cerebral physiologic dysfunction that are amenable to precision therapeutic intervention.
Advanced Multi-Modal Neuroimaging Modalities
TBI can be detected using various imaging techniques such as plain head computed tomography (CT), CT angiography (CTA), CT perfusion (CTP), and magnetic resonance imaging (MRI), during the acute phase of care. In the subacute and long-term phases of care, correlation between imaging and clinical phenotype can involve an expanded set of advanced neuroimaging modalities, such as: positron emission tomography (PET), MRI perfusion imaging, functional MRI (fMRI), and diffusion tensor imaging (DTI) to study brain metabolism, CBF, as well as functional and structural connectivity, respectively. Below, we briefly touch on these main categories of imaging modalities in TBI. Table 1 provides a summary overview of selected imaging modalities in TBI.
Computed Tomographic Based Approaches
Plain head CT evaluates the anatomical changes in the brain structures and CTA evaluates anatomical changes in the brain blood vessels, secondary to the traumatic injury. While plain CT and CTA are a good quick assessment of the anatomical changes, these do not provide any functional information of the brain. Furthermore, many of the interpretations are qualitative, as opposed to quantitative, though some advances in quantifiable artificial intelligence based interpretation have emerged (16, 72). In contrast to plain CT and CTA, CTP is an advanced imaging technique that is useful in the detection of TBI and brain death as it provides both an anatomical and functional assessment of the brain (19). In most emergency departments CT is the predominant imaging modality for patients with TBI as it is fast, widely available, and has minimal contraindications. While technologic improvements have reduced the degree of radiation exposure, this still remains a notable disadvantage of CT imaging.
CTP at hospital admission has been proposed as a means to predict the in-hospital mortality in patients with severe traumatic brain injury. However, this needs to be further validated and is currently the subject of an ongoing larger prospective study (NCT04318665). If found to be accurate, CTP may become a valuable initial prognostic tool at the time of presentation that helps inform appropriate clinical management. This is exemplified through the principle of brain death declaration by CTP, where a marked decrease in the CBF and cerebral blood volume (CBV) in the brainstem has been shown to be the most sensitive and specific imaging ancillary test with the highest positive predictive values (74–77). This could help reduce resource intensive but futile care in those who are already brain dead at the time of hospital admission and may facilitate the precious gift of organ donation.
Magnetic Resonance Imaging Approaches
Although MRI can provide superior tissue contrast and spatial resolution compared to CT, MRI is not the preferred modality of imaging in the emergency room setting due to its relatively high cost, limited availability within or immediately adjacent to the ER, and slow image acquisition. Additionally, screening for the presence of contraindications is often not possible in those with moderate and severe TBI at the time of presentation. However, when available and when possible, MRI can provide valuable anatomical information, and methods such as MRI-based susceptibility weighted imaging (SWI) have been shown to be particularly sensitive for detecting micro-hemorrhages in TBI patients. However, these microbleeds are not necessarily colocalized with the microstructural changes (78). Nonetheless, while CT continues to be predominantly used for assessment of TBI patients in the ER at present, MRI techniques hold significant promise for the future.
Magnetic Resonance Perfusion Approaches
Consideration of the dynamics of CBF/CBV and perfusion can be facilitated through advanced magnetic resonance perfusion (MRP). This is non-invasive and can be used to obtain the functional information like that from CTP to detect CBF. However, for the reasons listed above, MRP has yet to be commonly used in the clinical setting for severe TBI patients (53).
Diffusion Tensor Imaging Approaches
Diffusion tensor imaging (DTI) is an advanced MRI technique that is based on the diffusion of protons in neuronal axons and provides vital information on the structural connectivity within the brain. The two main clinically relevant DTI parameters are fractional anisotropy (FA) and mean diffusivity (MD). FA measures the anisotropic diffusion of protons within a voxel and MD depicts the average diffusion of protons over all sampled directions. Normal white matter of the brain usually demonstrates anisotropic diffusion along the direction of the axons resulting in a high FA and low MD. However, in patients with TBI, traumatic axonal injury results in altered FA and MD measurements in the various involved brain regions to a degree dependent on the severity of the brain injury (79). In one study, of the regions examined, 88 and 92% had significantly lower FA in mild and moderate/severe TBI subgroups, respectively, compared to the controls. MD was found to be higher in 95 and 100% of the brain regions examined in those with mild and moderate/severe TBI, respectively, when compared to controls. A recent meta-analysis showed that high FA and low MD is associated with better cognitive outcomes (80). However, more research is needed before this important technique is used in routine clinical practice to inform individual patient decision making (81).
Positron Emission Tomography Approaches
The biggest advantage of PET over other in vivo imaging methods (e.g., MRI or CT) is the possibility to investigate specific biochemical and cellular processes by modifying the tracer used. Fluorodeoxyglucose (FDG), a radiolabeled glucose analog, is the most widely used PET radiotracer as it allows one to estimate regional glucose uptake, which is useful for delineating hypermetabolic tumors. In TBI, FDG uptake is known to be elevated immediately following trauma for a short period of time (up to 3 h), followed by a longer term decrease lasting days to weeks (82). A reduced degree of hypometabolism, in the sub-acute phase, has been associated with better long term outcomes (83, 84). However, precise mechanistic attribution of FDG PET observations to singular events has been elusive as FDG uptake can be affected by several processes, including inflammation (increase), cell dysfunction (increase or decrease), atrophy (decrease), and neurotransmitter activities (increase or decrease).
Other radiotracers of interest in TBI research include those that are sensitive to tau proteins and translocator proteins (TSPO). Among many, tau PET tracers (e.g., [18F]flortaucipir) has garnered the most interest as it enables quantification of phosphorylated tau in vivo, and thus directly addresses accumulation of the neuropathological hallmark of chronic traumatic encephalopathy (85, 86). The biggest limitation of tau PET imaging is its unspecific binding to beta-sheets (e.g., beta-amyloid plaques and alpha-synuclein) and other molecular structures (87). TSPO is a surrogate marker for microglial activation and astrogliosis; thus, TSPO PET yields a new opportunity to quantify neuroinflammation and has gained traction in the field (88). However similar to tau PET tracers, TSPO tracers have not demonstrated the desired specificity (89), again limiting definitive mechanistic inferences. Additionally, 2nd generation TSPO tracers have anrs6971 polymorphism-dependent binding affinity which further limits their generalizability (90).
Functional Magnetic Resonance Imaging Approaches
Although there are actually different types of fMRI contrast (91), blood oxygen level-dependent (BOLD) fMRI is by far the most common, and is based on the principle that elevated regional CBF (and there for increased oxygen delivery) follows neuronal activation in the brain in order to meet locally increased metabolic demand (92). The resulting spatiotemporal increase in oxygenated/deoxygenated hemoglobin ratio can be sensitively measured by acquiring a series of T2- or T2*-weighted MRI images, allowing signal changes over time to be interpreted as changes in local neural (neuronal and astrocytic) activity (92, 93). In 1997, Biswal and colleagues reported that BOLD fMRI signals also fluctuate synchronously in distant brain regions even when an individual is not engaged in a particular task (94). Those brain regions with synchronously fluctuating activities form intrinsic connectivity networks (ICNs) (95) ICNs are often characterized by independent component analysis (ICA) of resting-state low-frequency (typically ranging from ~0.01 to 0.1 Hz) fMRI data. Among different ICNs, the abnormalities of default mode network (DMN) have been frequently associated with TBI (96–99), and a very recent systematic review revealed that the most frequently studied MRI-based brain connectivity biomarkers for mild TBI to date are global functional connectivity and DMN functional connectivity (along with DTI measures of FA) (100).
The DMN is one of the most extensively studied brain networks, the disturbance of which has been associated with many neurological and psychiatric disorders that affects consciousness (101, 102). The DMN is situated in the posterior cingulate cortex (PCC) and medial prefrontal cortex (mPFC) with prominent nodes in the medial temporal lobe (MTL) and the angular gyrus (103). Following partial recovery of consciousness after an acute TBI that resulted in coma, patients in a minimally conscious or confused state showed partially preserved intra-DMN connectivity while such connectivity was absent in those that remained comatose (97). The DMN connectivity returned to a normal level upon full recovery of consciousness (97), although persistently altered DMN connectivity (>1 year since injury) predicted poor emotion recognition and social integration (96) as well as cognitive dysfunction (104) in TBI patients. While promising, more longitudinal studies with larger sample size are warranted prior to the wide adoption of fMRI for clinical use in TBI.
Machine Learning Approaches
The basic concept of most neuroimaging machine learning techniques is to classify patients based on human-annotated datasets. For example, Mitra et al. (105) trained a random forest classifier that identifies diffuse axonal injury-containing FA maps (DTI measurement) of TBI patients (n = 179) from healthy controls (n = 146) with moderate accuracy (~68%). Better accuracy was achieved when resting-state fMRI (84.1%) was used with support vector machine than when FA maps (75.5%) were used in different cohort of TBI patients (n = 50; control n = 50) (106). While these studies demonstrate a proof-of-concept of how machine learning approaches, using neuroimaging data, can be applied in TBI care, the true advantage of machine learning is achieved if the human-annotated labels are taken from follow-ups and linked to the baseline imaging data, which is used to train a prognostic classifier that can be used at an earlier stage (107, 108). To fully benefit from these recent technical advances, a large longitudinal neuroimaging database is necessary. This will allow for detailed phenotypic data to be linked to appropriately sized training, validation, and test sets, therefore generating the most robust neuroimaging based machine learning models.
Serial Proteome of Serum, Cerebrospinal Fluid, and Extracellular Space
Protein biomarkers of tissue fate play a significant role in diagnostics, prognostics, and monitoring across a wide array of medical fields. In TBI, throughout the last decade, a number of proteins in serum have been suggested as having clinical utility. These include the primarily glial/astrocytic S100 calcium-binding protein B (S100B) and glial fibrillary acidic protein (GFAP), the neuronal Neuro-Specific Enolase (NSE) and Ubiquitin C-terminal Hydrolase L1 (UCH-L1) as well as the axonal Neurofilament-Light (NfL) and tau (109). By combining these markers with different cellular origins, as well as different serum kinetics (22), improved prognostication and assessment of injury severity can be achieved following moderate-to-severe TBI (110). S100B, which has been available in clinical assays for almost 20 years, has been implemented in guidelines in order to rule out the need for CT scanning in mild TBI (111). Further, it is used regionally to monitor unconscious TBI patients in order to detect lesion progression or development of neuronal insults during intensive care (112, 113). Other than S100B, only NSE exists in clinical assays [though GFAP was just approved in a point-of-care device (114)] and is used in guidelines for anoxic brain injuries following cardiac arrest (115). However, experimental clinical studies of longitudinal, serial sampling show that the other proteins exhibit similar capacity as S100B in detecting secondary neuronal insults in unconscious TBI patients (22). Additionally, S100B levels have been associated with the strength of resting-state brain connectivity in multiple resting-state networks on fMRI following severe TBI, highlighting its capacity as a global brain injury marker (116).
In terms of multiplexing the proteome following TBI, these studies are scarcer, and almost all have focused on profiling the proteome of either the CSF (117–120) or brain tissue (121) following injury. In a study of three TBI patients by Hanrieder et al. serial sampling of CSF, in the first 2 weeks following injury, using a shotgun proteomic approach showed that acute phase reactants, fibrinogens, as well as brain enriched proteins like GFAP and NSE demonstrated interesting temporal trends as tentative biomarkers (118). Proteomic approaches have been attempted by analyzing the brain extra-cellular fluid (ECF) cytokine response using multiplex techniques (24, 122–125), revealing interesting trends for some inflammatory proteins. Altogether, the field of proteomic profiling of brain fluids following TBI is still in its infancy as these mass spectrometry-based approaches are difficult to conduct on a larger scale, but new techniques in preliminary reports of both CSF and brain-ECF from TBI patients reveal several tentative markers of brain injury that may have clinical utility in the management of these patients.
Similarly, blood brain barrier (BBB) integrity and function may also be surveyed utilizing advanced proteomic approaches in TBI. Lindblad et al. demonstrated that the brain to blood clearance of S100B was associated with BBB integrity, while that for NSE was not (126), hence suggesting that different proteins might be more associated with BBB disruption than others. In another recent work by Lindblad et al. utilizing a protein-array targeting brain enriched and inflammatory proteins in 186 TBI patients, the extent of BBB damage was associated with increased complement proteins in CSF, presumably indicating an association between ongoing secondary injury, BBB disruption and a subsequent neuroinflammatory response (127). A similar trend could be seen in blood, but was more evident in the CSF compartment, highlighting the need for appropriate compartmental monitoring in order to accurately monitor pathology. Several inflammatory and structural proteins provided independent information in outcome prediction models. Thus, novel proteomic approaches reveal that BBB-disruption is a key event following TBI and seems to be associated with neuroinflammation, both clinically relevant secondary injuries that should be acknowledged for future research.
