- 1State Key Laboratory of Quality Research in Chinese Medicine, Institute of Chinese Medical Sciences, University of Macau, Macao, China
- 2School of Informatics, College of Science and Engineering, University of Edinburgh, Edinburgh, United Kingdom
Potential pathogenic factors, other than well-known APP, APOE4, and PSEN, can be further identified from transcriptomics studies of differentially expressed genes (DEGs) that are specific for Alzheimer’s disease (AD), but findings are often inconsistent or even contradictory. Evidence corroboration by combining meta-analysis and bioinformatics methods may help to resolve existing inconsistencies and contradictions. This study aimed to demonstrate a systematic workflow for evidence synthesis of transcriptomic studies using both meta-analysis and bioinformatics methods to identify potential pathogenic factors. Transcriptomic data were assessed from GEO and ArrayExpress after systematic searches. The DEGs and their dysregulation states from both DNA microarray and RNA sequencing datasets were analyzed and corroborated by meta-analysis. Statistically significant DEGs were used for enrichment analysis based on KEGG and protein–protein interaction network (PPIN) analysis based on STRING. AD-specific modules were further determined by the DIAMOnD algorithm, which identifies significant connectivity patterns between specific disease-associated proteins and non-specific proteins. Within AD-specific modules, the nodes of highest degrees (>95th percentile) were considered as potential pathogenic factors. After systematic searches of 225 datasets, extensive meta-analyses among 25 datasets (21 DNA microarray datasets and 4 RNA sequencing datasets) identified 9,298 DEGs. The dysregulated genes and pathways in AD were associated with impaired amyloid-β (Aβ) clearance. From the AD-specific module, Fyn, and EGFR were the most statistically significant and biologically relevant. This meta-analytical study suggested that the reduced Aβ clearance in AD pathogenesis was associated with the genes encoding Fyn and EGFR, which were key receptors in Aβ downstream signaling.
Introduction
Alzheimer’s disease is a neurodegenerative disease that is the major cause of dementia worldwide (Alzheimer’s Association, 2009). The AD brain is characterized by the distribution of amyloid plaques and neurofibrillary tangles, which are composed of Aβ and hyperphosphorylated tau proteins, respectively (Wang and Mandelkow, 2016; Wang J. et al., 2017). The generation of Aβ from amyloid precursor protein (APP) is a central theme in the field of AD. APP is a single transmembrane protein with a large extracellular domain that is generated in large quantities and efficiently metabolized. APP can be processed via non-amyloidogenic or amyloidogenic generation of Aβ (Chow et al., 2010). Aβ mainly exists in two forms, Aβ40 and a more hydrophobic Aβ42, which consist of 40 and 42 amino acids, respectively (Marina et al., 2003). The Aβ monomer is intrinsically disordered, and present as a dynamic conformational structure (Chen et al., 2017), which allows the monomer binds to numerous substrate with a high binding affinity. The monomers of Aβ aggregate into oligomers, fibrils, and plaques, when a critical concentration of amyloid is reached due to dysfunctional homeostasis (Knowles et al., 2014). The soluble Aβ oligomer is recognized as the major neurotoxic species, and exists in an equilibrium with fibrils (Yang et al., 2017). And the toxicity of Aβ oligomers is inversely correlated with their oligomer size (Sengupta et al., 2016). Aβ is directly neurotoxic and induces a series of cellular responses; for example, it can induce excitotoxic signaling by increasing glutamate release (Brito-Moreira et al., 2011), and alter Ca2+ homeostasis and synaptic functions in neurons, astrocytes, and microglia (Parihar and Brewer, 2010). Aβ located on the mitochondria generates ROS, thus increasing oxidative stress and disrupting oxidative phosphorylation (Caspersen et al., 2005). Aβ also activates protein kinase to phosphorylate tau protein, resulting in the formation of neurofibrillary tangles (Hernández and Avila, 2008). The toxicity of both Aβ and hyperphosphorylated tau proteins are dependent on one another; an absence of tau protein reduces Aβ-induced memory impairment, while increased Aβ levels promote tau pathology (Oddo et al., 2006; Roberson et al., 2007).
The amyloid cascade hypothesis was proposed in 1992, and depicts Aβ as the causative factor in AD development (Hardy and Higgins, 1992). This hypothesis has genetic-based support, because mutations in genes related to Aβ formation (APP and PSEN) and clearance (APOE4) induce early-onset AD (Rovelet-Lecrux et al., 2006; Kline, 2012; St George-Hyslop and Fraser, 2012). However, this hypothesis is challenged by two facts, although there are also counterarguments that have been proposed from other scientific findings. First, amyloid imaging reveals that Aβ deposition also occurs in healthy aging subjects, suggesting that Aβ deposition could be a normal phenomenon of aging (Edison et al., 2007). However, Esparza et al. (2013) suggested that Aβ oligomer levels, which are the neurotoxic form of Aβ deposition, are higher in AD brains compared with healthy subjects. Second, the degree of dementia does not correlate well with amyloid plaque formation in AD (Nelson et al., 2012). In fact, Aβ oligomers may exert their neurotoxic effects much earlier, before the formation of amyloid plaques (Broersen et al., 2010). Also, the drug development for the treatment of AD has been guided by the amyloid cascade hypothesis for the pass two decade, although most anti-Aβ drugs fail in clinical trials and no novel drugs have been brought to market since 2003 (Cummings et al., 2014). For example, Solanezumab (currently in a phase 3 trial) recognizes Aβ at the 13 to 28 amino acid position and lowers amyloid levels, but does not show beneficial effects in subjects with mild AD (Honig et al., 2018). Verubecestat (currently in a phase 3 trial), a β-secretase inhibitor, reduces Aβ levels in a dose-dependent manner (Kennedy et al., 2016). However, a recent clinical trial (Egan et al., 2019) indicated that verubecestat does not have clinical effects in AD subjects. The failure of development of anti-Aβ drugs does not discredit amyloid as the central theme for AD treatment, because the sporadic AD is caused by the changes of genes which potentiate the neurotoxicity of Aβ in the brain over years, rather than solely Aβ overproduction. Indeed, the cerebral Aβ42 kinetics were modified by Aβ accumulation (Potter et al., 2013), resulting in the disease preventative treatment required at least 95% lowering Aβ42 production if the treatment was started after Aβ accumulation (Roberts et al., 2017). This suggests that the development of anti-Aβ drugs should not be solely dependent on inhibition of Aβ42 production. However, the incomplete knowledge of AD pathogenic factors may hindered the investigation of AD (Scott et al., 2014), not just Aβ production, but also the Aβ-relevant receptors and their corresponding downstream signaling cascades.
Alzheimer’s disease is a complex disorder, expressed as a malfunction of defects in multiple genes. The discovery of AD pathogenic factors can be revealed by transcriptome profiling approach [i.e., DNA microarray and RNA-Seq (Sutherland et al., 2011)] to identify DEGs in AD subjects compared with healthy subjects. DEGs are genes that are potentially associated with disease pathology. Table 1 shows the publications using transcriptomic data and bioinformatics tools for identifying AD pathogenic factors. Puthiyedth et al. (2016) identified common DEGs from microarray datasets across six different brain regions. All datasets were integrated using the Colored (α, β)-κ Feature set approach (Puthiyedth et al., 2015), and a few common DEGs were identified across the brain regions, revealing an AD-specific signature. Kawalia et al. (2017) used the BC3Net10 algorithm to infer AD gene regulatory networks. This network was generated by the integration of literature-based knowledge and data-driven analysis. Inferences did not solely depend on the network topology, but also integrated information about DEGs and AD-related genes, after systematic searching from databases. However, the DEGs obtained from different transcriptomic studies of AD are sometimes conflicting because of many factors, such as the use of different experimental designs or statistical methods between studies. The integration of these studies based on meta-analysis therefore resolves inconclusive results and obtains a generalization (Haidich, 2010). The results of meta-analysis are presented as logORs based on the number of dysregulation events in both disease and control samples. The effect sizes are integrated to assess the overall effect size based on either fixed- or random-effect models. Meta-analysis has been applied in genomic analyses to assess the effectiveness of a particular gene in AD. Moradifard et al. (2018) conducted a meta-analysis using the R package RobustRankAggreg to identify statistically significant DEGs in AD across six microarray datasets. The DEGs were further corroborated with three RNA-Seq datasets and input for biological enrichment analysis to reveal the miRNAs that regulate the DEGs. Furthermore, Wang Q. et al. (2017) conducted a meta-analysis using the R package RankProd to explore the molecular mechanisms of AD across seven microarray datasets. They found 37 DEGs commonly shared by six brain regions, and most of these DEGs were downregulated. The significantly enriched pathways of DEGs were associated with mitochondrial oxidative phosphorylation and synaptic vesicle function. However, both studies only focused on a single microarray platform (Affymetrix), and the meta-analysis of DEGs did not include the gene expression profiles from RNA-Seq. For the study by Moradifard et al., the DEGs from RNA-Seq were only used to validate the results of microarray datasets; that is, the DEGs common to both the RNA-Seq and microarray datasets, in the same dysregulated direction. It seems that the results of currently available meta-analyses of DEGS for identifying AD pathogenic genes are limited by the use of microarray platforms and the inability to integrate results from both microarray and RNA-Seq.
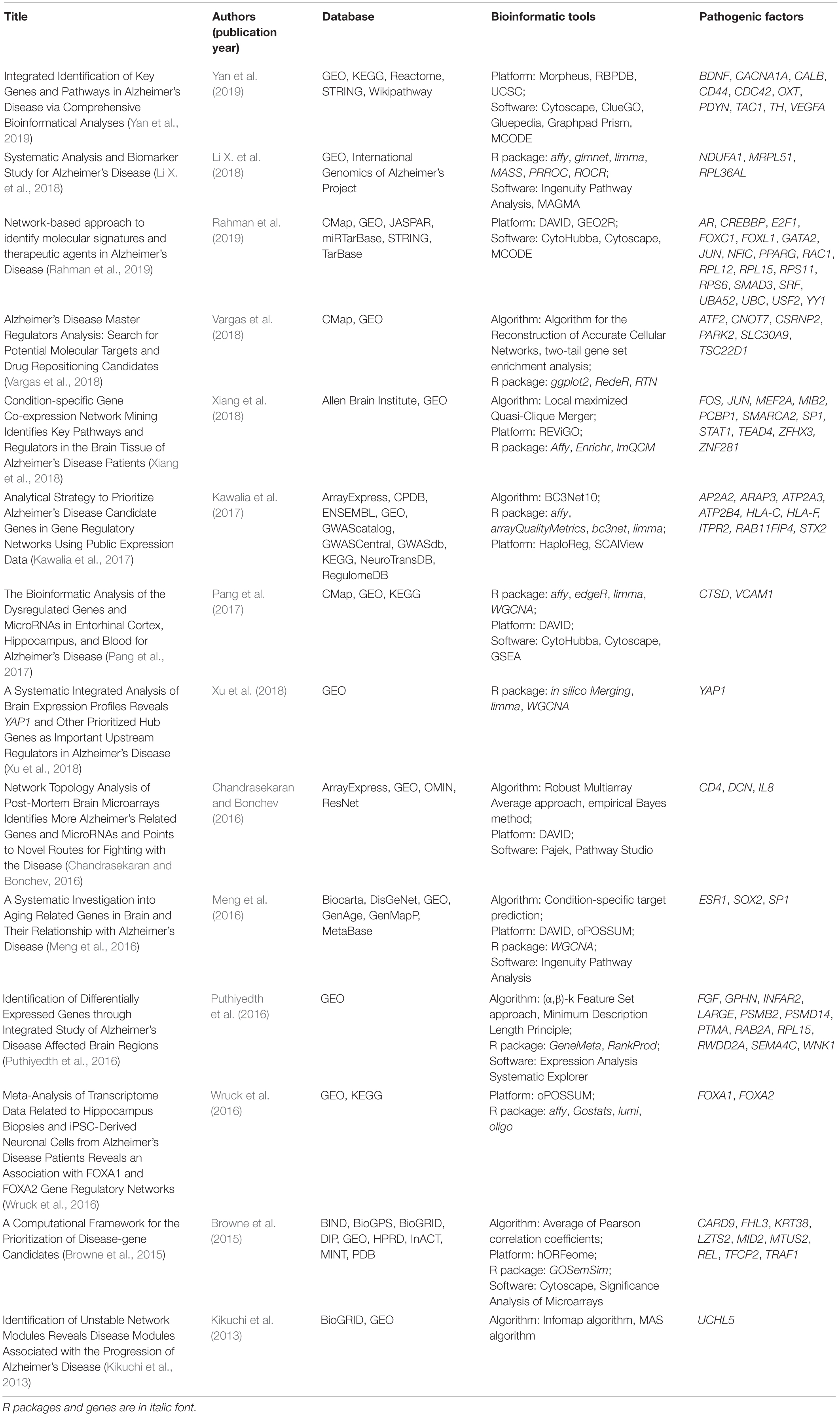
Table 1. The published transcriptomic data and bioinformatics tools for identifying AD pathogenic factors.
This study aimed to identify potential pathogenic factors based on the meta-analysis of transcriptomic data from different brain regions in AD. The meta-analysis was conducted according to PRISMA guidelines (Moher et al., 2009), including those for dataset search, dataset selection, and statistical analysis. The raw transcriptomic data were retrieved from databases after a systematic search and were analyzed using a consistent workflow, from quality control and normalization to DEG determination. A statistical meta-analysis was performed when more than one study reported the same DEG. The DEGs that remained statistically significant after P-value adjustment were collected to perform a biological enrichment analysis and subgroup analysis, and to construct a PPIN. The network information and AD seed genes, which were obtained from the GWAS Catalog (Welter et al., 2014), were collected for the DIseAse Module Detection (DIAMoND) algorithm (Ghiassian et al., 2015) to examine the significance of the connectivity patterns among network components. This algorithm identified an AD-specific module from the network, and the nodes within the module with a high degree were treated as potential pathogenic factors. The overall study design is illustrated in Figure 1.
Materials and Methods
Database Search and Dataset Selection
The datasets were collected from GEO1 (Barrett et al., 2012) and ArrayExpress2 (Kolesnikov et al., 2015) from their respective inception dates until January 6, 2019. The search strategy is shown in Supplementary File S1.
Raw DNA microarray and RNA-Seq data reporting the transcriptomes of brain regions from AD and healthy subjects were included. The datasets were excluded if they were (1) not case–control studies; (2) focused on other neurodegenerative diseases; (3) demonstrated treatment effects on AD; or (4) used cell-line or artificial AD models. Sample details were collected if they were provided. If the datasets contained several comparisons, DEGs were determined from each comparison independently.
Data Processing
Each microarray dataset was processed consistently and independently using R packages from Bioconductor. Each dataset was processed through background correction, quantile normalization, and log2-transformation of the averaged expression value of duplicate probes, using the affy (Gautier et al., 2004), oligo (Carvalho and Irizarry, 2010), or limma (Ritchie et al., 2015) package. For each dataset, the quality of each array was assessed using the arrayQualityMetrics (Kauffmann et al., 2009) package. The array was omitted from the subsequent analysis if it was detected as an outliner in any of the three metrics provided by the package. The gene expression ratios between AD and healthy subjects were determined for each dataset using the limma package. Genes with an adjusted P-value (by FDR) of less than 0.05 were considered to be statistically significant DEGs. The DEGs were annotated using the gene annotation table provided by GEO and ArrayExpress. The probes with missing gene symbols were removed. When multiple probes were annotated with the same gene symbol on the same array, the probe with the highest expression value was kept.
