- National Institute for Public Health and the Environment, Bilthoven, Netherlands
The increased availability of whole-genome-sequencing techniques generates a wealth of DNA data on numerous organisms, including foodborne pathogens such as Salmonella. However, how these data can be used to improve microbial risk assessment and understanding of Salmonella epidemiology remains a challenge. The aim of this study was to assess variability in in vitro virulence and genetic characteristics between and within different serovars. The phenotypic behavior of 59 strains of 32 different Salmonella enterica serovars from animal, human and food origin was assessed in an in vitro gastro-intestinal tract (GIT) system and they were analyzed for the presence of 233 putative virulence genes as markers for phenotypic prediction. The probability of in vitro infection, P(inf), defined as the fraction of infectious cells passing from inoculation to host cell invasion at the last stage of the GIT system, was interpreted as the in vitro virulence. Results showed that the (average) P(inf) of Salmonella serovars ranged from 5.3E-05 (S. Kedougou) to 5.2E-01 (S. Typhimurium). In general, a higher P(inf) on serovar level corresponded to higher reported human incidence from epidemiological reporting data. Of the 233 virulence genes investigated, only 101 showed variability in presence/absence among the strains. In vitro P(inf) was found to be positively associated with the presence of specific plasmid related virulence genes (mig-5, pef, rck, and spv). However, not all serovars with a relatively high P(inf), > 1E-02, could be linked with these specific genes. Moreover, some outbreak related strains (S. Heidelberg and S. Thompson) did not reveal this association with P(inf). No clear association with in vitro virulence P(inf) was identified when grouping serovars with the same virulence gene profile (virulence plasmid, Typhoid toxin, peg operon and stk operon). This study shows that the in vitro P(inf) variation among individual strains from the same serovar is larger than that found between serovars. Therefore, ranking P(inf) of S. enterica on serovar level alone, or in combination with a serovar specific virulence gene profile, cannot be recommended. The attribution of single biological phenomena to individual strains or serovars is not sufficient to improve the hazard characterization for S. enterica. Future microbial risk assessments, including virulence gene profiles, require a systematic approach linked to epidemiological studies rather than revealing differences in characteristics on serovar level alone.
Introduction
Recent years have seen a concerted effort in the scientific community and the food industry to analyze molecular data related to foodborne pathogens and understand their relevance for public health. The development in Next Generation Sequencing (NGS) techniques (e.g., Taboada et al., 2017) and algorithms (pipelines) for data analysis (e.g., Davis et al., 2015; Katz et al., 2017) has resulted in more precise and also quicker outbreak investigations (Gilchrist et al., 2015; Jackson et al., 2016), source attribution and genomic epidemiology (Franz et al., 2014, 2016). In addition, the International Life Science Institute (ILSI) has started several initiatives to share the views of different stakeholders in the application of NGS data in food safety management. For example, the “Microbiological Food Safety Task Force Expert Group: the use of NGS-translation into practice”1 and ILSI were engaged in a “Workshop on NG MRA (Microbiological Risk Assessment), integration of omics data into risk assessment.” This resulted in four position papers that are published (e.g., den Besten et al., 2017; Haddad et al., 2018). Most of these studies focus on surveillance and outbreak investigations. Research hardly addresses the application of omics data in microbial risk assessment (MRA). Some studies exist in which genomic analysis has led to novel insights in the ‘hazard identification’ step of the MRA framework. For example, Maury et al. (2016) identified novel virulence factors in Listeria monocytogenes by studying the genomes of several clinical strains. Pielaat et al. (2015) demonstrated the non-trivial computational complications when molecular data are used for MRA. Practical implications were illustrated in that paper with a case study: linking single nucleotide polymorphisms (SNP) data of Escherichia coli O157:H7 to phenotypic behavior.
Salmonella enterica serovars are among the major foodborne diarrhoeal disease agents worldwide (Havelaar et al., 2015). While some serovars (among more than 2500 in total) dominate the human disease incidence (i.e., Enteritidis and Typhimurium) others rarely cause disease but can be found in animals, food and/or the environment (European Food Safety Authority [EFSA] and European Centre for Disease Prevention and Control [ECDC], 2017). Although outbreaks associated with relatively rare serovars do occur (Brandwagt et al., 2018). Such an epidemiological situation of Salmonella serovars could be the reflection of differences in virulence, in exposure, or both. In this study the in vitro virulence as well as the genetic virulence profile of various Salmonella serovars were investigated. A total of 59 S. enterica strains (32 different serovars) were screened for the presence of 233 virulence genes of Salmonella. In addition, all strains were tested in an in vitro Gastro Intestinal Tract (GIT) model system to quantify infectivity by estimating a probability of infection (P(inf)) (Wijnands et al., 2017). Data analysis aimed to identify whether different Salmonella serovars have different phenotypic and/or genotypic characteristics; if different Salmonella strains of the same serovar share the same phenotypic and genotypic characteristics; whether the phenotypic result [P(inf)] for the different Salmonella strains can be explained by genotypic properties (presence/absence of genes); and to what extent the outcomes of these investigations can be related to surveillance data on human incidence.
Materials and Methods
A short description of the experimental set-up and data analysis are given in section “Gastrointestinal Passage” and “GIT System Data Analysis” below. Earlier, preliminary analysis were presented at the 9th International Conference on Predictive Modelling in Food (Pielaat et al., 2016). In that study, bacterial concentrations (counts per ml) were used for further calculations without the conversion of the dilutions in the sequential steps of the GIT system. In the present study, dilutions have been accounted for and a thorough procedure was used for quantifying the gastrointestinal passage system, including a Bayesian framework for statistical analysis is described in Wijnands et al. (2017). Additional aspects specific to the experimental set-up and data analysis of the Salmonella strains in the present study are given below.
Bacterial Strains
In total, 59 strains belonging to 32 different Salmonella serovars were used for the in vitro GIT experiments and NGS. Two additional strains of S. Enteritidis (broiler) were used for the NGS experiments (see Supplementary Appendix A). The strains were obtained from the National Institute for Public Health and the Environment (RIVM) in the Netherlands. The center for zoonosis and environmental microbiology (cZ&O) of the RIVM has build this Salmonella strain collection over the years from different research projects. The collection was now used to quantify variability among the strains in in vitro gastro intestinal tract characteristics in relation to genetic properties. Table 1 includes an overview of the various strain sources associated for the different serovars.
Gastrointestinal Passage (GIT)
The different stages of the in vitro GIT-model simulating the human gastrointestinal tract are shown in Figure 1 (Wijnands et al., 2017). The GIT system is sequential, meaning that the Salmonella culture is transferred from one stage (SGF) to the next (SIF, ATT, and INV) without intermediate culturing.
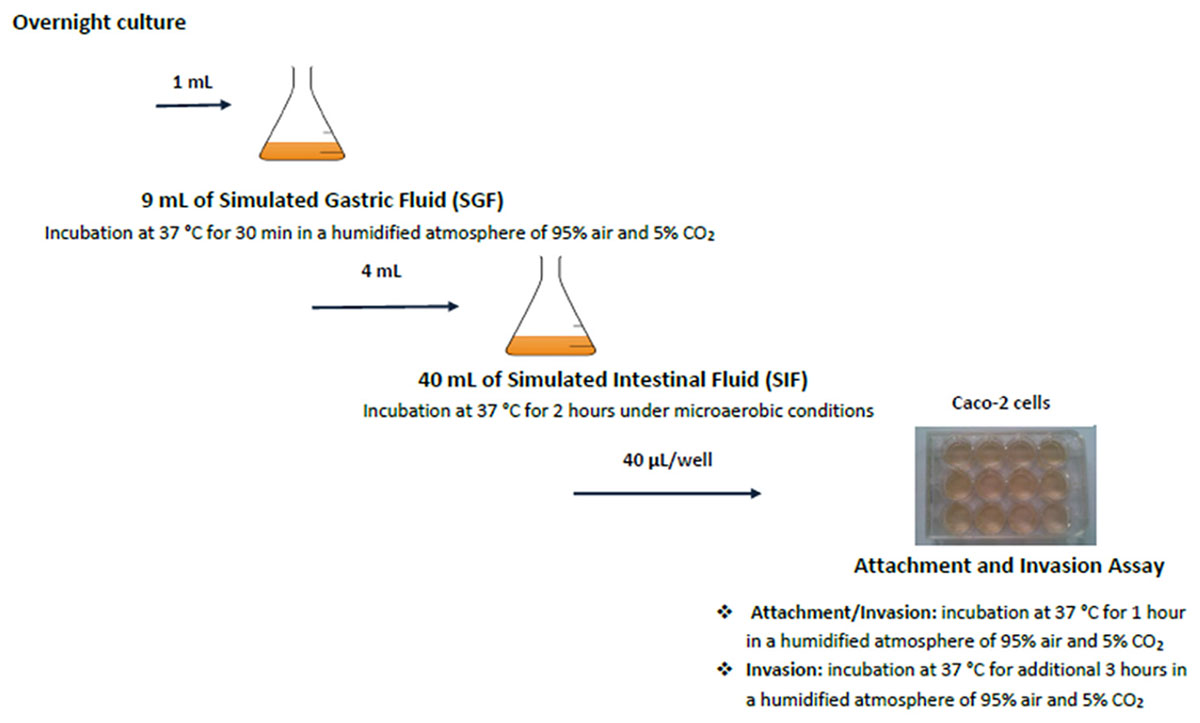
Figure 1. Schematic set-up of the simulated GIT-passage system. Replicate counts occur at all stages.
