- Department of Cognitive Science and Artificial Intelligence, Tilburg University, Tilburg, Netherlands
Introduction: There is a rising interest in using virtual reality (VR) applications in learning, yet different studies have reported different findings for their impact and effectiveness. The current paper addresses this heterogeneity in the results. Moreover, contrary to most studies, we use a VR application actually used in industry thereby addressing ecological validity of the findings.
Methods and Results of Study1: In two studies, we explored the effects of an industrial VR safety training application on learning. In our first study, we examined both interactive VR and passive monitor viewing. Using univariate, comparative, and correlational analytical approaches, the study demonstrated a significant increase in self-efficacy and knowledge scores in interactive VR but showed no significant differences when compared to passive monitor viewing. Unlike passive monitor viewing, however, the VR condition showed a positive relation between learning gains and self-efficacy.
Methods and Results of Study2: In our subsequent study, a Structural Equation Model (SEM) demonstrated that self-efficacy and users’ simulation performance predicted the learning gains in VR. We furthermore found that the VR hardware experience indirectly predicted learning gains through self-efficacy and user simulation performance factors.
Conclusion/Discussion of both studies: Conclusively, the findings of these studies suggest the central role of self-efficacy to explain learning gains generalizes from academic VR tasks to those in use in industry training. In addition, these results point to VR behavioral markers that are indicative of learning.
1 Introduction
Virtual reality (VR) has increasingly been used as a tool for training in a variety of domains, including education (De Back et al., 2020; van Limpt-Broers et al., 2020; Schloss et al., 2021), medicine (Yang et al., 2018; Behmadi et al., 2022), and industrial maintenance (Pedram et al., 2020; Makransky and Klingenberg, 2022). In addition to an effort to understand in what contexts and what aspects of VR training are more beneficial than other training methods (Buttussi and Chittaro, 2017; Makransky, Borre-Gude, et al., 2019; Radianti et al., 2020), there is an increasing focus on understanding the cognitive and affective factors that explain the variability of learning in VR (Makransky and Petersen, 2019).
Both immersive VR and 2D screen training methods, have the potential to leverage multimedia learning principles to facilitate more effective training by optimizing the integration of various visual and auditory information (Mayer, 2009; Mayer, 2014). While several studies point to immersive VR promoting a higher degree of learning than 2D screen solutions (e.g., Krokos et al., 2019; Johnson-Glenberg et al., 2021), others report no difference in learning effectiveness between VR and non-VR conditions (Greenwald et al., 2018; Madden et al., 2020; Souchet et al., 2022). Some studies have even reported a lower degree of learning in VR conditions compared to a 2D screen solution (Molina-Carmona et al., 2018; Makransky et al., 2019).
One explanation for these mixed findings might be the complex nature of learning, with a myriad of elements in the learning process needing to be considered together and not in isolation (Salzman et al., 1999). For instance, factors such as self-efficacy or perceived user confidence (Gegenfurtner et al., 2014), the training context (Hamilton et al., 2021), learners’ behavioral traits (Bailenson et al., 2008; Gavish et al., 2015; Pathan et al., 2020), as well as the quality of the interaction experience with the system (Salzman et al., 1999; Wang et al., 2017; Rupp et al., 2019) all play an important role both in the learning process and its outcome. And the aim of the current study is to advance the knowledge in both VR learning outcomes and the process of learning in VR.
Given the primary objective of understanding the complexity in VR learning process and learning outcomes, a foundational aspect to explore is self-efficacy. Self-efficacy, was defined by Bandura (1997) as the perceived confidence in conducting the trained task. Self-efficacy beliefs influence an individual’s level of motivation, their resilience in the face of challenges, and the amount of effort they invest in a task, according to Bandura’s social cognitive theory (Bandura, 1993). Consequently, learners with a higher sense of self-efficacy are more likely to persist in difficult situations, resulting in improved learning outcomes (Pajares, 1996; Zimmerman, 2000). Self-efficacy has a central role in many explanations of learning gains found in VR training tasks (Wang and Wu, 2008; Richardson et al., 2012; Gegenfurtner et al., 2013; Tai et al., 2022). Most notably, Makransky and Lilleholt (2018) and Makransky and Petersen (2019) used a wide array of cognitive and affective measures within structural equation modeling (SEM) frameworks—a statistical technique that combines factor analysis and multiple regression analysis (Kline, 2015)—to explain variability in learning gains. The conclusion in both studies was that most measures indirectly explained a degree of learning, but the strongest direct connection to learning was from self-efficacy measures. In their CAMIL model, Makransky and Petersen (2021) also, reported a positive relationship between self-efficacy and learning outcomes. Finally, Tai et al. (2022) presented a model in which self-efficacy explained learning through a positive relationship with VR-learning-interest and a negative relationship with VR-using-anxiety. In light of these findings, self-efficacy serves as a central factor in our study as well.
In line with our objective to unravel the complexities influencing learning in VR, it is essential to address the behavioral traits of a learner known to be a factor that correlates with learning gains in VR (Cheng et al., 2015). These traits refer to in-game, real-time, objective behavioral measures of the user. Researchers often convert these measures into performance and use them as assessment methods embodied in the VR environment, which is also known as stealth assessment (Shute, 2009; Alcañiz et al., 2018). However, it is important to distinguish between behavioral data collected during training versus testing phases in VR. This can be seen as analogously to student classroom behavior during regular training versus during an exam. Paying attention to a text for an extended time during a training session, is more focused on the learning process and can reveal personal characteristics, intrinsic motivations and interests, which then may result in more effective learning. In contrast, extended attention to a part of text during a test, is directly related to the learning outcomes, which may indicate understanding issues, and propose potentially lower learning outcomes. In our study, we focused on training-phase data to isolate and better understand how inherent behavioral traits influence learning in a VR training procedure, rather than simply measuring the end result of learning outcomes.
Various objective measures have been employed in different studies to assess performance. For instance, Salzman et al. (1999) used administrator observations, time on task, error types, and error rates as indicators of actual performance to characterize their other variables. Gavish et al. (2015) used task time, the number of picture clues required, and the number of unsolved errors to calculate a combined performance score. Similarly, Shi et al. (2020) applied accuracy and operation time as indicators of task performance and used machine learning methods to predict learning outcomes. Inspired by these studies, we introduced the latent variable “user simulation performance,” inferred from four objective measures we selected, including time on task, error count, question count, and fixation on the checklist. We believe that the combined insights from these references suggest a correlation between user simulation performance and learning gains. And a novel aspect of our study is defining this variable and exploring its relationship with learning gains and self-efficacy.
To further strengthen our understanding of the VR learning process, we turn our focus to another known factor to influence learning, the quality of the interaction experience with the VR training environment (Salzman et al., 1999), which we term as “VR hardware experience.” This is a latent variable, inferred from usability and simulator sickness. However, the literature is not clear about how direct is the relationship between this factor and learning gains. Jia et al. (2014) reported that usability has a positive correlation with learning in a memory-test, but Makransky and Peterson (2019), in their SEM model, indicated that usability explained learning indirectly through self-efficacy. This is similar for simulator sickness, with some studies reporting its direct effects on learning (Rupp et al., 2019), but others reporting no effect (Selzer et al., 2019).
There have been few studies to date that have tried to explain learning in VR simulations by measuring self-efficacy, user simulation performance, and hardware experience. Several studies have identified direct or indirect associations between usability and self-efficacy or perceived learning (Makransky and Petersen, 2019; Pedram et al., 2020; Song et al., 2021). Jia et al. (2014) reported a correlation between usability and task performance, and Johnson (2007) reported a correlation between simulator sickness scores and their participants’ statements that “discomfort hampers training.” This study is the first to evaluate all three as potential factors to explain learning gains in VR training environments.
