- 1The Laboratory of Ecological Genetics, Vavilov Institute of General Genetics, Russian Academy of Sciences, Moscow, Russia
- 2The Laboratory of Molecular Immunology, Rogachev National Research Center of Pediatric Hematology, Oncology and Immunology, Moscow, Russia
- 3The Laboratory of Clinical Pathophysiology of Critical Conditions, Federal Research and Clinical Center of Intensive Care Medicine and Rehabilitology, Moscow, Russia
The recent report of the International Union of Immunological Societies (IUIS) has provided the categorized list of 354 inborn errors of immunity. We performed a systematic analysis of genes and diseases from the IUIS report with the use of the OMIM, ORPHANET, and HPO resources. To measure phenotypic similarity we applied the Jaccard/Tanimoto (J/T) coefficient for HPO terms and top-level categories. Low J/T coefficients for HPO terms for OMIM or ORPHANET disease pairs associated with the same genes indicated high pleiotropy of these genes. Gene ORGANizer enrichment analysis demonstrated that gene sets related to HPO top-level categories were most often enriched in immune, lymphatic, and corresponding body systems (for example, genes from the category “Cardiovascular” were enriched in cardiovascular system). We presented available data on frequent and very frequent clinical signs and symptoms in inborn errors of immunity. With the use of DisGeNET, we generated the list of 25 IUIS/OMIM diseases with two or more relatively high score gene-disease associations, found for unrelated genes and/or for clusters of genes coding for interacting proteins. Our study showed the enrichment of gene sets related to several IUIS categories with neoplastic and autoimmune diseases from the GWAS Catalog and reported individual genes with phenotypic overlap between inborn errors of immunity and GWAS diseases/traits. We concluded that genetic background may play a role in phenotypic diversity of inborn errors of immunity.
Introduction
Rare diseases, being individually rare, altogether affect millions of people. Different estimates exist: rare diseases affect 10% of Americans (1) or 6–8% of Europeans (in total, more than 30 million citizens) (2). The prevalence of primary immunodeficiencies (PIDs) is reported at about 1:2,000 live births (3). Patients with rare diseases encounter with diagnostic difficulties. In the report produced by EURORDIS in 2007, 40% of rare disease patients first received an erroneous diagnosis, of which 25% had to wait for the correct diagnosis between 5 and 30 years. The patient or family was not informed on the genetic origin of the disease in 25% of cases (4). Since this publication, an enormous progress has been achieved in the genetic characterization of known diseases, in the search for new phenotypes and in the progress in rare disease differential diagnosis, however, the problem continues to be relevant. With the information explosion, both new opportunities and challenges constantly arise. The recent report of the International Union of Immunological Societies (IUIS) on inborn errors of immunity (5) has provided the categorized list of 354 inborn errors of immunity (as of 2017). Preliminary estimates indicate that in the next decade the number of PIDs may be as high as 3000 (6). As information accelerates, there is a constant need for the development of resources, algorithms and approaches to capture, process and analyze all this information.
With the manifold possible manifestations of inborn errors of immunity, careful characterization of gene-phenotype relationships is clinically relevant. Detailed reviews on rare individual disorders are available for many PIDs, however, the complex analysis of these disorders with the use of modern resources might shed light on some regularities in gene-disease interactions. The IUIS report may serve as a starting point for different types of in depth analysis of genes, diseases, and categories of inborn errors of immunity.
The study was designed to perform a systematic analysis of genes and diseases from the IUIS report. We set out to characterize gene-disease categories and the whole gene-disease set with the use of several resources widely known in the rare disease community: OMIM (7), ORPHANET (8), and HPO (9). We aimed to provide some new results for gene-disease associations available from public data and to attract the attention of researchers to the possible influence of common genetic variants on the phenotypic variability of inborn errors of immunity.
Materials and Methods
The OMIM, ORPHANET, and HPO Resources
OMIM and ORPHANET are the two largest rare disease public resources. OMIM is a continuously updated catalog of human genes and genetic phenotypes of all known Mendelian disorders (7). OMIM content is generated with the use of published peer-reviewed biomedical literature. It includes over 25,000 entries with approximately 9,000 of them related to phenotypes (as of 28 June 2019). OMIM information is provided as free text; genes and phenotypes are described in separate entries and have unique digit identifiers (MIM numbers). Orphanet is a European initiative aimed at collecting expert-vetted data on rare diseases and their associated genes from multiple information resources (8). ORPHANET provides data for 21,566 diseases with all available synonyms (as of 18 February 2019). ORPHANET contains rare diseases ontology (ORDO) with a systematic vocabulary for rare diseases, relationships between diseases, genes associated with these diseases and other relevant features. The Human Phenotype Ontology (HPO) is organized as a standardized vocabulary integrating data on phenotypic abnormalities from literature and rare disease resources (9). It currently contains over 13,000 terms and over 156,000 annotations to hereditary diseases (release date June 16, 2019). The HPO data may be queried for genes, HPO terms and diseases (separately for OMIM diseases and ORPHANET diseases).
We searched OMIM and ORPHANET for genes from the IUIS report and assessed the compliance of associated diseases and phenotypes with those presented in the IUIS report. The HPO database was first queried for genes and then for associated HPO terms and diseases (separately for OMIM diseases and ORPHANET diseases). For further analysis we exported disease-associated HPO term names together with their top-level categories.
The DisGeNET Resource
DisGeNET is one of the largest and comprehensive repositories of human gene-disease associations (10). It comprises data on 628,685 gene-disease associations between 17,549 genes and 24,166 diseases/traits (as of January 2019). DisGeNET includes associations for Mendelian, complex, environmental and rare diseases, and disease-related traits.
We queried DisGeNET separately for genes and OMIM and/or ORPHANET diseases for summaries of all gene-disease and variant-disease associations, which included, in particular, original metrics describing scores for these associations, disease specificity and pleiotropy indexes. To improve reliability, we mainly focused on records with gene-disease association score ≥ 0.3. The metrics were used to evaluate genotype-phenotype relationships.
Jaccard/Tanimoto Similarity Test
The Jaccard/Tanimoto coefficient is the ratio of intersection to union used to measure similarity between binary data (11). It estimates the ratio of the numbers of the elements in the intersecting and union sets as the measure of similarity (it equals to zero in the absence of intersecting elements and equals to one when all elements overlap). The Jaccard/Tanimoto coefficient was calculated by the equation
(Na- number of elements in set A; Nb- number of elements in set B; Nc- number of elements in intersecting set) for pairwise testing of means. Jaccard/Tanimoto coefficients significance was calculated with a CRAN R package (12). To correct for multiplicity we applied Benjamini and Hochberg false discovery rate (FDR) with a threshold < 0.05.
Gene Set Enrichment Analysis
Enrichment analysis is a leading computational method for analyzing gene sets in the context of prior biological knowledge. We implemented a phenotype-based tool Gene ORGANizer for the enrichment analysis focused on body systems (13). Gene ORGANizer comprises HPO and DisGeNET phenotype associations for more than 7,000 genes. For the IUIS/OMIM diseases with more than one high-score gene-disease association in the DisGeNET database we applied enrichment for protein-protein interactions (PPI) conducted with the Search Tool for the Retrieval of Interacting Genes (STRING) database (14). We analyzed human PPI dataset taking the combined score > 0.4 as the cut-off criterion. Overlap with gene set library from the NHGRI-EBI GWAS Catalog (GWAS Catalog 2019) was assessed with the Enrichr tool (15). We set Benjamini and Hochberg false discovery rate (FDR) to 5% (corrected for multiplicity P value < 0.05).
