- 1Department of Chemistry, City University of Hong Kong, Kowloon Tong, Hong Kong
- 2Croucher Institute for Environmental Sciences, Department of Biology, Hong Kong Baptist University, Kowloon Tong, Hong Kong
The gut microbiome is a dynamic ecosystem formed by thousands of diverse bacterial species. This bacterial diversity is acquired early in life and shaped over time by a combination of multiple factors, including dietary exposure to distinct nutrients and xenobiotics. Alterations of the gut microbiota composition and associated metabolic activities in the gut are linked to various immune and metabolic diseases. The microbiota could potentially interact with xenobiotics in the gut environment as a result of their board enzymatic capacities and thereby affect the bioavailability and toxicity of the xenobiotics in enterohepatic circulation. Consequently, microbiome-xenobiotic interactions might affect host health. Here, we aimed to investigate the effects of dietary perfluorooctane sulfonic acid (PFOS) exposure on gut microbiota in adult mice and examine the induced changes in animal metabolic functions. In mice exposed to dietary PFOS for 7 weeks, body PFOS and lipid contents were measured, and to elucidate the effects of PFOS exposure, the metabolic functions of the animals were assessed using oral glucose-tolerance test and intraperitoneal insulin-tolerance and pyruvate-tolerance tests; moreover, on Day 50, cecal bacterial DNA was isolated and subject to 16S rDNA sequencing. Our results demonstrated that PFOS exposure caused metabolic disturbances in the animals, particularly in lipid and glucose metabolism, but did not substantially affect the diversity of gut bacterial species. However, marked modulations were detected in the abundance of metabolism-associated bacteria belonging to the phyla Firmicutes, Bacteroidetes, Proteobacteria, and Cyanobacteria, including, at different taxonomic levels, Turicibacteraceae, Turicibacterales, Turicibacter, Dehalobacteriaceae, Dehalobacterium, Allobaculum, Bacteroides acidifaciens, Alphaproteobacteria, and 4Cod-2/YS2. The results of PICRUSt analysis further indicated that PFOS exposure perturbed gut metabolism, inducing notable changes in the metabolism of amino acids (arginine, proline, lysine), methane, and a short-chain fatty acid (butanoate), all of which are metabolites widely recognized to be associated with inflammation and metabolic functions. Collectively, our study findings provide key information regarding the biological relevance of microbiome–xenobiotic interactions associated with the ecology of gut microbiota and animal energy metabolism.
Introduction
The prevalence of non-communicable diseases (NCDs) is rapidly increasing, with cardiovascular diseases and diabetes being at the top of this list of NCDs, and multiple risk factors are widely recognized to be responsible for the increased incidences. Recently, the cumulative incidence of certain NCDs has been correlated to exposure to environmental chemicals (Gluckman et al., 2010). The past decades have witnessed the production of >150,000 synthetic chemicals, with approximately 2000 new chemicals being produced annually (Judson et al., 2009), and these heterogeneous chemical substances have been used for generating diverse industrial, agricultural, and commercial products. However, the release of these substances into the environment has adversely affected ecological and animal health. Depending on their chemical properties, these chemical substances have become dispersed in distinct environmental compartments and have contaminated food and water supplies. Retrospective analysis has revealed that exposure to various classes of environmental chemicals can occur through distinct routes and processes, including inhalation, dietary intake, and skin contact; this has resulted in the bodily accumulation of different environmental chemicals in the general population worldwide (Centers for Disease Control and Prevention, 2009), which indicates direct interactions of the exogenous chemicals within our body system.
The 2017 WHO global report on diabetes showed that >422 million adults were diagnosed with diabetes, underpinning the high prevalence of the disease and associated metabolic syndromes. People with a susceptible genetic background are predisposed to developing these diseases, and consumption of calorically dense diets and physical inactivity are the major risk factors associated with the disease development. However, these factors cannot account for the widespread prevalence of metabolic diseases in recent years, and thus additional investigation is required to reveal the pathogenesis of these diseases. Recently, scientific research has been focused on other potential risk factors that might disrupt body energy homeostasis, and considerable attention has been attracted by the roles of (1) gastrointestinal microbiota and (2) endocrine-disrupting chemicals (EDCs) as contributing factors.
The animal gut microbiome is a dynamic ecosystem formed by thousands of distinct bacterial species (Qin et al., 2010), and the remarkable metabolic activity in the gut environment is driven through a complex symbiotic interaction between these species. The gut bacterial diversity is shaped over time, with the complexity increasing due to the combined effect of multiple factors (such as genotype, diet composition, antibiotic therapy, and environmental exposure to xenobiotics) (Spor et al., 2011; Yatsunenko et al., 2012). The gut microbiota can potentially interact with environmental chemicals by altering the processes of absorption, disposition, metabolism, and excretion. Accordingly, gut bacteria have been widely reported to exhibit board ability to metabolize various environmental chemicals by using enzyme families (e.g., azoreductases, β-glucuronidases, β-lyases, nitroreductases, sulfatases) to catalyze diverse chemical reactions (e.g., reduction, hydrolysis, dehydroxylation, deacetylation, dinitration, deconjugation, demethylation) (Eriksson and Gustafsson, 1970; Williams et al., 1970; Bakke and Gustafsson, 1986; Rafii et al., 1990; Rafil et al., 1991; Roldan et al., 2008). A recent register of gut microbial biocatalytic reactions on xenobiotics listed 529 microorganisms that affect >1369 compounds (Gao et al., 2010); the study highlighted the capacity of gut microbes to transform diverse types of environmental chemicals. Notably, emerging evidence has indicated an association between body burden of environmental chemicals and gut microbial communities in the development of metabolic diseases (Alonso-Magdalena et al., 2011). In 2011, the U.S. National Toxicological Program studied the roles of environmental chemicals in the development of diabetes and obesity and reported positive correlations between EDC exposure and disease prevalence; the analysis prioritized the ten most predicted positive compounds across distinct biological processes: flusilazole, forchlorfenuron, d-cis/trans-allethrin, fentin, fludioxonil, niclosamide, prallethrin, thidiazuron, (Z,E)-fenpyroximate, and perfluorooctane sulfonic acid (PFOS). Among these chemicals, PFOS was listed as one of the risk factors for the development of metabolic diseases in the European research project OBELIX.
Alterations of gut microbiota composition are reported to be associated with various immune and metabolic diseases (e.g., inflammatory bowel disease, obesity, diabetes) (Cummings et al., 2003; Ley et al., 2006; Qin et al., 2012). However, few previous studies have investigated the interactions between environmental chemicals and gut microbiota and their toxicological relevance to the development of metabolic diseases. Here, we used a mouse model to assess the metabolic impact of dietary PFOS exposure. Physiological experiments and 16S rDNA metagenomic analyses were conducted to investigate the association among PFOS exposure, changes in gut bacterial community, and metabolic function.
Materials and Methods
Experimental Animals and Chemicals
Female CD-1 mice (6–8 weeks old), obtained from the Animal Unit of the University of Hong Kong, were housed in polypropylene cages containing sterilized bedding, maintained under a controlled temperature (23 ± 1°C, ambient temperature) and 12/12-h light/dark cycle, and provided ad libitum access to standard chow (LabDiet, 5001 Rodents Diet) and water (in glass bottles). The animal handling protocol was approved by the Committee on the Use of Human and Animal Subjects of the Hong Kong Baptist University (Permit no. 261812), in accordance with the Guidelines and Regulations of Department of Health, the Government of Hong Kong Special Administrative Region. The mice were acclimatized for 1 week before the PFOS-exposure experiments and then randomly divided into three groups (control, AC; low-dose PFOS, AL; high-dose PFOS, AH; at least four mice/group). PFOS (98% pure, Sigma-Aldrich) was dissolved in dimethyl sulfoxide (DMSO, Sigma-Aldrich) before mixing with corn oil; the final concentration of DMSO was <0.05% in all groups. The PFOS-exposure groups were weighed using an electronic balance (Shimadzu, Tokyo, Japan) and administered, every morning by oral gavage, 0.3 (AL) or 3 μg/g/day (AH) PFOS in corn oil for 7 weeks. The exposure doses were selected as described in our previous study (Lai et al., 2017), in reference with the human tolerable daily intake of PFOS established by the Scientific Panel on Contaminants in the Food Chain (European Food Safety Authority, 2008). The dose-range corresponded to the general population and occupational exposure levels. The control group (AC) received corn oil mixed with DMSO (0.05%).
Animals were sacrificed on Day 50 by cervical dislocation and cecal samples were collected. Blood samples were collected through cardiocentesis, and blood serum was prepared by centrifuging the samples at 3000 ×g for 15 min. The serum and the weighed liver samples were stored at -20°C and then used for triglyceride (TG) and PFOS measurements.
