- 1Shenzhen Public Platform for Screening and Application of Marine Microbial Resources, Graduate School at Shenzhen, Tsinghua University, Shenzhen, China
- 2Department of Biology, Woods Hole Oceanographic Institution, Woods Hole, MA, United States
Interactions between microorganisms and algae during bloom events significantly impacts their physiology, alters ambient chemistry, and shapes ecosystem diversity. The potential role these interactions have in bloom development and decline are also of particular interest given the ecosystem impacts of algal blooms. We hypothesized that microbial community structure and succession is linked to specific bloom stages, and reflects complex interactions among taxa comprising the phycosphere environment. This investigation used pyrosequencing and correlation approaches to assess patterns and associations among bacteria, archaea, and microeukaryotes during a spring bloom of the dinoflagellate Alexandrium catenella. Within the bacterial community, Gammaproteobacteria and Bacteroidetes were predominant during the initial bloom stage, while Alphaproteobacteria, Cyanobacteria, and Actinobacteria were the most abundant taxa present during bloom onset and termination. In the archaea biosphere, methanogenic members were present during the early bloom period while the majority of species identified in the late bloom stage were ammonia-oxidizing archaea and Halobacteriales. Dinoflagellates were the major eukaryotic group present during most stages of the bloom, whereas a mixed assemblage comprising diatoms, green-algae, rotifera, and other microzooplankton were present during bloom termination. Temperature and salinity were key environmental factors associated with changes in bacterial and archaeal community structure, respectively, whereas inorganic nitrogen and inorganic phosphate were associated with eukaryotic variation. The relative contribution of environmental parameters measured during the bloom to variability among samples was 35.3%. Interaction analysis showed that Maxillopoda, Spirotrichea, Dinoflagellata, and Halobacteria were keystone taxa within the positive-correlation network, while Halobacteria, Dictyochophyceae, Mamiellophyceae, and Gammaproteobacteria were the main contributors to the negative-correlation network. The positive and negative relationships were the primary drivers of mutualist and competitive interactions that impacted algal bloom fate, respectively. Functional predictions showed that blooms enhance microbial carbohydrate and energy metabolism, and alter the sulfur cycle. Our results suggest that microbial community structure is strongly linked to bloom progression, although specific drivers of community interactions and responses are not well understood. The importance of considering biotic interactions (e.g., competition, symbiosis, and predation) when investigating the link between microbial ecological behavior and an algal bloom’s trajectory is also highlighted.
Introduction
Phytoplankton are a fundamental component of the marine ecosystem, playing multiple roles in matter cycling and the support of global biological and geochemical processes (Lindh et al., 2013). Under certain conditions, phytoplankton can be a double-edged sword, ecologically speaking. On the one hand, phytoplankton account for less than 1% of overall photosynthetic biomass on Earth, yet contribute at least half of the world’s net oxygen production (Field et al., 1998; Monier et al., 2015). On the other hand, some phytoplankton taxa form harmful algal blooms (HABs), which can adversely impact ecosystems and human health. For example, Alexandrium is a cosmopolitan dinoflagellate genus that proliferates annually worldwide, often forming dense blooms in near-shore waters. Blooms of certain toxin-producing species in these genus are responsible for widespread and significant impacts, including animal mortality events, and can contaminate shellfish with toxins that threaten human health (Bagatini et al., 2014). Estimates of the economic costs of these blooms exceed $20 million USD (Anderson et al., 2012), largely due to losses suffered by tourism and aquaculture industries.
The increase in the frequency and global distribution of HABs in recent decades has prompted research to identify the ecological and physiological factors that trigger the initiation and influence the magnitude of these events. Abiotic factors influencing HAB occurrence and dynamics include hydrodynamic processes, environmental conditions, and nutrient availability (Bouchouicha Smida et al., 2012; Lewitus et al., 2012), whereas biotic factors include grazing, pathogenicity, and parasitism (Paerl and Otten, 2013; Carnicer et al., 2015). Among the array of biotic drivers, microbial community composition (particularly bacteria) is increasingly cited as influencing HAB development (Teeling et al., 2012, 2016; Klindworth et al., 2014; Needham and Fuhrman, 2016). This is due in part to the role microbial communities play in mediating biogeochemical cycling, micro-food web structure, and production of essential elements that stimulate algal growth (Doucette, 1995; Ferrier et al., 2002; Segev et al., 2016), as well as absorbing essential elements (Adachi et al., 2003), exhibiting algicidal activity (Demuez et al., 2015; Bloh et al., 2016), inhibiting sexual reproduction (Sanders, 2014), and regulating algae-bacteria signaling (such as quorum sensing) (Zhou et al., 2016b). Viruses also play a critical role in the regulation of phytoplankton biomass, including host infection and lysis (Mizumoto et al., 2008; Ory et al., 2010). Nagasaki (2008) summarized the ecological relationships between dinoflagellates and diatoms, and their viruses, and concluded that viral infection is a significant factor driving population dynamics of algae.
Bacterial community structure during phytoplankton blooms is complex and changes during bloom progression depending on the algal species, physiological status, environmental conditions, and bloom stage (Zheng, 2011). Several studies have reported changes in bacterial community structure during natural and semi-natural (mesocosm) phytoplankton blooms (Riemann et al., 2000; Jones et al., 2010; Theroux et al., 2012), and certain dominant bacteria groups (e.g., Roseobacter and Flavobacterium) have been associated with blooms (Buchan et al., 2014). The structure and metabolic properties of these communities influence their ecological functions, which can include nutrient provision, release of organic compounds, and even as a competitor with algae for a particular ecological niche (Amin et al., 2015). Together, these behaviors create a regulatory network that operates throughout bloom formation, duration, and collapse (Tan et al., 2015; Needham and Fuhrman, 2016).
Previous studies have largely focused on bacteria taxa, and to our knowledge none have examined the wider spectrum of the microbial biosphere (i.e., archaea and microeukaryotes) over the complete bloom cycle, i.e., from bloom initiation through termination (Kodama et al., 2006; Ramanan et al., 2016). In addition, despite a relatively mature understanding of microbial dynamics during HAB events, identifying potential microbial relationships remains a significant challenge (Fuhrman et al., 2015). Only a few studies have examined network interactions during algal blooms with respect to inter- and intra-species patterns (Yang et al., 2017), thus limiting our current understanding of the complex interactions between microbes and algae. To contribute herefore, it is necessary to study bacterioplankton dynamics and their relationships (positive or negative) during phytoplankton blooms to further our understanding of algal-bacteria interactions.
This investigation used high throughput sequencing and association networking methods to analyze prokaryotic and eukaryotic microbial community dynamics during a natural dinoflagellate bloom to better characterize patterns of interaction and related ecological behavior during HAB events. We hypothesized that microbial community structure and succession is linked to specific bloom stages, thus driving complex interactions among taxa comprising the phycosphere environment. The specific aims of this study were to explore the population dynamics of microorganisms during an algal bloom, and to identify and characterize correlations among microbial taxa using network analysis. The ultimate goal of this study was to advance our current understanding of community structure and associations among microbial populations during algal blooms, and provide insights into the mechanisms underlying these interactions and dynamics.
Materials and Methods
Study Site, Sampling, and Environmental Parameters
Field studies were carried out in Salt Pond in the Nauset Marsh System on Cape Cod (MA, United States). Salt Pond is a small kettle pond (∼82,200 m2 in area) located in Eastham, MA (41°32′35 N, 70°37′40 W). The pond is connected to the Nauset Estuary by a shallow and narrow tidal channel approximately 1–3 m in depth, which permits tidal exchange with the wider marsh system (Crespo et al., 2011; Richlen et al., 2012). Average and maximum depths in the pond are ∼3.4 and 9 m, respectively (Crespo et al., 2011). Freshwater enters Salt Pond solely through groundwater or precipitation, as there are no stream or river inputs. The flushing rate measured via dye tracers is about 0.4–0.7 day-1 (Anderson and Stolzenbach, 1985) and groundwater discharge to the pond has been estimated using radioisotope data (radon) and salinity at ∼3200–4500 m3 day-1 (Crusius et al., 2005). Blooms of the dinoflagellate Alexandrium catenella recur annually during the spring, and originate from the germination of resting cysts found within the pond (Crespo et al., 2011; Ralston et al., 2014). The physical features of this system provide a natural mesocosm for the study of algal blooms, thus providing an ideal study site to track microbial dynamics over the course of a bloom cycle.
Sampling was carried out in Salt Pond weekly from 19 March 2014 until 12 June 2014 (nine sampling time points; named W1 to W9; Figure 1). Water samples were collected in triplicate (2 L each) using a Niskin bottle from depths of about 0.5–1.0 m. Samples were first pre-filtered using a 300-mesh sieve, and were then collected on isopore filters with a diameter of 47 mm and pore size of 0.22 μm (Millipore, Burlington, MA, United States). Filters were stored at -80°C until analysis. A 50 mL fraction of each sample was preserved in 1% glutaraldehyde for enumerating total bacterial abundance. In addition, 500 mL of each sample was preserved with 1% Lugol’s iodine for phytoplankton identification and enumeration. This latter sample was further concentrated via settling and siphoning to produce a 25 mL concentrate. Algae were observed and counted under an optical microscope (×100 magnification).
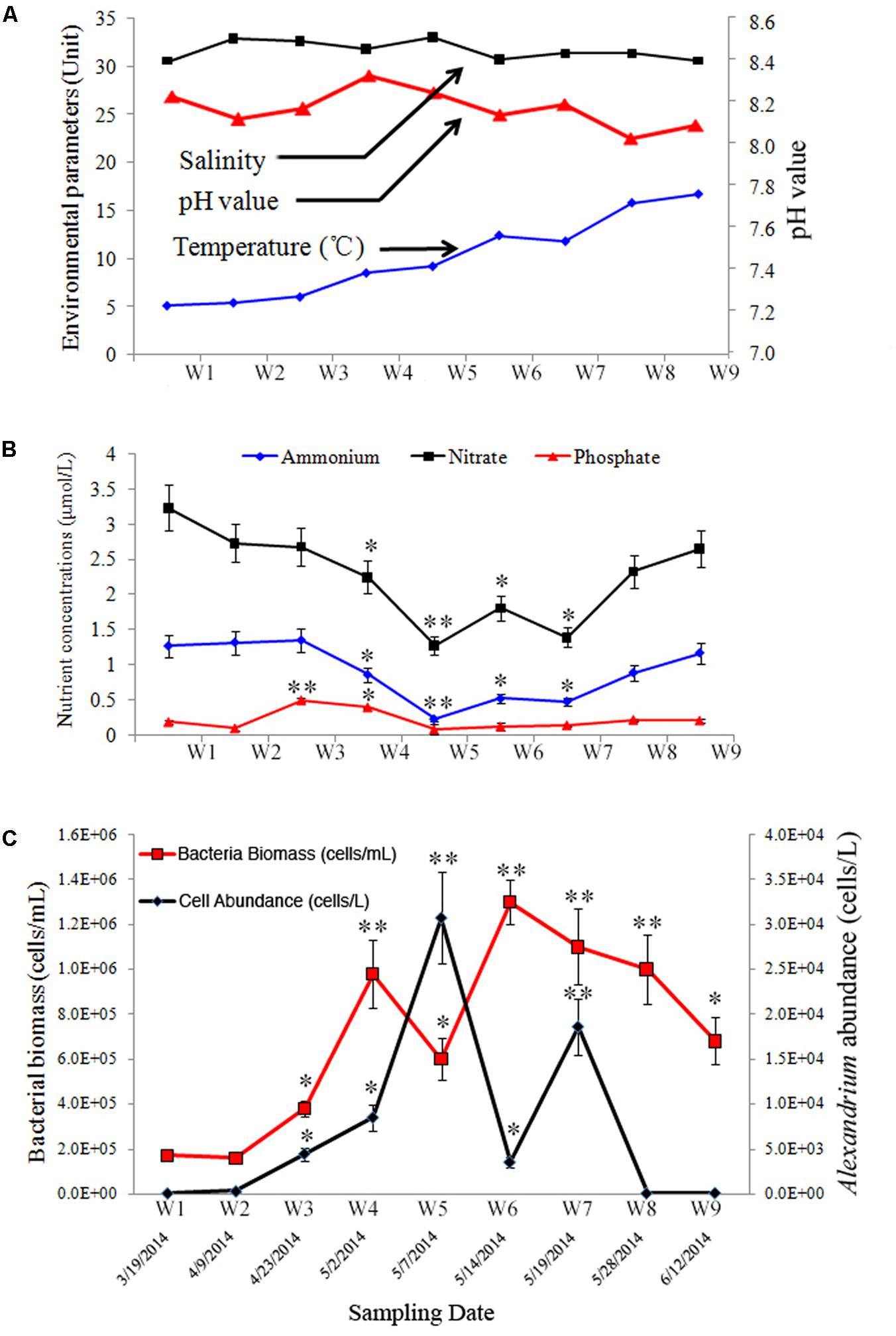
FIGURE 1. Environmental and biological parameters during a bloom of Alexandrium catenella in Salt Pond, Nauset Marsh, MA, United States. (A) temperature, salinity, and pH; (B) nutrients concentrations (nitrate [NO3-], ammonium [NH4+], and phosphate [PO43-]); (C) abundances of A. catenella (black line) and total bacterioplankton (red line) in water samples collected over the course of the A. catenella bloom. Data points represent the mean + SD of triplicate measurements. The single asterisk (∗) and double asterisk (∗∗) indicated the significant difference compared with the first time-point (W1) at P < 0.05 and P < 0.01 levels, respectively.
During each sampling event, physicochemical parameters of the seawater were recorded. In situ measurements of salinity, pH, and temperature (°C) were collected using an YSI Professional Pro Meter (YSI Inc., Yellow Springs, OH, United States). The detection range of salinity and precision were 1–60 and 0.1, respectively. Ammonium (NH4+), nitrate (NO3-), and phosphate (PO43-) were measured according to methods outlined below. NH4+ was measured according to the indophenol blue method using a spectrophotometer (DR/2800, Hach) (Hashihama et al., 2015). NO3- analyses followed protocols from the Center for Microbial Oceanography at the University of Hawaii1. Phosphorus was measured by spectrophotometry following the formation of phosphomolybdic acid according to Murphy and Riley (1962). Nutrient analyses were performed on a Seal Analytical Continuous-Flow AutoAnalyzer 3 (United Kingdom). The detection limits of the aforementioned nutrient parameters were 0.05, 0.05, and 0.01 μmol/L, respectively. Approximate inorganic nitrogen/inorganic phosphate (IN/IP) values were measured according to methods of Murphy and Riley (1962) and Greenberg et al. (1992). Standards for NO3-, NO2-, and PO43- were obtained from Merck KGaA Biochemical Co., Ltd (Germany), and the purity was ≥99%.
Bacterial Abundance
Seawater samples preserved in glutaraldehyde were stained with 4′-6′-diamidino-2-phenylindole (DAPI, Sigma, final concentration 1 μg/mL) for 15 min (Poter and Feig, 1980) and filtered onto 0.22 μm pore size polycarbonate filters (Millipore, Burlington, MA, United States). Bacterial cells were enumerated using an epifluorescent microscope (×1000 magnification). At least 10 fields of view and >50 cells were counted in each sample.
DNA Extraction and PCR Amplification
DNA was extracted using a Fast DNA Spin Kit (Bio101, QbioGene, United States) according to the manufacturer’s instructions, and eluted in 50 μL TE buffer. DNA quality was estimated by the ratio of OD260/OD280 to verify that the value was >1.8 (NanoDropTM 2000), and stored at -20°C until further use.
Prokaryote 16S rRNA genes (V4–V5 hypervariable region) were amplified as described by Fierer et al. (2008) using the primers F515 (5′-GTGCCAGCMGCCGCGG-3′) and R907 (5′-CCGTCAATTCMTTTRAGTTT-3′). These primers were demonstrated in silico to target nearly all archaea and bacteria (Bates et al., 2011), and provide sufficient resolution for the taxonomic classification of microbial sequences (Liu et al., 2007). An 8-bp barcode was added to the forward primer, and samples were amplified in triplicate as described by Fierer et al. (2008). Replicate PCRs for each sample were subsequently pooled and purified using a QIAquick Gel Extraction Kit (Qiagen, Hilden, Germany).
Amplification of eukaryotes was performed using primers 547F (5′-CCAGCASCYGCGGTAATTCC-3′) and 952R (5′-ACTTTCGTTCTTGATYRA-3′), which target the V4 region of the 18S RNA gene. Amplifications were carried out in triplicate 50 μL PCRs comprising 0.5 μM of each primer, 1× GoTaq Flexi Reaction Buffer (Promega, Madison, WI, United States), 2.5 mM MgCl2, 200 mM dNTPs, 0.8 μg/mL BSA, 2.5 U of GoTaq Flexi DNA Polymerase (Promega), and 10 ng of total DNA. PCR cycling conditions were as follows: 2 min at 94°C; 10 cycles 94°C for 30 s, 65°C for 30 s (decreasing 1°C per cycle for 30 s, and 2°C for 30 s); 18 cycles of 94°C for 30 s, 55°C for 30 s, and 72°C for 30 s; and a final extension of 72°C for 10 min.
