- 1Department of Radiology, Fujian Medical University Union Hospital, Fuzhou, China
- 2Department of Neurology, Center for Cognitive Neurology, Fujian Medical University Union Hospital, Fuzhou, China
- 3Fujian Institute of Geriatrics, Fujian Medical University Union Hospital, Fuzhou, China
- 4Institute of Clinical Neurology, Fujian Medical University, Fuzhou, China
- 5Fujian Key Laboratory of Molecular Neurology, Fujian Medical University, Fuzhou, China
Objective: This study aimed to investigated the microstructural integrity of WM and the relationship with the cognitive domains and cognition-related plasma biomarkers in low heart rate variability (HRV).
Methods: Our study recruited 44 high HRV and 44 low HRV (Grouping by median of HF). Diffusion Magnetic resonance imaging (MRI) was utilized for the calculation of neurite orientation dispersion and density imaging (NODDI) parameters, and tract-based spatial statistics (TBSS) were employed to explore differential clusters. The fibers covered by these clusters were defined as regions of interest (ROI) for the extraction of NODDI parameter values and the analysis of their correlation with cognitive domains and cognition-related plasma biomarkers.
Results: The TBSS analysis unveiled specific cerebral regions exhibiting disparities within the low HRV group high HRV group. These differences were evident in fractional isotropy (FISO; p < 0.05). The extracted values from these ROIs (p < 0.05) were mainly manifested in the anterior regions of the brain and corpus callosum. Further analysis showed that the abnormal white matter (WM) showed significant correlations with CDR, RCFT-I, RCFT-D, attention, memory, language, and cognition-related plasma biomarkers to varying degrees.
Conclusion: Patients with low HRV exhibit distinctive patterns of microstructural changes in the WM as revealed by the FISO which indicate a decline in white matter integrity, and the relationship between low HRV-related and worse cognitive performance may be attributed to damage of the frontal-corpus callosum pathways.
1 Introduction
The ECG of a healthy human exhibits subtle fluctuations from beat to beat. This phenomenon is known as heart rate variability (HRV), which refers to the variation in the duration of time separating consecutive heart contractions (1). The fluctuations in heart rate are characterized by HRV, a manifestation of the intricate interplay between the sympathetic and parasympathetic branches of the autonomic nervous system (2). HRV has emerged as a significant indicator of overall wellbeing (1, 3).
HRV can be measured by using methods from the time domain and frequency domain (4, 5). The standard time-domain measurements for HRV typically encompass the Standard Deviation of the N-N Intervals (SDNN), the Root Mean Square of the Successive Differences (RMSSD), and the percentage of successive normal sinus RR intervals more than 50 ms (pNN50). In the frequency domain, the key parameters are the total power (TP), high-frequency band (HF; 0.15 to 0.4 Hz), low-frequency band (LF; 0.04 to 0.15 Hz), very-low frequency (VLF; 0.0033 to 0.04 Hz), and ultralow frequencies (ULF; below 0.0033 Hz) (6). SDNN captures the overall variability in the heart rate across the entire recording period, including all the cyclic fluctuations. RMSSD reflects vagal tone. The pNN50 metric is correlated with both RMSSD and the HF, and it also serves as an indicator of the parasympathetic activity (7). ULF reflects overall autonomic activity, including core body temperature, metabolism and the renin-angiotensin system. VLF is an indicator of the combined activity of both the sympathetic and parasympathetic nervous systems, with a notable predominance of sympathetic influence. HF reflects the highest level of efferent parasympathetic (vagal) influence on the sinus node. LF reflects a mix of sympathetic and vagal nerve effects, it shows the influence of sympathetic and parasympathetic nerve branches. A shared aspect of these HRV parameters is their emphasis on autonomic nervous function, an area that is also central to the majority of HRV research studies. Despite a lack of consensus, cumulative studies have shown that lower HRV is reflective of autonomic nervous dysautonomia (8).
HRV is a widely utilized method for evaluating the state of the autonomic nervous system (9). Previous studies have shown that the autonomic nervous system is related to self-regulation in aspects such as cognition, emotion, social and health (10, 11). Therefore, the relationship between HRV and global cognitive performance is emerging as a hot topic of research. Multiple studies have established a connection between the autonomic nervous system and cognitive processes, showing that there is a beneficial association between cognitive performance and HRV (12–14). However, there has been controversy about the relationship between HRV and its connection to the broad cognitive domain. As is widely known, the cognitive domain encompasses a variety of mental functions, including but not limited to the following key areas: attention, memory, language, learning ability and visuospatial function. Hanse et al. (15) and Colzato et al. (16) found that higher HRV was associated with better memory and attention but other studies concluded that the relationship between HRV and memory was not significant (17). Britton et al. (18) enrolled 5,375 middle-aged individuals and found that reduced HRV was not related to cognitive disorders in the middle-aged population. From the studies aforementioned, many of these inconsistencies are probably due to diverse populations studied, inconsistency in HRV measurement or the different components of the cognitive domains included in these studies (4). Consequently, a quantitative evaluation method is needed.
Neuroimaging analysis can provide quantitative information of brain structure. Over the past years, despite the fact that voxel-based morphometry (VBM) and functional magnetic resonance imaging (fMRI) has been used to investigate the association between HRV and global cognitive performance (5, 19, 20), the signal of fMRI is susceptible to the influence of blood flow changes and heart rate (21), and VBM is not sensitive to subtle changes for brain structure. Diffusion imaging methods, such as neurite orientation dispersion and density imaging (NODDI) could be used to measure WM microstructure differences and how they were associated with cognitive impairment (22, 23). The microstructural environments of WM fiber bundle could be measured by neurite density index (NDI), orientation dispersion index (ODI), and fractional isotropy (FISO). Recently, WM is gradually becoming a focus of interest in HRV researches. Yu et al. (24) believes that cardiovascular diseases are risk factors for white matter hyperintensities. One recent study has shown that an inverse association between low nighttime HRV and white matter hyperintensities progression, and provide support for the role of sympathetic overactivity in this relationship (25). Tian et al. (26) also found that reduced RMSSD was associated with white matter hyperintensity. We speculate that changes in the autonomic nervous system may lead to alterations in the WM. Notably, a few studies (27, 28) consider cardiac autonomic dysfunction is associated with cognitive decline, but its underlying pathophysiological mechanisms may be related to white matter lesions in the brain. Therefore, we postulate the following hypothesis: HRV is associated with microstructural changes in WM. Fortunately, NODDI is more sensitive to the density of neurites and the degree of fiber used to describe changes in WM microstructure (29). Unlike DTI, NODDI has multi-compartment biophysical model representing each voxel and three different microstructural environments (30).
As far as we know, scarce studies were available to employ the NODDI model to synthesize assessments of WM damage in low HRV patients, with insufficient attention directed toward elucidating the correlation of cognitive implications in low HRV with combined WM damage. Examining the impact of white matter integrity may help elucidate how HRV impacts brain processes, including memory, attention, language, visuospatial function, and the sensorimotor system. These findings may provide novel insights into the relationship between HRV and cognition.
2 Materials and methods
2.1 Ethical approval statement
All procedures performed in studies involving human participants followed the ethical standards of the institutional research committee with the 1964 Helsinki Declaration and its later amendments or comparable ethical standards. The study protocol was approved by the Ethics Committee of Fujian Medical University Union Hospital (2021KJT001).
2.2 Study subjects
The enrollment process and flowchart are depicted in Figure 1. Participants in this study were consecutively recruited from the Cognitive Psychology Outpatient Department of Fujian Union Hospital from May 2022 to February 2023. Participants voluntarily sought cognitive testing with concerns about their own cognitive functions. The inclusion criteria required that participants have no significant cognitive deficits on standardized tests and clinical assessments. Participants went through a magnetic resonance imaging (MRI) examination on the day they completed the neuropsychological assessment. All participants signed informed consent forms before the MRI examination. Exclusion criteria as follows: other neurological disorders, cognitive impairment, obesity, severe heart disease, arrhythmias, atrial fibrillation, respiratory system diseases, mental illnesses (i.e., schizophrenia or depression), severe endocrine disorders, injuries or surgeries within the past 6 months, use of illegal anesthetics or alcohol abuse, uncorrected vision or hearing, pregnancy or breastfeeding, use of psychotropic drugs, anesthetic analgesics, benzodiazepines, and psychoactive substances were excluded from the study. Additionally, participants were instructed to abstain from caffeine and nicotine for at least 4 h and to avoid alcohol consumption for at least 12 h. Demographic characteristics were collected, including age, sex, education, and Fazekas scales.
