- 1Department of Neurology, Minhang Hospital, Fudan University, Shanghai, China
- 2Institute of Science and Technology for Brain-Inspired Intelligence (ISTBI), Fudan University, Shanghai, China
- 3Department of Geriatrics, Shanghai Geriatric Medical Center, Shanghai, China
Importance: phenylacetylglutamine (PAGln), a gut microbiota-derived metabolite, is linked to increased platelet reactivity and thrombosis risk. However, its relationship with age, particularly the non-linear patterns in ischemic stroke patients, remains unclear.
Objectives: To explore the non-linear relationship between age and plasma PAGln levels in ischemic stroke patients, focusing on identifying exponential growth trends and critical age thresholds.
Design, setting, and participants: This single-center, prospective cohort study was conducted at the Department of Neurology, Minhang Hospital, Fudan University, from January 2022 to December 2023. A total of 121 patients with ischemic stroke were consecutively enrolled. Demographic information, lifestyle factors, stroke characteristics, and comorbidities were systematically collected. Plasma PAGln levels were measured using rapid resolution liquid chromatography–quadrupole time-of-flight mass spectrometry. Generalized additive models and smoothing curve fitting were applied to assess non-linear relationships between age and PAGln levels, with threshold effect analysis used to identify age breakpoints. Multivariable regression models were applied to adjust for confounders, and subgroup analyses tested the robustness of findings.
Main outcomes and measures: Plasma PAGln levels and their association with age in ischemic stroke patients, evaluated through non-linear models and regression analysis.
Results: Significant differences in PAGln levels were found across age quartiles (P = 0.004), rising from 186.87 ± 95.49 μmol/L in the youngest quartile (35–54 years) to 433.11 ± 474.03 μmol/L in the oldest quartile (69–87 years). A non-linear association between age and PAGln levels was identified (P = 0.0006). Smoothing curve fitting revealed an exponential increase in PAGln levels with age. A threshold effect analysis pinpointed a breakpoint at 71 years. Below this age, no significant association between age and PAGln was observed (P = 0.5394), while above 71, a significant exponential increase in PAGln levels was detected (P < 0.0001). Subgroup analyses confirmed consistent results across various patient characteristics, with no significant interactions.
Conclusions and relevance: A non-linear exponential relationship exists between age and plasma PAGln levels in ischemic stroke patients, with a marked increase after 71 years. Elevated PAGln levels in elderly patients suggest significant metabolic dysregulation, potentially raising thrombosis risk. Monitoring PAGln levels in stroke patients over 71 years could provide valuable insights for personalized interventions to reduce thrombotic complications.
Introduction
Stroke remains a major global cause of death and disability, presenting an ongoing challenge for public health (1, 2). As the population ages, the incidence of stroke is rising, especially among older adults, who show significantly increased rates of both first-time and recurrent strokes (3). Recently, growing attention has been paid to the role of gut microbiota in neurological diseases, primarily through the “gut-brain axis,” which affects nervous system health and development (4, 5).
Phenylacetylglutamine (PAGln) is a key metabolite produced by the gut microbiota, recently linked to increased thrombosis risk (6). In 2020, Nemet et al. demonstrated that PAGln enhances platelet reactivity by modulating adrenergic receptors, thereby increasing thrombosis risk (7). This finding suggests that PAGln plays a key role in regulating the circulatory system. Additionally, the production of PAGln depends on the metabolic activity of the gut microbiota, and it is also considered a crucial marker reflecting the health of the gut and the metabolic burden of the host (8, 9).
Age is an inevitable factor that significantly influences both stroke onset and prognosis (10). With increasing age, the body's metabolism slows, immune regulation becomes less efficient, and vascular elasticity decreases. Furthermore, the composition of the gut microbiota in elderly populations is altered, characterized by reduced diversity and an increased proportion of pathogenic bacteria (11, 12). As a gut-derived metabolite, PAGln levels may exhibit a complex pattern of changes influenced by age and the composition of the microbiota. However, studies investigating the levels of PAGln in stroke patients and its relationship with age remain relatively scarce, particularly regarding whether this relationship exhibits a specific non-linear pattern.
This study aims to explore the non-linear relationship between age and PAGln levels in stroke patients, with a specific focus on whether an exponential growth trend exists. We hypothesize that PAGln levels may not simply accumulate linearly with age but instead undergo significant changes at certain age thresholds, exhibiting a non-linear exponential growth pattern. Based on this hypothesis, we conducted an in-depth analysis of clinical data from stroke patients and applied smoothing curve fitting and threshold effect analysis to uncover the potential complex associations between age and PAGln.