Finally, metabolic failure following TBI has been associated with different pathophysiological conditions, however the most detrimental is considered to be mitochondrial dysfunction (128). This is a condition that has been identified following TBI in animal models (129, 130), where different techniques exist to detect mitochondrial dysfunction. However, many of these laboratory techniques are not applicable in vivo in humans (131). Instead, in order to assess biochemical substances in the ECF in vivo, cerebral microdialysis (CMD) is commonly used (70). CMD consists of a probe of a semi-permeable membrane where a carrier fluid is being pumped through it, and in the process, extracting ECF substances through diffusion. This allows for serial sampling and thus consecutive bedside monitoring of focal cerebral metabolism. There have been several consensus meetings dealing with how to best optimize CMD as a clinical tool for metabolism monitoring, the most recent published in 2015 (132). The primary markers of metabolism that are monitored clinically in brain ECF are glucose, lactate and pyruvate.
In the case of a deranged cerebral metabolic redox state because of impaired mitochondria, there will be an accumulation of ECF brain lactate (as a surrogate marker for NADH) and a subsequent decrease of ECF pyruvate levels (a marker of NAD+) (133). An ECF lactate/pyruvate ratio (LPR) >25 has been independently associated with an unfavorable outcome following TBI (21). A deranged LPR retrieved from CMD has also been associated with an impaired phosphocreatine/ATP ratio, as a sign of an effect on the brain energy state (134). The association of brain ECF LPR and mitochondrial dysfunction is also supported in large animal experiments where cyanide is administrated, resulting in a prompt LPR increase (135). However, in CSF, while being suggested as a marker of mitochondrial disease (136), LPR has not been seen to be a similar marker of deranged metabolism following TBI. Studies either do not show any difference between TBI and healthy controls (137), or fail to see an association with outcome (138), stressing the necessity for compartmental monitoring in order to tailor treatment strategies following TBI (139).
Candidate Gene Studies and Genome-Wide Association Work
The role of genetic variations, such as single nucleotide genetic polymorphisms (SNPs), in the heterogeneity of outcomes following TBI has been known for some time and has been the focus of a recent review by Gomez and colleagues. Polymorphisms in candidate genes involved in neural repair, BBB integrity, neurotransmission, inflammation, as well as those associated with neurodegenerative disease have all been examined for their association with global, cognitive, and physiologic outcomes following injury (27). Table 2 provides a general overview of major categories of candidate SNPs that have been explored in TBI to date. We refer the interested reader to comprehensive reviews on the topic in TBI for more information if desired (25–28).
The APOE gene has been the subject of numerous outcome association studies due to its role in neurodegenerative diseases such as Alzheimer's disease. Three polymorphisms of the gene are commonly found, ε2, ε3, and ε4, of which the ε4 allele has been found to be associated with increased risk of late onset Alzheimer's disease (140). This led to its examination by Teasdale et al. in 1997, who found that those with an APOE ε4 allele were significantly more likely to have poor global outcomes at 6 months post-injury as measured by Glasgow Outcome Scale (GOS) (141). Since this seminal work, numerous studies, and meta-analyses have found the APOE ε4 allele to be associated with worse global outcomes following TBI (28, 142, 143). The ε4 allele has also been linked to worse cognitive outcomes following TBI with some studies finding the allele associated with worse memory, processing speed, and overall increased cognitive impairment (144–146). However, not all studies have found an association with worse cognitive outcome and so further work is needed (147–149). Currently, the association of the APOE ε4 allele with poor outcomes is thought to be mediated by abnormal lysing of its protein product resulting in neurotoxic byproducts that worsen secondary injury and impair recovery (28).
Genes involved in neuronal repair and survival have also been examined for their association with outcomes following TBI. The BDNF gene, which encodes brain-derived neurotropic factor (BDNF), has been examined for association between its polymorphisms and global and cognitive outcomes. Early work by Failla et al. point to an association with certain SNPs and global outcomes mediated by interactions with age and serum BDNF levels (150, 151). When examining associations with cognitive outcomes following injury, the rs6265 allele was found to be linked to improved recovery of executive function, working memory, processing speed and other cognitive domains when compared to wild-type alleles (152–154). However, conflicting results have also been reported with the variant allele being associated with worse cognitive outcomes (155, 156). The mechanism by which this may be mediated is poorly understood.
Association studies have also been conducted on genes encoding ATP-binding cassette (ABC) transporter proteins, which are integral in the function of the BBB. SNPs of the genes encoding some ABC proteins have been found to be associated with global and cognitive outcomes while others have not (157–159). Notably, polymorphisms in the gene encoding ABCC8, a member of the ABC transport protein family, have been associated with elevated ICP and CT findings of cerebral edema in the acute phase of TBI (158, 160).
Similarly, polymorphisms in the genes that encode proteins involved in neurotransmitter metabolism, such as catechol-O-methyltransferase (COMT), have yielded contradictory results (160, 161). Association studies examining polymorphisms in genes integral to inflammation have also failed to provide uniform results (162–164). However, one study did find that polymorphisms in the gene encoding the inflammation associated protein IL-1β carried different risks for post-traumatic seizures/epilepsy (165).
Conflicting results and replication issues in candidate gene studies are well-documented in the field of human genetics (166) and reflect the need for unbiased genome-wide association studies (GWAS) to be performed in large, comprehensively phenotyped TBI cohorts. GWAS findings for complex traits have been shown to be more reliably replicated and are able to give insights into novel biology (167). For example, they can identify novel drug targets, infer the most relevant cell types and define the genetic heritability of the traits being studied. Future studies should genotype TBI cohorts to assess the predictive capabilities of polygenic scores derived large-scale GWAS from TBI-related traits in stratifying clinical outcomes in a standardized manner (168). Large TBI cohorts would also allow for the identification of genome-wide significant loci that have the potential to be novel therapeutic targets (169). Current initiatives that can facilitate GWAS in TBI include the Genetic Associations in Neurotrauma (GAIN) Consortium, the Enhancing Neuroimaging Genetics through Meta-Analysis (ENIGMA) Consortium, and the TBI-related focus of the Psychiatric Genomics Consortium-PTSD Working Group (PGC-PTSD) (17, 170) However, more focus needs to be placed on efforts to recruit and genotype research participants that have experienced moderate/severe cases of TBI. Further, significant work needs to be done before the evaluation of target gene polymorphisms are integrated into TBI care. However, a better understanding of the contribution of genotypic variation in global and cognitive outcomes following injury may aid in prognostication and eventually may allow for more personalized therapeutic targets.
Limitations in the Application of Current Comprehensive OMICS Data in TBI
The main limitation associated with omics data streams in TBI is the overall complexities inherent with each data type. In general, integration of each omics data type is difficult given different temporal and spatial resolutions, requiring multi-disciplinary expert collaboratives in order to achieve such goals. This is also the case when adding baseline patient characteristics, treatment information, and serially assessed comprehensive outcomes. Aside from this, each data stream has its own specific considerations, which have limited their integration for precision medicine approaches in moderate/severe TBI.
Multi-modal high frequency physiologic data sets carry a few limitations. Data is typically streamed in full physiologic waveform format, leading to high data storage requirements, specialized data management, and biomedical engineering skills for processing and analysis. This is particularly the case for some of the more advanced derived metrics of cerebrovascular function, compensatory reserve assessments, and individualized CPP and ICP target characterization (34, 60–62, 65, 66). Further, with expansion of the various continuous cerebral physiologic monitoring possibilities, there now exists substantial volume of concurrent continuous data streams presented to the treating clinician, leading to information overload, and difficulties in knowing which information to act on. Finally, many of these physiologic data streams are only available during the acute phase of care, given their invasive nature. Thus, serial follow-up assessments of continuous cerebral physiology have classically not occurred. Though this has recently changed with advances in non-invasive monitoring and biomedical engineering techniques (171–174).
Advanced neuroimaging platforms suffer from a lack of temporal resolution, sacrificed for the sake of spatial resolution. As such, these snapshots of cerebral structure and connectome, have limited translatability to bedside care and integration with more continuous omics data streams. This is especially the case in the setting of critically ill moderate/severe TBI patients, where transport and care provision during lengthy advanced neuroimaging sequences are often not feasible, except for a few centers globally with advanced imaging platforms and critical care units co-located. Consequently, the costs associated with facility development, maintenance, need for specialized personnel (such as radiochemists for advance PET tracers, or MRI physicists for novel sequence development) and image acquisition at the individual study level, can be prohibitive for many centers globally.
Proteome biomarker data suffers from slightly different complexities. The lower sampling frequency of such serially measured samples makes it difficult to integrate with higher resolution physiologic data streams that typically drive bedside decision making in the acute phase of moderate/severe TBI (20, 22, 70). Though sampling frequency typically outstrips that of neuroimaging. Furthermore, given the above limitation of follow-up continuous physiologic assessments, many long-term serial biomarker studies have failed to be linked to cerebral physiology, limiting interpretability. Finally, most work to date has focused on small sets of protein biomarkers of interest, such as pro-inflammatory cytokine profiles (23, 123, 125), given the need for expertise in sample management, processing, and interpretation biologic significance. As such, proteome-wide analysis has not been conducted and may shed further light on molecular pathways driving secondary injury in TBI.
Finally, genomic and epigenomic data suffers two main issues in the TBI population. Sample size in TBI research has classically been quite small, in comparison to other genomic studies in non-neurological populations. Most moderate/severe TBI studies have <1,000 patients (26, 28). Thus, genome-wide approaches are left as exploratory initiatives, with limited conclusions drawn at the population level. Further to this, the volume of information obtained through current sequencing platforms is extensive and has limited investigations in this area to a few specialized centers. Integrating such data sets with other serially sampled omics data streams is also a challenge, particularly when attempting to comment on potential molecular pathways driving secondary injury.
Integrative Neuroinformatics: Future of Precision Medicine in TBI
Based on the above-mentioned complex data streams, and limitations associated with their application, there exists a clear need for the use of advanced data science strategies to facilitate better understanding. As mentioned, some preliminary application of machine learning techniques has been conducted in high-frequency multi-modal cerebral physiologic data, development of automated neuroimaging-based predictions, and prognostic model derivation in moderate/severe TBI. However, such advances have yet to translate to wide sweeping changes in bedside care, or personalized precision medicine. Given the intrinsic complexity of TBI and the massive amount of information “encrypted” in most data modalities, in practice, there are multiple challenges for patient screening and for obtaining a better explanation of the individual neuropathological mechanisms. That is, collecting the above-mentioned data streams is a necessary but not sufficient condition for achieving a better understanding of the underlying neuropathological mechanisms and preventing clinical deterioration. In practice, comprehensive integrative neuroinformatics platforms are required to drive precision prognostication and derivation of personalized therapeutics targeting secondary injury mechanisms. As such approaches would be novel in moderate/severe TBI, here we propose a brief roadmap for the adaptation of significant advances recently made in other neuropathological conditions, such as neurodegenerative processes.
First, predicting the individual course of a progressive neurological condition, such as moderate/severe TBI, is of crucial importance for early diagnosis and optimum treatment selection. The last decade has seen a tremendous advance in neuroinformatic methods aiming to track disease advance in the context of most-prevalent neurodegenerative disorders (175–178). Sophisticated mathematical and statistical models, including novel Artificial Intelligence (AI) techniques, are increasingly allowing to decode the “encrypted” patterns in big neuroscience data. In general, neuroinformatic models of disease progression (DP) combining multi-modal data (electrophysiological, peripheral, genetics, imaging, clinical, etc.) with advanced computational techniques can be classified in two main categories: empirical and mechanistic. Empirical DP models (177) focus on making disease predictions (e.g., which subjects will develop the disease vs. which subjects will not) but without offering a detailed biological explanation of the underlying neuropathological causes. On the other hand, mechanistic DP models (29, 179–181) may make predictions, but their primary goal is to clarify disease mechanisms (e.g., which genes or brain areas are driving the disease, and why).