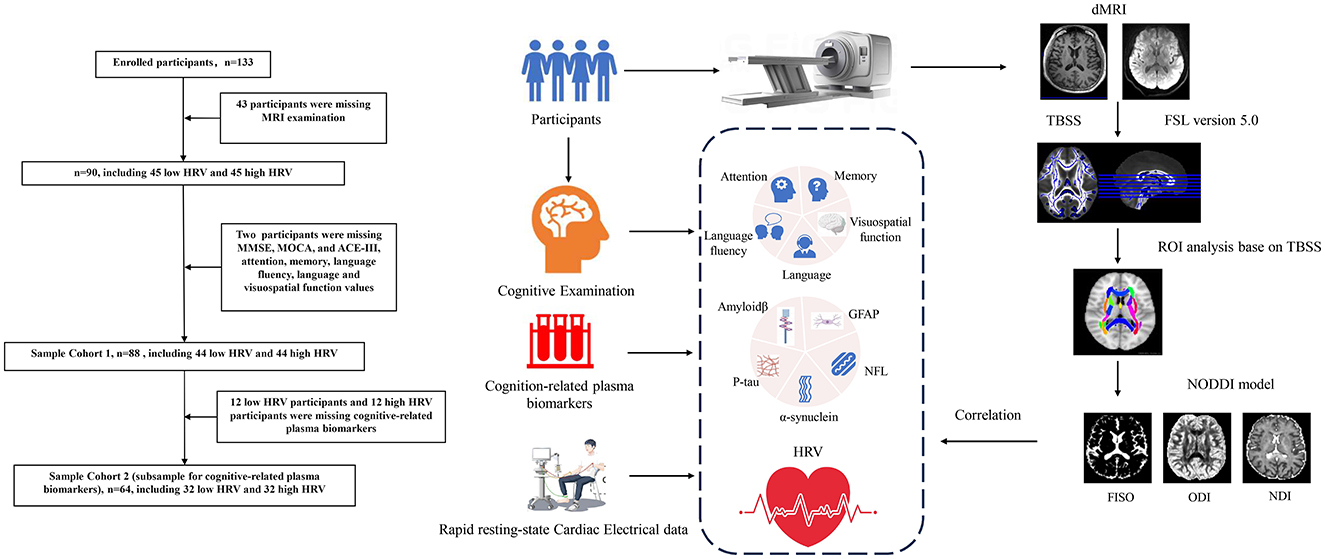
Figure 1. The enrollment process and flowchart of the participant. NODDI, neurite orientation dispersion and density imaging; TBSS, tract-based spatial statistics; HRV, heart rate viability; BPM, beat per minute; RMSSD, the root mean square of successive differences; SDNN, the standard deviation of all NN intervals; SDSD, the standard deviation of the differences between consecutive NN intervals; TP, total power; HF, high frequency; LF, low frequency; VLF, very low frequency; ULF, ultra-low frequency; pNN50, percentage of adjacent intervals that varied by >50 ms; MMSE, mini-mental state examination; MOCA, Montreal Cognitive Assessment; ACE-III, Addenbrooke's Cognitive Examination version III; RCFT-I, Rey Complex Figure Test-Immediate recall; RCFT-D, Rey Complex Figure Test-Delayed recall; CDR, Clinical Dementia Rating; Aβ42, Amyloidβ-42; Aβ40, Amyloidβ-40; GFAP, glial fibrillary acidic protein; NFL, Neurofilament light chain; P-tau, phospho-tau181.
2.3 Assessment of cognitive function
Global cognition, including Clinical Dementia Rating (CDR), Mini-mental State Examination (MMSE), Montreal Cognitive Assessment (MOCA), Rey Complex Figure Test-Immediate recall (RCFT-I), Rey Complex Figure Test-Delayed recall (RCFT-D) and Addenbrooke's Cognitive Examination version III (ACE-III) scales were conducted by a neurologist with 5 years of experience. Addenbrooke's Cognitive Examination version III (ACE-III) scales were used to assess cognitive function in five subdomains, including attention, memory, language, language fluency and visuospatial function (31).
2.4 Determination of plasma biomarkers
Blood samples were collected into EDTA-coated vacuum containers. After centrifugation, blood samples were stored at −80°C and thawed immediately before composition quantification. The levels of plasma amyloid β-42 (Aβ42), amyloid β-40 (Aβ40), glial fibrillary acidic protein (GFAP), neurofilament light chain (NFL), phospho-tau 181 (P-tau) and α-synuclein were measured using the Human Neurology 3-Plex A Assay (N3PA) kit on an automatic single-molecule-array (SIMOA) instrument (Quanterix Corp, MA, USA) according to the manufacturer's protocol.
2.5 MRI data acquisition
All the images were acquired using a 3T MR scanner (MAGNETOM Prisma, Siemens Healthcare) with a 64-channel head coil. The foam was placed around the head of each participant before the test to reduce head movement. All participants were asked to refrain from moving their heads during the MRI scan. Diffusion-weighted images were obtained by using a multi-shell echo-planar imaging sequence, which consisted of four b-values (0, 1,000, 2,000, and 3,000 s/mm2) along 6, 30, 30, and 30 gradient directions, respectively. Seventy-two slices with a thickness of 2 mm were used. The other scan parameters were as follows: repetition time (TR), 5,800 ms; time to echo (TE), 91 ms; field-of-view (FOV), 215 × 215 mm2; GeneRalized Autocalibrating Partial Parallel Acquisition, 2; slice acceleration factor, 2; number of averages, 1; voxel size, 2 × 2 × 2 mm3, without gap; and acquisition time, 9 min 44 s. High-resolution T1-weighted images were acquired using a Magnetization Prepared-Rapid Gradient Echo sequence with the following parameters: TR, 2,300 ms; TE, 2.32 ms; FOV, 240 × 240 mm2; number of averages, 1; voxel size, 0.9 × 0.9 × 0.9 mm3,192 slices; and acquisition time, 4 min 44 s. We used parallel imaging and phase partial fourier (7/8) to accelerate the scanning speed of the MP-RAGE sequence.
2.6 Postprocessing of MRI data
All images were scrutinized and any affected by artifacts, noise, or head movement were excluded. The remaining images were processed into raw, preprocessed files with specific indices. (1) The initial DICOM format images were converted into NIFTI files using the MRIcron software package (http://www.nitrc.org/projects/mricron). (2) An average b = 0 s/mm2 image from each diffusion-weighted shell served as a reference for subsequent steps. (3) We performed motion and eddy current correction using FSL's eddy tool in the FMRIB's Diffusion Toolbox (FDT). (4) Brain mask generation using a brain extraction tool (BET) and distortion correction via registration of individual T1W and dMRI data. (5) We used visual inspection to avoid severe geometric distortions, ensuring that all images met the quality control criteria before further analysis. (6) The FMRIB's Diffusion Toolbox (FSL-FDT, included in FSL version 5.0 (http://fsl.fmrib.ox.ac.uk/fsl/fslwiki/FDT), was utilized to remove the skull from the images. Following this, the NODDI parameters (including NDI, ODI, and FISO) were calculated with NODDI_toolbox (www.nitrc.org/projects/noddi_toolbox) in MatLab R2022b (The MathWorks Inc, Natick, MA, USA).
2.7 TBSS and ROI analysis
Tract-based spatial statistics (TBSS, part of FSL version 5.0, http://fsl.fmrib.ox.ac.uk/fsl/fslwiki/TBSS/UserGuide) was conducted with the FSL software. In our TBSS analysis pipeline, the skeleton projection of NDI, ODI, and FISO were based on the FA skeleton, which is consistent with the standard TBSS method. The main steps were as follows: 1) All individual FA images were non-linearly registered to the FMRIB58_FA template using FNIRT (FMRIB's Non-linear Registration Tool). The FMRIB58_FA template is already in Montreal Neurological Institute (MNI) 152 space standard space.; 2) An average FA image was created from continuous scan images of all subjects in this common space and refined to generate an average WM skeleton representing the center of all fiber bundles with a threshold >0.2; 3) Each subject's FA data is then projected onto a mean white matter skeleton by assigning each skeleton voxel the highest FA value from the local perpendicular tract, ensuring alignment across subjects. The NDI, ODI and FISO images were subjected to the same non-linear deformations at the individual level and projected onto the FA skeleton using nearest-neighbor projection. This ensures that the projection points for all indices are consistent with the voxels of the FA skeleton. Therefore, the values of NDI, ODI, and FISO on the skeleton originated from the same spatial locations, avoiding the situation where projection points for different indices come from different voxels; 4) The FSL was used to conduct double independent sample t-test and replacement test between the two groups (replacement times 5,000 times), and the differences in the NDI, ODI and FISO values of the WM between the two groups were detected. For the presentation of differences in specific brain regions, the ROIs from Johns Hopkins University ICBM-DTI-81 White-Matter Labels (32) were used in this analysis. The images metrics for NODDI were transformed to MNI152 space, and then the mean values of FISO, NDI, and ODI were extracted from each tract. Age and sex were added as covariates. The false discovery rate (FDR) was used for multiple-comparison corrections.