Methods
Study design and population
This single-center, prospective cohort study investigates the association between gut microbiota-derived metabolites and age in ischemic stroke patients. From January 2022 to December 2023, we consecutively enrolled 121 inpatients diagnosed with ischemic stroke from the Department of Neurology at Minhang Hospital, affiliated with Fudan University. The inclusion criteria were: age ≥ 18 years, National Institutes of Health Stroke Scale (NIHSS) score ≤ 5 upon admission, symptom onset within 7 days, and ischemic stroke diagnosis confirmed by magnetic resonance imaging (MRI). Exclusion criteria were: severe infections, autoimmune diseases, malignancies, recent use of antibiotics or probiotics within the past 3 months, and severe cardiac, hepatic, or renal insufficiency, and known digestive system diseases (e.g., inflammatory bowel disease, chronic diarrhea, gastrointestinal tumors, or history of major gastrointestinal surgery).
This study was conducted in strict accordance with the principles of the Declaration of Helsinki and was approved by the Ethics Committee of Minhang Hospital, Fudan University. All participants provided written informed consent.
Baseline data collection
Baseline data were systematically collected through standardized questionnaires and medical records, covering: Demographic information (age, sex, weight, height, and BMI); Lifestyle and behavioral characteristics (smoking history, alcohol consumption); Stroke characteristics (ischemic cerebral infarction history, subtype classified according to the TOAST criteria); Comorbidities (hypertension, diabetes, atrial fibrillation, lipid metabolism disorders, and other relevant conditions); and Stroke severity (assessed upon admission using the NIHSS score). To facilitate stratified baseline comparisons, age was divided into quartiles based on ascending order.
Measurement of gut microbiota-derived metabolites
Fasting venous blood samples were collected from patients within 24 h of admission using EDTA anticoagulant tubes. Samples were centrifuged at 1,000 × g for 10 min to separate plasma, which was aliquoted and stored at −80°C until analysis.
Metabolite measurement method
Rapid resolution liquid chromatography-quadrupole time-of-flight mass spectrometry (RRLC-QTOF/MS) was employed to quantify plasma levels of gut microbiota-derived metabolites, including phenylacetylglutamine (PAGln), trimethyllysine (TML), and trimethylamine N-oxide (TMAO). Isotopically labeled internal standards (d5-PAGln, d9-TML, and d9-TMAO) were utilized to enhance quantification accuracy and reliability.
Instrumentation and conditions
Liquid chromatography was performed using an Agilent 1,260 series rapid resolution system. Mass spectrometry detection was carried out using an Agilent 6,530 series quadrupole time-of-flight mass spectrometer equipped with a dual electrospray ionization source. Plasma and quality control samples were prepared according to standard operating procedures, which included protein precipitation, centrifugation, and filtration. Metabolite concentrations were determined by comparing peak area ratios of target metabolites to internal standards.
Statistical analysis
All statistical analyses were performed using R software (version 4.0.2). Statistical tests were two-sided, with P < 0.05 considered statistically significant. Continuous variables were summarized as mean ± standard deviation (Mean ± SD) for normally distributed data or as median and interquartile range (IQR) for non-normally distributed data. Categorical variables were expressed as frequencies and percentages. Generalized additive models (GAMs) were used to assess the non-linear relationship between age and PAGln levels, and smoothing curves were plotted to visualize this trend. A segmented regression model was employed to identify any potential threshold point between age and PAGln levels, using the least squares method to estimate the threshold and compare the rate of change in PAGln levels before and after this point. Multivariable regression models were used to adjust for potential confounders, including gender, BMI, smoking history, alcohol consumption, hypertension, and diabetes. Sensitivity analyses and interaction tests were performed to verify the robustness of the findings.
Results
Baseline characteristics and metabolite levels
Among the 121 stroke patients, a significant difference in gender distribution was observed across the age quartiles (P = 0.031). As shown in Table 1, in the first quartile (35–54 years), 88.0% were male, whereas in the fourth quartile (69–87 years), 52.8% were male. There were no statistically significant differences in smoking history (P = 0.098), alcohol consumption (P = 0.518), diabetes (P = 0.082), hypertension (P = 0.287), ischemic cerebral infarction history (P = 0.376), atrial fibrillation (P = 0.171), or lipid metabolism disorders (P = 0.669) across age groups. Similarly, no significant differences were found in BMI, NIHSS score at admission, systolic blood pressure (SBP), or diastolic blood pressure (DBP) among the age quartiles. Additionally, no statistically significant differences in TOAST classification were observed across the age quartiles (P = 0.055).