The raw sequence data were converted to FASTQ format using the SRA Toolkit (version 2.9.2) (Leinonen et al., 2011). Quality control on FASTQ was conducted using FastQC (version 0.11.8) (Andrews, 2010): (1) sequences with less than 80% of bases with at least quality of 25 were filtered; and (2) the bases with quality less than 25 were trimmed. The cleaner FASTQ files were aligned to the Homo.sapiens reference genome, GRCh38.94 (downloaded from Ensemble), using TopHat (version 2.1.1) (Kim et al., 2013). The aligned sequences were sorted based on the aligned position to the reference genome using SAMtools (version 1.9) (Li H. et al., 2009). After sorting, the count-based gene expression was obtained using HTSeq (version 0.8.0) (Anders et al., 2015), and the reads with alignment scores less than 10 were omitted. Differential expression was performed using the edgeR (Robinson et al., 2009) package for R software. RNA-Seq datasets were processed on a Linux-based HP ProLiant DL580 Gen8 workstation [Inter® Xeon® E7-4820 CPU v2 @ 2.00 GHz; 4 processors with 32 total cores enabled; 128 GB RAM].
Meta-Analysis, Biological Enrichment Analysis, and Subgroup Analysis
The list of DEGs and their dysregulation states (i.e., upregulated or downregulated) from each study were processed using the meta for Chung et al. (2015) package for R software. The meta-analysis was conducted under a random-effects model, and their outcomes were logORs with P-values. For each gene in the i-th study, the effect (θi) based on the numbers of dysregulation events in both AD and control samples was first calculated, then the overall effect was computed according to formula , where wi is the weight and is equal to 1/vi, where vi is the sample variance. The genes with logORs above or below 0 were considered upregulated or downregulated, respectively. The P-values were adjusted by FDR, and DEGs with adjusted P-values less than 0.05 were regarded as statistically significant. After meta-analysis, the statistically significant DEGs were used to perform a biological enrichment analysis. The enrichment analysis was conducted by the hypergeometric test using the clusterProfiler (Yu et al., 2012) package for R software, based on the KEGG3 (Kanehisa et al., 2012). The statistically significant DEGs after meta-analysis were collected as input for the enrichment analysis to identify biological pathways among them. The pathways with FDR-adjusted P-values less than 0.05 were considered statistically significant.
The DEGs were split into different subgroups based on which brain regions they were from, to compare expression profiles among brain regions. According to Roth et al. (2006), brain regions can be hierarchically clustered into three major branches based on cytological differences. The first branch includes the cerebellum. The second branch is sub-categorized into three sub-branches; the first sub-branch includes the putamen and nucleus accumbens, the second sub-branch includes the amygdala and hippocampus, and the third sub-branch includes the cerebral neocortex. The third branch includes the thalamus, brain stem, and spinal cord. Biological enrichment analysis was also conducted in each subgroup.
Protein–Protein Interaction Network and Potential Pathogenic Factors
After meta-analysis, statistically significant DEGs were used to construct a PPIN based on the data from STRING (version 114 ; Szklarczyk et al., 2011). Only interactions with the highest confidence (0.9) were kept. The PPIN was visualized using Cytoscape (Shannon et al., 2003). Disease-associated genes are not topologically interacted into a dense network module because disease can be the consequence of perturbation in many functional units. AD seed genes were retrieved from the GWAS Catalog (Welter et al., 2014)5 using the key word “AD” and the following selection criteria: a significance p-Value cutoff of no more than 1∗10–8. Genes with official gene symbols were kept as AD seed genes. The PPIN information (e.g., edge list in the co-expression network) and AD seed genes were put into the DIAMOnD algorithm (Ghiassian et al., 2015) to evaluate the significance of the connections that each node had with the seed genes in the biological network. In each iteration until the stopping condition was satisfied, the node with the lowest connectivity P-value was treated as the most significantly connected node for output. The nodes within the module that had a high degree (>95th percentile) were treated as potential pathogenic factors.
Risk of Bias
All studies were collected according to the publication information of datasets. If there was more than one publication for one dataset, the publication with the earlier publication year was selected. The risk of bias for the included studies was evaluated according to MIAME (Minimum information about a microarray experiment) (Brazma, 2009) and MINSEQE (Minimum information about a high-throughput nucleotide sequencing experiment6, proposed by FGED Society in 2012) guidelines by two authors (SY and HZ), independently. Disagreements between the authors were resolved by discussion with the third author (SWL). The MIAME and MINSEQE guidelines are designated to report the quality of DNA microarray and RNA-Seq studies, respectively. And the quality of transcriptomic parts of included studies was assessed, including raw data collection, result presentation, sample annotation, experimental design, array annotation, and data processing pipeline. Items with low risk were counted +1, suggesting high reproducibility; items with unclear risk were counted 0, suggesting ambiguous reproducibility; and items with high risk were counted –1, suggesting low reproducibility.
Results
Included Datasets
The selection process of the datasets is shown in Figure 2. A total of 225 datasets were initially identified from GEO and ArrayExpress. After removing duplicated datasets and datasets that met the exclusion criteria, 25 datasets (21 for microarray and 4 for RNA-Seq) met the eligibility criteria. The dataset characteristics are shown in Table 2. Among the included datasets, the hippocampus (n = 8) was the most extensively studied brain region, followed by the entorhinal cortex and prefrontal cortex (n = 3).
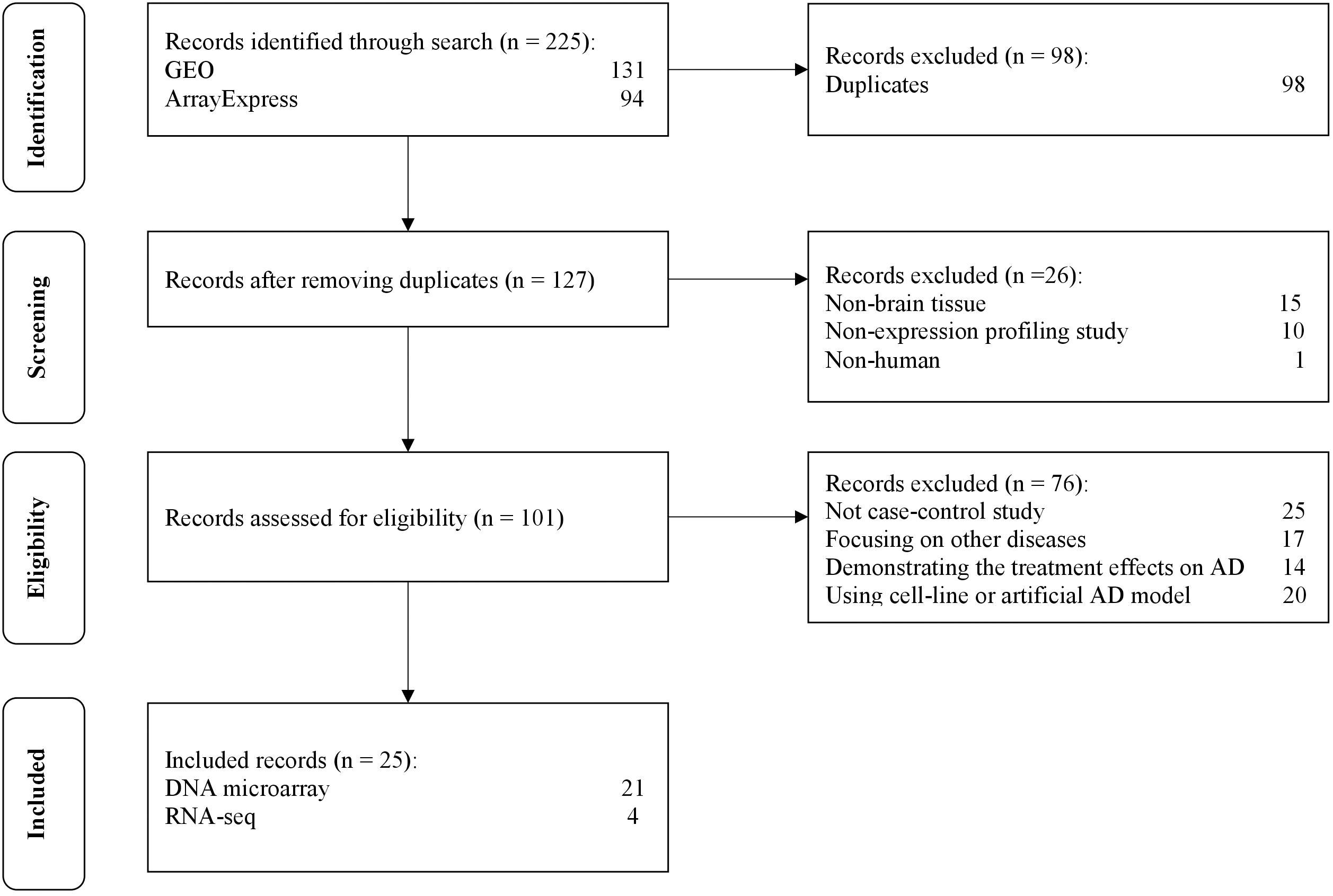
Figure 2. The flow diagram of datasets selection, including identification, screening, eligibility and inclusion stage.
Preprocessing of Datasets and Determination of Differentially Expressed Genes
After the selection process of the datasets, all included datasets contained complete transcriptomic raw data, and were undergone a quality control as a prioritization procedure. In the included microarray datasets, the quality of the normalized dataset was assessed using the R package arrayQualityMetrics. Different numbers of arrays were identified as outliers and omitted in the subsequent analysis (Table 2). In the included RNA-Seq datasets, the reads were mapped to the Homo.sapiens reference genome (GRCh38.94) after sequence quality assessment. The mapping rates were above 95% for the RNA-Seq datasets. From the mapped reads, the genes were counted to determine DEGs. After preprocessing steps, different numbers of DEGs were determined from each comparison, ranging from 0 to over 10,000 (Table 3). There were 21,064 DEGs in all comparisons that compared AD brain samples with healthy brain samples, and 16,810 DEGs (79.76%) were reported in at least two comparisons (Supplementary File S2). In the meta-analysis of DEGs, 9,298 DEGs were found to be statistically significant (Supplementary File S3); 4,960 genes were downregulated and 4,338 genes were upregulated. The most reported downregulated DEGs were DPP6 and FXYD7, which were reported in 16 comparisons; RHOQ was the most reported upregulated DEG among all 15 reported comparisons. A loss of DPP6 or FXYD7 is reported to dysregulate neuronal excitation (Hoos et al., 2013; Cacace et al., 2019), while RHOQ is reported to enhance Aβ oligomerization (Aguilar et al., 2017). Multiple DEGs involved in Aβ clearance were found statistically significant in meta-analysis (Table 4). These DEGs could be categorized into UPS, autophagy, and UPR, implying the downregulated Aβ clearance. The statistically significant DEGs in UPS and autophagy were mostly downregulated, while those in UPR were mostly upregulated, proposing a dysfunctional homeostasis. The impaired Aβ clearance resulting from dysfunctional homeostasis is suggested to induce Aβ accumulation in sporadic AD, in contrast with the overproduction of Aβ in familial AD (Mawuenyega et al., 2010; Potter et al., 2013).
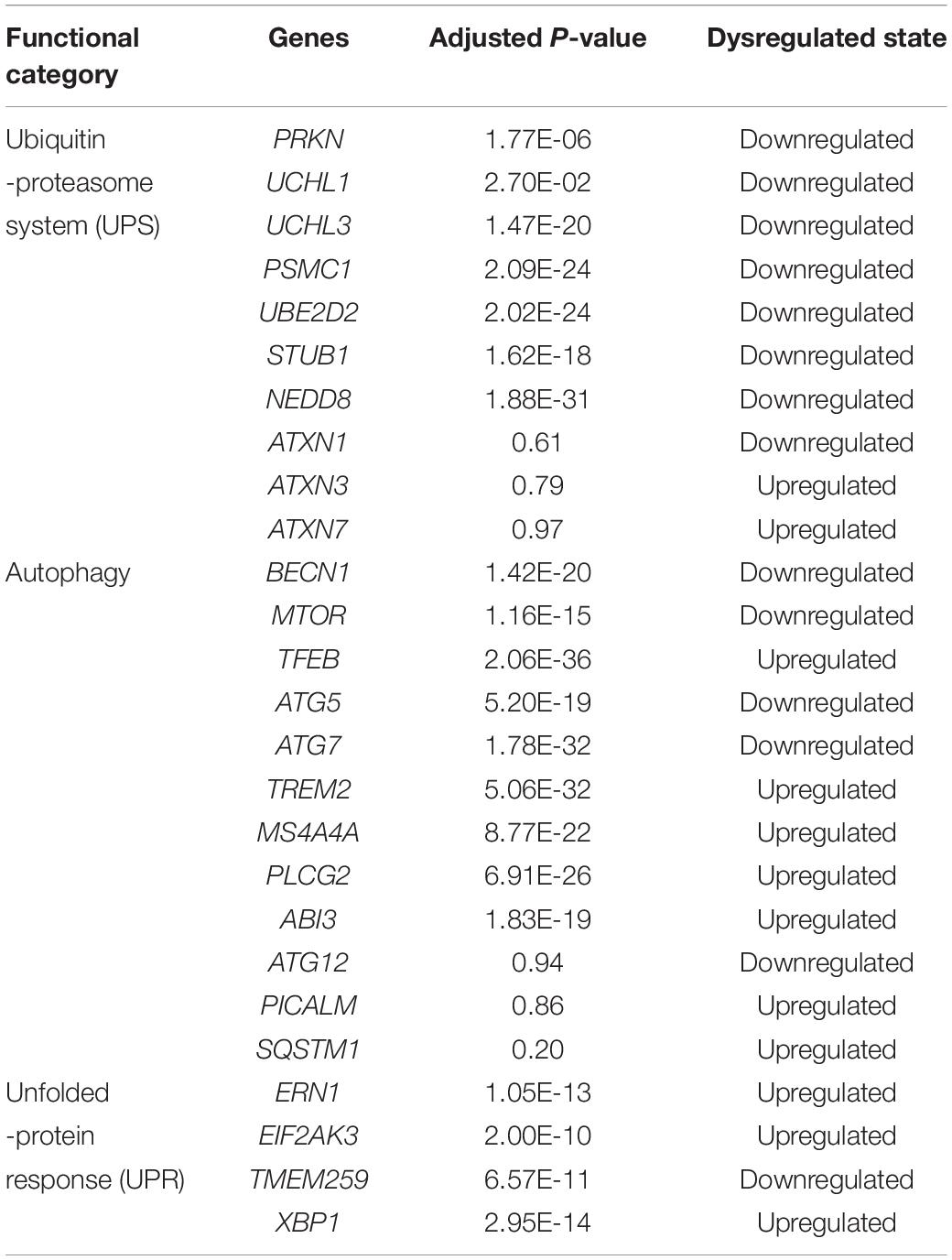
Table 4. The dysregulated status of genes involved in ubiquitin-proteasome system, autophagy, and unfolded-protein response for Aβ clearance.
However, several genes involved in Aβ clearance were not found statistically significant in meta-analysis, although they were reported as DEGs in separated comparisons. The heat shock proteins work coordinately with UPS for protein clearance, remodeling the misfolded proteins before the degradation by UPS. Both systems are upregulated to restore dysfunctional homeostasis at the beginning (Kästle and Grune, 2012). However, the genes for most heat shock proteins, such as ATXN1, ATXN3, and ATXN7, were not statistically significant (Supplementary File S3), suggesting that the early event of AD might not be truly reflected. PICALM and SQSTM1 which are involved in autophagosome formation (Moreau et al., 2014; Ntsapi and Loos, 2016), were not reported as DEGs in the meta-analysis. Several autophagic marker genes, MAP1LC3B and ATG12, associated with autophagosomal function (Ma et al., 2010), were also found as a statistically insignificant in the meta-analysis. The expression of TREM2 was upregulated, which was contradictory to the role of TREM2 reported in autophagy (Lucin et al., 2013), but consistent with that reported in Aβ plaque-activated microglia (Yuan et al., 2016; Yin et al., 2017). Meanwhile, those genes involved in Aβ plaque-activated microglia, MS4A4A, PLCG2, and ABI3, were also found significantly upregulated in the meta-analysis.