The PCR products were pooled, cleaned, and concentrated using a QIAquick PCR Purification Kit (Qiagen). A single composite sample for pyrosequencing was prepared by combining approximately equimolar amounts of PCR products from each sample. Sequencing was carried out by Biolinker Biotech. Co. Ltd. (Shanghai, China).
Processing of 16S/18S Sequences
Raw sequences were processed and checked using Mothur and QIIMe software packages (Schloss et al., 2009). Sequencing data were denoised using the commands “shhh.flows” (translation of PyroNoise algorithm; Quince et al., 2009) and “pre.cluster” (Huse et al., 2010) on the Mothur platform. Chimeric sequences were identified and removed using UCHIME with the de novo method (Edgar et al., 2011). The raw sequence reads were trimmed and filtered according to a previous method (Huse et al., 2007). After low-quality reads were removed, representative sequences were annotated using an alignment tool (BLAST) against the Ribosomal Database Project and the Silva database augmented with 16S and 18S rRNA sequences from major marine taxa. We assigned operational taxonomic units (OTUs) at a 3% gene identity threshold using the UPARSE (version 7.1)2 pipeline (Edgar, 2013). The sequence data reported here have been deposited in the NCBI GenBank database (accession number SRP114998).
Network Analysis
Interaction networks and co-occurrence patterns were examined according to Faust et al. (2012) and Yang et al. (2016). OTUs with average relative abundance of less than 0.1% were removed the microbial sequences, and a Spearman rank correlation matrix was created using the remaining data. Co-occurrence patterns were determined to be robust if the Spearman’s rank correlation coefficient (rho) was >0.6 and P < 0.05. These two filtering steps were applied to remove poorly represented OTUs and reduce network complexity, which assisted in determining the core microbial community in samples. An affiliation network was generated using the R package igraph, version 1.0.1. False positive correlations were filtered according to methods by Lima-Mendez et al. (2015) and Yambartsev et al. (2016). A network was constructed with edges consisting of correlations within an arbitrary P-value threshold. Unexpected links (a score below the thresholds) were identified, counted, and removed from the network. The false positives were discarded using the dirmult package in R. An affiliation network is defined as a network in which the members are affiliated with one another based on co-membership of a group or co-participation in some type of event. Direct connections among the different genera were extracted from the two-mode affiliation network using igraph’s bipartite.projection function. The nodes in the reconstructed network represented variable taxa, and the edges connecting the nodes represented correlations between genera. The topographic features of the network, including centrality and edge weights, were also analyzed using functions available in the igraph package. In addition, subsets of representative specialist genera from the samples were selected to help identify potential keystone taxa and their interactions within the network. Sub-networks were then generated from the meta-community networks using subgraph functions in the igraph package. Information on the target network was further organized in matrices and visualized in chord diagrams using the R package circlize, version 0.3.5.3
Statistical Analyses
The α- and β-diversity indices for each sample were calculated using Mothur (Schloss et al., 2009). Statistically significant differences in taxa abundance were identified using Welch’s t-test in the STAMP program (Parks et al., 2014). The structure of the bacterial/archaeal/microeukaryotal community in each sample was compared using the 2STAGE analysis in the PRIMER (v6, PRIMER-E Ltd, Lutton, United Kingdom) (Clarke and Gorley, 2006). A heatmap of microbial communities was created using the PHYLOTEMP tool4 developed by Polson (2007), whereby relative abundance data are clustered based on the Bray–Curtis similarity algorithm. Principal component analysis (PCA) was used to display and compare microbial community composition among different samples, and canonical correspondence analysis (CCA) was used to link variations in microbial communities to environmental properties. Both analyses were carried out using Canoco 5 software. A variation partitioning analysis (VPA) was conducted to examine the contribution of environmental factors in influencing microbial community structure as determined by CCA analysis. Significant differences were defined as a P < 0.05 or P < 0.01. Functional analysis was performed in which bacterial gene functions were predicted from 16S rRNA gene-based microbial compositions using the PICRUSt algorithm to make inferences from KEGG annotated databases (Langille et al., 2013). The predicted metabolic functions of microbial communities at different algal bloom stages were identified using the PICRUSt (v1.0.0) protocol. For the environmental parameters, differences in various data were determined using analysis of variance (ANOVA) at the P < 0.05 significance level. Data analyses were performed using the SPSS software package 13.0 (Armonk, NY, United States).
Results
Environmental Parameters and Bloom Characteristics
Five primary stages were documented over the course of the bloom: pre- [W1], onset [W2–W3], exponential growth [W4], peak [W5], and decline/termination stages [W6–W9]. Over the duration of the sampling period, temperature, salinity, and pH values ranged from 5.2 to 16.8°C, 29.8 to 33.1, and 8.06 to 8.36, respectively (Figure 1A). Nutrient concentrations at each time point are shown in Figure 1B. NH4++ NO3- and PO43- ranged from 1.52 to 4.53 μmol/L and 0.12 to 0.51 μmol/L, respectively. Highest and lowest concentrations of NH4+ and NO3- were detected during bloom onset and peak bloom stages, respectively (P < 0.01). A different trend was observed in PO43- concentrations in which PO43- was higher earlier in the bloom, and lower concentrations occurred during the peak- and decline bloom stages (P < 0.01). The approximate IN/IP ratio in surface waters ranged from 12.67 to 37.75.
Alexandrium catenella cell densities ranged from 1.5 × 102 to 3.1 × 104 cells/L over the sampling period (Figure 1C). The cellular morphology and size of A. catenella are shown in Supplementary Figure S1. Total abundance of bacteria ranged from 1.7 × 105 to 1.2 × 106 cells/mL, and the highest value appeared immediately after the bloom’s peak (Figure 1C) (P < 0.01). Micrographs of DAPI-stained filter samples are shown in Supplementary Figure S2. Total bacterial abundance was correlated with the A. catenella bloom, and a hysteresis phenomenon was observed in the population dynamics of the two groups.
Biodiversity
Using a 97% similarity cut-off, a total of 4,859 bacterial OTUs, 517 archaea OTUs, and 3452 eukaryotes OTUs were obtained from the field samples. To investigate changes in biodiversity, stage variations in Chao1, Shannon, and Simpson indices were calculated for the bacterioplankton communities (Figure 2A). The Chao1 index calculated for the bacterial group remained relatively constant throughout the A. catenella bloom, and only increased at the starting point of bloom termination. The Shannon index was relatively constant throughout the bloom; Simpson indices exhibited a similar trend, but decreased slightly over the course of the bloom.
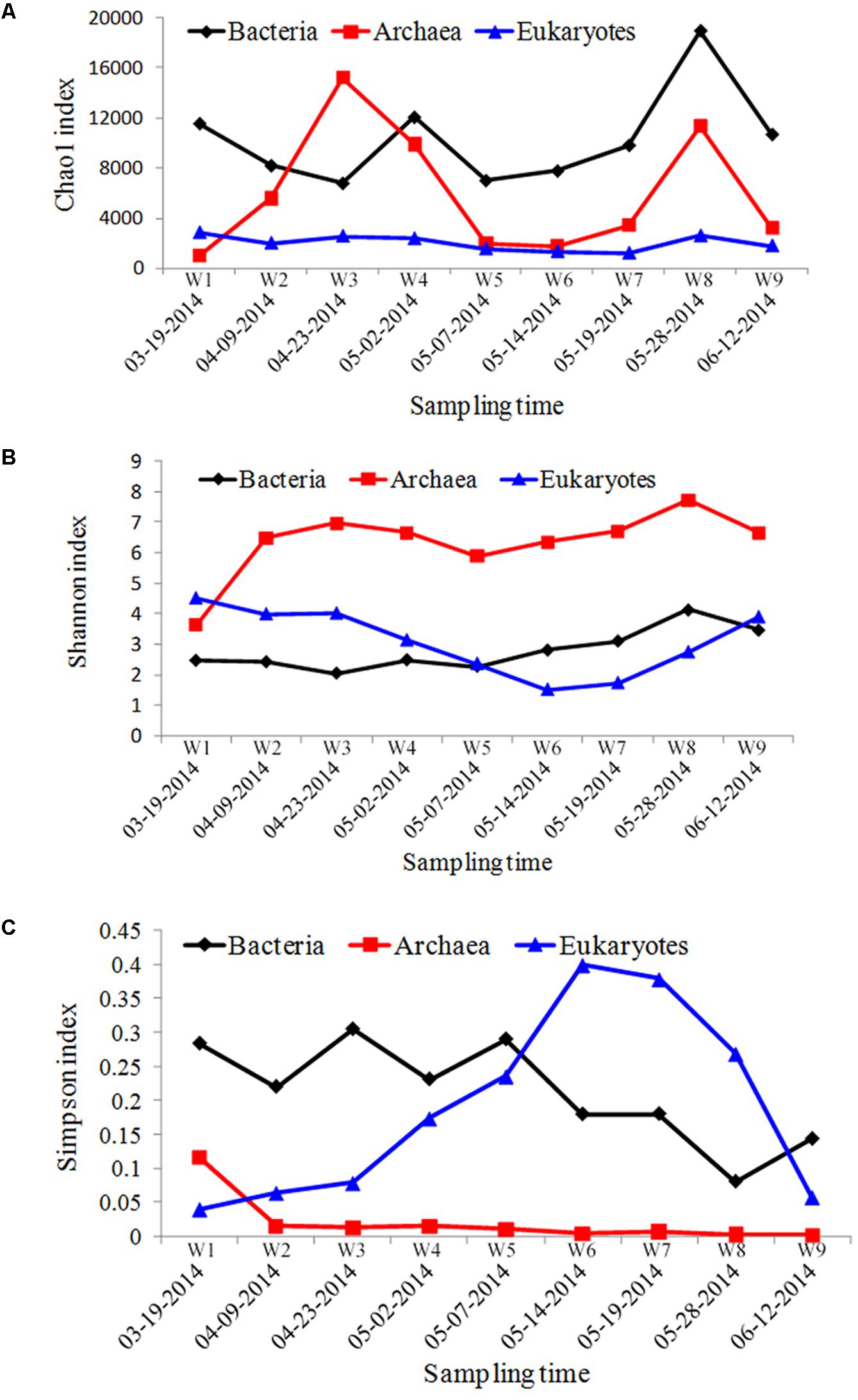
FIGURE 2. The α-diversity indices of microbial communities sampled from Salt Pond. (A) Chao1 index, (B) Shannon index, and (C) Simpson index.
In the archaeal biosphere, fluctuations were observed throughout the entire sampling period. The highest Chao1 diversity index value appeared during bloom onset, and the lowest value was observed at the bloom’s peak (Figure 2B). The Shannon index values increased during the bloom, starting at the second time point (W2, 04-09-2014), whereas Simpson index values decreased from this time point.
Chao1 indices calculated for eukaryotic communities were significantly lower compared with those calculated for bacterial/archaeal communities, and were relatively stable throughout the bloom. Fluctuations were observed, however, in the Shannon and Simpson indices calculated for the different sampling periods. The most obvious change appeared at beginning of the bloom’s decline (W6, 05-14-2014), in which the lowest Shannon and highest Simpson diversity indices were observed (Figure 2C).
Patterns of microbial community beta diversity (among-sample differences in OTU composition) are shown in Figure 3, in which the color intensity reflects similarity in species composition. In the bacteria, the general dissimilarity trend among sampling periods was group I (W1–W3) > group II (W4 and W8) > group III (W5–W7 and W9). The biggest difference in community composition across samples was found for W3, which was collected during bloom development (Figure 3A). Archaea samples clustered into three groups that largely corresponded to the time period sampled (e.g., early, mid, late bloom), in spite of some overlap. The biggest difference among sampling periods was found at the decline stage, which had a comparatively higher beta-diversity indices (W7 and W9) (group I); while the remaining samples exhibited similar community composition (group II) (Figure 3B). High similarity in eukaryotic species composition was found in a grouping comprised of samples from the early and late bloom stages (W1–W3 and W6–W9), whereas some differences appeared in samples collected during exponential growth (W4–W5). In this group, the highest beta-diversity index value was found at the peak stage of the bloom (Figure 3C).
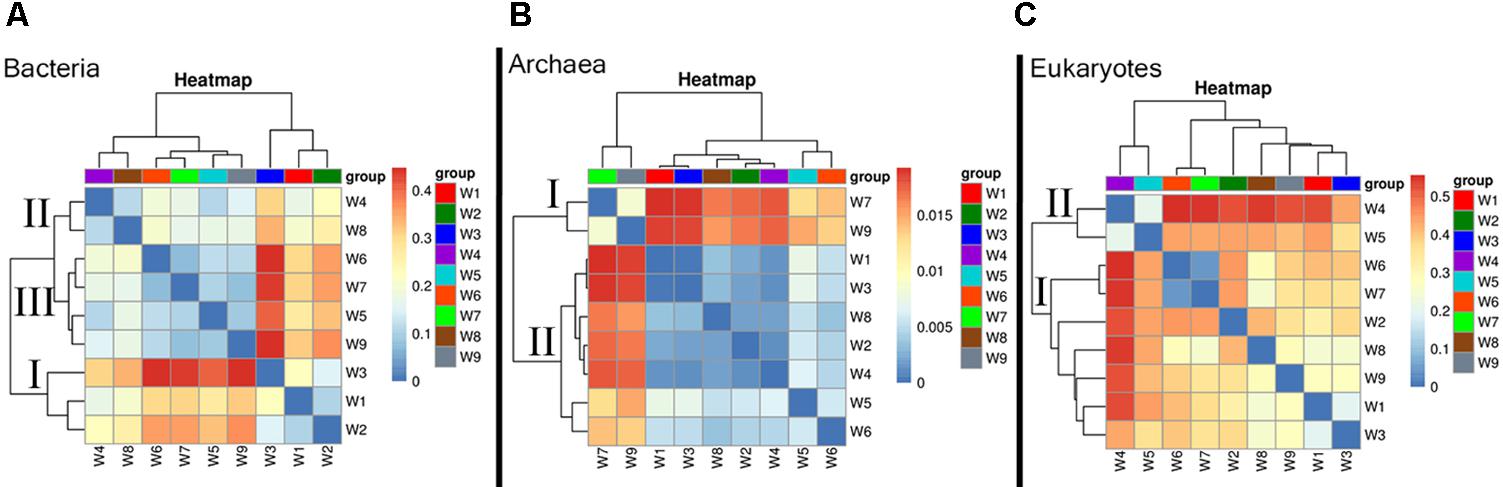
FIGURE 3. Heatmap illustrating Bray–Curtis similarities (β-diversity) based on taxonomic assignments (genus level). (A) Bacteria, (B) Archaea, and (C) Eukaryotes.
Microbial Community Dynamics
Bacterial community structure (phylum level) during the bloom is shown in Supplementary Figure S3A. Bacteroidetes and Proteobacteria were the two major dominant phyla during all bloom stages. The mean proportion of Bacteroidetes during the bloom’s peak (65.3%) was higher than during pre-bloom (30.6–51.5%) and post-bloom stages (21.5–53.1%). Proteobacteria exhibited an inverse pattern compared with Bacteroidetes; specifically, the Gammaproteobacteria dominated during the pre-bloom stage, while Alphaproteobacteria were predominant during the peak and decline stages (Supplementary Figure S3B). Betaproteobacteria and Deltaproteobacteria appeared during the post-bloom stage, and the proportional abundance of these groups was 5.1 and 2.8%, respectively. As the bloom progressed, significant changes in the bacterial community were observed. The Cyanobacteria cluster significantly increased (30%) during the onset of bloom decline (W6) and gradually decreased thereafter. Similarly, the Actinobacteria group gradually increased over time, and the maximum proportional abundance was observed during bloom decline (about 4.2–10.7%). Low proportions of Spirochaetae, Candidate division WS3, and Acidobacteria occupied <1% at different bloom phases (Supplementary Figure S3A).
The dominant bacterial clades (top 10, order level) in samples were Flavobacteriales, Alteromonadales, Rhodobacterales, Oceanospirillales, Thiotrichales, Sphingobacteriales, Campylobacterales, Desulfobacterales, Clostridiales, and Cytophagales, which contributed >98% of the total quality reads (Figure 4). Among them, some taxa displayed obvious variations during algal bloom development. Flavobacteriales gradually increased during the bloom’s onset, reached maximum proportional abundance during the peak stage, and then declined along with the bloom; interestingly, abundance subsequently increased concurrent with the bloom’s termination. Similar to Flavobacteriales, Rhodobacterales increased significantly during the early bloom stages, reached highest proportional abundance when the bloom began to decline (W7), and decreased subsequently. Alteromonadales was the third most proportionally abundant order, and showed an overall decline as the bloom progressed. Thiotrichales and Oceanospirillales exhibited gradually increasing and decreasing patterns with bloom progression, respectively.
Compared with bacteria, relatively fewer archaea were detected. The ratio of archaea was about 6–10% of the total prokaryotic community. The sequences obtained in this study primarily fall within the Marine Groups I (Thaumarchaeota) and II (Euryarchaeota) (Figure 5A). Among the most abundant OTUs (genus level), >50% belonged to methanogenic species (Methanomicrobiaceae, Methanocorpusculaceae, Methanoregullaceae, and Methanocellaceae); 11–21% belonged to ammonia-oxidizing archaea (AOA) (Nitrosocaldaceae and Nitrosocaldaceae); 5–30% belonged to Halobacteriaceae; 3–10% belonged to Thermoplasmatales; and 2–9% belonged to other species (Figure 5B). The Methanomicrobiales were dominant during the first sampling period, and their proportional abundance increased significantly (to 60%) from bloom onset to exponential growth stages. Halobacteriales and Thermoplasmatales were present during bloom decline. The proportional abundance of these taxa increased during the late bloom stage and comprised 35–40% of the total archaeal community (Figure 5A).