Serum and Liver TGs
Serum and liver TG levels were quantified using the method described in our previous study (Wan et al., 2012) and a TG assay kit (Cayman, United States). Briefly, tissue samples were homogenized in chloroform:methanol (2:1) solution and then 0.05% sulfuric acid was added for phase separation. The aqueous phase was discarded, and the organic phase was collected and blow-dried under nitrogen gas at room temperature. The pellet was reconstituted in deionized water for 30 min at 37°C and then used for TG measurement.
Chemicals and Instrumental Analysis for PFOS
We used a mass-labeled mixed standard solution for perfluorinated compounds (Product code: MPFAC-MXA; Lot number: MPFACMXA0714; >98% pure) from Wellington Laboratories (ON, Canada). Samples were extracted and analyzed as previously described (Wan et al., 2013). Briefly, each tissue sample was mixed with 2 ng of internal standard, 1 mL of 0.5 M tetrabutylammonium hydroxide solution, 2 mL of 0.25 M sodium carbonate buffer, and 5 mL of methyl tert-butyl ether, and this was followed by mixing in a reciprocating shaker (HS 501 digital shaker, Janke and Kunkel IKA Labortechnik) at 250 rpm for 20 min. The organic and aqueous layers were separated, and the organic phase was collected, and the extraction procedure was repeated and all organic phases were pooled. The solution was blow-dried under nitrogen gas (N2 ≥ 99.995%, Hong Kong Oxygen) in a nitrogen evaporator (N-EVAP112, Organomation Associates, Inc., MA, United States) and re-dissolved in 40% acetonitrile/60% 10 mM of ammonium acetate in Milli-Q water. An Agilent 1200 series liquid-chromatography system (Waldbronn, Germany) was used for PFOS detection. Chromatographic separation was performed using an Agilent ZORBAX Eclipse Plus C8 Narrow Bore guard column and an Agilent ZORBAX Eclipse Plus C8 Narrow Bore column. Tandem mass detection was conducted using an Agilent 6410B Triple Quadrupole mass spectrometer equipped with an Agilent Masshunter Workstation (version B.02.01) and an electrospray ionization source. The values of matrix recoveries were all 99%.
Physiological Analysis
Oral glucose-tolerance test (OGTT) and intraperitoneal (i.p.) insulin-tolerance test (ITT) and pyruvate-tolerance test (PTT) were conducted on Day 50 on control and PFOS-exposed mice, as described in our previous study (Wan et al., 2014). Briefly, for OGTT, 16-h-fasted mice were administered glucose (2 mg/g body weight); for PTT, 16-h-fasted mice received an i.p. injection of sodium pyruvate (2 mg/g of body weight); and for ITT, 12-h-fasted mice received an i.p. injection of insulin (1 IU/kg body weight). For measuring blood glucose, blood samples were collected by means of tail prick at 0, 15, 30, 60, and 120 min. Area under the curve (AUC) values for OGTT, PTT, and ITT were calculated to evaluate glucose tolerance, the total glucose synthesized from pyruvate, and insulin sensitivity, respectively.
16S rDNA Metagenomic Sequencing
Cecal bacterial DNA was isolated using DNeasy Blood & Tissue Kit (Qiagen), according to manufacturer instructions, and 30 ng of qualified DNA was used to construct the library for metagenomic sequencing. V3-V4 Dual-index Fusion PCR Primer Cocktail and PCR Master Mix were used to amplify the V3-V4 regions of 16S rDNA, and the PCR product was purified using Ampure XP beads (Agencourt). The library was quantified using real-time quantitative PCR and was quality-checked using an Agilent 2100 bioanalyzer instrument (EvaGreen). The normalized library was subject to Illumina MiSeq sequencing for 250-bp paired-end sequencing; sequencing data have been deposited in the NCBI Sequence Read Archive (SRA)1, accession code SRP156864.
Bioinformatics Analysis
To obtain accurate and reliable results in bioinformatics analyses, we used a dual-indexing approach (Fadrosh et al., 2014). Raw data were filtered to eliminate adapters and low-quality reads by using an in-house procedure; this included truncation of sequencing reads, based on the phred algorithm: the removed sequencing reads (1) were <75% of their original length and contained their paired reads; (2) included adapter sequences (default parameter: 15 bases overlapped by reads); (3) contained an ambiguous base (N base) and their paired reads; and (4) exhibited low complexity (default: reads containing the same base in 10 consecutive positions). For pooling the library with barcoded samples, the clean reads were assigned to corresponding samples by allowing 0 base mismatch to barcode sequences with in-house scripts. The data-processing results are listed in Supplementary Table S1. At least 2 Mbp of clean data were obtained from each sample, and the read-usage ratio was >70%. Paired-end reads featuring overlaps were merged to tags that were clustered to operational taxonomic units (OTUs) by using the scripts of USEARCH software (v7.0.1090) (Edgar, 2013). All tags were clustered to OTUs at 97% sequence similarity. Taxonomic ranks were assigned to OTU representative sequences by using Ribosomal Database Project (RDP) Naïve Bayesian Classifier v.2.2. Alpha-diversity analysis and the screening for different species were based on OTU and taxonomic ranks. Phylogenetic investigation of communities by reconstruction of unobserved states (PICRUSt) analysis was employed to predict functional capabilities by using our sequencing data (Langille et al., 2013).
Statistical Analysis
Data are presented as means ± SEM. Differences between treatment and respective control groups were analyzed using Student’s t-test; p < 0.05 was considered significant. Analyses were conducted using SigmaStat for Windows.
Results
Effect of Chronic Dietary PFOS Exposure on Liver Weight and TG Content
Upon completion of the PFOS-exposure study, on Day 50, the mean body weights were increased in the control (AC) group and the low-dose (AL) and high-dose (AH) PFOS-treatment groups, and the weights did not differ in a statistically significant manner among the groups. However, in the AH group, the liver was enlarged and the absolute liver weight was increased (Figure 1A), as was the ratio of liver weight to body weight (Figure 1B). The liver appeared yellowish in the AH-group mice (data not shown), which might be associated with lipid accumulation. Accordingly, measurement of liver TG content revealed a significant increase in the PFOS-exposed mice (Figure 1C), and the liver TG content was positively correlated with the increase in absolute liver weight. Intriguingly, serum TG content in the AH group was significantly decreased relative to control (Figure 1D). Table 1 shows the PFOS levels in both the liver and the serum in control and treatment groups; the accumulated PFOS levels were increased in a PFOS dose-dependent manner.
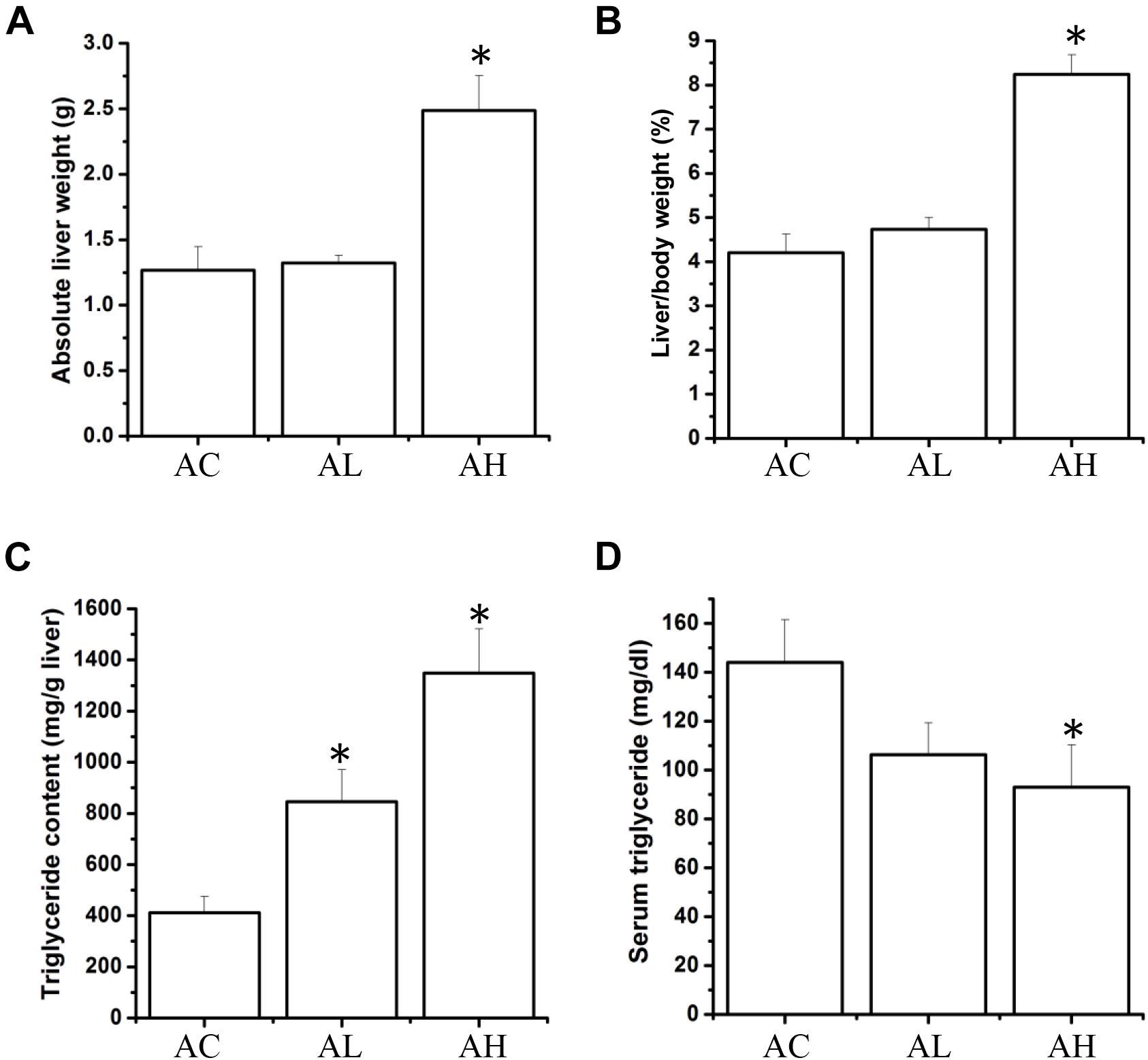
FIGURE 1. Effect of 7-week dietary PFOS exposure on liver weight and triglyceride content in mice. (A) Absolute liver weight, (B) liver index, (C) liver triglyceride level, and (D) serum triglyceride level were measured on Day 50 after PFOS treatment. Data are presented as means ± SD; ∗p < 0.05 versus control group. AC, control; AL, 0.3 μg/g body weight/day; AH, 3 μg/g body weight/day.