On the enrichment analysis, AD itself was identified as significant (Figure 3A). In the AD pathway, components related to the mitochondrial respiratory chain were downregulated, while components related to Ca2+ channels that transport Ca2+ from the ER into the cytoplasm were upregulated (Figure 3B). Several AD-related pathways, including proteasome, oxidative phosphorylation, and retrograde endocannabinoid signaling pathways, were also identified as statistically significant; most components in these pathways were downregulated (Figures 3C–E). Downregulation of the proteasome decreases the clearance of Aβ (Hong et al., 2014), while downregulation of oxidative phosphorylation decreases the efficacy of the mitochondrial respiratory chain, suggesting hypometabolism in the AD brain (Mosconi et al., 2008). The genes (e.g., MFN1, OPA1, and DNM1L) involved in mitochondrial fusion and fission were downregulated (Westermann, 2012), disturbing mitochondrial biogenesis to adopt energetic demands. The aberrant mitochondria were removed by the process, mitophagy. However, the genes responsible for mitophagy, PINK1 and PRKN (Narendra et al., 2008), were downregulated, which further increased the burden of damaged protein clearance. The regulation of genes involved in oxidative phosphorylation in mitochondria was severely impaired in the present study, including genes for NADH dehydrogenase, succinate dehydrogenase, cytochrome c reductase, and cytochrome c oxidase in the electron transport chain. eCBs are signaling molecules used among nearby cells over short distances, and they modulate neuronal transmission. There is a high density of cannabinoid receptors in presynaptic terminals in the hippocampus and limbic cortex, indicating a role for cannabinoids in cognitive information processing (Zou and Kumar, 2018). A disruption of eCB signaling has been reported in AD, which influences neurotransmitter release (Basavarajappa et al., 2017). In the current study, most members of the retrograde endocannabinoid signaling pathway were downregulated, interfering with the role of eCBs in the release of glutamate and GABA in synapses.
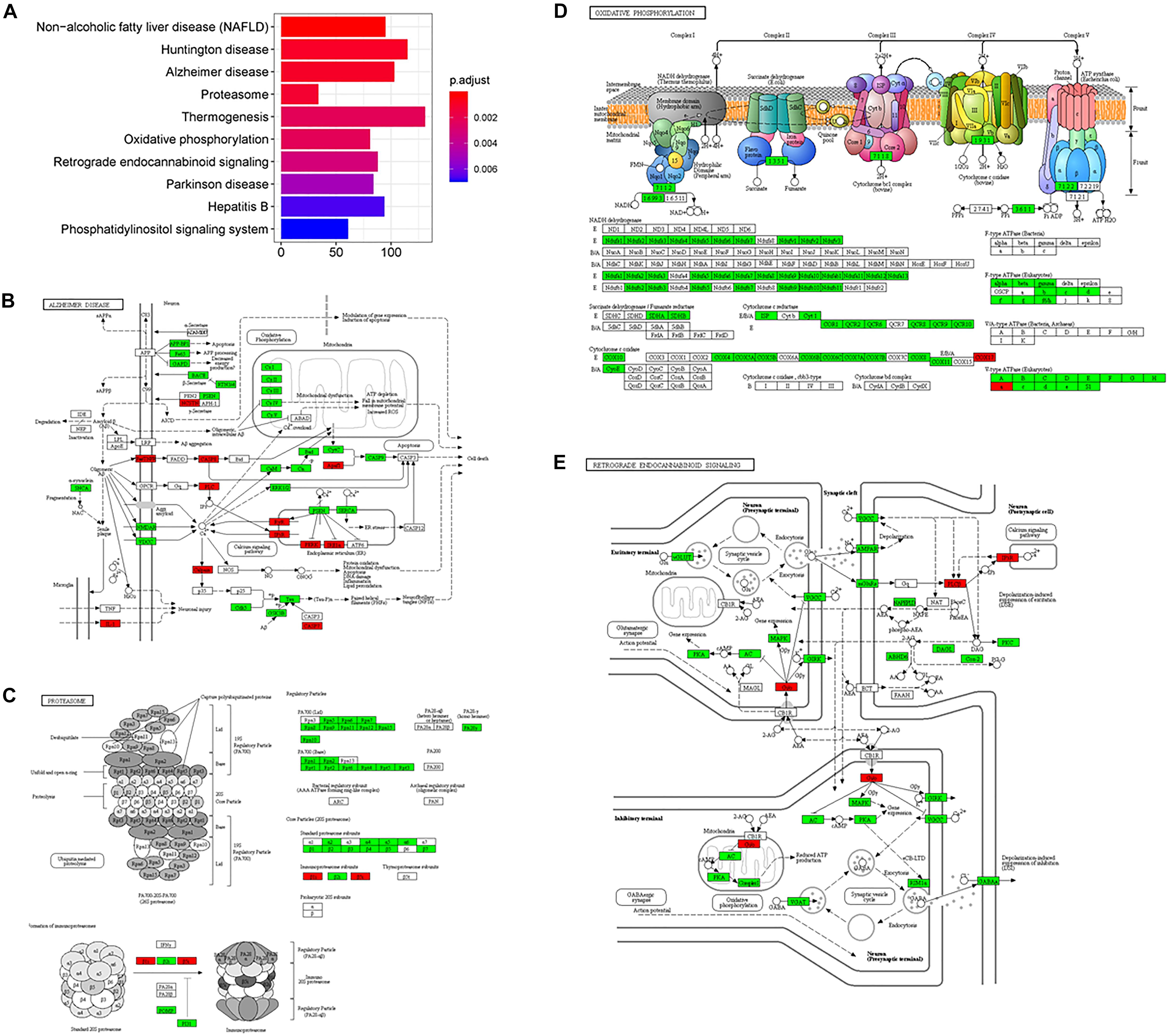
Figure 3. The AD-related biological pathways as impacted in health vs. AD subjects, (A) the top 10 statistically significant pathways from enrichment analysis result; (B) Alzheimer’s disease; (C) Proteasome; (D) Oxidative phosphorylation; and (E) Retrograde endocannabinoid signaling. For figure 3B-E, the red and green represent upregulation and downregulation, respectively.
Subgroup Analysis
Among 77 comparisons, 15 investigated the amygdala and hippocampus of AD subjects, while 28 focused on the cerebral cortex (Table 5). Statistically significant DEGs for each subgroup analysis are shown in Supplementary File S4. There were 2,260 DEGs that were reported as significant in the amygdala and hippocampus, while 6,636 DEGs were reported as significant in the cerebral cortex. Among the 1,390 commonly found DEGs, 359 upregulated and 1,020 downregulated DEGs were consistently found in two subgroups. Eleven DEGs were upregulated in one subgroup but downregulated in another subgroup. The most highly upregulated and downregulated DEGs were S100A6 and STMN4, respectively, in both subgroups. The protein calcyclin (encoded by S100A6) is a calcium-binding protein that is highly expressed in astrocytes surrounding Aβ deposits, and is involved in neuronal death via zinc depletion (Bartkowska et al., 2017). The protein stathmin (encoded by STMN4) binds tubulin to mediate microtubule dynamics, and dysregulation of stathmin-mediated microtubule stability induces memory loss (Uchida et al., 2014).
In the subgroup enrichment analyses, the pathways for proteasome and retrograde endocannabinoid signaling were found in both subgroups (Figures 4, 5). For the subgroup of the amygdala and hippocampus, the synaptic vesicle cycle was identified as statistically significant, and most components in this pathway were downregulated (Figure 4F). Downregulated synaptic functions decrease the release of neurotransmitters from neurons, inducing memory-related symptoms in AD. For the subgroup of the cerebral cortex, several synaptic signaling pathways were identified in AD, including the MAPK signaling pathway, dopaminergic synapses, and the insulin signaling pathway; the components in these pathways were mostly downregulated (Figures 5D–F). The role of the MAPK effector, JNK, is to dissociate Beclin 1 from Bcl-2 to mediate autophagy, and downregulation of JNK reduces autophagy ability (Zhou et al., 2015). The loss of dopaminergic synapses in the ventral tegmental area reduces the release of dopamine toward the cortex and hippocampus, and is reported to be associated with memory loss in AD before the formation of amyloid plaques (Nobili et al., 2017). Although all AD datasets collected in this study did not report suffering from T2DM, the dysfunctional insulin signaling was identified, suggesting an effect of Aβ in insulin signaling pathway. Aβ shares a common sequence with insulin, antagonizing the regulatory effects of insulin (Folch et al., 2018). In the canonical insulin signaling pathway, insulin binds receptors to activate Akt for mediating downstream effects, e.g., translocating glucose receptors to cellular membrane, activating mTOR for autophagy regulation, and inhibiting GSK3β for tau protein phosphorylation. Losing the regulatory effects of insulin may induce dysfunctional autophagy and tau protein hyperphosphorylation, potentiating AD development. The increased level of free insulin due to Aβ blockage reduces Aβ clearance via IDE, because insulin has a higher affinity to IDE than Aβ (Farris et al., 2003). On the other hand, the AD progression reduces the transport of insulin across BBB, decreasing insulin signaling in brain (Stanley et al., 2016).
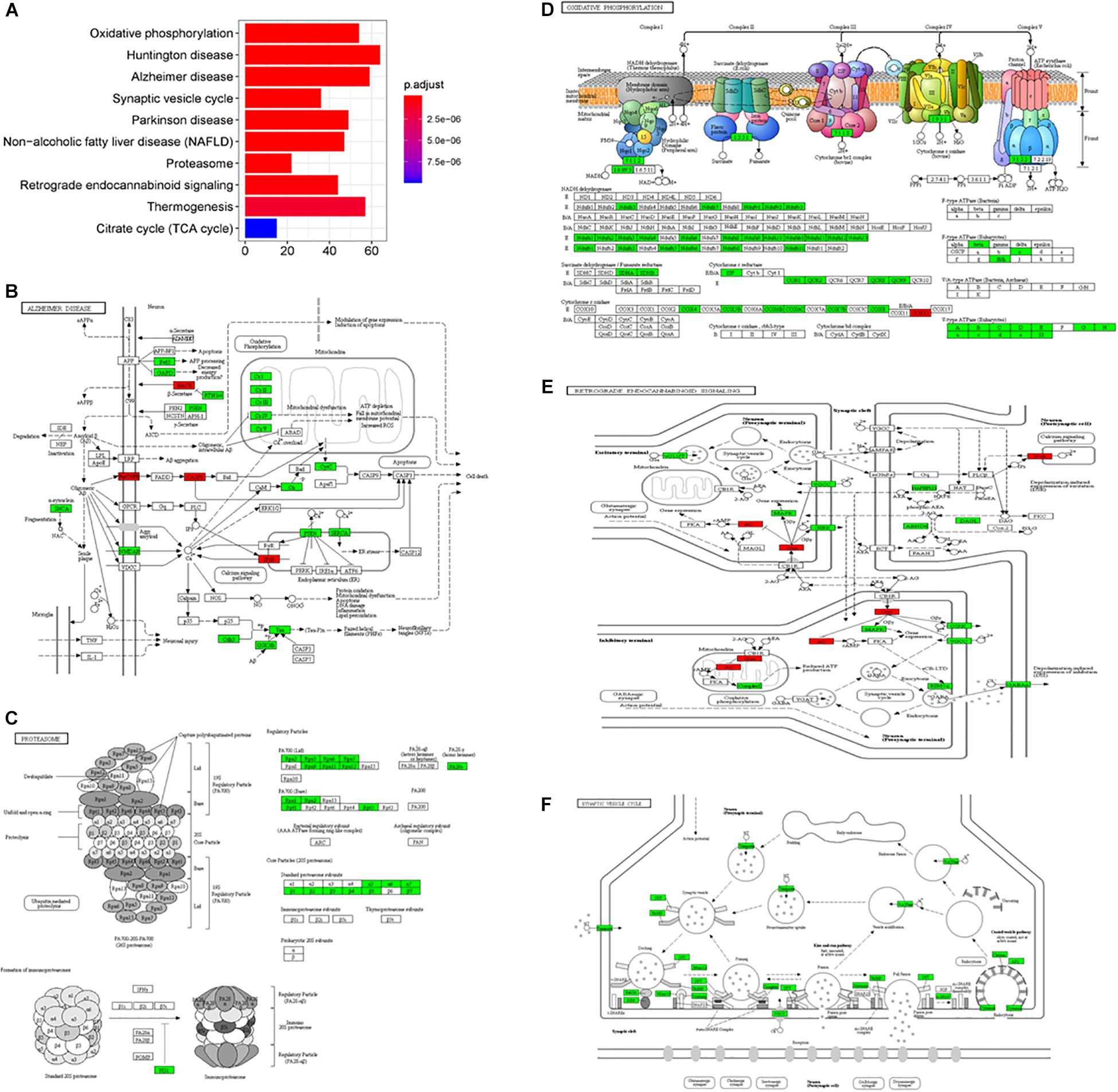
Figure 4. The AD-related biological pathways from the subgroup amydala and hippocampus, (A) the top 10 statistically significant pathways from enrichment analysis result; (B) Alzheimer’s disease; (C) Proteasome; (D) Oxidative phosphorylation; (E) Retrograde endocannabinoid signaling; and (F) Synaptic vesicle cycle. The red and green represent upregulation and downregulation, respectively.
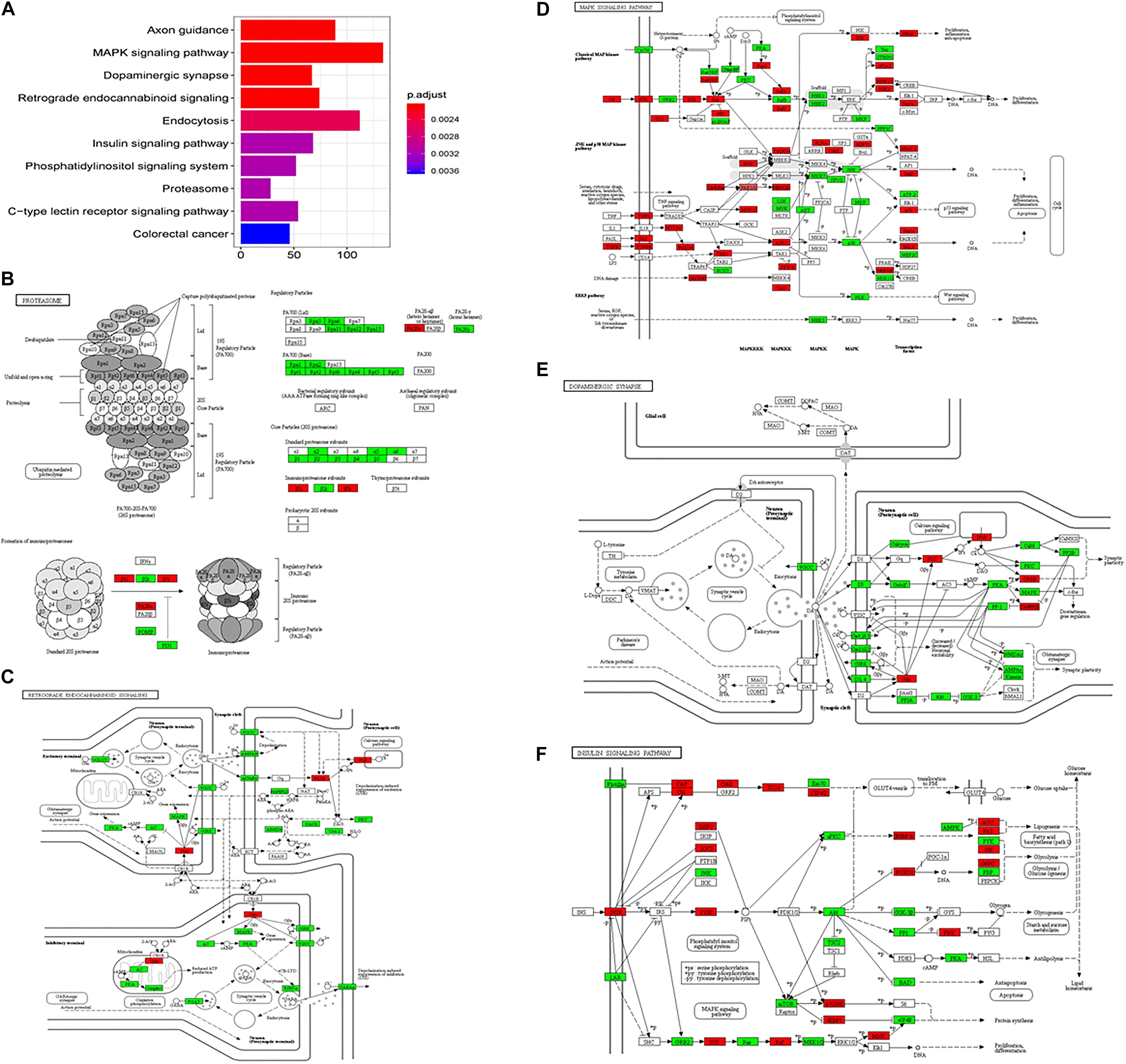
Figure 5. The AD-related biological pathways from the subgroup cerebral cortex, (A) the top 10 statistically significant pathways from enrichment analysis result; (B) Proteasome; (C) Retrograde endocannabinoid signaling; (D) MAPK signaling pathway; (E) Dopaminergic synapse; and (F) Insulin signaling pathways. The red and green represent upregulation and downregulation, respectively.