In conclusion, studies that investigate the role of interactive VR in learning often give insufficient consideration to the variety of factors that attribute to the complexity of the learning process as well as the interactions of these factors. This may explain why the literature has yielded mixed findings on the positive, neutral, and negative effects of VR on the outcome of the learning process. Moreover, the influence of training context and application domain on VR effectiveness has been demonstrated in the literature (Madden et al., 2020; Wu et al., 2020; Johnson-Glenberg et al., 2021), but most studies focus on research-designed simulations rather than industry-designed training actually being used, raising concerns about the generalizability and applicability of the findings. The current study addresses these gaps by examining the effectiveness of a pre-existing real-world industrial applications used for maintenance and safety training, and by investigating the interrelation of different factors affecting learning in this solution. The aim of our investigation is twofold: advancing the understanding of VR learning outcomes and exploring the complexities of the process of learning in VR. To meet these objectives we conducted two studies. The first study centers on learning outcomes, asking the question whether current VR training produces any learning gains and self-efficacy in interactive VR and passive monitor viewing? In the second study we map out the factors that may explain learning gains in interactive VR training scenarios by using structural equation modeling to address the question how the interrelation of different factors like self-efficacy, user simulation performance, and VR hardware experience can define the learning gain in VR. Makransky and Petersen (2019) demonstrated that two sets of measures—self-reported affective and self-reported cognitive measures—filtered through the measure of self-efficacy, affected learning gains. We added additional measures to the model to unravel the complexity of the above-mentioned factors that may affect learning in VR simulations.
2 Study 1. Training using monitor versus VR
A two-part study investigated the learning outcomes and self-efficacy of an industrial VR application for training electrical maintenance tasks. The simulation was presented either as an interactive 3D VR simulation or a passive viewing condition on a 2D screen. Learning and self-efficacy gains were evaluated for each condition, then compared across conditions. Finally, the relationship between self-efficacy and learning gains was evaluated in each condition separately.
2.1 Method
2.1.1 Participants
Sixty individuals (39 females, age M = 21.83, SD = 4.20) from Tilburg University participant pool participated in the study for course credits. The study received approval from the ethics committee at the university (REDC # 20201035). While we did not assess specific VR expertise of participants, none of the participants was familiar with the particular industrial VR solution. Inclusion criteria were that participants had to be 16 years of age or older, no uncorrected hearing or visual impairments, and had to have proficiency in English—the standard language of communication for Tilburg University students. The exclusion criterion consisted of the inability to complete the VR training task.
2.1.2 Materials
2.1.2.1 Interactive VR simulation
Before starting the VR simulation, participants completed a 5-min VR experience with a simple task to get familiarized with the VR controllers and head-mounted display. The interactive VR simulation utilized in this study represents a real-world industrial scenario in a factory, that aims to train participants in conducting electrical maintenance—specifically, disconnecting a main feed pump from the cooling tower in the control room and subsequently performing a megger test on the connections—while ensuring safety protocols. This VR solution, provided by an industry partner, was a pre-existing training tool for their field service engineers and maintenance staff and is part of their annual training. In the VR simulation, the task requires that participants progress through three rooms: the introduction room, the equipment room, and finally the maintenance operation room. These rooms must be visited in this order, and specific actions are required in each.
In the introduction room, participants listened to a detailed description of the task presented by an embodied agent (Figure 1A). After this description, participants were provided with a digital “work-permit” consisted of an in-application text panel which could be viewed by the user in VR as needed. This permit outlined all the tasks that were to be conducted, along with some necessary steps required to complete them. In the equipment room, participants collected the personal protective equipment (PPE) (Figure 1B) as outlined in the work-permit. Participants who did not collect all the necessary equipment were not permitted to proceed to the maintenance operation room. The final room is the maintenance operation room, in which all electrical maintenance steps had to be completed by using a set of tools present in the room (Figure 1C).
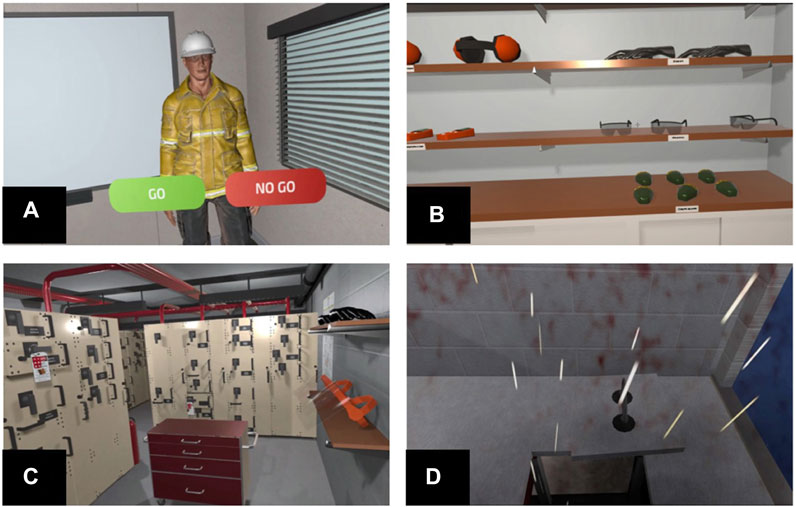
FIGURE 1. (A) Introduction Room; (B). PPE Room; (C). Operation Room; (D). Serious Error Stressor. Reproduced with permission.
The sequence of steps needed to complete the maintenance task could result in nine serious errors that could occur if the participant did not correctly follow the instructions. After each serious error, the participants experienced different stressors, such as an explosion or an evacuation alarm (Figure 1D) and were automatically teleported out of the maintenance operation room back into the equipment room. These stressors were inherent to the original VR solution provided by our industry partner, reflecting real-world industrial training scenarios. For each serious error made, the experimenter, who was the first author of the manuscript, verbally provided the participant with the corresponding guideline associated with the error and how to avoid it. To ensure uniform feedback for all participants, both the experimenter and the verbal feedback remained consistent across all sessions.
Following current training protocols, in which participants could ask questions to the experimenter, a set of 25 pre-written responses to frequently asked questions was created. These were based on queries from earlier trial-run sessions, intended to minimalize confusion across participants while ensuring standardization. Before starting the experiment, participants were informed that whenever they felt stuck, they could ask the experimenter a question. In this case, the question was recorded, and the most appropriate pre-written response was provided verbally by the experimenter. The experiment continued until either a 30-min time limitation was exceeded, or the user had completed the task without producing a serious error.
2.1.2.2 Hardware and technologies
In the VR condition, we used an HTC Vive Pro 2 head mounted display (HMD), up to 100° (horizontal) FOV, and 6-DOF trackers. We used this specific HMD to be consistent with the equipment used by our industry partner, who uses the same hardware and software for their annual training of their employees. Moreover, currently this HMD is one of the most prominent VR HMDs on the market and has been used in a variety of other studies (including Dey et al., 2019; van Limpt-Broers et al., 2020). The application was streamed through SteamVR to the HMD via the Windows 10 operating system.
2.1.2.3 Passive 2D monitor viewing
In the monitor condition, participants viewed a 7-min video of a user performing a walkthrough of the interactive VR simulation environment without committing any serious errors. Participants completed this experiment online at home using their personal setups, due to covid. The video was played in a full-screen mode automatically and all controls otherwise available to the participant (e.g., clicking, fast-forwarding, skipping, etc.) were deactivated by an embedded JavaScript code in the Qualtrics platform.