2.8 Acquisition of rapid resting-state cardiac electrical data
The assessments were conducted in a designated and tranquil room. All participants were mandated to obtain a restful night's sleep of 6 to 8 h before the examination. The data was consistently gathered at the same time in the early morning while participants were fasting. Before the tests, individuals were asked to sit in a relaxed position for a 5-min period. They were instructed to keep their eyes closed, remain conscious, and wear noise-canceling headphones. In the meantime, a 3-min segment of resting-state electrocardiogram (ECG) was simultaneously recorded.
Electrodes for limb leads (aVR, aVL, and aVF) were attached to the patient's wrists and one ankle to capture cardiac electrical activity. The KARDi2/4-B Autonomic Function Mapping ECG system, manufactured by NeuroMed in China, was utilized for both recording and analyzing the subtle millivolt-level fluctuations within the ECG signal. The system automatically identifies and calculates the mean of the three RR intervals preceding and following any normal beat, and applies markers to refine the data by excluding ectopic beats. Incorporating the Pan-Tompkins algorithm integrated within the system, it performed an analysis of time-domain heart rate variability (HRV) indices, such as pNN50, RMSSD, SDNN, and SDSD, as well as frequency-domain indices, which encompass TP, HF, LF, VLF, and ULF. Participants were split in two groups on the basis of HF [median = 111.97 ms2; low HRV = 40.99 ms2 (median =46.39 ms2); high HRV = 235.41 ms2 (median = 156.86 ms2)] as it has been recommended (4) and extensively used (33, 34).
2.9 Statistical analysis
All statistical analyses were performed using SPSS (Version 26.0; Chicago, Illinois, USA). All statistical analyses were defined as a two-sided p < 0.05. Data were tested for normal distribution by the Kolmogorov-Smirnov test. Continuous variables were expressed as the mean ± standard deviation by t-test. The non-normal distribution variables were expressed as median (interquartile range, IQR) and compared by a non-parametric test. The Chi-squared and Fisher's exact tests were used to compare categorical variables. Finally, Pearson's correlation analysis and partial correlation analysis (controlling for sex, age and total years of education) were utilized to determine associations between abnormal WMs and HRV, and cognitive function. The statistical significance threshold was set at p < 0.05.
3 Results
3.1 Demographic characteristics of participants
Sample cohort 1 included 44 Low HRV, and 44 High HRV participants (Table 1). The table showed no significant differences in sex, education, Fazekas scales, MMSE, CDR, attention, language fluency, and language (p > 0.05), but significant differences in age, BPM, RMSSD, SDSD, TP, HF, LF, VLF, ULF, pNN50, MOCA, ACE-III, RCFT-I, RCFT-D, memory, and visuospatial function between the two groups (p < 0.01).
Sample cohort 2 included 32 low HRV, and 32 high HRV participants, the table showed no significant differences in sex, education, Aβ42, Aβ40, P-tau, GFAP and α-synuclein (p > 0.05), but significant differences in age and Aβ42/Aβ40 between the two groups (p < 0.05; Table 2).
3.2 The results of TBSS analysis (sample cohort 1)
TBSS statistical analysis showed significant differences in FISO in several cerebral regions between low HRV and high HRV group, including the left and right anterior corona radiata (ACR), Body of corpus callosum (CCB), Genu of corpus callosum (CCG), Splenium of corpus callosum (CCS), left superior corona radiata (SCR), which were hereinafter referred to as comprehensive abnormal fiber bundles (p < 0.05).
There was no significant difference in the NDI and ODI values between the two groups (p > 0.05; Figure 2, Table 3).
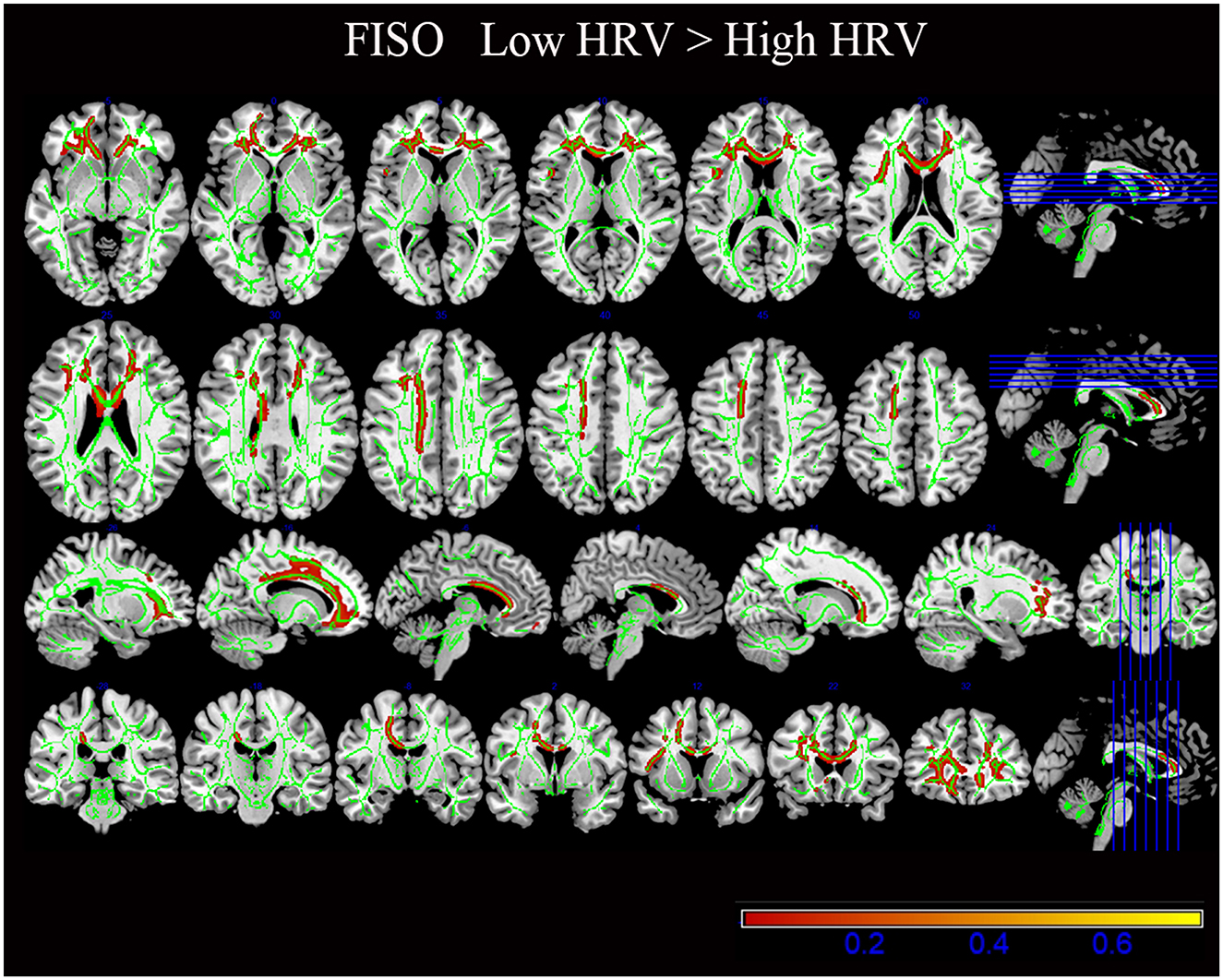
Figure 2. The results of diffusion metrics by tract-based spatial statistics (TBSS). Compared with the High HRV group, the white matter regions of the Low HRV patients showed increased FISO (P < 0.05). FISO, isotropic volume fraction; HRV, heart rate viability.
