- 1Laboratory for Movement Biomechanics, Institute for Biomechanics Department of Health Science and Technology, ETH Zürich, Zürich, Switzerland
- 2Department of Human Movement Sciences, Faculty of Behavioural and Movement Sciences, Vrije Universiteit Amsterdam, Amsterdam Movement Sciences, Amsterdam, Netherlands
- 3Graduate School of Biomedical Engineering, University of New South Wales, Sydney, NSW, Australia
- 4Department of Neurology, University Medical Center Schleswig-Holstein, Kiel, Germany
- 5Singapore-ETH Centre, Future Health Technologies Program, CREATE Campus, Singapore, Singapore
Background: Unstable gait leading to falls negatively impacts the quality of life in many people with Parkinson’s disease (PD). Systematic review evidence provides moderate to strong evidence of efficacy for a wide range of physiotherapy-based interventions to reduce gait impairment. However, outcomes have often focused on gait assessments conducted in controlled laboratory or clinical environments.
Objective: This perspective investigates the complexities and challenges of conducting real-world gait assessments in people with PD and the factors that may influence the translation from improved lab-assessed gait to improved real-world gait.
Methods: Through a thorough review of current literature, we present an in-depth analysis of current methodological approaches to real-world gait assessments and the challenges that may influence the translation of an intervention’s success from lab-based outcomes to improved walking during daily life.
Results: We identified six key factors that may influence the translation of intervention success into real-world environments at different stages of the process. These factors comprise the gait intervention, parameters analyzed, sensor setup, assessment protocols, characteristics of walking bouts, and medication status. We provide recommendations for each factor based on our synthesis of current literature.
Conclusion: This perspective emphasizes the importance of measuring intervention success outside of the laboratory environment using real-world gait assessments. Our findings support the need for future studies to bridge the gap between proven efficacy for gait as assessed in controlled laboratory environments and real-world impact for people with PD.
1 Introduction
Gait impairments are among the most common and disabling symptoms in Parkinson’s disease (PD) as they limit mobility and often lead to falls, reducing the quality of life (1). Approximately 60% of people with PD fall at least once per year (2). An estimated 50% of all falls in people with PD occur during walking, often caused by inability to adapt to environmental demands including perturbations or disturbances (3, 4). Following years of extensive research, we now have a robust understanding that gait impairments in PD are commonly characterized by reduced gait speed, shorter step length, longer double-support phase, higher stride-to-stride variability, and more steps to complete a turn (5, 6). Furthermore, the severity of these gait impairments increases as the disease advances leading to adverse mobility outcomes such as freezing of gait, that further exacerbates the risk of falling (7, 8).
A broad range of non-pharmacological interventions with the goal of managing motor symptoms, enhancing gait quality, and consequently, mitigating the risk of falls are available, including treadmill and overground gait training, resistance exercises, and other complementary therapies such as Tai Chi (9). A recent meta-analysis that included 191 trials generally found positive evidence supporting all these interventions in the management of PD (10). Among them, treadmill training emerged as the intervention with the most compelling evidence for improving gait parameters such as speed (18 out of 22 trials) and step length (14 out of 17). The ‘efficacy’ of such interventions is normally evaluated by the assessment of gait changes in gait parameters of interest inside the laboratory using motion capture systems (9–11). However, it is crucial to extend the assessment beyond the controlled laboratory to evaluate the ‘effectiveness’ of an intervention in broader, ecological, real-world settings. If an intervention is efficacious inside the laboratory, but proves ineffective outside of its controlled environment, consideration needs to be given to modifying existing gait interventions or exploring new ones.
Recent advances in wearable movement sensors have made the assessment of gait outside of the laboratory feasible. Several studies have compared walking performance between laboratory settings and daily life (12–14). These investigations have revealed a disparity between gait observed in laboratory settings and that in real-world scenarios, likely stemming from the intricate interactions we encounter in everyday life, resulting in reduced attention toward gait itself (12, 13, 15). Although gait is different in the real-world for healthy individuals, such differences are more pronounced in people with PD (13). In daily life, patients with PD can exhibit a reduction in gait speed by about 30% compared to laboratory settings, while differences in stride length can be around 20% (13). Moreover, in the real-world scenario, gait is constantly challenged by factors such as turns, stairs, or obstacles among others (15). In addition, variability and asymmetry in daily life gait may be evoked by, e.g., diverse furniture or a busy environment. Distractions and multitasking situations further influence these gait characteristics. Hence, evaluating gait in a laboratory setting can provide insights mostly into an individual’s capacity (what a person can do), while observing gait during daily life most likely offers a reflection of a person’s functional performance (what and how a person does) (16). This implies that assessments are required both in the laboratory and in real-world settings, as the information they provide is complementary and both must be taken into account for treatment decisions in PD.
The extent that interventions aimed at improving key indicators of PD disease severity in a laboratory translate to improvements in gait quality and quantity in daily life remains uncertain. In this context, ‘translation’ refers to the process of effectively transferring outcomes or findings observed in a controlled laboratory setting to real-world or daily life scenarios. It implies assessing whether (and by how much) the positive effects of interventions observed in a laboratory environment can effectively impact on the ‘same’ gait parameters or mechanisms in the real-world. In this understanding, no current intervention literature fits this scope, but a few papers have attempted to measure both laboratory and real-life gait. Rieger and coauthors report improved gait performance in a laboratory setting after a 4-week perturbation-based treadmill training with dual-tasks for healthy older adults, but no changes in daily-life gait quality or quantity (17). In people with PD, only one study has examined the effectiveness in terms of improvements in gait quality in real world contexts (18). The study by Cohen and coworkers found improvements in laboratory-based tests that are intended to reflect walking abilities (6MWT, 10MWT and TUG), after an 8-week multidisciplinary intensive outpatient rehabilitation program for PD patients. Real-world gait quantity (e.g., step count) or quality (e.g., gait speed, step length), however, did not show any (18). Another study will assess the effects of a turning intervention on clinical measures and on turning and gait quality in real-life among people with PD (19). Furthermore, many studies only report on daily life step count or the number of falls in daily life to evaluate effects after an intervention, while quality of gait is not considered (20–23). This raises the question whether we can anticipate a direct translation of the effects by training interventions for people with PD within the laboratory to gait in the real world. Psychological, physiological, pathological, cognitive, environmental, and technical factors influence the outcomes and may cause a disparity between laboratory assessments and real-world environments (15). Even though gait measures taken in a laboratory setting may not reflect those observed in daily life, there appears to be some evidence indicating that certain gait parameters, such as gait speed, exhibit similar associations between clinical and real-world settings in both PD patients and healthy controls (24). Also gait parameters derived from standardized tests in clinical and free-living settings seem to be related (25).
Given the significant impact of gait impairments on daily-life walking, achieving the translation from laboratory assessments to real-world gait through intervention is essential for enhancing quality of life and reducing the incidence of falls in people with PD. Addressing the challenge of successful translation is crucial for unraveling this real-world complexity and enhancing our ability to assess intervention effects in daily life. However, it might be due to the complexity of the real world, and thus the complexity of outcomes, that gait assessment remains often restricted to the laboratory, while it is refrained from attempting measurements in daily life. To achieve these translational goals, optimize existing treatments and increase intervention effectiveness, more real-world research in people with PD is required. This narrative review is offering a window into real-world complexity of gait assessment, particularly concerning the translation of intervention effects in PD. We provide approaches to navigate and address these complexities by identifying aspects that influence real-world assessment and may support researchers in managing real-world data. In this context, our focus was primarily on non-pharmacological interventions for gait and the translation of their effects to real-world settings. The search terms encompassed, but were not limited to, “real-world,” “daily life,” “Parkinson’s disease,” “intervention,” “rehabilitation,” “IMU,” “wearable sensor,” and “gait.” Given the limited number of real-world studies specifically focused on individuals with Parkinson’s disease, research involving healthy adults or stroke patients was also included. In particular, we focus on six factors that might have an influence on the translation of intervention effects in PD to the real world at different stages of the process: the gait intervention, analyzed parameters, number and placement of IMU sensors, assessment protocol, walking bouts and medication status. By identifying the conditions for each factor where the disparity between laboratory and real-world will be minimal and performing gait interventions under representative conditions as well as adapting the protocol for real-world assessments, we may be able to facilitate a translation. Achieving a direct translation could help understanding the effects of an intervention in daily life and lead to an optimization of current interventions in PD.