OGTT, ITT, and PTT
On Day 50, mice from the control and low- and high-dose PFOS-exposure groups were prepared for testing glucose metabolism and insulin function. OGTT results revealed that whereas the low-dose PFOS treatment did not significantly affect glucose tolerance (Figure 2A), the high-dose treatment elicited an earlier response in the reduction of blood glucose levels, at 15 and 30 min (p < 0.05), following glucose administration. The AUCs of OGTT were similar between the AC and AL groups, but the AUC of the AH group was significantly lower than that of the AC group (control).
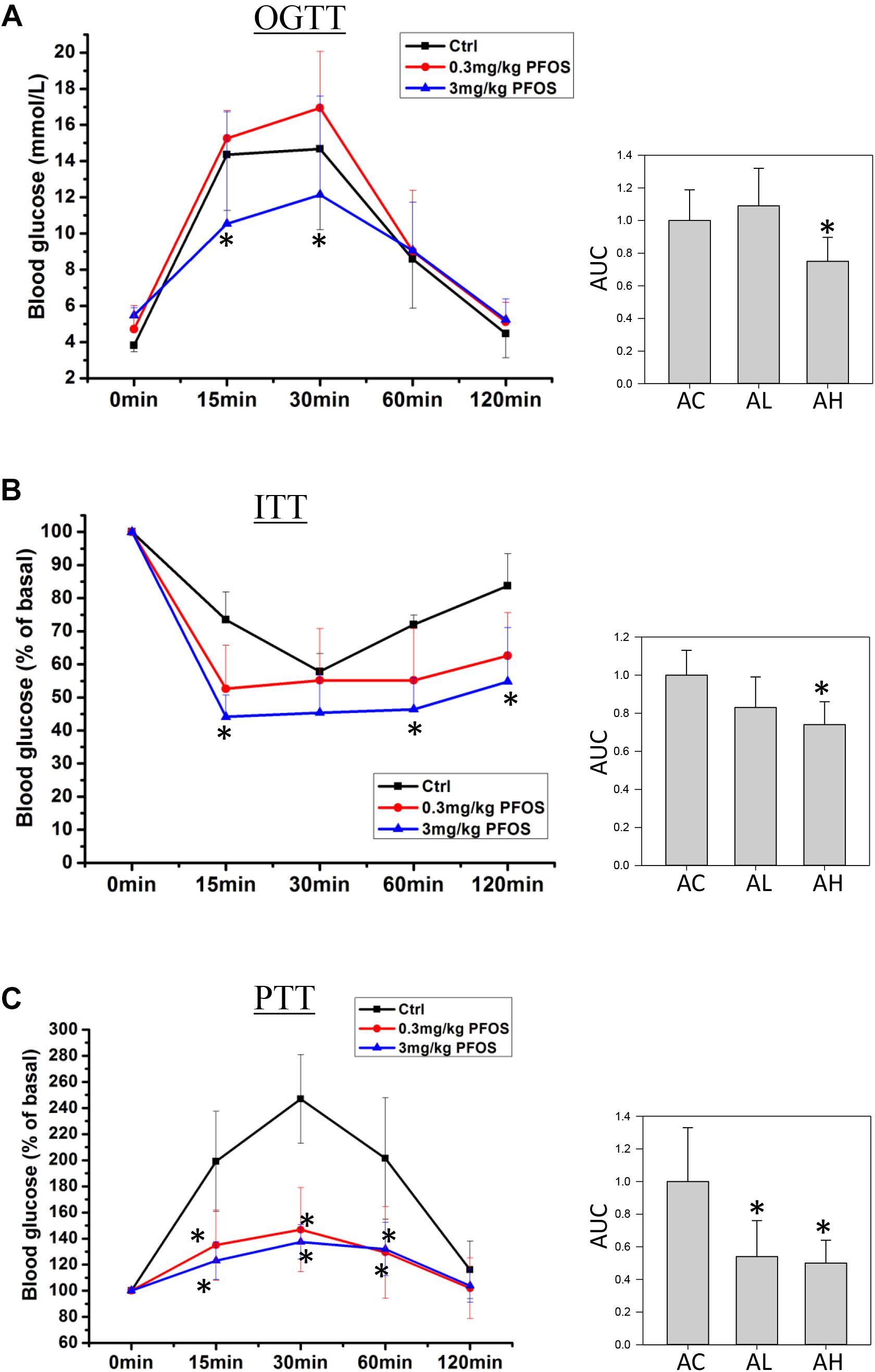
FIGURE 2. Effect of 7-week dietary PFOS exposure in mice, examined using (A) oral glucose-tolerance test (OGTT), (B) insulin-tolerance test (ITT), and (C) pyruvate-tolerance test (PTT). Left panels: changes in serum glucose levels against time in the assays; right panels: area under curve (AUC) values of the respective assays. Data are presented as means ± SD; ∗p < 0.05 versus control group. AC, control; AL, 0.3 μg/g body weight/day; AH, 3 μg/g body weight/day.
Next, ITT-based measurement of body insulin sensitivity revealed that the mice in the AC and AL groups showed similar rate and extent of responses (Figure 2B), but in the AH-group mice, plasma glucose after insulin treatment was significantly lower than that in the control group. Accordingly, the AUC of the AH group was significantly lower than that of the AC group.
Lastly, to measure the effect of PFOS exposure on gluconeogenesis, pyruvate (a gluconeogenic substrate) was administrated and the rate of pyruvate conversion to glucose was measured. The PTT results indicated that pyruvate conversion in the AL and AH groups was significantly decreased relative to that in the AC group (Figure 2C).
PFOS Exposure Exerted No Marked Effect on Gut Bacterial Species Diversity
To determine the changes in gut bacterial community caused by chronic dietary PFOS exposure, we performed metagenomic sequencing analysis on the V3-V4 regions of 16S rDNA; the DNA was collected from the ceca of mice in the AC, AL, and AH groups. In OTU analysis, we found that the degree of bacterial diversity was similar among the groups, and the predominant phyla included Firmicutes, Bacteroidetes, and Proteobacteria. In the sample AH4, the number of bacterial species was low (Table 2). Venn diagram analysis (Figure 3A) revealed that 395 OTUs were shared among the three groups. Alpha diversity was next applied for analyzing the complexity of species diversity in each sample by using several indices: observed species, Chao1, ACE, Shannon, and Simpson indices (Table 3). The rarefaction curves based on the observed species value and Chao1 and ACE data were used to evaluate the coverage of the sequencing. The result showed that the sequencing data were adequate for covering all the bacterial species in the community, which was reflected in the appearance of plateau regions in the curves from all the samples (Supplementary Figure S1). Moreover, comparison of the species diversity in the three groups revealed that the PFOS-exposure groups showed no significant differences in gut bacterial species diversity relative to the control group (Figure 3B).