3.3 ROI analysis results based on TBSS analysis (sample cohort 1)
Compared with the High HRV group, FISOs of CCB, CCG, fornix (FX), left sagittal stratum (SS), bilateral anterior limb of internal capsule (ALIC), left external capsule (EC) and left superior fronto-occipital fasciculus (SFOF) in the low HRV group increased to different degrees; (p < 0.05; Figure 3). In addition, no significant differences in other fiber bundle indicators were observed between the groups (p > 0.05).
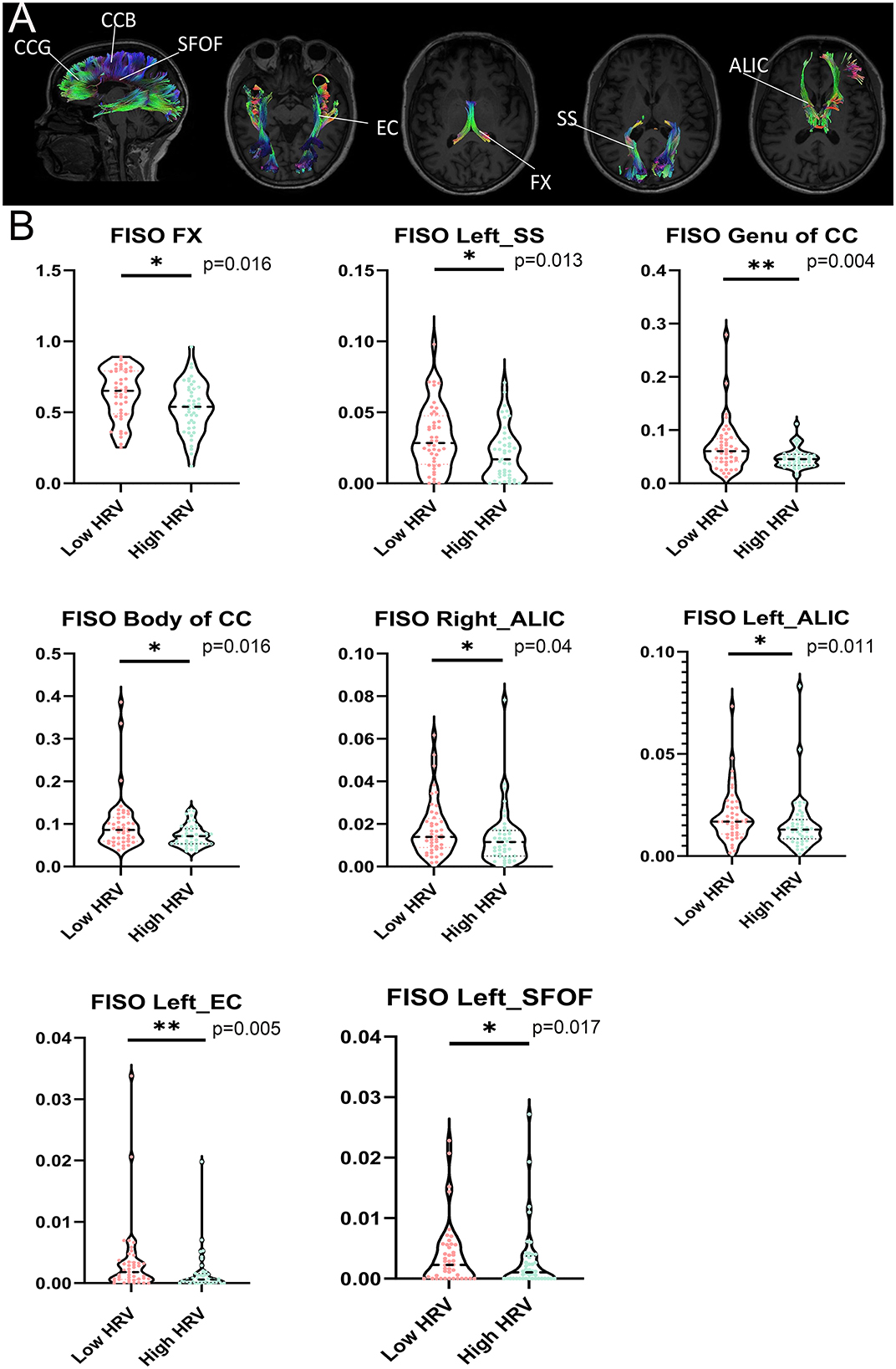
Figure 3. (A) Significant tracts from tract-of-interest analysis comparing between low HRV and High HRV. Tracts obtained using the JHU White Matter (WM) Tractography Atlas. (B) Violin plots show the important fiber bundles of FISO in the region of interest (ROI) by cluster-based spatial statistics (TBSS). False discovery rate (FDR) correction was performed for multiple comparisons, with statistical significance of P < 0.05. HRV, heart rate viability; CCB, Body of corpus callosum; CCG, Genu of corpus callosum; FX, fornix; SS, sagittal stratum; ALIC, anterior limb of internal capsule; EC, external capsule; SFOF, left superior fronto-occipital fasciculus. **P < 0.01, *P < 0.05.
3.4 Association of aberrant NODDI indicators with cognition (sample cohort 1)
Pearson's correlation analysis showed that abnormalities in the WM microstructure were closely associated with a wide range of cognitive domains (p < 0.05; Figure 4a).
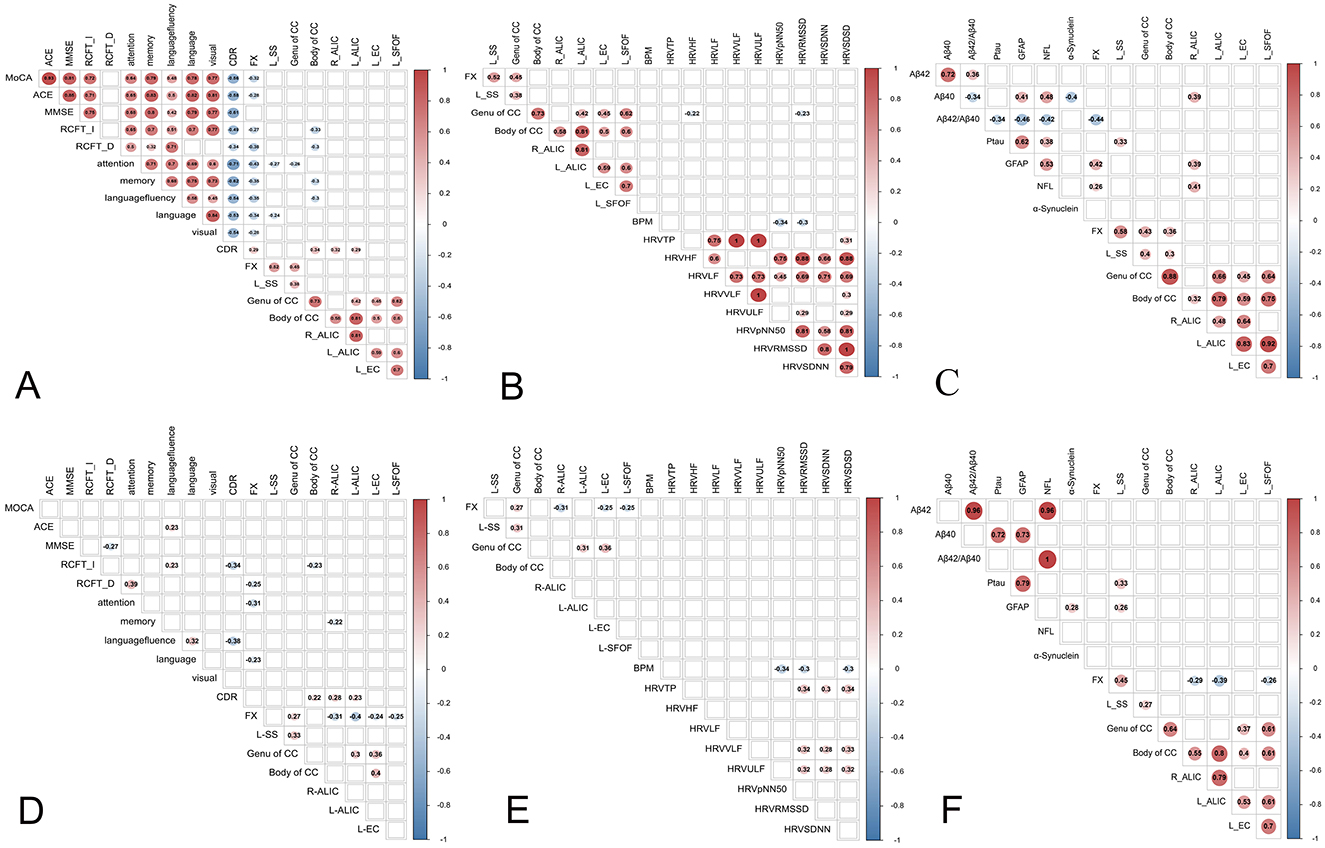
Figure 4. The results of pearson's correlation analysis (a–c) and partial correlation analysis (d–f) between abnormal WMs with cognitive function, heart rate viability (HRV) and cognition-related biomarkers. (a) Correlation between the abnormal fiber bundles and cognitive function. (b) Correlation between the abnormal fiber bundles and HRV. (c) Correlation between abnormal fiber bundles and cognition-related plasma biomarkers. (d) Partial correlation analysis between abnormal fiber bundles and cognition-related plasma biomarkers. (e) Partial correlation analysis between abnormal fiber bundles and HRV. (f) Partial correlation analysis between abnormal fiber bundles and cognition-related plasma biomarkers. HRV, heart rate viability; BPM, beat per minute; RMSSD, the root mean square of successive differences; SDNN, the standard deviation of all NN intervals; SDSD, the standard deviation of the differences between consecutive NN intervals; TP, total power; HF, high frequency; LF, low frequency, VLF, very low frequency; ULF, ultra-low frequency; pNN50, percentage of adjacent intervals that varied by >50 ms; MMSE, mini-mental state examination; MOCA, Montreal Cognitive Assessment; ACE-III, Addenbrooke's Cognitive Examination version III; RCFT-I, Rey Complex Figure Test-Immediate recall; RCFT-D, Rey Complex Figure Test-Delayed recall; CDR, Clinical Dementia Rating; Aβ42, Amyloidβ-42; Aβ40, Amyloidβ-40; GFAP, glial fibrillary acidic protein; NFL, Neurofilament light chain; P-tau, phospho-tau 181; CCB, Body of corpus callosum; CCG, Genu of corpus callosum; FX, fornix; SS, sagittal stratum; ALIC, anterior limb of internal capsule; EC, external capsule, SFOF, left superior fronto-occipital fasciculus.