2 Factors influencing translation of gait outcomes in PD
To bridge the gap between laboratory and real-world assessments, we identified and discuss six factors (Figure 1), addressing which may aid in achieving translation of gait interventions in PD.
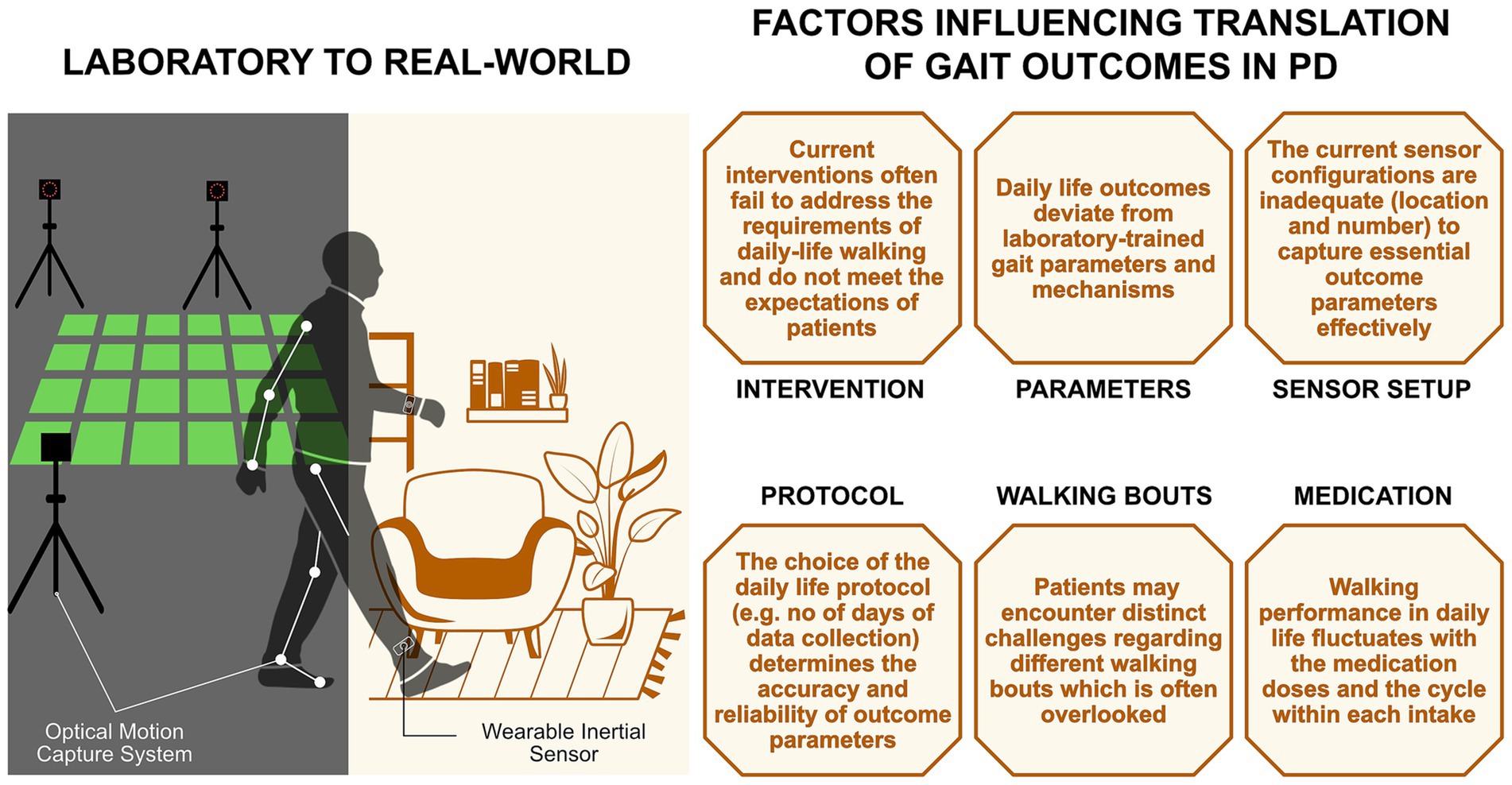
Figure 1. Factors influencing the translation of gait outcomes from the laboratory to the real-world in people with PD.
2.1 Intervention
A large number of studies investigated the effects of treadmill training on gait parameters, therefore having the largest evidence of positive effects (26–28). Treadmill training is a form of externally cued gait training that utilizes somatosensory cues through movement and speed to encourage stepping (26). Different types of treadmill training are frequently used, for example speed-dependent treadmill training with short intervals of fast speeds or treadmill training with progressive speed development over time. There is some evidence that treadmill training has (short-term) effects on gait parameters including gait speed, stride length, cadence or walking distance (26, 29) and PD patients seem to be able to transfer effects to overground walking in a laboratory (30). Treadmill training further seems to provide long-term effects for gait performance in the laboratory of three to 6 months (5). However, these effects might not provide practical benefits in daily life, as treadmill training does not reflect typical demands of a natural environment, which seems to be an important factor for translation of gait improvements in the laboratory to the real-world. Therefore, the effectiveness of treadmill training in translating benefits to real-world gait and other physical functions is yet to be determined. Various concepts were developed with the goal to mimic real-world situations, including virtual reality, obstacle crossing or external perturbations (31–33). Adding dual tasks, like a visual oddball or an auditory Stroop task while walking in a virtual reality simulation of the real world, mimics daily gait more closely (34, 35), as everyday activities often involve motor-cognitive challenges and put demand on executive processes, e.g., when walking while talking to others or carrying an object. Therefore, including dual tasks in the intervention might induce a better translation of intervention effects to real-world gait. Moreover, evidence suggests that task-specific training is necessary to achieve the largest training effects (9, 36). However, task-specificity may not be applicable to various real-world situations, where walking conditions constantly vary, potentially leading to less transferability to real-world gait (37).
2.2 Parameters
Most commonly, gait speed, step or stride length, and cadence are used as outcome parameters for evaluating the efficacy of an intervention in a laboratory (24). Here, these parameters frequently improve after an intervention (8, 24). While spatiotemporal parameters such as stride length variability or asymmetries are also employed, their utilization in daily life is less common. When evaluating the effectiveness in real-world settings several studies used questionnaires to evaluate quality of life or number of falls or investigate daily step count or walking time following an intervention. When only looking at studies calculating walking-related digital mobility outcomes, similar to the laboratory, gait speed is commonly evaluated, as it is often considered as the “final common expression” of locomotor control (38, 39). However, gait speed does not fully capture the underlying mechanisms governing locomotion (40). Therefore, as a first step, it is important to ascertain which specific gait parameter(s) the intervention is targeting directly. This gait parameter thus becomes the suitable outcome parameter for evaluating the efficacy (improvements in the laboratory) and effectiveness (improvements in daily life) of the intervention, thereby facilitating translation from laboratory settings to real-world (41).
In addition to the previously validated digital mobility outcomes, like gait speed or step length, that are related to future disease progression, falls, physical function, and cognition (24), further parameters need to be explored for application in real-world settings. Examining parameters that are less affected by environmental factors might be beneficial for observing and assessing translation. In this respect, a study involving young healthy adults showed that different settings (laboratory or real life) and testing conditions influence gait variability parameters, whereas gait stability parameters such as Sample Entropy, remain unaffected by such variations (42). Furthermore, in older healthy adults, Sample Entropy seems to be only weakly affected by walking speed (43). Therefore, including parameters less susceptible to environmental or other influences could prove beneficial for assessing translation. However, future studies, particularly focusing on individuals with PD, are necessary to comprehensively understand the potential benefits.