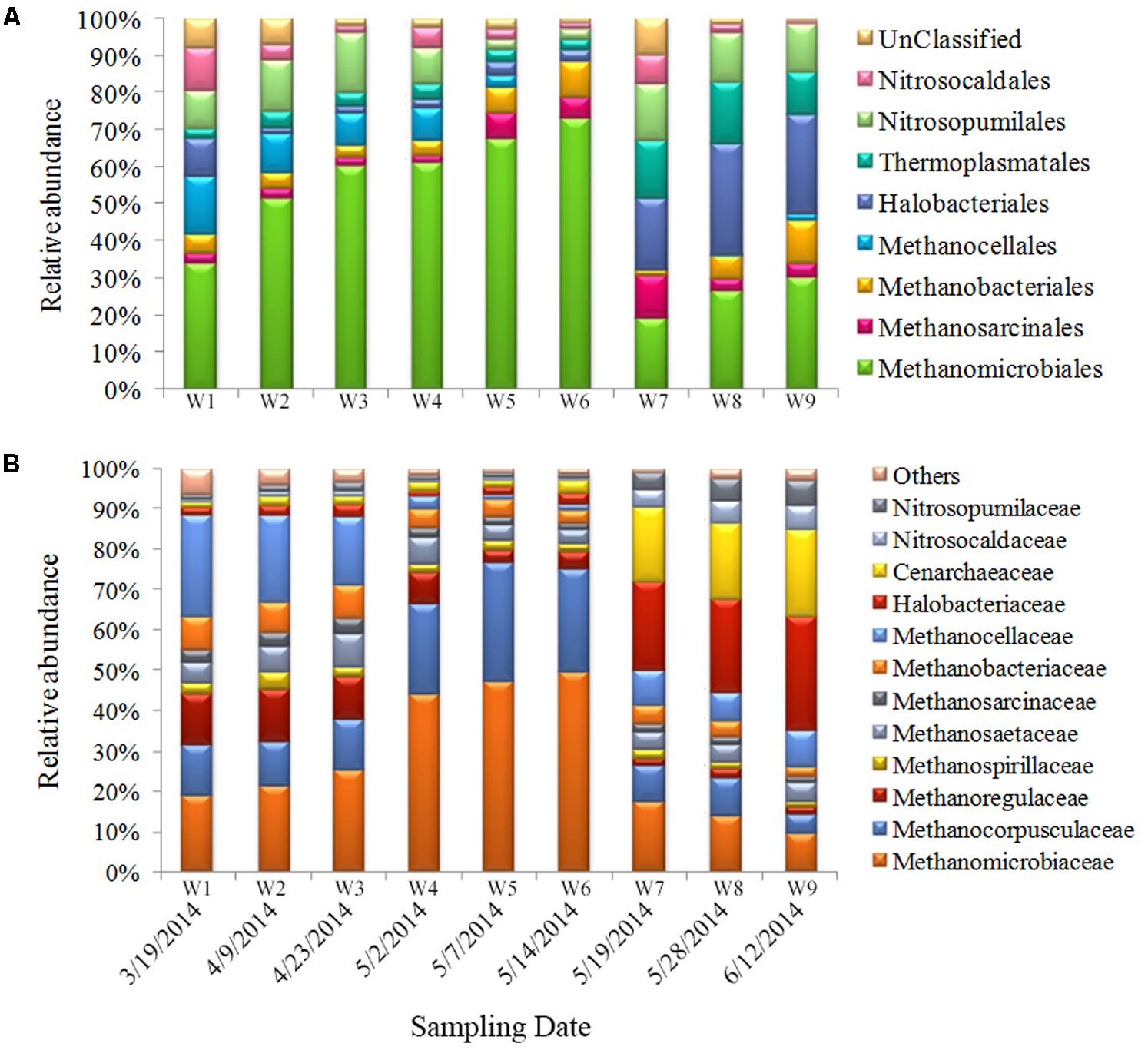
FIGURE 5. Archaeal community composition in samples. Relative abundances of the dominant groups at the order level (A) and family level (B).
Operational taxonomic units profiles of eukaryotic community structure during algal blooms in Salt Pond are shown in Figure 6. Dinophyta was clearly the major dominant phyla throughout the sampling period. During the pre-bloom stage, Chlorophyta, Diatomea, and Arthropoda species comprised the majority of the eukaryotic community. Over time, dinoflagellates (especially Alexandrium sp. and Heterocapsa sp.) significantly increased and reached peak abundance (>90%) at the height of the bloom (W5, 5/07/2014). During the later bloom stages (W7–W9), dinoflagellates were gradually replaced by other algal taxa, including Chlorophyta (mainly Tetraselmis sp. and Micromonas sp.), Diatomea (Thalassiosira sp. and Skeletonema sp.), and other low-abundance algae (Prymnesiophyceae, Phaeophyceae, and Streptophyta). In addition to the phytoplankton, zooplankton also exhibited distinct dynamics during the bloom. During the pre-bloom stage, Arthropoda, Apicomplexa, and some taxa (Nematoda, Annelida, Platyhelminthes, and Ascomycota) co-occurred. During the bloom’s peak stage, their abundances were significantly decreased and most of 18S OTUs were occupied by Alexandrium sp. When the algal bloom entered the termination stages, eukaryotic diversity gradually increased, and multiple organisms (algae, ciliate and fungi) co-occurred during this bloom phase (Figure 6).
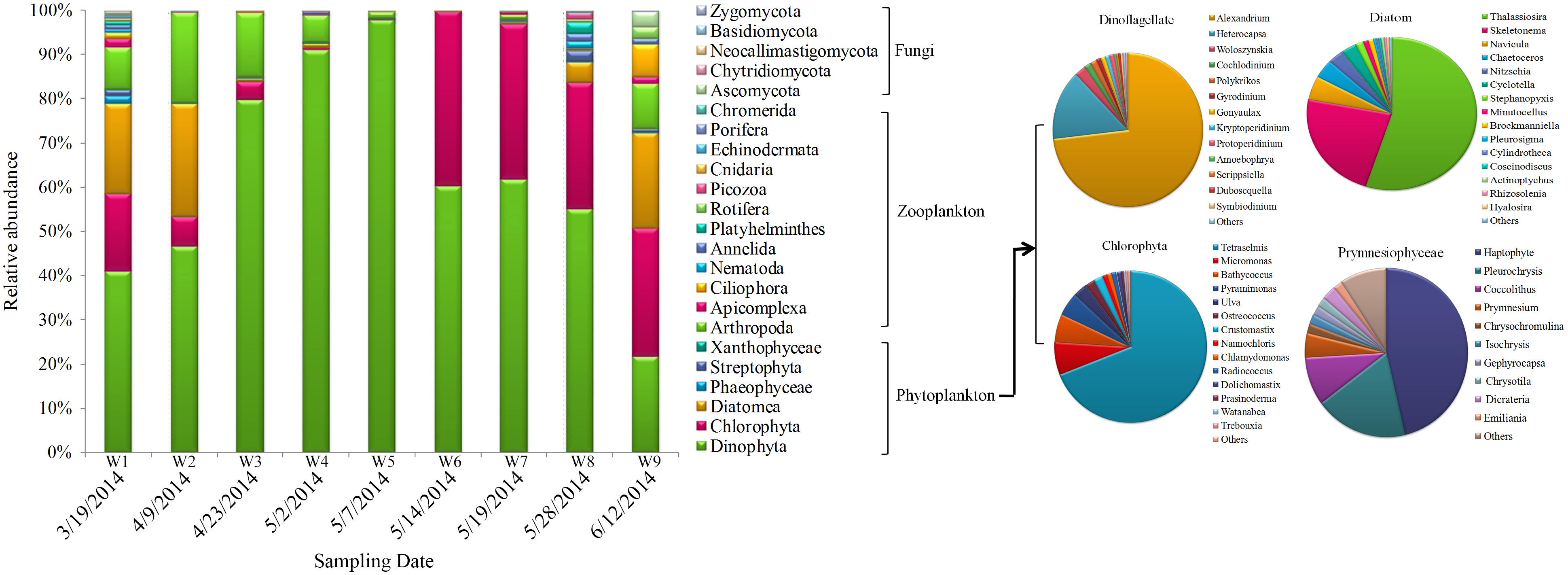
FIGURE 6. Eukaryotic community composition in samples. Relative abundances of the dominant groups at the phylum level. The right panel shows the composition of primary phytoplankton groups, including Dinoflagellata, Chlorophyta, diatoms, and Prymnesiophyceae.
Cluster Analysis
To determine whether the apparent differences in microbial community composition among samples significantly correlated with the algal bloom stage, cluster analyses were carried out. As shown in Figure 7A, the bacterial samples were divided into four clusters according to the different bloom stages (pre- [W1], onset and exponential growth [W2–W4], peak and initial decline [W5–W7], and termination stages [W8 and W9]).
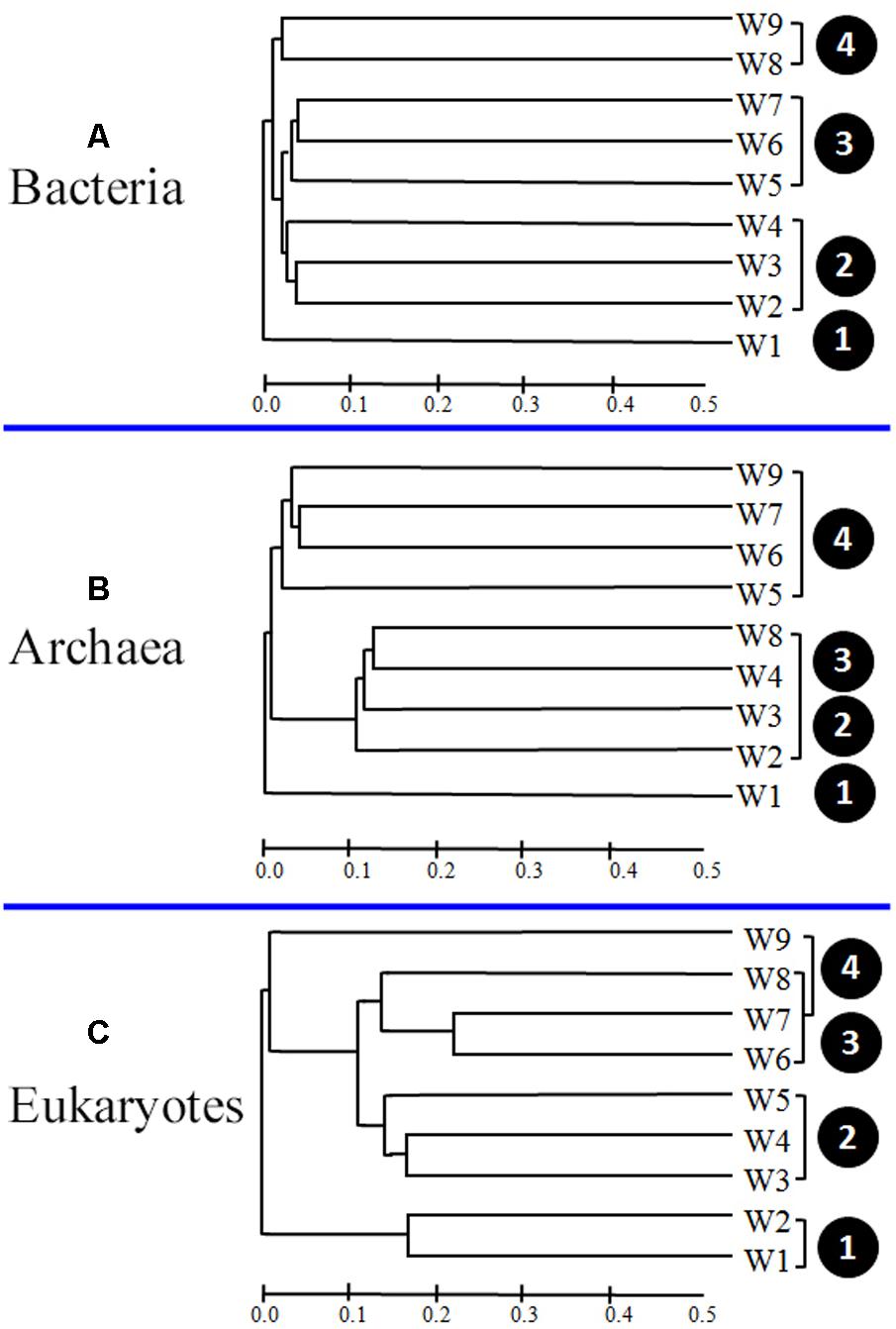
FIGURE 7. Bray–Curtis dissimilarity-based dendrogram at the operational taxonomic unit (OTU) level illustrating groups (A, Bacteria; B, Archaea; and C, Eukaryotes) in samples.
Differences in archaeal community composition among bloom stages were also depicted by assemblage analysis (Figure 7B). Samples from the pre-bloom stage (W1) were clustered together and separated from samples collected during bloom onset (W2–W4). Interestingly, a few samples (W8 and W9) exhibited some similarities with the samples collected during exponential growth and decline bloom stages (W5–W7).
Analysis of 18S OTU profiles also revealed obvious temporal structuring. W1 and W2 formed the first group (pre-bloom and initiation of bloom onset), W3–W5 formed the second group (onset and exponential growth stages), and third group comprised the remaining samples (W6–W9), collected during the bloom decline stages. The distribution showed a distinct temporal progression, with overlap at some phases (Figure 7C).
Correlation of Microbial Community Structure With Environmental Factors
Correspondence canonical analysis (CCA) was used to determine whether correlations in microbial structure were associated with environmental parameters (e.g., temperature, nutrients). Among the chemical factors, PO43- and NO3- contributed most to the variance in bacterial communities (Figure 8A). In the archaea, NH4+ and PO43- were the controlling factors associated with population variation (Figure 8B), whereas the strongest determinant of community structure in microeukaryotes was the IN/IP ratio (Figure 8C). Among the physical parameters, temperature and salinity were related to the composition of bacteria and archaea, respectively. There were no obvious negative or positive correlations between population dynamics and pH value. Additional information about the relationships between genera and environmental parameters as determined by correlation analysis (Pearson correlation coefficient) is shown in Supplementary Figures S4–S6.
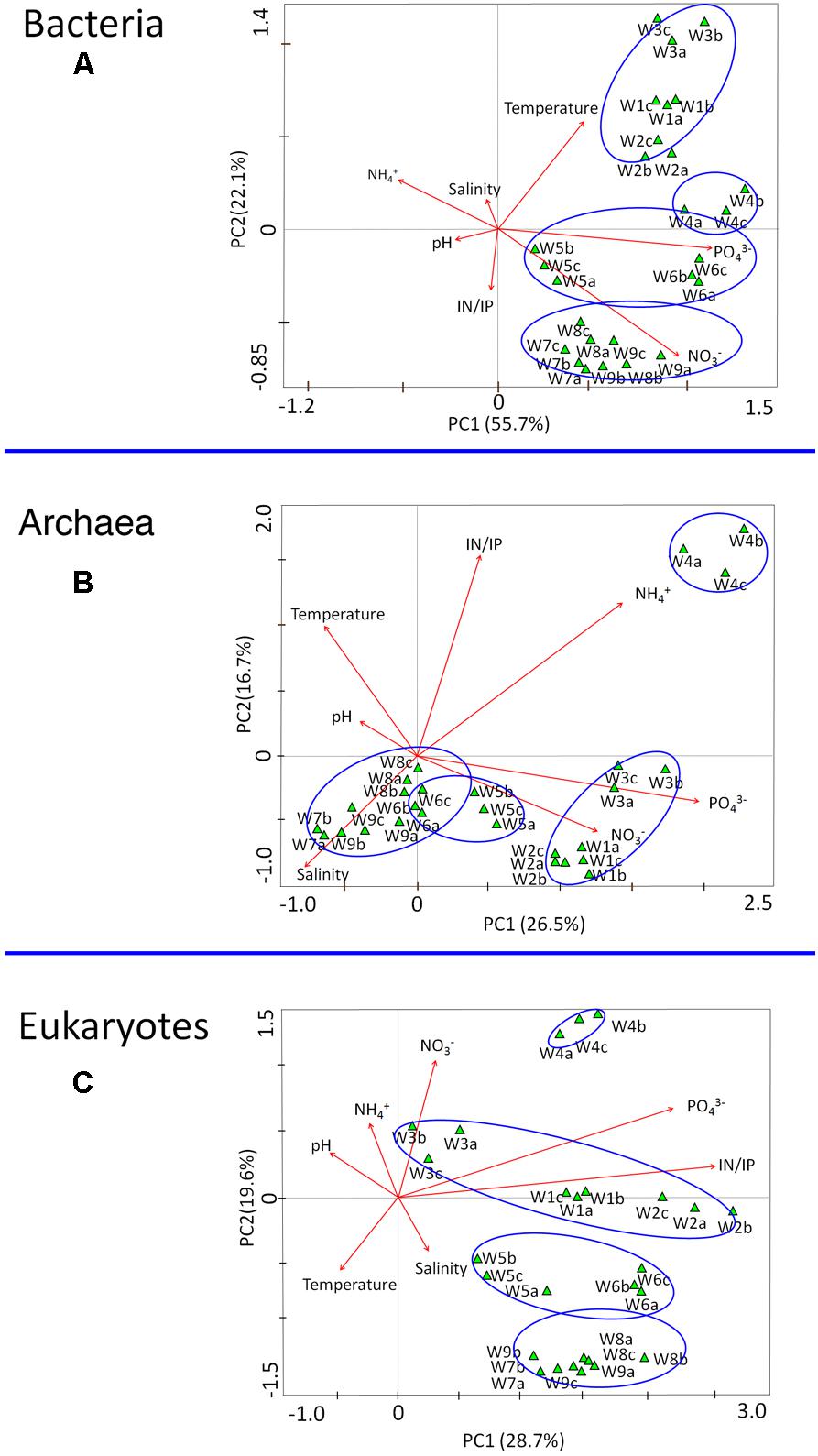
FIGURE 8. Correspondence canonical analysis (CCA) based on microbial communities (OTUs) and environmental parameters sampled during the bloom. (A) Bacteria, (B) Archaea, and (C) Eukaryotes. W1–W9 refer to the nine different collection time points.