Results
Disease Matching in the IUIS, OMIM, and ORPHANET Databases
We defined a total of 331 unique genes (further IUIS genes) linked to 300 unique diseases (further IUIS diseases) from eight of nine IUIS categories (5). One category “Phenocopies of inborn errors of immunity,” which includes non-inborn errors of immunity was not covered by our analysis. For disease matching, we used gene and disease names and associated features from the IUIS tables, and the OMIM and ORPHANET information. ORPHANET differs from OMIM and IUIS by the level of granularity. In the ORPHANET database, individual gene-linked disorders may be related to parent diseases associated with a group of genes. For example, seven OMIM Aicardi-Goutieres syndrome phenotypes (Aicardi-Goutieres syndrome 1–7) are merged into one ORPHANET disease “Aicardi-Goutières syndrome.” OMIM-ORPHANET matching was facilitated by the fact, that ORPHANET diseases have a link to the relevant phenotype in the OMIM database. Although the IUIS report also contains OMIM numbers for gene-disease pairs, these numbers are often related to gene, not phenotype descriptors. One gene may be associated with several clinical phenotypes indicated by Phenotype MIM number. For example, the IUIS disease “β actin deficiency” (gene ACTB) corresponds to MIM phenotypes “Baraitser-Winter syndrome 1” (243310) and “Dystonia, juvenile-onset” (607371), or “Adenosine deaminase (ADA) deficiency” (gene ADA) in the IUIS Report is related to “Omenn Syndrome” (603554) and “Severe Combined Immunodeficiency, Autosomal Recessive, T Cell-negative, B Cell-negative, NK Cell-negative, Due To Adenosine Deaminase Deficiency” (102700). In some cases, the IUIS-OMIM disease matching was a judgment call based on the analysis of literature and OMIM full text descriptions. All IUIS gene-disease pairs were matched with corresponding OMIM and/or ORPHANET gene-disease pairs (in total 300 gene-disease pairs in one or both databases). Matching of the IUIS diseases provided 329 MIM phenotypes and 262 ORPHANET diseases (Supplementary Table 1, Sheet “IUIS_Diseases”). The IUIS genes were also associated with some other rare diseases (Supplementary Table 1, Sheet “non-IUIS_Diseases”).
Representation and Characterization of the IUIS Diseases in the ORPHANET Database
ORPHANET annotations for the diseases associated with the IUIS genes are presented in Figure 1. These annotations were generated using information from Supplementary Table 1. ORPHANET level of granularity determines the association of one disease with one or several genes. This feature is reflected in Figures 1A,B displaying age of onset and mode of inheritance of the IUIS and non-IUIS diseases as indicated exactly and indicated among others (for multigene associations). The IUIS diseases predominantly manifest in neonatal age, infancy and childhood, while non-IUIS diseases may manifest throughout life (Figure 1A). Autosomal recessive (AR) and autosomal dominant (AD) modes of inheritance are prevalent among the IUIS diseases. AR, AD, and muligenic/multifactorial modes of inheritance are similar in frequency among non-IUIS diseases (Figure 1B). As expected, such ORPHANET classifications as rare genetic diseases, rare immunological diseases and rare disorders potentially indicated for transplant are related to the vast majority of the IUIS diseases. Among non-IUIS diseases, neurological and neoplastic diseases are relatively numerous (Figure 1C). The most rare disease prevalence (< 1/1,000,000) is typical for the IUIS diseases and not strongly prevails among non-IUIS diseases (Figure 1D). In contrast to non-IUIS diseases, the IUIS diseases are mainly associated with a single gene (Figure 1F). The distribution of the number of ORPHANET classifications for the IUIS and non-IUIS diseases is reflected in Figure 1G. A small overlap between the IUIS and non-IUIS diseases (Figure 1G) is explained by the ORPHANET level of granularity.
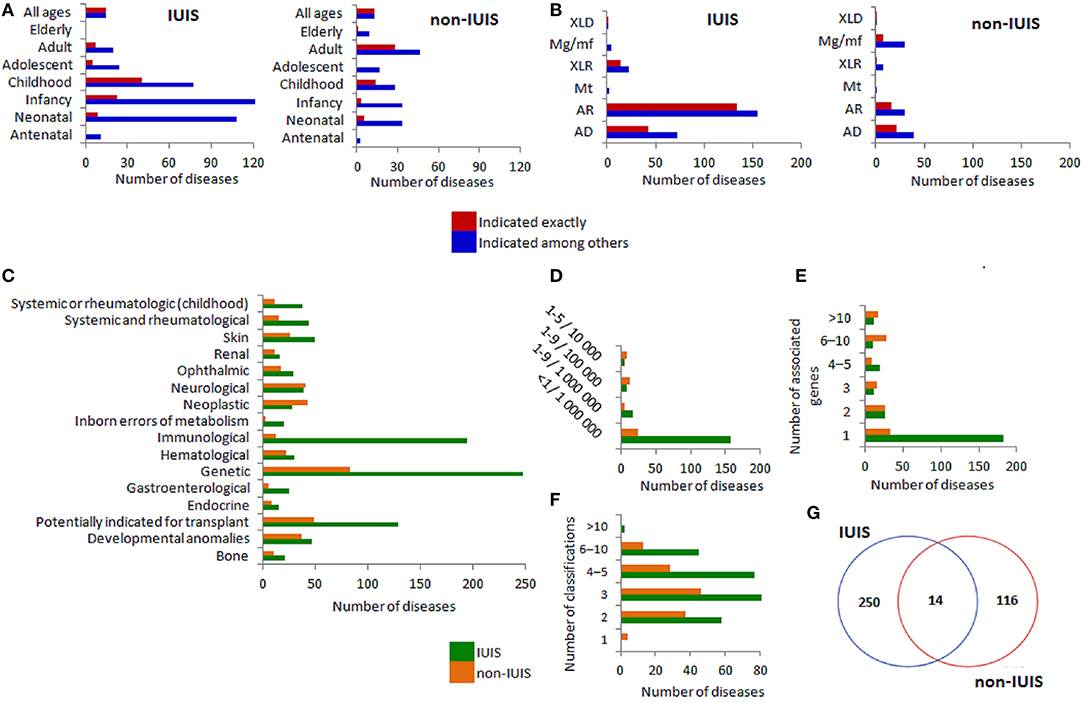
Figure 1. ORPHANET annotations for IUIS/non-IUIS diseases. The distribution of the diseases associated with the IUIS genes by (A) age of onset of the IUIS diseases (left) and non-IUIS diseases (right), (B) mode of inheritance for the IUIS diseases (left) and non-IUIS diseases (right), (C) ORPHANET classifications for rare diseases (classifications including 10 or more diseases are presented), (D) prevalence, (E) number of associated genes, (F) number of classifications per disease, (G) gene-centered Venn diagram for the IUIS- and non-IUIS diseases in the ORPHANET database. (A,B) Since many diseases were associated with more than one gene, we characterized found associations (Y-axis) as indicated among others (different genes are linked to several variants of disease manifestation) and indicated exactly (corresponds to the axis labels). AD, Autosomal dominant; AR, Autosomal recessive; Mt, Mitochondrial inheritance; XLR, X-linked recessive; Mg/mf, Multigenic/mulifactorial; XLD, X-linked dominant.
Summarizing ORPHANET data, we may state that inborn errors of immunity were mainly represented by immunological diseases with autosomal recessive or autosomal dominant inheritance, very rare prevalence (< 1/1,000,000) and early age of disease manifestation. The majority of diseases were related to more than two classifications.