2.1.2.4 Knowledge questionnaires and learning gains
An interactive design process involving the maintenance training specialists produced 23 intended learning objectives for the VR simulation. Two knowledge questions were created for each intended learning objective and were then randomly assigned to one of two sets. This produced two sets of 23 questions, with each set featuring one question that covered each learning objective. Both before and after training, participants answered a set of written questions using Qualtrics on a desktop computer platform, with the order of question sets being counterbalanced across participants.
Learning gains were computed based on the average-normalized gain between pre- and post-knowledge assessments. Learning gains were calculated as the ratio of the actual average gain (%post—%pre) to the maximum possible average gain (100—%pre) (Hake, 1998).
2.1.2.5 Self-report measures
2.1.2.5.1 Self-efficacy
Self-efficacy is a measure of people’s perceived confidence in their ability to perform a specific task (Gegenfurtner et al., 2014). Following Bandura (2006) and Luszczynska et al. (2005), we adapted six questions from the General Self-Efficacy Scale (Schwarzer and Jerusalem, 1995). This scale has demonstrated good internal consistency, with Cronbach’s alpha values ranging from .76 to .90, the majority of which are in the high .80s (Croasmun et al., 2011). Participants rated statements such as “I can do an electrical maintenance operation” and “I feel confident that I can do an electrical maintenance operation in a limited time” on a 7-point Likert scale from 0 (lowest ability) to 6 (highest ability). These questions measured participants’ confidence in their ability to successfully complete the electrical maintenance task. Aggregate unweighted scores were computed and normalized to 0 to 100.
2.1.2.5.2 System usability
System usability measured how participants perceived the usability of the computer systems they were using (Brooke, 2013). To measure system usability, we used the 10-item System Usability Scale (SUS) (Brooke, 1996), which has a very good reliability with a global Cronbach’s alpha of .91 (Peres et al., 2013). Sample items from the SUS include “I thought the training system was easy to use” and “I felt very confident using the training system.” These questions measured users’ perceptions of the usability of the VR training system, and participants rated each statement on a 5-point Likert scale from 0 (lowest usability) to 4 (highest usability). Aggregate unweighted SUS scores were computed, including reverse-coding, when necessary, with a final range of scores from 0 to 100 (Bangor et al., 2008; Bangor et al., 2009; Sauro, 2011).
2.1.2.5.3 Simulator sickness questionnaire (SSQ)
To measure participants’ discomfort level in VR, we used the original SSQ designed by Kennedy et al. (2003), taking into account the scoring modification suggested by Bimberg et al. (2020). The questionnaire consists of 16 questions and a good reliability based on Cronbach’s alpha of .94 (Sevinc and Berkman, 2020). Participants rated sample items such as ‘Nausea’, ‘Headache’, and ‘Oculomotor discomfort’ on a 4-point Likert scale from “none” to “severe.” Higher scores indicate a higher degree of simulator sickness. We calculated the total SSQ score by aggregating the three unweighted subscales proposed by Kennedy et al. (2003) taking into consideration that five items repeat across the subscales. We multiplied the sum by Kennedy et al.‘s recommended scaling factor of 3.74, which translates to a possible score range from 0 to 235.62.
2.1.3 Design and procedure
After signing the informed consent form, participants in both the interactive VR condition and the passive viewing 2D monitor condition followed the same overall procedure. First, they completed the knowledge test and self-efficacy questionnaire. Participants in the interactive VR group received training in the electrical maintenance task VR simulation, while those in the passive viewing group underwent training by watching a gameplay video of the same simulation, but on a 2D monitor. After the training, participants in both groups completed the simulator sickness questionnaire, followed by the post-training knowledge test, self-efficacy questionnaire, and system usability questionnaire.
2.1.4 Statistical analyses
Several statistical methods were used to evaluate the effectiveness of our training conditions and various related measures. All measures recorded and reported here were analyzed in both studies. We used one-sample t-tests to determine whether the observed changes in learning gains and self-efficacy from pre-to post-test significantly deviated from zero. We utilized independent t-tests to compare the VR and monitor conditions in terms of learning gains, self-efficacy, system usability, and simulator sickness. In addition, Pearson correlation analyses were used to examine the relationships between learning gains and self-efficacy, system usability, and simulator sickness in both conditions.
2.2 Results
2.2.1 Learning and self-efficacy gains
2.2.1.1 VR condition
Twenty-nine out of 30 participants showed an increase in their knowledge scores from pre-to post-test (M = 42.10, SD = 18.9). These learning gains were above zero, according to a one-sample t-test, t(29) = 12.20, p < .001, d = 2.22. Moreover, the self-efficacy of 22 out of 30 participants increased (M = 12.2, SD = 18.7), and the self-efficacy gains were significantly greater than zero t(29) = 3.58, p < .01, d = 0.65. Detailed statistics are provided in Table 1.
2.2.1.2 Monitor condition
Twenty-five out of 30 participants showed an increase in knowledge (M = 32.62, SD = 29.62) and a one-sample t-test showed these knowledge gains were significantly above chance, t (29) = 6.03, p < .001, d = 1.1. Self-efficacy also showed an increase in 17 out of 30 participants (M = 9.63, SD = 15.6). A one-sample t-test showed a significant increase in self-efficacy after monitor training, t(29) = 3.38, p < .01, d = 0.62. For comprehensive statistics, refer to Table 1.
2.2.1.3 Comparison between conditions
Despite larger effect sizes for both learning and self-efficacy gains in the VR condition compared to the monitor condition there was not a significant difference between the VR and monitor conditions in learning gains, t(49.19) = 1.46, p =.14, d = .38 or self-efficacy, t(56.22) = 0.58, p = .56, d = .15. A detailed comparison between VR and monitor conditions, considering all measures, is presented in Table 2.
2.2.2 System usability checks
2.2.2.1 VR condition
System usability scored just below the average satisfaction rate of 68 (Brooke, 2013; Joshi et al., 2021), with the average SUS score being 66.42 (SD = 16.73). Also, simulator sickness was operationalized by SSQ scores (M = 29.67, SD = 26.02) in VR.
2.2.2.2 Monitor condition
For the monitor condition, the SUS scores (M = 56.75, SD = 15.45), were well below the acceptable standard (Brooke, 2013; Joshi et al., 2021), suggesting that the participants did not experience this system as acceptably usable. Additionally, the SSQ scores (M = 40.64, SD = 32.40), were derived from participants from watching the video on the monitor.
2.2.2.3 Comparison between conditions
A significant difference between VR and monitor was found for SUS, t(57.63) = −2.32, p = .02, d = .60. However, there was no significant difference between the VR and monitor conditions in SSQ, t(55.41) = -1.45, p = .15, d = −.37. Although, SSQ in monitor was slightly higher than induced sickness by using VR.
2.2.3 Relationship between learning gains and other measures
2.2.3.1 VR condition
A Pearson correlation test indicated a significant positive correlation between learning gains and self-efficacy, r(28) = .47, p < .01. In contrast, the correlations between learning gains and SUS, r(28) = .29, p = .12, and between learning gains and SSQ, r(28) = −.24, p = .2, were not statistically significant.
2.2.3.2 Monitor condition
Conversely, a correlation test showed no significant relation between self-efficacy and learning gains, r(28) = .23, p = .22. As with the VR condition, there was no significant correlation between learning gains and SUS, r(28) = .23, p = .21, nor between learning gains and SSQ (r(28) = −.05, p = .79) in the monitor condition.