2.3 Sensors (number/placement)
Evaluating an individual’s gait quality in real-world conditions requires mobile sensors that are able to capture the relevant outcome parameter while also being worn comfortably by the patient over several days. However, the accuracy and reliability of gait parameters depend on the sensor setup, including the number of sensors and their position on the body. Algorithms need to be validated and improved, which is often still done in a laboratory with a rather low complexity of gait tasks and restricted observation period (44, 45). In the real-world, additional aspects such as gait speed and contextual factors like stairs or turns and use of walking aids may alter the gait pattern and influence the performance of algorithms used for calculating gait events (15). This further challenges the analysis of real-world data and undermines the reliable determination of gait parameters.
In previous studies, different IMU configurations were utilized, ranging from a single sensor to setups with as many as 11 sensors (46–50). Frequently used sensor locations include lower back, feet, wrists, or shanks, with wrist, ankle and lumbar sensors exhibiting high wearability among individuals with PD (50). When employing multiple sensors, there is still a lack of agreement on the ‘optimal’ setup, leading to many possible combinations (51). Currently, the most commonly used setup consists of a single sensor on the lower back (51). The assessment of spatio-temporal gait parameters requires the accurate detection of initial and final contact of the foot, which is essential for further determination of spatio-temporal gait parameters. Gait events can be reliably detected using only one sensor on the lower back, demonstrating accurate identification of initial contacts in both healthy individuals as well as in people with PD (52, 53). However, determination of the final foot contact using a lower back sensor is less accurate compared to shank-worn sensors, which provide higher accuracy due to their closer proximity to the point of contact between foot and ground (54–56). Consequently, the estimation of spatial parameters (e.g., step length) still seems to be challenging and presents higher errors than evaluated from sensors on the shank. This becomes even more important as errors in the estimated step length introduce errors in the estimation of gait speed (57). Furthermore, for measuring step-to-step variability, the accurate detection of gait events is crucial. Motor asymmetries often are the first motor symptoms of PD that tend to decrease with disease progression (8, 58). Assessing asymmetry with just one sensor can be challenging, while this can be accurately estimated with two sensors on the feet or shanks (59, 60). However, parameters such as the harmonic ratio, that try to estimate the symmetry of gait patterns, have been employed in real-world measurements with a single sensor (61, 62). Especially in challenging real-world situations such as turns or stair climbing, sensors placed on the shanks or feet currently provide reliable differentiation of left and right gait events. As current interventions likely only lead to small effects in real-life, it is critical to estimate gait parameters as accurately and reliably as possible (63). Therefore, including a sensor at the foot level in addition to a sensor at the lower back in the assessment of real-world gait might be beneficial in facilitating translation and enable the calculation of further relevant parameters such as foot clearance. Alternative wearable sensors, aside from IMUs, could also serve this purpose effectively. For instance, plantar pressure insoles offer accurate and reliable data on gait events (55, 64), while infrared distance sensors can detect the alternating movements of the lower extremities (65).
Nevertheless, the acceptability of a 3-sensor system needs to be considered as adherence by PD patients might be low. Achieving a high adherence requires the setup to be as imperceptible as possible, and the benefits should be communicated as they might not be directly perceivable by patients. Still, currently high quality and accuracy of data might outweigh the eventually perceived burden as many aspects and mechanisms in PD, especially in daily life, are still unknown and highly successful interventions are crucial to improve patients quality of life. Future studies should also add questionnaires about usability and wearability of the sensors to gather patient experience. This approach would facilitate the evaluation and enhancement of current sensor setups. This includes not only the number and placement of sensors but also factors such as wearing comfort, practicality in conditions such as warm or humid environments, the adjustability of sensor attachments, and participant requirements, such as removing and reattaching sensors for charging.
2.4 Protocol
Extensive research in the last years has led to rapid developments in the field of wearable devices and enables collecting data continuously over several days or weeks. Nevertheless, the duration required to reliably assess both movement characteristics in everyday physical activity and their day-to-day variability is still unknown (66–68). Patients with PD exhibit high day-to-day variability of performance measures such as steps per day (69). Therefore, it is necessary to collect data over multiple days to provide an accurate representation of gait characteristics (70). Furthermore, the amount of activity may vary between weekends and weekdays and could be influenced by weather conditions (71, 72). The number of days for data recording currently varies between three and 7 days (12, 47, 73–76). In healthy older adults, two consecutive days can already reliably assess many activities (e.g., sitting, standing) as well as parameters (e.g., number and length of walking bouts). However, the median walking bout duration requires 5 days of measurement to remain reliable (66). A minimum of 3 days may be sufficient in healthy older adults to ensure a reliable and valid assessment. However, in people with PD, three up to 7 days of recording are required to reliably estimate certain measures, such as symmetries, using a single sensor on the lower back (77). Given their increased day-to-day and within-day variability as well as reduced physical activity (78), longer recording durations are needed compared to healthy adults to ensure reliable assessment of different gait measures, potentially as long as it can be integrated with participants wear time compliance. In line with that, the recording should last five consecutive days of daily living, however, a minimum of 3 days is essential to ensure a reliable and valid assessment. Moreover, maintaining similar settings during pre-and post intervention assessment, while allowing participants to engage in their usual activities without restriction, is important. This involves having participants reside in the same environment for both measurements, preferably at their own homes. A questionnaire could be used to gather crucial information about any specific activities that the patient may engage in within or outside the home that might vary between the two assessments. Additionally, it could provide insight into the utilization of assistive devices such as walking aids. The influence of psychological and motivational aspects on daily activity might be difficult to collect objectively but needs to be considered when analyzing real-world gait data. Fear of falling often leads to a reduction in physical activity, resulting in reduced confidence, further increasing fall risk and reducing quality of life (79, 80). In addition, people with PD with low self-efficacy are less likely to participate in physical activity (81). On the other hand, gait impairments might be improved due to increased levels of motivation, as a result of switching to a goal-directed mode of control, e.g., when participating in a study (82). Therefore, a comprehensive understanding of these aspects could lead to more effectively customized interventions that address not only physical impairments but also the motivational and psychological barriers to mobility.
2.5 Walking bouts
Another important aspect is the dependency of reliability of gait parameters on the length of the walking bouts analyzed in daily life. A walking bout is defined as a period of continuous walking, but variations in the criteria for the number of consecutive steps/strides used to define a walking bout across studies make comparisons challenging (73, 83, 84).
In daily life measurements, most walking bouts have a duration shorter than 10s or fewer than 12 strides (12, 84). However, according to Del Din et al. and Rehman et al., shorter walking bouts do not discriminate between people with PD and healthy people and therefore, longer walking bouts (>30 s) should be investigated when evaluating daily life gait characteristics (12, 85). However, in their study, only around 12% of all walking bouts were longer than 30s (85). Therefore, only investigating those bout lengths would not give a complete picture of the actual walking performance. In contrast, Shah et al. found that differences between those two groups were more apparent in shorter bouts (84). The difference between the results might be explained by the utilization of different sensor setups in these studies: a single sensor on the lower back versus three sensors on the feet and the lower back. Sensors on the feet could enhance the accuracy of detection for short walking bouts (86). Nevertheless, to reliably capture gait variability measures in a laboratory, a minimum of 50 gait cycles is necessary (87). This is supported for real-world assessments by the findings of Micó-Amigo co-workers, who reported larger errors in gait event detection in walking bouts with a duration of less than 8 s using a single sensor at the lower back, which is crucial for a reliable estimation of gait variability (52). As gait parameters like gait speed or stride length increase with increasing bout lengths (84), including all gait bouts in the analysis, but separate them and compute gait parameters according to the walking bout length might provide additional information about gait characteristics in real life.