Significant differences in metabolite levels were observed across age groups. Table 1 shows that TMAO levels differed significantly (P = 0.036), with the first quartile having a mean level of 92.05 ± 42.26 μmol/L, which increased to 219.08 ± 232.91 μmol/L in the second quartile. PAGln levels also varied significantly among age groups (P = 0.004), increasing from 186.87 ± 95.49 μmol/L in the first quartile to 433.11 ± 474.03 μmol/L in the fourth quartile.
Non-linear relationship between age and PAGln levels
The non-linear relationship between age and PAGln levels was assessed using GAMs. After adjusting for multiple confounders, including gender, smoking, alcohol consumption, BMI, ischemic cerebral infarction history, atrial fibrillation, hypertension, diabetes, lipid metabolism disorders, TOAST classification, and NIHSS score, a significant non-linear association between age and PAGln levels was observed (P = 0.0006) (Figure 1). The fitted smooth curve indicated that PAGln levels followed a significant non-linear “exponential” growth pattern with increasing age.
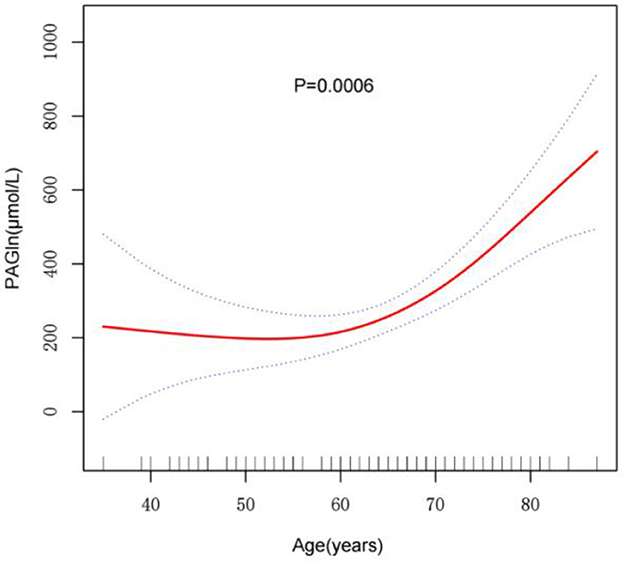
Figure 1. Smooth curve fitting of the relationship between age and PAGln levels. The relationship was modeled using a GAM adjusted for sGender, Alcohol, Smoking, BMI, Ischemic Cerebral Infarction History, Atrial Fibrillation, Hypertension, Diabetes, Lipid Metabolism Disorder, TOAST Classification, and NIHSS Score (Day 1). The red line indicates the smoothed trend of PAGln levels with age, and the blue dashed lines represent 95% confidence intervals. A significant non-linear association was observed (P = 0.0006***). ***p < 0.001.
A segmented regression model identified a threshold point at 71 years for the relationship between age and PAGln levels (Table 2). Below 71 years, the effect of age on PAGln levels was not statistically significant, with an effect size of 2.135 (95% CI: −4.659, 8.929, P = 0.5394). In contrast, above 71 years, the effect size significantly increased to 35.520 (95% CI: 19.042, 51.998, P < 0.0001), indicating a marked exponential rise in PAGln levels. The significance of this threshold effect was further validated by a likelihood ratio test (P < 0.001), confirming a highly significant non-linear association between age and PAGln levels after 71 years, with a rapid increase in this age range.
Subgroup analysis
A subgroup analysis was performed to assess the stability of the association between age and PAGln levels across different population characteristics. The results showed that this association remained consistent regardless of population characteristics. As presented in Table 3, none of the stratification variables—including NIHSS score, gender, TOAST classification, smoking, alcohol consumption, diabetes, hypertension, ischemic cerebral infarction history, atrial fibrillation, or lipid metabolism disorders—significantly affected the relationship between age and PAGln levels. All interaction P-values were >0.05, indicating that none of the covariates significantly modified the effect of age on PAGln levels.
Discussion
This study represents a significant advancement by revealing a non-linear exponential relationship between age and the gut microbiota-derived metabolite PAGln in stroke patients, especially noting a marked increase after the age of 71. These findings offer new insights into metabolic changes in stroke patients, with important implications for future clinical management, particularly for elderly populations.