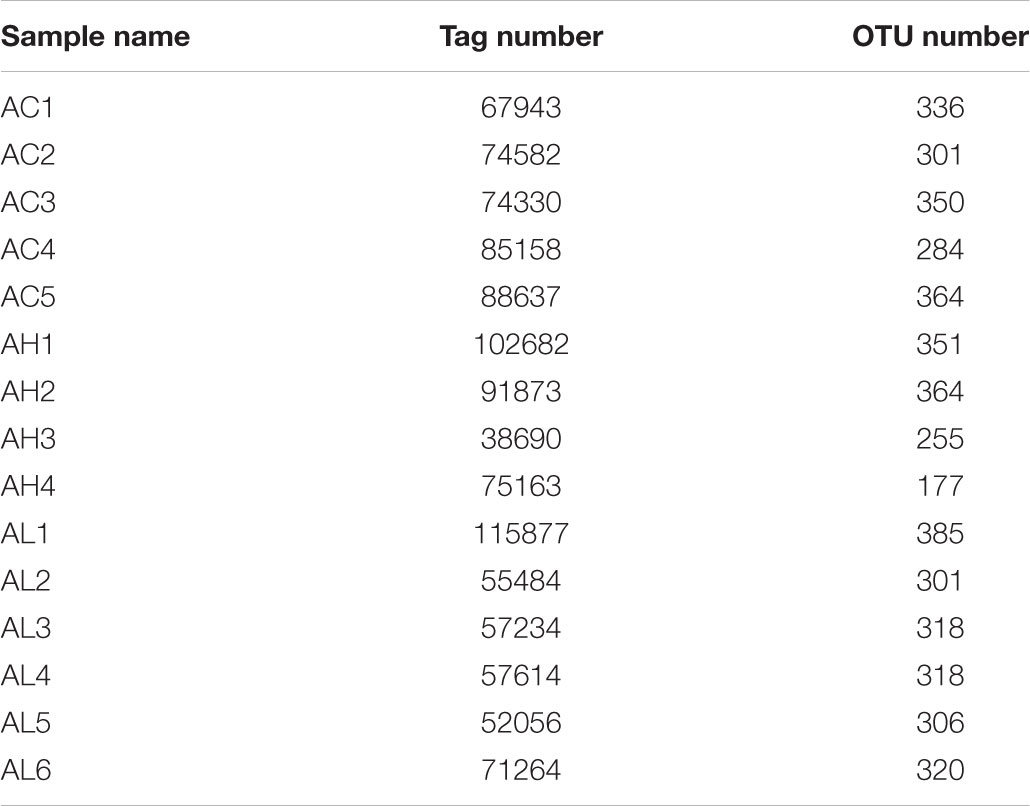
TABLE 2. Operational taxonomic unit (OTU) analysis on each sample from the control (AC), low-dose (AL), and high-dose (AH) PFOS-exposed groups.
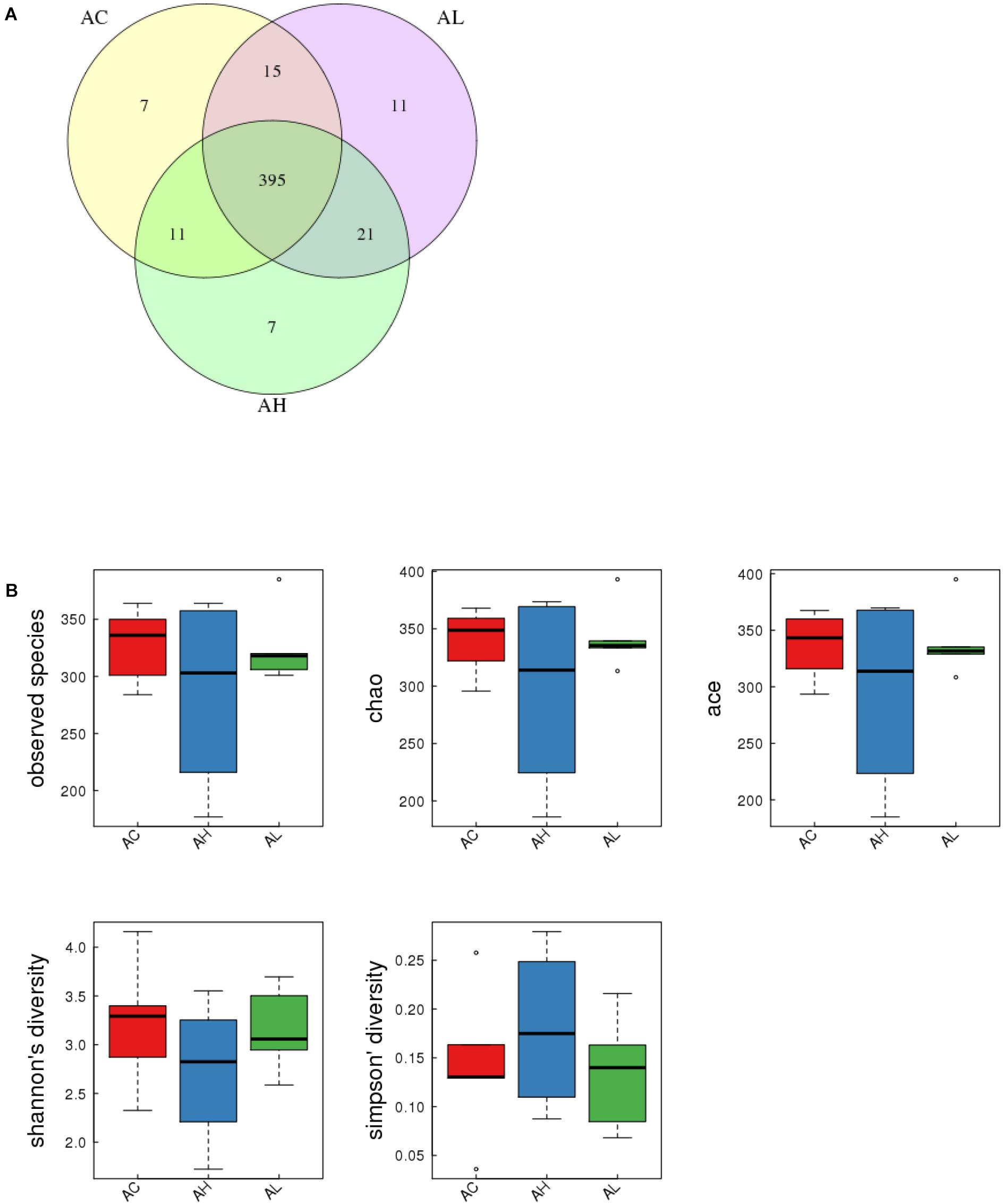
FIGURE 3. Effect of 7-week dietary PFOS exposure on gut bacterial structure in mice. (A) Comparison of operational taxonomic units (OTUs); different colors represent distinct groups: (i) control (AC), (ii) low-dose PFOS exposure (AL), and (iii) high-dose PFOS exposure (AH). The intersection represents the set of OTUs commonly present in the counterpart groups. Venn diagram was drawn using VennDiagram software R (v3.0.3). (B) Changes in observed species number, Chao1 index, Ace index, Shannon’s diversity, and Simpson’s diversity; the results suggest that dietary PFOS intake exerted no effect on the species diversity of the gut bacterial community.