Partial correlation analysis revealed that the FISO of CCB, right ALIC and left ALIC were positively associated with CDR, the FISO of FX was associated with RCFT-D, attention and language, the FISO of CCB was negatively associated with RCFT-I, the FISO of right ALIC were negatively associated with memory (p < 0.05; Figure 4d). Furthermore, no association was found between other fiber bundle indexes and cognitive function (p > 0.05; Figure 4d).
3.5 Association of aberrant NODDI indicators with HRV (sample cohort 1)
Pearson's correlation analysis showed that the FISO of CCG were negatively associated with RMSSD and SDSD (p < 0.05; Figure 4b).
Partial correlation analysis revealed that no association was found between fiber bundle indexes and HRV (p > 0.05; Figure 4e).
3.6 Association of aberrant NODDI indicators with cognition-related plasma biomarkers (sample cohort 2)
Pearson's correlation analysis found that the FISO of FX was negatively associated with Aβ42/Aβ40 and positively associated with NFL, GFAP and p-tau 181.The FISO of left SS and CCB were positively associated with p-tau 181. The FISO of right ALIC was positively associated with NFL, GFAP and p-tau 181 (p < 0.05; Figure 4c).
Partial correlation analysis revealed that the FISO of FX was positively associated with GFAP. The FISO of left SS and CCG were positively associated with p-tau 181 (p < 0.05; Figure 4f). The scatter plot was used to display the correlation between Fractional Isotropic Volume Fraction (FISO) and cognitive function, as well as cognition-related plasma biomarkers (Supplementary Figure S1).
4 Discussion
In this study, we utilized NODDI to analyze variations in the integrity and microstructure of WM in participants with low HRV and high HRV. We found that participants in the low HRV group exhibited WM discrepancies in various regions and severities, and these microstructure changes were associated with cognitive function, cognition-related plasma biomarkers to varying degrees. Taken together, these findings indicate that microstructural abnormalities in WM may be indicative of underlying histopathological changes linked to cognitive decline. These findings may provide novel insight into the effects of HRV on cognitive domains.
NODDI, as an innovative diffusion MRI approach, has the three most commonly derived NODDI metrics (ODI, NDI, and FISO). FISO reflects the content of free water in tissue, which is used to quantify the volume of voxels occupied by free-flowing cerebrospinal fluid (35). Cumulative studies have shown that the increase in FISO may indicate a decrease in the microstructural integrity of the WM (29, 36, 37). In this study, we found several microstructural anomalies in FISO, which implies axonal loss in the WM is the primary pathological change in the low HRV group. Indeed, the potential white matter tract injury may begin with axonal loss (38). Besides, the elevated FISO levels suggest an expansion of the extracellular fluid volume, a condition typically associated with neuroinflammatory (39) which has been suggested to contribute to neurodegenerative diseases and cognitive dysfunction (40).
Notably, TBSS and ROI analysis showed that the abnormal WM integrity was mainly manifested in the anterior regions of the brain and corpus callosum (CC), mainly linked to the frontal and corpus callosum. Our findings seem to support this theory of the frontal-corpus callosum pathways. The frontal region is a complex brain region through which a variety of WM fibers pass, mainly connecting and associative fibers. Multiple previous studies have confirmed that the front of the brain is related to attention, memory, motion, language, executive function (41). Damage or dysfunction of the frontal or corpus callosum may lead to impairment of any one or several of the aforementioned functions, which could significantly affect an individual's daily life and social functioning (41, 42). ROI analysis also found that the FISO values of FX, CCB, right ALIC and left ALIC were associated with the cognitive function and broad cognitive domains to varying degrees, which is consistent with the previous findings (43, 44). It seems that low HRV are prone to affect the WM in the anterior regions of the brain. This can be explained by several potential mechanisms. Changes in the integrity of the fibers (right and left ALIC, CC) in the regions associated with autonomic nervous function, such as the corpus callosum and basal ganglia (45), further support the basic theory that HRV is closely linked to the dysfunction of the autonomic regulatory regions. The frontal cortex is also the main region of autonomic regulation (46). ALIC is an important white matter structure in the brain that carries nerve fibers from the cerebral cortex to the brain stem. The brainstem is the key part of autonomic regulation (45). EC and SFOF are involved in connecting the cerebral cortex to deeper brain structures, including those involved in autonomic nervous system (ANS). Therefore, we speculate that the damage to WM in low HRV patients might result from the injury of ANS. In addition, despite a lack of consensus, cumulative studies have shown that low HRV were associated with levels of brain-derived neurotrophic factor (BDNF) in the frontal cortex (47, 48). BDNF is a widely distributed protein in the central nervous system that plays a critical role in the growth, survival, and differentiation of neurons (48). HRV could influence BDNF concentrations within the frontal cortex, potentially causing degeneration in white matter.
Although the microstructural changes detected by NODDI could not directly prove low HRV with cognitive impairments, our study results showed that abnormal fiber bundles in WM of low HRV group were associated with cognitive-related regions. This damage may lead to decreased efficiency in information transfer, thereby affecting the normal functioning of these cognitive abilities. Previous studies had also confirmed that WM damage in cognitive-related regions is closely related to the decline in executive function, memory, and attention, such as the corpus callosum, fornix, and internal capsule. A host of studies have documented the association of FX with long-term memory and spatial memory (49), that of SS with visual memory and spatial cognition (50), the role of SFOF in visual processing and attention (51), the role of CCB and CCG in attention, memory, and emotional processing (52), and that of EC and ALIC in memory and executive function (53). In a similar line, the damaged WM areas were also associated with neurological diseases which also supports that low HRV contributes to the occurrence of cognitive diseases (54, 55). Furthermore, most of the cognitive assessments showed significant differences between groups in our study (Table 1), which also confirmed the trend of cognitive decline in individuals with low HRV. This is similar to the findings of a recent study (56). Although MMSE, attention, and language did not show significant differences between groups, Cohen's d measurements (Supplementary Table S1) revealed small to medium effect sizes, indicating a trend toward cognitive decline. Additionally, WM abnormalities in FISO were only detected between groups, and FISO is known to be sensitive to early WM damage (57). We speculate that individuals with low HRV may be in the early stages of cognitive decline.