Our results indicate a notable increase in PAGln levels in patients aged 71 and above, suggesting significant changes in gut microbiota function and host physiology. Previous research has shown that aging is associated with reduced gut microbiota diversity and an increase in pathogenic bacteria, which may contribute to elevated PAGln production (13, 14). Yu et al. also reported a significant association between higher PAGln levels and increased white matter hyperintensities (WMH) burden, particularly in patients with moderate-severe WMH (15). This finding supports the potential role of PAGln as a biomarker for assessing metabolic dysfunction in stroke patients. GAMs analysis identified 71 years as a crucial threshold for significant changes in PAGln levels, indicating a point of potential metabolic dysregulation. Clinically, this threshold reflects an increased metabolic burden in older adults, which could elevate the risk of thrombosis and stroke-related complications.
This study emphasizes the effects of age on PAGln metabolism. Previous research has associated elevated PAGln levels with an increased risk of thrombosis, particularly in cardiovascular diseases (16, 17). Our study specifically examined age-related differences in PAGln levels among stroke patients. PAGln is known to enhance platelet reactivity, thereby increasing the risk of thrombosis, which is particularly concerning for elderly stroke survivors (18, 19). The marked rise in PAGln levels in patients over 71 suggests its potential use as a biomarker for assessing metabolic burden and thrombosis risk, providing valuable insights for individualized risk assessment. These findings point to clinical applications, such as monitoring and regulating PAGln levels in elderly patients to reduce thrombosis risk and improve stroke recovery.
PAGln levels display a non-linear growth pattern, reflecting the complexity of its age-dependent metabolic regulation. Before the age of 71, PAGln levels remain relatively stable, possibly due to effective metabolic stress regulation in younger and middle-aged individuals. With aging, reduced gut barrier function and increased intestinal permeability may lead to elevated PAGln production (20–22), which could explain the sharp rise observed after age 71. Our threshold effect analysis confirms this point as a critical turning point, beyond which PAGln levels rise significantly, indicating metabolic dysregulation and heightened thrombosis risk.
The subgroup analysis showed that PAGln levels increased exponentially in individuals aged over 71, irrespective of gender, smoking history, or alcohol consumption. These findings emphasize that age is the primary factor influencing PAGln metabolism, while other individual characteristics contribute minimally. Even after adjusting for multiple confounding factors, the rising trend in PAGln levels remained significant, indicating an independent association between age and PAGln levels. This highlights the potential of PAGln as a biomarker for assessing metabolic burden in elderly stroke patients, particularly in the absence of other notable risk factors, thereby enabling more targeted and individualized patient management. PAGln levels could thus serve as a key biomarker for predicting stroke recurrence, allowing for more personalized management approaches.
This study provides significant insights by revealing a non-linear exponential relationship between PAGln levels and age, particularly with a marked increase after age 71. These findings have important implications for clinical practice. PAGln could serve as a valuable biomarker for metabolic monitoring in stroke patients, especially among the elderly, providing early indications of metabolic dysfunction and thrombosis risk. Furthermore, patients with significantly elevated PAGln levels—particularly those over the age of 71-may benefit from personalized interventions, including dietary changes, modulation of gut microbiota, or pharmaceutical therapies to reduce metabolic burden and thrombosis risk.
Limitations
This study has several limitations. First, the sample size of 121 ischemic stroke patients is relatively small, which may affect the generalizability of the findings. Second, it is a single-center study, which may introduce selection bias. Third, although we adjusted for several confounders, factors such as dietary habits and gut microbiota composition were not considered, which could influence PAGln levels. Furthermore, the cross-sectional design of this study limits the ability to infer causality between age, PAGln levels, and thrombosis risk. Finally, the lack of long-term follow-up data means that we cannot determine whether elevated PAGln levels persist over time or whether they contribute to long-term stroke outcomes. These limitations highlight the need for future studies with larger sample sizes, multi-center data, and longitudinal follow-up to confirm our findings and explore the potential causal relationships between PAGln levels, stroke prognosis, and metabolic dysfunction.
Conclusion
This research offers new insights into the metabolic characteristics of elderly stroke patients by revealing a non-linear exponential relationship between PAGln levels and age. Specifically, the sharp increase in PAGln levels observed after the age of 71 suggests a heightened risk of thrombosis and stroke in this population. Thus, monitoring PAGln levels could provide valuable evidence for personalized management and early intervention strategies in elderly patients.
Data availability statement
The original contributions presented in the study are included in the article/Supplementary material, further inquiries can be directed to the corresponding authors.