For the GIT system, 1 ml overnight culture of Salmonella (ON) was mixed with 9 ml simulated gastric fluid (SGF). Samples (SGF-strain mixture) were incubated during 30 min at 37°C, after which 1 ml SGF-strain mixture was taken for microbiological analysis. From the remaining SGF-strain sample, 4 ml was mixed with 40 ml simulated intestinal fluid (SIF) and incubated during 2 h at 37°C. Salmonella cells surviving these SGF and SIF stages were incubated with fully differentiated Caco-2 cells to investigate their attachment (ATT) and invasion (INV) capability. This latter assay mimics the interaction with the small intestinal epithelium (Pinto et al., 1983; Hendricks et al., 1996). Caco-2 cells were obtained from the American Type Culture Collection (ATCC, HTB-37, United States). A 12-well plate (Corning, United States) was inoculated with 40 μl SIF-SGF-strain mixture per well, and incubated at 37°C during 1 h for the attachment assay (ATT). After aspiration of the medium, the cells were either lysed used for determining the number of attached and invaded Salmonella, or treated with gentamycin for the invasion assay(INV). For this invasion assay, the plates were incubated during 3 h at 37°C. The lysate was used for enumeration of the invaded Salmonella.
Surviving Salmonella cells were enumerated after every stage in the GIT model: ON, SGF, SIF, ATT, and INV (data not shown).
GIT System Data Analysis
A hierarchical Bayesian framework was used to analyze bacterial count data obtained from the gastro intestinal tract experiments. The methodology for this analysis has been described in Wijnands et al. (2017). In short, bacterial counts at any stage in the GIT system (ON, SGF, SIF, ATT, and INV) were assumed Poisson distributed. Concentrations were assumed lognormally distributed, and the changes in log concentrations at any stage were estimated, by employing Markov chain Monte Carlo (MCMC) sampling. Thus in vitro infectivity within and between strains can be quantified, separating biological variability from experimental uncertainty.
The sum of all log changes in concentration of Salmonella (ml−1) throughout the GIT system from ON up until concentration invasion into Caco-2 cells (INV) was defined as log P(inf), an estimate for in vitro infectivity. The mean P(inf), including a 95% credible interval (C.I.), was calculated for each strain tested in the GIT system. In addition, a mean P(inf), standard deviation (SD) and 95% C.I. were calculated at the serovar level averaging posterior samples of multiple individual strains when available (Table 1).
Whole Genome Sequencing (WGS)
DNA Isolation
Genomic DNA from studied strains was extracted using the MagAttract HMW DNA kit (Qiagen, Venlo, Netherlands) according to the manual “Purification of High Molecular Weight Genomic DNA from Gram Positive Bacteria” (including a lysozyme step). An extra concentration and wash step were included to increase the yield and purity of DNA (20–50 ng/μl).
The concentration and purity of the DNA were measured using a Quantus device (Promega, Leiden, Netherlands) according to the manual for using the QuantiFluor dsDNA kit (Promega).
Next Generation Sequencing (NGS)
Paired-end sequence reads were generated using the Illumina HiSeq 2500 system with standard Illumina filtering and quality control. FASTQ sequence files were produced using the Illumina Casava pipeline version 1.8.3.
Processing Sequence Reads
For downstream bioinformatic analyses, sequence reads were assembled using SPAdes (Bankevich et al., 2012) on the ARIES platform of the public Galaxy server at Istituto Superiore di Sanita2. The settings of the SPAdes assembler were; Run only assembly (without read error correction): No, Careful correction: Yes Automatically choose k-mer values: No, K-mers to use, separated by commas: 21, 33, 55, Coverage Cutoff: Off.
Quality of resulting assemblies was assessed by checking the total number the contigs, their maximum and average length, and N50 per strain (Supplementary Appendix A).
The sequence data of the isolates are available. Accession numbers are provided in Supplementary Appendix A.
Phylogenetic Analysis
The read-based, reference-free method kSNP (Gardner et al., 2015), a k-mer based approach for detecting single-nucleotide variants, was used for phylogenetic analyses. kSNP3 was applied via the Galaxy project Aries (see footnote 1). The kmer starting value was set at a lower limit of 19 and a final upper limit of 191 with a search step of 2.
Virulence Genes
Sequences from virulence genes listed in the Salmonella section of the virulence factor database3 and some additional ones found in the literature were downloaded (e.g., Bolton et al., 2012; Suez et al., 2013; Khoo et al., 2015). These conformed a set of 233 putative virulence genes.
The tested virulence gene sequences (chromosome and plasmid) were selected for S. Typhimurium LT2, S. Enteritidis P125109, S. Agona SL483, S. Heidelberg SL476 and S. Typhi CT18. The investigated virulence genes are given in Supplementary Appendix B.
The presence or absence of the 233 virulence genes/factors was investigated by individual BLASTn analysis in the “Align two or more sequences” option4 of the assembled NGS data. The algorithm parameters were set to: expect threshold 10; word size 28; max matches in a query range 0; match/mismatch scores 1, −2; gap costs linear. Genes were marked as present if the BLAST showed an alignment of sequences of a strain with >90% identity and a coverage of >95% (Laing et al., 2017).
Epidemiological Surveillance Data
Notification data for the Salmonella serovars used in this study were obtained from the national laboratory surveillance of RIVM (Zomer et al., 2014).
Linking in vitro Virulence to the Presence/Absence of Virulence Genes
A description of the thorough Bayesian statistical analysis of the in vitro virulence of the 59 Salmonella strains has been described in section “GIT System Data Analysis” with further reference to Wijnands et al. (2017). Based on these analyses, accounting for uncertainty in P(inf) estimates, the Salmonella strains could be grouped into four categories: Low: P(inf) < 10−3, mid-Low: 10−3 < P(inf) < 10−2, mid-High: 10−2 < P(inf) < 10−1 and High: P(inf) > 10−1. Subsequent descriptive statistics, simple linear regression and principal component analysis (details provided in Supplementary Appendix C) were used to visualize potential relations between the 233 investigated virulence genes and a variety of phenotypic characteristics: survival through the separate stages of the GIT system, inferred from the Bayesian statistical analysis of in vitro virulence, and human cases of illness.
Results
In vitro Virulence
The mean in vitro virulence P(inf) with 95% credible intervals (C.I.) calculated from the GIT experiments were sorted by average P(inf). Figure 2 shows P(inf) posteriors calculated for strains grouped at serovar level and Figure 3 shows P(inf) posteriors calculated for all individual strains. The associated values together with the standard deviation of the P(inf) posterior distributions on serovar level are provided in Table 1. Variability exists both within and between serovars. The average P(inf) ranges in the order of 1E-05 to 1E-01 for individually tested strains in the GIT system. A Salmonella Kedougou strain had the lowest P(inf), 5.3E-05 for an individual strain while a S. Typhimurium strain had the highest individual P(inf), 5.2E-01 and on average 1.7E-01 on serovar level. Individual strains of S. Typhimurium cover, however, a broad range of in vitro virulence; 1.2E-04 for one of the human strains and from 1.2E-02 up to 5.2E-01 for the other strains.
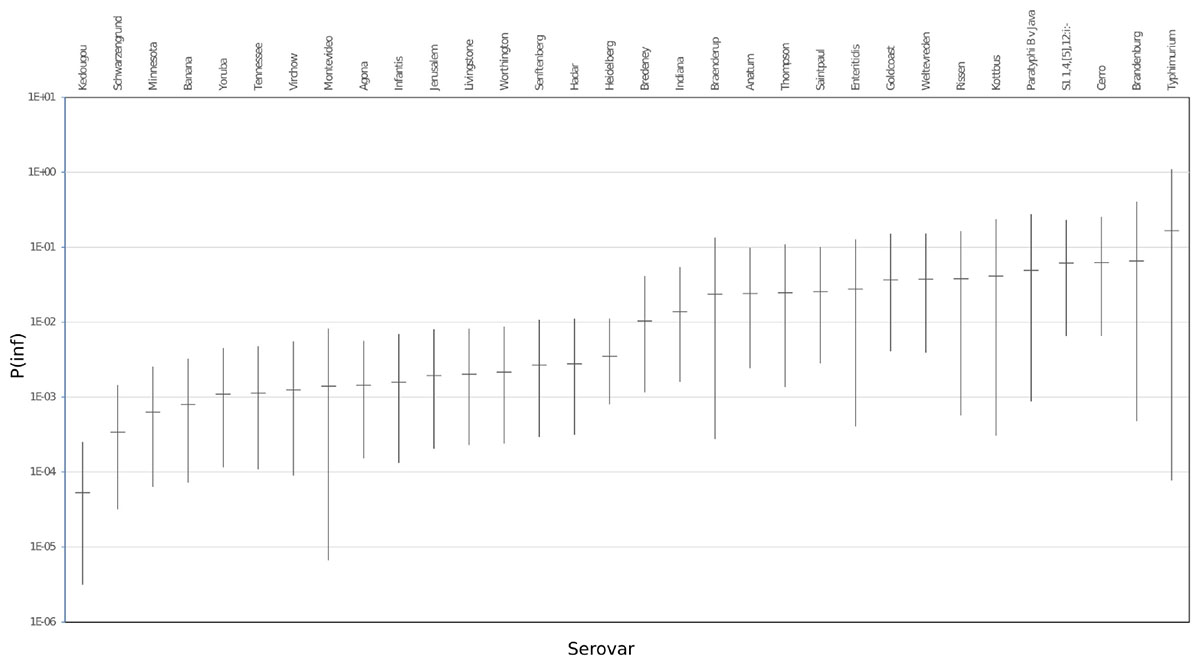
Figure 2. P(inf) at serovar level from low (left) to high (right) infectious serovars in the GIT system. The horizontal lines indicate the mean P(inf) and the bars represent the 95% C.I. See Table 1 for the associated values.