2.3 Discussion
When either actively exploring an immersive VR simulation or passively viewing a simulation on a monitor, the industry-designed VR training resulted in significant improvements in both knowledge gains and self-efficacy. These results are consistent with research-designed 2D-VR training environments (Smith et al., 2018; Madden et al., 2020) and interactive VR training environments, e.g., (Buttussi and Chittaro, 2017; Smith et al., 2018; Rupp et al., 2019). Additionally, this study found no significant difference between the two conditions regarding SSQ, consistent with previous research by Joshi et al. (2021), but a difference between VR and monitor was found for SUS, as also reported by Simões et al. (2020) and Othman et al. (2022).
The high fidelity of VR compared to the monitor condition made it rather surprising that our comparisons did not show a reliable difference between the two conditions, except for system usability. But perhaps this is not unexpected given the complex nature of learning (Salzman et al., 1999), with literature suggesting that several factors can explain this finding, such as differences in participant perceptions of their abilities, behavioral differences, or the quality of experience participants had.
The difference between VR and the monitor condition was much clearer in the relationship between self-efficacy and learning. In the monitor condition, there was no evidence of a significant correlation between learning and other measures, but in the VR condition, there was. The positive correlation in the VR condition echoes the results of structural equation modeling analyses on learning in other VR tasks (Makransky and Petersen, 2021). Makransky and Petersen (2019) found that a large range of cognitive and affective factors that might directly influence learning gains are more appropriate to consider as indirect factors, and the measure with the most direct impact on learning gains is self-efficacy. Expanding on this framework, in Study 2 we recorded behavioral data during the immersive VR task (Salzman et al., 1999; Gavish et al., 2015; Read and Saleem, 2017; Shi et al., 2020) and evaluated the relationship between learning gains, self-efficacy, system usability, simulator sickness, and behavioral measures.
3 Study 2. Training in VR
We followed Makransky and Peterson (2019) and used a structural equation model (SEM) to map out the factors that may influence learning in VR. The theoretical framework for this study is grounded in the existing literature presented in the introduction section, which suggests that self-efficacy, user simulation performance, and VR hardware experience can all influence learning outcomes in a VR training environment either directly or indirectly through self-efficacy. The hypothesized model in Figure 2 encompasses all these factors and the postulated relationships between them, in accordance with the theoretical framework.
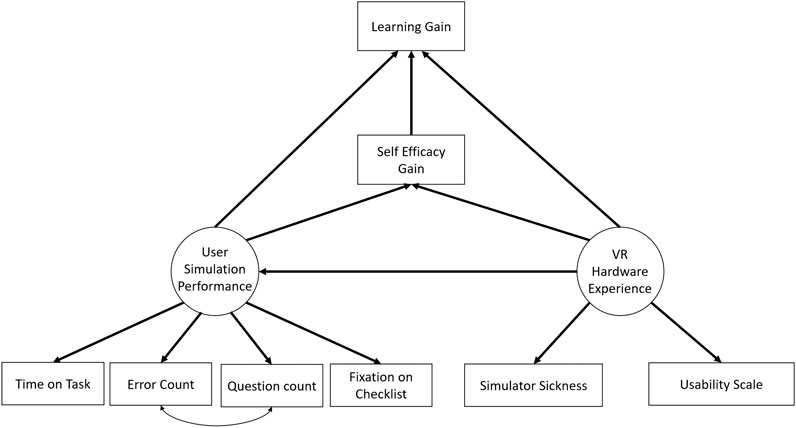
FIGURE 2. Priori SEM model of the learning process in VR. This initial model represents the hypothesized relationships based on theoretical underpinnings and previous research. Each path and node showcases our expected connections before data collection. This model serves as a foundation to compare against the final model (Figure 3) after the iterative fitting procedure.
As in the Makransky and Peterson (2019) analysis, there is hypothesized a connection from Self-Efficacy Gain to Learning Gains. This is in alignment with existing work showing self-efficacy has been found to have a significant influence on learning gains in VR (Wang and Wu, 2008; Richardson et al., 2012; Gegenfurtner et al., 2013; Makransky and Petersen, 2019; Tai et al., 2022). However, Figure 2 indicates the inclusion of connections from the User Simulation Performance and VR Hardware Experience latent factors to both the Self-Efficacy Gain and Learning Gains factors. Previous studies have shown that VR hardware experience, which encompasses the user’s interaction experience with the VR training environment, can explain learning outcomes (Salzman et al., 1999; Makransky and Petersen, 2019; Rupp et al., 2019; Selzer et al., 2019). However, usability and simulator sickness, components of VR hardware experience, have been found to have an ambiguous effect on learning (Jia et al., 2014; Makransky and Petersen, 2019). We expect that VR hardware experience may explain the learning gains and predict self-efficacy gains. Finally, we include a connection from VR Hardware Experience to User Simulation Performance as we expect VR hardware experience can explain user simulation performance (Johnson, 2007; Jia et al., 2014).
3.1 Method
3.1.1 Participants
To ensure sufficient statistical power for the SEM analysis, an additional 27 participants were recruited from the same participant pool. The original goal was to double the number of participants from the VR condition in Study 1 by recruiting 30 more. Three participants however had to be excluded due to their inability to complete the task. As a result, a total of 57 participants (30 from Study 1 and 27 new ones) were included in the SEM analysis (29 females, age M = 21.98, SD = 4.20).
Similar to Study 1, participants had no prior familiarity with our specific VR solution. The inclusion criteria was age 16 or older, no uncorrected hearing or visual impairments, and proficiency in English. The main exclusion criterion was the inability to complete the VR training task.
3.1.2 Materials
In Study 2, we focused exclusively on VR without any comparison to a monitor condition or anything else. This choice was informed by the results of Study 1, where the VR condition demonstrated a significant correlation between learning gains and other measures. Study 2 used the same materials and methods as Study 1, with the addition of the following behavioral measures.
3.1.2.1 Behavioral measures
3.1.2.1.1 Time on task
The duration of the task ranged from 0 to 30 min.
3.1.2.1.2 Question count
The number of questions participants asked the experimenter during the training. As before, all questions were answered with one of the 25 pre-determined FAQ responses.
3.1.2.1.3 Error count
The number of serious errors experienced during the training. This ranged from 0 to 9 errors which can occur if the user has not followed the instructions correctly.
3.1.2.1.4 Fixation on checklist
The percentage of time spent looking at the work-permit relative to the total time the user activated the work-permit (with a button press).
Descriptive statistics for these behavioral measures are provided in Table 3.
3.1.3 SEM statistical analyses
The list of all measures included in the SEM analysis and their correlation with each other is included in Table 4. The items were treated as scalar variables, and the proposed models are verified in terms of the suitability of the models using three indicators: Comparative Fit Index (CFI, Hatcher and O'Rourke, 2013), Discrepancy Divided by Degrees of Freedom (CMIN/DF, Hair et al., 2010), and Root Mean Square Error of Approximation (RMSEA, Hair et al., 2010). In this study, we performed SEM using IBM SPSS Amos version 28.0, and we followed the SEM method from Makransky and Petersen (2019) for pruning the non-significant paths according to the greatest misfit. After fitting, if a non-significant connection was present, the connection with the lowest significance was deleted and the model connections estimated again. This procedure was iteratively followed until all of the remaining paths were significant.
3.2 Results
We conducted a confirmatory factor analysis (CFA) on defined constructs to test the fitness of the hypothesized relationships shown in Figure 2. Our initial hypothesized model almost reached an acceptable fit (RMSEA = .079, CFI = .94, CMIN/DF = 1.35) but the resulting fit indicated two insignificant paths were present. After the iterative procedure removed these two connections, a simplified and more robust model was obtained (Figure 3) with an acceptable fit (RMSEA = .078; CFI = .93; CMIN/DF = 1.34). All standardized path coefficients shown in Figure 3 are significant at an alpha level of .05. Table 4 indicates the descriptive statistics of all factor loadings.