2.6 Medication status
Unsupervised measurements in daily life encompass all phases of the medication cycle, including ON and OFF phases. Due to greater regimen complexity with increasing number of daily dose intakes and cognitive deficits, medication adherence becomes more challenging in people with PD (88, 89). In addition, it has been shown that walking patterns differ when measured during peak dose (at least 30 min after medication intake) or at the end of the dose (90). To the contrary, interventions in the laboratory are usually performed during (peak) ON medication. Therefore, determining average gait parameters throughout an entire day does not provide comparable results, making it challenging to evaluate the translation and effectiveness of an intervention. To adequately quantify the impact of the medication state, successfully translating the effects may entail observing the patient under similar conditions during both unsupervised assessment and intervention phases. Additionally, during pre-and post-assessments, variations in medication intake must be accounted for, as the dosage may differ between assessment days. Documenting times of medication intake, e.g., by using a diary or tracking with a smartphone app, would facilitate the identification of phases with similar medication levels (91). However, despite being the current standard for assessing medication intake and motor fluctuations, diaries may not be feasible for reliable documentation (92). Instead, current implementations involve the monitoring of medication adherence using a smartwatch, where patients receive reminders for their medication intake times and can either confirm or decline the intake, or utilizing machine learning techniques to differentiate between ON and OFF phases (93, 94). Another successful approach could be measuring the optimum state during the real-life assessment, including the best 10% of an individual’s distribution (15). Both approaches would facilitate the comparison of gait patterns at equivalent mobility levels and minimize the influence of medication on gait outcomes. Moreover, this allows tracking to identify in which phase of the medication cycle interventions can improve gait outcomes and may lead to a refinement of interventions, enabling improvement in gait patterns throughout the day.
3 Conclusion
Despite the growing evidence that gait interventions in laboratory settings can improve gait among individuals with PD within that controlled environment, it remains uncertain whether these effects translate to real-world scenarios. In this context, we propose defining ‘translation’ as ‘the process of effectively transferring outcomes or findings observed in a controlled laboratory setting to the ‘same’ gait parameters or mechanisms in real-world or daily life scenarios.’ Achieving a translation is important to improve current gait interventions and evaluate their real-world effectiveness. Currently, only a limited number of studies investigate intervention effects in both laboratory and real-world settings. Alongside the previously mentioned intervention studies involving individuals with PD and healthy adults, further evidence exists in multiple sclerosis (MS). A multidisciplinary inpatient rehabilitation program for people with MS has shown similar outcomes, improving gait capacity in the laboratory but not translating to enhanced gait performance in daily life (95). The authors proposed incorporating behavioral and psychological factors, such as motivation and self-efficacy, into rehabilitation programs, which may contribute to achieving long-term effects with increased physical activity. In addition to these factors, disease severity and environmental challenges also need to be accounted for while motivating individuals toward increasing the level of participation in physical activity.
Furthermore, none of these studies align with our definition of translation. Instead of clearly defining which gait mechanisms and corresponding gait parameters the intervention targets, and then analyzing them in both laboratory and real-world settings, no direct comparison or analysis of the same gait outcomes has been made. Most commonly, real-world evaluations following an intervention have focused on gait speed, fall occurrences, or activity level measured by steps per day. Gait speed is often considered as the “final common expression” of locomotor control, while falls and physical activity are closely linked to quality of life. Consequently, these metrics are typically viewed as the ultimate indicators of intervention success. However, improvements in these “final outcomes” are often the result of changes in specific underlying gait mechanisms or gait quality. To date, it remains unclear whether the same gait mechanisms are involved in both laboratory and daily life settings. Gait interventions are effective because they target and improve specific mechanisms through training. Therefore, it is essential to evaluate both the direct translation of these improvements and the underlying mechanisms that facilitate the translation of intervention effects to daily life. This is particularly important in PD, where motor learning processes and the ability to transfer skills to different tasks may be impaired. Understanding this relationship is crucial for optimizing gait interventions and ensuring their long-term effectiveness in improving daily functional outcomes. As there still seems to be reluctance toward real-world assessment, potentially due to the challenges posed by the complexity of the environment and the multitude of influencing factors, we provide potential solutions to unravel the real-world complexity (Table 1). By that, we aim to provide the basis from which concrete recommendations will emerge, guiding best practices in this area.
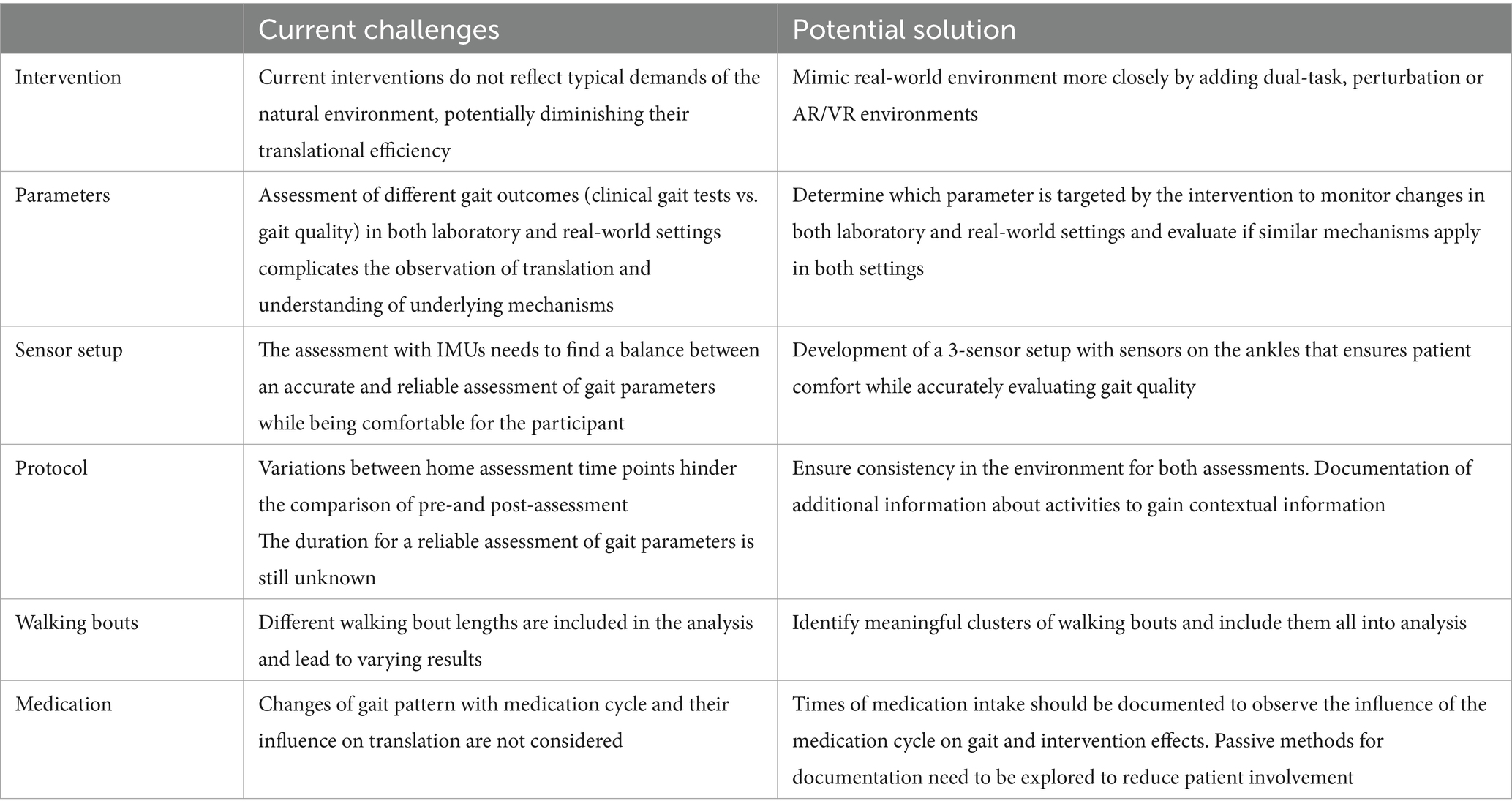
Table 1. Challenges and potential solutions to unravel real-world complexity and advance the application of daily life assessment in the evaluation of gait interventions in PD.