In the moderate/severe TBI context, it is critical to consider the distinction between these two DP modeling categories, while empirical approaches can represent powerful predictive tools, they constitute “black-boxes.” This handicap is not common to mechanistic models, which (in part to remain interpretable) often achieve a lower predictive capacity. In TBI applications, in order to maximize the tradeoff between the models' clinical predictability and biological interpretability, it will be essential to combine the advantages of empirical and mechanistic brain disease models (182). For example, in a recent neurodegeneration study (183), state-of-the-art machine learning advances for exploring and visualizing high dimensional data (184) were used to define contrasted disease trajectories and clinically screen the patients. This semi-unsupervised method (named contrasted Trajectories Inference [cTI]) uncovers the underlying neurodegenerative path in large-scale omics data, accurately identifying the series of sequential molecular states (genetic alterations) covering decades of disease progression, and subsequently revealing the relative position of each individual subject in that path. When applied to in-vivo microarray gene expression data from the blood of 744 subjects in the late-onset Alzheimer's disease (LOAD) spectrum (ADNI data; Figures 4A,B), cTI automatically identifies disease-associated patterns of genes that mirror neuropathological and clinical alterations (Figure 4C), and subsequently detects the relative ordering of individuals that better align with those patterns (Figure 4D). That is, by finding the relative position of each subject on the long-term disease “timeline” (Figure 4D), cTI provides a personalized molecular disease index that significantly predicts individual neuropathology (tau, amyloid and infarct positivity), cognitive deterioration and future clinical conversion (Figures 4E,F). Similarly, when evaluated on 1225 post-mortem brains in the spectrum of AD and Huntington's disease (ROSMAP and Harvard Brain Tissue Resource Center data [HBTRC]), it strongly predicts neuropathological severity and comorbidity (Braak, Amyloid, and Vonsattel stages). A notable attribute of the cTI approach is that it allows estimating the specific contribution of each gene on the identified disease “timeline” and the obtained personalized molecular disease index, based on the analysis of the model's resulting weights/loadings for each biomarker included in the original data (i.e., ~40,000 gene transcripts). Simply put, this predictive and semi-mechanistic technique overcomes the traditional AI “black-box” limitation, allowing the direct discovery of genes and related molecular pathways underlying disease evolution.
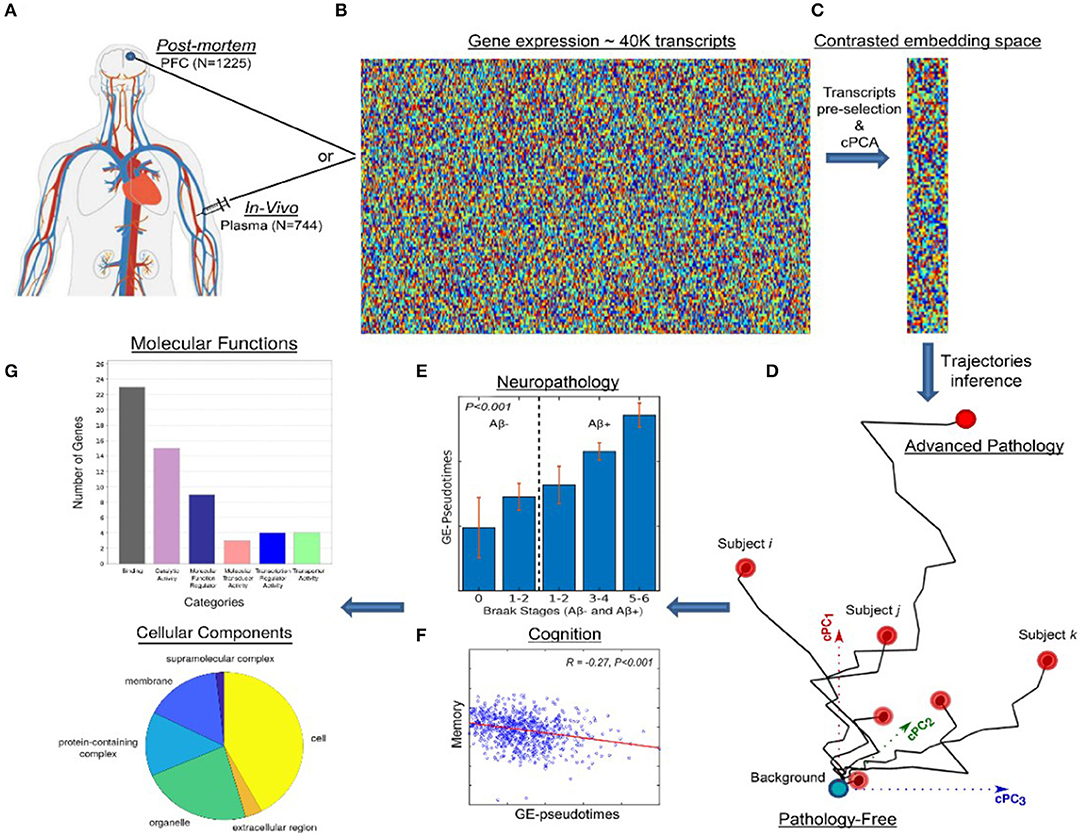
Figure 4. Schematic approach for detecting molecular disease-associated patterns and patient staging. In-vivo blood (N = 744; ADNI) and post-mortem brain (N = 1,225; ROSMAP, HBTRC) tissues (A) are screened to measure the activity of ~40,000 transcripts (B). This high dimensional data is reduced to a set of disease-associated components (C) via contrastive PCA and/or variational autoencoders 0.171. This allows to represent each subject in a disease-associated space (D) where the corresponding position reflects her/his pathological state (with proximity to the left bottom corner implying a pathology-free state and to the right top corner implying advanced pathology). An individual molecular disease score is then calculated, reflecting how advanced is each subject in his/her disease-trajectory. This score significantly predicts neuropathological (E) and cognitive measurements (F). Finally, the resulting model weights allow the direct identification and posterior functional analysis of most influential genes (G). Image adapted with permission from Iturria-Medina et al. (183).
Computationally, it is also feasible to go in the inverse methodological direction (from characterizing mechanisms to performing treatment-effect predictions). In another recent neurodegeneration study by the same group, a neuroimaging-based personalized Therapeutic Intervention Fingerprint (pTIF; Figure 5) was introduced, aiming to characterize brain mechanisms for subsequently predicting individual treatment needs (185). Based on spatiotemporal analysis of multi-modal imaging data (i.e., PET, MRI, etc.), the pTIF demonstrates that the patients may need different treatments, not only depending on their brain's unifactorial alterations (e.g., tau and amyloid accumulation or not, dopamine alteration or not, atrophy or not) but also on their individual multifactorial brain dynamics: how the different biological factors interact and how they would respond (at the individual level) to potential clinical perturbations. In summary, after quantitatively characterizing basic disease mechanisms at the individual level (e.g., intra-brain spreading of tau and amyloid proteins, and their toxic interaction with vascular and structural factors), the pTIF integrates large amounts of data (e.g., millions of multi-modal brain imaging measurements) into a simplified individual patient profile of the quantitative biological factor modifications needed to control disease evolution. Results in aging and LOAD (ADNI data, N = 331; see Figure 5) support that pTIF allows to categorize the patients into distinctive therapy-based subtypes. These subtypes were relevant by comparing them to the patients' individual genetic profiles, finding that each pTIF-subtype presents a distinctive/characteristic pattern of gene expression alterations in the blood.
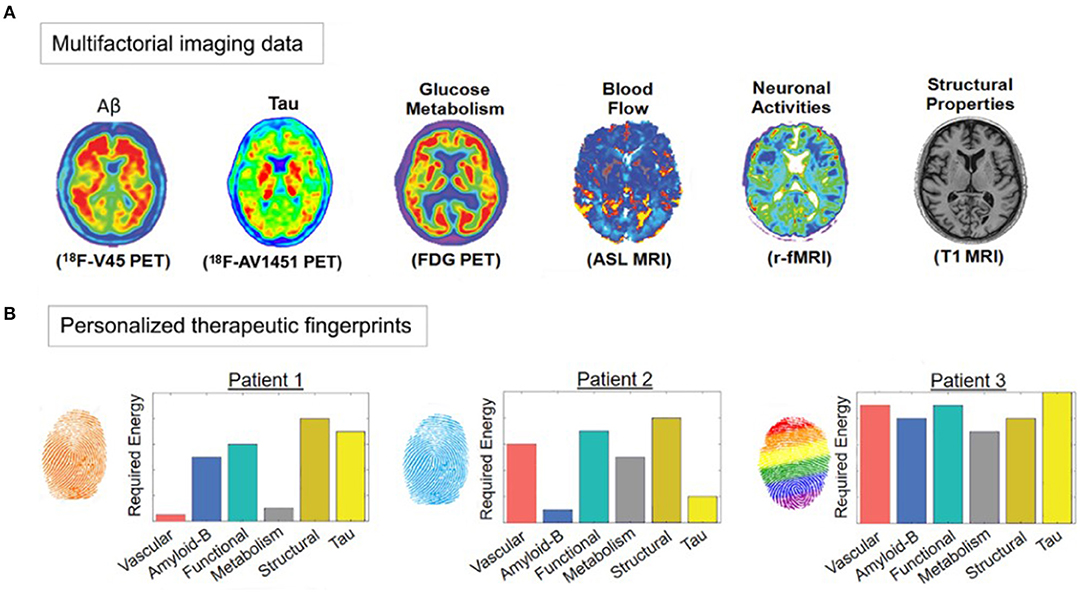
Figure 5. Imaging-based therapeutic intervention fingerprinting approach (A) Amyloid, tau, and F-fluorodeoxyglucose (FDG) PET, and Cerebral Blood Flow (CBF), functional activity at rest and gray matter density from MRI. A network-based approach allows individual characterization of the intra-brain direct factor-factor biological interactions and the spreading mechanisms through vascular/anatomical connections. Inverting the model's equations allow estimation of the optimum required to produce a desired clinical state (e.g., healthy). (B) Different pTIF patterns for three participants with similar clinical status. Note that Patient 1 requires lower cost-energy values for vascular and metabolic interventions, while Patient 2 requires lower values for anti-Aß and anti-tau interventions, suggesting the identification of specific single-target therapies that may benefit these patients (e.g., physical exercise and aducanumab, respectively). However, Patient 3 requires high cost-energy for all the single-target interventions, suggesting that combinatorial (and not single-target) treatments will be most beneficial. Image adapted with permission from Iturria-Medina et al. (185).
In summary, to achieve true personalized care in moderate/severe TBI, it is vital to use advanced neuroinformatic tools. For this, the combination of both empirical (highly predictive) and mechanistic (biologically interpretable) disease progression models is an indispensable associated step, as important as the acquisition of multifactorial data (molecular, electrophysiologic, neuroimaging, clinical, etc). Deep mathematical and computational modeling of multidimensional neurological disorders is, however, complex and time demanding. The TBI research and clinical community could take advantage of the growing number of techniques initially developed for other neurological conditions (Alzheimer's, Parkinson's, depression), which have been tested and validated in large-scale heterogenous datasets. As such, the use of already available Open-Access neuroinformatic tools (177, 178, 182, 186), integrating a large variety of biological data for a better understanding of individual disease progression and treatment needs, may accelerate the adaptation and improvement of advance computational approaches in the moderate/severe TBI context.
Leveraging Integrative Neuroinformatics Discoveries for Precision Medicine
Integrating comprehensive serial physiome, neuroimaging and proteome information, with genome/epigenome data from multicenter clinical data sets, will facilitate highlighting various pathways of cerebral physiologic dysfunction of interest which are driving current poor outcomes. Using this information in a top-down fashion, cellular/small animal and large animal models could then be developed to explore these in more detail. Here, detailed probing of molecular drivers of secondary injury could occur, with comprehensive pathway elucidation and characterization of connectomic and histopathological consequences of dysfunction. Understanding here would be expected to lead to discovery of precision targets aimed at prevention and intervention for such secondary injury mechanisms. Precision therapeutics would then need to be further explored in controlled healthy and TBI small animal models, to gain understanding of connectomic, physiologic, and tissue consequences of such interventions. However, in order to circumvent past failure of clinical translation from cellular/small animal models, large animal TBI models would need to be employed as well.
Cellular/small animal and large animal platforms would be complementary and poised to inform one another in the process of target elucidation and precision therapeutics development. Comprehensive multi-modal high-resolution cerebral physiologic monitoring would be applied in large animal models, with serial serum and extracellular fluid sampling for protein biomarkers and whole brain explantation post-experiment for evaluation of histopathological correlates. Such platforms would facilitate directed validation of emerging personalized medicine approaches to TBI care, exploration of precision therapeutics directed at secondary injury pathways, and assessment of histopathological consequences of various interventions in detail on a model which closely represents the human cerebrum in structure and physiology. Such large animal platforms would focus on moderate/severe TBI simulation and therapeutics development, to bridge the existing gap in translating small animal model discoveries to humans. Further, this platform would serve as the ultimate pre-clinical means of precision therapeutics investigation prior to implementation in humans. Discoveries here would lead to translatable changes to bedside care, focused on individualized care plans, and transitioning away from failed guideline approaches toward personalized medicine in TBI care.