Our study also found a significant difference in the Aβ42/Aβ40 ratio between the Low HRV group and the High HRV group. The decreased Aβ42/Aβ40 ratio may indicate that individuals in the low HRV group are more susceptible to cognitive impairment. The mechanism may be related to neuroinflammation and vascular regulation. First, amyloid beta may be associated with neuroinflammation (58) and the changes in both amyloid beta and neuroinflammation tend to happen relatively early in the process, perhaps even before signs of cognitive impairment (59). We speculate that low HRV may be associated with higher inflammatory markers or may have a role in decreased clearance of Aβ42. Second, autonomic nervous system (ANS) imbalance caused by reduced HRV can exacerbate neuroinflammatory responses. Dong-Hun Lee found that the ANS and the hypothalamic-pituitary-adrenal (HPA) axis can induce excessive inflammatory responses in the central nervous system (CNS), leading to neuroinflammation (60), a finding also confirmed by Aleksandar Sic's study (61). HRV, an indicator of autonomic nervous system regulation of cardiovascular function, might affect cerebral blood flow and blood-brain barrier function, reducing the clearance efficiency of Aβ (62) and accelerating the deposition of Aβ42 in the vascular walls, which further lowers the Aβ42/Aβ40 ratio.
Although the intergroup difference of NFL did not reach the threshold of statistical significance, its trend is consistent with other indicators of neurodegeneration and cognitive decline. Reduced HRV may exacerbate neuronal stress through chronic inflammation or vascular damage (63), leading to increased release of NFL (64). Additionally, studies have found that abnormal Aβ metabolism can further lead to increased NFL release by promoting neuronal damage (65). We speculate that HRV may establish a “double-hit” mechanism on AD pathology: simultaneously promoting amyloid toxicity (decreased Aβ42/Aβ40) and neurodegeneration (increased NFL).
Strengths of our study included the recruitment of subjects with complete clinical, neuropsychological data, and plasma biomarker data, which were integrated with DWI data. In addition, for the first time, our study applied NODDI, which facilitates an accurate assessment of the WM integrity in low HRV patients. We also explain the mechanism by which HRV leads to cognitive decline from the perspective of the integrity of WM.
Our study was not without limitations. First, the association between WM integrity and cognition-related plasma biomarkers was investigated in a small sample. Second, the results were derived from Chinese population and caution needs to be taken when they are generalized to other populations. Third, owing to its cross-sectional design, selection bias was inevitable. Fourth, the MNI152 template is mostly applied to young individuals. Although the subjects in this study were older, we employed rigorous visual inspection and eddy current correction to avoid misregistration. In future work, we will attempt to use more appropriate templates for our population (such as “most representative subject” in https://fsl.fmrib.ox.ac.uk/fsl/docs/#/diffusion/tbss?id=user-guide or study-specific templates in https://dti-tk.sourceforge.net/pmwiki/pmwiki.php?n=Documentation). Fifth, EPI-induced geometric distortion correction was not applied due to the lack of available field maps or reverse phase encoding acquisitions. However, we performed visual inspection of all diffusion images to ensure that no severe distortions were present, and all images met the quality control criteria. Future studies could benefit from incorporating EPI distortion correction techniques, to further improve the accuracy of white matter microstructural analysis. Sixth, considering that white matter hyperintensities (WMH) may affect the measured NODDI metrics, we will further investigate WMH volume in future work to better understand these differences.
In conclusion, our study evidences that the microstructural changes of WM in Low HRV are associated with cognitive domains and cognition-related plasma biomarkers. Low HRV may lead to worse cognitive performance through the damage of the genu of the corpus callosum pathway.
Data availability statement
The original contributions presented in the study are included in the article/Supplementary material, further inquiries can be directed to the corresponding author.
Ethics statement
The studies involving humans were approved by the Ethics Committee of Fujian Medical University Union Hospital (2021KJT001). The studies were conducted in accordance with the local legislation and institutional requirements. The participants provided their written informed consent to participate in this study. Written informed consent was obtained from the individual(s) for the publication of any potentially identifiable images or data included in this article.
Author contributions
SJ: Data curation, Formal analysis, Investigation, Writing – original draft. YC: Formal analysis, Investigation, Visualization, Writing – original draft. LL: Investigation, Visualization, Writing – original draft. RJ: Formal analysis, Investigation, Visualization, Writing – original draft. XP: Conceptualization, Data curation, Formal analysis, Funding acquisition, Investigation, Supervision, Writing – original draft. YX: Conceptualization, Project administration, Writing – review & editing.
Funding
The author(s) declare that financial support was received for the research and/or publication of this article. This work was supported by the Guiding project of Fujian province (NO.2021Y0020), the Natural Science Foundation of Fujian Province (No. 2020Y9057), and the Startup Fund for Scientific Research, Fujian Medical University (No.2020QH1069).
Acknowledgments
We would like to thank all the participants and Fujian Medical University Union Hospital for their support and collaboration in data collection and management.
Conflict of interest
The authors declare that the research was conducted in the absence of any commercial or financial relationships that could be construed as a potential conflict of interest.
Generative AI statement
The author(s) declare that no Gen AI was used in the creation of this manuscript.
Publisher's note
All claims expressed in this article are solely those of the authors and do not necessarily represent those of their affiliated organizations, or those of the publisher, the editors and the reviewers. Any product that may be evaluated in this article, or claim that may be made by its manufacturer, is not guaranteed or endorsed by the publisher.
Supplementary material
The Supplementary Material for this article can be found online at: https://www.frontiersin.org/articles/10.3389/fneur.2025.1503599/full#supplementary-material
Supplementary Figure S1 | The correlation between FISO and cognitive function, as well as cognition-related plasma biomarkers. FISO, fractional isotropy; RCFT-I, Rey Complex Figure Test-Immediate recall; RCFT-D, Rey Complex Figure Test-Delayed recall; CDR, Clinical Dementia Rating global rating; GFAP, glial fibrillary acidic protein; P-tau, phospho-tau 181.
Supplementary Table S1 | The Cohen's d of cognitive assessments between groups. Cohen's d is a standardized measure used to quantify the difference in means between two groups and is commonly employed to assess effect size. Specifically, d = 0.2 indicates a small effect, d = 0.5 indicates a medium effect, and d = 0.8 indicates a large effect. The formula for calculating Cohen's d: Cohen's d = M1 and M2 are the means of the two groups of data; Spooled is the pooled standard deviation. . N1 and N2 are the sample sizes of the two groups of data; SD1 and SD2 are the standard deviations of the two groups of data.