A VPA was carried out to partition the contributions of environmental parameters. Results of this analysis showed that temperature (T), nutrients (N), and “salinity + pH” (SP) explained 35.3% of microbial community variation in the OTU data (Figure 9), indicating that they were significant factors in determining microbial composition. Independently, T, N, and SP were able to explain 13.9% (P = 0.01), 12.2% (P = 0.05), and 3.3% (P = 0.05) of the total variation observed, respectively. Interactions between T and N, T and SP, and N and SP explained 2.9, 1.8, and 1.2% of variation, respectively. About 64.7% of the microbial community variation in OTU data was not explained by these environmental parameters, indicating that other biotic and/or abiotic factors contribute to these processes.
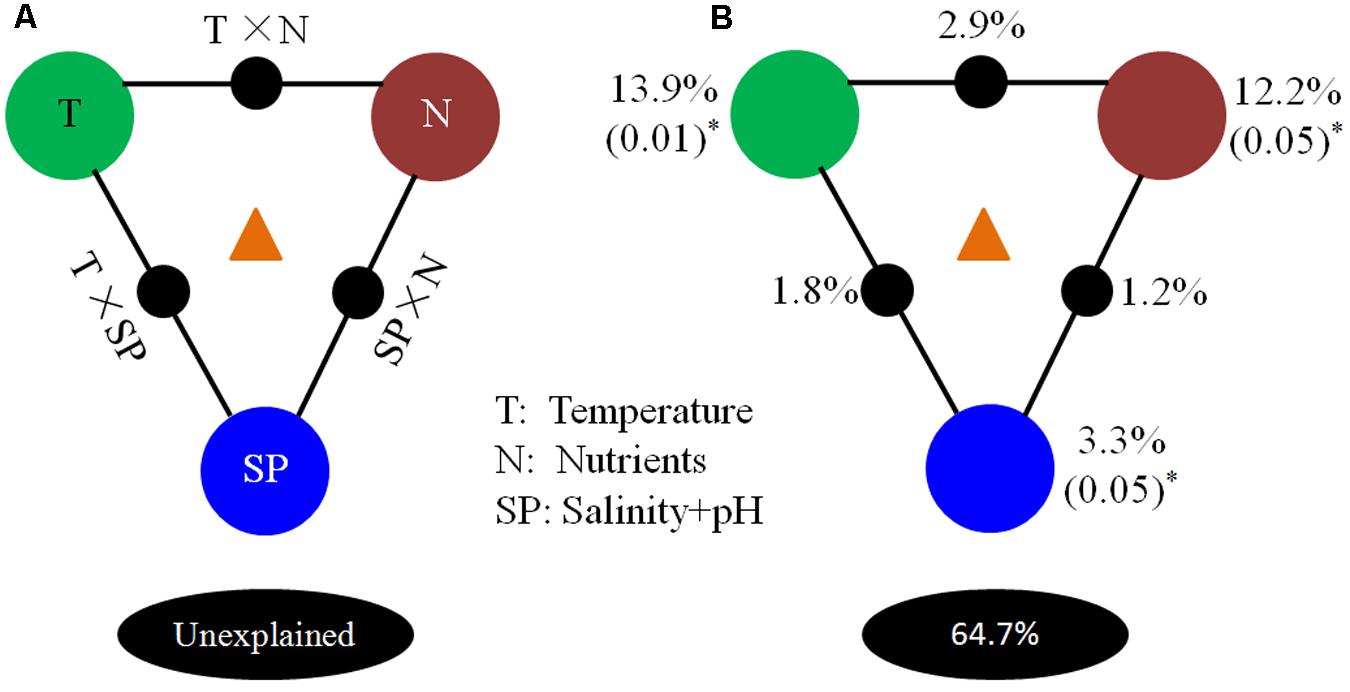
FIGURE 9. Variation partitioning analysis of microbial distribution explained by environmental factors. (A) General outline; (B) test environmental parameters, including temperature (T), nutrients (N), and “salinity + pH value” (SP). Each diagram represents the variation partitioned into the relative effects of each factor or combination of factors, in which area is proportional to the respective percentages of variation explained. The edges of the triangles represent the variation explained by each factor alone. The sides of the triangles represent interactions of any two factors, and the middle parts of the triangles represent interactions of all parameters.
Association Network
An interaction network based on community correlations is shown in Figure 10. Among the 15 types of microorganisms analyzed at the class level, there were 14,331 associations, of which 57.6% were positive and 42.4% were negative. These associations represented inter- and intra-phyla interactions in the co-occurring subcommunities, including 663 pairs, 3,521 triplets, and 4,008 quadruplets. Among the positive relationships, all taxa were connected with each other in the network, but Halobacteria, dinoflagellates, Spirotrichea, Maxillopoda, and Gammaproteobacteria were keystone taxa. In pairwise analyses, these five groups co-occurred most frequently with the other members, particularly with dinoflagellates and Halobacteria (Figure 10A) (P < 0.01). Active interplay was observed in the negative relationships, which appeared to the highest degree in the archaea. The Halobacteria was strongly negatively correlated (P < 0.01) with several organisms, including Dictyochophyceae, Mamiellophyceae, Gammaproteobacteria, Flavobacteria, and some zooplankton (such as Maxillopoda) (Figure 10B).
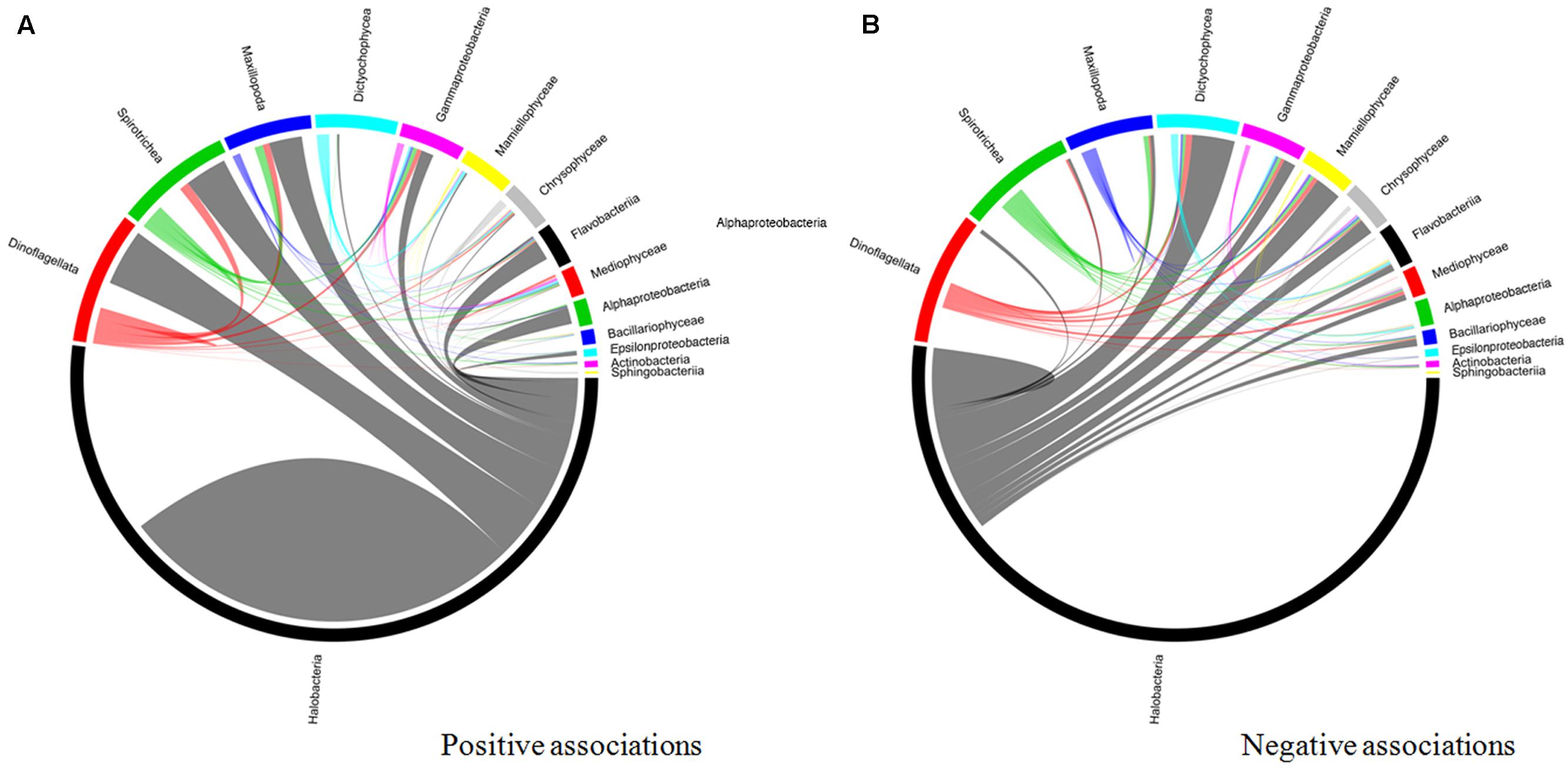
FIGURE 10. Taxonomic patterns identified within the co-occurrence network. The pop 14 interacting taxon groups are depicted as colored segments in a chord diagram, in which ribbons connecting two segments indicate co-presence (A) and exclusion (B) links, respectively. Size of the ribbon is proportional to the number of links (co-presence and exclusion) between the OTUs assigned to the respective segments, and color is segment (of the two involved) with the more total links. Links are dominated by Alphaproteobacteria, Gammaproteobacteria, Flavobacteria, and Thermoplasmata. The chord diagram was generated using the circlized package in R based on the adjacency matrix for the taxon of interest.
To further investigate the correlations between microbial species and the bloom-forming dinoflagellate A. catenella, a network was constructed based on significant OTUs (Figure 11). For simplicity, we selected the top 30 OTUs, which were analyzed at the genus level. Among the bacterial groups, three members (Caldithrix, Spirochaeta, and Sulfurovum) had strong positive correlations with A. catenella; five members (Glaciecola, Reineken, Owenweeksia, Candidatus Aquiluna, and Thiomicrospira) were significantly negatively correlated with A. catenella; and the remaining species (Marinobacterium and Pseudospirillum) exhibited weak correlations with the target algae. Within the archaea, three main members (Methanolobus, Nitrosopumilus, and Nitrosoarchaeum) were correlated with bloom-forming Alexandrium sp., whereas other archaea were not significantly associated with algal dynamics. In the microeukaryote biosphere, Thalassiosira and Woloszynskia directly influenced algal abundance, whereas Helicostomella displayed negative effects on algae concentration through grazing or parasitic infection (Figure 11). An interesting finding was that three species (Woloszynakia, Navicula, and Methanolobus) were correlated with both bloom formation and collapse, and exhibited both positive and negative correlations with A. catenella.
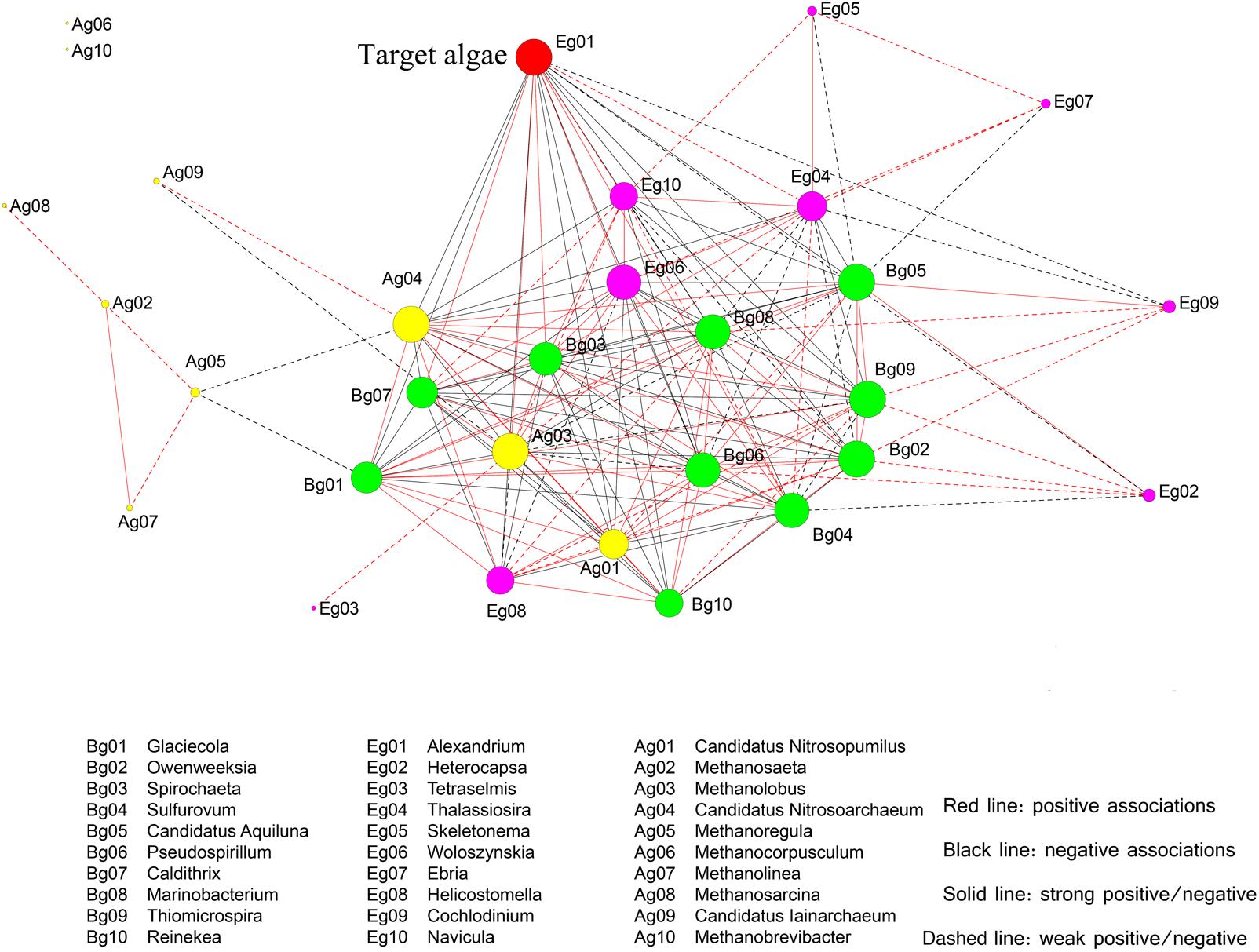
FIGURE 11. Correlation relationship with the top 30 genera comprising microeukaryotes and target algae (A. catenella), based on significant Spearman rank correlation coefficients. The deep red nodes represent target algae (Eg01, the bloom-forming species A. catenella); green nodes indicate bacterial taxa; yellow nodes indicate archaea; and the light red nodes indicate eukaryotes. Circle size reflects the number of connections the OTUs has with other OTUs. Red bold lines indicate strong positive correlations and red dotted lines indicate positive associations; black bold lines indicate strong negative correlations, black dotted lines indicate negative associations.
Functional Prediction
Based on the detection of functional genes, differences in the functional potential of bacterial communities were found among the different bloom stages. The three groups representing major functions (cell proliferation, cofactor and vitamin metabolism, and glycan biosynthesis) showed higher activity in the onset/exponential growth stage [W2–W4] compared with the pre-bloom stage [W1]. Amino acid and carbohydrate metabolism were the main pathways present during the bloom’s peak, leading into decline [W5–W6]. Interestingly, when the bloom began to decline [W7–W9], these metabolic pathways were diminished, whereas sulfur, lipid metabolism, and environmental adaptation genes were enriched during this phase (Figure 12).