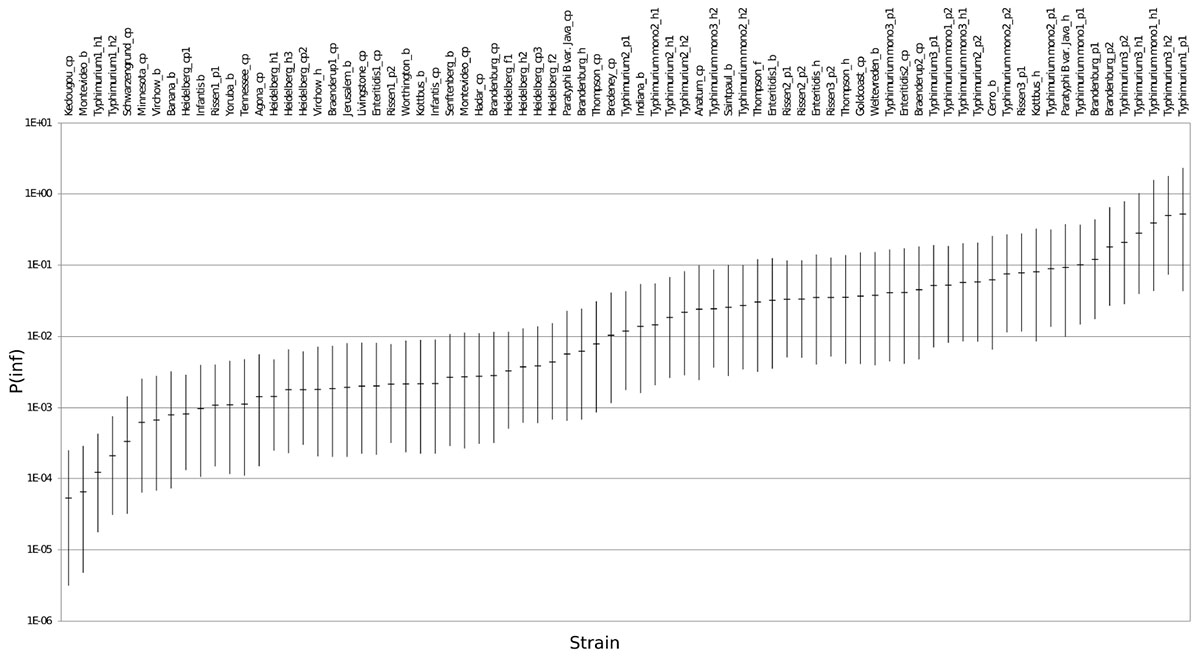
Figure 3. P(inf) for all individual test strains from low (left) to high (right) infectious strains in the GIT system. The horizontal lines indicate the mean P(inf) and the bars represent the 95% C.I. See Table 1 for additional strain information (b, broiler; cp, chicken product; f, food; h, human; p, pork). Strains of the same serovar and source have equal indicators (e.g., Typhimurium1_cp). Replicates of the same strain are numbered as indicated by the underscore (e.g., _cp1).
Figure 3 shows that the 95% C.I. describing the experimental P(inf) uncertainty were within the same range for individual strains. The 95% C.I.’s were larger at serovar level for some serovars compared to the 95% C.I.’s of the underlying strains. This indicates a larger variability in P(inf) for individual strains of the same serovar compared to those of other serovars was observed. For example, S. Montevideo showed a relatively large 95% C.I. which can be explained by the relatively large difference in P(inf) for the individual strains, one broiler strain with a P(inf) of 6.5E-05 and one chicken product strain with a P(inf) of 2.7E-03. On the other hand, S. Infantis showed a relatively small 95% C.I. at serovar level with both broiler and chicken product strains. S. Heidelberg also showed a relatively small 95% C.I. at serovar level. This is caused by a relatively large number of replicates with three test strains and a narrow range in mean P(inf): 8.3E-04 – 4.4E-03 for the individual test strains. The food (f) and human (h) S. Heidelberg strains stem from the same outbreak, and the chicken product (cp) strain was not related to the outbreak, but still had a comparable P(inf).
All replicate experiments using the same Salmonella strain had overlapping 95% C.I.’s. For example, S. Rissen consists of three pork strains that have all been tested twice, expressed as Rissen(1) with a P(inf) of 1.1E-03 and 2.1E-03, Rissen(2) with both a P(inf) of 3.3E-02 and Rissen(3) with a P(inf) of 3.5E-02 and 7.8E-02 (Figure 3).
Overall, Figure 2 shows variability in P(inf) between different Salmonella serovars, where S. Kedougou is characterized by the lowest P(inf) (<1E-04), followed by a group with a P(inf) in the range of 1E-03 -1E-02 and a group with a P(inf) > 1E-02. The second group contains one serovar (S. Infantis) often associated with human cases of illness, whereas the third group contained Typhimurium and Enteritidis which have the highest human disease incidence. However, also serovars with low incidence (for example Gold Coast and Kottbus) can be found in highest range of P(inf) and large variability in P(inf) can be seen between individual Salmonella strains (Figure 3).
Virulence Genes
Among the 233 investigated virulence factors, variability in presence/absence in the strains was concentrated to 101 genes, because 14 were absent and 118 were present in all tested strains (see Supplementary Appendix B). A gene was assigned “present” when it was detected in >70% of the tested strains for each serovar. The five serovars with the highest number of virulence genes in individual strains were: Heidelberg (181), Typhimurium and Enteritidis (both 179), Virchow (177) and Thompson (176). The five serovars with the lowest number of virulence genes in individual strains are: Schwarzengrund (151), Bredeney (150), Cerro (148), Montevideo (144), and Brandenburg (142).
Figure 4 shows the presence of the 101 determinant virulence genes for all strains and for those related to the different categories (low, low-mid, mid-high, and high) of the in vitro virulence of the GIT system. There is no straight forward association between gene presence and P(inf) when comparing gene presence in all the categories with the gene presence for each category separated, especially those in the extremes, whose presence is either close to a value of 1 or 0. Only a few genes are evidently increasing in presence as the P(inf) increases to higher categories (safA, gogB, ssel.srfH, STM1043, sodCI, stdA, sseK2, and the sef operon) and another few are increasing in absence as the P(inf) category increases (the stk operon and the ste operon).
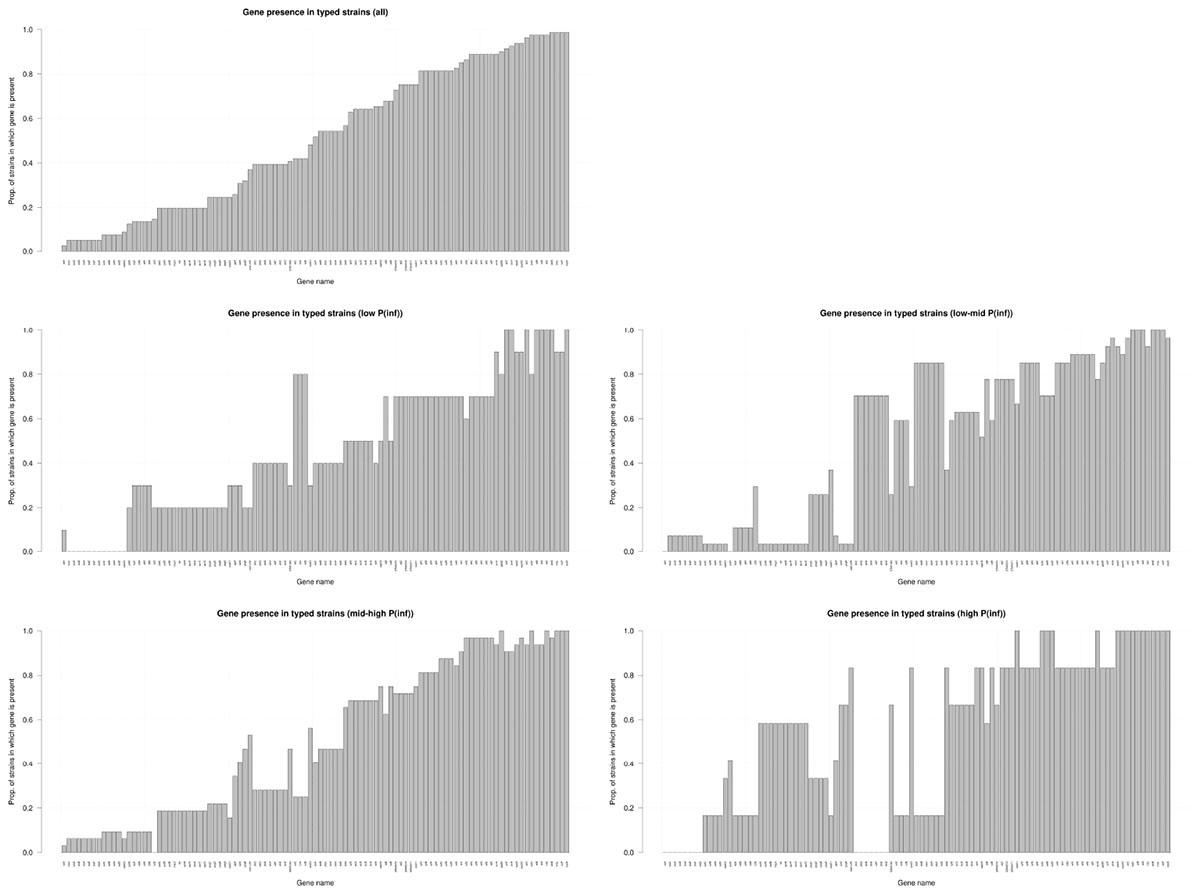
Figure 4. Presence/absence of virulence genes in all strains investigated (n = 59); low: P(inf) < 1E-03 (n = 8), low-mid: 1E-03 < P(inf) < 1E-02(n = 22), mid-high: 1E-02 < P(inf) < 1E-01(n = 23), high: P(inf) > 1E-01 (n = 6). Genes that were either present or absent in all strains are not shown.