HPO Terms and Top-Level Categories for the Genes Associated With Inborn Errors of Immunity
The HPO data on phenotypic abnormalities, associated with the IUIS genes, are presented in Figure 2. In total, there were 10,506 HPO terms, among them, 2,229 terms were unique. Ten top-level HPO categories (blood and blood-forming tissues; cardiovascular; digestive system; eye; genitourinary system; head and neck; immunology; nervous system; skeletal system; skin, hair, and nails) included the largest numbers of HPO terms (in total, and unique terms) (Figure 2A). The distribution of the top ten HPO terms mapped to these HPO categories is presented in Supplementary Figure 1. The greatest number and variety of HPO terms is related to three top-level categories (nervous system, head and neck, and immunology) (Figure 2A). The enrichment analysis focused on 12 body systems showed that gene sets related to HPO categories were most often enriched in immune, lymphatic and corresponding body systems (for example, genes from the category “Cardiovascular” were enriched in cardiovascular system). The unexpected exclusion was “Nervous system” (Figure 2B). The genetic similarity between pairs of 11 HPO categories assessed by the Jaccard/Tanimoto coefficient was relatively high and significant (with one exception) (Figure 2C). The Jaccard/Tanimoto coefficient was used to measure the phenotypic similarity of diseases associated with the same gene. In the OMIM and ORPHANET databases, 99 and 43 genes related to ≥ 2 diseases were linked to 236 and 110 unique disease pairs, respectively. This gene-based approach revealed a weak similarity at the HPO term level and a rather high similarity at the HPO category level (Figure 2D). The Jaccared/Tanimoto coefficients were relatively high for the OMIM-ORPHANET matched disease pairs (n = 136) (Figure 2E). The Jaccared/Tanimoto coefficients for the HPO terms and categories are presented in Figure 2F. At the level of HPO terms, significant differences were revealed between the Jaccared/Tanimoto coefficients related to OMIM (gene-based comparison) and OMIM-ORPHANET (disease-based comparison) (Mann-Whitney test: P = 3.185E−11) and ORPHANET (gene-based comparison) and OMIM-ORPHANET (disease-based comparison) (Mann-Whitney test: P = 5.98E−12). At the level of HPO categories, the differences were less pronounced (OMIM and OMIM-ORPHANET P = 5.75E-04; ORPHANET; and OMIM-ORPHANET P = 6.10E-02, non-significant). Despite the differences in the OMIM and ORPHANET hierarchy for disease classification, the comparison yielded higher Jaccared/Tanimoto coefficients for the matched OMIM-ORPHANET diseases than for the disease pairs associated with the same genes.
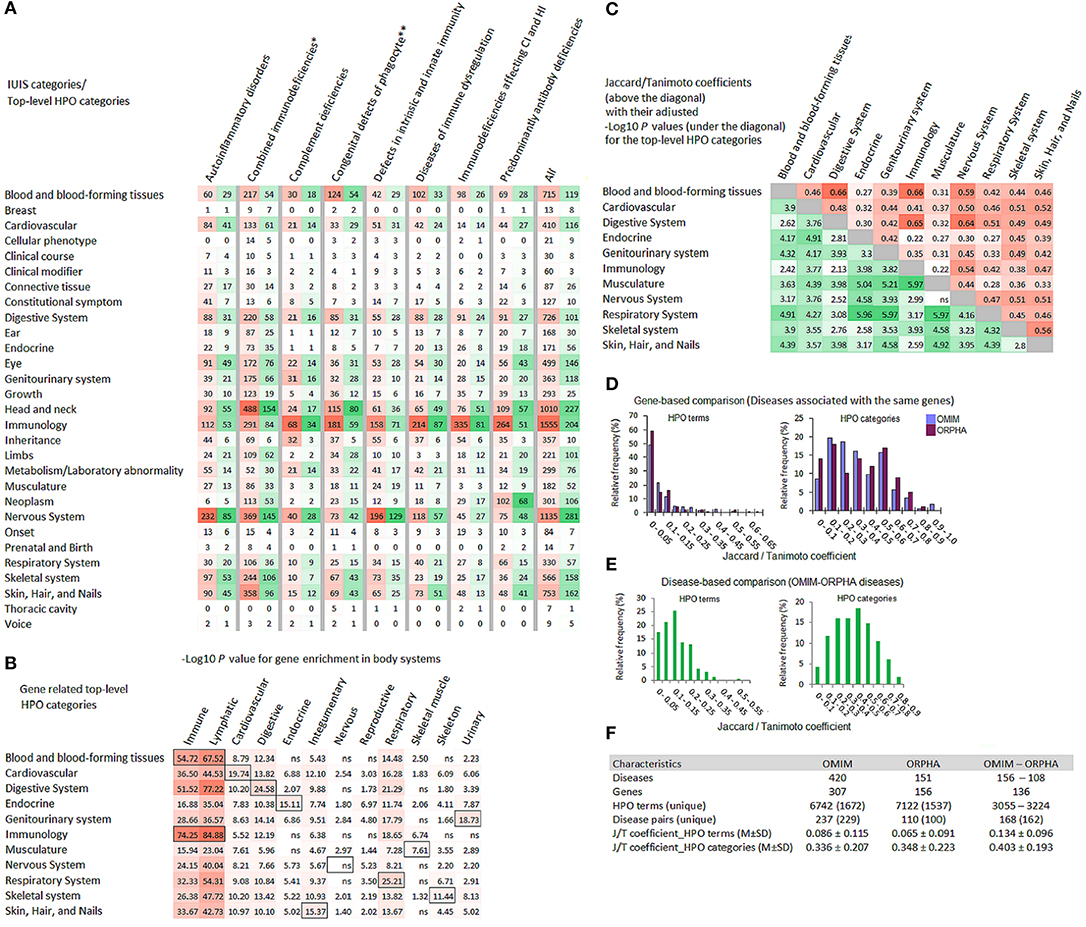
Figure 2. HPO annotations for the genes associated with inborn errors of immunity. (A) Multiple heat-map matrix for HPO terms related to top-level HPO categories (Y-axis) in the diseases grouped by the IUIS categories (X-axis). Since many HPO terms were associated with several genes, the data are presented as sum of their occurrences, i.e., the total number (red) and unique number (green) of HPO terms. For the IUIS categories all heat-maps are independent to each other. (B) Heat-map matrix for the results of Gene ORGANizer enrichment analysis. Gene lists related to top-level HPO categories (Y-axis) were analyzed for their overrepresentation in body systems (X-axis). FDR adjusted enrichment P values (threshold < 0.05) represented as –Log10 (threshold > 1.30) are plotted against HPO categories. Cells with P values for body systems matched with HPO categories are highlighted with black boundaries. (C) Heat-map matrix for the genetic similarity between selected top-level HPO categories. Jaccard/Tanimoto coefficient values are colored in red; their FDR adjusted P values represented as –Log10 are colored in green. (D) The Jaccard/ Tanimoto coefficient distribution for HPO terms and top-level HPO-categories for OMIM and ORPHANET diseases associated with the same genes. (E) The Jaccard/Tanimoto coefficient distribution for HPO terms and top-level HPO-categories for matched OMIM-ORPHANET disease pairs. (F) Summary statistics for the overlap measures. CI, cellular immunity; FDR, false discovery rate; HI, humoral immunity; J/T, Jaccard/Tanimoto; ns, non-significant; ORPHA, ORPHANET. *Combined immunodeficiencies with associated or syndromic features; **Congenital defects of phagocyte number or function.
Briefly, this part of our study presents an overview of HPO terms and their top-level categories associated with the IUIS genes. The enrichment analysis via Gene ORGANizer provided information on the overrepresentation of HPO terms at the level of 12 body systems assessed in a genomic context. Different HPO categories were represented by highly overlapped gene spectra. The analysis of phenotypic similarity measured by the Jaccard/Tanimoto coefficient showed that although diseases associated with the same genes are very different in their clinical signs and symptoms, the latter are related to the limited number of HPO top-level categories, i.e., mainly affect certain body systems.
Frequent and Very Frequent Clinical Signs and Symptoms in the Inborn Errors of Immunity
ORPHANET provides data on symptom frequency. Currently, these data are available for 110 inborn errors of immunity. The list of 110 diseases is given in Supplementary Table 2. Among the disease categories, the largest number of annotated diseases is related to “Combined immunodeficiencies with associated or syndromic features” (Figure 3A). All frequent and very frequent clinical signs and symptoms occurring in three or more diseases are provided in Figures 3B–S. Frequent symptoms occur in 79%−30% of patients; very frequent symptoms are typical for 80%−99% of patients. The greatest symptom variety is revealed for the following top-level HPO categories: “immunology,” n = 38; “head and neck,” n = 33; and “nervous system,” n = 20.