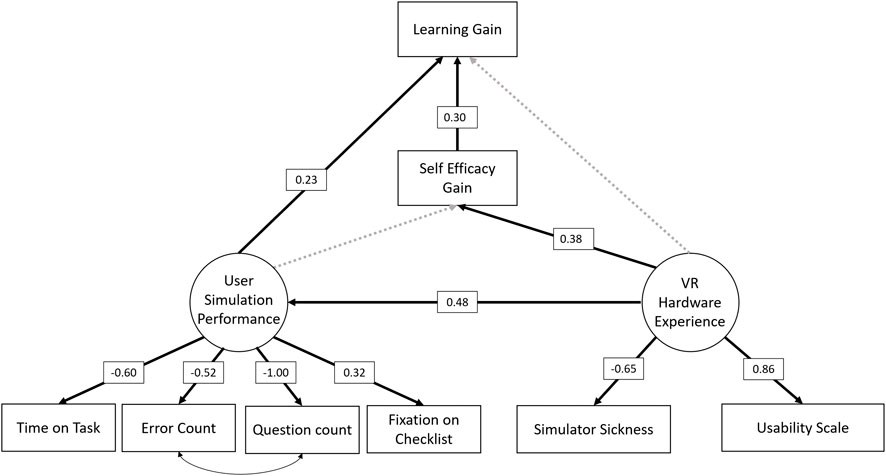
FIGURE 3. Final model. The dashed line represents a connection that was not significant and was eventually pruned in the iterative fitting procedure (Makransky and Petersen, 2019). The continuous line represents significant paths that remain from the initial hypothesized model (Figure 2).
3.3 Discussion
In Study 2, the resulting model exhibited consistent constructs, with “user simulation performance” representing in-game behavioral performance measures and “VR hardware experience” encompassing self-reported usability and sickness. These latent factors had significant loadings on all observed measures hypothesized to be connected to them. A strong, direct connection was found between self-efficacy and learning gains. This is consistent with other studies evaluating VR training in the domains of education, academic assessments, and occupational skill development (Richardson et al., 2012; Makransky and Petersen, 2019; Tai et al., 2022).
The hypothesized direct connection from VR hardware experience to learning gains was not significant in the final SEM (beta = .137, p = .33). This finding is somewhat surprising, considering the results reporting the direct effect of usability or simulator sickness on learning in Jia et al. (2014) and Rupp et al. (2019), however Salzman et al. (1999), noted mixed results in the literature regarding usability and simulator sickness impacts on learning. Instead of a direct connection to the learning gains, our model suggests a positive indirect effect of the VR hardware experience on learning by affecting self-efficacy, in line with the findings in Makransky and Petersen (2019), where usability connects to the learning passing through cognitive variables and self-efficacy, and Pedram et al. (2020), where they showed both usability and self-efficacy can explain learning indirectly through different paths. The direct connection from usability to self-efficacy is consistent with Song et al. (2021).
Finally, our model sheds light on the effect of user simulation performance on learning gains. Unlike Makransky and Petersen (2019), who did not include behavioral measures, we incorporated them and identified a factor beyond self-efficacy that directly impacts learning gains. Behavioral measures recorded during training, including time on task, error count, question count, and fixation duration, constitute a latent variable that directly explains a significant proportion of the variance in learning gains. A portion of the variance in user simulation performance does seem to be directly explained by VR hardware experience, which is in line with Jia et al. (2014), who reported the correlation between usability and task performance, and Johnson (2007), who reported a correlation between SSQ score and agreement with the statement that “discomfort hampers training.”
4 General discussion
Our finding in Study 1 regarding the parity of the effect of a 2D screen and interactive VR on learning outcomes is in line with several studies (Buttussi and Chittaro, 2017; Greenwald et al., 2018; Joshi et al., 2021) but in contrast with others (Krokos et al., 2019; Kyrlitsias et al., 2020). To explain this parity and the existing discrepancy in the literature, factors such as training context and task-technology fit might be helpful. VR has been shown to perform better in assessments involving spatial memorization (Sowndararajan et al., 2008; Ragan et al., 2010), or spatial ability (Yang et al., 2018), as well as in studies focused on skill-based rather than knowledge-based training (Kozhevnikov et al., 2013). Thus, the immersion and interactivity which make VR effective for spatial and skill-based tasks, may make it less efficient for purely knowledge-based learning, where traditional methods can be more direct and less distracting.
The generalization of multimedia learning principles (Mayer, 2009; 2014; Mayer and Fiorella, 2014) to VR may explain cases where extraneous materials and features in VR environments can cause cognitive overload, depleting learners’ limited cognitive capacity (Parong and Mayer, 2018). A goal-oriented design with an appropriate task-technology fit can mitigate these distractions (Zhang et al., 2017). Considering the training context and task-technology fit, in our study we used a safety training application primarily designed for interactive VR. Thus, the observed parity between 2D screens and interactive VR is more likely due to the focus on knowledge-based rather than skill-based training contexts and assessments.
The findings of Study 1 suggest VR should not to be seen as a one-size-fits-all approach. This conclusion is in line with Johnson-Glenberg et al.’s (2021) argument that “platform is not destiny”, which suggests that only using new platforms like VR will not guarantee the effectiveness of the training. Instead, as the current study has shown, research into VR for training purposes should consider a variety of factors working together, such as context, self-efficacy, user simulation performance, and VR hardware experience.
The results of our SEM analysis in Study 2 indicate that self-efficacy is a central predictor of learning gains. The resulting beta value of .3 from the SEM in Study 2, along with the correlation of .47 in Study 1, are consistent with previous findings. For instance, Makransky and Petersen (2019) found a beta value of .579 for the same relationship in their SEM analysis. Likewise, Gegenfurtner et al. (2013) in their meta-analysis reported an uncorrected mean correlation of .34 between self-efficacy and transfer of learning. Richardson et al. (2012) also observed a medium correlation of .31 between GPA and academic self-efficacy, with a 95% confidence interval of [0.28, 0.34].
Though previous SEM analyses of learning gains on VR training found self-efficacy to be the only factor directly predicting learning gains (Makransky and Petersen, 2019), our analysis suggests a direct connection from the latent factor User Simulation Performance that captures the four behavioral markers of performance in the simulation. This suggests that behavior provides information about the degree of learning above and beyond what people are aware that they are capable of (self-efficacy). In addition, it demonstrates that people who display “correct” behavior in the VR experience (completing the task faster, with fewer errors, while asking fewer questions, but looking at the information sheet more) improve more based on the training. This result suggests future avenues of adaptive training that focus more on scaffolding the environment to facilitate correct behavior and thus more learning (Vygotsky, 1978) and less on finding the level of desirable difficulty to promote errors (Bjork, 1994).
The quality of the VR hardware experience, as indicated by factors such as simulator sickness and perceived usability, did now show a direct connection with learning gains. Instead, the VR hardware experience directly impacted both self-efficacy and user simulation performance, and thus indirectly had an impact on learning. Unsurprisingly, people who had a more negative experience had both lower self-efficacy and worse performance, leading to less learning. This highlights the importance of usability when designing virtual training environments (Pedram et al., 2020) or task-technology fit (Zhang et al., 2017).
These results focus on short-term knowledge gains from a VR simulation designed as part of an annual refresher training program for employees. However, insights in the duration of knowledge retention, its generalizability and transfer to the other situations, and the effect of participant expertise (Chi et al., 2014) require further research.