The presence/absence of virulence genes on strain level was submitted to a principal component analysis (PCA, Figure 5). Only those 101 virulence genes that showed variability in their presence/absence among the strains were included. Based on the PCA analysis, different clusters could be identified based on the presence of virulence genes, which could be grouped under different virulence profiles (Figure 6). Profile 1, the virulence genes located on a plasmid; mig-5 and operons of pef, rck and spv were only present in serovars with a high P(inf), S. Typhimurium and S. Enteritidis. Profile 2, the typhoid toxin genes cdtB, hlyE, plt, and taiA operon were only found in the Salmonella serovars Brandenburg, Bredeney, Gold Coast, Indiana, Minnesota, Montevideo, and Schwarzengrund. Profile 3, the stk operon virulence genes were present in the outbreak strains of S. Heidelberg and S. Thompson, but also in serovars like Banana, Braenderup, Hadar, Indiana, Paratyphi B var. Java (cp), Rissen, Saintpaul, Senftenberg, Virchow, Worthington and Yoruba. And profile 4, the peg operon fimbrial virulence genes were not only present in one of the most relevant serovars from an epidemiological viewpoint, S. Enteritidis, but also in serovars; Anatum, Brandenburg, Bredeney, Livingstone, Minnesota, Schwarzengrund, Thompson, Worthington and Yoruba.
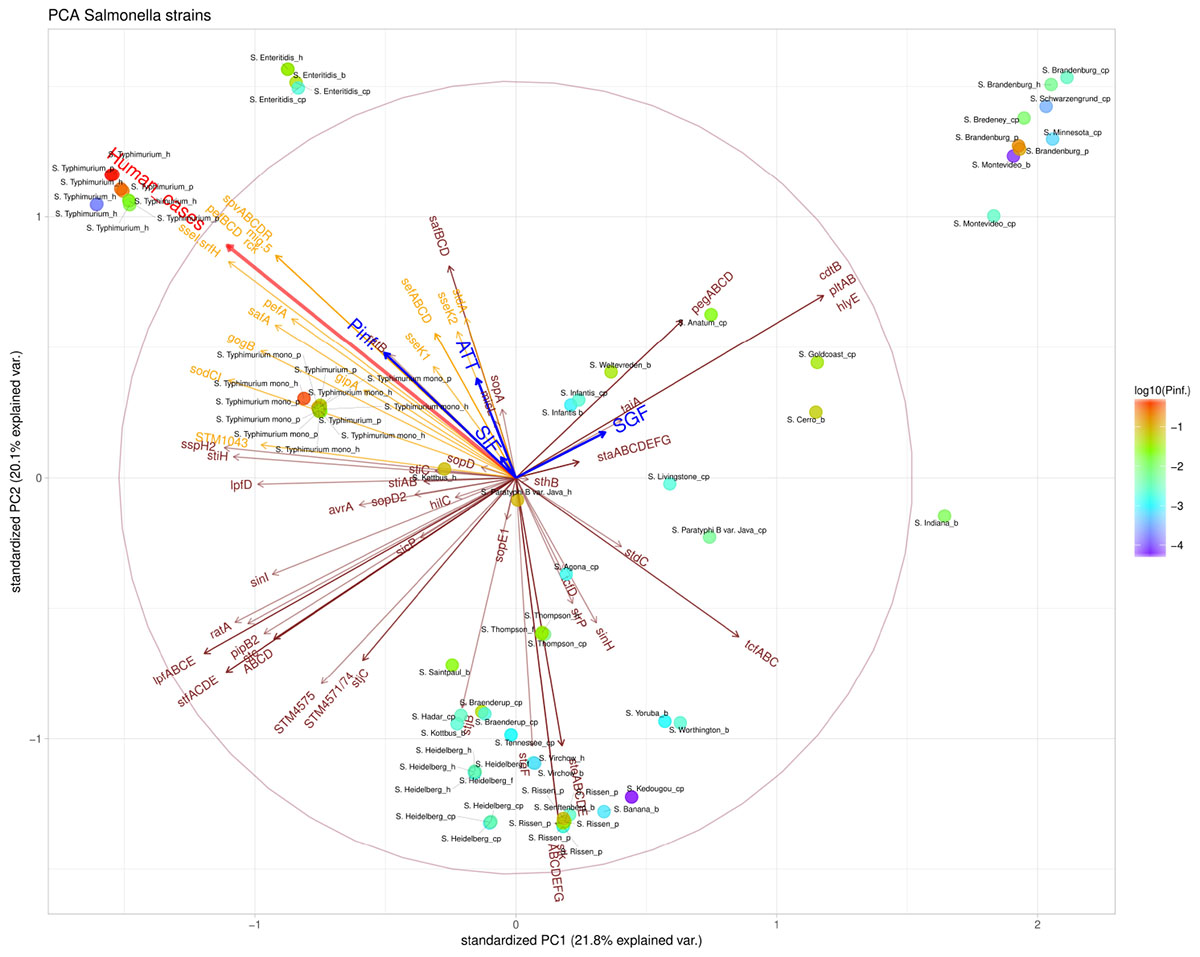
Figure 5. Principal component analysis (PCA) scatterplot calculated from the presence/absence of the various virulence genes (brown and orange arrows) in the Salmonella strains under investigation. The number of human cases (shown in red arrow) and survival up to the indicated stages SGF, SIF. ATT and INV [indicated as P(inf)] (shown in blue arrows). The color scale of the dots characterizes P(inf) of individual strains (indicated in the legend). Orange virulence genes are those associated with a positive P(inf) effect (p-value < 0.05) (see section “Genetic Variability Linked to in vitro Virulence,” Table 2). The length and direction of the variable arrows indicate how those variables contribute to the separation of the data when projected on the two components which explain the most data variance, i.e., PC1 and PC2. An arrow reaching the large circle means that it is fully projected on the two principal component plane. See Table 1 for additional strain information (b, broiler; cp, chicken product; f, food; h, human; p, pork). Strains of the same serovar and source have equal indicators (e.g., Typhimurium1_cp).