In the present study, several datasets focused on the hippocampus and entorhinal cortex because these two regions suffer the most significant neuronal loss in the early stage of AD, while no significant neuronal loss is observed in these regions during normal aging. The transcriptome from these brain regions therefore represents the chronic response to the causes and consequences, or the treatment, of AD.
Quality Assessment of Studies
MIAME and MINSEQE guidelines were used to assess the transcriptomic analysis of studies (Blalock et al., 2004, 2011; Liang et al., 2007; Berchtold et al., 2008; Bronner et al., 2009; Williams et al., 2009; Nunez-Iglesias et al., 2010; Tan et al., 2010; Durrenberger et al., 2012; Antonell et al., 2013; Fischer et al., 2013; Janssen et al., 2013; Miller et al., 2013; Zhang et al., 2013; Hokama et al., 2014; Narayanan et al., 2014; Magistri et al., 2015; Scheckel et al., 2016; Wang et al., 2016; Lachen-Montes et al., 2017; Friedman et al., 2018; Nativio et al., 2018; Stopa et al., 2018) that published the datasets that were used in the present study. The results of quality assessments are shown in Figure 6. Among the 19 studies that published the microarray datasets, 80% did not provide the brain sample size or the weight used for RNA extraction for sample annotation and experimental variables, and only two studies provided sufficient information about experimental design, including quality control of samples. Furthermore, the annotations of array designs, including microarray quality indicators, were not fully reported for most studies (95%). For 40% of the studies, experimental and data processing details, including normalization methods and cut-offs for DEGs, were not fully reported. Among the four studies that published the RNA-Seq datasets, no study provided the brain sample size or the weight used for RNA extraction, or the details of experimental parameters used for RNA-Seq procedures. Furthermore, half of the studies did not provide sufficient information about experimental and data processing protocols, including the cut-offs for DEGs.
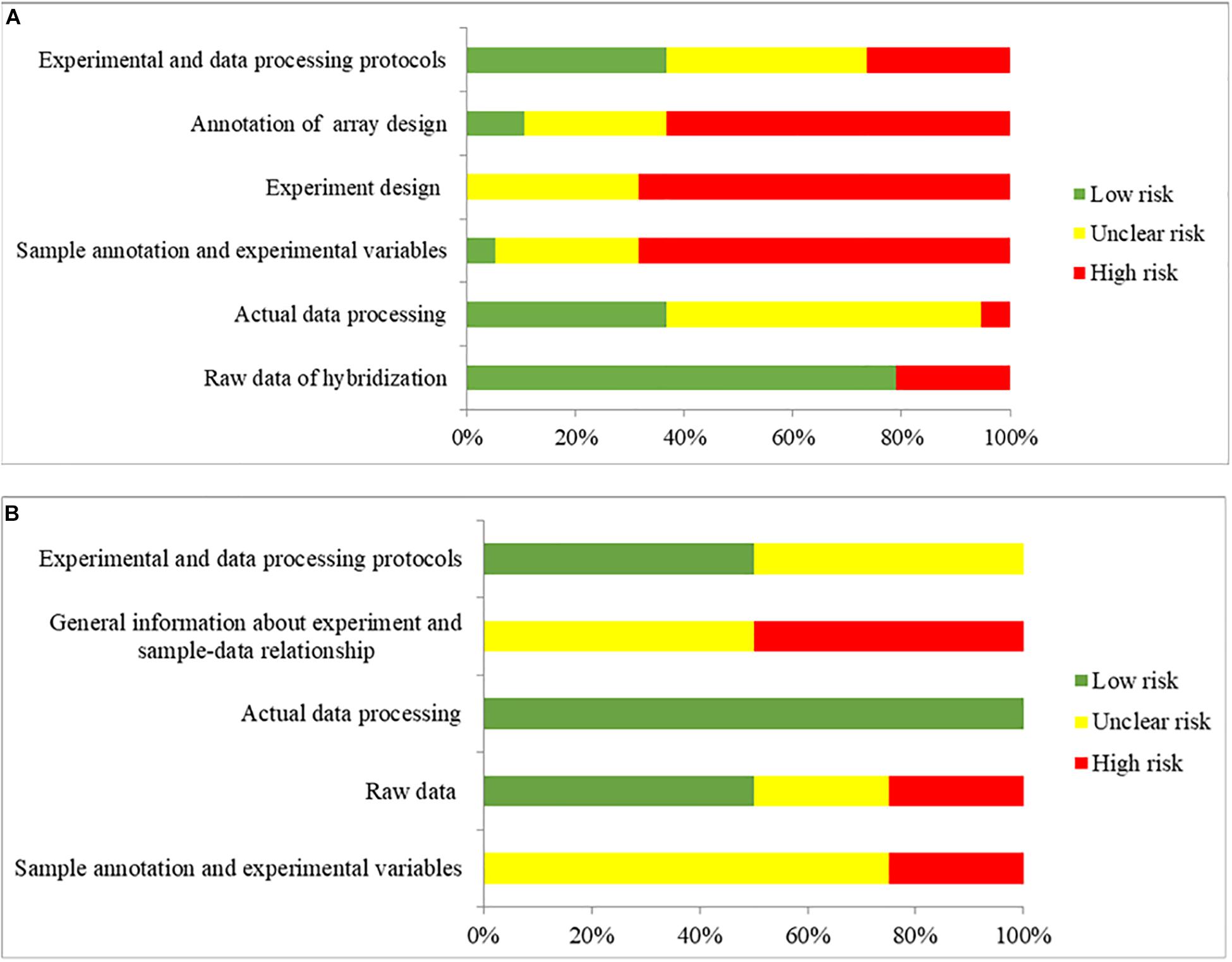
Figure 6. The overall quality assessment for (A) 19 studies for DNA microarray and (B) 4 studies for RNA-seq. Green color represents low risk of bias, which the authors clearly provided the information with full detail. Yellow color represents unclear risk of bias, which the authors provided the information without full detail. Red color represents high risk of bias, which the authors did not provide the correct information.
The relatively low quality of the studies indicates the insufficient information related to transcriptomic analysis provided in the studies, raising the reproducibility issue. Also, the transcriptomic results might be influenced, blurring some pathological events in the postmortem AD brains. The genes encoded heat shock proteins (e.g., ATXN1, ATXN3, and ATXN7) were not found statistically significant in the meta-analysis, while the multiple genes involved in neuroinflammation (e.g., CXCL3, IFNG, IL6, IL13, and CXCL9) (Garwood et al., 2011) were only reported as DEGs in one dataset, and therefore were not collected for meta-analysis. Although both heat shock proteins recruitment and neuroinflammation are associated with AD development, their pathological roles in AD development might not fully reflected in this study.
Construction of a Protein–Protein Interaction Network to Identify Potential Pathogenic Factors
The DEGs from the meta-analysis were used to retrieve the corresponding proteins in interactions according to STRING. The PPIN consisted of 4,781 nodes (2,083 upregulated nodes and 2,698 downregulated nodes) and 51,076 edges (Figure 7A) and there were 105 AD seed genes (Supplementary File S5) retrieved from the GWAS Catalog. The PPIN exhibited scale free topology with the degree distribution following a power law distribution (y = 3779.2x–1.481) and the most proteins were closely linked. In the PPIN, polyubiquitin-C (endcoded by UBC) and E3 ubiquitin-protein ligase RBX1 (encoded by RBX1), were the two proteins with the highest degree (372 and 222, respectively). These proteins were downregulated and are parts of UPS for protein degradation. The dysfunctional proteasome is regarded as an early event of AD (Manavalan et al., 2013), e.g., the decreased proteasomal clearance enhances mitochondrial dysfunction (Braun et al., 2015).
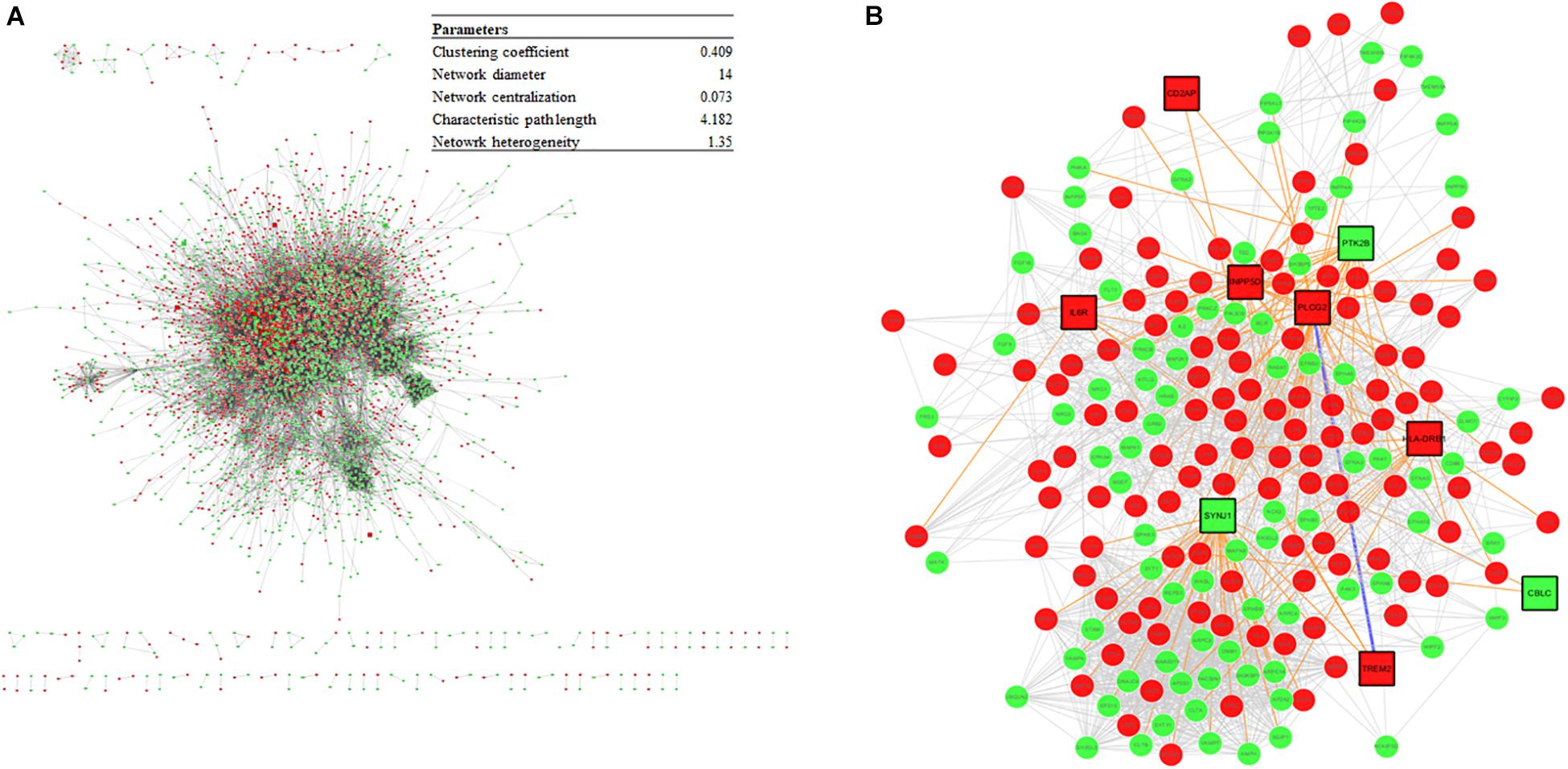
Figure 7. (A) The PPIN constructed from DEGs and the PPIN topology parameters. The red and green represent upregulation and downregulation, respectively. (B) A disease module, derived from the DIAMOnD algorithm, consisting of AD seed proteins (represented as squares) and their corresponding DIAMOnD proteins (represented as circles). The colors of the nodes represent upregulation (red), and downregulation (green). The edges represent seed-seed interaction (dark green), seed-DIAMOnD interaction (purple), and DIAMOnD-DIAMOnD interaction (pink).
The PPIN for each protein pair was extracted for the subsequent DIAMOnD algorithm with AD seed genes to obtain a disease module. Not every seed gene was biologically linked to AD; some were involved in AD comorbidity disease. Among the disease modules (Figure 7B), the two nodes with a high degree (>95th percentile) were selected as potential pathogenic factors, and included EGFR and Fyn. The EGFR was the Aβ oligomer receptor, in which Aβ oligomer is regarded as the causative toxic species. The hydrophobic residues of Aβ oligomers are more easily accessible and interacting with cellular proteins, compared with Aβ fibrils (William et al., 2011). Fyn is involved in Aβ signal transduction and tau protein phosphorylation, and is changed in synaptic signaling pathways, including MAPK signaling pathway. These two proteins are both Aβ-relevant receptors, mediating the Aβ oligomers downstream neurotoxicity.
Discussion
We used a meta-analysis approach based on DNA microarray and RNA-Seq datasets from multiple brain regions to reveal potential pathogenic factors of AD. Perturbations in the genes involved in the proteasome, oxidative phosphorylation, and retrograde endocannabinoid signaling pathways were identified. The proteasome is required to degrade the damaged proteins to maintain the cellular functionality. The decreased proteasome activity results in an accumulation of ubiquitinated and damaged proteins (Ciechanover, 2015). The ubiquitination degradation of APP by the proteasome includes several key genes, such as PRKN, NEDD8, PSMC1, UCHL1, and UCHL3 (Bedford et al., 2008; Chen et al., 2012; Zhang et al., 2015; Nomura et al., 2016). Tau-specific ubiquitin–proteasome related genes, including UBE2D2 (Shimura et al., 2004) and STUB1 (Saidi et al., 2015), were also downregulated. The downregulation of these genes might result in impaired Aβ and phosphorylated tau protein clearance (Table 4). The oligomeric Aβ itself disrupts the catalytic activity of proteasome (Tseng et al., 2008), forming a positive feedback loop to worsen the Aβ clearance by proteasome. The disrupted catalytic activity of proteasome may also lose its inhibition of presenilin complex, which is the catalytic complex for γ-secretase, enhancing Aβ production (Chadwick et al., 2012). The accumulated Aβ, located on the mitochondria, deleteriously impairs oxidative phosphorylation, decreasing ATP production in the mitochondria (Chen and Du Yan, 2007; Moreira et al., 2010). The ATP-dependent proteins (i.e., the Ca2+ pumps located on the endoplasmic reticulum (ER) and ATP-dependent ion pumps) are unable to maintain their physiological functions, interfering neurotransmitter release and memory formation (Sudhof, 2012). The ATP-dependent molecular chaperones are also failed to work coordinately with UPS to remodel the misfolded proteins (Mattoo and Goloubinoff, 2014).