References
1. Shaffer F, Ginsberg JP. An overview of heart rate variability metrics and norms. Front Public Health. (2017) 5:258. doi: 10.3389/fpubh.2017.00258
2. Billman GE. Heart rate variability? A historical perspective. Front Physiol. (2011) 2:86. doi: 10.3389/fphys.2011.00086
3. Shaffer F, McCraty R, Zerr CL. A healthy heart is not a metronome: an integrative review of the heart's anatomy and heart rate variability. Front Psychol. (2014) 5:1040. doi: 10.3389/fpsyg.2014.01040
4. Laborde S, Mosley E, Thayer JF. Heart rate variability and cardiac vagal tone in psychophysiological research – recommendations for experiment planning. Data analysis, and data reporting. Front Psychol. (2017) 8:213. doi: 10.3389/fpsyg.2017.00213
5. Tupitsa E, Egbuniwe I, Lloyd WK, Puertollano M, Macdonald B, Joanknecht K, et al. Heart rate variability covaries with amygdala functional connectivity during voluntary emotion regulation. NeuroImage. (2023) 274:120136. doi: 10.1016/j.neuroimage.2023.120136
6. Alharbi EA, Jones JM, Alomainy A. Non-invasive solutions to identify distinctions between healthy and mild cognitive impairments participants. IEEE Journal of Translational Engineering in Health and Medicine. (2022) 10:1–6. doi: 10.1109/JTEHM.2022.3175361
7. Abdelbasset WK, Picard M, Tauveron I, Magdasy S, Benichou T, Bagheri R, et al. Effect of exercise training on heart rate variability in type 2 diabetes mellitus patients: a systematic review and meta-analysis. PLoS ONE. (2021) 16:251863. doi: 10.1371/journal.pone.0251863
8. Shah B, Kunal S, Bansal A, Jain J, Poundrik S, Shetty MK, et al. Heart rate variability as a marker of cardiovascular dysautonomia in post-COVID-19 syndrome using artificial intelligence. Indian Pacing Electrophysiol J. (2022) 22:70–6. doi: 10.1016/j.ipej.2022.01.004
9. Klimontov VV, Myakina NE, Tyan NV. Heart rate variability is associated with interstitial glucose fluctuations in type 2 diabetic women treated with insulin. Springerplus. (2016) 5:337. doi: 10.1186/s40064-016-1932-z
10. Blase K, Vermetten E, Lehrer P, Gevirtz R. Neurophysiological approach by self-control of your stress-related autonomic nervous system with depression, stress and anxiety patients. Int J Environ Res Public Health. (2021) 18:3329. doi: 10.3390/ijerph18073329
11. Schwarz KG, Vicencio SC, Inestrosa NC, Villaseca P, Del Rio R. Autonomic nervous system dysfunction throughout menopausal transition: a potential mechanism underpinning cardiovascular and cognitive alterations during female ageing. J Physiol. (2023) 602:263–80. doi: 10.1113/JP285126
12. Forte G, Favieri F, Casagrande M. Heart rate variability and cognitive function: a systematic review. Front Neurosci. (2019) 13:710. doi: 10.3389/fnins.2019.00710
13. Forte G, Morelli M, Casagrande M. Heart rate variability and decision-making: autonomic responses in making decisions. Brain Sci. (2021) 11:243. doi: 10.3390/brainsci11020243
14. Gathright EC, Walter FA, Hawkins MAW, Spitznagel MB, Hughes JW, Gunstad J. Executive function moderates the relationship between depressive symptoms and resting heart rate variability in heart failure. J Behav Med. (2015) 39:192–200. doi: 10.1007/s10865-015-9684-8
15. Hansen AL, Johnsen BH, Thayer JF. Vagal influence on working memory and attention. Int J Psychophysiol. (2003) 48:263–74. doi: 10.1016/S0167-8760(03)00073-4
16. Colzato LS, Jongkees BJ, de Wit M, van der Molen MJW, Steenbergen L. Variable heart rate and a flexible mind: higher resting-state heart rate variability predicts better task-switching. Cogn Affect Behav Neurosci. (2018) 18:730–8. doi: 10.3758/s13415-018-0600-x
17. Stenfors CUD, Hanson LM, Theorell T, Osika WS. Executive cognitive functioning and cardiovascular autonomic regulation in a population-based sample of working adults. Front Psychol. (2016) 7:1536. doi: 10.3389/fpsyg.2016.01536
18. Britton A, Singh-Manoux A, Hnatkova K, Malik M, Marmot MG, Shipley M. The association between heart rate variability and cognitive impairment in middle-aged men and women. Neuroepidemiology. (2008) 31:115–21. doi: 10.1159/000148257
19. Schumann A, Suttkus S, Bär K-J. Estimating resting HRV during fMRI: a comparison between laboratory and scanner environment. Sensors. (2021) 21:766. doi: 10.3390/s21227663
20. Legaz A, Yoris A, Sedeño L, Abrevaya S, Martorell M, Alifano F, et al. Heart–brain interactions during social and cognitive stress in hypertensive disease: a multidimensional approach. Eur J Neurosci. (2020) 55:2836–50. doi: 10.1111/ejn.14979
21. Glover GH. Overview of functional magnetic resonance imaging. Neurosurg Clin N Am. (2011) 22:133–9. doi: 10.1016/j.nec.2010.11.001
22. Fu X, Wang X, Zhang Y, Li T, Tan Z, Chen Y, et al. Brain microstructural changes in patients with amnestic mild cognitive impairment. Clin Neuroradiol. (2022) 33:445–53. doi: 10.1007/s00062-022-01226-2
23. Zhong S, Lou J, Ma K, Shu Z, Chen L, Li C, et al. Disentangling in-vivo microstructural changes of white and gray matter in mild cognitive impairment and Alzheimer's disease: a systematic review and meta-analysis. Brain Imaging Behav. (2023) 17:764–77. doi: 10.1007/s11682-023-00805-2
24. Yu L, Li Y, Wang Y, Gao Y, Li S, Yu W, et al. Association of nocturnal heart rate with white matter hyperintensity burden in cerebral small vessel disease patients. Curr Neurovasc Res. (2021) 18:565–71. doi: 10.2174/1567202619666211231092630
25. Del Brutto OH, Mera RM, Costa AF, Rumbea DA, Recalde BY, Peñaherrera E, et al. Decreased nighttime heart rate variability and progression of white matter hyperintensities of presumed vascular origin. A prospective study in community-dwelling older adults. J Stroke Cerebrovasc Dis. (2022) 31:106479. doi: 10.1016/j.jstrokecerebrovasdis.2022.106479
26. Tian Y, Yao D, Pan Y, Wang M, Meng X, Zhao X, et al. Implication of heart rate variability on cerebral small vessel disease: a potential therapeutic target. CNS Neurosci Ther. (2023) 29:1379–91. doi: 10.1111/cns.14111
27. Galluzzi S, Nicosia F, Geroldi C, Alicandri A, Bonetti M, Romanelli G, et al. Cardiac autonomic dysfunction is associated with white matter lesions in patients with mild cognitive impairment. J Gerontol A Biol Sci Med Sci. (2009) 64:1312–5. doi: 10.1093/gerona/glp105
28. Xue S, Li M-F, Leng B, Yao R, Sun Z, Yang Y, et al. Complement activation mainly mediates the association of heart rate variability and cognitive impairment in adults with obstructive sleep apnea without dementia. Sleep. (2023) 46:zsac146. doi: 10.1093/sleep/zsac146
29. Palacios EM, Owen JP, Yuh EL, Wang MB, Vassar MJ, Ferguson AR, et al. The evolution of white matter microstructural changes after mild traumatic brain injury: a longitudinal DTI and NODDI study. Sci Adv. (2020) 6:eaaz6892. doi: 10.1126/sciadv.aaz6892
30. Evans SL, Dowell NG, Prowse F, Tabet N, King SL, Rusted JM. Mid age APOE ε4 carriers show memory-related functional differences and disrupted structure-function relationships in hippocampal regions. Sci Rep. (2020) 10:3110. doi: 10.1038/s41598-020-59272-0
31. Bruno D, Slachevsky A, Fiorentino N, Rueda DS, Bruno G, Tagle AR, et al. Validación argentino-chilena de la versión en español del test addenbrooke's cognitive examination III para el diagnóstico de demencia. Neurología. (2020) 35:82–8. doi: 10.1016/j.nrl.2017.06.004
32. Hua K, Zhang J, Wakana S, Jiang H, Li X, Reich DS, et al. Tract probability maps in stereotaxic spaces: analyses of white matter anatomy and tract-specific quantification. Neuroimage. (2008) 39:336–47. doi: 10.1016/j.neuroimage.2007.07.053
33. Hinojosa JA, Ruiz-Padial E, Mercado F. In exogenous attention, time is the clue: brain and heart interactions to survive threatening stimuli. PLoS ONE. (2021) 16:243117. doi: 10.1371/journal.pone.0243117
34. Ruiz-Padial E, Thayer JF. Resting heart rate variability and the startle reflex to briefly presented affective pictures. Int J Psychophysiol. (2014) 94:329–35. doi: 10.1016/j.ijpsycho.2014.10.005
35. Anaby D, Shrot S, Belenky E, Ben-Zeev B, Tzadok M. Neurite density of white matter significantly correlates with tuberous sclerosis complex disease severity. NeuroImage. (2022) 35:103085. doi: 10.1016/j.nicl.2022.103085