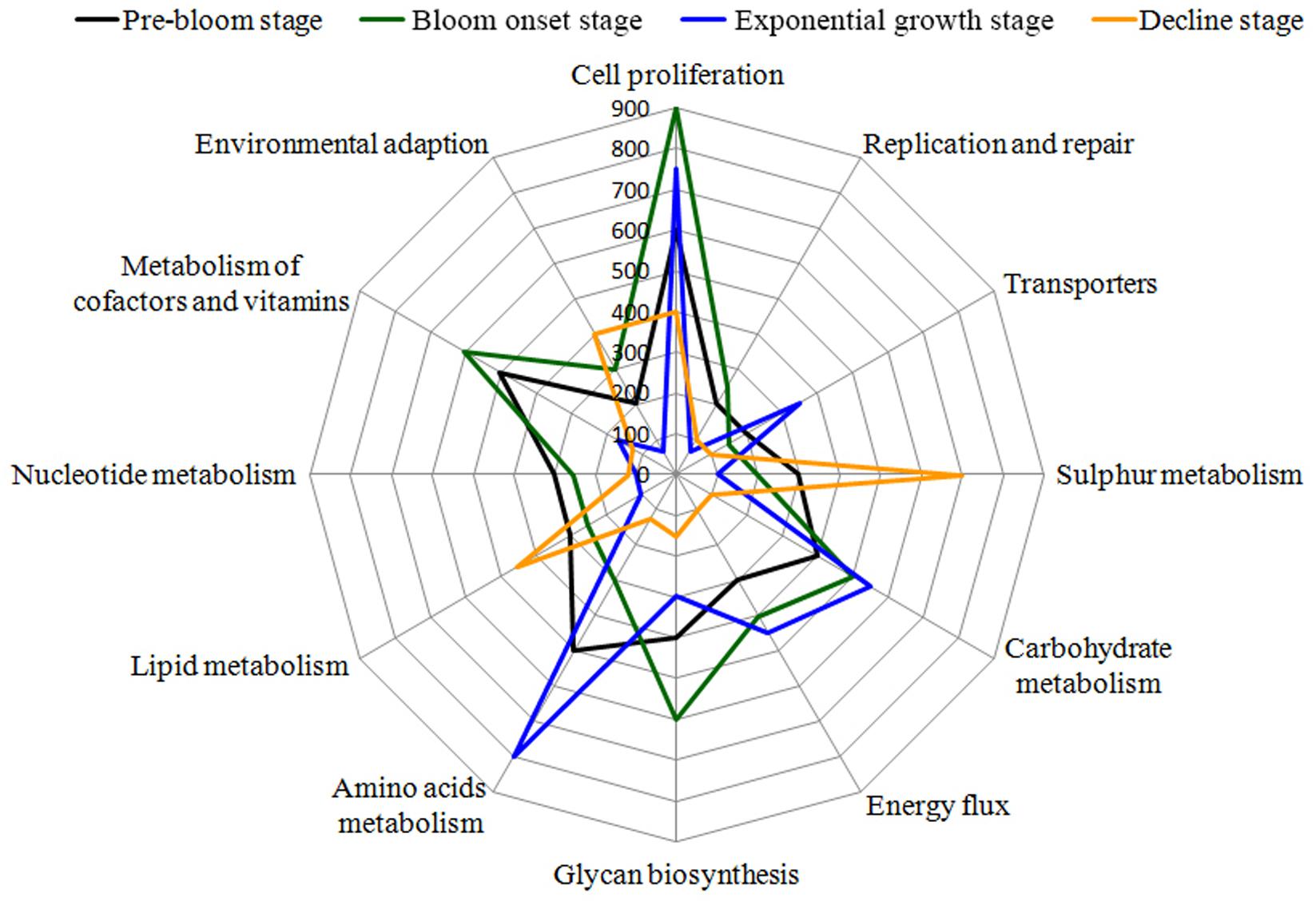
FIGURE 12. Bacterial taxa associated with algal bloom status are related to several gene functional pathways. Gene functions were predicted from 16S rRNA gene-based microbial compositions using the PICRUSt algorithm to make inferences from KEGG annotated databases. Relative signal intensity was normalized by the number of the genes for each indicated metabolic pathway. The main KEGG pathways, including proteins involved in carbohydrate metabolism, energy flux, lipid metabolism, environmental adaption, cell growth, signal transaction and related physiological behavior are included in the radar graph.
Discussion
Ecological studies have suggested the presence of specific algal-microbial interactions based on their co-occurrence in marine systems, which have the potential to influence biogeochemical cycles and shape the community structure of these groups (Amin et al., 2012; Kouzuma and Watanabe, 2015). However, much is yet unknown regarding the mechanisms and dynamics of these interactions, and this remains an area of active research and debate. In this work, we investigated temporal algal and microbial community structure during a bloom of A. catenella in an isolated salt pond in the Nauset Estuary on Cape Cod, MA, United States. Self-seeding and annual phytoplankton blooms occur every spring in this system, making Salt Pond an ideal “natural laboratory” for studying algal-microbial dynamics (Crespo et al., 2011; Ralston et al., 2014). Our analysis focused on documenting microbial community structure during algal blooms, and identifying specific associations among bacteria, archaea, and microeukaryotes to provide insights into mechanisms underlying microbial interactions and dynamics.
Bacterial Community Dynamics
During the earliest bloom stages, the primary bloom-associated bacterial groups were Gammaproteobacteria, Alphaproteobacteria, and Bacteroidetes. Among these taxa, Gammaproteobacteria were the most abundant in OTU sequences. Comprised of predominantly SAR86 and SAR92 clusters, this group is often defined as opportunistic and has a broad generalist substrate spectrum (Skerratt et al., 2002). During the early bloom stages, phytoplankton produce and release carbohydrates, sugar alcohols, and amino/organic acids, carbohydrates, which serve as chemoattractants for these bacterial groups (Buchan et al., 2014). Prior analysis of in situ data documented high expression levels of transporters for phosphates, peptides, monosaccharides, and other monomers in these taxa during phytoplankton blooms, indicating that they may be specialized for substrate utilization of algal-derived organic matter (Teeling et al., 2012). In studies of bacterium–A. catenella associations the Gulf of Maine (Northwest Atlantic), Hasegawa et al. (2007) found that a dominant and diverse group of Gammaproteobacteria was closely associated with A. catenella. Our results are similar to this observation and suggest that Gammaproteobacteria have a taxon-specific selective capability in the phycosphere niche. In addition, we observed that Gammaproteobacteria were still a dominant species during the later bloom stages (Figure 4). Gammaproteobacteria, and particularly members of the Alteromonas–Pseudomonas–Vibrio group, are frequently characterized as r-strategists (Weinbauer et al., 2006), colonizing quickly and promoting growth of more specialized taxa. Hence, Gammaproteobacteria provide a certain degree of buffering, which may have promoted stability in the microbial community structure as the Alexandrium bloom progressed.
During bloom onset, the bacterial community composition changed, and was dominated by Bacteroidetes (especially Flavobacteria) and Alphaproteobacteria (such as Roseobacter and SAR11). Flavobacteria are highly diverse, and are capable of degrading high-molecular-weight organics (e.g., cellulose, chitin, and pectin) (Kirchman, 2002). This group is thus regarded as an important component of the microbial loop in coastal phytoplankton blooms (Jones et al., 2010; Williams et al., 2013; Tully et al., 2014). Indeed, Taylor et al. (2014) demonstrated that exopolymer particles (due to algal lysis) area niche occupied by Flavobacteriales. Phytoplankton-derived polymeric organic matter is processed by actively hydrolyzing bacteria, as well as a wide variety of opportunistic bacteria that are capable of quenching the products of hydrolysis (Teeling et al., 2012). Prior field studies have reported that certain Flavobacteria are abundant during stationary growth and bloom decline (Newton et al., 2011). In this work, Flavobacteriaceae were present during all phases of the A. catenella bloom and exhibited pronounced fluctuations, perhaps reflecting their role in the processing of organic matter during blooms (Pinhassi et al., 2004). In addition to Bacteroidetes, Roseobacter and SAR11 are two other actors in A. catenella bloom onset. Roseobacter exhibits a high degree of physiological and phenotypic differentiation (Lucena et al., 2012), and has been reported to degrade a variety of dissolved low-molecular-weight organics during phytoplankton blooms, including those associated with HAB species such as Alexandrium sp., Gymnodinium catenatum, and Prorocentrum lima (Lafay et al., 1995; Green et al., 2004; Jasti et al., 2005; Wagner-Dobler and Biebl, 2006). During bloom development, Roseobacter is a “pioneer” bacterium, and plays two critical ecological roles in the phycosphere. One is its role as the dominant species in the natural environment, which is achieved through its ability to compete and thrive under low-nutrient conditions (Pinhassi and Berman, 2003), and the second is its provision of sulfur compounds that stimulate algal growth (Tan et al., 2015). These roles reflect both ecological strategies and contributions of this dominant group. The SAR11 (such as Rhizobiales) is another active producer of S and N during blooms (Teeling et al., 2012). Shifts in OTU numbers we observed corroborates the hypothesis that members of the Rhizobiales clade are capable of rapidly changing metabolic functioning in response to changing conditions during phytoplankton blooms, thus enhancing their competitive ability (Gao, 2015).
When an algal bloom begins to decline, bacterial community structure becomes more diverse and complex. Phytoplankton release macromolecules and small molecules (e.g., polysaccharides, proteins, lipids, and other material from cell lysis) in response to nutrient-limiting conditions, which further stimulates heterotrophic bacterial activity (Buchan et al., 2014). Indeed, this phenomenon was observed in the current study, exemplified by the substantial increase in Actinobacteria and Cyanobacteria (Supplementary Figure S3A). In the oceanic environment, Actinobacteria helps to decompose organic matter (e.g., dead algae) for uptake by phytoplankton. Several researchers have reported that Actinobacteria are closely linked to the diatom/dinoflagellate blooms (Eckert et al., 2012; Bunse et al., 2016), which could help to explain their wide distribution and high abundances following blooms. Cyanobacteria are fast-growing nutrient opportunists in the phycosphere environment, and can compete with other slow-growing nutrient specialists via exploitative resource competition (Vallina et al., 2014). This is largely governed by substrate availability, as algal-derived substrates provide ecological niches for specific populations and concurrently generate a selective advantage for bacteria that are fundamentally opportunistic (Taylor et al., 2014). In addition, photosynthetic cyanobacterial taxa such as Synechococcus, Chroococcales, and Oscillatoriales were detected in samples collected during bloom decline and contributed about 10–30% to overall abundance (data not shown), which is in line with previous reports suggesting a role for Cyanobacteria in sinking particle flux (as decomposers of organic material from dead algae) (Palenik, 2015).
It is important to note that some bacteria exhibit dual roles during different stages of the bloom, such as Cytophaga–Flavobacterium–Bacteroides (CFB) and Rhodobacterales. In this work, the most marked fluctuations were found in heterotrophic taxa, including the CFB members, which may reflect their ability to quickly establish and grow under changing conditions (algal lysis, grazing, and nutrient depletion) during the bloom. Previously, Rinta-Kanto et al. (2012) demonstrated that heterotrophic microbes decrease energy conservation in response to blooms, effectively shifting bioreactive compounds assimilated from the DOM (dissolved organic matter) pool, and altering the surfaces of cells in a manner that effectively promotes both adhesion and particle formation. This is a survival strategy in response to increasing competition for resources. In addition, CFB are also the most common algicidal bacteria (Kodama et al., 2006), which may also explain why CFB are found during the decay of the blooms (Zhou et al., 2016a). Another widespread and commonly found group is Rhodobacterales, which comprise large fractions of the total bacterioplankton. Given its antagonism toward other bacteria, this group may be an important regulator of HAB dynamics (Roth P. A. et al., 2008; Roth P. B. et al., 2008; Li et al., 2013). Our results showed that Rhodobacterales can wax and wane with Alexandrium sp., thus exhibiting non-equilibrium coexistence.
Archaeal Community Structure
Archaea have been largely ignored as potential agents in phytoplankton dynamics, although a few studies indicated a euryarchaeal association with eukaryotic phytoplankton (Needham and Fuhrman, 2016). In this work, we found that most archaeal members belonged to Marine Group II (Figure 5), and their communities during the bloom were less complex compared with bacterioplankton. Changes in archaeal community composition during blooms have been sporadically reported in blooms of diatoms (Kim et al., 2014), cyanobacteria (Xing et al., 2012), and dinoflagellates (such as Scrippsiella trochoidea) (Lumarie, 2006; Tan et al., 2015); and most demonstrated that methanogens were predominant members of the archaeal biosphere. Similar to the aforementioned results, we also found that the dominant group throughout the bloom was comprised of methanogenic archaea (nearly 50%) (Figure 5). During the pre-bloom period, Methanomicrobiales and Methanocellales were the predominant genera. The Methanocellales are phylogenetically diverse, and may play a significant role in ecosystem functioning through their ability to process complex compounds into simpler molecules in both anaerobic and aerobic habitats (Chouari et al., 2015). This ability to convert polysaccharides into compounds that are usable by other taxa might allow Methanocellales to be more competitive than groups reliant on other energy sources. Our observations suggest that the occurrence of Methanocellales at the beginning of the bloom may have been associated with the less eutrophic conditions present at that time.
Two additional methane-metabolizing members, Methanosarcinales and Methanobacteriales, co-occurred in the mid- and post-bloom stages, potentially as syntrophic partners inorganic biomass degradation (Chauhan et al., 2006). In addition, it should be noted that different algal hosts may have been associated with different methanogenic archaea. In diatoms, Methanomicrobiales constituted the resident population due to its fast growth response to nitrate and phosphate availability (den Haan et al., 2013), whereas in our study, Methanobacteriales and Methanomicrobiales were both commonly associated with Alexandrium sp. This may reflect their growth responses to the variety of organic compounds produced by different phytoplankton host, which selects for different archaeal communities (Schwarz et al., 2008).
During the post-bloom stage, AOA emerged, and Nitrosopumilaceae was particularly abundant. AOA activity provides an efficient way of removing ammonium waste secreted by the host and prevents toxic NH4+ accumulation, thus strengthening the relationship between phytoplankton and their symbionts. Pearman et al. (2016) further demonstrated that this recycling of ammonia is the predominant driving force in AOA community composition. One additional observation of note pertains to the Halobacteriales, which were enriched during the bloom’s terminal phase. The AOA archaeal communities present in Salt Pond late in the bloom were consistently associated with A. catenella, suggesting that these symbionts may play a key role in NH4+ detoxification for their host, and thus could significantly impact the nitrogen cycle in the phycosphere. One additional observation of note pertains to the Halobacteriales, which were enriched during the bloom’s terminal phase. Certain taxa in this group are capable of growing both aerobically and anaerobically, and they have a novel means of synthesizing energy through photosynthesis. In addition, Halobacteriales actively degrades organic matter in salty environments. The higher abundance of Halobacteria may thus indicate involvement in organic matter degradation, thus supplying inorganic compounds for algae (Zhou et al., 2014). This could be beneficial to resident algae since dissolved organic matter and nutrients concentrations are typically low during the pre-bloom stage.
Microeukaryote Community Structure
In the phytoplankton biosphere, obvious variations in community structure associated with bloom dynamics were observed. Significant differences in the Shannon– and Simpson indices were found among the different HAB stages (Figure 2), which indicated a lower level of α-diversity in whole bloom period. A significant shift in the phytoplankton community structure was also reflected in β-diversity measures (Figure 3). Pre-bloom phytoplankton communities were characterized by high diversity, which included both diatom and dinoflagellate taxa. Upon bloom initiation, diatom abundance declined markedly. This suggests that diatom growth may have been negatively influenced by the high abundance of Alexandrium sp. Allelopathic and auto-inhibition effects play important roles in competition between algae, and may have contributed to the dynamics we observed between these groups (Yamasaki et al., 2007; Wang et al., 2013).
During the early stages of the bloom, communities were dominated by Dinophyceae (Alexandrium, Heterocapsa, Woloszynskia, Cochlodinium, and Polykrikos), with various green algae or diatoms (Tetraselmis, Micromonas, Bathycoccus, Thalassiosira, Skeletonema, and Navicula) as minor contributors (Figure 6). Several days later, the number of algal cells peaked, and the eukaryotic community was comprised almost exclusively of Alexandrium sp. (90%). Subsequent to the bloom’s peak, multiple phytoplankton groups co-occurred, ranging from nutrient specialists to nutrient opportunists (such as green algae). During bloom decline, diatoms and green algae comprised nearly 50% of eukaryotic OTUs. Algal activities led to rapid exhaustion of nutrients, and in conjunction with other taxa (e.g., diatoms, eukaryote grazers), contributed to bloom termination. Other unmeasured hydrochemical characteristics and/or factors not examined in this study likely contributed as well. Algal mortality during this stage dramatically increased the substrate available to other microeukaryotic communities, and the most obvious shift in community composition occurred during bloom termination.
Other non-abundant Prymnesiophyceae species, haptophytes, comprised < 3% of the eukaryotic community and only appeared during the post-bloom stage. Despite high levels of haptophytes reported during blooms of other dinoflagellates (Friocourt et al., 2012), comparatively low haptophyte OTUs were observed in the dataset collected during this Alexandrium bloom. Several reasons for this finding are possible: (1) different hosts have different associated microeukaryotic communities; (2) higher copy numbers of ciliate and diatom 18S rRNA gene sequences dwarfed haptophyte DNA; and (3) primer mismatches or difficulties amplifying GC-rich haptophyte DNA, which resulted in low haptophyte tag yield. Despite the relatively small OTU tag numbers in our dataset, haptophytes are known to be opportunistic, and utilize urea and phosphonate as alternative sources of N and P (Pearman et al., 2016). In this study, a negative correlation was observed between haptophytes and Alexandrium (Figure 6), which may indicate that these species have the ability to compete with algae for nutrients and thus contribute to Alexandrium bloom termination.