The reduced activity of proteasome enhances the burden of Aβ clearance on the autophagy and UPR. Autophagy is the process of degrading redundant cellular components by delivering them to lysosomes and forming autophagosomes. Beclin 1 (encoded by BECN1) is an essential component of autophagososomal structure (Jaeger and Wyss-Coray, 2010), but its autophagy function is inhibited by interacting with Bcl-2 (encoded by BCL2) leading to cellular death (Marquez and Xu, 2012). The downregulation of BECN1 and upregulation of BCL2 in the present study indicated autophagic flux failure. Autophagy is inhibited by the upregulation of mTOR and activated by the downregulation of TFEB (Caccamo et al., 2010; Polito et al., 2014). The downregulation of mTOR and upregulation of TFEB in the present study induced the formation of immature autophagosomes, impairing Aβ clearance. In addition, the downregulation of mTOR is associated with AD development (François et al., 2014), memory impairment (Slipczuk et al., 2009), and neuronal apoptosis (Yin et al., 2011). The depletion of autophagy-related genes, including Atg5 and Atg17, impairs the elongation of phagopores to form autophagosomes, resulting in Aβ accumulation (Hara et al., 2006; Nilsson et al., 2013). Accumulated Aβ on the ER membrane induces ER stress, which is relieved by the activation of UPR. The activation of UPR is induced by the accumulation of misfolded proteins (Stutzbach et al., 2013), and has been reported in AD subjects (Scheper and Hoozemans, 2015). Two main UPR proteins, including PERK (encoded by EIF2AK3) and IRE-1 (encoded by ERN1), were upregulated and activated in AD due to misfolded Aβ oligomers (Joshi et al., 2016), although a recent critical review suggested that the involvement of ER stress in AD might be exaggerated by the misuse of APP/PS1-overexpressing AD mouse models (Hashimoto and Saido, 2018). The activation of UPR is a significant approach to prevent the accumulation of misfolded proteins, but may also increase Aβ production (Scheper and Hoozemans, 2015), and result in synaptic loss (Gong et al., 2016). Taken together, disturbed Aβ clearance could be the upstream event for AD pathological development, to induce Aβ accumulation, altering intracellular ionic gradients and resulting in insufficient oxidative phosphorylation.
The two top pathogenic factors determined in the present study, Fyn and EGFR, are key receptors in Aβ downstream signaling. Fyn is a protein tyrosine kinase belonging to the Src family, and it mediates synaptic plasticity in the central nervous system (Kaufman et al., 2015). The soluble Aβ oligomer binds to PrPC with high affinity (Laurén et al., 2009), and interrupts the PrPC’s autoinhibitory mechanism (Wu et al., 2017), overactivating Fyn to increase glutamate release (Trepanier et al., 2012). Overactivation of Fyn, however, alters NMDA receptor function and intracellular Ca2+ homeostasis, rendering neurons vulnerable to Aβ-induced neurotoxicity. The Aβ-activated Fyn can phosphorylate downstream tau proteins at Tyr18 (Lee, 2004), and tau proteins participate in transporting Fyn to post-synaptic densities around glutamate receptors (Ittner et al., 2010). Fyn is a key protein that links between extracellular Aβ and intracellular tau protein, and unites these two pathologies in AD. The activity of Fyn is gene-dose-dependent (Um et al., 2012); that is, downregulation of FYN reduces Aβ-induced neurotoxicity, while upregulation of FYN exacerbates Aβ-induced neurotoxicity. In previous studies, overexpression of FYN has also been reported to accelerate synaptic loss, making Fyn inhibition a potential therapeutic treatment for AD (Chin et al., 2004; Chin, 2005). Saracatinib (AZD0530) is a Src kinase inhibitor that is being tested clinically for the treatment of AD. Saracatinib blocks Fyn activation to rescue memory deficits, and exhibits well-tolerated effects in mild-to-moderate AD subjects (Nygaard et al., 2015). A recently published clinical trial (VanDyck et al., 2019) revealed that saracatinib does not slow the decline in the CMRgl, and does not exhibit beneficial effects on several cognitive assessments, compared with the placebo group. However, a trend for slowing the reduction of volumetric measure in brain is observed, and a post hoc exploratory analysis deduces a statistically significant decline in CMRgl in the entorhinal cortex. Although the frequent adverse event of saracatinib, e.g., diarrhea, may limit the feasibility of increasing 125 mg daily to a higher dosage, the authors proposed that a higher dosage of saracatinib may be beneficial to slow decline of CMRgl in AD participants, who have greater tolerability.
EGFR is more commonly associated with cancer than with AD. However, the expression of EGFR is statistically correlated with the expression of γ-secretase, suggesting a significant role of EGFR in AD (Zhang et al., 2007). EGFR is widely distributed in the hippocampus and cerebral cortex (Fu et al., 2003), and EGFR signaling exerts neurotrophic functions to regulate synaptic architecture in the central nervous system (Oyagi et al., 2011). The expression of EGFR is reported to be upregulated in the brains of AD animals, and overactivation of EGFR by Aβ oligomers induces memory loss (Wang et al., 2012). The overactivation of EGFR can also be induced by the prolong UPR (Papa and Germain, 2011). The levels of overactivated EGFR can be suppressed by treatment with the EGFR inhibitor gefitnib to rescue memory loss. Activation of EGFR also induces downstream neuroinflammatory cascades in response to Aβ-induced neurotoxicity. Afatinib, an orally available EGFR tyrosine kinase inhibitor, inhibits EGFR activation to alleviate neuroinflammation by reducing caspase 1 activation and interleukin-1β levels in neurodegenerative diseases (Chen et al., 2019). Antagonizing Aβ-induced activation of EGFR may have beneficial effects to slow memory loss and alleviate neuroinflammation. Figure 8 summarizes the mechanism of action of Aβ-mediated Fyn and EGFR in AD, as described above.
Besides the genes involved in Aβ downstream neurotoxicity, the genes related to amyloid clearance proteins (e.g., ECE1 and NEU1) are reported as pathogenic factors of AD by other studies. Endothelin 1 (encoded by ECE1) is implicated in Aβ degradation and downregulation of ECE1 enhances Aβ deposition (Pacheco-Quinto and Eckman, 2013). However, upregulation of ECE1 was found in the present study, suggesting that the pathophysiological role of ECE1 was not solely involved in Aβ metabolism, but also associated with the increase Aβ-induced vasoconstriction in AD (Palmer et al., 2013). Sialidase (encoded by NEU1) is a lysosomal enzyme for Aβ clearance through lysosomal exocytosis (Annunziata et al., 2013). Deficit of NEU1 causes Aβ-induced proteasome inhibition, which was consistent with our results. Disruption of Aβ clearance proteins may be the secondary events of AD progression, rather than the primary cause. Increasing evidences show a correlation between T2DM and higher risk of developing AD, through increasing BBB permeability by chronic peripheral inflammation (Holmes et al., 2009). The BBB leakage was supported by the dysregulated genes in this study, including OCLN, TJP1, CDH5, and CTNNB1, which encode the tight junction proteins. The compromised BBB can be exacerbated by chronic hypertension (Santos et al., 2017) and microbial pathogens (Li H. et al., 2018), and alters peripheral immune cell trafficking to the brain and induces neuroinflammation. The neuroinflammation is mediated by p38 (encoded by MAPK14), which is a protein found in MAPK pathway. p38 is also a kinase to phosphorylate tau protein, making p38 as a potential pathogenic factor of AD. However, upregulation of p38 was not observed in this study, since p38 participates in numerous cellular events not only neuroinflammation. This can be supported by that although the inhibition of p38 alleviates neuroinflammation in AD (Yasuda et al., 2012; Ashabi et al., 2013), most p38 inhibitors are failed in clinical trials due to off-target effects. A gene converting glucose into lactate in glycolysis, LDHA, is regarded as a relevant pathogenic factor in AD, although it may not be specific to AD pathogenesis. Overexpression of LDHA is reported to prevent neurons from Aβ neurotoxicity by shifting mitochondrial glucose metabolism to lactate production, and decreasing ROS production (Newington et al., 2012). However, elevated lactate level may be detrimental to neurons (Harris et al., 2016). Although the expression of LDHA was insignificantly downregulated in this study, the opposing neuroprotective and neurotoxic effects of LDHA suggested that neurons might have its own defense mechanism against Aβ in the early onset of AD.
The strength of this study is the integration of transcriptomic data from both DNA microarrays and RNA-Seq using a systematic search and consistent workflow. However, there are some limitations. First, the articles that published the datasets did not provide full details of the experimental procedures, making the transcriptomic results less reliable and more difficult to repeat. In addition, the transcriptomic data retrieved from postmortem brain tissue using different methods might be biased. For example, laser-microdissection mainly targets neuronal soma, but may miss transcripts transporting to the pre- or post-synapses. Lacking of sufficient information about disposal of transcriptomic analysis, resulting in relatively low quality of the selected studies, might raise a reproducibility issue. Second, the confounding factors of postmortem brain tissues include age, sex, and postmortem interval, and may blur the results, e.g., neuroinflammation. Third, most included datasets in this study focused on hippocampus and entorhinal cortex, in which the brain region AD are believed to start. However, AD might start differently from those two brain regions, e.g., the impaired UPS as an early AD event was reported in brainstem (Irmler et al., 2012). Transcriptomic analysis in different regions of postmortem AD brains would provide more novel insights of AD development.
Conclusion
This meta-analytical study suggested that the reduced Aβ clearance in AD pathogenesis was associated with the genes encoding Fyn and EGFR, which were key receptors in Aβ downstream signaling.
Data Availability Statement
The raw data of this paper are available in the public database, GEO and ArrayExpress. The data that support the findings of this paper are available in the published article and its additional files.
Author Contributions
SL and SY conceived and designed the study and wrote the manuscript. SY and HZ extracted and analyzed the data. SL and HZ verified the data and revised the manuscript. All authors read and approved the final version of the manuscript.
Funding
Part of this authors’ work and publication fees of this paper were supported by a grant (MYRG2019-00159-ICMS) received from the University of Macau.
Conflict of Interest
The authors declare that the research was conducted in the absence of any commercial or financial relationships that could be construed as a potential conflict of interest.
Acknowledgments
We thank Bronwen Gardner, Ph.D., from the Edanz Group (www.edanzediting.com/ac) for editing a draft of this manuscript.
Supplementary Material
The Supplementary Material for this article can be found online at: https://www.frontiersin.org/articles/10.3389/fnins.2020.00209/full#supplementary-material
FILE S1 | The search strategy used in the database GEO and ArrayExpress.
FILE S2 | The DEGs from each comparison.
FILE S3 | The DEGs after meta-analysis found statistically significant in this study.
FILE S4 | The statistical significant DEGs found in each subgroup.
FILE S5 | The AD seed genes used in the DIAMOnD algorithm.
Abbreviations
Aβ, amyloid- β; AD, Alzheimer’s disease; BBB, blood-brain barrier; CMRgl, cerebral metabolic rate for glucose; DEGs, differentially expressed genes; DIAMoND, DIseAse Module Detection algorithm; eCBs, endocannabinoids; FDR, false discovery rate; IDE, insulin-degrading enzyme; KEGG, Kyoto Encyclopedia of Genes and Genomes; logORs, loge odds ratios; PPIN, protein–protein interaction network; PrPC, cellular prion protein; ROS, reactive oxygen species; RNA-Seq, RNA-sequencing; T2DM, type 2 diabetes mellitus; UPR, unfolded-protein response; UPS, ubiquitin-proteasome system.
Footnotes
- ^ https://www.ncbi.nlm.nih.gov/geo
- ^ https://www.ebi.ac.uk/arrayexpress/
- ^ http://www.genome.jp/kegg/
- ^ https://string-db.org/
- ^ https://www.ebi.ac.uk/gwas/
- ^ http://fged.org/projects/minseqe/
References
Aguilar, B. J., Zhu, Y., and Lu, Q. (2017). Rho GTPases as therapeutic targets in Alzheimer’s disease. Alzheimers. Res. Ther. 9:97. doi: 10.1186/s13195-017-0320-4
Anders, S., Pyl, P. T., and Huber, W. (2015). HTSeq–a Python framework to work with high-throughput sequencing data. Bioinformatics 31, 166–169. doi: 10.1093/bioinformatics/btu638
Andrews, S. (2010). FastQC: A Quality Control Tool for High Throughput Sequence Data. Available online at: http://www.bioinformatics.babraham.ac.uk/projects/fastqc.