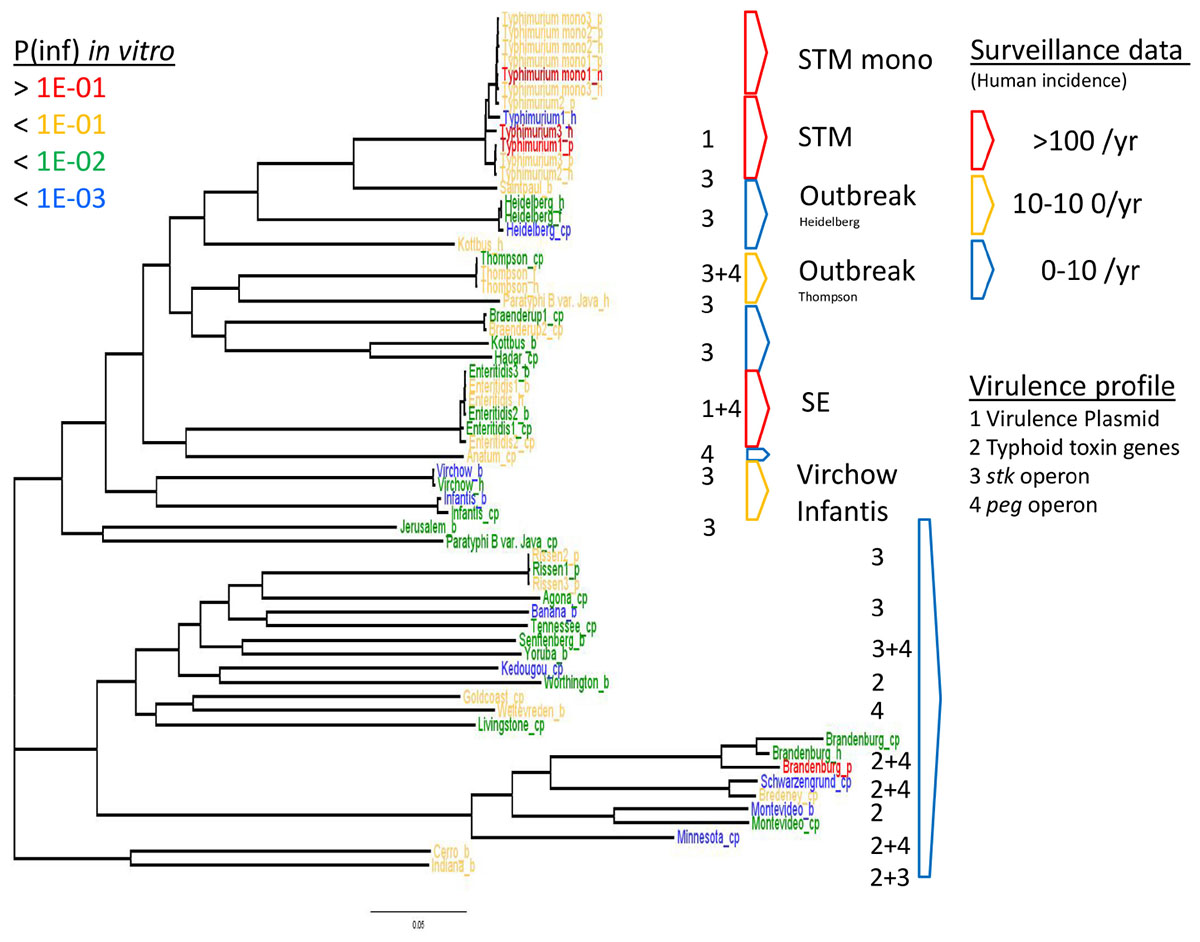
Figure 6. WGS based phylogeny related to in vitro virulence of the GIT system (in this study) and surveillance data. The serovar names of the Salmonella strains with the highest P(inf) are written in red (>1E-01), mid-high (<1E-01) in yellow, low-mid (<1E-02) in green and the lowest in blue (<1E-03). The Salmonella top five serovars related to human cases are marked in red (>100/year) and yellow (10–100/year), the lowest number of human cases (0–10/year) are blue (Zomer et al., 2014). See Table 1 for additional strain information (b, broiler; cp, chicken product; f, food; h, human; p, pork). Strains of the same serovar and source have equal indicators (e.g., Typhimurium1_cp). Numbers 1, 2, 3, and 4 indicate the virulence profiles found as clusters in the PCA analysis, present in each strain and grouped by serovar.
The GIT System
Figure 5 shows that stomach survival (shown as SGF) was positively associated with the presence of Typhoid toxin genes. Survival through other stages of the GIT system [shown as SIF, ATT, and P(inf), respectively] were associated with higher human disease incidence and more specifically, with S. Typhimurium, S. Enteritidis and monophasic S. Typhimurium.
Genetic Variability Linked to in vitro Virulence P(inf)
The distribution of the presence/absence of genes among strains with a relatively high P(inf), > 1E-01 was compared to strains with a relatively low P(inf), <1E-03 (Figure 4). Highly in vitro infectious strains did not possess any of the stk genes. Genes associated with highly in vitro infectious strains were gogB, mig-5, safA, STM1043 and operons pef, rck and spv.
A simple linear regression model (Pielaat et al., 2015) was applied in an attempt to identify those genes which could be associated with high P(inf) (Table 2). All plasmid related genes (mig-5 and operons of pef, rck and spv) were part of this group and were only present in the human cases associated serovars Typhimurium and Enteritidis. All the virulence genes present in more than 50% of the strains with the highest P(inf) (gogB, mig-5, pef, rck, safA, spv, and STM1043) are mentioned in Table 2 as the genes that have a positive association with P(inf). The results from the linear regression model were used as an identification tool rather than for testing an hypothesis on whether the studied genes are responsible for high P(inf) values. Results suggest that not all strains with a relatively high P(inf), > 1E-02, possessed the identified virulence genes having a gene effect with p-value < 0.05 (marked with an asterisk in Supplementary Appendix B). For example, S. Rissen with a comparable P(inf) to S. Enteritidis [P(inf) of 3.8E-02] possesses none of the 19 virulence genes with p-value < 0.05, while 13 of these genes were present in S. Enteritidis (only gipA, gogB, pefA, safA, stdA, and STM1043 were absent).
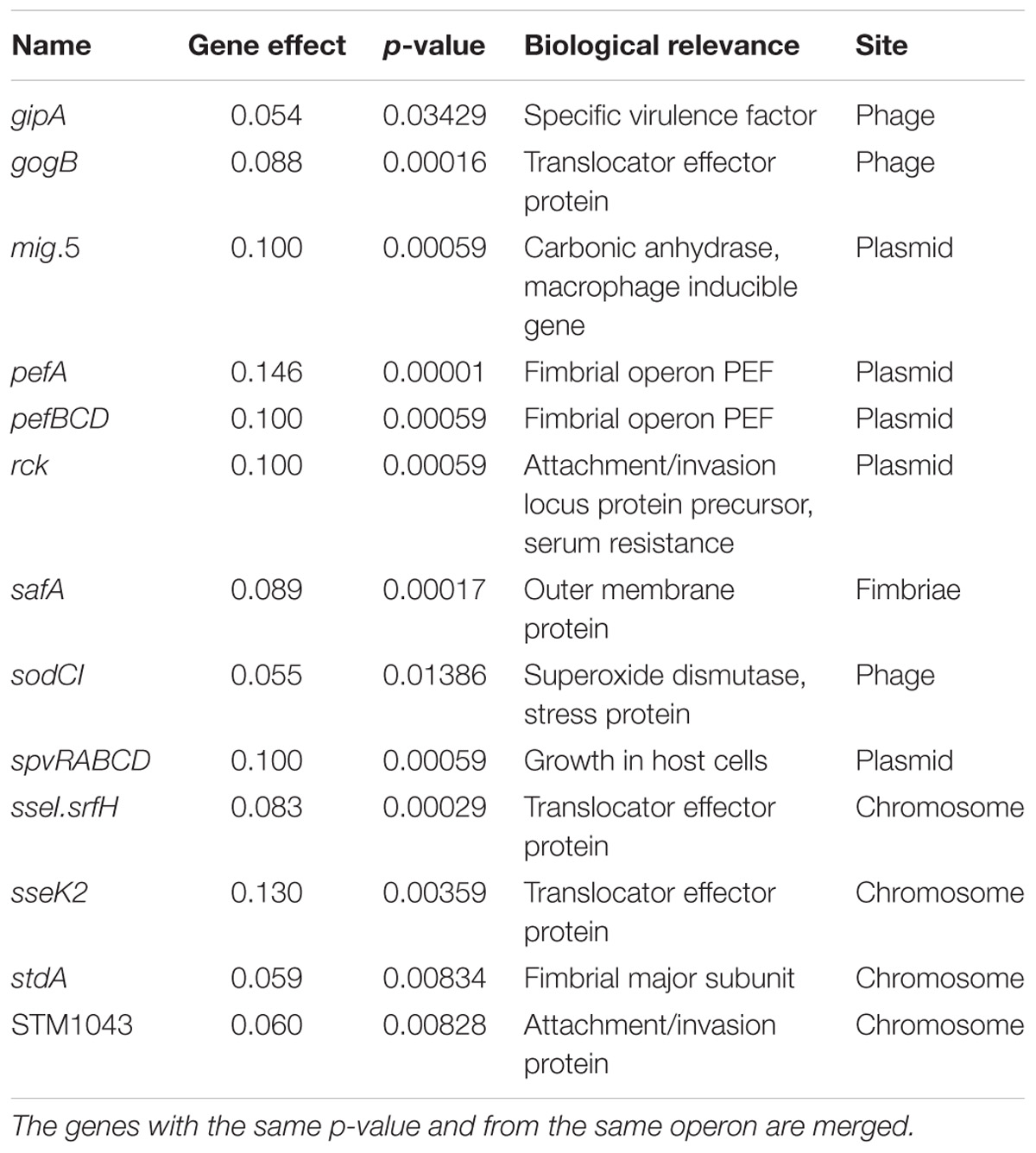
Table 2. Parameter values of the gene effect (n = 19) with a p-value < 0.05 which are positively associated with P(inf).
Phylogeny Related to in vitro Virulence and Surveillance Data
The relation of the phylogenetic tree based on the WGS data of all tested Salmonella strains to in vitro virulence expressed in different P(inf) categories, showed no clear phylogenetic relationship regarding virulence (Figure 6). Serovars with a P(inf) > 1E-02 show a tendence to be clustered [(monophasic) Typhimurium, the outbreak strains (Thompson and Heidelberg) and Enteritidis]. The relation of human salmonellosis surveillance data on serovar level of the Dutch population (Zomer et al., 2014) to the phylogenetic data suggests that serovars with low number of human cases (0–10 per year) in the same part of the phylogenetic tree (e.g., Rissen and Brandenburg serovars in Figure 6). There was no apparent relation between the serovars possessing the same virulence profiles to the phylogenetic tree. An exception were the serovars with typhoid toxin genes virulence profile, which most could be located in the same part of the phylogenic tree (Figure 6).