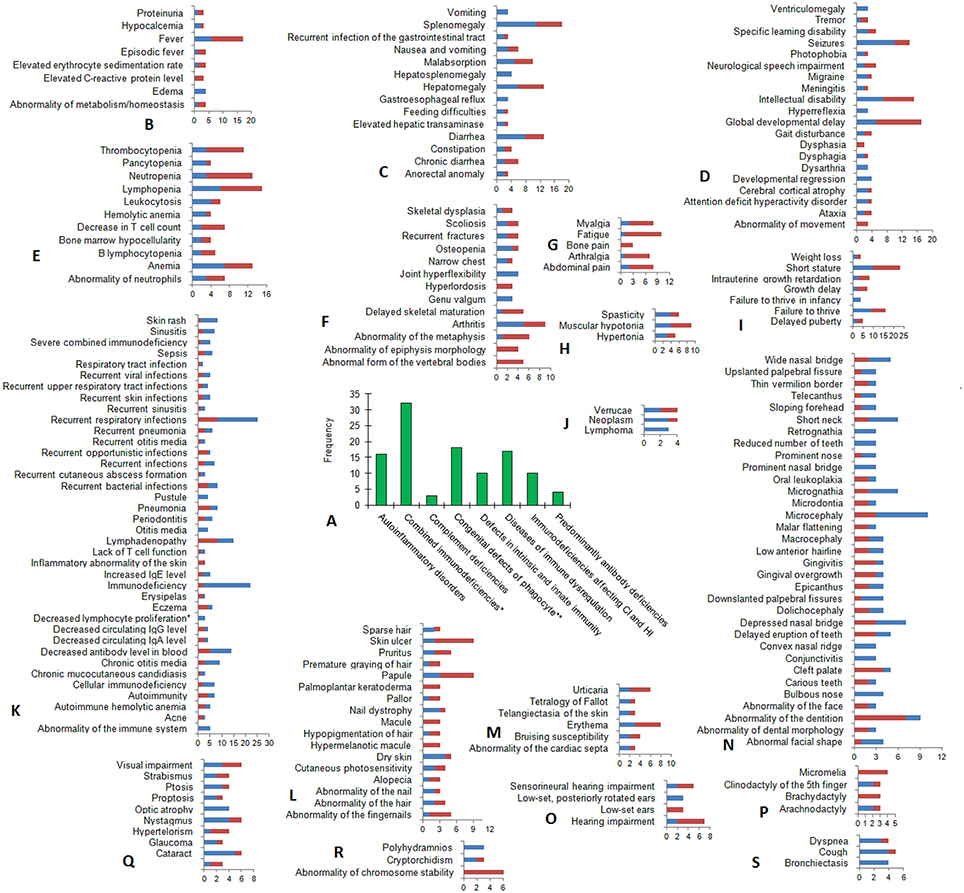
Figure 3. Frequent and very frequent clinical signs and symptoms in diseases matched between IUIS and ORPHANET. (A) The distribution of diseases within the IUIS categories (for 110 diseases with available annotations on symptom frequency). (B–S) The frequency of occurrence (X-axis) of frequent (dark red) and very frequent (blue) clinical signs and symptoms (Y-axis) is mapped to HPO term names within top-level HPO categories: (B) Metabolism/Laboratory abnormality, (C) Digestive System, (D) Nervous System, (E) Blood and blood-forming tissues, (F) Skeletal system, (G) Constitutional Symptom, (H) Musculature, (I) Growth, (J) Neoplasm, (K) Immunology, (L) Skin, Hair, and Nails, (M) Cardiovascular, (N) Head and neck, (O) Ear, (P) Limbs, (Q) Eye, (R) Miscellaneous: Genitourinary system (Cryptorchidism), Prenatal and Birth (Polyhydramnios) and Cellular phenotype (Abnormality of chromosome stability), (S) Respiratory System. *Combined immunodeficiencies with associated or syndromic features; **Congenital defects of phagocyte number or function. CI, cellular immunity; HI, humoral immunity.
Gene-Disease Association Score and IUIS/OMIM Diseases With More Than One High-Score Association
The DisGeNET resource collects data on gene-disease associations for a wide range of diseases, including rare diseases. It ranges gene-disease associations by score, which depends on the number and type of sources and the number of publications in support of the association. We divided all gene-disease associations found for inborn errors of immunity in the DisGeNET resource (in total, 179 diseases) into three groups: S1, with gene-disease association score ≥ 0.3; S2, with gene-disease association score 0.1– 0.29; S3 with gene-disease association score < 0.1. The full list of the genetic associations and their scores is presented in Supplementary Table 3. Inborn errors of immunity (n = 25) with more than one high-score association in the DisGeNET database are listed in Table 1. The PPI enrichment analysis showed that these high score gene-disease associations were found for both, clusters of encoded interacting proteins and unrelated proteins. The IUIS/OMIM gene-disease associations usually have the highest scores; however, other disease associations may also matter.
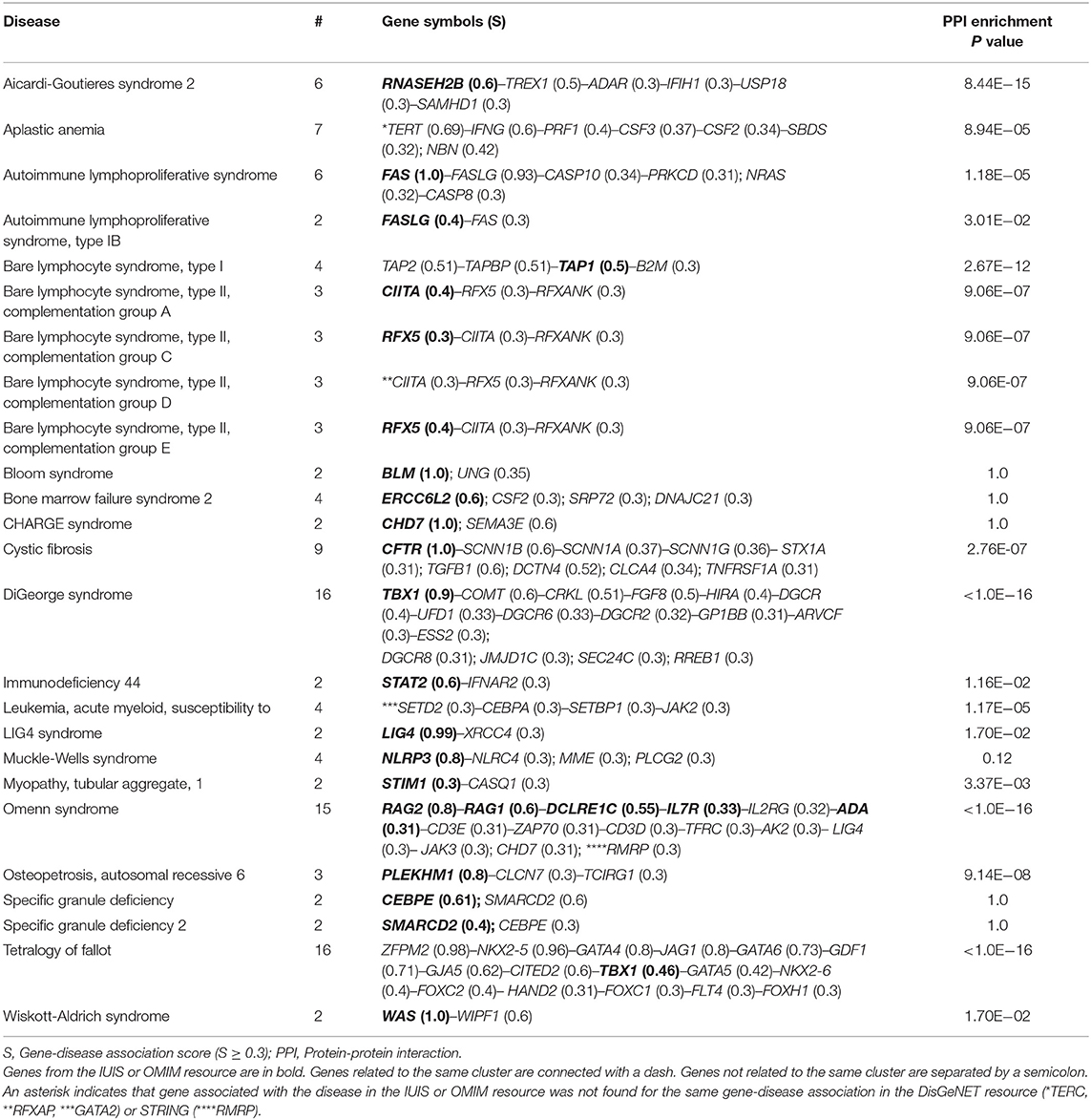
Table 1. IUIS/OMIM diseases with more than one high-score gene-disease association in the DisGeNET database.