Fundamental Requirements for Future Work
Based on the integrative neuroinformatics framework presented here, there are some basic requirements to facilitate this in moderate/severe TBI. First, the comprehensive clinical phenotyping requires multi-center collaborations, in order to build enough power to adequately inform such prognostic models and top-down exploration of molecular pathways of secondary injury. This requires that each site has the capacity for serial high-resolution data capture for the physiome, proteome and neuroimaging. Similarly, it requires the basic infrastructure and personnel support to ensure complete data acquisition and proper bio sample storage. With the aid of existing specialized TBI research groups, such as the Canadian Traumatic Brain Injury Research Consortium (CRTC) and the CAnadian High-Resolution TBI (CAHR-TBI) research collaborative (33), such comprehensive data collection strategies are feasible. Second, multi-disciplinary groups of specialist clinicians and scientists are required. Expertise in epidemiology, data science, genetics, proteomics, advanced neuroimaging, biomedical engineering, cerebral physiology, and neuroscience are required to facilitate success with such integrative neuroinformatics approaches. Third, comprehensive pre-clinical platforms are necessary to facilitate exploration of molecular pathways of secondary injury. It is through cellular, small and large animal models of neural injury, that pathways uncovered by neuroinformatic approaches applied to comprehensive clinical data, can be explored in more detail. Cellular and small animal platforms would facilitate exploration of such molecular pathways, derive therapeutic targets, and highlight histopathological correlates. Similarly, given issues with translatability of findings from cellular/small animal TBI platforms in the past, large animal models of TBI are also needed to allow for further pre-clinical investigation of therapeutic targets, interventions and histopathological outcomes on cerebral structures more closely related to humans (187). Such large models are key in bridging the current bench-to-bedside gap in TBI research. Fifth, based on discoveries from the top-down approach to precision therapeutic development, translation to bedside care and trials is necessary. Study of such personalized approaches will differ from standard clinical trials, as intervention for each patient will be based on their individual phenotypic signature identified from presenting and early-phase omics data. Finally, clinical end-users are critical to involve in the entire top-down process, as their real-world bedside experience in caring for such complex patients can provide crucial insight into what may or may not be feasible for personalized precision medicine in this population.
Conclusions
Current therapeutic treatment paradigms in moderate/severe TBI have failed to lead to substantial impacts on morbidity or mortality in the past three decades. Similarly, the ability to prognosticate in this population currently accounts for limited variance seen in outcomes. Recent expansions in phenotypic characterization of this population using current omics approaches have led to a rapid expansion to the data available to treating clinicians. The future of moderate/severe TBI care necessitates precision prognostication and therapeutics, tailored to the individual patient based on comprehensive physiome, advanced neuroimaging, proteome, and genome/epigenome. Such work will rely on integrative neuroinformatics platforms for precision prognostication and a “top-down” development of personalized therapeutics directed as secondary injury mechanisms driving morbidity and mortality.
Author Contributions
FZ, YI-M, and CA: responsible for concept, design, and manuscript preparation. ET, JS, JK, CF, and GW: undertook review and screening of the literature as well as aided in preparing the manuscript. AG: undertook review and screening of the literature as well as aided in preparing the manuscript and figures. All authors contributed to the article and approved the submitted version.
Funding
FZ receives research support from the Manitoba Public Insurance (MPI) Neuroscience/TBI Research Endowment, the Health Sciences Centre Foundation Winnipeg, the United States National Institutes of Health (NIH) through the National Institute of Neurological Disorders and Stroke (NINDS)(Grant #: R03NS114335-01), the Canadian Institutes of Health Research (CIHR)(Grant #: 432061), the Canada Foundation for Innovation (CFI)(Project #: 38583), Research Manitoba (Grant #: 3906), the University of Manitoba VPRI Research Investment Fund (RIF), the University of Manitoba Centre on Aging, and the University of Manitoba Rudy Falk Clinician-Scientist Professorship.
Conflict of Interest
The authors declare that the research was conducted in the absence of any commercial or financial relationships that could be construed as a potential conflict of interest.
Publisher's Note
All claims expressed in this article are solely those of the authors and do not necessarily represent those of their affiliated organizations, or those of the publisher, the editors and the reviewers. Any product that may be evaluated in this article, or claim that may be made by its manufacturer, is not guaranteed or endorsed by the publisher.
Acknowledgments
ET acknowledges funding from StratNeuro (Karolinska Institutet), The Erling-Persson Family Foundation, Region Stockholm (Clinical Research Appointment) and the Swedish Brain Foundation. CF acknowledges funding from the Canadian Natural Sciences and Engineering Research Council (NSERC), Research Manitoba, and Paul H.T. Thorlakson Foundation Fund. GW acknowledges funding from the Canada Research Chairs (CRC) Program (Tier 2 CRC in Neurogenomics) and the Health Sciences Centre Foundation Winnipeg. CA acknowledges funding from the Manitoba Research Chairs Program (Research Manitoba) and the Brain Canada Foundation.
References
1. Maas AIR, Menon DK, Adelson PD, Andelic N, Bell MJ, Belli A, et al. Traumatic brain injury: integrated approaches to improve prevention, clinical care, and research. Lancet Neurol. (2017) 16:987–1048. doi: 10.1016/S1474-4422(17)30371-X
2. Carney N, Totten AM, O'Reilly C, Ullman JS, Hawryluk GWJ, Bell MJ, et al. Guidelines for the management of severe traumatic brain injury, fourth edition. Neurosurgery. (2017) 80:6–15. doi: 10.1227/NEU.0000000000001432
3. Donnelly J, Czosnyka M, Adams H, Cardim D, Kolias AG, Zeiler FA, et al. Twenty-Five Years of Intracranial Pressure Monitoring After Severe Traumatic Brain Injury: A Retrospective, Single-Center Analysis. Neurosurgery. (2019) 85:E75–E82. doi: 10.1093/neuros/nyy468
4. Le Roux P, Menon DK, Citerio G, Vespa P, Bader MK, Brophy GM, et al. Consensus summary statement of the International Multidisciplinary Consensus Conference on Multimodality Monitoring in Neurocritical Care : a statement for healthcare professionals from the Neurocritical Care Society and the European Society of Intensive Care Medicine. Intensive Care Med. (2014) 40:1189–209. doi: 10.1007/s00134-014-3369-6
5. Depreitere B, Citerio G, Smith M, Adelson PD, Aries MJ, Bleck TP, et al. Cerebrovascular autoregulation monitoring in the management of adult severe traumatic brain injury: a delphi consensus of clinicians. Neurocrit Care. (2021) 34:731–8. doi: 10.1007/s12028-020-01185-x
6. Okonkwo DO, Shutter LA, Moore C, Temkin NR, Puccio AM, Madden CJ, et al. Brain oxygen optimization in severe traumatic brain injury Phase-II: a Phase II randomized trial. Crit. Care Med. (2017) 45:1907–14. doi: 10.1097/CCM.0000000000002619
7. Zeiler FA, Beqiri E, Cabeleira M, Hutchinson PJ, Stocchetti N, Menon DK, et al. Brain tissue oxygen cerebrovascular reactivity in traumatic brain injury: a collaborative european neurotrauma effectiveness research in traumatic brain injury exploratory analysis of insult burden. J Neurotrauma. (2020) 37:1854–63. doi: 10.1089/neu.2020.7024
8. Klein SP, Fieuws S, Meyfroidt G, Depreitere B. Effects of norepinephrine, propofol hemoglobin concentration on dynamic measurements of cerebrovascular reactivity in acute brain injury. J Neurotrauma. (2020) 38:506–12. doi: 10.1089/neu.2020.7160
9. Froese L, Dian J, Batson C, Gomez A, Unger B, Zeiler FA. The impact of hypertonic saline on cerebrovascular reactivity and compensatory reserve in traumatic brain injury: an exploratory analysis. Acta Neurochir. (2020) 162:2683–93. doi: 10.1007/s00701-020-04579-0
10. Froese L, Dian J, Batson C, Gomez A, Alarifi N, Unger B, et al. The impact of vasopressor and sedative agents on cerebrovascular reactivity and compensatory reserve in traumatic brain injury: an exploratory analysis. Neurotrauma Rep. (2020) 1:157–68. doi: 10.1089/neur.2020.0028
11. Froese L, Batson C, Gomez A, Dian J, Zeiler FA. The limited impact of current therapeutic interventions on cerebrovascular reactivity in traumatic brain injury: a narrative overview. Neurocrit Care. (2020) 34:325–35. doi: 10.1007/s12028-020-01003-4
12. Zeiler FA, Ercole A, Beqiri E, Cabeleira M, Aries M, Zoerle T, et al. Cerebrovascular reactivity is not associated with therapeutic intensity in adult traumatic brain injury: a CENTER-TBI analysis. Acta Neurochir. (2019) 161:1955–64. doi: 10.1007/s00701-019-03980-8
13. Steyerberg EW, Mushkudiani N, Perel P, Butcher I, Lu J, McHugh GS, et al. Predicting outcome after traumatic brain injury: development and international validation of prognostic scores based on admission characteristics. PLoS Med. (2008) 5:e165; discussion e165. doi: 10.1371/journal.pmed.0050165
14. Dijkland SA, Helmrich I.R.A.R., Nieboer D, van der Jagt M, Dippel DWJ, et al. Outcome prediction after moderate severe traumatic brain injury: external validation of two established prognostic models in 1742 European patients. J Neurotrauma. (2020) 38:1377–88. doi: 10.1089/neu.2020.7300
15. Richter S, Winzeck S, Kornaropoulos EN, Das T, Vande Vyvere T, Verheyden J, et al. Neuroanatomical substrates and symptoms associated with magnetic resonance imaging of patients with mild traumatic brain injury. JAMA Netw Open. (2021) 4:e210994. doi: 10.1001/jamanetworkopen.2021.0994
16. Monteiro M, Newcombe VFJ, Mathieu F, Adatia K, Kamnitsas K, Ferrante E, et al. Multiclass semantic segmentation and quantification of traumatic brain injury lesions on head CT using deep learning: an algorithm development and multicentre validation study. Lancet Digit Health. (2020) 2:e314–22. doi: 10.1016/S2589-7500(20)30085-6
17. Olsen A, Babikian T, Bigler ED, Caeyenberghs K, Conde V, Dams-O'Connor K, et al. Toward a global and reproducible science for brain imaging in neurotrauma: the ENIGMA adult moderate/severe traumatic brain injury working group. Brain Imaging Behav. (2021) 15:526–54. doi: 10.1007/s11682-020-00313-7
18. Launey Y, Fryer TD, Hong YT, Steiner LA, Nortje J, Veenith TV, et al. Spatial and temporal pattern of ischemia and abnormal vascular function following traumatic brain injury. JAMA Neurol. (2020) 77:339–49. doi: 10.1001/jamaneurol.2019.3854
19. Shankar JJS, Green R, Virani K, Wong H, Eddy K, Vandorpe R. Admission perfusion CT for classifying early in-hospital mortality of patients with severe traumatic brain injury: a pilot study. AJR Am J Roentgenol. (2020) 214:872–6. doi: 10.2214/AJR.19.21599
20. Timofeev I, Czosnyka M, Carpenter KLH, Nortje J, Kirkpatrick PJ, Al-Rawi PG, et al. Interaction between brain chemistry and physiology after traumatic brain injury: impact of autoregulation and microdialysis catheter location. J. Neurotrauma. (2011) 28:849–60. doi: 10.1089/neu.2010.1656
21. Timofeev I, Carpenter KLH, Nortje J, Al-Rawi PG, O'Connell MT, Czosnyka M, et al. Cerebral extracellular chemistry and outcome following traumatic brain injury: a microdialysis study of 223 patients. Brain J Neurol. (2011) 134:484–94. doi: 10.1093/brain/awq353
22. Thelin EP, Zeiler FA, Ercole A, Mondello S, Büki A, Bellander M, et al. Serial sampling of serum protein biomarkers for monitoring human traumatic brain injury dynamics: a systematic review. Front Neurol. (2017) 8:300. doi: 10.3389/fneur.2017.00300
23. Thelin EP, Tajsic T, Zeiler FA, Menon DK, Hutchinson PJA, Carpenter KLH, et al. Monitoring the neuroinflammatory response following acute brain injury. Front Neurol. (2017) 8:351. doi: 10.3389/fneur.2017.00351
24. Zeiler FA, Thelin EP, Czosnyka M, Hutchinson PJ, Menon DK, Helmy A. Cerebrospinal fluid and microdialysis cytokines in severe traumatic brain injury: a scoping systematic review. Front Neurol. (2017) 8:331. doi: 10.3389/fneur.2017.00331
25. Zeiler FA, Thelin EP, Donnelly J, Stevens AR, Smielewski P, Czosnyka M, et al. Genetic drivers of cerebral blood flow dysfunction in TBI: a speculative synthesis. Nat. Rev. Neurol. (2019) 15:25–9. doi: 10.1038/s41582-018-0105-9
26. Zeiler FA, McFadyen C, Newcombe VFJ, Synnot A, Donoghue EL, Ripatti S, et al. Genetic influences on patient-oriented outcomes in traumatic brain injury: a living systematic review of non-apolipoprotein e single-nucleotide polymorphisms. J Neurotrauma. (2021) 38:1107–23. doi: 10.1089/neu.2017.5583
27. Gomez A, Batson C, Froese L, Zeiler FA. Genetic variation and impact on outcome in traumatic brain injury: an overview of recent discoveries. Curr Neurol Neurosci Rep. (2021) 21:19. doi: 10.1007/s11910-021-01106-1
28. McFadyen CA, Zeiler FA, Newcombe V, Synnot A, Steyerberg E, Gruen RL, et al. Apolipoprotein E4 Polymorphism and outcomes from traumatic brain injury: a living systematic review and meta-analysis. J Neurotrauma. (2021) 38:1124–36. doi: 10.1089/neu.2018.6052
29. Iturria-Medina Y, Carbonell FM, Sotero RC, Chouinard-Decorte F, Evans AC, Alzheimer's Disease Neuroimaging Initiative. Multifactorial causal model of brain (dis)organization and therapeutic intervention: application to Alzheimer's disease. NeuroImage. (2017) 152:60–77. doi: 10.1016/j.neuroimage.2017.02.058
30. Sanchez-Rodriguez LM, Iturria-Medina Y, Mouches P, Sotero RC. Detecting brain network communities: considering the role of information flow and its different temporal scales. NeuroImage. (2021) 225:117431. doi: 10.1016/j.neuroimage.2020.117431
31. Shu N, Iturria-Medina Y, Sotero RC. From micro- to macroscopic brain connectivity using multiple modalities. BioMed Res Int. (2016) 2016:8128095. doi: 10.1155/2016/8128095
32. Zeiler FA, Unger B, West M, Kazina CJ, Berrington N, Ellis MJ. Manitoba cranial neurotrauma research – past, present and future. J Neurotrauma. (2018) 35:1999–2001.