36. Fu X, Shrestha S, Sun M, Wu Q, Luo Y, Zhang X, et al. Microstructural white matter alterations in mild cognitive impairment and Alzheimer's disease. Clin Neuroradiol. (2019) 30:569–79. doi: 10.1007/s00062-019-00805-0
37. Liu Z, Bian B, Wang G, Tian C, Lv Z, Shao Z, et al. Evaluation of microstructural changes in spinal cord of patients with degenerative cervical myelopathy by diffusion kurtosis imaging and investigate the correlation with JOA score. BMC Neurol. (2020) 20:185. doi: 10.1186/s12883-020-01752-x
38. Jang I, Chun IY, Brosch JR, Bari S, Zou Y, Cummiskey BR, et al. Every hit matters: white matter diffusivity changes in high school football athletes are correlated with repetitive head acceleration event exposure. NeuroImage. (2019) 24:101930. doi: 10.1016/j.nicl.2019.101930
39. Andica C, Kamagata K, Kirino E, Uchida W, Irie R, Murata S, et al. Neurite orientation dispersion and density imaging reveals white matter microstructural alterations in adults with autism. Mol Autism. (2021) 12:48. doi: 10.1186/s13229-021-00456-4
40. Zhao J, Bi W, Xiao S, Lan X, Cheng X, Zhang J, et al. Neuroinflammation induced by lipopolysaccharide causes cognitive impairment in mice. Sci Rep. (2019) 9:5790. doi: 10.1038/s41598-019-42286-8
41. Li X, Chen X, Yu R, Dai L, Ai M, Huang Q, et al. Changes in gray matter volume following electroconvulsive therapy in adolescent depression with suicidal ideation: a longitudinal structural magnetic resonance imaging study. Front Psychiatry. (2022) 13:944520. doi: 10.3389/fpsyt.2022.944520
42. Xie H, Guo Q, Duan J, Jia X, Zhou W, Sun H, et al. Disrupted causal connectivity anchored on the right anterior insula in drug-naive first-episode patients with depressive disorder. Front Psychiatry. (2022) 13:858768. doi: 10.3389/fpsyt.2022.858768
43. Xie H, Zhang Q, Jiang Y, Bai Y, Zhang J. Parkinson's disease with mild cognitive impairment may has a lower risk of cognitive decline after subthalamic nucleus deep brain stimulation: a retrospective cohort study. Front Hum Neurosci. (2022) 16:943472. doi: 10.3389/fnhum.2022.943472
44. Wan Z, Rolls ET, Feng J, Cheng W. Brain functional connectivities that mediate the association between childhood traumatic events, and adult mental health and cognition. eBioMedicine. (2022) 79:104002. doi: 10.1016/j.ebiom.2022.104002
45. Cheng Y, Lin L, Jiang S, Huang P, Zhang J, Xin J, et al. Aberrant microstructural integrity of white matter in mild and severe orthostatic hypotension: a NODDI study. CNS Neurosci Ther. (2024) 30:e14586. doi: 10.1111/cns.14586
46. Du X, Liu S, Jia P, Wang X, Gan J, Hu W, et al. Epidemiology of constipation in elderly people in parts of China: a multicenter study. Front Public Health. (2022) 10:823987. doi: 10.3389/fpubh.2022.823987
47. Dutt R, Shankar N, Srivastava S, Yadav A, Ahmed RS. Cardiac autonomic tone, plasma BDNF levels and paroxetine response in newly diagnosed patients of generalised anxiety disorder. Int J Psychiatry Clin Pract. (2020) 24:135–42. doi: 10.1080/13651501.2020.1723642
48. Tiwari R, Kumar R, Malik S, Raj T, Kumar P. Analysis of heart rate variability and implication of different factors on heart rate variability. Curr Cardiol Rev. (2021) 17:e160721189770. doi: 10.2174/1573403X16999201231203854
49. Senova S, Fomenko A, Gondard E, Lozano AM. Anatomy and function of the fornix in the context of its potential as a therapeutic target. J Neurol Neurosurg Psychiatry. (2020) 91:547–59. doi: 10.1136/jnnp-2019-322375
50. Maldonado IL, Destrieux C, Ribas EC, Siqueira de Abreu Brito Guimarães B, Cruz PP, Duffau H. Composition and organization of the sagittal stratum in the human brain: a fiber dissection study. J Neurosurg. (2021) 135:1214–22. doi: 10.3171/2020.7.JNS192846
51. Meola A, Comert A, Yeh FC, Stefaneanu L, Fernandez-Miranda JC. The controversial existence of the human superior fronto-occipital fasciculus: connectome-based tractographic study with microdissection validation. Hum Brain Mapp. (2015) 36:4964–71. doi: 10.1002/hbm.22990
52. Bubb EJ, Metzler-Baddeley C, Aggleton JP. The cingulum bundle: anatomy, function, and dysfunction. Neurosci Biobehav Rev. (2018) 92:104–27. doi: 10.1016/j.neubiorev.2018.05.008
53. Maldonado IL, Parente de Matos V, Castro Cuesta TA, Herbet G, Destrieux C. The human cingulum: from the limbic tract to the connectionist paradigm. Neuropsychologia. (2020) 144:107487. doi: 10.1016/j.neuropsychologia.2020.107487
54. Nowrangi MA, Rosenberg PB. The fornix in mild cognitive impairment and Alzheimer's disease. Front Aging Neurosci. (2015) 7:1. doi: 10.3389/fnagi.2015.00001
55. Bao Y, Wang Y, Wang W, Wang Y. The superior fronto-occipital fasciculus in the human brain revealed by diffusion spectrum imaging tractography: an anatomical reality or a methodological artifact? Front Neuroanat. (2017) 11:119. doi: 10.3389/fnana.2017.00119
56. Forte G, Casagrande M. Explaining cognitive decline related to hypertension: the role of heart rate variability in the stairway to cognitive impairment. Physiol Behav. (2025) 292:114825. doi: 10.1016/j.physbeh.2025.114825
57. Stein A, Vinh To X, Nasrallah FA, Barlow KM. Evidence of ongoing cerebral microstructural reorganization in children with persisting symptoms following mild traumatic brain injury: a NODDI DTI analysis. J Neurotrauma. (2024) 41:41–58. doi: 10.1089/neu.2023.0196
58. Azargoonjahromi A. The duality of amyloid-β: its role in normal and Alzheimer's disease states. Mol Brain. (2024) 17:44. doi: 10.1186/s13041-024-01118-1
59. Wang J, Qiao F, Shang S, Li P, Chen C, Dang L, et al. Elevation of plasma amyloid-β level is more significant in early stage of cognitive impairment: a population-based cross-sectional study. J Alzheimers Dis. (2018) 64:61–9. doi: 10.3233/JAD-180140
60. Lee D-H, Lee J-Y, Hong D-Y, Lee E-C, Park S-W, Lee Y-K, et al. Pharmacological treatment for neuroinflammation in stress-related disorder. Biomedicines. (2022) 10:2518. doi: 10.3390/biomedicines10102518
61. Sic A, Bogicevic M, Brezic N, Nemr C, Knezevic NN. Chronic stress and headaches: the role of the HPA axis and autonomic nervous system. Biomedicines. (2025) 13:463. doi: 10.3390/biomedicines13020463
62. Daneshpour A, Nasiri H, Motamed AK, Heidarzadeh N, Fard AM, Koleini S, et al. Uncovering cerebral blood flow patterns corresponding to amyloid-beta accumulations in patients across the Alzheimer's disease continuum using the arterial spin labeling. Neurol Sci. (2025) 46:2081–90. doi: 10.1007/s10072-025-07992-4
63. Pavlov VA, Tracey KJ. The vagus nerve and the inflammatory reflex—linking immunity and metabolism. Nat Rev Endocrinol. (2012) 8:743–54. doi: 10.1038/nrendo.2012.189
64. Khalil M, Teunissen CE, Otto M, Piehl F, Sormani MP, Gattringer T, et al. Neurofilaments as biomarkers in neurological disorders. Nat Rev Neurol. (2018) 14:577–89. doi: 10.1038/s41582-018-0058-z
Keywords: NODDI, cognition, plasma biomarker, MRI, HRV
Citation: Jiang S, Cheng Y, Luo L, Jiang R, Pan X and Xue Y (2025) Cognitive implications of white matter microstructural changes in individuals with low heart rate variability: a NODDI study. Front. Neurol. 16:1503599. doi: 10.3389/fneur.2025.1503599
Received: 29 September 2024; Accepted: 14 April 2025;
Published: 09 May 2025.
Edited by:
Laura Lorenzo-López, University of A Coruña, SpainReviewed by:
David Cash, University College London, United KingdomFlavio DellAcqua, King's College London, United Kingdom
Copyright © 2025 Jiang, Cheng, Luo, Jiang, Pan and Xue. This is an open-access article distributed under the terms of the Creative Commons Attribution License (CC BY). The use, distribution or reproduction in other forums is permitted, provided the original author(s) and the copyright owner(s) are credited and that the original publication in this journal is cited, in accordance with accepted academic practice. No use, distribution or reproduction is permitted which does not comply with these terms.
*Correspondence: Yunjing Xue, eHVleXVuamluZ0AxMjYuY29t
†These authors have contributed equally to this work
‡ORCID: Shaofan Jiang orcid.org/0000-0001-8480-7343
Yingzhe Cheng orcid.org/0000-0003-1572-8689
Rifeng Jiang orcid.org/0000-0001-6959-0027
Xiaodong Pan orcid.org/0000-0001-9511-6986
Yunjing Xue orcid.org/0000-0003-4563-4763