Given these results, the most prominent gene candidates for inborn errors of immunity with unidentified genetic abnormalities in known genes are often represented by other genes involved in the PPI network.
Specificity and Pleiotropy of Genes Associated With Inborn Errors of Immunity
DisGeNET comprises 6,780 disease associations for the IUIS genes with in total 36,169 gene-disease association pairs. To address the item on gene specificity and pleiotropy, we used DisGeNET metrics, which include, in particular, the Disease Sensitivity Index (DSI) and the Disease Pleiotropy index (DPI). DSI is inversely proportional to the number of diseases associated with the corresponding gene. DPI is calculated taking into account whether the diseases associated with the gene are similar or completely different and refer to different classes of diseases. DSI and DPI were available for 255 IUIS genes. The distribution of DSI and DPI for these genes is presented in Figures 4A,B. The scatterplot displaying the relationship between DSI and DPI is produced in Figure 4C. Ten top values are indicated for DSI (15 genes) and DPI (16 genes). The distribution of the number of associated diseases is shown in Figure 4D. Large numbers of associated diseases per gene are explained by the fact that all associations are taken into account regardless of their scores.
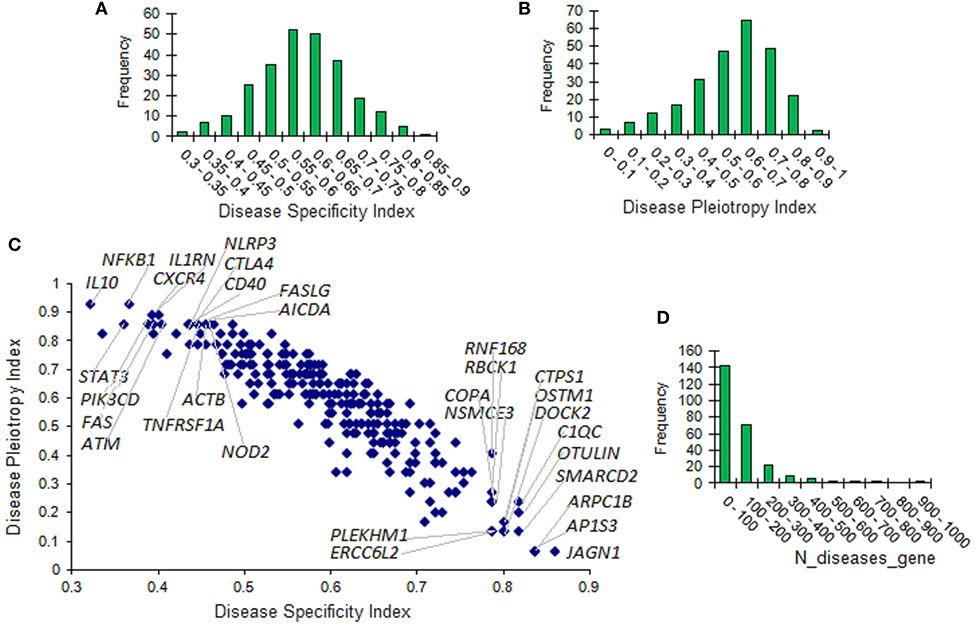
Figure 4. Disease specificity and disease pleiotropy indexes of genes associated with inborn errors of immunity. (A) The distribution of the disease specificity index (DSI). (B) The distribution of the disease pleiotropy index (DPI). (C) Scatterplot of DSI and DPI. Ten top values are presented for DSI (15 genes) and DPI (16 genes). (D) The distribution of the number of associated diseases.
Besides gene-disease associations, DisGeNET also provides information on variant-disease associations. From the total of 11,884 variants in the IUIS genes, 5,084 variants had data on allele frequency. The minimum alternative allele frequency (AF) was 3.18E−05; 2,999 variants had AF > 0.05. Variant-disease association scores in the range of 0.65–1.0 were registered for 2,657 of 5,084 variants. These data are presented in Supplementary Figure 2.
Phenotypic and Genetic Overlap Between Inborn Errors of Immunity and Complex Traits
Given that all IUIS genes are associated with several or many diseases, and a lot of these associations have been found for common genetic variants, we searched the NHGRI-EBI GWAS Catalog (16) for the genes of inborn errors of immunity in order to see if there is phenotypic and genetic overlap between inborn errors of immunity and complex traits. A comparison of HPO terms and GWAS mapped traits yielded 35 genes with overlapping phenotypes, among them autoimmune and neoplastic phenotypes were highly represented (Table 2). The largest number of associations with neoplasms has been registered for the TERT gene (22 phenotypes).
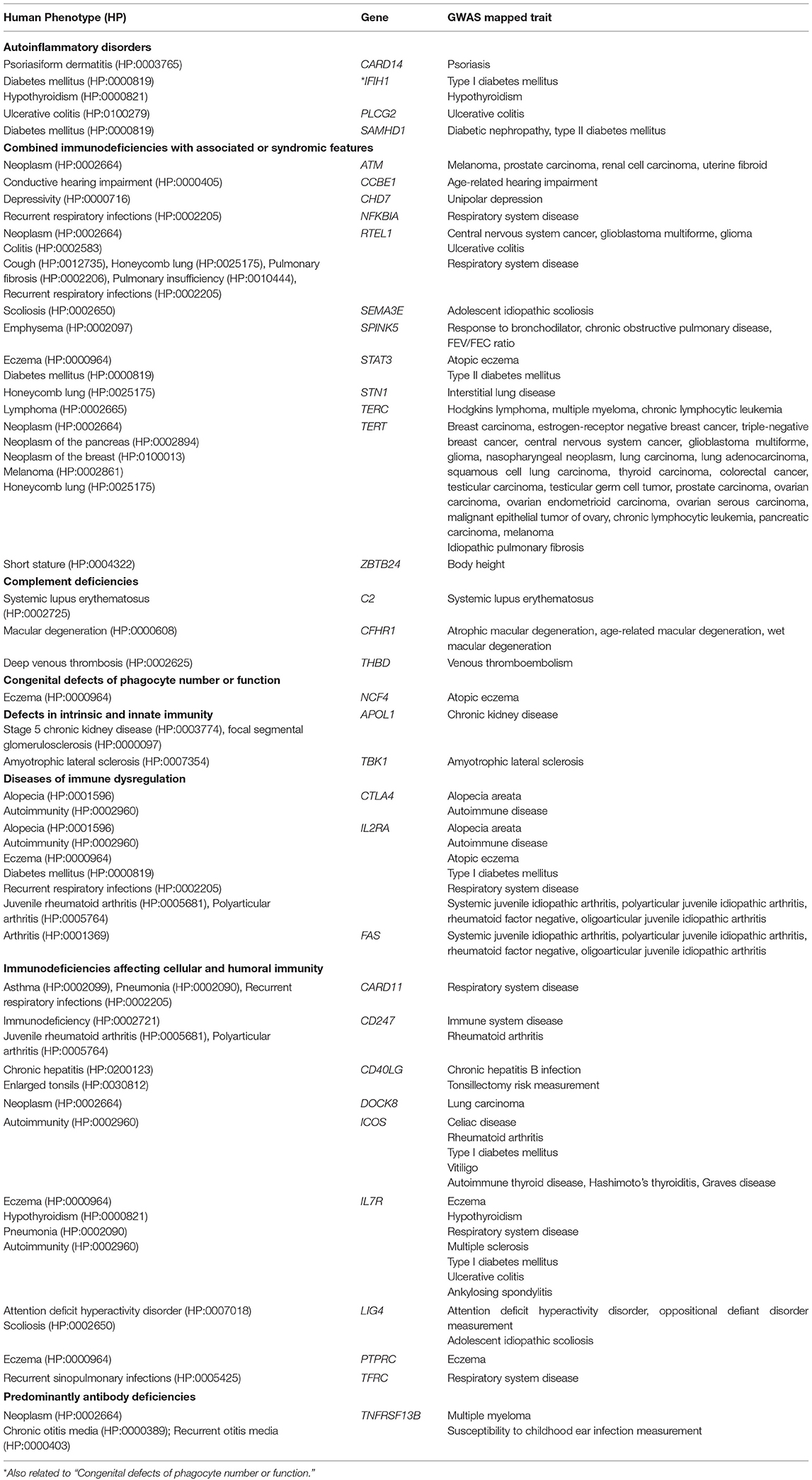
Table 2. Overlap between HPO terms and GWAS mapped traits for the genes of inborn errors of immunity.