33. Bernard F, Gallagher C, Griesdale D, Kramer A, Sekhon M, Zeiler FA. The Canadian High-Resolution Traumatic Brain Injury (CAHR-TBI) research collaborative. Can J Neurol Sci. (2020) 47:551–6. doi: 10.1017/cjn.2020.54
34. Froese L, Dian J, Gomez A, Zeiler FA. Sedation and cerebrovascular reactivity in traumatic brain injury: another potential avenue for personalized approaches in neurocritical care? Acta Neurochir. (2021) 163:1383–9. doi: 10.1007/s00701-020-04662-6
35. Thelin EP, Raj R, Bellander B.-M., Nelson D, Piippo-Karjalainen A, et al. Comparison of high versus low frequency cerebral physiology for cerebrovascular reactivity assessment in traumatic brain injury: a multi-center pilot study. J Clin Monit Comput. (2019) 34:971–94. doi: 10.1007/s10877-019-00392-y
36. Mathieu F, Khellaf A, Ku JC, Donnelly J, Thelin EP, Zeiler FA. Continuous near-infrared spectroscopy monitoring in adult traumatic brain injury: a systematic review. J Neurosurg Anesthesiol. (2020) 32:288–99. doi: 10.1097/ANA.0000000000000620
37. Hawryluk GWJ, Phan N, Ferguson AR, Morabito D, Derugin N, Stewart CL, et al. Brain tissue oxygen tension and its response to physiological manipulations: influence of distance from injury site in a swine model of traumatic brain injury. J Neurosurg. (2016) 125:1217–28. doi: 10.3171/2015.7.JNS15809
38. Rosenthal G, Hemphill JC, Sorani M, Martin C, Morabito D, Obrist WD, et al. Brain tissue oxygen tension is more indicative of oxygen diffusion than oxygen delivery and metabolism in patients with traumatic brain injury. Crit Care Med. (2008) 36:1917–24. doi: 10.1097/CCM.0b013e3181743d77
39. Mathieu F, Khellaf A, Thelin EP, Zeiler FA. Continuous thermal diffusion-based cerebral blood flow monitoring in adult traumatic brain injury: a scoping systematic review. J Neurotrauma. (2019) 36:1707–23. doi: 10.1089/neu.2018.6309
40. Czosnyka M, Matta BF, Smielewski P, Kirkpatrick PJ, Pickard JD. Cerebral perfusion pressure in head-injured patients: a noninvasive assessment using transcranial Doppler ultrasonography. J Neurosurg. (1998) 88:802–8. doi: 10.3171/jns.1998.88.5.0802
41. Robba C, Cardim D, Sekhon M, Budohoski K, Czosnyka M. Transcranial Doppler: a stethoscope for the brain-neurocritical care use. J Neurosci Res. (2018) 96:720–30. doi: 10.1002/jnr.24148
42. Gomez A, Batson C, Froese L, Sainbhi AS, Zeiler FA. Utility of transcranial Doppler in moderate severe traumatic brain injury: a narrative review of cerebral physiologic metrics. J Neurotrauma. (2021) 38:2206–20. doi: 10.1089/neu.2020.7523
43. Haveman ME, Van Putten M.J.A.M., Hom HW, Eertman-Meyer CJ, Beishuizen A, Tjepkema-Cloostermans MC. Predicting outcome in patients with moderate to severe traumatic brain injury using electroencephalography. Crit Care Lond Engl. (2019) 23:401. doi: 10.1186/s13054-019-2656-6
44. Soldozy S, Sharifi KA, Desai B, Giraldo D, Yeghyayan M, Liu L, et al. Cortical spreading depression in the setting of traumatic brain injury. World Neurosurg. (2020) 134:50–7. doi: 10.1016/j.wneu.2019.10.048
45. Toth P, Szarka N, Farkas E, Ezer E, Czeiter E, Amrein K, et al. Traumatic brain injury-induced autoregulatory dysfunction and spreading depression-related neurovascular uncoupling: pathomechanisms, perspectives, therapeutic implications. Am J Physiol Heart Circ Physiol. (2016) 311:H1118–31. doi: 10.1152/ajpheart.00267.2016
46. Zeiler FA, Donnelly J, Calviello L, Smielewski P, Menon DK, Czosnyka M. Pressure autoregulation measurement techniques in adult traumatic brain injury, part ii: a scoping review of continuous methods. J Neurotrauma. (2017) 34:3224–37. doi: 10.1089/neu.2017.5086
47. Czosnyka M, Smielewski P, Kirkpatrick P, Laing RJ, Menon D, Pickard JD. Continuous assessment of the cerebral vasomotor reactivity in head injury. Neurosurgery. (1997) 41:11–7; discussion 17–19. doi: 10.1097/00006123-199707000-00005
48. Zeiler FA, Ercole A, Beqiri E, Cabeleira M, Thelin EP, Stocchetti N, et al. Association between cerebrovascular reactivity monitoring mortality is preserved when adjusting for baseline admission characteristics in Adult TBI: a CENTER-TBI study. J Neurotrauma. (2019) 37:1233–41. doi: 10.1089/neu.2019.6808
49. Bennis FC, Teeuwen B, Zeiler FA, Elting JW, van der Naalt J, Bonizzi P, et al. Improving prediction of favourable outcome after 6 months in patients with severe traumatic brain injury using physiological cerebral parameters in a multivariable logistic regression model. Neurocrit Care. (2020) 33:542–51. doi: 10.1007/s12028-020-00930-6
50. Hawryluk GWJ, Aguilera S, Buki A, Bulger E, Citerio G, Cooper DJ, et al. A management algorithm for patients with intracranial pressure monitoring: the Seattle International Severe Traumatic Brain Injury Consensus Conference (SIBICC). Intensive Care Med. (2019) 45:1783–94. doi: 10.1007/s00134-019-05805-9
51. Czosnyka M, Miller C, Participants in the International Multidisciplinary Consensus Conference on Multimodality Monitoring. Monitoring of cerebral autoregulation. Neurocrit Care. (2014) 21(Suppl. 2):S95–102. doi: 10.1007/s12028-014-0046-0
52. Hasen M, Almojuela A, Zeiler FA. Autonomic dysfunction and associations with functional and neurophysiological outcome in moderate/severe traumatic brain injury: a scoping review. J Neurotrauma. (2019) 36:1491–504. doi: 10.1089/neu.2018.6073
53. Zeiler FA, Donnelly J, Calviello L, Menon DK, Smielewski P, Czosnyka M. Pressure autoregulation measurement techniques in adult traumatic brain injury, Part I: a scoping review of intermittent/semi-intermittent methods. J Neurotrauma. (2017) 34:3207–23. doi: 10.1089/neu.2017.5085
54. Calviello L, Donnelly J, Cardim D, Robba C, Zeiler FA, Smielewski P, et al. Compensatory-reserve-weighted intracranial pressure and its association with outcome after traumatic brain injury. Neurocrit Care. (2018) 28:212–20. doi: 10.1007/s12028-017-0475-7
55. Zeiler FA, Ercole A, Cabeleira M, Beqiri E, Zoerle T, Carbonara M, et al. Compensatory-reserve-weighted intracranial pressure versus intracranial pressure for outcome association in adult traumatic brain injury: a CENTER-TBI validation study. Acta Neurochir. (2019) 161:1275–84. doi: 10.1007/s00701-019-03915-3
56. Zeiler FA, Ercole A, Placek MM, Hutchinson PJ, Stocchetti N, Czosnyka M, et al. Association between physiological signal complexity outcomes in moderate severe traumatic brain injury: a CENTER-TBI exploratory analysis of multi-scale entropy. J Neurotrauma. (2020) 38:272–82. doi: 10.1089/neu.2020.7249
57. Lu CW, Czosnyka M, Shieh JS, Smielewska A, Pickard JD, Smielewski P. Complexity of intracranial pressure correlates with outcome after traumatic brain injury. Brain J Neurol. (2012) 135:2399–408. doi: 10.1093/brain/aws155
58. Gao L, Smielewski P, Czosnyka M, Ercole A. Cerebrovascular signal complexity six hours after intensive care unit admission correlates with outcome after severe traumatic brain injury. J Neurotrauma. (2016) 33:2011–8. doi: 10.1089/neu.2015.4228
59. Zeiler FA, Donnelly J, Smielewski P, Menon DK, Hutchinson PJ, Czosnyka M. Critical thresholds of intracranial pressure-derived continuous cerebrovascular reactivity indices for outcome prediction in noncraniectomized patients with traumatic brain injury. J Neurotrauma. (2018) 35:1107–15. doi: 10.1089/neu.2017.5472
60. Steiner LA, Czosnyka M, Piechnik SK, Smielewski P, Chatfield D, Menon DK, et al. Continuous monitoring of cerebrovascular pressure reactivity allows determination of optimal cerebral perfusion pressure in patients with traumatic brain injury. Crit Care Med. (2002) 30:733–8. doi: 10.1097/00003246-200204000-00002
61. Aries MJH, Czosnyka M, Budohoski KP, Steiner LA, Lavinio A, Kolias AG, et al. Continuous determination of optimal cerebral perfusion pressure in traumatic brain injury. Crit Care Med. (2012) 40:2456–63. doi: 10.1097/CCM.0b013e3182514eb6
62. Beqiri E, Smielewski P, Robba C, Czosnyka M, Cabeleira MT, Tas J, et al. Feasibility of individualised severe traumatic brain injury management using an automated assessment of optimal cerebral perfusion pressure: the COGiTATE phase II study protocol. BMJ Open. (2019) 9:e030727. doi: 10.1136/bmjopen-2019-030727
63. Zeiler FA, Ercole A, Cabeleira M, Carbonara M, Stocchetti N, Menon DK, et al. Comparison of performance of different optimal cerebral perfusion pressure parameters for outcome prediction in adult traumatic brain injury: a Collaborative European NeuroTrauma Effectiveness Research in Traumatic Brain Injury (CENTER-TBI) Study. J Neurotrauma. (2019) 36:1505–17. doi: 10.1089/neu.2018.6182
64. Donnelly J, Czosnyka M, Adams H, Robba C, Steiner LA, Cardim D, et al. Individualizing thresholds of cerebral perfusion pressure using estimated limits of autoregulation. Crit Care Med. (2017) 45:1464–71. doi: 10.1097/CCM.0000000000002575
65. Lazaridis C, DeSantis SM, Smielewski P, Menon DK, Hutchinson P, Pickard JD, et al. Patient-specific thresholds of intracranial pressure in severe traumatic brain injury. J. Neurosurg. (2014) 120:893–900. doi: 10.3171/2014.1.JNS131292
66. Zeiler FA, Ercole A, Cabeleira M, Beqiri E, Zoerle T, Carbonara M, et al. Patient-specific ICP epidemiologic thresholds in adult traumatic brain injury: a CENTER-TBI validation study. J Neurosurg Anesthesiol. (2021) 33:28–38. doi: 10.1097/ANA.0000000000000616
67. Needham E, McFadyen C, Newcombe V, Synnot AJ, Czosnyka M, Menon D. Cerebral perfusion pressure targets individualized to pressure-reactivity index in moderate to severe traumatic brain injury: a systematic review. J Neurotrauma. (2017) 34:963–70. doi: 10.1089/neu.2016.4450
68. Zeiler FA, Donnelly J, Menon DK, Smielewski P, Zweifel C, Brady K, et al. Continuous autoregulatory indices derived from multi-modal monitoring: each one is not like the other. J Neurotrauma. (2017) 34:3070–80. doi: 10.1089/neu.2017.5129
69. Zeiler FA, Smielewski P, Stevens A, Czosnyka M, Menon DK, Ercole A. Non-invasive pressure reactivity index using doppler systolic flow parameters: a pilot analysis. J Neurotrauma. (2019) 36:713–20. doi: 10.1089/neu.2018.5987