Among the zooplankton communities, the most abundant taxa were protists (Apicomplexa), Arthropoda (Maxillopoda), ciliate (Ciliophora), and rotifera. Their abundance was enriched during the bloom termination and post-bloom stages. Most of these taxa are phytoplankton grazers, and are the primary drivers in top–down control that can play a critical role in bloom decline. Our results are similar to those reported by Alacid et al. (2015), i.e., that protists (such as the dinoflagellate parasitoid Parvilucifera sinerae) may be an important factor in the termination of Alexandrium minutum blooms in the natural environment. Regarding the observed increase in ciliates during the post-stage, one possible reason is the availability of easily digestible taxa such as green algae and diatoms, both of which were dominant at the end of the bloom (Figure 6). Fungi were another cornerstone taxa during the transition from a phytoplankton dominated community to non-phytoplankton-dominated community. In this work, Basidiomycota and Ascomycota were the primary members detected, and their abundance was increased during bloom termination. These results are similar to our previous observations (Sun et al., 2017), and suggest that there might be a saprophytic association between fungi and the decomposition of A. catenella biomass.
In addition to bacteria, archaea, and microeukaryotes, viruses are another important regulator of algal bloom activity. Tomaru et al. (2007) reported that viral infection by HcRNAV significantly impacts the population dynamics of H. circularisquama, both in terms of population abundance (biomass) and structure (clonal composition). Recent work further indicates that viruses influence algal host physiology and behavior (Park et al., 2004; Cai et al., 2011). The present work did not carry out an analysis of viral impacts; however, more detailed knowledge is clearly needed regarding the interactions between viruses and their algal hosts, and the ecological implications for algal bloom events.
Environmental Factors Regulating Microbial Structure
Among the environmental parameters analyzed, we found that temperature was strongly correlated with bacterial diversity, and also influenced archaeal communities (Figures 8A,B). These results indicate that temperature was the major abiotic force shaping microbial community structure in Salt Pond, which is consistent with past studies that documented a pronounced impact of temperature on plankton composition (Niu et al., 2011; Hampton et al., 2014; Frenken et al., 2016). For example, in the German Bight Lucas et al. (2015) identified temperature as a major niche-defining factor that indirectly influenced patterns of short-term bacterioplankton succession during phytoplankton blooms. Together, these findings indicate that temperature-mediated succession of the plankton community drives variation in microbial community composition (Pomeroy and Wiebe, 2001). In the present study, salinity was also identified as a primary physical factor driving 16S diversity, particularly archaeal community composition (Figure 8B), which may exhibit sensitivity to osmotic pressure. Salinity was previously shown to be a principal driving force of archaeal community patterns at the global scale (Auguet et al., 2010), these results demonstrate the importance of salinity in determining archaeal community structure at local scales. It is worth noting that although other environmental factors measured in this study were not associated with community structure, it is certainly possible that other unmeasured water chemistry parameters participated in community dynamics. Similar to previous findings (Bolhuis and Stal, 2011), salinity was related to the archaeal dynamics in Salt Pond, but is only one of the factors determining archaeal community structure. Future studies into the influence of salinity on these communities, including the effect of different ionic compounds and the buffering capacity of seawater, would be helpful in clarifying the relevance of salinity to archaeal populations.
In addition to physical factors, N and P are two key chemical elements that influence microbial communities through their effects on substance utilization and growth (Garcia et al., 2016). The acquisition and efficiency of N/P are linked to specific nutrient stoichiometry, thus determining the succession of bloom-forming phytoplankton taxa such as Alexandrium sp. (Tsuchiya et al., 2014). In this work, we observed an inverse relationship between phytoplankton biomass and nutrient concentrations during the bloom. Following bloom initiation, concentrations of NO3- and NH4+ decreased during bloom development (Figures 1B,C), presumably due to uptake and utilization by Alexandrium sp. (Ji et al., 2018), and increased thereafter as the bloom declined. During this same time period, P concentrations also decreased at the bloom’s peak stage. Phytoplankton communities may thus have experienced P-limitation due to its depletion during the exponential growth phase (Ji et al., 2018). Microbial biomass was highest following the bloom’s peak, when nutrient levels again rose. These results support prior findings in which algal bloom community succession was regulated by nutrient availability, which contributes to bottom–up control over both phytoplankton and microbial populations (Teeling et al., 2012).
The environmental data were then analyzed to determine which parameters drive community variability. These analyses included temperature, nutrients, and hydrochemical parameters (pH and salinity) as explanatory variables. These factors independently explained 13.9, 12.2, and 3.3% of the total observed variations, respectively (Figure 9). It partly supports the principle of “everything is everywhere, but the environment selects” (O’Malley, 2008). Abiotic (physical conditions and nutrient availability) and biotic factors (co-occurrence or exclusion) have long been regarded as the primary drivers shaping the distribution of microbial communities during algal events; the former have been considered to have a stronger effect (Rohwer and Thurber, 2009; Smetacek, 2012; Worden et al., 2015). However, Lima-Mendez et al. (2015) reported that environmental factors contributed to just 18% of community variation of microbes at the global ocean scale. Similarly, in this study, just 35.3% of microbial community variation in the OTU data was explained by above-mentioned parameters, indicating that other abiotic factors not sampled (such as DOC, DOM, or ROS abundance) or biotic parameters (e.g., uncovered community interactions) may be important drivers of community structure. These findings, however, may also indicate that the effect of abiotic factors on community structure is more limited than was previously assumed (Worden et al., 2015). Further investigation of the influence of physical-chemical parameters, particularly over longer time scales, is needed to better characterize the relative contribution of these factors on community variation.
Association Network Correlations
Network analysis has been shown to be an effective tool for characterizing biotic interactions and associations at various taxonomic levels (Lupatini et al., 2014). In this study, we observed a variety of different co-occurrence patterns among dominating prokaryotes and eukaryotes. In bacterial communities, diverse inter- and intra-relationships were observed at the class level (Figures 10A,B). Furthermore, the putative keystone nodes in the network were highly abundant (e.g., Spirotrichea, Maxillopoda, and Gammaproteobacteria), suggesting that these groups are important in maintaining network structures in the microbial community. Several examples of interplay (positive or negative) among these groups have been reported previously (Chaffron et al., 2010), which support the biological relevance of the findings reported here. In the archaea, more negative than positive interactions were observed in Halobacteria, suggesting that competition may occur more frequently than cooperation between archaea and other bacterioplankton. Notably, Zheng et al. (2013) showed that patterns of diversity in archaea are primarily driven by stochastic processes; our results lend support to this view and suggest that competition contributed to the random process of archaeal distribution. In the eukaryotes, relatively low connectivity (focusing on dinoflagellates) was observed in the network, indicating that eukaryotes were less affected by surrounding microorganisms and thus exhibited higher stability (Zhou et al., 2010). Overall, our results suggest that community composition was shaped by both competitive and cooperative forces. Although the exact contribution of biotic interactions with community variations is not presently known, this work provides possible clues to foster a better understanding regarding which keystone taxa may be present in the microbial communities during blooms, how they interact, and which bioprocesses they influence in the phycosphere environment.
A multiplicity of inter- and intra-specific associations (direct or indirect) within the network were further analyzed in order to illuminate correlations between the primary microbes and the bloom-forming algae Alexandrium sp. (Figure 11). Among the bacterial groups, positive correlations were observed during the bloom that suggested certain functional interdependencies between bacteria and algae. In this study, Alexandrium sp. was positively correlated with Caldithrix, Spirochaeta, and Sulfurovum. These keystone species are characterized as opportunistic and play multiple roles, including the degradation of organic matter (Seyedsayamdost et al., 2011), metabolizing dimethylsulfoniopropionate (DMSP) (Moran et al., 2012). And essentially serving as a nutrient “cooker” (producing vitamins, iron, and dissolved organic material) (Jones et al., 2010). These positive correlations indicated that these species would have been adapted to the surrounding environment, and may have played a role in algal bloom duration (Tan et al., 2015). In contrast, certain negative correlations between bacteria (such as Glaciecola, Reineken, and Owenweeksia) and Alexandrium sp. were also observed, reflecting the appearance of nutrient competitors or algicidal bacteria during the blooms. The former are more efficient than algae at acquiring P and N from organic compounds (Løvdal et al., 2008), while the latter can release molecules that kill or lyse algal cells (Mu et al., 2007; Amin et al., 2012).
Similar to bacteria, certain archaeal species were directly or indirectly correlated with Alexandrium sp. dynamics. Methanolobus was the primary group present during bloom formation, whereas Nitrosopumilus and Nitrosoarchaeum were the key members during bloom decline and termination. As a member of the methanogenic archaea, Methanolobus exhibit positive effects as symbionts, due in part to their participation in the N cycle in the phycosphere environment (Needham and Fuhrman, 2016). Nitrosopumilus and Nitrosoarchaeum likely were the major N-source competitors with algae because of their ability to utilize and oxidize ammonia (Metcalf et al., 2012). In addition to the aforementioned species, certain archaea exhibited strong interdependence with other taxa. These “hub species” (highly linked species within their own module) may function to promote the stability and maintenance of the network. In the microeukaryotic biosphere, positive correlations with Alexandrium sp. were observed during the algal bloom period, including Thalassiosira and Woloszynskia, but negative correlations were more frequent. Helicostomella exhibited the most significantly negative correlations with Alexandrium sp., indicating that competitors are primary drivers in the top–down process. An additional and interesting finding was that three genera (Woloszynakia, Navicula, and Methanolobus) had a dual correlation with bloom growth and collapse, suggesting the ability to adapt the changing environmental conditions associated during the algal bloom, based on their documented genotypic and metabolic diversity (Cram, 2015). It should be noted that the correlations between microorganisms and Alexandrium sp. reported in this study are based on relative abundance; as improved quantification methods become available to researchers, it will be possible to investigate these relationships using a more statistically robust approach.
Potential Microbial Function
To further investigate the ecological role of phycosphere microbiota, functional predictions were analyzed using the PICRUSt algorithm (Langille et al., 2013). To reduce complexity, only bacteria were analyzed. From the functional modules, we found that genes related to cell proliferation, carbohydrate metabolism, and energy flux were highly abundant during bloom onset, whereas functional modules involved in lipid, sulfur metabolism, and environmental adaption were over-represented during the bloom decline stages (Figure 12). Using Geochip, Yang et al. (2016) found that carbon degradation activity significant increased during a bloom of Akashiwo sanguinea, which was consistent with our results. Energy metabolism was maintained at relatively high levels during the onset and exponential stages, which may have contributed to the Alexandrium bloom’s duration. Similar results were observed in A. sanguinea, and through promotion of nitrogen (ammonium) accumulation, organic acid utilization, and carbon assimilation (Kudela et al., 2008; Rinta-Kanto et al., 2012). In this study, genes related to lipid and sulfur metabolism were mainly enriched in the onset and decline phases, which indicate that S and P availability were important for both bloom initiation and termination. Additionally, modules for amino acids metabolism and active transporters were over-represented in log-phase and during the beginning stage of bloom termination. These results show that bacterial functions affect phycosphere metabolic activity and algal bloom development trajectories.
Conclusion
This work analyzed microbial (bacteria, archaea, and microeukaryotes) assemblages from the natural environment during a phytoplankton bloom event. The microbial response to algal blooms exhibited high heterogeneity and complex dynamics, which comprised the succession of distinct populations exhibiting distinct functional profiles. Further, the association networks showed that algal and microbial community structure observed during the bloom was shaped by environmental factors as well as biotic interactions. The results highlight the importance of microbial network interactions as a robust tool to help understand the fate of HABs. It should be noted that we could not investigate the metaproteomes or metabolomes of phycosphere microorganisms in the present study. Future research using integrated approaches, such as flow cytometry and “Omics” methods, will continue to improve our understanding of microbial community interactions during HAB events, and could be used to further explore causal relationships between microbial diversity and activity, and algal blooms.
Author Contributions
JZ and ZC performed the experiments. JZ and MR drafted the manuscript. TS and DK collected the samples and analyzed the data. JZ and MR prepared the figures and tables. DA, ZC, and DK completed critical comments and revision.
Funding
This work was supported by NSFC (41476092, 41741015), S&T Projects of Shenzhen Science and Technology Innovation Committee (JCYJ20150831192329178, JCYJ20170817160708491, and JCYJ20170412171959157), Key Research and Development Plan of Ministry of Science and Technology of China (2017YFC1403600), as well as by the Woods Hole Center for Oceans and Human Health through the National Science Foundation (Grant OCE-1314642), and National Institute of Environmental Health Sciences (Grant 1-P01-ES021923-01).
Conflict of Interest Statement
The authors declare that the research was conducted in the absence of any commercial or financial relationships that could be construed as a potential conflict of interest.
Acknowledgments
Special thanks to Judy Kleindinst, Yihua Lyu, Yan Gao, and other members of Dr. Anderson’s laboratory at WHOI for assistance in sample preparation and software use.
Supplementary Material
The Supplementary Material for this article can be found online at: https://www.frontiersin.org/articles/10.3389/fmicb.2018.01201/full#supplementary-material
FIGURE S1 | Morphology of Alexandrium catenella under light microscopy.
FIGURE S2 | Photographs of DAPI-strained filter samples under fluorescence microscopy (1000×). 1 mL of fixed sample was filtered onto 0.22 μm pore-size polycarbonate filters. The blue spots show bacteria particles in the sample (circles show the smaller bacteria). The scale bar is 10 μm.
FIGURE S3 | Relative abundance of bacteria at phylum level (A), and Proteobacteria at class level (B).
FIGURE S4 | Correlation analysis between relative abundances of the 100 most abundant bacterial OTUs and environmental variables based on Pearson correlations. Correlation values depict r-values of Pearson correlations. Statistical significance levels: ∗P < 0.05, ∗∗P < 0.01, and ∗∗∗P < 0.001.
FIGURE S5 | Correlation analysis between relative abundances of the most abundant archaeal OTUs and environmental variables based on Pearson correlations. Correlation values depict r-values of Pearson correlations. Statistical significance levels: ∗P < 0.05, ∗∗P < 0.01, and ∗∗∗P < 0.001.
FIGURE S6 | Correlation analysis between relative abundances of the 100 most abundant microeukaryote OTUs and environmental variables based on Pearson correlations. Correlation values depict r-values of Pearson correlations. Statistical significance levels: ∗P < 0.05, ∗∗P < 0.01, and ∗∗∗P < 0.001.