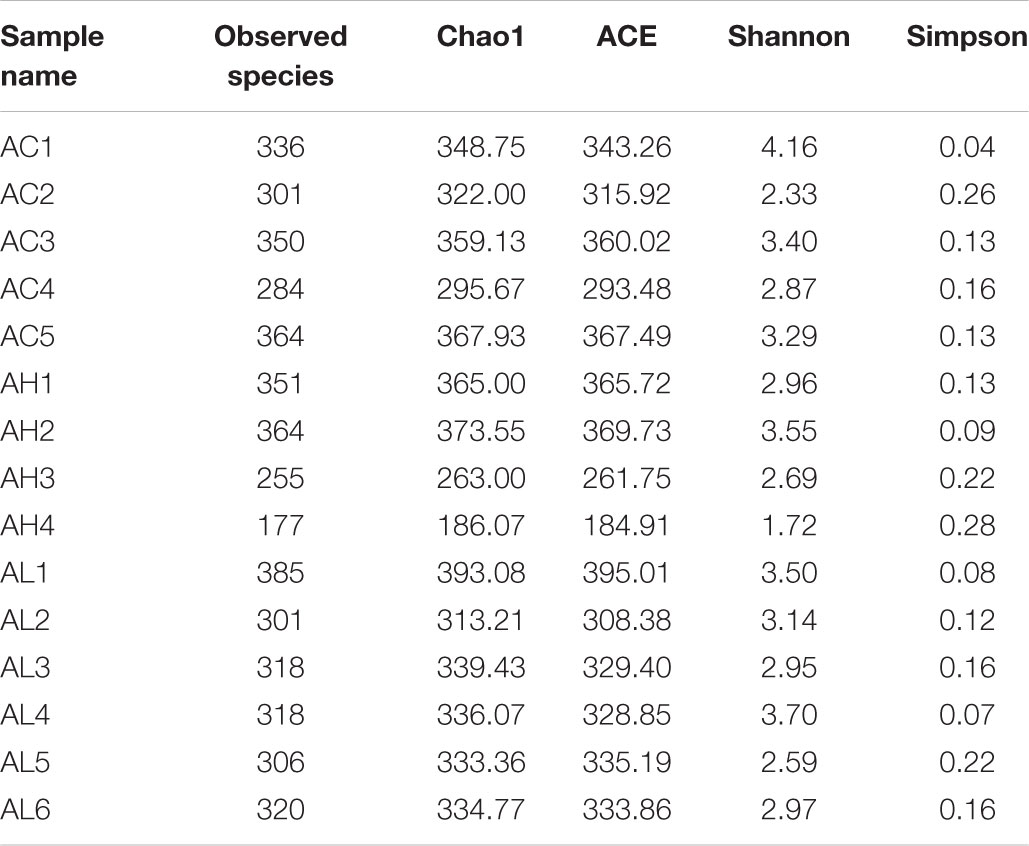
TABLE 3. Alpha diversity statistics in each samples from the control (AC), low-dose (AL), and high-dose (AH) PFOS-exposed groups.
PFOS Exposure Altered Gut Microbiome Community at Different Taxonomic Levels
We compared the composition of the cecal microbiota at distinct taxonomic levels after dietary PFOS exposure. In the AL and AH groups, PFOS exposure produced similar and consistent effects in terms of changes in the abundance of certain microbial communities (Table 4). These changes included a significant increase at the level of the order Turicibacterales (belonging to the phylum Firmicutes) and a reduction of the species Bacteroides acidifaciens (phylum Bacteroidetes) (Figure 4A and Table 4); the increase in Turicibacterales was mainly contributed by an induction of the family Turicibacteraceae and genus Turicibacter (Figure 4A and Table 4). However, the abundance of certain other microbes was increased in either the AL group or the AH group: In the AL group, we identified a significant induction of the phylum Cyanobacteria (Figure 4B and Table 4), increases in 4Cod-2 (Cyanobacteria-like lineage) and the class Alphaproteobacteria (phylum Proteobacteria) (Figure 4C and Table 4), and an induction of the order YS2 (phylum Cyanobacteria) (Figure 4D and Table 4). Conversely, in the AH group, we detected a significant reduction in the family Dehalobacteriaceae (phylum Firmicutes) (Figure 4E and Table 4) and the genus Dehalobacterium (Figure 4F and Table 4). Collectively, our results demonstrated that dietary PFOS exposure led to changes in the abundance of specific members of the gut-microbiome bacterial community.
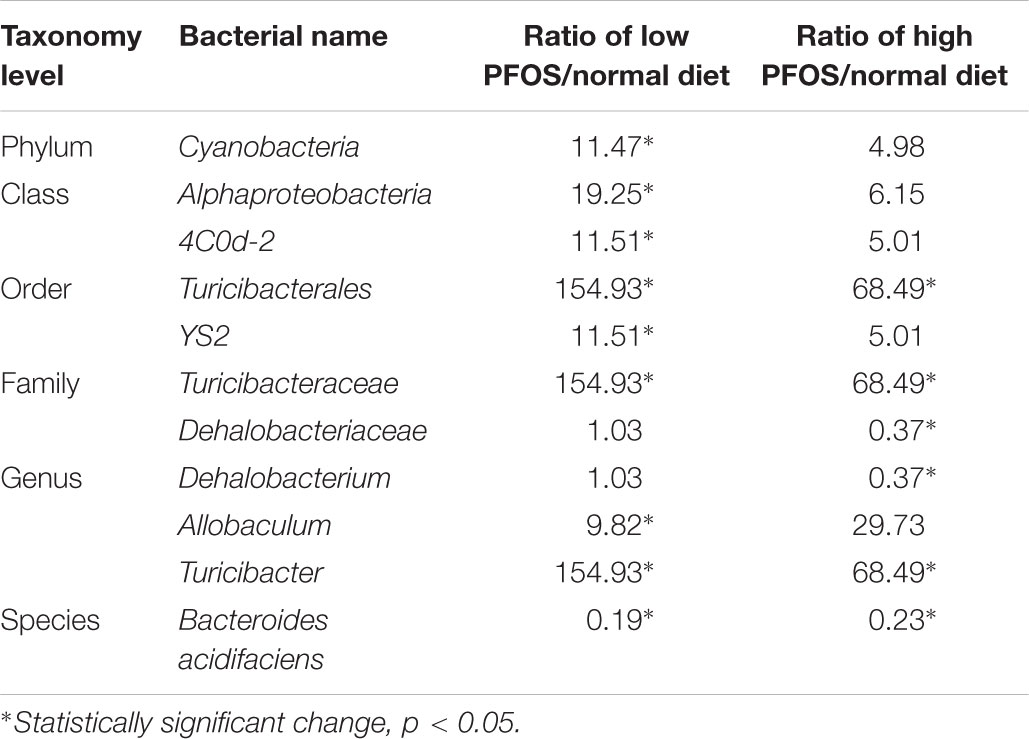
TABLE 4. Alteration of gut microbiome community at different taxonomy levels caused by dietary PFOS exposure.
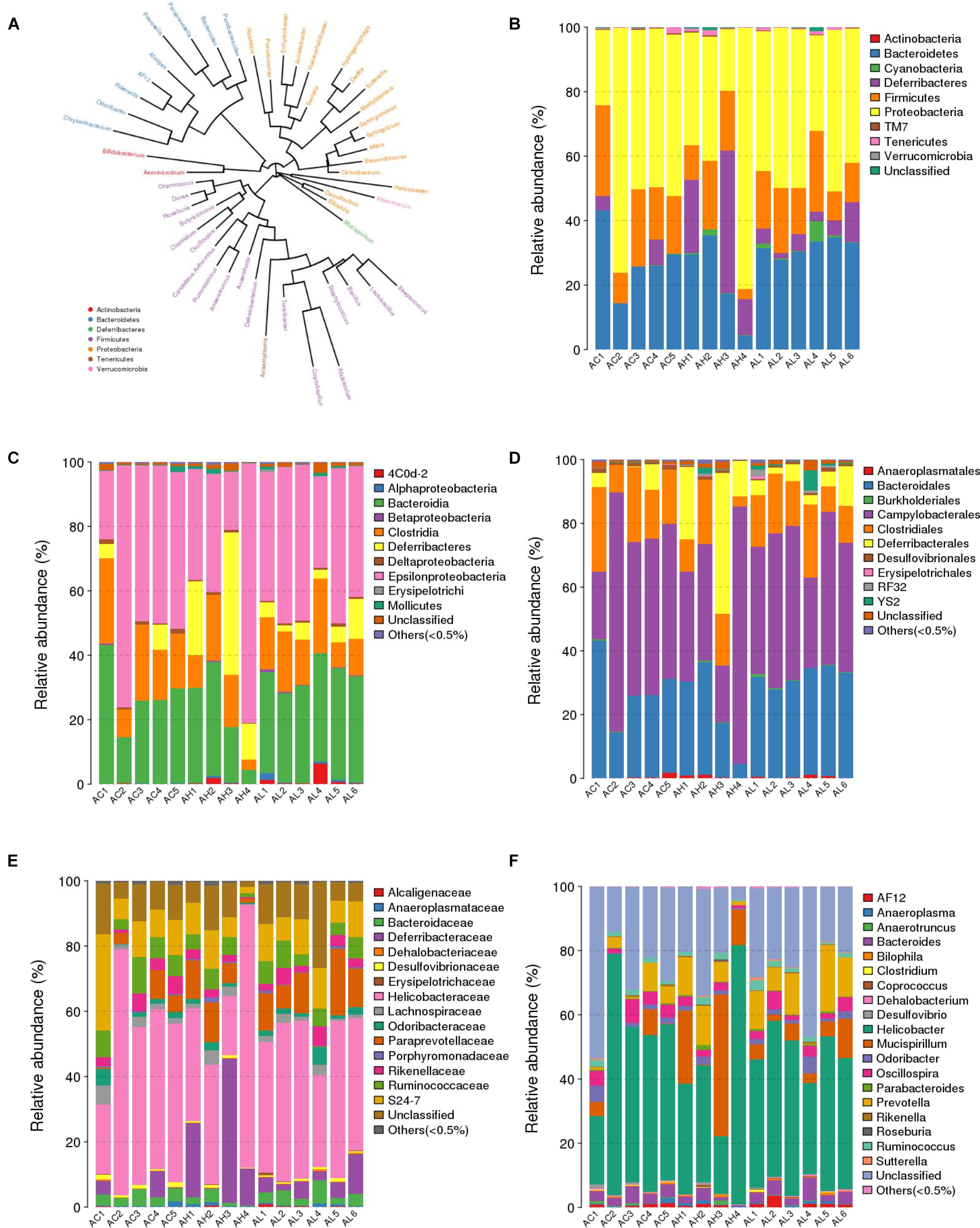
FIGURE 4. Effect of 7-week dietary PFOS exposure on gut microbiome community at distinct taxonomic levels. (A) Phylogenetic tree diagram at genus level. The same color indicates the same phylum. Taxonomic composition distributions in control (AC), low-dose PFOS-exposure (AL), and high-dose PFOS-exposure (AH) groups are shown at the levels of (B) phylum, (C) class, (D) order, (E) family, and (F) genus.