By identifying the factors influencing real-world assessment in PD, this paper proposes possible solutions to mitigate the disparities between laboratory and real-world settings, particularly for gait interventions in PD and their translation to real-life. This might allow to improve our understanding of current gait interventions, their effectiveness in the real world and the optimization of these treatments. However, it is important to recognize that for patients, translation or improvement of gait parameters, such as gait speed, may hold different significance. For them, the objective might rather entail improvements like being able to walk to the store. Hence, it is essential to also consider patient reported outcomes and connect them to gait parameters to obtain a comprehensive understanding of translation and real-world effectiveness. In lab-based assessments, minimal clinically important differences are established to indicate the meaningful impact of an intervention. However, these thresholds still require reassessment in real-world environments. Certain gait parameters, like stride time variability, show substantial increases in real-world settings compared to laboratory environments (e.g., treadmill assessments) (61). These discrepancies must be considered when assessing the effectiveness of an intervention. To identify a meaningful change, one approach might be to compare the distributions of data collected in and outside the lab, while adjusting for differences in their respective standard errors. Alternatively, combining real-world gait outcomes with patient-reported outcomes may signify a meaningful change if improvements in gait outcomes can also be perceived by the patient. By exploring this in future studies and examining how changes in gait outcomes impact quality of life and activities of daily living, it may become possible to connect subjective patient reports with objective gait data, thereby improving effectiveness through a more patient-centered approach.
Author contributions
CL: Conceptualization, Writing – original draft, Writing – review & editing. JD: Funding acquisition, Project administration, Writing – review & editing. MB: Writing – review & editing. JW: Writing – review & editing. WM: Conceptualization, Writing – review & editing. NS: Writing – review & editing. DR: Conceptualization, Visualization, Writing – original draft, Writing – review & editing.
Funding
The author(s) declare that financial support was received for the research, authorship, and/or publication of this article. This research was financially supported by StepuP: Steps against the burden of Parkinson’s Disease, grant number JPND2022-128. Open access funding by ETH Zurich.
Acknowledgments
We thank William R Taylor for supporting the StepuP project.
Conflict of interest
The authors declare that the research was conducted in the absence of any commercial or financial relationships that could be construed as a potential conflict of interest.
The author(s) declared that they were an editorial board member of Frontiers, at the time of submission. This had no impact on the peer review process and the final decision.
Publisher’s note
All claims expressed in this article are solely those of the authors and do not necessarily represent those of their affiliated organizations, or those of the publisher, the editors and the reviewers. Any product that may be evaluated in this article, or claim that may be made by its manufacturer, is not guaranteed or endorsed by the publisher.
References
1. Fasano, A, Canning, CG, Hausdorff, JM, Lord, S, and Rochester, L. Falls in Parkinson’s disease: a complex and evolving picture. Mov Disord. (2017) 32:1524–36. doi: 10.1002/mds.27195
2. Allen, NE, Schwarzel, AK, and Canning, CG. Recurrent falls in Parkinson’s disease: a systematic review. Parkinsons Dis. (2013) 2013:906274:1–16. doi: 10.1155/2013/906274
3. Ashburn, A, Stack, E, Ballinger, C, Fazakarley, L, and Fitton, C. The circumstances of falls among people with Parkinson’s disease and the use of falls diaries to facilitate reporting. Disabil Rehabil. (2008) 30:1205–12. doi: 10.1080/09638280701828930
4. Dennison, AC, Noorigian, JV, Robinson, KM, Fisman, DN, Cianci, HJ, Moberg, P, et al. Falling in Parkinson disease: identifying and prioritizing risk factors in recurrent fallers. Am J Phys Med Rehabil. (2007) 86:621–32. doi: 10.1097/PHM.0b013e311611583
5. Mak, MK, Wong-Yu, IS, Shen, X, and Chung, CL. Long-term effects of exercise and physical therapy in people with Parkinson disease. Nat Rev Neurol. (2017) 13:689–703. doi: 10.1038/nrneurol.2017.128
6. Zanardi, APJ, da Silva, ES, Costa, RR, Passos-Monteiro, E, Dos Santos, IO, Kruel, LFM, et al. Gait parameters of Parkinson's disease compared with healthy controls: a systematic review and meta-analysis. Sci Rep. (2021) 11:752. doi: 10.1038/s41598-020-80768-2
7. Welzel, J, Wendtland, D, Warmerdam, E, Romijnders, R, Elshehabi, M, Geritz, J, et al. Step length is a promising progression marker in Parkinson's disease. Sensors. (2021) 21:2292. doi: 10.3390/s21072292
8. Mirelman, A, Bonato, P, Camicioli, R, Ellis, TD, Giladi, N, Hamilton, JL, et al. Gait impairments in Parkinson's disease. Lancet Neurol. (2019) 18:697–708. doi: 10.1016/S1474-4422(19)30044-4
9. Ni, M, Hazzard, JB, Signorile, JF, and Luca, C. Exercise guidelines for gait function in Parkinson's disease: a systematic review and Meta-analysis. Neurorehabil Neural Repair. (2018) 32:872–86. doi: 10.1177/1545968318801558
10. Radder, DLM, Silva, L, de Lima, A, Domingos, J, Keus, SHJ, van Nimwegen, M, et al. Physiotherapy in Parkinson's disease: a Meta-analysis of present treatment modalities. Neurorehabil Neural Repair. (2020) 34:871–80. doi: 10.1177/1545968320952799
11. Hvingelby, VS, Glud, AN, Sorensen, JCH, Tai, Y, Andersen, ASM, Johnsen, E, et al. Interventions to improve gait in Parkinson's disease: a systematic review of randomized controlled trials and network meta-analysis. J Neurol. (2022) 269:4068–79. doi: 10.1007/s00415-022-11091-1
12. Del Din, S, Godfrey, A, Galna, B, Lord, S, and Rochester, L. Free-living gait characteristics in ageing and Parkinson's disease: impact of environment and ambulatory bout length. J Neuroeng Rehabil. (2016) 13:46. doi: 10.1186/s12984-016-0154-5
13. Shah, VV, McNames, J, Mancini, M, Carlson-Kuhta, P, Spain, RI, Nutt, JG, et al. Laboratory versus daily life gait characteristics in patients with multiple sclerosis, Parkinson's disease, and matched controls. J Neuroeng Rehabil. (2020) 17:159. doi: 10.1186/s12984-020-00781-4
14. Toosizadeh, N, Mohler, J, Lei, H, Parvaneh, S, Sherman, S, and Najafi, B. Motor performance assessment in Parkinson’s disease: association between objective in-clinic, objective in-home, and subjective/semi-objective measures. PLoS One. (2015) 10:e0124763. doi: 10.1371/journal.pone.0124763
15. Warmerdam, E, Hausdorff, JM, Atrsaei, A, Zhou, YH, Mirelman, A, Aminian, K, et al. Long-term unsupervised mobility assessment in movement disorders. Lancet Neurol. (2020) 19:462–70. doi: 10.1016/S1474-4422(19)30397-7
16. Brodie, MA, Coppens, MJ, Lord, SR, Lovell, NH, Gschwind, YJ, Redmond, SJ, et al. Wearable pendant device monitoring using new wavelet-based methods shows daily life and laboratory gaits are different. Med Biol Eng Comput. (2016) 54:663–74. doi: 10.1007/s11517-015-1357-9