Footnotes
- ^ http://cmore.soest.hawaii.edu
- ^ http://drive5.com/uparse/
- ^ https://cran.r-project.org/web/packages/circlize/index.html
- ^ http://www.phylotemp.microeco.org
References
Adachi, M., Kanno, T., Okamoto, R., Itakura, S., Yamaguchi, M., and Nishijima, T. (2003). Population structure of Alexandrium (Dinophyceae) cyst formation-promoting bacteria in Hiroshima Bay, Japan. Appl. Environ. Microbiol. 69, 6560–6568. doi: 10.1128/AEM.69.11.6560-6568.2003
Alacid, E., Reñé, A., and Garcés, E. (2015). New insights into the parasitoid Parvilucifera sinerae life cycle: the development and kinetics of infection of a bloom-forming dinoflagellate host. Protist 166, 677–699. doi: 10.1016/j.protis.2015.09.001
Amin, S. A., Hmelo, L. R., van Tol, H. M., Durham, B. P., Carlson, L. T., Heal, K. R., et al. (2015). Interaction and signaling between a cosmopolitan phytoplankton and associated bacteria. Nature 522, 98–101. doi: 10.1038/nature14488
Amin, S. A., Parker, M. S., and Armbrust, E. V. (2012). Interactions between diatoms and bacteria. Microbiol. Mol. Biol. Rev. 76, 667–684. doi: 10.1128/MMBR.00007-12
Anderson, D. M., Alpermann, T. J., Cembella, A. D., Collos, Y., Masseret, E., and Montresor, M. (2012). The globally distributed genus Alexandrium: multifaceted roles in marine ecosystems and impacts on human health. Harmful Algae 14, 10–35. doi: 10.1016/j.hal.2011.10.012
Anderson, D. M., and Stolzenbach, K. D. (1985). Selective retention of two dinoflagellates in a well-mixed estuarine embayment: the importance of diel vertical migration and surface avoidance. Mar. Ecol. Prog. Ser. 25, 39–50. doi: 10.3354/meps025039
Auguet, J. C., Barbera, A., and Casamayor, E. O. (2010). Global ecological patterns in uncultured archaea. ISME J. 4, 182–190. doi: 10.1038/ismej.2009.109
Bagatini, I. L., Eiler, A., Bertilsson, S., Klaveness, D., Tessarolli, L. P., and Vieira, A. A. (2014). Host-specificity and dynamics in bacterial communities associated with bloom-forming freshwater phytoplankton. PLoS One 9:e85950. doi: 10.1371/journal.pone.0085950
Bates, S. T., Berg-Lyons, D., Caporaso, J. G., Walters, W. A., Knight, R., and Fierer, N. (2011). Examining the global distribution of dominant archaeal populations in soil. ISME J. 5, 908–917. doi: 10.1038/ismej.2010.171
Bloh, A. H., Usup, G., and Ahmad, A. (2016). Loktanella spp. Gb03 as an algicidal bacterium, isolated from the culture of dinoflagellate Gambierdiscus belizeanus. Vet. World 9, 142–146. doi: 10.14202/vetworld.2016.142-146
Bolhuis, H., and Stal, L. J. (2011). Analysis of bacterial and archaeal diversity in coastal microbial mats using massive parallel 16S rRNA gene tag sequencing. ISME J. 5, 1701–1712. doi: 10.1038/ismej.2011.52
Bouchouicha Smida, D., Sahraoui, I., Mabrouk, H. H., and HlailiSakka, A. (2012). Seasonal dynamics of genus Alexandrium (potentially toxic dinoflagellate) in the lagoon of Bizerte (North of Tunisia) and controls by the abiotic factors. Crit. Rev. Biol. 335, 406–416. doi: 10.1016/j.crvi.2012.04.007
Buchan, A., LeCleir, G. R., Gulvik, C. A., and González, J. M. (2014). Master recyclers: features and functions of bacteria associated with phytoplankton blooms. Nat. Rev. Microbiol. 12, 686–698. doi: 10.1038/nrmicro3326
Bunse, C., Bertos-Fortis, M., Sassenhagen, I., Sildever, S., Sjöqvist, C., Godhe, A., et al. (2016). Spatiotemporal interdependence of bacteria and phytoplankton during a Baltic Sea spring bloom. Front. Microbiol. 7:517. doi: 10.3389/fmicb.2016.00517
Cai, W., Wang, H., Tian, Y., Chen, F., and Zheng, T. L. (2011). Influence of a bacteriophage on the population dynamics of toxic dinoflagellates by lysis of algicidal bacteria. Appl. Environ. Microbiol. 77, 7837–7840. doi: 10.1128/AEM.05783-11
Carnicer, O., Guallar, C., Andree, K. B., Diogène, J., and Fernández-Tejedor, M. (2015). Ostreopsis cf. ovata dynamics in the NW Mediterranean Sea in relation to biotic and abiotic factors. Environ. Res. 143(Pt B), 89–99. doi: 10.1016/j.envres.2015.08.023
Chaffron, S., Rehrauer, H., Pernthaler, J., and von Mering, C. (2010). A global network of coexisting microbes from environmental and whole-genome sequence data. Genome Res. 20, 947–959. doi: 10.1101/gr.104521.109
Chauhan, A., Reddy, K. R., and Ogram, A. V. (2006). Syntrophic-archaeal associations in a nutrient-impacted freshwater marsh. J. Appl. Microbiol. 100, 73–84. doi: 10.1111/j.1365-2672.2005.02751.x
Chouari, R., Guermazi, S., and Sghir, A. (2015). Co-occurence of crenarchaeota, thermoplasmata and methanogens in anaerobic sludge digesters. World J. Microbiol. Biotechnol. 31, 805–812. doi: 10.1007/s11274-015-1834-1
Cram, J. A. (2015). New insights into relationships between active and dormant organisms, phylogenetic diversity and ecosystem productivity. Mol. Ecol. 24, 5767–5769. doi: 10.1111/mec.13449
Crespo, B. G., Keafer, B. A., Ralston, D. K., Lind, H., Farber, D., and Anderson, D. M. (2011). Dynamics of Alexandrium catenella blooms and shellfish toxicity in the Nauset Marsh System of Cape Cod (Massachusetts). Harmful Algae 12, 26–38. doi: 10.1016/j.hal.2011.08.009
Crusius, J., Koopmans, D., Bratton, J. F., Charette, M. A., Kroeger, K., Henderson, P., et al. (2005). Submarine groundwater discharge to a small estuary estimated from radon and salinity measurements and a box model. Biogeosciences 2, 141–157. doi: 10.5194/bg-2-141-2005
Demuez, M., González-Fernández, C., and Ballesteros, M. (2015). Algicidal microorganisms and secreted algicides: new tools to induce microalgal cell disruption. Biotechnol. Adv. 33, 1615–1625. doi: 10.1016/j.biotechadv.2015.08.003
den Haan, J., Huisman, J., Dekker, F., ten Brinke, J. L., Ford, A. K., van Ooijen, J., et al. (2013). Fast detection of nutrient limitation in macroalgae and seagrass with nutrient-induced fluorescence. PLoS One 8:e68834. doi: 10.1371/journal.pone.0068834
Doucette, G. J. (1995). Interactions between bacteria and harmful algae: a review. Nat. Toxins 3, 65–74. doi: 10.1002/nt.2620030202
Eckert, E. M., Salcher, M. M., Posch, T., Eugster, B., and Pernthaler, J. (2012). Rapid successions affect microbial N-acetyl-glucosamine uptake patterns during a lacustrine spring phytoplankton bloom. Environ. Microbiol. 14, 794–806. doi: 10.1111/j.1462-2920.2011.02639.x
Edgar, R. C. (2013). UPARSE: highly accurate OTU sequences from microbial amplicon reads. Nat. Methods 10, 996–998. doi: 10.1038/nmeth.2604
Edgar, R. C., Haas, B. J., Clemente, J. C., Quince, C., and Knight, R. (2011). UCHIME improves sensitivity and speed of chimera detection. Bioinformatics 27, 2194–2200. doi: 10.1093/bioinformatics/btr381
Faust, K., Sathirapongsasuti, J. F., Izard, J., Segata, N., Gevers, D., Raes, J., et al. (2012). Microbial Co-occurrence relationships in the human microbiome. PLoS Comput. Biol. 8:e1002606. doi: 10.1371/journal.pcbi.1002606
Ferrier, M., Martin, J. L., and Rooney-Varga, J. N. (2002). Stimulation of Alexandrium fundyense growth by bacterial assemblages from the Bay of Fundy. J. Appl. Microbiol. 92, 706–716. doi: 10.1046/j.1365-2672.2002.01576.x
Field, C. B., Behrenfeld, M. J., Randerson, J. T., and Falkowski, P. (1998). Primary production of the biosphere: integrating terrestrial and oceanic components. Science 281, 237–240. doi: 10.1126/science.281.5374.237
Fierer, N., Hamady, M., Lauber, C. L., and Knight, R. (2008). The influence of sex, handedness, and washing on the diversity of hand surface bacteria. Proc. Natl. Acad. Sci. U.S.A. 105, 17994–17999. doi: 10.1073/pnas.0807920105
Frenken, T., Velthuis, M., SenerpontDomis, L. N., Stephan, S., Aben, R., Kosten, S., et al. (2016). Warming accelerates termination of a phytoplankton spring bloom by fungal parasites. Glob. Change Biol. 22, 299–309. doi: 10.1111/gcb.13095
Friocourt, Y. F., Skogen, M., Stolte, W., and Albretsen, J. (2012). Marine downscaling of a future climate scenario in the North Sea and possible effects on dinoflagellate harmful algal blooms. Food Addit. Contam. Part A Chem. Anal. Control Expo. Risk Assess. 29, 1630–1646. doi: 10.1080/19440049.2012.714079
Fuhrman, J. A., Cram, J. A., and Needham, D. M. (2015). Marine microbial community dynamics and their ecological interpretation. Nat. Rev. Microbiol. 13, 133–146. doi: 10.1038/nrmicro3417
Gao, Y. (2015). Application of Quantitative PCR and Imaging Flow Cytobot in the Study of Alexandrium Blooms. Ph.D. thesis, University of Chinese Academy of Sciences, Huairou.
Garcia, N. S., Bonachela, J. A., and Martiny, A. C. (2016). Interactions between growth-dependent changes in cell size, nutrient supply and cellular elemental stoichiometry of marine Synechococcus. ISME J. 10, 2715–2724. doi: 10.1038/ismej.2016.50
Green, D. H., Llewellyn, L. E., Negri, A. P., Blackburn, S. I., and Bolch, C. J. S. (2004). Phylogenetic and functional diversity of the cultivable bacterial community associated with the paralytic shellfish poisoning dinoflagellate Gymnodinium catenatum. FEMS Microbiol. Ecol. 47, 345–357. doi: 10.1016/S0168-6496(03)00298-8
Greenberg, A. E., Clesceri, L. S., and Eaton, A. D. (1992). Standard Methods for the Examination of Water and Wastewater. Washington, DC: American Public Health Association.
Hampton, S. E., Gray, D. K., Izmest’eva, L. R., Moore, M. V., and Ozersky, T. (2014). The rise and fall of plankton: long-term changes in the vertical distribution of algae and grazers in Lake Baikal, Siberia. PLoS One 9:e88920. doi: 10.1371/journal.pone.0088920
Hasegawa, Y., Martin, J. L., Giewat, M. W., and Rooney-Varga, J. N. (2007). Microbial community diversity in the phycosphere of natural populations of the toxic alga, Alexandrium catenella. Environ. Microbiol. 9, 3108–3121. doi: 10.1111/j.1462-2920.2007.01421.x
Hashihama, F., Kanda, J., Tauchi, A., Kodama, T., Saito, H., and Furuya, K. (2015). Liquid waveguide spectrophotometric measurement of nanomolar ammonium in seawater based on the indophenol reaction with o-phenylphenol (OPP). Talanta 143, 374–380. doi: 10.1016/j.talanta.2015.05.007
Huse, S. M., Huber, J. A., Morrison, H. G., Sogin, M. L., and Welch, D. M. (2007). Accuracy and quality of massively parallel DNA pyrosequencing. Genome Biol. 8:R143. doi: 10.1186/gb-2007-8-7-r143
Huse, S. M., Welch, D. M., Morrison, H. G., and Sogin, M. L. (2010). Ironing out the wrinkles in the rare biosphere through improved OTU clustering. Environ. Microbiol. 12, 1889–1898. doi: 10.1111/j.1462-2920.2010.02193.x
Jasti, S., Sieracki, M. E., Poulton, N. J., Giewat, M. W., and Rooney-Varga, J. N. (2005). Phylogenetic diversity and specificity of bacteria closely associated with Alexandrium spp. and other phytoplankton. Appl. Environ. Microbiol. 71, 3483–3494. doi: 10.1128/AEM.71.7.3483-3494.2005
Ji, N., Lin, L., Li, L., Yu, L., Zhang, Y., Luo, H., et al. (2018). Metatranscriptome analysis reveals environmental and diel regulation of a Heterosigma akashiwo (raphidophyceae) bloom. Environ. Microbiol. 20, 1078–1094. doi: 10.1111/1462-2920.14045
Jones, K. L., Mikulski, C. M., Barnhorst, A., and Doucette, G. J. (2010). Comparative analysis of bacterioplankton assemblages from Karenia brevis bloom and nonbloom water on the west Florida shelf (Gulf of Mexico, USA) using 16S rRNA gene clone libraries. FEMS Microbiol. Ecol. 73, 468–485. doi: 10.1111/j.1574-6941.2010.00914.x
Kim, J. G., Park, S. J., Quan, Z. X., Jung, M. Y., Cha, I. T., Kim, S. J., et al. (2014). Unveiling abundance and distribution of planktonic bacteria and archaea in a polynya in Amundsen Sea, Antarctica. Environ. Microbiol. 16, 1566–1578. doi: 10.1111/1462-2920.12287
Kirchman, D. (2002). The ecology of Cytophaga–Flavobacteria in aquatic environments. FEMS Microbiol. Ecol. 39, 91–100. doi: 10.1016/S0168-6496(01)00206-9
Klindworth, A., Mann, A. J., Huang, S., Wichels, A., Quast, C., Waldmann, J., et al. (2014). Diversity and activity of marine bacterioplankton during a diatom bloom in the North Sea assessed by total RNA and pyrotag sequencing. Mar. Genomics 18(Pt B), 185–192. doi: 10.1016/j.margen.2014.08.007
Kodama, M., Doucette, G. J., and Green, D. H. (2006). “Relationships between bacteria and harmful algae,” in Ecology of Harmful Algae, Ecological Studies, Vol. 189, eds E. Granéli and J. T. Turner (Berlin: Springer-Verlag), 243–255. doi: 10.1007/978-3-540-32210-8_19
Kouzuma, A., and Watanabe, K. (2015). Exploring the potential of algae/bacteria interactions. Curr. Opin. Biotechnol. 33, 125–129. doi: 10.1016/j.copbio.2015.02.007
Kudela, R. M., Lane, J. Q., and Cochlan, W. P. (2008). The potential role of anthropogenically derived nitrogen in the growth of harmful algae in California, USA. Harmful Algae 8, 103–110. doi: 10.1016/j.hal.2008.08.019
Lafay, B., Ruimy, R., Rausch de Traubenberg, C., Breittmayer, V., Gauthier, M. J., and Christen, R. (1995). Roseobacter algicola sp. nov., a new marine bacterium isolated from the phycosphere of the toxin-producing dinoflagellate Prorocentrum lima. Int. J. Syst. Bacteriol. 45, 290–296. doi: 10.1099/00207713-45-2-290
Langille, M. G., Zaneveld, J., Caporaso, J. G., McDonald, D., Knights, D., Reyes, J. A., et al. (2013). Predictive functional profiling of microbial communities using 16S rRNA marker gene sequences. Nat. Biotechnol. 31, 814–821. doi: 10.1038/nbt.2676
Lewitus, A. J., Horner, R. A., Caron, D. A., Garcia-Mendoza, E., and Hickey, B. M. (2012). Harmful algal blooms along the North American west coast region: history, trends, causes, and impacts. Harmful Algae 19, 133–159. doi: 10.1016/j.hal.2012.06.009
Li, D., Li, Y., and Zheng, T. L. (2013). Advance in the research of marine algicidal functional bacteria and their algicidal mechanism. Adv. Earth Sci. 28, 243–252.
Lima-Mendez, G., Faust, K., Henry, N., Decelle, J., Colin, S., Carcillo, F., et al. (2015). Ocean plankton. Determinants of community structure in the global plankton interactome. Science 348:1262073. doi: 10.1126/science.1262073
Lindh, M. V., Riemann, L., Baltar, F., Romero-Oliva, C., Salomon, P. S., Granéli, E., et al. (2013). Consequences of increased temperature and acidification on bacterioplankton community composition during a mesocosm spring bloom in the Baltic Sea. Environ. Microbiol. Rep. 5, 252–262. doi: 10.1111/1758-2229.12009
Liu, Z., Lozupone, C., Hamady, M., Bushman, F. D., and Knight, R. (2007). Short pyrosequencing reads suffice for accurate microbial community analysis. Nucleic Acids Res. 35:e120. doi: 10.1093/nar/gkm541
Løvdal, T., Skjoldal, E. F., Heldal, M., Norland, S., and Thingstad, T. F. (2008). Changes in morphology and elemental composition of V. splendidus along a gradient from carbon-limited to phosphate-limited growth. Microb. Ecol. 55, 152–161. doi: 10.1007/s00248-007-9262-x
Lucas, J., Wichels, A., Teeling, H., Chafee, M., Scharfe, M., and Gerdts, G. (2015). Annual dynamics of North Sea bacterioplankton: seasonal variability superimposes short-term variation. FEMS Microbiol. Ecol. 91:fiv099. doi: 10.1093/femsec/fiv099
Lucena, T., Pujalte, M. J., Ruvira, M. A., Garay, E., Macián, M. C., and Arahal, D. R. (2012). Tropicibacter multivorans sp. nov., an aerobic alphaproteobacterium isolated from surface seawater. Int. J. Syst. Evol. Microbiol. 62, 844–848. doi: 10.1099/ijs.0.030973-0
Lumarie, P. G. (2006). Common Bacterial Symbionts in Clonal Cultures of the Toxic Dinoflagellate Ostreopsis lenticularis. Master thesis, University of Puerto Rico Mayagüez Campus, Mayagüez.