PFOS Exposure Altered Gut Metabolism
Phylogenetic investigation of communities by reconstruction of unobserved states analysis was conducted to predict the functional profiling of gut bacterial communities in response to PFOS exposure. Our result demonstrated that both high- and low-dose PFOS exposure led to significant suppression of arginine and proline metabolism (Table 5). Moreover, high-dose PFOS exposure significantly reduced lysine biosynthesis and methane metabolism but induced butanoate metabolism. Taken together, these data suggest that PFOS exposure resulted in the alteration of gut metabolism.
Discussion
Perfluorooctane sulfonic acid represents a risk factor for the development of metabolic diseases. A cross-sectional study conducted using data from the U.S. National Health and Nutrition Examination Survey 1999–2000 and 2003–2004, which examined 474 adolescents and 969 adults, reported that high plasma concentrations of PFOS were associated with increased blood insulin levels (Lin et al., 2009). In an evaluation of a potential link between plasma PFOS levels in 571 Taiwanese workers and the risk of diabetes, elevated levels of the chemical were correlated with impaired glucose homeostasis and increased prevalence of diabetes (Su et al., 2016). Furthermore, experimental studies in animal and cell models have demonstrated that PFOS exposure alters glucose and/or lipid metabolism through perturbations of pancreatic β-cells, adipocytes, and liver function, and in studies on adult-stage animals, chronic PFOS exposure has been found to reduce body weight and fat, accompanied by an increase in liver mass (Lau et al., 2007; Martin et al., 2007; Zhang et al., 2008; Cui et al., 2009). In the previous studies, most experiments were conducted using high-dose and acute PFOS exposure, and the experimental setting was thus unlike that in the real-world scenario, where low-dose and chronic exposure occurs. Moreover, limited information on the roles of gut microbes in PFOS-exposed animals is currently available. Therefore, our study was designed to address this knowledge gap. In the biochemical analysis of body TG content, our data revealed hepatomegaly and lipid accumulation in the liver of AH-group mice. The observation of liver enlargement and lipid accumulation agreed with the results of our previous study in which we used higher PFOS doses (5 and 10 μg/g body weight/day) but a shorter exposure time (21 days) (Wan et al., 2012). The hepatic lipid content might be increased because of the impairment of lipid catabolism and/or hepatic lipid export; the reduction in lipid catabolism probably occurred due to an inhibition of β-oxidation, whereas the reduction in lipid transport was related to a downregulation of apolipoprotein B (Wan et al., 2012). This correlation was further supported by the results obtained in this study, which showed a marked reduction in serum TG level in the AH group. The perturbation of lipid metabolism could have affected glucose metabolism and insulin secretion (Antinozzi et al., 1998). Thus, we conducted physiological tests to evaluate the impact of PFOS exposure on glucose tolerance, insulin sensitivity, and hepatic gluconeogenesis. Our results showed statistically significant changes in the responses measured in OGTT and ITT in the AH group. The findings of both assays suggested that the high-dose PFOS exposure induced insulin hypersensitivity in mice, with the evidence indicating an increased rate of reduction of plasma glucose levels and a decreased rate of gluconeogenesis. The observation is supported by a previous study showing that exposure of mice to PFOA (perfluorooctanoic acid, a member of the PFOS family) led to an elevation of insulin sensitivity (Yan et al., 2015). One of the recognized physiological functions of insulin is to promote hepatic fatty acid synthesis. The high liver lipid content in the AH group appeared to be the biological outcome of this effect. Dietary PFOS exposure would lead to direct interaction of the chemical with the bacteria in the gut environment, and, intriguingly, this physiological outcome correlated with the changes in gut bacterial diversity assessed using 16S metagenomic analysis.
In our previous study in mice, we showed that daily intake of an environmental obesogen, bisphenol A, altered the gut bacterial structure (Lai et al., 2016). The pattern of the alteration was similar to that in high-fat-diet-fed mice. This observation supports the notion that environmental chemicals can perturb gut bacterial communities. In this study, we extended our investigation to address the effects of PFOS exposure on gut bacterial structure. Our results showed that chronic PFOS exposure (0.3 and 3 μg/g body weight, for 49 days) exerted no effect on gut bacterial diversity in general. However, when we examined specific taxonomic levels, we found that both low-dose and high-dose of PFOS exposure altered the abundances of distinct gut bacteria belonging to the phyla Firmicutes, Bacteroidetes, Proteobacteria, and Cyanobacteria. Some of these changes were reported to be associated with the symptoms of metabolic perturbations. For instance, PFOS exposure caused a marked induction of microbes in the order Turicibacterales, which was due to the growth of the bacteria in the family Turicibacteraceae and genus Turicibacter, and this induction was stronger in the low-dose PFOS-exposure group than in the high-dose group. A previous study showed that Turicibacter was increased in mice fed with a high-cholesterol diet, as compared with the level in the control group (Dimova et al., 2017); the data implied that Turicibacter was increased in response to the abundance of dietary cholesterol. Intriguingly, the results of an epidemiological analysis showed a positive correlation between serum PFOS and total cholesterol levels (Nelson et al., 2010). Moreover, other studies suggested that an increase in Turicibacter was correlated with dietary fat content, although the observations were inconclusive (Everard et al., 2014; Zhong et al., 2015). Nonetheless, the increase we observed here in the abundance of Turicibacter was likely related to the perturbing effects of PFOS on lipid metabolism. Another study on host–microbiota relationship in glucose-metabolism disorder demonstrated a positive association with Turicibacteraceae (Lippert et al., 2017). This association was observed here in our OGTT, ITT, and PTT data, particularly in the case of high-dose PFOS exposure. Moreover, following low-dose PFOS exposure, the abundance of the genus Allobaculum was increased substantially. Allobaculum, a putative short-chain fatty-acid-producing bacterium, was suggested to contribute to insulin resistance and obesity (Zhang et al., 2015). Besides this increase of bacterial abundance, our data revealed a marked reduction in the proportion of B. acidifaciens in the gut of mice in the PFOS-exposed groups, as compared with the proportion in the control group. B. acidifaciens is one of the predominant bacterial species responsible for promoting IgA production in the large intestine and is a specific commensal bacterium associated with amelioration of metabolic disorders in mice (Yanagibashi et al., 2013; Yang et al., 2017). The abundance of B. acidifaciens was found to be negatively correlated with liver TG levels in mice fed with a high-fat diet (Blasco-Baque et al., 2017), which supports our data indicating negative correlation between the levels of hepatic and serum TG in PFOS-exposed mice. The family Dehalobacteriaceae showed reduced abundance only in the high-dose group, which was contributed by the decrease in Dehalobacterium. In a study of 416 twin-pairs from the Twins population, a low abundance of Dehalobacterium was associated with a high body mass index and high blood lipid levels (Fu et al., 2015). The involvement of the gut microbiota in multiple metabolic pathways in the host is widely recognized, and, accordingly, the results of our PICRUSt analysis showed that PFOS exposure altered the microbial community functions, specifically in the metabolism of amino acids (arginine, proline, lysine), methane, and a short-chain fatty acid (butanoate). Alternations in the metabolism of these metabolites in intestinal bacteria were reported to affect host physiology (Dai et al., 2011; Nicholson et al., 2012); changes in arginine and proline metabolism were associated with coronary heart disease (Feng et al., 2016), whereas perturbations of butyrate and methane metabolism were related to inflammatory diseases (Morgan et al., 2012) and Type I diabetes (Brown et al., 2011). Furthermore, the GPR-43 receptor for short-chain fatty acids was demonstrated to be linked with fat accumulation in the host (Kimura et al., 2013). Retrospectively, we can conclude that our data on the changes in the abundance of gut bacteria and their metabolism in the PFOS-exposed groups were associated with the observed metabolic perturbations.