Annunziata, I., Patterson, A., Helton, D., Hu, H., Moshiach, S., Gomero, E., et al. (2013). Lysosomal NEU1 deficiency affects amyloid precursor protein levels and amyloid-β secretion via deregulated lysosomal exocytosis. Nat. Commun. 4:2734. doi: 10.1038/ncomms3734
Antonell, A., Lladó, A., Altirriba, J., Botta-Orfila, T., Balasa, M., Fernández, M., et al. (2013). A preliminary study of the whole-genome expression profile of sporadic and monogenic early-onset Alzheimer’s disease. Neurobiol. Aging 34, 1772–1778. doi: 10.1016/j.neurobiolaging.2012.12.026
Ashabi, G., Alamdary, S. Z., Ramin, M., and Khodagholi, F. (2013). Reduction of hippocampal apoptosis by intracerebroventricular administration of extracellular signal-regulated protein kinase and/or p38 inhibitors in amyloid beta rat model of Alzheimer’s disease: involvement of nuclear-related factor-2 and nuclear factor-kB. Basic Clin. Pharmacol. Toxicol. 112, 145–155. doi: 10.1111/bcpt.12000
Alzheimer’s Association, (2009). 2009 Alzheimer’s disease facts and figures. Alzheimers Dement. 5, 234–270. doi: 10.1016/j.jalz.2009.03.001
Barrett, T., Wilhite, S. E., Ledoux, P., Evangelista, C., Kim, I. F., Tomashevsky, M., et al. (2012). NCBI GEO: archive for functional genomics data sets—update. Nucleic Acids Res. 41, D991–D995. doi: 10.1093/nar/gks1193
Bartkowska, K., Swiatek, I., Aniszewska, A., Jurewicz, E., Turlejski, K., Filipek, A., et al. (2017). Stress-dependent changes in the CacyBP/SIP interacting protein S100A6 in the mouse brain. PLoS One 12:e0169760. doi: 10.1371/journal.pone.0169760
Basavarajappa, B. S., Shivakumar, M., Joshi, V., and Subbanna, S. (2017). Endocannabinoid system in neurodegenerative disorders. J. Neurochem. 142, 624–648. doi: 10.1111/jnc.14098
Bedford, L., Hay, D., Devoy, A., Paine, S., Powe, D. G., Seth, R., et al. (2008). Depletion of 26S proteasomes in mouse brain neurons causes neurodegeneration and lewy-like inclusions resembling human pale bodies. J. Neurosci. 28, 8189–8198. doi: 10.1523/JNEUROSCI.2218-08.2008
Berchtold, N. C., Cribbs, D. H., Coleman, P. D., Rogers, J., Head, E., Kim, R., et al. (2008). Gene expression changes in the course of normal brain aging are sexually dimorphic. Proc. Natl. Acad. Sci. U.S.A. 105, 15605–15610. doi: 10.1073/pnas.0806883105
Blalock, E. M., Buechel, H. M., Popovic, J., Geddes, J. W., and Landfield, P. W. (2011). Microarray analyses of laser-captured hippocampus reveal distinct gray and white matter signatures associated with incipient Alzheimer’s disease. J. Chem. Neuroanat. 42, 118–126. doi: 10.1016/j.jchemneu.2011.06.007
Blalock, E. M., Geddes, J. W., Chen, K. C., Porter, N. M., Markesbery, W. R., and Landfield, P. W. (2004). Incipient Alzheimer’s disease: microarray correlation analyses reveal major transcriptional and tumor suppressor responses. Proc. Natl. Acad. Sci. U.S.A. 101, 2173–2178. doi: 10.1073/pnas.0308512100
Braun, R. J., Sommer, C., Leibiger, C., Gentier, R. J. G., Dumit, I., Paduch, K., et al. (2015). Accumulation of basic amino acids at mitochondria dictates the cytotoxicity of aberrant ubiquitin. Cell Rep. 10, 1557–1571. doi: 10.1016/j.celrep.2015.02.009
Brazma, A. (2009). Minimum information about a microarray experiment (MIAME)–successes, failures, challenges. ScientificWorldJournal 9, 420–423. doi: 10.1100/tsw.2009.57
Brito-Moreira, J., Paula-Lima, A. C., Bomfim, T. R., Oliveira, F. B., Sepulveda, F. J., De Mello, F. G., et al. (2011). Aβ oligomers induce glutamate release from hippocampal neurons. Curr. Alzheimer Res. 8, 552–562. doi: 10.2174/156720511796391917
Broersen, K., Rousseau, F., and Schymkowitz, J. (2010). The culprit behind amyloid beta peptide related neurotoxicity in Alzheimer’s disease: oligomer size or conformation? Alzheimers. Res. Ther. 2:12. doi: 10.1186/alzrt36
Bronner, I. F., Bochdanovits, Z., Rizzu, P., Kamphorst, W., Ravid, R., vanSwieten, J. C., et al. (2009). Comprehensive mRNA expression profiling distinguishes tauopathies and identifies shared molecular pathways. PLoS One 4:e6826. doi: 10.1371/journal.pone.0006826
Browne, F., Wang, H., and Zheng, H. (2015). A computational framework for the prioritization of disease-gene candidates. BMC Genomics 16(Suppl. 9):S2. doi: 10.1186/1471-2164-16-S9-S2
Cacace, R., Heeman, B., VanMossevelde, S., DeRoeck, A., Hoogmartens, J., DeRijk, P., et al. (2019). Loss of DPP6 in neurodegenerative dementia: a genetic player in the dysfunction of neuronal excitability. Acta Neuropathol. 137, 901–918. doi: 10.1007/s00401-019-01976-3
Caccamo, A., Majumder, S., Richardson, A., Strong, R., and Oddo, S. (2010). Molecular interplay between mammalian target of rapamycin (mTOR), amyloid-β, and tau. J. Biol. Chem. 285, 13107–13120. doi: 10.1074/jbc.M110.100420
Carvalho, B. S., and Irizarry, R. A. (2010). A framework for oligonucleotide microarray preprocessing. Bioinformatics 26, 2363–2367. doi: 10.1093/bioinformatics/btq431
Caspersen, C., Wang, N., Yao, J., Sosunov, A., Chen, X., Lustbader, J. W., et al. (2005). Mitochondrial Aβ: a potential focal point for neuronal metabolic dysfunction in Alzheimer’s disease. FASEB J. 19, 2040–2041. doi: 10.1096/fj.05-3735fje
Chadwick, L., Gentle, L., Strachan, J., and Layfield, R. (2012). Review: Unchained maladie – a reassessment of the role of Ubb +1-capped polyubiquitin chains in Alzheimer’s disease. Neuropathol. Appl. Neurobiol. 38, 118–131. doi: 10.1111/j.1365-2990.2011.01236.x
Chandrasekaran, S., and Bonchev, D. (2016). Network topology analysis of post-mortem brain microarrays identifies more Alzheimer’s related genes and micrornas and points to novel routes for fighting with the disease. PLoS One 11:e0144052. doi: 10.1371/journal.pone.0144052
Chen, G. F., Xu, T. H., Yan, Y., Zhou, Y. R., Jiang, Y., Melcher, K., et al. (2017). Amyloid beta: structure, biology and structure-based therapeutic development. Acta Pharmacol. Sin. 38, 1205–1235. doi: 10.1038/aps.2017.28
Chen, J. X., and Du Yan, S. (2007). Amyloid-β-induced mitochondrial dysfunction. J. Alzheimer’s Dis. 12, 177–184. doi: 10.3233/JAD-2007-12208
Chen, Y., Neve, R. L., and Liu, H. (2012). Neddylation dysfunction in Alzheimer’s disease. J. Cell. Mol. Med. 16, 2583–2591. doi: 10.1111/j.1582-4934.2012.01604.x
Chen, Y.-J., Hsu, C.-C., Shiao, Y.-J., Wang, H.-T., Lo, Y.-L., and Lin, A. M. Y. (2019). Anti-inflammatory effect of afatinib (an EGFR-TKI) on OGD-induced neuroinflammation. Sci. Rep. 9:2516. doi: 10.1038/s41598-019-38676-7
Chin, J. (2005). Fyn kinase induces synaptic and cognitive impairments in a transgenic mouse model of Alzheimer’s disease. J. Neurosci. 25, 9694–9703. doi: 10.1523/JNEUROSCI.2980-05.2005
Chin, J., Palop, J. J., Yu, G. Q., Kojima, N., Masliah, E., and Mucke, L. (2004). Fyn kinase modulates synaptotoxicity, but not aberrant sprouting, in human amyloid precursor protein transgenic mice. J. Neurosci. 24, 4692–4697. doi: 10.1523/JNEUROSCI.0277-04.2004
Chow, V. W., Mattson, M. P., Wong, P. C., and Gleichmann, M. (2010). An overview of APP processing enzymes and products. Neuromolecular Med. 12, 1–12. doi: 10.1007/s12017-009-8104-z
Chung, S. S., Sun, K., and Bolech, C. J. (2015). Matrix product ansatz for Fermi fields in one dimension. Phys. Rev. B 91:121108. doi: 10.1103/PhysRevB.91.121108
Ciechanover, A. (2015). The unravelling of the ubiquitin system. Nat. Rev. Mol. Cell Biol. 16, 322–324. doi: 10.1038/nrm3982
Cummings, J. L., Morstorf, T., and Zhong, K. (2014). Alzheimer’s disease drug-development pipeline: few candidates, frequent failures. Alzheimers. Res. Ther. 6:37. doi: 10.1186/alzrt269
Durrenberger, P. F., Fernando, F. S., Magliozzi, R., Kashefi, S. N., Bonnert, T. P., Ferrer, I., et al. (2012). Selection of novel reference genes for use in the human central nervous system: a brainnet europe study. Acta Neuropathol. 124, 893–903. doi: 10.1007/s00401-012-1027-z
Edison, P., Archer, H. A., Hinz, R., Hammers, A., Pavese, N., Tai, Y. F., et al. (2007). Amyloid, hypometabolism, and cognition in Alzheimer disease: an [11C]PIB and [18F]FDG PET study. Neurology 68, 501–508. doi: 10.1212/01.wnl.0000244749.20056.d4
Egan, M. F., Kost, J., Voss, T., Mukai, Y., Aisen, P. S., Cummings, J. L., et al. (2019). Randomized trial of verubecestat for prodromal Alzheimer’s disease. N. Engl. J. Med. 380, 1408–1420. doi: 10.1056/NEJMoa1812840
Esparza, T. J., Zhao, H., Cirrito, J. R., Cairns, N. J., Bateman, R. J., Holtzman, D. M., et al. (2013). Amyloid-beta oligomerization in Alzheimer dementia versus high-pathology controls. Ann. Neurol. 73, 104–119. doi: 10.1002/ana.23748
Farris, W., Mansourian, S., Chang, Y., Lindsley, L., Eckman, E. A., Frosch, M. P., et al. (2003). Insulin-degrading enzyme regulates the levels of insulin, amyloid -protein, and the -amyloid precursor protein intracellular domain in vivo. Proc. Natl. Acad. Sci. U.S.A. 100, 4162–4167. doi: 10.1073/pnas.0230450100
Fischer, M. T., Wimmer, I., Höftberger, R., Gerlach, S., Haider, L., Zrzavy, T., et al. (2013). Disease-specific molecular events in cortical multiple sclerosis lesions. Brain 136, 1799–1815. doi: 10.1093/brain/awt110
Folch, J., Ettcheto, M., Busquets, O., Sánchez-López, E., Castro-Torres, R. D., Verdaguer, E., et al. (2018). The implication of the brain insulin receptor in late onset Alzheimer’s disease dementia. Pharmaceuticals 11:11. doi: 10.3390/ph11010011
François, A., Rioux Bilan, A., Quellard, N., Fernandez, B., Janet, T., Chassaing, D., et al. (2014). Longitudinal follow-up of autophagy and inflammation in brain of APPswePS1dE9 transgenic mice. J. Neuroinflammation 11:139. doi: 10.1186/s12974-014-0139-x
Friedman, B. A., Srinivasan, K., Ayalon, G., Meilandt, W. J., Lin, H., Huntley, M. A., et al. (2018). Diverse brain myeloid expression profiles reveal distinct microglial activation states and aspects of Alzheimer’s disease not evident in mouse models. Cell Rep. 22, 832–847. doi: 10.1016/j.celrep.2017.12.066
Fu, L., Abu-Khalil, A., Morrison, R. S., Geschwind, D. H., and Kornblum, I. (2003). Expression patterns of epidermal growth factor receptor and fibroblast growth factor receptor 1 mRNA in fetal human brain. J. Comp. Neurol. 462, 265–273. doi: 10.1002/cne.10727
Garwood, C. J., Pooler, A. M., Atherton, J., Hanger, D. P., and Noble, W. (2011). Astrocytes are important mediators of Aβ-induced neurotoxicity and tau phosphorylation in primary culture. Cell Death. Dis. 2:e167. doi: 10.1038/cddis.2011.50
Gautier, L., Cope, L., Bolstad, B. M., and Irizarry, R. A. (2004). Affy – Analysis of affymetrix genechip data at the probe level. Bioinformatics 20, 307–315. doi: 10.1093/bioinformatics/btg405
Ghiassian, S. D., Menche, J., and Barabási, A. L. (2015). A DIseAse MOdule detection (DIAMOnD) algorithm derived from a systematic analysis of connectivity patterns of disease proteins in the human interactome. PLoS Comput. Biol. 11:e1004120. doi: 10.1371/journal.pcbi.1004120
Gong, B., Radulovic, M., Figueiredo-Pereira, M. E., and Cardozo, C. (2016). The ubiquitin-proteasome system: Potential therapeutic targets for Alzheimer’s disease and spinal cord injury. Front. Mol. Neurosci. 9:4. doi: 10.3389/fnmol.2016.00004
Haidich, A. B. (2010). Meta-analysis in medical research. Hippokratia 14, 29–37. doi: 10.5005/jp/books/10519
Hara, T., Nakamura, K., Matsui, M., Yamamoto, A., Nakahara, Y., Suzuki-Migishima, R., et al. (2006). Suppression of basal autophagy in neural cells causes neurodegenerative disease in mice. Nature 441, 885–889. doi: 10.1038/nature04724
Hardy, J., and Higgins, G. (1992). Alzheimer’s disease: the amyloid cascade hypothesis. Science 256, 184–185. doi: 10.1126/science.1566067
Harris, R. A., Tindale, L., Lone, A., Singh, O., Macauley, S. L., Stanley, M., et al. (2016). Aerobic glycolysis in the frontal cortex correlates with memory performance in wild-type mice but not the APP/PS1 mouse model of cerebral amyloidosis. J. Neurosci. 36, 1871–1878. doi: 10.1523/JNEUROSCI.3131-15.2016
Hashimoto, S., and Saido, T. C. (2018). Critical review: involvement of endoplasmic reticulum stress in the aetiology of Alzheimer’s disease. Open Biol. 8:180024. doi: 10.1098/rsob.180024
Hernández, F., and Avila, J. (2008). The role of glycogen synthase kinase 3 in the early stages of Alzheimers’ disease. FEBS Lett. 582, 3848–3854. doi: 10.1016/j.febslet.2008.10.026
Hokama, M., Oka, S., Leon, J., Ninomiya, T., Honda, H., Sasaki, K., et al. (2014). Altered expression of diabetes-related genes in Alzheimer’s disease brains: the hisayama study. Cereb. Cortex 24, 2476–2488. doi: 10.1093/cercor/bht101
Holmes, C., Cunningham, C., Zotova, E., Woolford, J., Dean, C., Kerr, S., et al. (2009). Systemic inflammation and disease progression in alzheimer disease. Neurology 73, 768–774. doi: 10.1212/WNL.0b013e3181b6bb95
Hong, L., Huang, H.-C., and Jiang, Z.-F. (2014). Relationship between amyloid-beta and the ubiquitin–proteasome system in Alzheimer’s disease. Neurol. Res. 36, 276–282. doi: 10.1179/1743132813Y.0000000288
Honig, L. S., Vellas, B., Woodward, M., Boada, M., Bullock, R., Borrie, M., et al. (2018). Trial of solanezumab for mild dementia due to Alzheimer’s disease. N. Engl. J. Med. 378, 321–330. doi: 10.1056/NEJMoa1705971
Hoos, M. D., Richardson, B. M., Foster, M. W., Everhart, A., Thompson, J. W., Moseley, M. A., et al. (2013). Longitudinal study of differential protein expression in an Alzheimer’s mouse model lacking inducible nitric oxide synthase. J. Proteome Res. 12, 4462–4477. doi: 10.1021/pr4005103
Irmler, M., Gentier, R. J. G., Dennissen, F. J. A., Schulz, H., Bolle, I., Hölter, S. M., et al. (2012). Long-term proteasomal inhibition in transgenic mice by UBB+1 expression results in dysfunction of central respiration control reminiscent of brainstem neuropathology in Alzheimer patients. Acta Neuropathol. 124, 187–197. doi: 10.1007/s00401-012-1003-7
Ittner, L. M., Ke, Y. D., Delerue, F., Bi, M., Gladbach, A., vanEersel, J., et al. (2010). Dendritic function of tau mediates amyloid-β toxicity in alzheimer’s disease mouse models. Cell 142, 387–397. doi: 10.1016/j.cell.2010.06.036
Jaeger, P. A., and Wyss-Coray, T. (2010). Beclin 1 complex in autophagy and Alzheimer disease. Arch. Neurol. 67, 1181–1184. doi: 10.1001/archneurol.2010.258
Janssen, S. F., van derSpek, S. J. F., Tenbrink, J. B., Essing, A. H. W., Gorgels, T. G., van derSpek, P. J., et al. (2013). Gene expression and functional annotation of the human and mouse choroid plexus epithelium. PLoS One 8:e83345. doi: 10.1371/journal.pone.0083345
Joshi, A. U., Kornfeld, O. S., and Mochly-Rosen, D. (2016). The entangled ER-mitochondrial axis as a potential therapeutic strategy in neurodegeneration: a tangled duo unchained. Cell Calcium 60, 218–234. doi: 10.1016/j.ceca.2016.04.010
Kanehisa, M., Goto, S., Sato, Y., Furumichi, M., and Tanabe, M. (2012). KEGG for integration and interpretation of large-scale molecular data sets. Nucleic Acids Res. 40, D109–D114. doi: 10.1093/nar/gkr988
Kästle, M., and Grune, T. (2012). Interactions of the proteasomal system with chaperones: Protein triage and protein quality control. Prog. Mol. Biol. Transl. Sci. 109, 113–160. doi: 10.1016/B978-0-12-397863-9.00004-3
Kauffmann, A., Gentleman, R., and Huber, W. (2009). arrayQualityMetrics—a bioconductor package for quality assessment of microarray data. Bioinformatics 25, 415–416. doi: 10.1093/bioinformatics/btn647
Kaufman, A. C., Salazar, S. V., Haas, L. T., Yang, J., Kostylev, M. A., Jeng, A. T., et al. (2015). Fyn inhibition rescues established memory and synapse loss in Alzheimer mice. Ann. Neurol. 77, 953–971. doi: 10.1002/ana.24394
Kawalia, S. B., Raschka, T., Naz, M., deMatos Simoes, R., Senger, P., and Hofmann-Apitius, M. (2017). Analytical strategy to prioritize Alzheimer’s disease candidate genes in gene regulatory networks using public expression data. J. Alzheimers Dis. 59, 1237–1254. doi: 10.3233/JAD-170011
Kennedy, M. E., Stamford, A. W., Chen, X., Cox, K., Cumming, J. N., Dockendorf, M. F., et al. (2016). The BACE1 inhibitor verubecestat (MK-8931) reduces CNS -amyloid in animal models and in Alzheimers disease patients. Sci. Transl. Med. 8:363ra150. doi: 10.1126/scitranslmed.aad9704