Lupatini, M., Suleiman, A. K. A., Jacques, R. J. S., Antoniolli, Z. I., de Siqueira Ferreira, A., Kuramae, E. E., et al. (2014). Network topology reveals high connectance levels and few key microbial genera within soils. Front. Environ. Sci. 2:10. doi: 10.3389/fenvs.2014.00010
Metcalf, W. W., Griffin, B. M., Cicchillo, R. M., Gao, J., Janga, S. C., Cooke, H. A., et al. (2012). Synthesis of methylphosphonic acid by marine microbes: a source for methane in the Aerobic Ocean. Science 337, 1104–1107. doi: 10.1126/science.1219875
Mizumoto, H., Tomaru, Y., Takao, Y., Shirai, Y., and Nagasaki, K. (2008). Diverse responses of the bivalve-killing dinoflagellate Heterocapsa circularisquama to infection by a single-stranded RNA virus. Appl. Environ. Microbiol. 74, 3105–3111. doi: 10.1128/AEM.02190-07
Monier, A., Comte, J., Babin, M., Forest, A., Matsuoka, A., and Lovejoy, C. (2015). Oceanographic structure drives the assembly processes of microbial eukaryotic communities. ISME J. 9, 990–1002. doi: 10.1038/ismej.2014.197
Moran, M. A., Reisch, C. R., Kiene, R. P., and Whitman, W. B. (2012). Genomic insights into bacterial DMSP transformations. Ann. Rev. Mar. Sci. 4, 523–542. doi: 10.1146/annurev-marine-120710-100827
Mu, R. M., Fan, Z. Q., Pei, H. Y., Yuan, X. L., Liu, S. X., and Wang, X. R. (2007). Isolation and algae-lysing characteristics of the algicidal bacterium B5. J. Environ. Sci. 19, 1336–1340. doi: 10.1016/S1001-0742(07)60218-6
Murphy, J., and Riley, J. P. (1962). A modified single solution method for the determination of phosphate in natural waters. Anal. Chim. Acta 27, 31–36. doi: 10.1016/S0003-2670(00)88444-5
Nagasaki, K. (2008). Dinoflagellates, diatoms, and their viruses. J. Microbiol. 46, 235–243. doi: 10.1007/s12275-008-0098-y
Needham, D. M., and Fuhrman, J. A. (2016). Pronounced daily succession of phytoplankton, archaea and bacteria following a spring bloom. Nat. Microbiol. 1, 1–7. doi: 10.1038/nmicrobiol.2016.5
Newton, R. J., Jones, S. E., Eiler, A., McMahon, K. D., and Bertilsson, S. (2011). A guide to the natural history of freshwater lake bacteria. Microbiol. Mol. Biol. Rev. 75, 14–49. doi: 10.1128/MMBR.00028-10
Niu, Y., Shen, H., Chen, J., Xie, P., Yang, X., Tao, M., et al. (2011). Phytoplankton community succession shaping bacterioplankton community composition in Lake Taihu, China. Water Res. 45, 4169–4182. doi: 10.1016/j.watres.2011.05.022
O’Malley, M. A. (2008). Everything is everywhere: but the environment selects: ubiquitous distribution and ecological determinism in microbial biogeography. Stud. Hist. Philos. Biol. Biomed. Sci. 39, 314–325. doi: 10.1016/j.shpsc.2008.06.005
Ory, P., Hartmann, H. J., Jude, F., Dupuy, C., Del Amo, Y., Catala, P., et al. (2010). Pelagic food web patterns: do they modulate virus and nanoflagellate effects on picoplankton during the phytoplankton spring bloom? Environ. Microbiol. 12, 2755–2772. doi: 10.1111/j.1462-2920.2010.02243.x
Paerl, H. W., and Otten, T. G. (2013). Harmful cyanobacterial blooms: causes, consequences, and controls. Microb. Ecol. 65, 995–1010. doi: 10.1007/s00248-012-0159-y
Palenik, B. (2015). Molecular mechanisms by which marine phytoplankton respond to their dynamic chemical environment. Ann. Rev. Mar. Sci. 7, 325–340. doi: 10.1146/annurev-marine-010814-015639
Park, M. G., Yih, W., and Coats, D. W. (2004). Parasites and phytoplankton, with special emphasis on dinoflagellate infections. J. Eukaryot. Microbiol. 51, 145–155. doi: 10.1111/j.1550-7408.2004.tb00539.x
Parks, D. H., Tyson, G. W., Hugenholtz, P., and Beiko, R. G. (2014). STAMP: statistical analysis of taxonomic and functional profiles. Bioinformatics 30, 3123–3124. doi: 10.1093/bioinformatics/btu494
Pearman, J. K., Casas, L., Merle, T., Michell, C., and Irigoien, X. (2016). Bacterial and protist community changes during a phytoplankton bloom. Limnol. Oceanogr. 61, 198–213. doi: 10.1038/ismej.2015.135
Pinhassi, J., and Berman, T. (2003). Differential growth response of colony-forming alpha- and gamma-proteobacteria in dilution culture and nutrient addition experiments from Lake Kinneret (Israel), the eastern Mediterranean Sea, and the Gulf of Eilat. Appl. Environ. Microbiol. 69, 199–211. doi: 10.1128/AEM.69.1.199-211.2003
Pinhassi, J., Sala, M. M., Havskum, H., Peters, F., Guadayol, O., Malits, A., et al. (2004). Changes in bacterioplankton composition under different phytoplankton regimens. Appl. Environ. Microbiol. 70, 6753–6766. doi: 10.1128/AEM.70.11.6753-6766.2004
Polson, S. W. (2007). Comparative Analysis of Microbial Community Structure Associated with Acroporid Corals During a Disease Outbreak in the Florida Reef Tract. Ph.D. thesis, Medical University of South Carolina, Charleston, SC.
Pomeroy, L. R., and Wiebe, W. J. (2001). Temperature and substrates as interactive limiting factors for marine hetero-trophic bacteria. Aquat. Microb. Ecol. 23, 187–204. doi: 10.3354/ame023187
Poter, K. G., and Feig, Y. S. (1980). The use of DAPI for identifying and counting aquatic microflora. Limnol. Oceanogr. 25, 943–948. doi: 10.4319/lo.1980.25.5.0943
Quince, C., Lanzén, A., Curtis, T. P., Davenport, R. J., Hall, N., Head, I. M., et al. (2009). Accurate determination of microbial diversity from 454 pyrosequencing data. Nat. Methods 6, 639–641. doi: 10.1038/nmeth.1361
Ralston, D. K., Keafer, B. A., Brosnahan, M. L., and Anderson, D. M. (2014). Temperature dependence of an estuarine harmful algal bloom: resolving interannual variability in bloom dynamics using a degree day approach. Limnol. Oceanogr. 59, 1112–1126. doi: 10.4319/lo.2014.59.4.1112
Ramanan, R., Kim, B. H., Cho, D. H., Oh, H. M., and Kim, H. S. (2016). Algae-bacteria interactions: evolution, ecology and emerging applications. Biotechnol. Adv. 34, 14–29. doi: 10.1016/j.biotechadv.2015.12.003
Richlen, M. L., Erdner, D. L., McCauley, L. A., Libera, K., and Anderson, D. M. (2012). Extensive genetic diversity and rapid population differentiation during blooms of Alexandrium catenella (Dinophyceae) in an isolated salt pond on Cape Cod, MA, USA. Ecol. Evol. 2, 2588–2599. doi: 10.1002/ece3.373
Riemann, L., Steward, G. F., and Azam, F. (2000). Dynamics of bacterial community composition and activity during a mesocosm diatom bloom. Appl. Environ. Microbiol. 66, 578–587. doi: 10.1128/AEM.66.2.578-587.2000
Rinta-Kanto, J. M., Sun, S., Sharma, S., Kiene, R. P., and Moran, M. A. (2012). Bacterial community transcription patterns during a marine phytoplankton bloom. Environ. Microbiol. 14, 228–239. doi: 10.1111/j.1462-2920.2011.02602.x
Rohwer, F., and Thurber, R. V. (2009). Viruses manipulate the marine environment. Nature 459, 207–212. doi: 10.1038/nature08060
Roth, P. A., Mikulski, C. M., and Doucette, G. J. (2008). The influence of microbial interactions on the susceptibility of Karenia species to algicidal bacteria. Aquat. Microb. Ecol. 50, 251–259. doi: 10.3354/ame01167
Roth, P. B., Twiner, M. J., Mikulski, C. M., Barnhorst, A. B., and Doucette, G. J. (2008). Comparative analysis of two algicidal bacteria active against the red tide dinoflagellate Karenia brevis. Harmful Algae 7, 682–691. doi: 10.1016/j.hal.2008.02.002
Sanders, W. B. (2014). Complete life cycle of the lichen fungus Calopadia puiggarii (Pilocarpaceae, Ascomycetes) documented in situ: propagule dispersal, establishment of symbiosis, thallus development, and formation of sexual and asexual reproductive structures. Am. J. Bot. 101, 1836–1848. doi: 10.3732/ajb.1400272
Schloss, P. D., Westcott, S. L., Ryabin, T., Hall, J. R., Hartmann, M., Hollister, E. B., et al. (2009). Introducing mothur: open-source, platform-independent, community-supported software for describing and comparing microbial communities. Appl. Environ. Microbiol. 75, 7537–7541. doi: 10.1128/AEM.01541-09
Schwarz, J. I. K., Eckert, W., and Conrad, R. (2008). Response of the methanogenic microbial community of a profundal lake sediment (Lake Kinneret, Israel) to algal deposition. Limnol. Oceanogr. 53, 113–121. doi: 10.4319/lo.2008.53.1.0113
Segev, E., Wyche, T. P., Kim, K. H., Petersen, J., Ellebrandt, C., Vlamakis, H., et al. (2016). Dynamic metabolic exchange governs a marine algal-bacterial interaction. eLife 5:e17473. doi: 10.7554/eLife.17473
Seyedsayamdost, M. R., Case, R. J., Kolter, R., and Clardy, J. (2011). The Jekyll-and-Hyde chemistry of Phaeobacter gallaciensis. Nat. Chem. 3, 331–335. doi: 10.1038/nchem.1002
Skerratt, J. H., Bowman, J. P., Hallegraeff, G., James, S., and Nichols, P. D. (2002). Algicidal bacteria associated with blooms of a toxic dinoflagellate in a temperate Australian estuary. Mar. Ecol. Prog. Ser. 244, 1–15. doi: 10.3354/meps244001
Smetacek, V. (2012). Making sense of ocean biota: how evolution and biodiversity of land organisms differ from that of the plankton. J. Biosci. 37, 589–607. doi: 10.1007/s12038-012-9240-4
Sun, J. Y., Song, Y., Ma, Z. P., Zhang, H. J., Yang, Z. D., Cai, Z. H., et al. (2017). Fungal community dynamics during a marine dinoflagellate (Noctiluca scintillans) bloom. Mar. Environ. Res. 131, 183–194. doi: 10.1016/j.marenvres.2017.10.002
Tan, S. J., Zhou, J., Zhu, X. S., Xu, S. C., Zhang, W. G., Wang, B., et al. (2015). An association network analysis among microeukaryotes and bacterioplankton reveals algal bloom dynamics. J. Phycol. 51, 120–132. doi: 10.1111/jpy.12259
Taylor, J. D., Cottingham, S. D., Billinge, J., and Cunliffe, M. (2014). Seasonal microbial community dynamics correlate with phytoplankton-derived polysaccharides in surface coastal waters. ISME J. 8, 245–248. doi: 10.1038/ismej.2013.178
Teeling, H., Fuchs, B. M., Becher, D., Klockow, C., Gardebrecht, A., Bennke, C. M., et al. (2012). Substrate-controlled succession of marine bacterioplankton populations induced by a phytoplankton bloom. Science 336, 608–611. doi: 10.1126/science.1218344
Teeling, H., Fuchs, B. M., Becher, D., Klockow, C., Gardebrecht, A., Bennke, C. M., et al. (2016). Recurring patterns in bacterioplankton dynamics during coastal spring algae blooms. eLife 5:e11888. doi: 10.7554/eLife.11888
Theroux, S., Huang, Y., and Amaral-Zettler, L. (2012). Comparative molecular microbial ecology of the spring haptophyte bloom in a Greenland arctic oligosaline lake. Front. Microbiol. 3:415. doi: 10.3389/fmicb.2012.00415
Tomaru, Y., Hata, N., Masuda, T., Tsuji, M., Igata, K., Masuda, Y., et al. (2007). Ecological dynamics of the bivalve-killing dinoflagellate Heterocapsa circularisquama and its infectious viruses in different locations of western Japan. Environ. Microbiol. 9, 1376–1383. doi: 10.1111/j.1462-2920.2007.01252.x
Tsuchiya, K., Kuwahara, V. S., Yoshiki, T., Nakajima, R., Miyaguchi, H., Kumekawa, N., et al. (2014). Phytoplankton community response and succession in relation to typhoon passages in the coastal waters of Japan. J. Plankton Res. 36, 424–438. doi: 10.1093/plankt/fbt127
Tully, B. J., Sachdeva, R., Heidelberg, K. B., and Heidelberg, J. F. (2014). Comparative genomics of planktonic flavobacteriaceae from the Gulf of Maine using metagenomic data. Microbiome 2:34. doi: 10.1186/2049-2618-2-34
Vallina, S. M., Follows, M. J., Dutkiewicz, S., Montoya, J. M., Cermeno, P., and Loreau, M. (2014). Global relationship between phytoplankton diversity and productivity in the ocean. Nat. Commun. 5:4299. doi: 10.1038/ncomms5299
Wagner-Dobler, I., and Biebl, H. (2006). Environmental biology of the marine Roseobacter lineage. Annu. Rev. Microbiol. 60, 255–280. doi: 10.1146/annurev.micro.60.080805.142115
Wang, J., Zhang, Y., Li, H., and Cao, J. (2013). Competitive interaction between diatom Skeletonema costatum and dinoflagellate Prorocentrum donghaiense in laboratory culture. J. Plankton Res. 35, 367–378. doi: 10.1093/plankt/fbs098
Weinbauer, M. G., Christen, R., and Hofle, M. G. (2006). The response of Vibrio- and Rhodobacter-related populations of the NW Mediterranean Sea to additions of dissolved organic matter, phages, or dilution. Microb. Ecol. 51, 336–344. doi: 10.1007/s00248-006-9028-x
Williams, T. J., Wilkins, D., Long, E., Evans, F., DeMaere, M. Z., Raftery, M. J., et al. (2013). The role of planktonic Flavobacteria in processing algal organic matter in coastal East Antarctica revealed using metagenomics and metaproteomics. Environ. Microbiol. 15, 1302–1317. doi: 10.1111/1462-2920.12017
Worden, A. Z., Follows, M. J., Giovannoni, S. J., Wilken, S., Zimmerman, A. E., and Keeling, P. J. (2015). Rethinking the marine carbon cycle: factoring in the multifarious lifestyles of microbes. Science 347:1257594. doi: 10.1126/science.1257594
Xing, P., Li, H., Liu, Q., and Zheng, J. (2012). Composition of the archaeal community involved in methane production during the decomposition of Microcystis blooms in the laboratory. Can. J. Microbiol. 58, 1153–1158. doi: 10.1139/w2012-097
Yamasaki, Y., Nagasoe, S., Matsubara, T., Shikata, T., Shimasaki, Y., Oshima, Y., et al. (2007). Allelopathic interactions between the bacillariophyte Skeletonema costatum and the raphidophyte Heterosigma akashiwo. Mar. Ecol. Prog. Ser. 339, 83–92. doi: 10.3354/meps339083
Yambartsev, A., Perlin, M. A., Kovchegov, Y., Shulzhenko, N., Mine, K. L., Dong, X., et al. (2016). Unexpected links reflect the noise in networks. Biol. Direct 11:52. doi: 10.1186/s13062-016-0155-0
Yang, C., Wang, Q., Simon, P. N., Liu, J., Liu, L., Dai, X., et al. (2017). Distinct network interactions in particle-associated and free-living bacterial communities during a Microcystis aeruginosa bloom in a Plateau Lake. Front. Microbiol. 8:1202. doi: 10.3389/fmicb.2017.01202
Yang, C. Y., Li, Y., Zhou, Y. Y., Lei, X., Zheng, W., Tian, Y., et al. (2016). A comprehensive insight into functional profiles of free-living microbial community responses to a toxic Akashiwo sanguinea bloom. Sci. Rep. 6:34645. doi: 10.1038/srep34645
Zheng, T. L. (ed.). (2011). The Microbial Control of Harmful Algal Bloom. Xiamen: Xiamen University.
Zheng, Y. M., Cao, P., Fu, B., Hughes, J. M., and He, J. Z. (2013). Ecological drivers of biogeographic patterns of soil archaeal community. PLoS One 8:e63375. doi: 10.1371/journal.pone.0063375
Zhou, J., Chen, G. F., Zhu, X. S., Chen, L., and Cai, Z. H. (2014). A review of the relationship between algae and bacteria in harmful algal blooms. Acta Ecol. Sin. 34, 269–281.
Zhou, J., Deng, Y., Luo, F., He, Z., Tu, Q., and Zhi, X. (2010). Functional molecular ecological networks. mBio 1:e00169-10. doi: 10.1128/mBio.00169-10
Zhou, J., Lin, G. H., and Cai, Z. H. (2016a). The roles of microbes in matter cycles in phycosphere niche. Chin. J. Appl. Ecol. 27, 2708–2716. doi: 10.13287/j.1001-9332.201608.007
Keywords: microbial community, algal bloom, dynamic process, network interaction, ecological function
Citation: Zhou J, Richlen ML, Sehein TR, Kulis DM, Anderson DM and Cai Z (2018) Microbial Community Structure and Associations During a Marine Dinoflagellate Bloom. Front. Microbiol. 9:1201. doi: 10.3389/fmicb.2018.01201
Received: 28 September 2017; Accepted: 16 May 2018;
Published: 06 June 2018.
Edited by:
Debora Iglesias-Rodriguez, University of California, Santa Barbara, United StatesReviewed by:
Peter Croot, National University of Ireland Galway, IrelandDouwe Maat, Royal Netherlands Institute for Sea Research (NIOZ), Netherlands
Copyright © 2018 Zhou, Richlen, Sehein, Kulis, Anderson and Cai. This is an open-access article distributed under the terms of the Creative Commons Attribution License (CC BY). The use, distribution or reproduction in other forums is permitted, provided the original author(s) and the copyright owner are credited and that the original publication in this journal is cited, in accordance with accepted academic practice. No use, distribution or reproduction is permitted which does not comply with these terms.
*Correspondence: Zhonghua Cai, Y2FpemhAc3oudHNpbmdodWEuZWR1LmNu
†These authors have contributed equally to this work.