Ethics statement
The studies involving humans were approved by the Ethics Committee of Minhang Hospital, Fudan University. The studies were conducted in accordance with the local legislation and institutional requirements. The participants provided their written informed consent to participate in this study.
Author contributions
YL: Conceptualization, Writing – original draft. MC: Formal analysis, Writing – review & editing. DW: Formal analysis, Writing – review & editing. QL: Data curation, Writing – review & editing. JL: Supervision, Visualization, Writing – review & editing. JZ: Project administration, Supervision, Writing – review & editing.
Funding
The author(s) declare that financial support was received for the research and/or publication of this article. This work was supported by the National Natural Science Foundation of China (Grant Nos. 81973157 and 82173646 [to JZ]); Shanghai Municipal Health Commission Medical New Technology Research and Transformation Seed Project (Grant No. 2024ZZ2072 [to YL]); Natural Science Research Project in Minhang District, Shanghai City (Grant No. 2023MHZ046 [to YL]); and Training Program for High-Level Specialist Physicians under the Collaborative Health Service System of Education, Research, and Clinical Practice in Minhang District, Shanghai City (2024-2027) (Grant No. 2024MZYS09 [to YL]).
Conflict of interest
The authors declare that the research was conducted in the absence of any commercial or financial relationships that could be construed as a potential conflict of interest.
Generative AI statement
The author(s) declare that no Gen AI was used in the creation of this manuscript.
Publisher's note
All claims expressed in this article are solely those of the authors and do not necessarily represent those of their affiliated organizations, or those of the publisher, the editors and the reviewers. Any product that may be evaluated in this article, or claim that may be made by its manufacturer, is not guaranteed or endorsed by the publisher.
Supplementary material
The Supplementary Material for this article can be found online at: https://www.frontiersin.org/articles/10.3389/fneur.2025.1576777/full#supplementary-material
References
1. Fan J, Li X, Yu X, Liu Z, Jiang Y, Fang Y, et al. Global Burden, Risk Factor Analysis, and Prediction Study of Ischemic Stroke, 1990-2030. Neurology. (2023) 101:e137–50. doi: 10.1212/WNL.0000000000207387
2. Prust ML, Forman R, Ovbiagele B. Addressing disparities in the global epidemiology of stroke. Nat Rev Neurol. (2024) 20:207–21. doi: 10.1038/s41582-023-00921-z
3. GBD 2021 Diseases and Injuries Collaborators. Global incidence, prevalence, years lived with disability (YLDs), disability-adjusted life-years (DALYs), and healthy life expectancy (HALE) for 371 diseases and injuries in 204 countries and territories and 811 subnational locations, 1990-2021: a systematic analysis for the Global Burden of Disease Study 2021. Lancet. (2024) 403:2133–2161. doi: 10.1016/S0140-6736(24)00757-8
4. Park J, Kim CH. Regulation of common neurological disorders by gut microbial metabolites. Exp Mol Med. (2021) 53:1821–33. doi: 10.1038/s12276-021-00703-x
5. C. Willyard. How gut microbes could drive brain disorders. Nature. (2021) 590:22–5. doi: 10.1038/d41586-021-00260-3
6. Thierfelder H, Sherwin CP. Phenylacetyl-glutamin, ein Stoffwechsel-Produkt des menschlichen Körpers nach Eingabe von Phenyl-essigsäure. Berichte der deutschen chemischen Gesellschaft. (1914) 47:2630–4. doi: 10.1002/cber.19140470341
7. Nemet I, Saha PP, Gupta N, Zhu W, Romano KA, Skye SM, et al. A cardiovascular disease-linked gut microbial metabolite acts via adrenergic receptors. Cell. (2020) 180:862–877. doi: 10.1016/j.cell.2020.02.016
8. Song Y, Wei H, Zhou Z, Wang H, Hang W, Wu J, et al. Gut microbiota-dependent phenylacetylglutamine in cardiovascular disease: current knowledge and new insights. Front Med. (2024) 18:31–45. doi: 10.1007/s11684-024-1055-9