Kikuchi, M., Ogishima, S., Miyamoto, T., Miyashita, A., Kuwano, R., Nakaya, J., et al. (2013). Identification of unstable network modules reveals disease modules associated with the progression of Alzheimer’s disease. PLoS One 8:e76162. doi: 10.1371/journal.pone.0076162
Kim, D., Pertea, G., Trapnell, C., Pimentel, H., Kelley, R., and Salzberg, S. L. (2013). TopHat2: accurate alignment of transcriptomes in the presence of insertions, deletions and gene fusions. Genome Biol. 14:R36. doi: 10.1186/gb-2013-14-4-r36
Kline, A. (2012). Apolipoprotein E, amyloid-ß clearance and therapeutic opportunities in Alzheimer’s disease. Alzheimers. Res. Ther. 4:32. doi: 10.1186/alzrt135
Knowles, T. P. J., Vendruscolo, M., and Dobson, C. M. (2014). The amyloid state and its association with protein misfolding diseases. Nat. Rev. Mol. Cell Biol. 15, 384–396. doi: 10.1038/nrm3810
Kolesnikov, N., Hastings, E., Keays, M., Melnichuk, O., Tang, Y. A., Williams, E., et al. (2015). ArrayExpress update–simplifying data submissions. Nucleic Acids Res. 43, D1113–D1116. doi: 10.1093/nar/gku1057
Lachen-Montes, M., Zelaya, M. V., Segura, V., Fernández-Irigoyen, J., and Santamaría, E. (2017). Progressive modulation of the human olfactory bulb transcriptome during Alzheimer’s disease evolution: novel insights into the olfactory signaling across proteinopathies. Oncotarget 8, 69663–69679. doi: 10.18632/oncotarget.18193
Laurén, J., Gimbel, D. A., Nygaard, H. B., Gilbert, J. W., and Strittmatter, S. M. (2009). Cellular prion protein mediates impairment of synaptic plasticity by amyloid-β oligomers. Nature 457, 1128–1132. doi: 10.1038/nature07761
Lee, G. (2004). Phosphorylation of Tau by Fyn: implications for Alzheimer’s Disease. J. Neurosci. 24, 2304–2312. doi: 10.1523/JNEUROSCI.4162-03.2004
Leinonen, R., Sugawara, H., and Shumway, M. (2011). The sequence read archive. Nucleic Acids Res. 39, D19–D21. doi: 10.1093/nar/gkq1019
Li, H., Handsaker, B., Wysoker, A., Fennell, T., Ruan, J., Homer, N., et al. (2009). The sequence alignment/map format and SAMtools. Bioinformatics 25, 2078–2079. doi: 10.1093/bioinformatics/btp352
Li, H., Liu, C. C., Zheng, H., and Huang, T. Y. (2018). Amyloid, tau, pathogen infection and antimicrobial protection in Alzheimer’s disease -conformist, nonconformist, and realistic prospects for AD pathogenesis. Transl. Neurodegener. 7:34. doi: 10.1186/s40035-018-0139-3
Li, X., Wang, H., Long, J., Pan, G., He, T., Anichtchik, O., et al. (2018). Systematic Analysis and Biomarker Study for Alzheimer’s Disease. Sci. Rep. 8:17394. doi: 10.1038/s41598-018-35789-3
Liang, W. S., Dunckley, T., Beach, T. G., Grover, A., Mastroeni, D., Walker, D. G., et al. (2007). Gene expression profiles in anatomically and functionally distinct regions of the normal aged human brain. Physiol. Genomics 28, 311–322. doi: 10.1152/physiolgenomics.00208.2006
Lucin, K. M., O’Brien, C. E., Bieri, G., Czirr, E., Mosher, I., Abbey, R. J., et al. (2013). Microglial beclin 1 regulates retromer trafficking and phagocytosis and is impaired in Alzheimer’s disease. Neuron 79, 873–886. doi: 10.1016/j.neuron.2013.06.046
Ma, J. F., Huang, Y., Chen, S. D., and Halliday, G. (2010). Immunohistochemical evidence for macroautophagy in neurones and endothelial cells in Alzheimer’s disease. Neuropathol. Appl. Neurobiol. 36, 312–319. doi: 10.1111/j.1365-2990.2010.01067.x
Magistri, M., Velmeshev, D., Makhmutova, M., and Faghihi, M. A. (2015). Transcriptomics profiling of Alzheimer’s disease reveal neurovascular defects, altered amyloid-β homeostasis, and deregulated expression of long noncoding RNAs. J. Alzheimers Dis. 48, 647–665. doi: 10.3233/JAD-150398
Manavalan, A., Mishra, M., Feng, L., Sze, S. K., Akatsu, H., and Heese, K. (2013). Brain site-specific proteome changes in aging-related dementia. 45:e39. doi: 10.1038/emm.2013.76
Marina, G. B., Kirkitadze, D., Lomakin, A., Vollers, S. S., Benedek, G. B., and Teplow, D. B. (2003). Amyloid β-protein (Aβ) assembly: Aβ40 and Aβ42 oligomerize through distinct pathways. Proc. Natl. Acad. Sci. U.S.A. 100, 330–335. doi: 10.1073/pnas.222681699
Marquez, R. T., and Xu, L. (2012). Bcl-2:Beclin 1 complex: multiple, mechanisms regulating autophagy/apoptosis toggle switch. Am. J. Cancer Res. 2, 214–221.
Mattoo, R. U. H., and Goloubinoff, P. (2014). Molecular chaperones are nanomachines that catalytically unfold misfolded and alternatively folded proteins. Cell. Mol. Life Sci. 71, 3311–3325. doi: 10.1007/s00018-014-1627-y
Mawuenyega, K. G., Sigurdson, W., Ovod, V., Munsell, L., Kasten, T., Morris, J. C., et al. (2010). Decreased clearance of CNS β-amyloid in Alzheimer’s disease. Science 330:1774. doi: 10.1126/science.1197623
Meng, G., Zhong, X., and Mei, H. (2016). A systematic investigation into Aging related genes in brain and their relationship with Alzheimer’s disease. PLoS One 11:e0150624. doi: 10.1371/journal.pone.0150624
Miller, J. A., Woltjer, R. L., Goodenbour, J. M., Horvath, S., and Geschwind, D. H. (2013). Genes and pathways underlying regional and cell type changes in Alzheimer’s disease. Genome Med. 5:48. doi: 10.1186/gm452
Moher, D., Liberati, A., Tetzlaff, J., Altman, D. G., and Prisma Group. (2009). Preferred reporting items for systematic reviews and meta-analyses: the PRISMA statement. PLoS Med. 6:e1000097. doi: 10.1371/journal.pmed.1000097
Moradifard, S., Hoseinbeyki, M., Ganji, S. M., and Minuchehr, Z. (2018). Analysis of microRNA and gene expression profiles in Alzheimer’s disease: a meta-analysis approach. Sci. Rep. 8:4767. doi: 10.1038/s41598-018-20959-0
Moreau, K., Fleming, A., Imarisio, S., Lopez Ramirez, A., Mercer, J. L., Jimenez-Sanchez, M., et al. (2014). PICALM modulates autophagy activity and tau accumulation. Nat. Commun. 5:4998. doi: 10.1038/ncomms5998
Moreira, P. I, Carvalho, C., Zhu, X., Smith, M. A., and Perry, G. (2010). Mitochondrial dysfunction is a trigger of Alzheimer’s disease pathophysiology. Biochim. Biophys. Acta Mol. Basis Dis. 1802, 2–10. doi: 10.1016/j.bbadis.2009.10.006
Mosconi, L., Pupi, A., and DeLeon, M. J. (2008). Brain glucose hypometabolism and oxidative stress in preclinical Alzheimer’s disease. Ann. N. Y. Acad. Sci. 1147, 180–195. doi: 10.1196/annals.1427.007
Narayanan, M., Huynh, J. L., Wang, K., Yang, X., Yoo, S., McElwee, J., et al. (2014). Common dysregulation network in the human prefrontal cortex underlies two neurodegenerative diseases. Mol. Syst. Biol. 10:743. doi: 10.15252/msb.20145304
Narendra, D., Tanaka, A., Suen, D. F., and Youle, R. J. (2008). Parkin is recruited selectively to impaired mitochondria and promotes their autophagy. J. Cell Biol. 183, 795–803. doi: 10.1083/jcb.200809125
Nativio, R., Donahue, G., Berson, A., Lan, Y., Amlie-Wolf, A., Tuzer, F., et al. (2018). Publisher correction: dysregulation of the epigenetic landscape of normal aging in Alzheimer’s disease. Nat. Neurosci. 21, 1018–1018. doi: 10.1038/s41593-018-0124-2
Nelson, P. T., Alafuzoff, I., Bigio, E. H., Bouras, C., Braak, H., Cairns, N. J., et al. (2012). Correlation of Alzheimer disease neuropathologic changes with cognitive status: a review of the literature. J. Neuropathol. Exp. Neurol. 71, 362–381. doi: 10.1097/NEN.0b013e31825018f7
Newington, J. T., Rappon, T., Albers, S., Wong, D. Y., Rylett, R. J., and Cumming, R. C. (2012). Overexpression of pyruvate dehydrogenase kinase 1 and lactate dehydrogenase A in nerve cells confers resistance to amyloid β and other toxins by decreasing mitochondrial respiration and reactive oxygen species production. J. Biol. Chem. 287, 37245–37258. doi: 10.1074/jbc.M112.366195
Nilsson, P., Loganathan, K., Sekiguchi, M., Matsuba, Y., Hui, K., Tsubuki, S., et al. (2013). Aβ secretion and plaque formation depend on autophagy. Cell Rep. 5, 61–69. doi: 10.1016/j.celrep.2013.08.042
Nobili, A., Latagliata, E. C., Viscomi, M. T., Cavallucci, V., Cutuli, D., Giacovazzo, G., et al. (2017). Dopamine neuronal loss contributes to memory and reward dysfunction in a model of Alzheimer’s disease. Nat. Commun. 8:14727. doi: 10.1038/ncomms14727
Nomura, J., Hosoi, T., Kaneko, M., Ozawa, K., Nishi, A., and Nomura, Y. (2016). Neuroprotection by endoplasmic reticulum stress-induced HRD1 and chaperones: possible therapeutic targets for Alzheimer’s and Parkinson’s disease. Med. Sci 4:14. doi: 10.3390/medsci4030014
Ntsapi, C., and Loos, B. (2016). Caloric restriction and the precision-control of autophagy: a strategy for delaying neurodegenerative disease progression. Exp. Gerontol. 83, 97–111. doi: 10.1016/j.exger.2016.07.014
Nunez-Iglesias, J., Liu, C.-C., Morgan, T. E., Finch, C. E., and Zhou, X. J. (2010). Joint genome-wide profiling of miRNA and mRNA expression in Alzheimer’s disease cortex reveals altered miRNA regulation. PLoS One 5:e8898. doi: 10.1371/journal.pone.0008898
Nygaard, H. B., Wagner, A. F., Bowen, G. S., Good, S. P., MacAvoy, M. G., Strittmatter, K. A., et al. (2015). A phase Ib multiple ascending dose study of the safety, tolerability, and central nervous system availability of AZD0530 (saracatinib) in Alzheimer’s disease. Alzheimers. Res. Ther. 7:35. doi: 10.1186/s13195-015-0119-0
Oddo, S., Vasilevko, V., Caccamo, A., Kitazawa, M., Cribbs, D. H., and LaFerla, F. M. (2006). Reduction of soluble Aβ and Tau, but not soluble Aβ Alone, ameliorates cognitive decline in transgenic mice with plaques and tangles. J. Biol. Chem. 281, 39413–39423. doi: 10.1074/jbc.M608485200
Oyagi, A., Moriguchi, S., Nitta, A., Murata, K., Oida, Y., Tsuruma, K., et al. (2011). Heparin-binding EGF-like growth factor is required for synaptic plasticity and memory formation. Brain Res. 1419, 97–104. doi: 10.1016/j.brainres.2011.09.003
Pacheco-Quinto, J., and Eckman, E. A. (2013). Endothelin-converting enzymes degrade intracellular β-amyloid produced within the endosomal/lysosomal pathway and autophagosomes. J. Biol. Chem. 288, 5606–5615. doi: 10.1074/jbc.M112.422964
Palmer, J. C., Tayler, H. M., and Love, S. (2013). Endothelin-converting enzyme-1 activity, endothelin-1 production, and free radical-dependent vasoconstriction in Alzheimer’s disease. J. Alzheimers Dis. 36, 577–587. doi: 10.3233/JAD-130383
Pang, X., Zhao, Y., Wang, J., Zhou, Q., Xu, L., Kang, D., et al. (2017). The Bioinformatic analysis of the dysregulated genes and MicroRNAs in entorhinal cortex, hippocampus, and blood for Alzheimer’s disease. Biomed Res. Int. 2017:9084507. doi: 10.1155/2017/9084507
Papa, L., and Germain, D. (2011). Estrogen receptor mediates a distinct mitochondrial unfolded protein response. J. Cell Sci. 124 (Pt 9), 1396–1402. doi: 10.1242/jcs.078220
Parihar, M. S., and Brewer, G. J. (2010). Amyloid-β as a modulator of synaptic plasticity. J. Alzheimers Dis. 22, 741–763. doi: 10.3233/JAD-2010-101020
Polito, V. A., Li, H., Martini−Stoica, H., Wang, B., Yang, L., Xu, Y., et al. (2014). Selective clearance of aberrant tau proteins and rescue of neurotoxicity by transcription factor EB. EMBO Mol. Med. 6, 1142–1160. doi: 10.15252/emmm.201303671
Potter, R., Patterson, B. W., Elbert, D. L., Ovod, V., Kasten, T., Sigurdson, W., et al. (2013). Increased in vivo amyloid-b42 production, exchange, and loss in presenilin mutation carriers. Sci. Transl. Med. 5, 189ra77. doi: 10.1126/scitranslmed.3005615
Puthiyedth, N., Riveros, C., Berretta, R., and Moscato, P. (2015). A new combinatorial optimization approach for integrated feature selection using different datasets: a prostate cancer transcriptomic study. PLoS One 10:e0127702. doi: 10.1371/journal.pone.0127702
Puthiyedth, N., Riveros, C., Berretta, R., and Moscato, P. (2016). Identification of differentially expressed genes through integrated study of Alzheimer’s disease affected brain regions. PLoS One 11:e0152342. doi: 10.1371/journal.pone.0152342
Rahman, M. R., Islam, T., Turanli, B., Zaman, T., Faruquee, H. M., Rahman, M. M., et al. (2019). Network-based approach to identify molecular signatures and therapeutic agents in Alzheimer’s disease. Comput. Biol. Chem. 78, 431–439. doi: 10.1016/j.compbiolchem.2018.12.011
Ritchie, M. E., Phipson, B., Wu, D., Hu, Y., Law, C. W., Shi, W., et al. (2015). Limma powers differential expression analyses for RNA-sequencing and microarray studies. Nucleic Acids Res. 43:e47. doi: 10.1093/nar/gkv007
Roberson, E. D., Scearce-Levie, K., Palop, J. J., Yan, F., Cheng, I. H., Wu, T., et al. (2007). Reducing endogenous tau ameliorates amyloid beta-induced deficits in an Alzheimer’s disease mouse model. Science 316, 750–754. doi: 10.1126/science.1141736
Roberts, B. R., Lind, M., Wagen, A. Z., Rembach, A., Frugier, T., Li, Q. X., et al. (2017). Biochemically-defined pools of amyloid-β in sporadic Alzheimer’s disease: correlation with amyloid PET. Brain 140, 1486–1498. doi: 10.1093/brain/awx057
Robinson, M. D., McCarthy, D. J., and Smyth, G. K. (2009). edgeR: a bioconductor package for differential expression analysis of digital gene expression data. Bioinformatics 26, 139–140. doi: 10.1093/bioinformatics/btp616
Roth, R. B., Hevezi, P., Lee, J., Willhite, D., Lechner, S. M., Foster, A. C., et al. (2006). Gene expression analyses reveal molecular relationships among 20 regions of the human CNS. Neurogenetics 7, 67–80. doi: 10.1007/s10048-006-0032-6
Rovelet-Lecrux, A., Hannequin, D., Raux, G., Meur, N. Le, Laquerrière, A., Vital, A., et al. (2006). APP locus duplication causes autosomal dominant early-onset Alzheimer disease with cerebral amyloid angiopathy. Nat. Genet. 38, 24–26. doi: 10.1038/ng1718
Saidi, L. J., Polydoro, M., Kay, K. R., Sanchez, L., Mandelkow, E. M., Hyman, B. T., et al. (2015). Carboxy terminus heat shock protein 70 interacting protein reduces tau-associated degenerative changes. J. Alzheimers Dis. 44, 937–947. doi: 10.3233/JAD-142094
Santos, C. Y., Snyder, P. J., Wu, W. C., Zhang, M., Echeverria, A., and Alber, J. (2017). Pathophysiologic relationship between Alzheimer’s disease, cerebrovascular disease, and cardiovascular risk: a review and synthesis. Alzheimers Dement. (Amst) 7, 69–87. doi: 10.1016/j.dadm.2017.01.005
Scheckel, C., Drapeau, E., Frias, M. A., Park, C. Y., Fak, J., Zucker-Scharff, I., et al. (2016). Regulatory consequences of neuronal ELAV-like protein binding to coding and non-coding RNAs in human brain. elife 5:e10421. doi: 10.7554/eLife.10421
Scheper, W., and Hoozemans, J. J. M. (2015). The unfolded protein response in neurodegenerative diseases: a neuropathological perspective. Acta Neuropathol. 130, 315–331. doi: 10.1007/s00401-015-1462-8
Scott, T. J., O’Connor, A. C., Link, A. N., and Beaulieu, T. J. (2014). Economic analysis of opportunities to accelerate Alzheimer’s disease research and development. Ann. N. Y. Acad. Sci. 1313, 17–34. doi: 10.1111/nyas.12417
Sengupta, U., Nilson, A. N., and Kayed, R. (2016). The role of amyloid-β oligomers in toxicity, propagation, and immunotherapy. EBioMedicine 6, 42–49. doi: 10.1016/j.ebiom.2016.03.035
Shannon, P., Markiel, A., Ozier, O., Baliga, N. S., Wang, J. T., Ramage, D., et al. (2003). Cytoscape: a software environment for integrated models of biomolecular interaction networks. Genome Res. 13, 2498–2504. doi: 10.1101/gr.1239303
Shimura, H., Schwartz, D., Gygi, S. P., and Kosik, K. S. (2004). CHIP-Hsc70 complex ubiquitinates phosphorylated tau and enhances cell survival. J. Biol. Chem. 279, 4869–4876. doi: 10.1074/jbc.M305838200
Slipczuk, L., Bekinschtein, P., Katche, C., Cammarota, M., Izquierdo, I., and Medina, J. H. (2009). BDNF activates mTOR to regulate GluR1 expression required for memory formation. PLoS One 4:e6007. doi: 10.1371/journal.pone.0006007
St George-Hyslop, P., and Fraser, P. E. (2012). Assembly of the presenilin γ-/ε-secretase complex. J. Neurochem. 120, 84–88. doi: 10.1111/j.1471-4159.2011.07505.x
Stanley, M., Macauley, S. L., and Holtzman, D. M. (2016). Changes in insulin and insulin signaling in Alzheimer’s disease: cause or consequence? J. Exp. Med.