The current study used an industry VR application with university students as participants. One may argue that this is a limitation of the study, as ideally employees involved in the annual training would serve as better participants. There are two reasons for our decision, one theoretical and one practical. In order to compare the findings of our studies with those in other published studies, it would be desirable to not vary both VR application and participant group. With most of the published studies using university participants, we opted to keep this factor constant. These findings would then pave the way for more in-depth studies. This brings us to the practical reason: For obvious reasons it is harder to have employees participate in an experiment, and only ask them to participate once a foundation is put in place of the findings of a prior study.
Our future research will involve actual employees. In fact, currently we are conducting a follow-up study that focuses on employees, allowing us to compare the findings with those from university students. Other lines of further research will focus on gaining more insight in the dependent variables, by including physiological measures such as EEG and eye-tracking in addition to questionnaires, in order to compare the results from offline measures with online measures.
In conclusion, our study shows that an industrial safety training simulation produces significant gains in knowledge and self-efficacy, in both VR and monitor viewing conditions. In addition, further analysis of the VR data replicated the finding that self-efficacy is the best predictor of learning, something that had not yet been shown in a real-world application designed and used by the industry. Apart from generalizing the importance of self-efficacy for learning to industrial applications, our findings provide evidence that people whose behavior in the simulation is congruent with doing the task well (asking fewer questions, making fewer errors, completing faster) learn more, and that this effect is in addition to what can be explained by self-efficacy. This novel result highlights the potential for behavioral markers to indicate learning in VR settings. Our study adds to the growing body of literature on the use of VR for industrial training and has practical implications for the design of VR training programs.
Data availability statement
The data that support the findings of this study are openly available in DataversNL at https://doi.org/10.34894/T1VAKP.
Ethics statement
The studies involving humans were approved by the ethics committee at the Tilburg University (REDC # 20201035). The studies were conducted in accordance with the local legislation and institutional requirements. The participants provided their written informed consent to participate in this study.
Author contributions
SM, conceptualized and designed the experiments, performed the data collection and analysis, and wrote the manuscript. AH and MML contributed to the experimental design, suggested analysis plans, supervised the analysis and writing process. WP, contributed to the experimental design, and supervised the writing process. All authors contributed to the article and approved the submitted version.
Funding
This research is part of the MasterMinds project, funded by the RegionDeal Mid- and West-Brabant, and is co-funded by the Ministry of Economic Affairs and Municipality of Tilburg awarded to MML.
Acknowledgments
We would like to thank Actemium for their help in this research.
Conflict of interest
The authors declare that the research was conducted in the absence of any commercial or financial relationships that could be construed as a potential conflict of interest.
Publisher’s note
All claims expressed in this article are solely those of the authors and do not necessarily represent those of their affiliated organizations, or those of the publisher, the editors and the reviewers. Any product that may be evaluated in this article, or claim that may be made by its manufacturer, is not guaranteed or endorsed by the publisher.
References
Alcañiz, M., Parra, E., and Chicchi Giglioli, I. A. (2018). Virtual reality as an emerging methodology for leadership assessment and training. Front. Psychol. 9, 1658. doi:10.3389/fpsyg.2018.01658
Bailenson, J., Patel, K., Nielsen, A., Bajscy, R., Jung, S.-H., and Kurillo, G. (2008). The effect of interactivity on learning physical actions in virtual reality. Media Psychol. 11 (3), 354–376. doi:10.1080/15213260802285214
Bandura, A. (2006). “Guide for constructing self-efficacy scales,” in Self-efficacy beliefs of adolescents. Editors F. Pajares, and T. C. Urdan (Information Age Publishing), 307–337.
Bandura, A. (1993). Perceived self-efficacy in cognitive development and functioning. Educ. Psychol. 28 (2), 117–148. doi:10.1207/s15326985ep2802_3
Bandura, A. (1997). Self-efficacy: toward a unifying theory of behavioral change. Psychol. Rev. 84, 191–215. doi:10.1037/0033-295x.84.2.191
Bangor, A., Kortum, P., and Miller, J. (2009). Determining what individual SUS scores mean: adding an adjective rating scale. J. Usability Stud. 4 (3), 114–123.
Bangor, A., Kortum, P. T., and Miller, J. T. (2008). An empirical evaluation of the system usability scale. Int. J. Human-Computer Interact. 24 (6), 574–594. doi:10.1080/10447310802205776
Behmadi, S., Asadi, F., Okhovati, M., and Sarabi, R. E. (2022). Virtual reality-based medical education versus lecture-based method in teaching start triage lessons in emergency medical students: virtual reality in medical education. J. Adv. Med. Educ. Prof. 10 (1), 48–53. doi:10.30476/JAMP.2021.89269.1370
Bimberg, P., Weissker, T., and Kulik, A. (2020). “On the usage of the simulator sickness questionnaire for virtual reality research,” in 2020 IEEE Conference on Virtual Reality and 3D User Interfaces (VRW), Atlanta, GA, USA, March 26 2020, 464–467.
Bjork, R. A. (1994). Memory and metamemory considerations in the. Metacognition Knowing about knowing 185 (7). doi:10.7551/mitpress/4561.001.0001
Buttussi, F., and Chittaro, L. (2017). Effects of different types of virtual reality display on presence and learning in a safety training scenario. IEEE Trans. Vis. Comput. Graph. 24 (2), 1063–1076. doi:10.1109/tvcg.2017.2653117
Cheng, M. T., Lin, Y. W., and She, H. C. (2015). Learning through playing Virtual Age: exploring the interactions among student concept learning, gaming performance, in-game behaviors, and the use of in-game characters. Comput. Educ. 86, 18–29. doi:10.1016/j.compedu.2015.03.007
Chi, M. T., Glaser, R., and Farr, M. J. (2014). The nature of expertise. England, United Kingdom: Psychology Press.
Croasmun, J. T., and Ostrom, L. (2011). Using likert-type scales in the social sciences. J. adult Educ. 40 (1), 19–22.
De Back, T. T., Tinga, A. M., Nguyen, P., and Louwerse, M. M. (2020). Benefits of immersive collaborative learning in cave-based virtual reality. Int. J. Educ. Technol. High. Educ. 17 (1), 51–18. doi:10.1186/s41239-020-00228-9
Dey, A., Chatburn, A., and Billinghurst, M. (2019). “Exploration of an EEG-based cognitively adaptive training system in virtual reality,” in 2019 IEEE conference on virtual reality and 3D user interfaces (VR), Atlanta, GA, USA, March 22-26, 2020 (IEEE), 220–226.
Gavish, N., Guti´errez, T., Webel, S., Rodr´ıguez, J., Peveri, M., Bockholt, U., et al. (2015). Evaluating virtual reality and augmented reality training for industrial maintenance and assembly tasks. Interact. Learn. Environ. 23 (6), 778–798. doi:10.1080/10494820.2013.815221
Gegenfurtner, A., Quesada-Pallar`es, C., and Knogler, M. (2014). Digital simulation-based training: A meta-analysis. Br. J. Educ. Technol. 45 (6), 1097–1114. doi:10.1111/bjet.12188
Gegenfurtner, A., Veermans, K., and Vauras, M. (2013). Effects of computer support, collaboration, and time lag on performance self-efficacy and transfer of training: A longitudinal meta-analysis. Educ. Res. Rev. 8, 75–89. doi:10.1016/j.edurev.2012.04.001
Gonzalez-Franco, M., Pizarro, R., Cermeron, J., Li, K., Thorn, J., Hutabarat, W., et al. (2017). Immersive mixed reality for manufacturing training. Front. Robotics AI 4, 3. doi:10.3389/frobt.2017.00003
Greenwald, S. W., Corning, W., Funk, M., and Maes, P. (2018). Comparing learning in virtual reality with learning on a 2d screen using electrostatics activities. J. Universe. Comput. Sci. 24 (2), 220–245.