Next, we performed the enrichment analysis with the GWAS Catalog 2019 gene set library within the Enrichr tool. The analysis was accomplished for eight IUIS categories and for the whole gene set (Figure 5, Supplementary Table 4). Disease classification was based on the Experimental Factor Ontology (EFO), the resource within the GWAS Catalog documentation. Two main results supporting previous findings are worthy of attention. First, enriched immune-related phenotypes were mainly represented by autoimmune conditions. Second, different combinations of ten genes (RTEL1, TERT, TERC, STN1, ATM, SP110, SEMA3E, TBX1, DNMT3B, CDCA7) related to the category “Combined immunodeficiencies with associated or syndromic features” were overrepresented in 11 neoplastic diseases/traits. “Other” mapped trait for this category was “telomere length.”
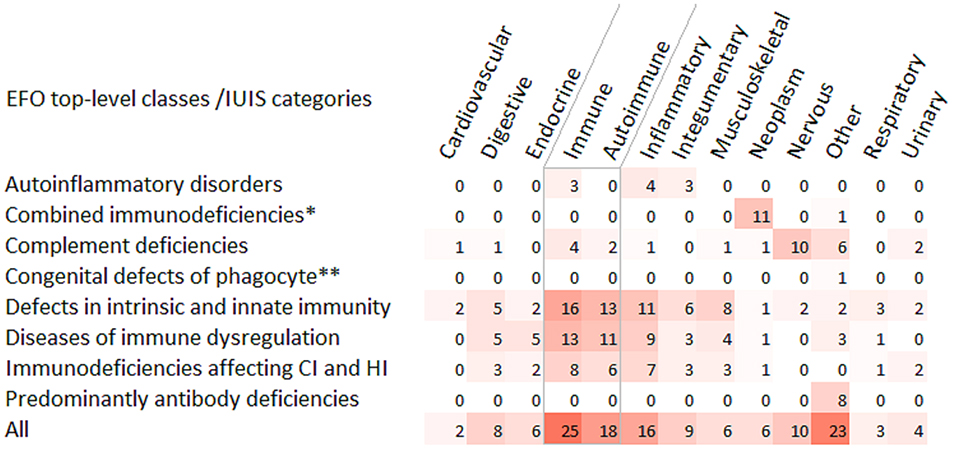
Figure 5. Heat-map matrix for the GWAS Catalog 2019 gene-set enrichment analysis. The IUIS genes were analyzed for their overrepresentation in common diseases/traits. The diseases/traits were classified according the Experimental Factor Ontology (EFO).The number of overrepresented common diseases/traits was indicated for EFO top-level categories (X-axis) within the IUIS categories of diseases (Y-axis). In the group of immune diseases, the subgroup of autoimmune conditions is highlighted with gray boundaries. *Combined immunodeficiencies with associated or syndromic features; **Congenital defects of phagocyte number or function. CI, cellular immunity; HI, humoral immunity.
Findings presented in this section of our analysis are useful for understanding of phenotypic diversity of inborn errors of immunity. Gene sequencing techniques have become more widely available and will be soon routine methods. The analysis of genes whose genetic variants may influence clinical course of the genetic disease will be useful for management of patients with primary immunodeficiencies.
Discussion
In this work, we provided some additional information on inborn errors of immunity summarized in the recent IUIS report (5). Using the ORPHANET resource we compared and characterized the IUIS and non-IUIS rare diseases associated with the IUIS genes. These genes were linked with a lot of phenotypes related to 29 top-level HPO categories in the HPO database. We estimated the phenotypic similarity between disease pairs associated with the same genes and between matched OMIM-ORPHANET disease pairs. Although the majority of inborn errors of immunity are considered monogenic, it is not always possible to identify damage in the corresponding gene. As far as medical and genomic data on rare diseases is progressively increasing, some of monogenic disorders may turn out to be associated with more than one gene. We reported additional relatively high score gene-disease associations for inborn errors of immunity. We found that all genes from the IUIS report were associated with several or many complex diseases. Genetic overlap between rare and common diseases is an important and clinically relevant problem, which is currently at the forefront of research (17, 18). We showed the enrichment of gene sets related to several IUIS categories with neoplastic and autoimmune diseases from the GWAS Catalog and reported individual genes with phenotypic overlap between inborn errors of immunity and GWAS diseases/traits. Our work was aimed not only at gaining new knowledge, but also at demonstrating the possibilities and the necessity of communication between clinicians and geneticists to ensure progress in the direction of precision medicine of primary immunodeficiencies. We conclude with highlighting several important aspects contributing to the overview of phenotypic diversity of inborn errors of immunity.
Linking genes of inborn errors of immunity with the HPO terms showed a huge variety of symptoms with the involvement of many organs and systems. With the use of a phenotype-based tool Gene ORGANizer, we demonstrated that gene sets related to HPO categories were most often enriched in immune, lymphatic, and corresponding body systems (cardiovascular genes in cardiovascular system, endocrine genes in endocrine system, etc.); however no enrichment was revealed for nervous system. The latter fact may be due to the nervous system related content of background information in Gene ORGANizer, which is discussed in the relevant paper (13). Besides, we assumed that certain nervous system abnormalities may be secondary relative abnormalities in other body systems. Neurological complications may occur as a result of infections (19), vascular problems (20), metabolic disturbances (21), treatment complications (22) and other health problems in inborn errors of immunity. The diagnostic and therapeutic delay in PIDs may lead to the development of irreversible organ damage (23–25). The diversity of symptoms complicates their diagnosis as features accompanying primary immunodeficiencies (26). The correct and quick diagnosis of a rare disease strongly depends on the associated clinical phenotypes and their recognition by physicians (1). We believe that our report on frequent and very frequent symptoms in inborn errors of immunity may be useful for accelerated and effective management of patients with primary immunodeficiencies.
An interesting finding in our work stems from the analysis of the phenotypic similarity between gene-related phenotypes. The Jaccard/Tanimoto coefficients for the HPO terms related to different rare diseases associated with the same gene were low, pointing to high pleiotropy. These results correlate with high genetic pleiotropy in diseases and traits from DisGeNET, however, pleiotropy due to rare alleles might be more pronounced (27). In contrast to the low Jaccard/Tanimoto coefficients for the HPO terms, the coefficients for the top-level HPO categories were relatively high. This observation supports a modular view of pleiotropy (28). A modular character of gene–trait relationships in particular means that mutations in a certain gene may lead to the development of different, partially overlapping combinations of clinical signs and symptoms with non-random clustering (29). Diseases associated with the same gene might share common biological pathways and affect the same anatomical systems.