To our knowledge, this the first integrative study to report the effects of PFOS exposure on animal metabolism and gut bacterial community. Our data revealed that chronic PFOS exposure at 3 μg/g body weight/day induced insulin sensitivity, which was associated with an increase in hepatic lipid content but a reduction in hepatic gluconeogenesis. The results of intestinal 16S metagenomic analysis demonstrated marked changes in the abundances of bacteria at distinct taxonomic levels, including Turicibacter, Allobaculum, B. acidifaciens, and Dehalobacteriaceae; changes in the abundance of these bacteria are known to be associated with perturbations of glucose and lipid metabolism. Collectively, the results from this study implied that dietary PFOS exposure affected not only the glucose and lipid metabolism of the host animals, but also caused disturbance to the gut bacterial ecosystem. However, certain questions remain unresolved, such as the mechanistic interactions between PFOS and gut microbes and the changes in the production of bacterial metabolites, and further investigation in necessary to clarify the potential correlation between these changes and PFOS exposure.
Data Availability
Sequence data generated in this study have been deposited in the NCBI Sequence Read Archive (SRA) (http://www.ncbi.nlm.nih.gov/sra); accession code: SRP156864.
Author Contributions
KL participated in metagenomic sequencings, analyzed the data, and drafted the manuscript. HW, CL, and RL carried out the animal works and sample preparation. AW was involved in chemical analysis. AN carried out the bioinformatic data analysis. CW conceived the idea, formulated the hypothesis, and drafted the manuscript.
Funding
This work was supported by the Strategic Research Fund to CW (Hong Kong Baptist University) (RC-ICRS/17-18/01).
Conflict of Interest Statement
The authors declare that the research was conducted in the absence of any commercial or financial relationships that could be construed as a potential conflict of interest.
Supplementary Material
The Supplementary Material for this article can be found online at: https://www.frontiersin.org/articles/10.3389/fmicb.2018.02552/full#supplementary-material
FIGURE S1 | Rarefaction curves based on Chao1 index, Ace index, and observed species values, showing that the data volume covered all species in the gut bacterial community.
TABLE S1 | The sequencing statistic of metagenomics sequencing.
Footnotes
References
Alonso-Magdalena, P., Quesada, I., and Nadal, A. (2011). Endocrine disruptors in the etiology of type 2 diabetes mellitus. Nat. Rev. Endocrinol. 7, 346–353. doi: 10.1038/nrendo.2011.56
Antinozzi, P. A., Segall, L., Prentki, M., McGarry, J. D., and Newgard, C. B. (1998). Molecular or pharmacologic perturbation of the link between glucose and lipid metabolism is without effect on glucose-stimulated insulin secretion. A re-evaluation of the long-chain acyl-CoA hypothesis. J. Biol. Chem. 273, 16146–16154. doi: 10.1074/jbc.273.26.16146
Bakke, J. E., and Gustafsson, J. A. (1986). Role of intestinal flora in metabolism of agrochemicals conjugated with glutathione. Xenobiotica 16, 1047–1056. doi: 10.3109/00498258609038982
Blasco-Baque, V., Coupe, B., Fabre, A., Handgraaf, S., Gourdy, P., Arnal, J. F., et al. (2017). Associations between hepatic miRNA expression, liver triacylglycerols and gut microbiota during metabolic adaptation to high-fat diet in mice. Diabetologia 60, 690–700. doi: 10.1007/s00125-017-4209-3
Brown, C. T., Davis-Richardson, A. G., Giongo, A., Gano, K. A., Crabb, D. B., Mukherjee, N., et al. (2011). Gut microbiome metagenomics analysis suggests a functional model for the development of autoimmunity for type 1 diabetes. PLoS.One. 6:e25792. doi: 10.1371/journal.pone.0025792
Centers for Disease Control and Prevention. (2009). Fourth National Report on Human Exposure to Environmental Chemicals, Department of Health and Human Services. Atlanta, GA: Centers for Disease Control and Prevention.
Cui, L., Zhou, Q. F., Liao, C. Y., Fu, J. J., and Jiang, G. B. (2009). Studies on the toxicological effects of PFOA and PFOS on rats using histological observation and chemical analysis. Arch. Environ. Contam. Toxicol. 56, 338–349. doi: 10.1007/s00244-008-9194-6
Cummings, J. H., Macfarlane, G. T., and Macfarlane, S. (2003). Intestinal bacteria and ulcerative colitis. Curr. Issues Intest. Microbiol. 4, 9–20.
Dai, Z. L., Wu, G., and Zhu, W. Y. (2011). Amino acid metabolism in intestinal bacteria: links between gut ecology and host health. Front. Biosci. 16, 1768–1786. doi: 10.2741/3820
Dimova, L. G., Zlatkov, N., Verkade, H. J., Uhlin, B. E., and Tietge, U. J. F. (2017). High-cholesterol diet does not alter gut microbiota composition in mice. Nutr. Metab. 14:15. doi: 10.1186/s12986-017-0170-x
Edgar, R. C. (2013). UPARSE: highly accurate OTU sequences from microbial amplicon reads. Nat. Methods 10, 996–998. doi: 10.1038/nmeth.2604
Eriksson, H., and Gustafsson, J. A. (1970). Steroids in germfree and conventional rats. Sulpho- and glucuronohydrolase activities of caecal contents from conventional rats. Eur. J. Biochem. 13, 198–202. doi: 10.1111/j.1432-1033.1970.tb00919.x
European Food Safety Authority. (2008). Perfluorooctane sulfonate (PFOS), perfluorooctanic acid (PFOA) and their salts - scientific opinion of the panel on contaminants in the food chain. EFSA J. 653, 1–131.
Everard, A., Lazarevic, V., Gaia, N., Johansson, M., Stahlman, M., Backhed, F., et al. (2014). Microbiome of prebiotic-treated mice reveals novel targets involved in host response during obesity. ISME J. 8, 2116–2130. doi: 10.1038/ismej.2014.45
Fadrosh, D. W., Ma, B., Gajer, P., Sengamalay, N., Ott, S., Brotman, R. M., et al. (2014). An improved dual-indexing approach for multiplexed 16S rRNA gene sequencing on the Illumina MiSeq platform. Microbiome 2:6. doi: 10.1186/2049-2618-2-6
Feng, Q., Liu, Z., Zhong, S., Li, R., Xia, H., Jie, Z., et al. (2016). Integrated metabolomics and metagenomics analysis of plasma and urine identified microbial metabolites associated with coronary heart disease. Sci. Rep. 6:22525. doi: 10.1038/srep22525
Fu, J., Bonder, M. J., Cenit, M. C., Tigchelaar, E. F., Maatman, A., Dekens, J. A., et al. (2015). The gut microbiome contributes to a substantial proportion of the variation in blood lipids. Circ. Res. 117, 817–824. doi: 10.1161/CIRCRESAHA.115.306807
Gao, J., Ellis, L. B., and Wackett, L. P. (2010). The university of minnesota biocatalysis/biodegradation database: improving public access. Nucleic Acids Res. 38, D488–D491. doi: 10.1093/nar/gkp771
Gluckman, P. D., Hanson, M. A., and Mitchell, M. D. (2010). Developmental origins of health and disease: reducing the burden of chronic disease in the next generation. Genome Med. 2:14. doi: 10.1186/gm135
Judson, R., Richard, A., Dix, D. J., Houck, K., Martin, M., Kavlock, R., et al. (2009). The toxicity data landscape for environmental chemicals. Environ. Health Perspect. 117, 685–695. doi: 10.1289/ehp.0800168
Kimura, I., Ozawa, K., Inoue, D., Imamura, T., Kimura, K., Maeda, T., et al. (2013). The gut microbiota suppresses insulin-mediated fat accumulation via the short-chain fatty acid receptor GPR43. Nat. Commun. 4:1829. doi: 10.1038/ncomms2852
Lai, K. P., Chung, Y. T., Li, R., Wan, H. T., and Wong, C. K. (2016). Bisphenol A alters gut microbiome: comparative metagenomics analysis. Environ. Pollut. 218, 923–930. doi: 10.1016/j.envpol.2016.08.039