70. Zeiler FA, Thelin EP, Helmy A, Czosnyka M, Hutchinson PJA, Menon DK. A systematic review of cerebral microdialysis and outcomes in TBI: relationships to patient functional outcome, neurophysiologic measures, tissue outcome. Acta Neurochir. (2017) 159:2245–73. doi: 10.1007/s00701-017-3338-2
71. Mathieu F, Zeiler FA, Whitehouse DP, Das T, Ercole A, Smielewski P, et al. Relationship between measures of cerebrovascular reactivity and intracranial lesion progression in acute TBI patients: an exploratory analysis. Neurocrit Care. (2019) 32:373–82. doi: 10.1007/s12028-019-00885-3
72. Mathieu F, Zeiler FA, Ercole A, Monteiro MAB, Kamnitsas K, Glocker B, et al. Relationship between measures of cerebrovascular reactivity intracranial lesion progression in acute TBI patients: a CENTER-TBI study. J Neurotrauma. (2020) 37:1556–65. doi: 10.1089/neu.2019.6814
73. Zeiler FA, Donnelly J, Nourallah B, Thelin EP, Calviello L, Smielewski P, et al. Intracranial and extracranial injury burden as drivers of impaired cerebrovascular reactivity in traumatic brain injury. J Neurotrauma. (2018) 35:1569–77. doi: 10.1089/neu.2017.5595
74. MacDonald D, Stewart-Perrin B, Shankar JJS. The role of neuroimaging in the determination of brain death. J Neuroimaging. (2018) 28:374–9. doi: 10.1111/jon.12516
75. Shankar JJS, Banfield JC. Comments on Shemie et al. : international guideline development for the determination of death. Intensive Care Med. (2015) 41:571. doi: 10.1007/s00134-015-3679-3
76. Shankar JJS, Vandorpe R. CT perfusion for confirmation of brain death. AJNR Am J Neuroradiol. (2013) 34:1175–9. doi: 10.3174/ajnr.A3376
77. Shankar JJS, Stewart-Perrin B, Quraishi AUR, Bata I, Vandorpe R. Computed tomography perfusion aids in the prognostication of comatose postcardiac arrest patients. Am J Cardiol. (2018) 121:874–8. doi: 10.1016/j.amjcard.2017.12.027
78. Andreasen SH, Andersen KW, Conde V, Dyrby TB, Puonti O, Kammersgaard LP, et al. Limited colocalization of microbleeds and microstructural changes after severe traumatic brain injury. J Neurotrauma. (2020) 37:581–92. doi: 10.1089/neu.2019.6608
79. Wallace EJ, Mathias JL, Ward L. Diffusion tensor imaging changes following mild, moderate and severe adult traumatic brain injury: a meta-analysis. Brain Imaging Behav. (2018) 12:1607–21. doi: 10.1007/s11682-018-9823-2
80. Wallace EJ, Mathias JL, Ward L. The relationship between diffusion tensor imaging findings and cognitive outcomes following adult traumatic brain injury: a meta-analysis. Neurosci Biobehav Rev. (2018) 92:93–103. doi: 10.1016/j.neubiorev.2018.05.023
81. Jolly AE, Bălăet M, Azor A, Friedland D, Sandrone S, Graham NSN, et al. Detecting axonal injury in individual patients after traumatic brain injury. Brain J Neurol. (2021) 144:92–113. doi: 10.1093/brain/awaa372
82. Byrnes KR, Wilson CM, Brabazon F, von Leden R, Jurgens JS, Oakes TR, et al. FDG-PET imaging in mild traumatic brain injury: a critical review. Front Neuroenergetics. (2014) 5:13. doi: 10.3389/fnene.2013.00013
83. Yamaki T, Imahori Y, Ohmori Y, Yoshino E, Hohri T, Ebisu T, et al. Cerebral hemodynamics and metabolism of severe diffuse brain injury measured by PET. J Nucl Med. (1996) 37:1166–70.
84. Worley G, Hoffman JM, Paine SS, Kalman SL, Claerhout SJ, Boyko OB, et al. 18-Fluorodeoxyglucose positron emission tomography in children and adolescents with traumatic brain injury. Dev Med Child Neurol. (1995) 37:213–20. doi: 10.1111/j.1469-8749.1995.tb11994.x
85. Gorgoraptis N, Li LM, Whittington A, Zimmerman KA, Maclean LM, McLeod C, et al. In vivo detection of cerebral tau pathology in long-term survivors of traumatic brain injury. Sci Transl Med. (2019) 11:eaaw1993. doi: 10.1126/scitranslmed.aaw1993
86. Robinson ME, McKee AC, Salat DH, Rasmusson AM, Radigan LJ, Catana C, et al. Positron emission tomography of tau in Iraq and Afghanistan Veterans with blast neurotrauma. NeuroImage Clin. (2019) 21:101651. doi: 10.1016/j.nicl.2019.101651
87. Leuzy A, Chiotis K, Lemoine L, Gillberg P.-G., Almkvist O, Rodriguez-Vieitez E, et al. Tau PET imaging in neurodegenerative tauopathies-still a challenge. Mol Psychiatry. (2019) 24:1112–34. doi: 10.1038/s41380-018-0342-8
88. Coughlin JM, Wang Y, Munro CA, Ma S, Yue C, Chen S, et al. Neuroinflammation and brain atrophy in former NFL players: an in vivo multimodal imaging pilot study. Neurobiol Dis. (2015) 74:58–65. doi: 10.1016/j.nbd.2014.10.019
89. Best L, Ghadery C, Pavese N, Tai YF, Strafella AP. New and Old TSPO PET radioligands for imaging brain microglial activation in neurodegenerative disease. Curr Neurol Neurosci Rep. (2019) 19:24. doi: 10.1007/s11910-019-0934-y
90. Owen DR, Yeo AJ, Gunn RN, Song K, Wadsworth G, Lewis A, et al. An 18-kDa translocator protein (TSPO) polymorphism explains differences in binding affinity of the PET radioligand PBR28. J Cereb Blood Flow Metab. (2012) 32:1–5. doi: 10.1038/jcbfm.2011.147
91. Figley CR, Leitch JK, Stroman PW. In contrast to BOLD: signal enhancement by extravascular water protons as an alternative mechanism of endogenous fMRI signal change. Magn Reson Imaging. (2010) 28:1234–43. doi: 10.1016/j.mri.2010.01.005
92. Raichle ME, Mintun MA. Brain work and brain imaging. Annu Rev Neurosci. (2006) 29:449–76. doi: 10.1146/annurev.neuro.29.051605.112819
93. Figley CR, Stroman PW. The role(s) of astrocytes and astrocyte activity in neurometabolism, neurovascular coupling, and the production of functional neuroimaging signals. Eur J Neurosci. (2011) 33:577–88. doi: 10.1111/j.1460-9568.2010.07584.x
94. Biswal BB, Van Kylen J, Hyde JS. Simultaneous assessment of flow and BOLD signals in resting-state functional connectivity maps. NMR Biomed. (1997) 10:165–70. doi: 10.1002/(SICI)1099-1492(199706/08)10:4/5<165::AID-NBM454>3.0.CO;2-7
95. Damoiseaux JS, Rombouts SA, Barkhof F, Scheltens P, Stam CJ, Smith SM, et al. Consistent resting-state networks across healthy subjects. Proc Natl Acad Sci USA. (2006) 103:13848–53. doi: 10.1073/pnas.0601417103
96. Lancaster K, Venkatesan UM, Lengenfelder J, Genova HM. Default mode network connectivity predicts emotion recognition and social integration after traumatic brain injury. Front Neurol. (2019) 10:825. doi: 10.3389/fneur.2019.00825
97. Threlkeld ZD, Bodien YG, Rosenthal ES, Giacino JT, Nieto-Castanon A, Wu O, et al. Functional networks reemerge during recovery of consciousness after acute severe traumatic brain injury. Cortex J Devoted Study Nerv Syst Behav. (2018) 106:299–308. doi: 10.1016/j.cortex.2018.05.004
98. Grossner EC, Bernier RA, Brenner EK, Chiou KS, Hong J, Hillary FG. Enhanced default mode connectivity predicts metacognitive accuracy in traumatic brain injury. Neuropsychology. (2019) 33:922–33. doi: 10.1037/neu0000559
99. Han K, Chapman SB, Krawczyk DC. Disrupted intrinsic connectivity among default, dorsal attention, and frontoparietal control networks in individuals with chronic traumatic brain injury. J Int Neuropsychol Soc. (2016) 22:263–79. doi: 10.1017/S1355617715001393
100. Puig J, Ellis MJ, Kornelsen J, Figley TD, Figley CR, Daunis-I-Estadella P, et al. Magnetic resonance imaging biomarkers of brain connectivity in predicting outcome after mild traumatic brain injury: a systematic review. J Neurotrauma. (2020) 37:1761–76. doi: 10.1089/neu.2019.6623
101. Fernández-Espejo D, Soddu A, Cruse D, Palacios EM, Junque C, Vanhaudenhuyse A, et al. A role for the default mode network in the bases of disorders of consciousness. Ann Neurol. (2012) 72:335–43. doi: 10.1002/ana.23635
102. Bodien YG, Chatelle C, Edlow BL. Functional networks in disorders of consciousness. Semin Neurol. (2017) 37:485–502. doi: 10.1055/s-0037-1607310
103. Raichle ME. The brain's default mode network. Annu Rev Neurosci. (2015) 38:433–47. doi: 10.1146/annurev-neuro-071013-014030
104. Irimia A, Maher AS, Chaudhari NN, Chowdhury NF, Jacobs EB, Alzheimer's Disease Neuroimaging Initiative. Acute cognitive deficits after traumatic brain injury predict Alzheimer's disease-like degradation of the human default mode network. GeroScience. (2020) 42:1411–29. doi: 10.1007/s11357-020-00245-6
105. Mitra J, Shen KK, Ghose S, Bourgeat P, Fripp J, Salvado O, et al. Statistical machine learning to identify traumatic brain injury (TBI) from structural disconnections of white matter networks. NeuroImage. (2016) 129:247–59. doi: 10.1016/j.neuroimage.2016.01.056
106. Vergara VM, Mayer AR, Damaraju E, Kiehl KA, Calhoun V. Detection of mild traumatic brain injury by machine learning classification using resting state functional network connectivity and fractional anisotropy. J Neurotrauma. (2017) 34:1045–53. doi: 10.1089/neu.2016.4526
107. Amorim RL, Oliveira LM, Malbouisson LM, Nagumo MM, Simoes M, Miranda L, et al. Prediction of early TBI mortality using a machine learning approach in a LMIC population. Front Neurol. (2019) 10:1366. doi: 10.3389/fneur.2019.01366
108. Gravesteijn BY, Nieboer D, Ercole A, Lingsma HF, Nelson D, van Calster B, et al. Machine learning algorithms performed no better than regression models for prognostication in traumatic brain injury. J Clin Epidemiol. (2020) 122:95–107. doi: 10.1016/j.jclinepi.2020.03.005
109. Zetterberg H, Smith DH, Blennow K. Biomarkers of mild traumatic brain injury in cerebrospinal fluid and blood. Nat Rev Neurol. (2013) 9:201–10. doi: 10.1038/nrneurol.2013.9
110. Thelin E, Al Nimer F, Frostell A, Zetterberg H, Blennow K, Nyström HM, et al. A serum protein biomarker panel improves outcome prediction in human traumatic brain injury. J Neurotrauma. (2019) 36:2850–62. doi: 10.1089/neu.2019.6375
111. Undén J, Ingebrigtsen T, Romner B, Scandinavian Neurotrauma Committee (SNC). Scandinavian guidelines for initial management of minimal, mild and moderate head injuries in adults: an evidence and consensus-based update. BMC Med. (2013) 11:50. doi: 10.1186/1741-7015-11-50