Hair, J., Black, W., Babin, B., and Anderson, R. (2010). Multivariate data analysis. 7. Upper Saddle River, NJ: Pearson Prentice Hall, Inc.
Hake, R. R. (1998). Interactive-engagement versus traditional methods: A six-thousand-student survey of mechanics test data for introductory physics courses. Am. J. Phys. 66 (1), 64–74. doi:10.1119/1.18809
Hamilton, D., McKechnie, J., Edgerton, E., and Wilson, C. (2021). Immersive virtual reality as a pedagogical tool in education: A systematic literature review of quantitative learning outcomes and experimental design. J. Comput. Educ. 8 (1), 1–32. doi:10.1007/s40692-020-00169-2
Hatcher, L., and O’Rourke, N. (2013). A step-by-step approach to using SAS for factor analysis and structural equation modeling. North Carolina, United States: SAS Institute.
Jia, D., Bhatti, A., and Nahavandi, S. (2014). The impact of self-efficacy and perceived system efficacy on effectiveness of virtual training systems. Behav. Inf. Technol. 33 (1), 16–35. doi:10.1080/0144929x.2012.681067
Johnson, D. M. (2007). Simulator sickness research summary. Alabama: US Army Research Institute for the Behavioral and Social Science Ft Rucker.
Johnson-Glenberg, M. C., Bartolomea, H., and Kalina, E. (2021). Platform is not destiny: embodied learning effects comparing 2d desktop to 3d virtual reality stem experiences. J. Comput. Assisted Learn. 37 (5), 1263–1284. doi:10.1111/jcal.12567
Joshi, S., Hamilton, M., Warren, R., Faucett, D., Tian, W., Wang, Y., et al. (2021). Implementing virtual reality technology for safety training in the precast/prestressed concrete industry. Appl. Ergon. 90, 103286. doi:10.1016/j.apergo.2020.103286
Kennedy, R., Drexler, J., Compton, D., Stanney, K., Lanham, D., and Harm, D. (2003). “Configural scoring of simulator sickness, cybersickness and space adaptation syndrome: similarities and differences,” in Virtual and adaptive environments: Applications, implications, and human performance issues. Editors L. J. Hettinger, and M. W. Haas (Mahwah, NJ: Lawrence Erlbaum Associates), 247–278.
Kline, R. B. (2015). Principles and practice of structural equation modeling. New York City: Guilford publications.
Kozhevnikov, M., Gurlitt, J., and Kozhevnikov, M. (2013). Learning relative motion concepts in immersive and non-immersive virtual environments. J. Sci. Educ. Technol. 22 (6), 952–962. doi:10.1007/s10956-013-9441-0
Krokos, E., Plaisant, C., and Varshney, A. (2019). Virtual memory palaces: immersion aids recall. Virtual Real. 23 (1), 1–15. doi:10.1007/s10055-018-0346-3
Kyrlitsias, C., Christofi, M., Michael-Grigoriou, D., Banakou, D., and Ioannou, A. (2020). A virtual tour of a hardly accessible archaeological site: the effect of immersive virtual reality on user experience, learning and attitude change. Front. Comput. Sci. 2, 23. doi:10.3389/fcomp.2020.00023
Luszczynska, A., Scholz, U., and Schwarzer, R. (2005). The general self-efficacy scale: multicultural validation studies. J. Psychol. 139 (5), 439–457. doi:10.3200/jrlp.139.5.439-457
Madden, J., Pandita, S., Schuldt, J., Kim, B., Won, A., and Holmes, N. (2020). Ready student one: exploring the predictors of student learning in virtual reality. PloS One 15 (3), e0229788. doi:10.1371/journal.pone.0229788
Makransky, G., Borre-Gude, S., and Mayer, R. E. (2019). Motivational and cognitive benefits of training in immersive virtual reality based on multiple assessments. J. Comput. Assisted Learn. 35 (6), 691–707. doi:10.1111/jcal.12375
Makransky, G., and Klingenberg, S. (2022). Virtual reality enhances safety training in the maritime industry: an organizational training experiment with a non-weird sample. J. Comput. Assisted Learn. 38 (4), 1127–1140. doi:10.1111/jcal.12670
Makransky, G., and Lilleholt, L. (2018). A structural equation modeling investigation of the emotional value of immersive virtual reality in education. Educ. Technol. Res. Dev 66 (5), 1141–1164. doi:10.1007/s11423-018-9581-2
Makransky, G., and Petersen, G. B. (2019). Investigating the process of learning with desktop virtual reality: A structural equation modeling approach. Comput. Educ 134, 15–30. doi:10.1016/j.compedu.2019.02.002
Makransky, G., and Petersen, G. B. (2021). The cognitive-affective model of immersive learning (CAMIL): A theoretical research-based model of learning in immersive virtual reality. Educ. Psychol. Rev 33 (3), 937–958. doi:10.1007/s10648-020-09586-2
Mayer, R. E. (2014). “Cognitive theory of multimedia learning,” in The Cambridge handbook of multimedia learning Editor R. E. Mayer 2 (New York, NY: Cambridge University Press) 43–71.
Mayer, R. E., and Fiorella, L. (2014). “Principles for reducing extraneous processing in multimedia learning: coherence, signaling, redundancy, spatial contiguity, and temporal contiguity principles,” in The Cambridge handbook of multimedia learning Editor R. E. Mayer 2 (New York, NY: Cambridge University Press) 279–315.
Molina-Carmona, R., Pertegal-Felices, M. L., Jimeno-Morenilla, A., and MoraMora, H. (2018). Virtual reality learning activities for multimedia students to enhance spatial ability. Sustainability 10 (4), 1074. doi:10.3390/su10041074
Othman, M. K., Nogoibaeva, A., Leong, L. S., and Barawi, M. H. (2022). Usability evaluation of a virtual reality smartphone app for a living museum. Univers. Access Inf. Soc. 21 (4), 995–1012. doi:10.1007/s10209-021-00820-4
Pajares, F. (1996). Self-efficacy beliefs in academic settings. Rev. Educ. Res. 66 (4), 543–578. doi:10.3102/00346543066004543
Parong, J., and Mayer, R. E. (2018). Learning science in immersive virtual reality. J. Educ. Psychol. 110 (6), 785–797. doi:10.1037/edu0000241
Pathan, R., Rajendran, R., and Murthy, S. (2020). Mechanism to capture learner’s interaction in VR-based learning environment: design and application. Smart Learn. Environ. 7 (1), 35–15. doi:10.1186/s40561-020-00143-6
Pedram, S., Palmisano, S., Skarbez, R., Perez, P., and Farrelly, M. (2020). Investigating the process of mine rescuers’ safety training with immersive virtual reality: A structural equation modelling approach. Comput. Educ. 153, 103891. doi:10.1016/j.compedu.2020.103891
Peres, S. C., Pham, T., and Phillips, R. (2013). Validation of the system usability scale (SUS): SUS in the wild. Proc. Hum. factors ergonomics Soc. Annu. Meet. 57 (1), 192–196. SAGE Publications. doi:10.1177/1541931213571043
Radianti, J., Majchrzak, T. A., Fromm, J., and Wohlgenannt, I. (2020). A systematic review of immersive virtual reality applications for higher education: design elements, lessons learned, and research agenda. Comput. Educ. 147, 103778. doi:10.1016/j.compedu.2019.103778
Ragan, E. D., Sowndararajan, A., Kopper, R., and Bowman, D. A. (2010). The effects of higher levels of immersion on procedure memorization performance and implications for educational virtual environments. Presence Teleoperators Virtual Environ. 19 (6), 527–543. doi:10.1162/pres_a_00016
Read, J. M., and Saleem, J. J. (2017). Task performance and situation awareness with a virtual reality head-mounted display. Proc. Hum. Factors Ergonomics Soc. Annu. Meet. 61 (1), 2105–2109. doi:10.1177/1541931213602008
Richardson, M., Abraham, C., and Bond, R. (2012). Psychological correlates of university students’ academic performance: A systematic review and meta-analysis. Psychol. Bull. 138 (2), 353–387. doi:10.1037/a0026838
Rupp, M. A., Odette, K. L., Kozachuk, J., Michaelis, J. R., Smither, J. A., and McConnell, D. S. (2019). Investigating learning outcomes and subjective experiences in 360-degree videos. Comput. Educ. 128, 256–268. doi:10.1016/j.compedu.2018.09.015
Salzman, M. C., Dede, C., Loftin, R. B., and Chen, J. (1999). A model for understanding how virtual reality aids complex conceptual learning. Presence Teleoperators Virtual Environ. 8 (3), 293–316. doi:10.1162/105474699566242
Sauro, J. (2011). A practical guide to the system usability scale: background, benchmarks & best practices. Denver, Colorado: Measuring Usability LLC.