9. Krishnamoorthy NK, Kalyan M, Hediyal TA, Anand N, Kendaganna PH, Pendyala G, et al. Role of the gut bacteria-derived metabolite phenylacetylglutamine in health and diseases. ACS Omega. (2024) 9:3164–72. doi: 10.1021/acsomega.3c08184
10. Madsen TE, Khoury JC, Leppert M, Alwell K, Moomaw CJ, Sucharew H, et al. Temporal trends in stroke incidence over time by sex and age in the GCNKSS. Stroke. (2020) 51:1070–6. doi: 10.1161/STROKEAHA.120.028910
11. Nuszkiewicz J, Kukulska-Pawluczuk B, Piec K, Jarek DJ, Motolko K, Szewczyk-Golec K, et al. Intersecting pathways: the role of metabolic dysregulation, gastrointestinal microbiome, and inflammation in acute ischemic stroke pathogenesis and outcomes. J Clin Med. (2024) 13:4258. doi: 10.3390/jcm13144258
12. Honarpisheh P, Bryan RM, McCullough LD. Aging microbiota-gut-brain axis in stroke risk and outcome. Circ Res. (2022) 130:1112–44. doi: 10.1161/CIRCRESAHA.122.319983
13. de la Cuesta-Zuluaga J, Kelley ST, Chen Y, Escobar JS, Mueller NT, Ley RE, et al. Age- and sex-dependent patterns of gut microbial diversity in human adults. mSystems. (2019) 4:e00261–19. doi: 10.1128/mSystems.00261-19
14. Mossad O, Batut B, Yilmaz B, Dokalis N, Mezo C, Nent E, et al. Gut microbiota drives age-related oxidative stress and mitochondrial damage in microglia via the metabolite N(6)-carboxymethyllysine. Nat Neurosci. (2022) 25:295–305. doi: 10.1038/s41593-022-01027-3
15. Yu F, Feng X, Li X, Luo Y, Wei M, Zhao T, et al. Gut-derived metabolite phenylacetylglutamine and white matter hyperintensities in patients with acute ischemic stroke. Front Aging Neurosci. (2021) 13:675158. doi: 10.3389/fnagi.2021.675158
16. Fu Y, Yang Y, Fang C, Liu X, Dong Y, Xu L, et al. Prognostic value of plasma phenylalanine and gut microbiota-derived metabolite phenylacetylglutamine in coronary in-stent restenosis. Front Cardiovasc Med. (2022) 9:944155. doi: 10.3389/fcvm.2022.944155
17. Tang W, Nemet I, Li XS, Wu Y, Haghikia A, Witkowski M, et al. Prognostic value of gut microbe-generated metabolite phenylacetylglutamine in patients with heart failure. Eur J Heart Fail. (2024) 26:233–41. doi: 10.1002/ejhf.3111
18. Parra-Izquierdo I, Bradley R, Aslan JE. Platelets get gutted by PAG. Platelets. (2020) 31:618–20. doi: 10.1080/09537104.2020.1759793
19. Fang C, Zuo K, Fu Y, Li J, Wang H, Xu L, et al. Dysbiosis of gut microbiota and metabolite phenylacetylglutamine in coronary artery disease patients with stent stenosis. Front Cardiovasc Med. (2022) 9:832092. doi: 10.3389/fcvm.2022.832092
20. Ling Z, Liu X, Cheng Y, Yan X, Wu S. Gut microbiota and aging. Crit Rev Food Sci Nutr. (2022) 62:3509–34. doi: 10.1080/10408398.2020.1867054
21. Zou Y, Song X, Liu N, Sun W, Liu B. Intestinal flora: a potential new regulator of cardiovascular disease. Aging Dis. (2022) 13:753–72. doi: 10.14336/AD.2021.1022
Keywords: phenylacetylglutamine (PAGln), ischemic stroke, age, gut microbiota, thrombosis risk
Citation: Liu Y, Chu M, Wang D, Li Q, Lin J and Zhao J (2025) Threshold of phenylacetylglutamine changes: exponential growth between age and gut microbiota in stroke patients. Front. Neurol. 16:1576777. doi: 10.3389/fneur.2025.1576777
Received: 14 February 2025; Accepted: 21 April 2025;
Published: 21 May 2025.
Edited by:
Jean-Claude Baron, University of Cambridge, United KingdomReviewed by:
Ozge Altintas Kadirhan, Kırklareli University, TürkiyeZeli Zhang, Shandong University, China
Copyright © 2025 Liu, Chu, Wang, Li, Lin and Zhao. This is an open-access article distributed under the terms of the Creative Commons Attribution License (CC BY). The use, distribution or reproduction in other forums is permitted, provided the original author(s) and the copyright owner(s) are credited and that the original publication in this journal is cited, in accordance with accepted academic practice. No use, distribution or reproduction is permitted which does not comply with these terms.
*Correspondence: Jing Zhao, emhhb19qaW5nQGZ1ZGFuLmVkdS5jbg==; Jixian Lin, bGluaml4aWFuQGZ1ZGFuLmVkdS5jbg==
†These authors share first authorship