112. Thelin EP, Johannesson L, Nelson D, Bellander B.-M. S100B is an important outcome predictor in traumatic brain injury. J Neurotrauma. (2013) 30:519–28. doi: 10.1089/neu.2012.2553
113. Thelin EP, Nelson DW, Bellander B-M. Secondary peaks of S100B in serum relate to subsequent radiological pathology in traumatic brain injury. Neurocrit Care. (2014) 20:217–29. doi: 10.1007/s12028-013-9916-0
114. Okonkwo DO, Puffer RC, Puccio AM, Yuh EL, Yue JK, Diaz-Arrastia R, et al. Point-of-care platform blood biomarker testing of glial fibrillary acidic protein versus S100 calcium-binding protein b for prediction of traumatic brain injuries: a transforming research clinical knowledge in traumatic brain injury study. J Neurotrauma. (2020) 37:2460–7. doi: 10.1089/neu.2020.7140
115. Nolan JP, Soar J, Cariou A, Cronberg T, Moulaert VRM, Deakin CD, et al. European resuscitation council and European society of intensive care medicine 2015 guidelines for post-resuscitation care. Intensive Care Med. (2015) 41:2039–56. doi: 10.1007/s00134-015-4051-3
116. Thompson WH, Thelin EP, Lilja A, Bellander B.-M., Fransson P. Functional resting-state fMRI connectivity correlates with serum levels of the S100B protein in the acute phase of traumatic brain injury. NeuroImage Clin. (2016) 12:1004–12. doi: 10.1016/j.nicl.2016.05.005
117. Sjödin MOD, Bergquist J, Wetterhall M. Mining ventricular cerebrospinal fluid from patients with traumatic brain injury using hexapeptide ligand libraries to search for trauma biomarkers. J Chromatogr B Analyt Technol Biomed Life Sci. (2010) 878:2003–12. doi: 10.1016/j.jchromb.2010.05.036
118. Hanrieder J, Wetterhall M, Enblad P, Hillered L, Bergquist J. Temporally resolved differential proteomic analysis of human ventricular CSF for monitoring traumatic brain injury biomarker candidates. J Neurosci Methods. (2009) 177:469–78. doi: 10.1016/j.jneumeth.2008.10.038
119. Connor DE, Chaitanya GV, Chittiboina P, McCarthy P, Scott LK, Schrott L, et al. Variations in the cerebrospinal fluid proteome following traumatic brain injury and subarachnoid hemorrhage. Pathophysiology. (2017) 24:169–83. doi: 10.1016/j.pathophys.2017.04.003
120. Conti A, Sanchez-Ruiz Y, Bachi A, Beretta L, Grandi E, Beltramo M, et al. Proteome study of human cerebrospinal fluid following traumatic brain injury indicates fibrin(ogen) degradation products as trauma-associated markers. J Neurotrauma. (2004) 21:854–63. doi: 10.1089/0897715041526212
121. Xu B, Tian R, Wang X, Zhan S, Wang R, Guo Y, et al. Protein profile changes in the frontotemporal lobes in human severe traumatic brain injury. Brain Res. (2016) 1642:344–52. doi: 10.1016/j.brainres.2016.04.008
122. Dyhrfort P, Shen Q, Clausen F, Thulin M, Enblad P, Kamali-Moghaddam M, et al. Monitoring of protein biomarkers of inflammation in human traumatic brain injury using microdialysis and proximity extension assay technology in neurointensive care. J Neurotrauma. (2019) 36:2872–85. doi: 10.1089/neu.2018.6320
123. Helmy A, De Simoni M.-G., Guilfoyle MR, Carpenter KLH, Hutchinson PJ. Cytokines and innate inflammation in the pathogenesis of human traumatic brain injury. Prog Neurobiol. (2011) 95:352–72. doi: 10.1016/j.pneurobio.2011.09.003
124. Helmy A, Antoniades CA, Guilfoyle MR, Carpenter KLH, Hutchinson PJ. Principal component analysis of the cytokine and chemokine response to human traumatic brain injury. PLoS ONE. (2012) 7:e39677. doi: 10.1371/journal.pone.0039677
125. Alam A, Thelin EP, Tajsic T, Khan DZ, Khellaf A, Patani R, et al. Cellular infiltration in traumatic brain injury. J Neuroinflammation. (2020) 17:328. doi: 10.1186/s12974-020-02005-x
126. Lindblad C, Nelson DW, Zeiler FA, Ercole A, Ghatan PH, von Horn H, et al. Influence of blood-brain barrier integrity on brain protein biomarker clearance in severe traumatic brain injury: a longitudinal prospective study. J Neurotrauma. (2020) 37:1381–91. doi: 10.1089/neu.2019.6741
127. Lindblad C, Pin E, Just D, Al Nimer F, Nilsson P, Bellander M, et al. Fluid proteomics of CSF and serum reveal important neuroinflammatory proteins in blood-brain barrier disruption and outcome prediction following severe traumatic brain injury: a prospective, observational study. Crit Care Lond. Engl. (2021) 25:103. doi: 10.1186/s13054-021-03503-x
128. Hiebert JB, Shen Q, Thimmesch AR, Pierce JD. Traumatic brain injury and mitochondrial dysfunction. Am J Med Sci. (2015) 350:132–38. doi: 10.1097/MAJ.0000000000000506
129. Vink R, Head VA, Rogers PJ, McIntosh TK, Faden AI. Mitochondrial metabolism following traumatic brain injury in rats. J Neurotrauma. (1990) 7:21–7. doi: 10.1089/neu.1990.7.21
130. Singh IN, Sullivan PG, Deng Y, Mbye LH, Hall ED. Time course of post-traumatic mitochondrial oxidative damage and dysfunction in a mouse model of focal traumatic brain injury: implications for neuroprotective therapy. J Cereb Blood Flow Metab. (2006) 26:1407–18. doi: 10.1038/sj.jcbfm.9600297
131. Redmann M, Benavides GA, Wani WY, Berryhill TF, Ouyang X, Johnson MS, et al. Methods for assessing mitochondrial quality control mechanisms and cellular consequences in cell culture. Redox Biol. (2018) 17:59–69. doi: 10.1016/j.redox.2018.04.005
132. Hutchinson PJ, Jalloh I, Helmy A, Carpenter KLH, Rostami E, Bellander M, et al. Consensus statement from the 2014 International Microdialysis Forum. Intensive Care Med. (2015) 41:1517–28. doi: 10.1007/s00134-015-3930-y
133. Nordström CH, Koskinen LO, Olivecrona M. Aspects on the physiological and biochemical foundations of neurocritical care. Front Neurol. (2017) 8:274. doi: 10.3389/fneur.2017.00274
134. Stovell MG, Mada MO, Helmy A, Carpenter TA, Thelin EP, Yan L, et al. The effect of succinate on brain NADH/NAD+ redox state and high energy phosphate metabolism in acute traumatic brain injury. Sci Rep. (2018) 8:11140. doi: 10.1038/s41598-018-29255-3
135. Nielsen TH, Olsen NV, Toft P, Nordström CH. Cerebral energy metabolism during mitochondrial dysfunction induced by cyanide in piglets. Acta Anaesthesiol Scand. (2013) 57:793–801. doi: 10.1111/aas.12092
136. Parikh S, Goldstein A, Koenig MK, Scaglia F, Enns GM, Saneto R, et al. Diagnosis and management of mitochondrial disease: a consensus statement from the Mitochondrial Medicine Society. Genet Med. (2015) 17:689–701. doi: 10.1038/gim.2014.177
137. Toczyłowska B, Chalimoniuk M, Wodowska M, Mayzner-Zawadzk E. Changes in concentration of cerebrospinal fluid components in patients with traumatic brain injury. Brain Res. (2006) 1104:183–9. doi: 10.1016/j.brainres.2006.05.057
138. Thelin EP, Nelson DW, Ghatan PH, Bellander BM. Microdialysis monitoring of CSF parameters in severe traumatic brain injury patients: a novel approach. Front Neurol. (2014) 5:159. doi: 10.3389/fneur.2014.00159
139. Thelin EP, Carpenter KLH, Hutchinson PJ, Helmy A. Microdialysis monitoring in clinical traumatic brain injury and its role in neuroprotective drug development. AAPS J. (2017) 19:367–76. doi: 10.1208/s12248-016-0027-7
140. Verghese PB, Castellano JM, Holtzman DM. Apolipoprotein E in Alzheimer's disease and other neurological disorders. Lancet Neurol. (2011) 10:241–52. doi: 10.1016/S1474-4422(10)70325-2
141. Teasdale GM, Nicoll JA, Murray G, Fiddes M. Association of apolipoprotein E polymorphism with outcome after head injury. Lancet Lond Engl. (1997) 350:1069–71. doi: 10.1016/S0140-6736(97)04318-3
142. Zhou W, Xu D, Peng X, Zhang Q, Jia J, Crutcher KA. Meta-analysis of APOE4 allele and outcome after traumatic brain injury. J Neurotrauma. (2008) 25:279–90. doi: 10.1089/neu.2007.0489
143. Zeng S, Jiang JX, Xu MH, Xu S, Shen GJ, Zhang AQ, et al. Prognostic value of apolipoprotein E epsilon4 allele in patients with traumatic brain injury: a meta-analysis and meta-regression. Genet Test Mol Biomark. (2014) 18:202–10. doi: 10.1089/gtmb.2013.0421
144. Yue JK, Robinson CK, Burke JF, Winkler EA, Deng H, Cnossen MC, et al. Apolipoprotein E epsilon 4 (APOE-ε4) genotype is associated with decreased 6-month verbal memory performance after mild traumatic brain injury. Brain Behav. (2017) 7:e00791. doi: 10.1002/brb3.791
145. Merritt VC, Clark AL, Sorg SF, Evangelista ND, Werhane M, Bondi MW, et al. Apolipoprotein E ε4 genotype is associated with elevated psychiatric distress in veterans with a history of mild to moderate traumatic brain injury. J Neurotrauma. (2018) 35:2272–82. doi: 10.1089/neu.2017.5372
146. Merritt VC, Clark AL, Sorg SF, Evangelista ND, Werhane ML, Bondi MW, et al. Apolipoprotein E (APOE) ε4 genotype is associated with reduced neuropsychological performance in military veterans with a history of mild traumatic brain injury. J Clin Exp Neuropsychol. (2018) 40:1050–61. doi: 10.1080/13803395.2018.1508555
147. Padgett CR, Summers MJ, Vickers JC, McCormack GH, Skilbeck CE. Exploring the effect of the apolipoprotein E (APOE) gene on executive function, working memory, and processing speed during the early recovery period following traumatic brain injury. J Clin Exp Neuropsychol. (2016) 38:551–60. doi: 10.1080/13803395.2015.1137557
148. Banks SJ, Miller JB, Rissman RA, Bernick CB. Lack of influence of apolipoprotein e status on cognition or brain structure in professional fighters. J Neurotrauma. (2017) 34:380–4. doi: 10.1089/neu.2016.4453
149. Merritt VC, Rabinowitz AR, Arnett PA. The influence of the Apolipoprotein E (APOE) gene on subacute post-concussion neurocognitive performance in college athletes. Arc. Clin Neuropsychol. (2018) 33:36–46. doi: 10.1093/arclin/acx051
150. Failla MD, Kumar RG, Peitzman AB, Conley YP, Ferrell RE, Wagner AK. Variation in the BDNF gene interacts with age to predict mortality in a prospective, longitudinal cohort with severe TBI. Neurorehabil Neural Repair. (2015) 29:234–46. doi: 10.1177/1545968314542617
151. Failla MD, Conley YP, Wagner AK. Brain-Derived Neurotrophic Factor (BDNF) in traumatic brain injury-related mortality: interrelationships between genetics and acute systemic and central nervous system BDNF profiles. Neurorehabil Neural Repair. (2016) 30:83–93. doi: 10.1177/1545968315586465