Lai, K. P., Lee, J. C., Wan, H. T., Li, J. W., Wong, A. Y., Chan, T. F., et al. (2017). Effects of in utero PFOS exposure on transcriptome, lipidome, and function of mouse testis. Environ. Sci. Technol. 51, 8782–8794. doi: 10.1021/acs.est.7b02102
Langille, M. G., Zaneveld, J., Caporaso, J. G., McDonald, D., Knights, D., Reyes, J. A., et al. (2013). Predictive functional profiling of microbial communities using 16S rRNA marker gene sequences. Nat. Biotechnol. 31, 814–821. doi: 10.1038/nbt.2676
Lau, C., Anitole, K., Hodes, C., Lai, D., Pfahles-Hutchens, A., and Seed, J. (2007). Perfluoroalkyl acids: a review of monitoring and toxicological findings. Toxicol. Sci. 99, 366–394. doi: 10.1093/toxsci/kfm128
Ley, R. E., Turnbaugh, P. J., Klein, S., and Gordon, J. I. (2006). Microbial ecology: human gut microbes associated with obesity. Nature 444, 1022–1023. doi: 10.1038/4441022a
Lin, C. Y., Chen, P. C., Lin, Y. C., and Lin, L. Y. (2009). Association among serum perfluoroalkyl chemicals, glucose homeostasis, and metabolic syndrome in adolescents and adults. Diabetes Care 32, 702–707. doi: 10.2337/dc08-1816
Lippert, K., Kedenko, L., Antonielli, L., Kedenko, I., Gemeier, C., Leitner, M., et al. (2017). Gut microbiota dysbiosis associated with glucose metabolism disorders and the metabolic syndrome in older adults. Benef. Microbes. 8, 545–556. doi: 10.3920/BM2016.0184
Martin, M. T., Brennan, R. J., Hu, W., Ayanoglu, E., Lau, C., Ren, H., et al. (2007). Toxicogenomic study of triazole fungicides and perfluoroalkyl acids in rat livers predicts toxicity and categorizes chemicals based on mechanisms of toxicity. Toxicol. Sci. 97, 595–613. doi: 10.1093/toxsci/kfm065
Morgan, X. C., Tickle, T. L., Sokol, H., Gevers, D., Devaney, K. L., Ward, D. V., et al. (2012). Dysfunction of the intestinal microbiome in inflammatory bowel disease and treatment. Genome Biol. 13:R79. doi: 10.1186/gb-2012-13-9-r79
Nelson, J. W., Hatch, E. E., and Webster, T. F. (2010). Exposure to polyfluoroalkyl chemicals and cholesterol, body weight, and insulin resistance in the general U.S. population. Environ. Health Perspect. 118, 197–202. doi: 10.1289/ehp.0901165
Nicholson, J. K., Holmes, E., Kinross, J., Burcelin, R., Gibson, G., Jia, W., et al. (2012). Host-gut microbiota metabolic interactions. Science 336, 1262–1267. doi: 10.1126/science.1223813
Qin, J., Li, R., Raes, J., Arumugam, M., Burgdorf, K. S., Manichanh, C., et al. (2010). A human gut microbial gene catalogue established by metagenomic sequencing. Nature 464, 59–65. doi: 10.1038/nature08821
Qin, J., Li, Y., Cai, Z., Li, S., Zhu, J., Zhang, F., et al. (2012). A metagenome-wide association study of gut microbiota in type 2 diabetes. Nature 490, 55–60. doi: 10.1038/nature11450
Rafii, F., Franklin, W., and Cerniglia, C. E. (1990). Azoreductase activity of anaerobic bacteria isolated from human intestinal microflora. Appl. Environ. Microbiol. 56, 2146–2151.
Rafil, F., Franklin, W., Heflich, R. H., and Cerniglia, C. E. (1991). Reduction of nitroaromatic compounds by anaerobic bacteria isolated from the human gastrointestinal tract. Appl. Environ. Microbiol. 57, 962–968.
Roldan, M. D., Perez-Reinado, E., Castillo, F., and Moreno-Vivian, C. (2008). Reduction of polynitroaromatic compounds: the bacterial nitroreductases. FEMS Microbiol. Rev. 32, 474–500. doi: 10.1111/j.1574-6976.2008.00107.x
Spor, A., Koren, O., and Ley, R. (2011). Unravelling the effects of the environment and host genotype on the gut microbiome. Nat. Rev. Microbiol. 9, 279–290. doi: 10.1038/nrmicro2540
Su, T. C., Kuo, C. C., Hwang, J. J., Lien, G. W., Chen, M. F., and Chen, P. C. (2016). Serum perfluorinated chemicals, glucose homeostasis and the risk of diabetes in working-aged Taiwanese adults. Environ. Int. 88, 15–22. doi: 10.1016/j.envint.2015.11.016
Wan, H. T., Leung, P. Y., Zhao, Y. G., Wei, X., Wong, M. H., and Wong, C. K. (2013). Blood plasma concentrations of endocrine disrupting chemicals in Hong Kong populations. J. Hazard. Mater. 261, 763–769. doi: 10.1016/j.jhazmat.2013.01.034
Wan, H. T., Zhao, Y. G., Leung, P. Y., and Wong, C. K. (2014). Perinatal exposure to perfluorooctane sulfonate affects glucose metabolism in adult offspring. PLoS.One. 9:e87137. doi: 10.1371/journal.pone.0087137
Wan, H. T., Zhao, Y. G., Wei, X., Hui, K. Y., Giesy, J. P., and Wong, C. K. (2012). PFOS-induced hepatic steatosis, the mechanistic actions on beta-oxidation and lipid transport. Biochim. Biophys. Acta 1820, 1092–1101. doi: 10.1016/j.bbagen.2012.03.010
Williams, J. R. Jr., Grantham, P. H., Marsh, H. H. III, Weisburger, J. H., and Weisburger, E. K. (1970). Participation of liver fractions and of intestinal bacteria in the metabolism of N-hydroxy-N-2-fluorenylacetamide in the rat. Biochem. Pharmacol. 19, 173–188. doi: 10.1016/0006-2952(70)90338-2
Yan, S., Zhang, H., Zheng, F., Sheng, N., Guo, X., and Dai, J. (2015). Perfluorooctanoic acid exposure for 28 days affects glucose homeostasis and induces insulin hypersensitivity in mice. Sci. Rep. 5:11029. doi: 10.1038/srep11029
Yanagibashi, T., Hosono, A., Oyama, A., Tsuda, M., Suzuki, A., Hachimura, S., et al. (2013). IgA production in the large intestine is modulated by a different mechanism than in the small intestine: Bacteroides acidifaciens promotes IgA production in the large intestine by inducing germinal center formation and increasing the number of IgA + B cells. Immunobiology 218, 645–651. doi: 10.1016/j.imbio.2012.07.033
Yang, J. Y., Lee, Y. S., Kim, Y., Lee, S. H., Ryu, S., Fukuda, S., et al. (2017). Gut commensal Bacteroides acidifaciens prevents obesity and improves insulin sensitivity in mice. Mucosal. Immunol. 10, 104–116. doi: 10.1038/mi.2016.42
Yatsunenko, T., Rey, F. E., Manary, M. J., Trehan, I., Dominguez-Bello, M. G., Contreras, M., et al. (2012). Human gut microbiome viewed across age and geography. Nature 486, 222–227. doi: 10.1038/nature11053
Zhang, H., Shi, Z., Liu, Y., Wei, Y., and Dai, J. (2008). Lipid homeostasis and oxidative stress in the liver of male rats exposed to perfluorododecanoic acid. Toxicol. Appl. Pharmacol. 227, 16–25. doi: 10.1016/j.taap.2007.09.026
Zhang, X., Zhao, Y., Xu, J., Xue, Z., Zhang, M., Pang, X., et al. (2015). Modulation of gut microbiota by berberine and metformin during the treatment of high-fat diet-induced obesity in rats. Sci. Rep. 5:14405. doi: 10.1038/srep14405
Keywords: gut microbiome, bacterial diversity, microbiome-xenobiotic interaction, PFOs, energy metabolism
Citation: Lai KP, Ng AH-M, Wan HT, Wong AY-M, Leung CC-T, Li R and Wong CK-C (2018) Dietary Exposure to the Environmental Chemical, PFOS on the Diversity of Gut Microbiota, Associated With the Development of Metabolic Syndrome. Front. Microbiol. 9:2552. doi: 10.3389/fmicb.2018.02552
Received: 22 June 2018; Accepted: 05 October 2018;
Published: 24 October 2018.
Edited by:
Hongsheng Liu, Liaoning University, ChinaReviewed by:
Alinne Castro, Universidade Católica Dom Bosco (UCDB), BrazilAndrea Barbarossa, Università degli Studi di Bologna, Italy
Copyright © 2018 Lai, Ng, Wan, Wong, Leung, Li and Wong. This is an open-access article distributed under the terms of the Creative Commons Attribution License (CC BY). The use, distribution or reproduction in other forums is permitted, provided the original author(s) and the copyright owner(s) are credited and that the original publication in this journal is cited, in accordance with accepted academic practice. No use, distribution or reproduction is permitted which does not comply with these terms.
*Correspondence: Chris Kong-Chu Wong, Y2tjd29uZ0Boa2J1LmVkdS5oaw==