Our study reported 25 inborn errors of immunity associated with more than one gene. These inborn errors of immunity were associated with two or more unrelated genes, gene clusters or unrelated genes along with gene clusters (20 gene clusters of 2 to 16 genes). Gene clusters were retrieved from protein-protein interaction (PPI) information, which is increasingly used for the identification of new disease-related proteins since the disease-related genes tend to form closely interconnected clusters in the protein network (30, 31). Some “monogenic” diseases (e.g., Aicardi-Goutieres syndrome 2 (AGS2), Bare lymphocyte syndrome, type II, complementation group A/C/D/E) were associated with one top gene and several else genes more closely linked to another disorder from a given phenotypic spectrum. According DisGeNET, genes TREX1, ADAR, IFIH1, and SAMHD1 associated with AGS1, AGS6, AGS7, and AGS5, respectively, may be also implicated in AGS2. These genes are interconnected within a biological network including also USP18 and the top associated gene RNASEH2B.
Investigations of phenotypic and genetic overlap between monogenic and common diseases are focused on expanding knowledge on both common and rare diseases. Genes associated with rare diseases are usually functionally related to disease-relevant pathways. It has been recently hypothesized that phenotypic overlap might be a general trend for rare and common diseases associated with the same gene (17). Phenotypic overlap between rare and common diseases linked by genomic location indirectly supports the common variant association with the common disease. Genes with common variants associated with a common trait might also carry large-effect variants at the extremes of the trait (32). For most traits, an inverse relationship between the effect size and the allele frequency has been shown (33), however, many small-effect genetic variants in sum may play a greater role than rare large-effect mutations (34). Given these observations, we speculate that common variants in relevant genes and relevant metabolic pathways might modify monogenic diseases in terms of their clinical course and response to treatment. The influence of genetic background on the “monogenic” disease phenotype has been shown for several diseases (35). We reported here that common variants in three genes with gene function related to telomere maintenance (RTEL1, Regulator of Telomere Elongation Helicase 1; TERC, Telomerase RNA Component; TERT, Telomerase Reverse Transcriptase) have been associated with telomere length and multiple neoplasms in GWAS. Both HPO terms and ORPHANET classifications for rare diseases associated with these genes include neoplasms. The second example from our study is related to autoimmune conditions. Four genes CTLA4, IL2RA, ICOS, and IL7R have been associated with a group of autoimmune diseases in GWAS and with autoimmunity in the HPO database. We assume that mutations, rare large-effect variants and polymorphisms influencing the same pathogenic processes might cooperate in determining the severity of the rare disease phenotype.
The main findings of the study and their possible implications are as follows: (i) Genes associated with inborn errors of immunity are pleiotropic being involved in a broad, however non-random spectrum of phenotypic abnormalities. (ii) Summary of frequent and very frequent clinical signs and symptoms may be useful for diagnosis in non-obvious cases and for early detection of possible complications. (iii) Some, if not many inborn errors of immunity can actually be associated with more than one gene. If genetic damage is unknown, the search for causal genes can first start among those encoding interacting proteins. (iv) Phenotypic diversity of inborn errors of immunity depends not only on time of diagnosis and treatment characteristics, but also on mutation penetrance and expressivity, the latter being linked, in particular, to genetic background. Common genetic variants in certain genes might contribute to even greater risk of the development of autoimmune and neoplastic diseases in inborn errors of immunity.
In summary, based on the analysis of gene-disease data from the recent IUIS report (5) we presented some additional knowledge on the relevant genes and phenotypes of inborn errors of immunity. The rapid development of genomic technologies is making possible promotion of personalized medicine. We exemplified the possibilities of using of genomic technologies in the field of inborn errors of immunity. This information and instances may be useful for clinical differential diagnostics and for the development of translational and basic research of primary immunodeficiencies.
Data Availability Statement
The datasets for this manuscript are available in Supplementary Materials or upon request.
Ethics Statement
Ethical review and approval was not required for the study on human participants in accordance with the local legislation and institutional requirements. Written informed consent for participation was not required for this study in accordance with the national legislation and the institutional requirements.
Author Contributions
LS designed the study. EC and LA collected data and prepared figures. LS and SL analyzed and interpreted data. LS wrote the manuscript. LS, EC, LA, and SL approved the final version.
Conflict of Interest
The authors declare that the research was conducted in the absence of any commercial or financial relationships that could be construed as a potential conflict of interest.
Supplementary Material
The Supplementary Material for this article can be found online at: https://www.frontiersin.org/articles/10.3389/fimmu.2019.02475/full#supplementary-material
References
1. Shen F, Zhao Y, Wang L, Mojarad MR, Wang Y, Liu S, et al. Rare disease knowledge enrichment through a data-driven approach. BMC Med Inform Decis Mak. (2019) 19:32. doi: 10.1186/s12911-019-0752-9
2. Rodríguez-Caso L, Reyes-Palomares A, Sánchez-Jiménez F, Quesada AR, Medina MÁ. What is known on angiogenesis-related rare diseases? A systematic review of literature. J Cell Mol Med. (2012) 16:2872–93. doi: 10.1111/j.1582-4934.2012.01616.x
3. Bonilla FA, Khan DA, Ballas ZK, Chinen J, Frank MM, Hsu JT, et al. Practice parameter for the diagnosis and management of primary immunodeficiency. J Allergy Clin Immunol. (2015) 136:1186–205.e1–78. doi: 10.1016/j.jaci.2015.04.049
4. Survey of the delay in diagnosis for 8 rare diseases in Europe. Available online at: https://www.eurordis.org/sites/default/files/publications/Fact_Sheet_Eurordiscare2.pdf (accessed October 8, 2019).
5. Picard C, Bobby Gaspar H, Al-Herz W, Bousfiha A, Casanova JL, Chatila T, et al. International Union of Immunological Societies: 2017 primary immunodeficiency diseases committee report on inborn errors of immunity. J Clin Immunol. (2018) 38:96–128. doi: 10.1007/s10875-017-0464-9
6. Conley ME, Notarangelo LD, Casanova JL. Definition of primary immunodeficiency in 2011: a “trialogue” among friends. Ann N Y Acad Sci. (2011) 1238:1–6. doi: 10.1111/j.1749-6632.2011.06212.x
7. Amberger JS, Bocchini CA, Schiettecatte F, Scott AF, Hamosh A. OMIM.org: online mendelian inheritance in man (OMIM(R)), an online catalog of human genes and genetic disorders. Nucleic Acids Res. (2015) 43:D789–98. doi: 10.1093/nar/gku1205
8. Rath A, Olry A, Dhombres F, Brandt MMC, Urbero B, Ayme S. Representation of rare diseases in health information systems: the Orphanet approach to serve a wide range of end users. Hum Mutat. (2012) 33:803–8. doi: 10.1002/humu.22078
9. Köhler S, Carmody L, Vasilevsky N, Jacobsen JOB, Danis D, Gourdine JP, et al. Expansion of the Human Phenotype Ontology (HPO) knowledge base and resources. Nucleic Acids Res. (2019) 47:D1018–27. doi: 10.1093/nar/gky1105
10. Piñero J, Bravo À, Queralt-Rosinach N, Gutiérrez-Sacristán A, Deu-Pons J, Centeno E, et al. DisGeNET: a comprehensive platform integrating information on human disease-associatedgenes and variants. Nucleic Acids Res. (2017) 45:D833–9. doi: 10.1093/nar/gkw943
11. Rácz A, Bajusz D, Héberger K. Life beyond the Tanimoto coefficient: similarity measures for interaction fingerprints. J Cheminform. (2018) 10:48. doi: 10.1186/s13321-018-0302-y
12. Hornik K. The R FAQ (2018). Available online at: https://CRAN.R-project.org/doc/FAQ/R-FAQ.html (accessed October 8, 2019).