152. Krueger F, Pardini M, Huey ED, Raymont V, Solomon J, Lipsky RH, et al. The role of the Met66 brain-derived neurotrophic factor allele in the recovery of executive functioning after combat-related traumatic brain injury. J Neurosci. (2011) 31:598–606. doi: 10.1523/JNEUROSCI.1399-10.2011
153. McAllister TW, Tyler AL, Flashman LA, Rhodes CH, McDonald BC, Saykin AJ, et al. Polymorphisms in the brain-derived neurotrophic factor gene influence memory and processing speed one month after brain injury. J Neurotrauma. (2012) 29:1111–8. doi: 10.1089/neu.2011.1930
154. Barbey AK, Colom R, Paul E, Forbes C, Krueger F, Goldman D, et al. Preservation of general intelligence following traumatic brain injury: contributions of the Met66 brain-derived neurotrophic factor. PLoS ONE. (2014) 9:e88733. doi: 10.1371/journal.pone.0088733
155. Bagnato S, Minafra L, Bravatà V, Boccagni C, Sant'angelo A, Castiglione A, et al. Brain-derived neurotrophic factor (Val66Met) polymorphism does not influence recovery from a post-traumatic vegetative state: a blinded retrospective multi-centric study. J Neurotrauma. (2012) 29:2050–9. doi: 10.1089/neu.2011.2184
156. Narayanan V, Veeramuthu V, Ahmad-Annuar A, Ramli N, Waran V, Chinna K, et al. Missense mutation of Brain Derived Neurotrophic Factor (BDNF) alters neurocognitive performance in patients with mild traumatic brain injury: a longitudinal study. PLoS ONE. (2016) 11:e0158838. doi: 10.1371/journal.pone.0158838
157. Adams SM, Conley YP, Ren D, Okonkwo DO, Puccio AM, Dixon CE, et al. ABCG2 c.421C>A is associated with outcomes after severe traumatic brain injury. J Neurotrauma. (2018) 35:48–53. doi: 10.1089/neu.2017.5000
158. Jha RM, Koleck TA, Puccio AM, Okonkwo DO, Park SY, Zusman BE, et al. Regionally clustered ABCC8 polymorphisms in a prospective cohort predict cerebral oedema and outcome in severe traumatic brain injury. J Neurol Neurosurg Psychiatry. (2018) 89:1152–62. doi: 10.1136/jnnp-2017-317741
159. Wang ZL, Xu DS, Wang YX, Qin H, Geng D. Effect of single nucleotide polymorphisms in the ATP-binding cassette B1 gene on the clinical outcome of traumatic brain injury. Genet Mol Res. (2015) 14:10948–53. doi: 10.4238/2015.September.21.6
160. Winkler EA, Yue JK, Ferguson AR, Temkin NR, Stein MB, Barber J, et al. COMT Val158Met polymorphism is associated with post-traumatic stress disorder functional outcome following mild traumatic brain injury. J Clin Neurosci. (2017) 35:109–16. doi: 10.1016/j.jocn.2016.09.017
161. Willmott C, Withiel T, Ponsford J, Burke R. COMT Val158Met and cognitive and functional outcomes after traumatic brain injury. J Neurotrauma. (2014) 31:1507–14. doi: 10.1089/neu.2013.3308
162. Dardiotis E, Dardioti M, Hadjigeorgiou GM, Paterakis K. Re: lack of association between the IL1A gene (-889) polymorphism and outcome after head injury. Tanriverdi T et al. Surg Neurol. 2006 65:7–10; discussion 10. Surg Neurol. (2006) 66:334–35. doi: 10.1016/j.surneu.2006.05.042
163. Uzan M, Tanriverdi T, Baykara O, Kafadar A, Sanus GZ, Tureci E, et al. Association between interleukin-1 beta (IL-1beta) gene polymorphism and outcome after head injury: an early report. Acta Neurochir. (2005) 147:715–20; discussion 720. doi: 10.1007/s00701-005-0529-z
164. Waters RJ, Murray GD, Teasdale GM, Stewart J, Day I, Lee RJ, et al. Cytokine gene polymorphisms and outcome after traumatic brain injury. J Neurotrauma. (2013) 30:1710–6. doi: 10.1089/neu.2012.2792
165. Diamond ML, Ritter AC, Failla MD, Boles JA, Conley YP, Kochanek PM, et al. IL-1β associations with posttraumatic epilepsy development: a genetics and biomarker cohort study. Epilepsia. (2014) 55:1109–19. doi: 10.1111/epi.12628
166. Claussnitzer M, Cho JH, Collins R, Cox NJ, Dermitzakis ET, Hurles ME, et al. A brief history of human disease genetics. Nature. (2020) 577:179–89. doi: 10.1038/s41586-019-1879-7
167. Visscher PM, Wray NR, Zhang Q, Sklar P, McCarthy MI, Brown MA, et al. 10 years of GWAS discovery: biology, function, translation. Am J Hum Genet. (2017) 101:5–22. doi: 10.1016/j.ajhg.2017.06.005
168. Wand H, Lambert SA, Tamburro C, Iacocca MA, O'Sullivan JW, Sillari C, et al. Improving reporting standards for polygenic scores in risk prediction studies. Nature. (2021) 591:211–9. doi: 10.1038/s41586-021-03243-6
169. Nelson MR, Tipney H, Painter JL, Shen J, Nicoletti P, Shen Y, et al. The support of human genetic evidence for approved drug indications. Nat Genet. (2015) 47:856–60. doi: 10.1038/ng.3314
170. Cortes D, Pera MF. The genetic basis of inter-individual variation in recovery from traumatic brain injury. NPJ Regen Med. (2021) 6:5. doi: 10.1038/s41536-020-00114-y
171. Gomez A, Dian J, Froese L, Zeiler FA. Near-infrared cerebrovascular reactivity for monitoring cerebral autoregulation and predicting outcomes in moderate to severe traumatic brain injury: proposal for a pilot observational study. JMIR Res Protoc. (2020) 9:e18740. doi: 10.2196/18740
172. Gomez A, Dian J, Zeiler FA. Continuous entirely non-invasive method for cerebrovascular reactivity assessment: technique implications. J Clin Monit Comput. (2020) 35:307–15. doi: 10.1007/s10877-020-00472-4
173. Khan DZ, Placek MM, Smielewski P, Budohoski KP, Anwar F, Hutchinson PJA, et al. Robotic semi-automated transcranial doppler assessment of cerebrovascular autoregulation in post-concussion syndrome: methodological considerations. Neurotrauma Rep. (2020) 1:218–31. doi: 10.1089/neur.2020.0021
174. Zeiler FA, Smielewski P. Application of robotic transcranial Doppler for extended duration recording in moderate/severe traumatic brain injury: first experiences. Crit Ultrasound J. (2018) 10:16. doi: 10.1186/s13089-018-0097-0
175. Gaiteri C, Dawe R, Mostafavi S, Blizinsky KD, Tasaki S, Komashko V, et al. Gene expression and DNA methylation are extensively coordinated with MRI-based brain microstructural characteristics. Brain Imaging Behav. (2019) 13:963–72. doi: 10.1007/s11682-018-9910-4
176. Mostafavi S, Gaiteri C, Sullivan SE, White CC, Tasaki S, Xu JU, et al. A molecular network of the aging human brain provides insights into the pathology and cognitive decline of Alzheimer's disease. Nat Neurosci. (2018) 21:811–9. doi: 10.1038/s41593-018-0154-9
177. Young AL, Marinescu RV, Oxtoby NP, Bocchetta M, Yong K, Firth NC, et al. Uncovering the heterogeneity and temporal complexity of neurodegenerative diseases with Subtype and Stage Inference. Nat Commun. (2018) 9:4273. doi: 10.1038/s41467-018-05892-0
178. Wu W, Zhang Y, Jiang J, Lucas MV, Fonzo GA, Rolle CE, et al. An electroencephalographic signature predicts antidepressant response in major depression. Nat Biotechnol. (2020) 38:439–47. doi: 10.1038/s41587-019-0397-3
179. Adewale Q, Khan AF, Carbonell F, Iturria-Medina Y, Alzheimer's Disease Neuroimaging Initiative. Integrated transcriptomic and neuroimaging brain model decodes biological mechanisms in aging and Alzheimer's disease. eLife. (2021) 10:e62589. doi: 10.7554/eLife.62589.sa2
180. Iturria-Medina Y, Sotero RC, Toussaint PJ, Evans AC, Alzheimer's Disease Neuroimaging Initiative. Epidemic spreading model to characterize misfolded proteins propagation in aging and associated neurodegenerative disorders. PLoS Comput Biol. (2014) 10:e1003956. doi: 10.1371/journal.pcbi.1003956
181. Carbonell F, Iturria-Medina Y, Evans AC. Mathematical modeling of protein misfolding mechanisms in neurological diseases: a historical overview. Front Neurol. (2018) 9:37. doi: 10.3389/fneur.2018.00037
182. Iturria-Medina Y, Carbonell F, Assadi A, Adewale Q, Khan AF, Baumeister TR, et al. Integrating molecular, histopathological, neuroimaging and clinical neuroscience data with NeuroPM-box. Commun Biol. (2021) 4:614. doi: 10.1038/s42003-021-02133-x
183. Iturria-Medina Y, Khan AF, Adewale Q, Shirazi AH, Alzheimer's Disease Neuroimaging Initiative. Blood brain gene expression trajectories mirror neuropathology clinical deterioration in neurodegeneration. Brain J Neurol. (2020) 143:661–73. doi: 10.1093/brain/awz400
184. Abid A, Zhang MJ, Bagaria VK, Zou J. Exploring patterns enriched in a dataset with contrastive principal component analysis. Nat Commun. (2018) 9:2134. doi: 10.1038/s41467-018-04608-8
185. Iturria-Medina Y, Carbonell FM, Evans AC, Alzheimer's Disease Neuroimaging Initiative. Multimodal imaging-based therapeutic fingerprints for optimizing personalized interventions: application to neurodegeneration. NeuroImage. (2018) 179:40–50. doi: 10.1016/j.neuroimage.2018.06.028
186. Park JY, Na HK, Kim S, Kim H, Kim HJ, Seo SW, et al. Robust Identification of Alzheimer's Disease subtypes based on cortical atrophy patterns. Sci Rep. (2017) 7:43270. doi: 10.1038/srep43270
Keywords: multi-modal data, neuroinformatics, precision medicine, traumatic brain injury, big data
Citation: Zeiler FA, Iturria-Medina Y, Thelin EP, Gomez A, Shankar JJ, Ko JH, Figley CR, Wright GEB and Anderson CM (2021) Integrative Neuroinformatics for Precision Prognostication and Personalized Therapeutics in Moderate and Severe Traumatic Brain Injury. Front. Neurol. 12:729184. doi: 10.3389/fneur.2021.729184
Received: 22 June 2021; Accepted: 09 August 2021;
Published: 07 September 2021.
Edited by:
Robert David Stevens, Johns Hopkins University, United StatesReviewed by:
Jonathan Kenneth James Rhodes, University of Edinburgh, United KingdomMarco Fidel Avila-Rodriguez, Universidad del Tolima, Colombia
Copyright © 2021 Zeiler, Iturria-Medina, Thelin, Gomez, Shankar, Ko, Figley, Wright and Anderson. This is an open-access article distributed under the terms of the Creative Commons Attribution License (CC BY). The use, distribution or reproduction in other forums is permitted, provided the original author(s) and the copyright owner(s) are credited and that the original publication in this journal is cited, in accordance with accepted academic practice. No use, distribution or reproduction is permitted which does not comply with these terms.
*Correspondence: Frederick A. Zeiler, ZnJlZGVyaWNrLnplaWxlckB1bWFuaXRvYmEuY2E=