Conclusion
The virulence of in total 59 strains of 32 different Salmonella serovars was assessed in an in vitro gastrointestinal tract system including attachment and invasion into cultured human intestinal epithelial cells. Statistical association with the presence/absence of 233 virulence genes in these strains was studied, and the results compared with surveillance data of human salmonellosis.
The in vitro infectivity estimate (P(inf)) was quantified and strains were differentiated based on their variability in P(inf).
Although many of the human associated serovars (e.g., S. Typhimurium and S. Enteritidis) clustered in the relatively high P(inf) group, still, large variability in P(inf) could be seen between individual Salmonella strains.
Based on sequence data, it was possible to cluster serovars with the same profile of virulence genes (virulence plasmid, Typhoid toxin, peg operon and stk operon.) but there was no clear association with phenotypic virulence P(inf). Overall, individual strains showed larger variability in P(inf) in association with virulence genes than could be explained by serovar alone. Ranking a public health risk of different Salmonella using point estimates in in vitro P(inf) on serovar level alone without considering biological variability of the underlying strains is, therefore, not recommended.
Finally, we would like to stress that a systematic approach in which molecular data is linked to other strain characteristics is a crucial step for future MRA studies. The aggregation of molecular data based on biologically relevant information will decrease the uncertainty in microbiological risk assessments. It will also prevent the initiation of a disaggregation problem in which the number of hazards will increase exponentially when a human risk assessment is based on the analysis of molecular data alone and each strain forms a distinct hazard.
Discussion
Traditional MRA methodologies based on the Codex principles for risk analysis (Codex Alimentarius Commission [CAC] (1999)) are currently revised by the scientific community in the light of the increasing amount of available molecular data. Pielaat et al. (2015) suggested that the integration of one dimensional in vitro testing (e.g., invasion into Caco-2 cells) with multiple dimensional interpretation of effects on a molecular level is a step forward to solve the mapping problem of translating this high dimensional information on molecular level to a single measure of risk (e.g., number of ill cases). In this study, we used descriptive statistics, simple linear regression and principal component analysis to visualize this integration of in vitro testing (passage through the GIT system) with human cases of illness and 233 investigated virulence genes. This type of analysis enabled us to identify a clustering of potentially relevant biological associations between phenotypic and genotypic data for microbiological risk assessment of Salmonella. This approach forms the basis to ultimately be able to build a valid, non-overparameterized, statistical model to identify marker genes for risk assessment.
In this study biological variability in in vitro infectivity was integrated with genetic diversity and human salmonellosis surveillance data to reveal potential new insights in the public health risk of different Salmonella strains. Results show (Figure 3) that in vitro infectivity, P(inf), for human Salmonella strains within one serovar is higher than for strains from other non-human sources within the same serovar [except for S. Typhimurium with high variability among all individual strains and monophasic S. Typhimurium, variable but all strains have a relatively high P(inf)]. This is in accordance with an assumption by Oliveira et al. (2011) that the history of a strain may have influence on its virulence. Further research would be needed with more strains per serovar to quantify specific within and between in vitro variability for all individual serovars as used in this study. However, concluding that the in vitro P(inf) variation among individual strains from the same serovar is larger than the P(inf) variation found between serovars still holds. This because the unequal number of strains per serovar has been already accounted for by the presented 95% credible intervals, which represent uncertainty on the true P(inf) at serovar level. Moreover, our experiments show a gradual range in in vitro virulence from 10−5 to 10−1. It represents the minimal range for measuring in vitro virulence as input for biologically relevant risk assessment for Salmonella. Including more strains will not necessarily change the overall conclusions.
The P(inf) agrees with human surveillance data where serovars with a high P(inf) have a high number of reported human cases [S. Typhimurium and the monophasic S. Typhimurium both with the highest average P(inf) and number of human cases, followed by S. Enteritidis (see Figure 6)]. S. Infantis and S. Virchow are an exception with a relatively low P(inf), but still between 10–100 confirmed human cases (Zomer et al., 2014). Strains associated with outbreaks caused by S. Heidelberg and S. Thompson from both food and human origin have comparable P(inf). In both cases, the P(inf)s of the outbreak-related strains are higher than the P(inf) of non-outbreak related strains originating from chicken products. The outbreak-related strains S. Heidelberg and S. Thompson (Friesema et al., 2014; van Rijckevorsel et al., 2015) did not show any specific association with survival through the stages in the GIT system (Wijnands et al., 2017; Figure 5). Of course, in this study we only consider in vitro P(inf) as explanatory variable for human cases, whereas other pathogen/host factors like prevalence, growth potential, acquired immunity, etc., influence the number of human cases.
Among the 233 investigated virulence genes, 101 showed variability in presence/absence in the Salmonella strains (see Supplementary Appendix B). In vitro P(inf) is slightly positively associated with the reported number of S. Typhimurium and S. Enteritidis human cases and specific plasmid related virulence genes (mig-5, operons of pef, rck and spv). However, not all serovars with a relatively high P(inf), > 1E-02, are associated with the identified virulence genes having positive association with P(inf) (gene effect with p-value < 0.05). More strains with an equal distribution over different serovars would need to be investigated to make any further statements about phenotypes and associated genes inside serovars. Moreover, a pangenomic approach on a larger strain set representing the total (molecular) variability of Salmonella would increase the probability to detect genes highly associated with P(inf).
The presence of virulence genes among different Salmonella strains as investigated in this study (see Figure 5 and Supplementary Appendix B) can be discussed against other published studies with a main focus on those genes identified to be potentially positively associated with P(inf) (see Table 2). These genes or operons will be addressed below one by one:
- The gipA gene is involved in Peyer’s patch survival and it concurs to Salmonella colonization of the M cells in the Peyer’s patch (Stanley et al., 2000). In our study gipA was present in strains of S. Typhimurium (h and p), monophasic S. Typhimurium (h and p), S. Virchow (h and p), S. Brandenburg (cp), and S. Weltevreden (p). Huehn et al. (2010) showed comparable results with the gipA gene being present in a part of the tested Typhimurium and Enteritidis strains and almost all Virchow strains. Beutlich et al. (2011) found the gipA gene in one Typhimurium strain.
- The gene sodC1 (superoxide dismutase), a virulence associated stress protein located on prophage, was present in S. Typhimurium (h and p), S. Heidelberg (cp, f and h), S. Enteritidis (cp, h and p), monophasic S. Typhimurium (h and p), S. Weltevreden (p) and absent in all strains with a low P(inf). Huehn et al. (2010) and Osman et al. (2014) also showed presence of sodC1 in S. Typhimurium and S. Enteritidis.
- The spv (Salmonella plasmid virulence) operon was found to be associated with Salmonella survival and growth in macrophages by Rychlik et al. (2006), and appeared to be only present in serovars Typhimurium and Enteritidis in our study. Huehn et al. (2010), Guedda et al. (2014), and Hoffmann et al. (2014) all showed comparable results with the spv operon being present in Typhimurium and Enteritidis, but absent in other serovars under investigation.
In addition to the relation of our findings compared to those in other studies concerning the genes in Table 2, the following remarkable results were observed:
- The staA gene, originally identified uniquely in serovar Typhi (Townsend et al., 2001; Al-Dahhan et al., 2015), was now found to be present in four of the investigated strains all isolated from a chicken product: S. Agona, S. Anatum, S. Livingstone, and S. Gold Coast. Huehn et al. (2010), did not find the staA gene in any of the 77 investigated strains [serovars Enteritidis (19), Hadar (14), Infantis (11), Typhimurium (21), and Virchow(11)].
- The stk fimbrial operon was present in multiple serovars, but remarkable was that the stk genes were present in all the investigated Heidelberg and Thompson including outbreak strains. However, Foley et al. (2011) also mentioned the presence of stk genes in isolates of S. Heidelberg but indicated that genes were differentially expressed among the S. Heidelberg strains examined.
- Typhoid toxin genes known from S. Typhi CT18 (cdtB, hlyE, and pltAB) were present in 10 strains, among the serovars Brandenburg, Bredeney, Gold Coast, Indiana, Minnesota, Montevideo and Schwarze Grund while taiA (Typhi-associated invasin A) was only present in Gold Coast, and Minnesota. The presence of cdtB and pltAB in isolates of Montevideo, Schwarzengrund, Bredeney was also found by Suez et al. (2013). Fuentes et al. (2008) found that hlyE (pore-forming hemolysin) mutants show impaired invasion of human epithelial cells in vitro, and its expression in S. Typhimurium improves the colonization of deep organs in mice, demonstrating that the HlyE hemolysin is a virulence determinant.
- The peg operon showed to be present in most important serovars from an epidemiological viewpoint. The role of peg operon in colonization and virulence has been established in a number of model experiments (Silva et al., 2012).