17. Rieger, MM, Papegaaij, S, Steenbrink, F, van Dieen, JH, and Pijnappels, M. Effects of perturbation-based treadmill training on balance performance, daily-life gait, and falls in older adults: REACT randomized controlled trial. Phys Ther. (2023) 104:pzad136. doi: 10.1093/ptj/pzad136
18. Cohen, M, Herman, T, Ganz, N, Badichi, I, Gurevich, T, and Hausdorff, JM. Multidisciplinary intensive rehabilitation program for people with Parkinson’s disease: gaps between the clinic and real-world mobility. Int J Environ Res Public Health. (2023) 20:3806. doi: 10.3390/ijerph20053806
19. King, LA, Carlson-Kuhta, P, Wilhelm, JL, Lapidus, JA, Dale, ML, Talman, LS, et al. TURN-IT: a novel turning intervention program to improve quality of turning in daily life in people with Parkinson's disease. BMC Neurol. (2022) 22:442. doi: 10.1186/s12883-022-02934-5
20. Conradsson, D, Löfgren, N, Nero, H, Hagströmer, M, Ståhle, A, Lökk, J, et al. The effects of highly challenging balance training in elderly with Parkinson's disease: a randomized controlled trial. Neurorehab Neural Res. (2015) 29:827–36. doi: 10.1177/1545968314567150
21. Leavy, B, Joseph, C, Löfgren, N, Johansson, H, Hagströmer, M, and Franzén, E. Outcome evaluation of highly challenging balance training for people with Parkinson disease: a multicenter effectiveness-implementation study. J Neurol Phys Ther. (2020) 44:15–22. doi: 10.1097/NPT.0000000000000298
22. Lim, I, van Wegen, E, Jones, D, Rochester, L, Nieuwboer, A, Willems, A-M, et al. Does cueing training improve physical activity in patients with Parkinson’s disease? Neurorehab Neural Res. (2010) 24:469–77. doi: 10.1177/1545968309356294
23. van Nimwegen, M, Speelman, AD, Overeem, S, van de Warrenburg, BP, Smulders, K, Dontje, ML, et al. Promotion of physical activity and fitness in sedentary patients with Parkinson's disease: randomised controlled trial. BMJ. (2013) 346:346. doi: 10.1136/bmj.f576
24. Polhemus, A, Ortiz, LD, Brittain, G, Chynkiamis, N, Salis, F, Gassner, H, et al. Walking on common ground: a cross-disciplinary scoping review on the clinical utility of digital mobility outcomes. NPJ Digit Med. (2021) 4:149. doi: 10.1038/s41746-021-00513-5
25. Gassner, H, Sanders, P, Dietrich, A, Marxreiter, F, Eskofier, BM, Winkler, J, et al. Clinical relevance of standardized Mobile gait tests. Reliability analysis between gait recordings at hospital and home in Parkinson's disease: a pilot study. J Parkinsons Dis. (2020) 10:1763–73. doi: 10.3233/JPD-202129
26. Harro, CC, Shoemaker, MJ, Frey, OJ, Gamble, AC, Harring, KB, Karl, KL, et al. The effects of speed-dependent treadmill training and rhythmic auditory-cued overground walking on gait function and fall risk in individuals with idiopathic Parkinson's disease: a randomized controlled trial. NeuroRehabilitation. (2014) 34:557–72. doi: 10.3233/NRE-141051
27. Bello, O, Sanchez, JA, Lopez-Alonso, V, Marquez, G, Morenilla, L, Castro, X, et al. The effects of treadmill or overground walking training program on gait in Parkinson's disease. Gait Posture. (2013) 38:590–5. doi: 10.1016/j.gaitpost.2013.02.005
28. Fisher, BE, Wu, AD, Salem, GJ, Song, J, Lin, CH, Yip, J, et al. The effect of exercise training in improving motor performance and corticomotor excitability in people with early Parkinson's disease. Arch Phys Med Rehabil. (2008) 89:1221–9. doi: 10.1016/j.apmr.2008.01.013
29. Schlick, C, Ernst, A, Botzel, K, Plate, A, Pelykh, O, and Ilmberger, J. Visual cues combined with treadmill training to improve gait performance in Parkinson's disease: a pilot randomized controlled trial. Clin Rehabil. (2016) 30:463–71. doi: 10.1177/0269215515588836
30. Klamroth, S, Steib, S, Gassner, H, Gossler, J, Winkler, J, Eskofier, B, et al. Immediate effects of perturbation treadmill training on gait and postural control in patients with Parkinson's disease. Gait Posture. (2016) 50:102–8. doi: 10.1016/j.gaitpost.2016.08.020
31. Bohil, CJ, Alicea, B, and Biocca, FA. Virtual reality in neuroscience research and therapy. Nat Rev Neurosci. (2011) 12:752–62. doi: 10.1038/nrn3122
32. Kim, A, Schweighofer, N, and Finley, JM. Locomotor skill acquisition in virtual reality shows sustained transfer to the real world. J Neuroeng Rehabil. (2019) 16:113. doi: 10.1186/s12984-019-0584-y
33. Hulzinga, F, de Rond, V, Vandendoorent, B, Gilat, M, Ginis, P, D'Cruz, N, et al. Repeated gait perturbation training in Parkinson's disease and healthy older adults: a systematic review and Meta-analysis. Front Hum Neurosci. (2021) 15:732648. doi: 10.3389/fnhum.2021.732648
34. Geerse, DJ, Roerdink, M, Marinus, J, and van Hilten, JJ. Assessing walking adaptability in Parkinson's disease: "the interactive walkway". Front Neurol. (2018) 9:1096. doi: 10.3389/fneur.2018.01096
35. Killane, I, Fearon, C, Newman, L, McDonnell, C, Waechter, SM, Sons, K, et al. Dual motor-cognitive virtual reality training impacts dual-task performance in freezing of gait. IEEE J Biomed Health. (2015) 19:1855–61. doi: 10.1109/JBHI.2015.2479625
36. Hubbard, IJ, Parsons, MW, Neilson, C, and Carey, LM. Task-specific training: evidence for and translation to clinical practice. Occup Ther Int. (2009) 16:175–89. doi: 10.1002/oti.275
37. Song, PYH, Sturnieks, DL, Davis, MK, Lord, SR, and Okubo, Y. Perturbation-based balance training using repeated trips on a walkway vs. belt accelerations on a treadmill: a cross-over randomised controlled trial in community-dwelling older adults. Front Sports Act Living. (2021) 3:702320. doi: 10.3389/fspor.2021.702320
38. Studenski, S, Perera, S, Patel, K, Rosano, C, Faulkner, K, Inzitari, M, et al. Gait speed and survival in older adults. JAMA. (2011) 305:50–8. doi: 10.1001/jama.2010.1923
39. Nordin, AD, Hairston, WD, and Ferris, DP. Faster gait speeds reduce alpha and Beta EEG spectral power from human sensorimotor cortex. IEEE T Bio Med Eng. (2020) 67:842–53. doi: 10.1109/TBME.2019.2921766
40. Lord, S, Galna, B, and Rochester, L. Moving forward on gait measurement: toward a more refined approach. Mov Disord. (2013) 28:1534–43. doi: 10.1002/mds.25545
41. Porzsolt, F, Wiedemann, F, Phlippen, M, Weiss, C, Weiss, M, Schmaling, K, et al. The terminology conflict on efficacy and effectiveness in healthcare. J Comp Effect Res. (2020) 9:1171–8. doi: 10.2217/cer-2020-0149
42. Tamburini, P, Storm, F, Buckley, C, Bisi, MC, Stagni, R, and Mazzà, C. Moving from laboratory to real life conditions: influence on the assessment of variability and stability of gait. Gait Posture. (2018) 59:248–52. doi: 10.1016/j.gaitpost.2017.10.024
43. Huijben, B, van Schooten, KS, van Dieen, JH, and Pijnappels, M. The effect of walking speed on quality of gait in older adults. Gait Posture. (2018) 65:112–6. doi: 10.1016/j.gaitpost.2018.07.004
44. Bertuletti, S, Cereatti, A, Comotti, D, Caldara, M, and Della, CU. Static and dynamic accuracy of an innovative miniaturized wearable platform for short range distance measurements for human movement applications. Sensors. (2017) 17:1492. doi: 10.3390/s17071492
45. Soltani, A, Dejnabadi, H, Savary, M, and Aminian, K. Real-world gait speed estimation using wrist sensor: a personalized approach. IEEE J Biomed Health. (2020) 24:658–68. doi: 10.1109/JBHI.2019.2914940
46. Zhou, L, Tunca, C, Fischer, E, Brahms, CM, Ersoy, C, Granacher, U, et al. Validation of an IMU gait analysis algorithm for gait monitoring in daily life situations. Proceedings of the 42nd annual international conferences of the IEEE engineering in medicine and biology society. Montreal, QC, Canada: Enabling innovative Technologies for Global Healthcare Embc’20. (2020).