Schloss, K. B., Schoenlein, M. A., Tredinnick, R., Smith, S., Miller, N., Racey, C., et al. (2021). The UW virtual brain project: an immersive approach to teaching functional neuroanatomy. Transl. Issues Psychol. Sci. 7 (3), 297–314. doi:10.1037/tps0000281
Schwarzer, R., and Jerusalem, M. (1995). “Generalized self-efficacy scale,” in Measures in health psychology: A user’s portfolio. Causal and control beliefs. Editors J. Weinman, S. Wright, and M. Johnston (Windsor, United Kingdom: NFER-NELSON), 35–37.
Selzer, M. N., Gazcon, N. F., and Larrea, M. L. (2019). Effects of virtual presence and learning outcome using low-end virtual reality systems. Displays 59, 9–15. doi:10.1016/j.displa.2019.04.002
Sevinc, V., and Berkman, M. I. (2020). Psychometric evaluation of Simulator Sickness Questionnaire and its variants as a measure of cybersickness in consumer virtual environments. Appl. Ergon. 82, 102958. doi:10.1016/j.apergo.2019.102958
Shi, Y., Zhu, Y., Mehta, R. K., and Du, J. (2020). A neurophysiological approach to assess training outcome under stress: A virtual reality experiment of industrial shutdown maintenance using Functional Near-Infrared Spectroscopy (fNIRS). Adv. Eng. Inf. 46, 101153. doi:10.1016/j.aei.2020.101153
Shute, V. J. (2009). Simply assessment. Int. J. Learn. Media 1 (2), 1–11. doi:10.1162/ijlm.2009.0014
Simões, B., Creus, C., Carretero, M. d. P., and Guinea Ochaíta, Á. (2020). Streamlining XR technology into industrial training and maintenance processes. 25th Int. Conf. 3D Web Technol., 1–7. doi:10.1145/3424616.3424711
Smith, S. J., Farra, S. L., Ulrich, D. L., Hodgson, E., Nicely, S., and Mickle, A. (2018). Effectiveness of two varying levels of virtual reality simulation. Nurs. Educ. Perspect. 39 (6), E10–E15. doi:10.1097/01.nep.0000000000000369
Song, H., Kim, T., Kim, J., Ahn, D., and Kang, Y. (2021). Effectiveness of VR crane training with head-mounted display: double mediation of presence and perceived usefulness. Automation Constr. 122, 103506. doi:10.1016/j.autcon.2020.103506
Souchet, A. D., Philippe, S., Lévêque, A., Ober, F., and Leroy, L. (2022). Short and long-term learning of job interview with a serious game in virtual reality: influence of eyestrain, stereoscopy, and apparatus. Virtual Real. 26 (2), 583–600. doi:10.1007/s10055-021-00548-9
Sowndararajan, A., Wang, R., and Bowman, D. A. (2008). “Quantifying the benefits of immersion for procedural training,” in Proceedings of the 2008 Workshop on Immersive Projection Technologies/Emerging Display Technologies, Los Angeles, California, August 9 - 10, 2008, 1–4.
Tai, K.-H., Hong, J.-C., Tsai, C.-R., Lin, C.-Z., and Hung, Y.-H. (2022). Virtual reality for car-detailing skill development: learning outcomes of procedural accuracy and performance quality predicted by vr self-efficacy, vr using anxiety, vr learning interest and flow experience. Comput. Educ. 182, 104458. doi:10.1016/j.compedu.2022.104458
van Limpt-Broers, H., Louwerse, M. M., and Postma, M. (2020). Awe yields learning: A virtual reality study. CogSci.
Vygotsky, L. S., and Cole, M. (1978). Mind in society: development of higher psychological processes. Massachusetts, United States: Harvard University Press.
Wang, R., Li, F., Cheng, N., Xiao, B., Wang, J., and Du, C. (2017). “How does web-based virtual reality affect learning: evidences from a quasi-experiment,” in Proceedings of the ACM Turing 50th celebration conference-China, Shanghai, China, May 12 - 14, 2017, 1–7.
Wang, S.-L., and Wu, P.-Y. (2008). The role of feedback and self-efficacy on web-based learning: the social cognitive perspective. Comput. Educ. 51 (4), 1589–1598. doi:10.1016/j.compedu.2008.03.004
Wu, B., Yu, X., and Gu, X. (2020). Effectiveness of immersive virtual reality using head-mounted displays on learning performance: A meta-analysis. Br. J. Educ. Technol. 51 (6), 1991–2005. doi:10.1111/bjet.13023
Yang, C., Kalinitschenko, U., Helmert, J. R., Weitz, J., Reissfelder, C., and Mees, S. T. (2018). Transferability of laparoscopic skills using the virtual reality simulator. Surg. Endosc. 32 (10), 4132–4137. doi:10.1007/s00464-018-6156-6
Zell, E., and Krizan, Z. (2014). Do people have insight into their abilities? A metasynthesis. Perspect. Psychol. Sci. 9 (2), 111–125. doi:10.1177/1745691613518075
Zhang, X., Jiang, S., Ordonez de Pablos, P., Lytras, M. D., and Sun, Y. (2017). How virtual reality affects perceived learning effectiveness: A task–technology fit perspective. Behav. Inf. Technol. 36 (5), 548–556. doi:10.1080/0144929x.2016.1268647
Keywords: learning, self-efficacy, behavior, virtual reality, structural equation modeling
Citation: Mousavi SMA, Powell W, Louwerse MM and Hendrickson AT (2023) Behavior and self-efficacy modulate learning in virtual reality simulations for training: a structural equation modeling approach. Front. Virtual Real. 4:1250823. doi: 10.3389/frvir.2023.1250823
Received: 30 June 2023; Accepted: 27 September 2023;
Published: 23 October 2023.
Edited by:
Heng Luo, Central China Normal University, ChinaReviewed by:
Katharina Petri, Otto von Guericke University Magdeburg, GermanyAdnan Fateh, University of Central Punjab, Pakistan
Copyright © 2023 Mousavi, Powell, Louwerse and Hendrickson. This is an open-access article distributed under the terms of the Creative Commons Attribution License (CC BY). The use, distribution or reproduction in other forums is permitted, provided the original author(s) and the copyright owner(s) are credited and that the original publication in this journal is cited, in accordance with accepted academic practice. No use, distribution or reproduction is permitted which does not comply with these terms.
*Correspondence: S. M. Ali Mousavi, c21vdXNhdmlAdXZ0Lm5s