Most of the virulence genes that have a positive association with P(inf) were plasmid and phage associated genes (Table 2). Cheng et al. (2015) mentioned that the disease incidence of poultry strains in humans may be attributable to the distribution of phage-associated virulence genes sodCI, sopE, and sseI and the plasmid located spvB in S. enterica serovars.
Some differences were observed in the possession of virulence genes in strains of Typhimurium and monophasic Typhimurium, both with a high P(inf) and high incidence in the surveillance data. Most remarkable was the absence of plasmid related virulence genes (mig-5 and pef, rck and spv operons) in all the monophasic Typhimurium strains and also in one Typhimurium strain (still with a high P(inf)). There was more variation in the number of virulence genes within the Typhimurium strains (169–179 of the 233) compared to monophasic Typhimurium strains which all possess the same total (169 of the 233). García et al. (2014) reported that most isolates of Typhimurium strains contained a virulence plasmid but they did not find it in isolates belonging to the monophasic variant. Still, it should be noticed that currently applied fragmented assembly procedures may induce false negative results for the absence of genes screened by blast. However, the probability of having false negative results will be limited in our study. An additional screening on virulence genes partly present on two different assemblies has been performed and still included as a positive result in further analysis.
Independent of serovar, an association was shown between certain virulence genes in the strains and their in vitro virulence (Figure 4). Further in depth investigation is needed to assess how much of the variability in P(inf) in the in vitro GIT system can be explained by one or a combination of virulence genes as identified in this study. This would involve the phenotypic analysis of a larger set of strains representing the whole (molecular) Salmonella population resulting in sufficient power to build a valid statistical model for causal analysis. Finally, biological confirmation (e.g., with gene knock-out experiments) and reproducibility of the analysis is a prerequisite to confirm any causal relationship between genotypic and phenotypic characteristics.
Author Contributions
AK was in charge of all practical work concerning the NGS data and GIT system lab analysis and NGS analysis, data interpretation, and the design, and data generation during the whole study. ABM developed together with AP the latest Bayesian statistical framework for the calculations on the GIT system, and is responsible for statistical calculations under that framework and for part of the results from the NGS data and PCA analysis. LW was responsible/supervisor for all the work on the GIT system. ED-v was responsible for the practical lab work on the strains and the GIT system. AvH was supervisor for all the work on the obtained NGS data. AP and EF planned and designed the whole project. AP was project-leader and responsible for the study design, the statistical calculations and supervision of finance. AK and AP have written the manuscript. All authors read, commented, and approved the final manuscript.
Funding
This work has been made possible by the Dutch Food and Consumer Safety Authority (NVWA) and funded by the research program of the RIVM.
Conflict of Interest Statement
The authors declare that the research was conducted in the absence of any commercial or financial relationships that could be construed as a potential conflict of interest.
Acknowledgments
We thank Peter Teunis for carefully reading and critical comments on the manuscript.
Supplementary Material
The Supplementary Material for this article can be found online at: https://www.frontiersin.org/articles/10.3389/fmicb.2018.03182/full#supplementary-material
Footnotes
- ^ http://ilsi.eu/task-forces/food-safety/microbiological-food-safety/
- ^ https://w3.iss.it/site/aries
- ^ http://www.mgc.ac.cn/VFs/main.htm
- ^ https://blast.ncbi.nlm.nih.gov/Blast.cgi
References
Al-Dahhan, H. A., Muhammad Ali, A. J., and Al-Ammer, M. H. (2015). Phenotypic and genotypic characterization of Salmonella Typhi virulence factors isolated from patients with typhoid fever in Najaf province / Iraq. Int. J. Res. Stud. Biosci. 3, 77–84.
Bankevich, A., Nurk, S., Antipov, D., Gurevich, A. A., Dvorkin, M., Kulikov, A. S., et al. (2012). SPAdes: a new genome assembly algorithm and its applications to single-cell sequencing. J. Comput. Biol. 19, 455–477. doi: 10.1089/cmb.2012.0021
Beutlich, J., Jahn, S., Malorny, B., Hauser, E., Hühn, S., Schroeter, A., et al. (2011). Antimicrobial resistance and virulence determinants in European Salmonella genomic island 1-positive Salmonella enterica isolates from different origins. Appl. Environ. Microbiol. 77, 5655–5664. doi: 10.1128/aem.00425-11
Bolton, D. J., O’Neill, C. J., and Fanning, S. (2012). A preliminary study of Salmonella, verocytotoxigenic Escherichia coli/Escherichia coli O157 and Campylobacter on four mixed farms. Zoonoses Public Health 59, 217–228. doi: 10.1111/j.1863-2378.2011.01438.x
Brandwagt, D., Wijngaard, van den, C., Tulen, A. D., Mulder, A. C., Hofhuis, A., et al. (2018). Outbreak of Salmonella Bovismorbificans associated with the consumption of uncooked ham products, the Netherlands, 2016 to 2017. Euro Surveill. 23:17-00335. doi: 10.2807/1560-7917.ES.2018.23.1.17-00335
Cheng, Y., Pedroso, A. A., Porwollik, S., McClelland, M., Lee, M. D., Kwan, T., et al. (2015). rpoS-Regulated core genes involved in the competitive fitness of Salmonella enterica serovar Kentucky in the intestines of chickens. Appl. Environ. Microbiol. 81, 502–514. doi: 10.1128/AEM.03219-14
Codex Alimentarius Commission [CAC], (1999). Principles and Guidelines for the Conduct of Microbiological Risk Assessment. Available at: http://www.fao.org/docrep/004/y1579e/y1579e05.htm
Davis, S., Pettengill, J. B., Luo, Y., Payne, J., Shpuntoff, A., Rand, H., et al. (2015). CFSAN SNP Pipeline: an automated method for constructing SNP matrices from next-generation sequence data. PeerJ Comput. Sci. 1:e20. doi: 10.7717/peerj-cs.20
den Besten, H. M. W., Amézquita, A., Bover-Cid, S., Dagnas, S., Ellouze, M., Guillou, S., et al. (2017). Next generation of microbiological risk assessment: potential of omics data for exposure assessment. Int. J. Food Microbiol. 287, 18–27. doi: 10.1016/j.ijfoodmicro.2017.10.006
European Food Safety Authority [EFSA], and European Centre for Disease Prevention and Control [ECDC] (2017). The European Union summary report on trends and sources of zoonoses, zoonotic agents and food-borne outbreaks in 2016. EFSA J. 15:5077. doi: 10.2903/j.efsa.2017.5077
Foley, S. L., Nayak, R., Hanning, I. B., Johnson, T. J., Han, J., and Ricke, S. C. (2011). Population dynamics of Salmonella enterica serotypes in commercial egg and poultry. Appl. Environ. Microbiol. 77, 4273–4279. doi: 10.1128/AEM.00598-11
Franz, E., Delaquis, P., Morabito, S., Beutin, L., Gobius, K., Rasko, D. A., et al. (2014). Exploiting the explosion of information associated with whole genome sequencing to tackle Shiga toxin-producing Escherichia coli (STEC) in global food production systems. Int. J. Food Microbiol. 187, 57–72. doi: 10.1016/j.ijfoodmicro.2014.07.002
Franz, E., Mughini Gras, L., and Dallman, T. (2016). Significance of whole genome sequencing for surveillance, source attribution and microbial risk assessment. Crit. Rev. Food Sci. Technol. 8, 74–79. doi: 10.1016/j.cofs.2016.04.004
Friesema, I., de Jong, A., Hofhuis, A., Heck, M., van den Kerkhof, H., de Jonge, R., et al. (2014). Large outbreak of Salmonella Thompson related to smoked salmon in The Netherlands. Euro Surveill. 19:20918. doi: 10.2807/1560-7917.ES2014.19.39.20918
Fuentes, J. A., Villagra, N., Castillo-Ruiz, M., and Mora, G. C. (2008). The Salmonella Typhi hlyE gene plays a role in invasion of cultured epithelial cells and its functional transfer to S. Typhimurium promotes deep organ infection in mice. Res. Microbiol. 159, 279–287. doi: 10.1016/j.resmic.2008.02.006
García, P., Hopkins, K. L., García, V., Beutlich, J., Mendoza, M. C., Threlfall, J., et al. (2014). Diversity of plasmids encoding virulence and resistance functions in Salmonella enterica subsp. enterica serovar Typhimurium monophasic variant 4,[5],12:i:- Strains circulating in Europe. PLoS One 9:e89635. doi: 10.1371/journal.pone.0089635
Gardner, S. N., Slezak, T., and Hall, B. G. (2015). kSNP3.0: SNP detection and phylogenetic analysis of genomes without genome alignment or reference genome. Bioinformatics 31, 2877–2878. doi: 10.1093/bioinformatics/btv271
Gilchrist, C. A., Turner, S. D., Riley, M. F., Petri, W. A. Jr., and Hewlett, E. L. (2015). Whole-genome sequencing in outbreak analysis. Clin. Microbiol. Rev. 28, 541–563. doi: 10.1128/CMR.00075-13
Guedda, I., Taminiau, B., Ferjani, A., Boukadida, J., Bertrand, S., and Daube, G. (2014). Antimicrobial and molecular analysis of Salmonella serovar Livingstone strains isolated from humans in Tunisia and Belgium. J. Infect. Dev. Ctries. 8, 973–980. doi: 10.3855/jidc.3989
Haddad, N., Johnson, N., Kathariou, S., Métris, A., Phister, T., Pielaat, A., et al. (2018). Next generation microbiological risk assessment – Potential of omics data for hazard characterization. Int. J. Food Microbiol. 287, 28–39. doi: 10.1016/j.ijfoodmicro.2018.04.015
Havelaar, A. H., Kirk, M. D., Torgerson, P. R., Gibb, H. J., Hald, T., Lake, R. J., et al. (2015). World health organization global estimates and regional comparisons of the burden of foodborne disease in 2010. PLoS Med. 12:e1001923. doi: 10.1371/journal.pmed.1001923
Hendricks, H., van Asten, A., Koninkx, J., Kok, W., van der Zeijst, B., and van Dijk, J. (1996). “Interactions between Salmonella enteritidis and the enterocyte-like human carcinoma cell line Caco-2,” in Effects of Nutrients on the Nutritional Value of Legume Diets, eds S. Bardocz, F. V. Nekrep, and A. Pustazi (Luxembourg: Official Publications European Communities), 137–139.