47. Renggli, D, Graf, C, Tachatos, N, Singh, N, Meboldt, M, Taylor, WR, et al. Wearable inertial measurement units for assessing gait in real-world environments. Front Physiol. (2020) 11:11. doi: 10.3389/fphys.2020.00090
48. Salis, F, Bertuletti, S, Scott, K, Caruso, M, Bonci, T, Buckley, E, et al. A wearable multi-sensor system for real world gait analysis. 43rd Annual International Conference of the IEEE Engineering in Medicine & Biology Society (EMBC), Mexico, (2021) 7020–7023.
49. Romijnders, R, Warmerdam, E, Hansen, C, Welzel, J, Schmidt, G, and Maetzler, W. Validation of IMU-based gait event detection during curved walking and turning in older adults and Parkinson's disease patients. J Neuroeng Rehabil. (2021) 18:28. doi: 10.1186/s12984-021-00828-0
50. O'Day, J, Lee, M, Seagers, K, Hoffman, S, Jih-Schiff, A, Kidzinski, L, et al. Assessing inertial measurement unit locations for freezing of gait detection and patient preference. J Neuroeng Rehabil. (2022) 19. doi: 10.1186/s12984-022-00992-x
51. Brognara, L, Palumbo, P, Grimm, B, and Palmerini, L. Assessing gait in Parkinson's disease using wearable motion sensors: a systematic review. Diseases. (2019) 7:18. doi: 10.3390/diseases7010018
52. Micó-Amigo, ME, Bonci, T, Paraschiv-Ionescu, A, Ullrich, M, Kirk, C, Soltani, A, et al. Assessing real-world gait with digital technology? Validation, insights and recommendations from the Mobilise-D consortium. J Neuroeng Rehabil. (2023) 20:78. doi: 10.1186/s12984-023-01198-5
53. Panebianco, GP, Bisi, MC, Stagni, R, and Fantozzi, S. Analysis of the performance of 17 algorithms from a systematic review: influence of sensor position, analysed variable and computational approach in gait timing estimation from IMU measurements. Gait Posture. (2018) 66:76–82. doi: 10.1016/j.gaitpost.2018.08.025
54. Trojaniello, D, Cereatti, A, Pelosin, E, Avanzino, L, Mirelman, A, Hausdorff, JM, et al. Estimation of step-by-step spatio-temporal parameters of normal and impaired gait using shank-mounted magneto-inertial sensors: application to elderly, hemiparetic, parkinsonian and choreic gait. J Neuroeng Rehabil. (2014) 11:152. doi: 10.1186/1743-0003-11-152
55. Storm, FA, Buckley, CJ, and Mazzà, C. Gait event detection in laboratory and real life settings: accuracy of ankle and waist sensor based methods. Gait Posture. (2016) 50:42–6. doi: 10.1016/j.gaitpost.2016.08.012
56. Alvarez, JC, Alvarez, D, Lopez, A, and Gonzalez, RC. Pedestrian navigation based on a waist-worn inertial sensor. Sensors. (2012) 12:10536–49. doi: 10.3390/s120810536
57. Kirk, C, Kuederle, A, Mico-Amigo, ME, Bonci, T, Paraschiv-Ionescu, A, Ullrich, M, et al. Estimating real-world walking speed from a single wearable device: analytical pipeline, results and lessons learnt from the Mobilise-D technical validation study. (2023). Available at: https://www.researchsquare.com/article/rs-2965670/v1.
58. Miller-Patterson, C, Buesa, R, McLaughlin, N, Jones, R, Akbar, U, and Friedman, JH. Motor asymmetry over time in Parkinson's disease. J Neurol Sci. (2018) 393:14–7. doi: 10.1016/j.jns.2018.08.001
59. Del Din, S, Hickey, A, Hurwitz, N, Mathers, JC, Rochester, L, and Godfrey, A. Measuring gait with an accelerometer-based wearable: influence of device location, testing protocol and age. Physiol Meas. (2016) 37:1785–97. doi: 10.1088/0967-3334/37/10/1785
60. Mobbs, RJ, Perring, J, Raj, SM, Maharaj, M, Yoong, NKM, Sy, LW, et al. Gait metrics analysis utilizing single-point inertial measurement units: a systematic review. Mhealth. (2022) 8:9. doi: 10.21037/mhealth-21-17
61. Rispens, SM, Van Dieën, JH, Van Schooten, KS, Lizama, LEC, Daffertshofer, A, Beek, PJ, et al. Fall-related gait characteristics on the treadmill and in daily life. J Neuroeng Rehabil. (2016) 13:12. doi: 10.1186/s12984-016-0118-9
62. Menz, HB, Lord, SR, and Fitzpatrick, RC. Acceleration patterns of the head and pelvis when walking on level and irregular surfaces. Gait Posture. (2003) 18:35–46. doi: 10.1016/S0966-6362(02)00159-5
63. Ilg, W, Seemann, J, Sarvestan, J, Del, D, Synofzik, M, and Alcock, L. Inertial sensors on the feet, rather than lumbar sensor only, increase sensitivity of spatio-temporal gait measures to longitudinal progression in ataxia. International Symposium on Posture and Gait Research, Brisbane, Australia. (2023)
64. Roth, N, Martindale, CF, Eskofier, BM, Gaßner, H, Kohl, Z, and Klucken, J. Synchronized sensor insoles for clinical gait analysis in home-monitoring applications. Curr Direct Biomed Eng. (2018) 4:433–7. doi: 10.1515/cdbme-2018-0103
65. Salis, F, Bertuletti, S, Bonci, T, Caruso, M, Scott, K, Alcock, L, et al. A multi-sensor wearable system for the assessment of diseased gait in real-world conditions. Front Bioeng Biotechnol. (2023) 11:1143248. doi: 10.3389/fbioe.2023.1143248
66. van Schooten, KS, Rispens, SM, Elders, PJM, Lips, P, van Dieën, JH, and Pijnappels, M. Assessing physical activity in older adults: required days of trunk accelerometer measurements for reliable estimation. J Aging Phys Activ. (2015) 23:9–17. doi: 10.1123/JAPA.2013-0103
67. Ilg, W, Milne, S, Schmitz-Hubsch, T, Alcock, L, Beichert, L, Bertini, E, et al. Quantitative gait and balance outcomes for Ataxia trials: consensus recommendations by the Ataxia global initiative working group on digital-motor biomarkers. Cerebellum. (2023) 23:1566–92. doi: 10.1007/s12311-023-01625-2
68. Meyer, BM, Depetrillo, P, Franco, J, Donahue, N, Fox, SR, O’Leary, A, et al. How much data is enough? A reliable methodology to examine long-term wearable data acquisition in gait and postural sway. Sensors. (2022) 22:6982. doi: 10.3390/s22186982
69. Quinn, NP . Classification of fluctuations in patients with Parkinson's disease. Neurology. (1998) 51:S25–9. doi: 10.1212/WNL.51.2_Suppl_2.S25
70. Holleran, CL, Bland, MD, Reisman, DS, Ellis, TD, Earhart, GM, and Lang, CE. Day-to-day variability of walking performance measures in individuals post-stroke and individuals with Parkinson disease. J Neurol Phys Ther. (2020) 44:241–7. doi: 10.1097/NPT.0000000000000327
71. Klenk, J, Büchele, G, Rapp, K, Franke, S, Peter, R, and Grp, AS. Walking on sunshine: effect of weather conditions on physical activity in older people. J Epidemiol Commun H. (2012) 66:474–6. doi: 10.1136/jech.2010.128090
72. Carcreff, L, Gerber, CN, Paraschiv-Ionescu, A, De Coulon, G, Aminian, K, Newman, CJ, et al. Walking speed of children and adolescents with cerebral palsy: laboratory versus daily life. Front Bioeng Biotechnol. (2020) 8:812. doi: 10.3389/fbioe.2020.00812
73. Hillel, I, Gazit, E, Nieuwboer, A, Avanzino, L, Rochester, L, Cereatti, A, et al. Is every-day walking in older adults more analogous to dual-task walking or to usual walking? Elucidating the gaps between gait performance in the lab and during 24/7 monitoring. Eur Rev Aging Phys A. (2019) 16:12. doi: 10.1186/s11556-019-0214-5
74. Roos, MA, Rudolph, KS, and Reisman, DS. The structure of walking activity in people after stroke compared with older adults without disability: a cross-sectional study. Phys Ther. (2012) 92:1141–7. doi: 10.2522/ptj.20120034
75. Weiss, A, Herman, T, Giladi, N, and Hausdorff, JM. Objective assessment of fall risk in Parkinson's disease using a body-fixed sensor worn for 3 days. PLoS One. (2014) 9:e96675. doi: 10.1371/journal.pone.0096675
76. Pilotto, A, Rizzardi, A, Zatti, C, Hansen, C, Donini, A, Romijnders, R, et al. Unsupervised but not supervised gait parameters are related to fatigue in Parkinson's disease: a pilot study. Front Aging Neurosci. (2023) 15:1279722. doi: 10.3389/fnagi.2023.1279722
* 77. Gazit, E, Garcia-Aymerich, J, Buekers, J, Del Din, S, Mueller, A, Maetzler, W, et al. How many days are required to reliably estimate diverse digital mobility outcomes from various gait domains with a lower back sensor in people with Parkinson's disease? [Abstract]. Movement Disorders (2024), 39(Suppl. 1). (2024) Available at: https://www.mdsabstracts.org/abstract/how-many-days-are-required-to-reliably-estimate-diverse-digital-mobility-outcomes-from-various-gait-domains-with-a-lower-back-sensor-in-people-with-parkinsons-disease/. (Accessed October 2, 2024).