Stopa, E. G., Tanis, K. Q., Miller, M. C., Nikonova, E. V., Podtelezhnikov, A. A., Finney, E. M., et al. (2018). Comparative transcriptomics of choroid plexus in Alzheimer’s disease, frontotemporal dementia and Huntington’s disease: implications for CSF homeostasis. Fluids Barriers CNS 15:18. doi: 10.1186/s12987-018-0102-9
Stutzbach, L. D., Xie, S. X., Naj, A. C., Albin, R., Gilman, S., Lee, V. M. Y., et al. (2013). The unfolded protein response is activated in disease-affected brain regions in progressive supranuclear palsy and Alzheimer’s disease. Acta Neuropathol. Commun. 1:31. doi: 10.1186/2051-5960-1-31
Sudhof, T. C. (2012). Calcium control of neurotransmitter release. Cold Spring Harb. Perspect. Biol. 4:a011353. doi: 10.1101/cshperspect.a011353
Sutherland, G. T., Janitz, M., and Kril, J. J. (2011). Understanding the pathogenesis of Alzheimer’s disease: will RNA-Seq realize the promise of transcriptomics? J. Neurochem. 116, 937–946. doi: 10.1111/j.1471-4159.2010.07157.x
Szklarczyk, D., Franceschini, A., Kuhn, M., Simonovic, M., Roth, A., Minguez, P., et al. (2011). The STRING database in 2011: functional interaction networks of proteins, globally integrated and scored. Nucleic Acids Res. 39, D561–D568. doi: 10.1093/nar/gkq973
Tan, M. G., Chua, W. T., Esiri, M. M., Smith, A. D., Vinters, H. V., and Lai, M. K. (2010). Genome wide profiling of altered gene expression in the neocortex of Alzheimer’s disease. J. Neurosci. Res. 88, 1157–1169. doi: 10.1002/jnr.22290
Trepanier, C. H., Jackson, M. F., and MacDonald, J. F. (2012). Regulation of NMDA receptors by the tyrosine kinase Fyn. FEBS J. 279, 12–19. doi: 10.1111/j.1742-4658.2011.08391.x
Tseng, B. P., Green, K. N., Chan, J. L., Blurton-Jones, M., and LaFerla, F. M. (2008). Aβ inhibits the proteasome and enhances amyloid and tau accumulation. Neurobiol. Aging 29, 1607–1618. doi: 10.1016/j.neurobiolaging.2007.04.014
Uchida, S., Martel, G., Pavlowsky, A., Takizawa, S., Hevi, C., Watanabe, Y., et al. (2014). Learning-induced and stathmin-dependent changes in microtubule stability are critical for memory and disrupted in ageing. Nat. Commun. 5:4389. doi: 10.1038/ncomms5389
Um, J. W., Nygaard, H. B., Heiss, J. K., Kostylev, M. A., Stagi, M., Vortmeyer, A., et al. (2012). Alzheimer amyloid-β oligomer bound to postsynaptic prion protein activates Fyn to impair neurons. Nat. Neurosci. 15, 1227–1235. doi: 10.1038/nn.3178
VanDyck, C. H., Nygaard, H. B., Chen, K., Donohue, M. C., Raman, R., Rissman, R. A., et al. (2019). Effect of AZD0530 on cerebral metabolic decline in alzheimer disease: a randomized clinical trial. JAMA Neurol. 76, 1219–1229. doi: 10.1001/jamaneurol.2019.2050
Vargas, D. M., DeBastiani, M. A., Zimmer, E. R., and Klamt, F. (2018). Alzheimer’s disease master regulators analysis: search for potential molecular targets and drug repositioning candidates. Alzheimers. Res. Ther. 10:59. doi: 10.1186/s13195-018-0394-7
Wang, J., Gu, B. J., Masters, C. L., and Wang, Y.-J. (2017). A systemic view of Alzheimer disease — insights from amyloid-β metabolism beyond the brain. Nat. Rev. Neurol. 13, 612–623. doi: 10.1038/nrneurol.2017.111
Wang, L., Chiang, H.-C., Wu, W., Liang, B., Xie, Z., Yao, X., et al. (2012). Epidermal growth factor receptor is a preferred target for treating Amyloid–induced memory loss. Proc. Natl. Acad. Sci. U.S.A. 109, 16743–16748. doi: 10.1073/pnas.1208011109
Wang, M., Roussos, P., McKenzie, A., Zhou, X., Kajiwara, Y., Brennand, K. J., et al. (2016). Integrative network analysis of nineteen brain regions identifies molecular signatures and networks underlying selective regional vulnerability to Alzheimer’s disease. Genome Med. 8:104. doi: 10.1186/s13073-016-0355-3
Wang, Q., Li, W. X., Dai, S. X., Guo, Y. C., Han, F. F., Zheng, J. J., et al. (2017). Meta-analysis of Parkinson’s disease and Alzheimer’s disease revealed commonly impaired pathways and dysregulation of NRF2-dependent genes. J. Alzheimers Dis. 56, 1525–1539. doi: 10.3233/JAD-161032
Wang, Y., and Mandelkow, E. (2016). Tau in physiology and pathology. Nat. Rev. Neurosci. 17, 22–35. doi: 10.1038/nrn.2015.1
Welter, D., MacArthur, J., Morales, J., Burdett, T., Hall, P., Junkins, H., et al. (2014). The NHGRI GWAS catalog, a curated resource of SNP-trait associations. Nucleic Acids Res. 42, D1001–D1006. doi: 10.1093/nar/gkt1229
Westermann, B. (2012). Bioenergetic role of mitochondrial fusion and fission. Biochim. Biophys. Acta Bioenerg. 1817, 1833–1838. doi: 10.1016/j.bbabio.2012.02.033
William, T. L., Johnson, B. R. G., Urbanc, B., Jenkins, A. T. A., Connell, S. D. A., and Serpell, L. C. (2011). Aβ42 oligomers, but not fibrils, simultaneously bind to and cause damage to ganglioside-containing lipid membranes. Biochem. J. 439, 67–77. doi: 10.1042/BJ20110750
Williams, C., Mehrian Shai, R., Wu, Y., Hsu, Y.-H., Sitzer, T., Spann, B., et al. (2009). Transcriptome analysis of synaptoneurosomes identifies neuroplasticity genes overexpressed in incipient Alzheimer’s disease. PLoS One 4:e4936. doi: 10.1371/journal.pone.0004936
Wruck, W., Schröter, F., and Adjaye, J. (2016). Meta-analysis of transcriptome data related to hippocampus biopsies and iPSC-derived neuronal cells from Alzheimer’s disease patients reveals an association with FOXA1 and FOXA2 gene regulatory networks. J. Alzheimers Dis. 50, 1065–1082. doi: 10.3233/JAD-150733
Wu, B., McDonald, A. J., Markham, K., Rich, C. B., McHugh, K. P., Tatzelt, J., et al. (2017). The N-terminus of the prion protein is a toxic effector regulated by the C-terminus. elife 6:e23473. doi: 10.7554/eLife.23473
Xiang, S., Huang, Z., Wang, T., Han, Z., Yu, C. Y., Ni, D., et al. (2018). Condition-specific gene co-expression network mining identifies key pathways and regulators in the brain tissue of Alzheimer’s disease patients. BMC Med. Genomics 11:115. doi: 10.1186/s12920-018-0431-1
Xu, M., Zhang, D. F., Luo, R., Wu, Y., Zhou, H., Kong, L. L., et al. (2018). A systematic integrated analysis of brain expression profiles reveals YAP1 and other prioritized hub genes as important upstream regulators in Alzheimer’s disease. Alzheimers Dement. 14, 215–229. doi: 10.1016/j.jalz.2017.08.012
Yan, T., Ding, F., and Zhao, Y. (2019). Integrated identification of key genes and pathways in Alzheimer’s disease via comprehensive bioinformatical analyses. Hereditas 156:25. doi: 10.1186/s41065-019-0101-0
Yang, T., Li, S., Xu, H., Walsh, D. M., and Selkoe, D. J. (2017). Large soluble oligomers of amyloid β-protein from alzheimer brain are far less neuroactive than the smaller oligomers to which they dissociate. J. Neurosci. 37, 152–163. doi: 10.1523/JNEUROSCI.1698-16.2016
Yasuda, S., Sugiura, H., Tanaka, H., Takigami, S., and Yamagata, K. (2012). p38 MAP kinase inhibitors as potential therapeutic drugs for neural diseases. Cent. Nerv. Syst. Agents Med. Chem. 11, 45–59. doi: 10.2174/187152411794961040
Yin, G., Li, L. Y., Qu, M., Luo, H. B., Wang, J. Z., and Zhou, X. W. (2011). Upregulation of AKT attenuates amyloid-β-induced cell apoptosis. J. Alzheimers Dis. 25, 337–345. doi: 10.3233/JAD-2011-110104
Yin, Z., Raj, D., Saiepour, N., VanDam, D., Brouwer, N., Holtman, I. R., et al. (2017). Immune hyperreactivity of Aβ plaque-associated microglia in Alzheimer’s disease. Neurobiol. Aging 55, 115–122. doi: 10.1016/j.neurobiolaging.2017.03.021
Yu, G., Wang, L.-G., Han, Y., and He, Q.-Y. (2012). clusterProfiler: an R package for comparing biological themes among gene clusters. OMICS 16, 284–287. doi: 10.1089/omi.2011.0118
Yuan, P., Condello, C., Keene, C. D., Wang, Y., Bird, T. D., Paul, S. M., et al. (2016). TREM2 haplodeficiency in mice and humans impairs the microglia barrier function leading to decreased amyloid compaction and severe axonal dystrophy. Neuron 90, 724–739. doi: 10.1016/j.neuron.2016.05.003
Zhang, B., Gaiteri, C., Bodea, L. G., Wang, Z., McElwee, J., Podtelezhnikov, A. A., et al. (2013). Integrated systems approach identifies genetic nodes and networks in late-onset Alzheimer’s disease. Cell 153, 707–720. doi: 10.1016/j.cell.2013.03.030
Zhang, M., Cai, F., Zhang, S., Zhang, S., and Song, W. (2015). Overexpression of ubiquitin carboxyl-terminal hydrolase L1 (UCHL1) delays Alzheimer’s progression in vivo. Sci. Rep. 4:7298. doi: 10.1038/srep07298
Zhang, Y. W., Wang, R., Liu, Q., Zhang, H., Liao, F. F., and Xu, H. (2007). Presenilin/γ-secretase-dependent processing of β-amyloid precursor protein regulates EGF receptor expression. Proc. Natl. Acad. Sci. U.S.A. 104, 10613–10618. doi: 10.1073/pnas.0703903104
Zhou, Y. Y., Li, Y., Jiang, W. Q., and Zhou, L. F. (2015). MAPK/JNK signalling: a potential autophagy regulation pathway. Biosci. Rep. 35:e00199. doi: 10.1042/BSR20140141
Keywords: Alzheimer’s disease, meta-analysis, microarray analysis, RNA sequence analysis, bioinformatics
Citation: Yuen SC, Zhu H and Leung S (2020) A Systematic Bioinformatics Workflow With Meta-Analytics Identified Potential Pathogenic Factors of Alzheimer’s Disease. Front. Neurosci. 14:209. doi: 10.3389/fnins.2020.00209
Received: 28 October 2019; Accepted: 25 February 2020;
Published: 13 March 2020.
Edited by:
Woon-Man Kung, Chinese Culture University, TaiwanReviewed by:
Chung-Feng Kao, National Chung Hsing University, TaiwanJudith Potashkin, Rosalind Franklin University of Medicine and Science, United States
Copyright © 2020 Yuen, Zhu and Leung. This is an open-access article distributed under the terms of the Creative Commons Attribution License (CC BY). The use, distribution or reproduction in other forums is permitted, provided the original author(s) and the copyright owner(s) are credited and that the original publication in this journal is cited, in accordance with accepted academic practice. No use, distribution or reproduction is permitted which does not comply with these terms.
*Correspondence: Siu-wai Leung, siuwai.leung@gmail.com