Hoffmann, M., Zhao, S., Pettengill, J., Luo, Y., Monday, S. R., Abbott, J., et al. (2014). Comparative genomic analysis and virulence differences in closely related Salmonella enterica serotype Heidelberg isolates from humans, retail meats, and animals. Genome Biol. Evol. 6, 1046–1068. doi: 10.1093/gbe/evu079
Huehn, S., La Ragione, R. M., Anjum, M., Saunders, M., Woodward, M. J., Bunge, C., et al. (2010). Virulotyping and antimicrobial resistance typing of Salmonella enterica serovars relevant to human health in Europe. Foodborne Pathog. Dis. 7, 523–535. doi: 10.1089/fpd.2009.0447
Jackson, B. R., Tarr, C., Strain, E., Jackson, K. A., Conrad, A., Carleton, H., et al. (2016). Implementation of nationwide real-time whole-genome sequencing to enhance listeriosis outbreak detection and investigation. Clin. Infect. Dis. 63, 380–386. doi: 10.1093/cid/ciw242
Katz, L. S., Griswold, T., Williams-Newkirk, A. J., Wagner, D., Petkau, A., Sieffert, C., et al. (2017). A comparative analysis of the Lyve-SET phylogenomics pipeline for genomic epidemiology of foodborne pathogens. Front. Microbiol. 8:375. doi: 10.3389/fmicb.2017.00375
Khoo, C.-H., Sim, J.-H., Salleh, N. A., and Cheah, Y.-K. (2015). Pathogenicity and phenotypic analysis of sopB, sopD and pipD virulence factors in Salmonella enterica serovar Typhimurium and Salmonella enterica serovar Agona. Antonie Van Leeuwenhoek 107, 23–37. doi: 10.1007/s10482-014-0300-7
Laing, C. R., Whiteside, M. D., and Gannon, V. P. J. (2017). Pan-genome analyses of the species Salmonella enterica, and identification of genomic markers predictive for species, subspecies, and serovar. Front. Microbiol. 8:1345. doi: 10.3389/fmicb.2017.01345
Maury, M. M., Tsai, Y.-H., Charlier, C., Touchon, M., Chenal-Francisque, V., Leclercq, A., et al. (2016). Uncovering Listeria monocytogenes hypervirulence by harnessing its biodiversity. Nat. Genet. 48, 308–313. doi: 10.1038/ng.3501
Oliveira, M., Wijnands, L., Abadias, M., Aarts, H., and Franz, E. (2011). Pathogenic potential of Salmonella typhimurium DT104 following sequential passage through soil, packaged fresh-cut lettuce and a model gastrointestinal tract. Int. J. Food Microbiol. 148, 149–155. doi: 10.1016/j.ijfoodmicro.2011.05.013
Osman, K. M., Marouf, S. H., Zolnikov, T. R., and AlAtfeehy, N. (2014). Isolation and characterization of Salmonella enterica in day-old ducklings in Egypt. Pathog. Glob. Health 108, 37–48. doi: 10.1179/2047773213Y.0000000118
Pielaat, A., Boer, M. P., Wijnands, L. M., van Hoek, A. H. A. M., Bouw, E., Barker, G. C., et al. (2015). First step in using molecular data for microbial food safety risk assessment; hazard identification of Escherichia coli O157:H7 by coupling genomic data with in vitro adherence to human epithelial cells. Int. J. Food Microbiol. 213, 130–138. doi: 10.1016/j.ijfoodmicro.2015.04.009
Pielaat, A., Kuijpers, A. F. A., Delfgou-van Asch, E., van Pelt, W., and Wijnands, L. M. (2016). Phenotypic behavior of 35 Salmonella enterica serovars compared to epidemiological and genomic data. Procedia Food Sci. 7, 53–58. doi: 10.1016/j.profoo.2016.02.085
Pinto, M., Robine, S., and Appay, M. D. (1983). Enterocyte-like differentiation and polarization of the human colon carcinoma cell line Caco-2 in culture. Biol. Cell 47, 323–330.
Rychlik, I., Gregorova, D., and Hradecka, H. (2006). Distribution and function of plasmids in Salmonella enterica. Vet. Microbiol. 112, 1–10. doi: 10.1016/j.vetmic.2005.10.030
Silva, C. A., Blondel, C. J., Quezada, C. P., Porwollik, S., Andrews-Polymenis, H. L., Toro, C. S., et al. (2012). Infection of mice by Salmonella enterica serovar Enteritidis involves additional genes that are absent in the genome of serovar Typhimurium. Infect. Immun. 80, 839–849. doi: 10.1128/iai.05497-11
Stanley, T. L., Ellermeier, C. D., and Slauch, J. M. (2000). Tissue-specific gene expression identifies a gene in the lysogenic phage Gifsy-1 that affects Salmonella enterica serovar Typhimurium survival in peyer’s patches. J. Bacteriol. 182, 4406–4413. doi: 10.1128/JB.182.16.4406-4413.2000
Suez, J., Porwollik, S., Dagan, A., Marzel, A., Schorr, Y. I., Desai, P. T., et al. (2013). Virulence gene profiling and pathogenicity characterization of non-typhoidal Salmonella accounted for invasive disease in humans. PLoS One 8:e58449. doi: 10.1371/journal.pone.0058449
Taboada, E. N., Graham, M. R., Carriço, J. A., and Van Domselaar, G. (2017). Food safety in the age of next generation sequencing, bioinformatics, and open data access. Front. Microbiol. 8:909. doi: 10.3389/fmicb.2017.00909
Townsend, S. M., Kramer, N. E., Edwards, R., Baker, S., Hamlin, N., Simmonds, M., et al. (2001). Salmonella enterica serovar Typhi possesses a unique repertoire of fimbrial gene sequences. Infect. Immun. 69, 2894–2901. doi: 10.1128/IAI.69.5.2894-2901.2001
van Rijckevorsel, G. G. C., Mieras, L., Bovée, L. P. M. J., van Dijk, C., Scholing, M., Swaan, C. M., et al. (2015). A foodborne outbreak of Salmonella Heidelberg in nurseries (in Dutch). Infect. Bull. 26, 118–120.
Wijnands, L. M., Teunis, P., Kuijpers, A. F. A., Delfgou-Van Asch, E. H. M., and Pielaat, A. (2017). Quantification of Salmonella survival and infection in an in vitro model of the human intestinal tract as proxy for foodborne pathogens. Front. Microbiol. 8:11398. doi: 10.3389/fmicb.2017.01139
Keywords: Bayesian approach, gastro-intestinal tract, phenotypic virulence, quantitative risk assessment, virulence genes, WGS
Citation: Kuijpers AFA, Bonacic Marinovic AA, Wijnands LM, Delfgou-van Asch EHM, van Hoek AHAM, Franz E and Pielaat A (2019) Phenotypic Prediction: Linking in vitro Virulence to the Genomics of 59 Salmonella enterica Strains. Front. Microbiol. 9:3182. doi: 10.3389/fmicb.2018.03182
Received: 13 September 2018; Accepted: 07 December 2018;
Published: 09 January 2019.
Edited by:
Jean-Christophe Augustin, École Nationale Vétérinaire d’Alfort, FranceReviewed by:
Regis Pouillot, Independent Consultant, Buenos Aires, ArgentinaNicolas Radomski, Agence Nationale de Sécurité Sanitaire de l’Alimentation, de l’Environnement et du Travail (ANSES), France
Copyright © 2019 Kuijpers, Bonacic Marinovic, Wijnands, Delfgou-van Asch, van Hoek, Franz and Pielaat. This is an open-access article distributed under the terms of the Creative Commons Attribution License (CC BY). The use, distribution or reproduction in other forums is permitted, provided the original author(s) and the copyright owner(s) are credited and that the original publication in this journal is cited, in accordance with accepted academic practice. No use, distribution or reproduction is permitted which does not comply with these terms.
*Correspondence: Annemarie Pielaat, annemarie.pielaat@unilever.com
†Present address: Angelina F. A. Kuijpers, NIZO Food Research, Ede, Netherlands; Annemarie Pielaat, Unilever R&D, Vlaardingen, Netherlands