13. Gokhman D, Kelman G, Amartely A, Gershon G, Tsur S, Carmel L. Gene ORGANizer: linking genes to the organs they affect. Nucleic Acids Res. (2017) 45:W138–45. doi: 10.1093/nar/gkx302
14. Szklarczyk D, Franceschini A, Wyder S, Forslund K, Heller D, Huerta-Cepas J, et al. STRING v10: protein-protein interaction networks, integrated over the tree of life. Nucleic Acids Res. (2015) 43:D447–52. doi: 10.1093/nar/gku1003
15. Kuleshov MV, Jones MR, Rouillard AD, Fernandez NF, Duan Q, Wang Z, et al. Enrichr: a comprehensive gene set enrichment analysis web server 2016 update. Nucleic Acids Res. (2016) 44:W90–7. doi: 10.1093/nar/gkw377
16. Buniello A, MacArthur JAL, Cerezo M, Harris LW, Hayhurst J, Malangone C, et al. The NHGRI-EBI GWAS Catalog of published genome-wide association studies, targeted arrays and summary statistics 2019. Nucleic Acids Res. (2019) 47:D1005–12. doi: 10.1093/nar/gky1120
17. Groza T, Köhler S, Moldenhauer D, Vasilevsky N, Baynam G, Zemojtel T, et al. The human phenotype ontology: semantic unification of common and rare disease. Am J Hum Genet. (2015) 97:111–24. doi: 10.1016/j.ajhg.2015.05.020
18. Freund MK, Burch KS, Shi H, Mancuso N, Kichaev G, Garske KM, et al. Phenotype-specific enrichment of mendelian disorder genes near GWAS regions across 62 complex traits. Am J Hum Genet. (2018) 103:535–52. doi: 10.1016/j.ajhg.2018.08.017
19. Lanternier F, Mahdaviani SA, Barbati E, Chaussade H, Koumar Y, Levy R, et al. Inherited CARD9 deficiency in otherwise healthy children and adults with Candida species-induced meningoencephalitis, colitis, or both. J Allergy Clin Immunol. (2015) 135:1558–68.e2. doi: 10.1016/j.jaci.2014.12.1930
20. Westbury SK, Turro E, Greene D, Lentaigne C, Kelly AM, Bariana TK, et al. Human phenotype ontology annotation and cluster analysis to unravel genetic defects in 707 cases with unexplained bleeding and platelet disorders. Genome Med. (2015) 7:36. doi: 10.1186/s13073-015-0151-5
21. Aslam A, Misbah SA, Talbot K, Chapel H. Vitamin E deficiency induced neurological disease in common variable immunodeficiency: two cases and a review of the literature of vitamin E deficiency. Clin Immunol. (2004) 112:24–9. doi: 10.1016/j.clim.2004.02.001
22. Ziegner UH, Kobayashi RH, Cunningham-Rundles C, Español T, Fasth A, Huttenlocher A, et al. Progressive neurodegeneration in patients with primary immunodeficiency disease on IVIG treatment. Clin Immunol. (2002) 102:19–24. doi: 10.1006/clim.2001.5140
23. Plebani A, Soresina A, Rondelli R, Amato GM, Azzari C, Cardinale F, et al. Clinical, immunological, and molecular analysis in a large cohort of patients with X-linked agammaglobulinemia: an Italian multicenter study. Clin Immunol. (2002) 104:221–30. doi: 10.1006/clim.2002.5241
24. Maarschalk-Ellerbroek LJ, Hoepelman AI, van Montfrans JM, Ellerbroek PM. The spectrum of disease manifestations in patients with common variable immunodeficiency disorders and partial antibody deficiency in a university hospital. J Clin Immunol. (2012) 32:907–21. doi: 10.1007/s10875-012-9671-6
25. Chapel H, Prevot J, Gaspar HB, Español T, Bonilla FA, Solis L, et al. Primary immune deficiencies - principles of care. Front Immunol. (2014) 5:627. doi: 10.3389/fimmu.2014.00627
26. Costa-Carvalho BT, Grumach AS, Franco JL, Espinosa-Rosales FJ, Leiva LE, King A, et al. Attending to warning signs of primary immunodeficiency diseases across the range of clinical practice. J Clin Immunol. (2014) 34:10–22. doi: 10.1007/s10875-013-9954-6
27. Broadaway KA, Cutler DJ, Duncan R, Moore JL, Ware EB, Jhun MA, et al. A statistical approach for testing cross-phenotype effects of rare variants. Am J Hum Genet. (2016) 98:525–40. doi: 10.1016/j.ajhg.2016.01.017
28. Tyler AL, Crawford DC, Pendergrass SA. Detecting and characterizing pleiotropy: new methods for uncovering the connection between the complexity of genomic architecture and multiple phenotypes. Pac Symp Biocomput. (2014) 183–7. doi: 10.1142/9789814583220_0018
29. Wang Z, Liao BY, Zhang J. Genomic patterns of pleiotropy and the evolution of complexity. Proc Natl Acad Sci USA. (2010) 107:18034–9. doi: 10.1073/pnas.1004666107
30. Lage K, Karlberg EO, Størling ZM, Ólason PÍ, Pedersen AG, Rigina O, et al. A human phenome-interactome network of protein complexes implicated in genetic disorders. Nat Biotechnol. (2007) 25:309–16. doi: 10.1038/nbt1295
31. Vanunu O, Magger O, Ruppin E, Shlomi T, Sharan R. Associating genes and protein complexes with disease via network propagation. PLoS Comput Biol. (2010) 6:e1000641. doi: 10.1371/journal.pcbi.1000641
32. Tsai CT, Hsieh CS, Chang SN, Chuang EY, Juang JM, Lin LY, et al. Next-generation sequencing of nine atrial fibrillation candidate genes identified novel de novo mutations in patients with extreme trait of atrial fibrillation. J Med Genet. (2015) 52:28–36. doi: 10.1136/jmedgenet-2014-102618
33. Bomba L, Walter K, Soranzo N. The impact of rare and low-frequency genetic variants in common disease. Genome Biol. (2017) 18:77. doi: 10.1186/s13059-017-1212-4
34. Khera AV, Chaffin M, Aragam KG, Haas ME, Roselli C, Choi SH, et al. Genome-wide polygenic scores for common diseases identify individuals with risk equivalent to monogenic mutations. Nat Genet. (2018) 50:1219–24. doi: 10.1038/s41588-018-0183-z
Keywords: international union of immunological societies, inborn errors of immunity, OMIM, ORPHANET, human phenotype ontology, clinical signs and symptoms, pleiotropy, gene-disease association
Citation: Salnikova LE, Chernyshova EV, Anastasevich LA and Larin SS (2019) Gene- and Disease-Based Expansion of the Knowledge on Inborn Errors of Immunity. Front. Immunol. 10:2475. doi: 10.3389/fimmu.2019.02475
Received: 31 July 2019; Accepted: 03 October 2019;
Published: 21 October 2019.
Edited by:
Antonio Condino-Neto, University of São Paulo, BrazilReviewed by:
Amos Etzioni, University of Haifa, IsraelMichel J. Massaad, American University of Beirut Medical Center, Lebanon
Copyright © 2019 Salnikova, Chernyshova, Anastasevich and Larin. This is an open-access article distributed under the terms of the Creative Commons Attribution License (CC BY). The use, distribution or reproduction in other forums is permitted, provided the original author(s) and the copyright owner(s) are credited and that the original publication in this journal is cited, in accordance with accepted academic practice. No use, distribution or reproduction is permitted which does not comply with these terms.
*Correspondence: Lyubov E. Salnikova, c2Fsbmlrb3ZhbHl1Ym92QGdtYWlsLmNvbQ==