78. van Nimwegen, M, Speelman, AD, Hofman-van Rossum, EJ, Overeem, S, Deeg, DJ, Borm, GF, et al. Physical inactivity in Parkinson's disease. J Neurol. (2011) 258:2214–21. doi: 10.1007/s00415-011-6097-7
79. Bloem, BR, Grimbergen, YA, Cramer, M, Willemsen, M, and Zwinderman, AH. Prospective assessment of falls in Parkinson's disease. J Neurol. (2001) 248:950–8. doi: 10.1007/s004150170047
80. Schoene, D, Heller, C, Aung, YN, Sieber, CC, Kemmler, W, and Freiberger, E. A systematic review on the influence of fear of falling on quality of life in older people: is there a role for falls? Clin Interv Aging. (2019) 14:701–19. doi: 10.2147/CIA.S197857
81. Ellis, T, Cavanaugh, JT, Earhart, GM, Ford, MP, Foreman, KB, Fredman, L, et al. Factors associated with exercise behavior in people with Parkinson disease. Phys Ther. (2011) 91:1838–48. doi: 10.2522/ptj.20100390
82. Nonnekes, J, Ruzicka, E, Nieuwboer, A, Hallett, M, Fasano, A, and Bloem, BR. Compensation strategies for gait impairments in Parkinson disease: a review. JAMA Neurol. (2019) 76:718–25. doi: 10.1001/jamaneurol.2019.0033
83. Galperin, I, Hillel, I, Del Din, S, Bekkers, EMJ, Nieuwboer, A, Abbruzzese, G, et al. Associations between daily-living physical activity and laboratory-based assessments of motor severity in patients with falls and Parkinson's disease. Parkinsonism Relat D. (2019) 62:85–90. doi: 10.1016/j.parkreldis.2019.01.022
84. Shah, VV, McNames, J, Harker, G, Mancini, M, Carlson-Kuhta, P, Nutt, JG, et al. Effect of bout length on gait measures in people with and without Parkinson's disease during daily life. Sensors. (2020) 20:5769. doi: 10.3390/s20205769
85. Rehman, RZU, Guan, Y, Shi, JQ, Alcock, L, Yarnall, AJ, Rochester, L, et al. Investigating the impact of environment and data aggregation by walking bout duration on Parkinson's disease classification using machine learning. Front Aging Neurosci. (2022) 14:14. doi: 10.3389/fnagi.2022.808518
86. Ullrich, M. Fall risk prediction in Parkinson’s disease using machine learning and real-world inertial sensor-based gait analysis: Doctoral dissertation, Dissertation, Erlangen, Friedrich-Alexander-Universität Erlangen-Nürnberg (FAU). (2023).
87. König, N, Singh, NB, Von Beckerath, J, Janke, L, and Taylor, WR. Is gait variability reliable? An assessment of spatio-temporal parameters of gait variability during continuous overground walking. Gait Posture. (2014) 39:615–7. doi: 10.1016/j.gaitpost.2013.06.014
88. Malek, N, and Grosset, DG. Medication adherence in patients with Parkinson’s disease. CNS Drugs. (2015) 29:47–53. doi: 10.1007/s40263-014-0220-0
89. Zhang, H, Xu, C, Li, H, Rathore, AS, Song, C, Yan, Z, et al. PDMove: towards passive medication adherence monitoring of Parkinson’s disease using smartphone-based gait assessment. Proc ACM Interact Mob Wearable Ubiquitous Technol. (2019) 3:1–23. doi: 10.1145/3351281
90. Morris, ME, Matyas, TA, Iansek, R, and Summers, JJ. Temporal stability of gait in Parkinson’s disease. Phys Ther. (1996) 76:763–77. doi: 10.1093/ptj/76.7.763
91. Hauser, RA, Friedlander, J, Zesiewicz, TA, Adler, CH, Seeberger, LC, O'Brien, CF, et al. A home diary to assess functional status in patients with Parkinson’s disease with motor fluctuations and dyskinesia. Clin Neuropharmacol. (2000) 23:75–81. doi: 10.1097/00002826-200003000-00003
92. Löhle, M, Bremer, A, Gandor, F, Timpka, J, Odin, P, Ebersbach, G, et al. Validation of the PD home diary for assessment of motor fluctuations in advanced Parkinson's disease. NPJ Parkinsons Dis. (2022) 8:69. doi: 10.1038/s41531-022-00331-w
93. Debelle, H, Packer, E, Beales, E, Bailey, HGB, Mc Ardle, R, Brown, P, et al. Feasibility and usability of a digital health technology system to monitor mobility and assess medication adherence in mild-to-moderate Parkinson's disease. Front Neurol. (2023) 14:1111260. doi: 10.3389/fneur.2023.1111260
94. Moradi, H, Roth, N, Seifer, AK, and Eskofier, BM. Detection of distorted gait and wearing-off phenomenon in Parkinson’s disease patients during levodopa therapy. 2022 IEEE-Embs international conference on biomedical and health informatics (BHI) jointly organised with the IEEE-EMBS international conference on wearable and implantable body sensor networks (Bsn'22) Ioannina, Greece. (2022).
Keywords: Parkinson’s disease, gait, intervention, free-living, laboratory
Citation: Lang C, van Dieen JH, Brodie MA, Welzel J, Maetzler W, Singh NB and Ravi DK (2024) Complexities and challenges of translating intervention success to real world gait in people with Parkinson’s disease. Front. Neurol. 15:1455692. doi: 10.3389/fneur.2024.1455692
Edited by:
Simone Carozzo, Sant’Anna Crotone Institute, ItalyReviewed by:
Julia M. Leach, Synergic Medical Technologies, Inc., United StatesMichela Russo, University of Naples Federico II, Italy
Copyright © 2024 Lang, van Dieen, Brodie, Welzel, Maetzler, Singh and Ravi. This is an open-access article distributed under the terms of the Creative Commons Attribution License (CC BY). The use, distribution or reproduction in other forums is permitted, provided the original author(s) and the copyright owner(s) are credited and that the original publication in this journal is cited, in accordance with accepted academic practice. No use, distribution or reproduction is permitted which does not comply with these terms.
*Correspondence: Deepak K. Ravi, ZGVlcGFrLnJhdmlAaGVzdC5ldGh6LmNo