- 1Potsdam Institute for Climate Impact Research (PIK), Member of the Leibniz Association, Potsdam, Germany
- 2Agroecosystem Analysis and Modelling, Faculty of Organic Agricultural Sciences, University of Kassel, Kassel, Germany
Agroforestry is a promising adaptation measure for climate change, especially for low external inputs smallholder maize farming systems. However, due to its long-term nature and heterogeneity across farms and landscapes, it is difficult to quantitatively evaluate its contribution in building the resilience of farming systems to climate change over large areas. In this study, we developed an approach to simulate and emulate the shading, micro-climate regulation and biomass effects of multi-purpose trees agroforestry system on maize yields using APSIM, taking Ethiopia as a case study. Applying the model to simulate climate change impacts showed that at national level, maize yield will increase by 7.5 and 3.1 % by 2050 under RCP2.6 and RCP8.5, respectively. This projected increase in national-level maize yield is driven by maize yield increases in six administrative zones whereas yield losses are expected in other five zones (mean of −6.8% for RCP2.6 and −11.7% for RCP8.5), with yields in the other four zones remaining stable overtime. Applying the emulated agroforestry leads to increase in maize yield under current and future climatic conditions compared to maize monocultures, particularly in regions for which yield losses under climate change are expected. A 10% agroforestry shade will reduce maize yield losses by 6.9% (RCP2.6) and 4.2 % (RCP8.5) while 20% shade will reduce maize yield losses by 11.5% (RCP2.6) and 11% (RCP8.5) for projected loss zones. Overall, our results show quantitatively that agroforestry buffers yield losses for areas projected to have yield losses under climate change in Ethiopia, and therefore should be part of building climate-resilient agricultural systems.
Introduction
Climate change will have severe impacts on food production in many tropical areas as conditions become marginal or highly variable (Hoegh-Guldberg et al., 2018). As such, building a resilient agricultural system in the tropics is needed to cope up with the impacts of the changing climate yet remaining within planetary boundaries. The capacity of a system to recover from climate-related shocks like droughts, heavy precipitation, or floods will be crucial with ongoing climate and global change. Such resilience of agricultural systems should be established, among others, through climate adaptation measures. Adaptation measures should ideally contribute to reducing the greenhouse gases emissions, stabilize effects on the agricultural production, improve or stabilize agro-ecological functioning, and have local ownership among other standards (Osbahr et al., 2010; Conway and Schipper, 2011). Agroforestry is one of few measures that fit into the foregoing description and criteria.
Due to its huge potential, agroforestry features in climate change adaptation discourses especially in resource constrained vulnerable smallholder systems in Africa and Asia. This is because agroforestry contributes to sustainable agricultural development through increased yields and ecosystem services under low external inputs use, underpinning both sustainable development and climate change resilience (Mbow et al., 2014; Ong and Kho, 2015). Agroforestry is also important for stabilizing slopes and reducing erosion (Keesstra et al., 2016; Cerda et al., 2018) and increasing biodiversity of farmlands (Pardon et al., 2019; Udawatta et al., 2019). In addition, regenerative agroforestry is important for soil fertility, biodiversity, carbon sequestration and crop production, which are directly linked to the achievement of sustainable development goals such as on poverty (SDG1), hunger (SDG2), climate action (SDG13), and life on land (SDG15). There is a consensus that agroforestry is important for climate resilience despite the fact that most studies are based on discrete experimental field studies and thus difficult to transfer or upscale. This is especially important given the heterogeneous agro-ecological systems under which maize is produced in many countries. In Ethiopia for example, there is a growing realization that trees are important and linked to many biophysical processes including for regulating temperature and rainfall regimes (Muluneh et al., 2017b) and for crop buffering (Kassie, 2018; Sida et al., 2018). This is even more important with increasing risks of climate change on crop production (Araya et al., 2017; Bedeke et al., 2020).
Efforts to quantify agroforestry contributions to agriculture are focused on productivity because this aspect is most easily quantified and monetized. There is however a lack of spatially explicit quantitative information on yield stabilizing effects of agroforestry across heterogeneous environments (Dagar and Tewari, 2016; Lal et al., 2016). In addition, there is paucity of data on the spatial upscaling potential and/or uncertainty in its functioning. There are also some limitations in modeling future performance of agroforestry systems to provide confidence in its stability and role under projected climatic conditions (de Sousa et al., 2019). This lack of quantitative assessments make it difficult to explicitly include it in the Paris Agreement's National Determined Contributions (NDCs) investment plans and National Adaptation Plans (NAPs) that require such information for local level planning (Luedeling et al., 2014; Dagar and Tewari, 2016).
As a result of the demand for more information about agroforestry, there is a growing interest to incorporate agroforestry dynamics in climate risk assessment tools such as crop models. For example, Lott et al. (2009) reported significant changes in maize yield under an agroforestry system attributed to thermal and radiation amelioration. In another study, Smethurst et al. (2017) analyzed the field-level maize growth and yield effects of crop-tree interactions in two contrasting environments in a dynamic crop simulation model with satisfactory skill. Many field-level studies on the impact of agroforestry on crop production potential under climate change have also been reported. For example, Dilla et al. (2018) showed that it is possible to use APSIM to simulate maize total above-ground biomass, leaf area index and grain yield at various levels of shading. Similarly, Sida et al. (2018) observed that agroforestry systems with Faidherbia albida trees have increased soil mineral N, water use efficiency and reduced heat stress, increasing wheat yield significantly. In another study Pardon et al. (2018) concluded that in adult tree agroforestry systems, summer crops (maize and potato) are impaired when planted too close to the tree because of reduced of radiation, with these effects less in young and middle-aged systems. There are generally three principal ways through which agroforestry contributes to agricultural adaptation. These are: (i) soil and water conservation through reduced evapotranspiration, enhanced water-use efficiency and increased soil moisture retention (Cannavo et al., 2011; Zhao et al., 2012), (ii) microclimate regulation where it prevents extremes of temperature (Lott et al., 2009; Moreno et al., 2018; Qiao et al., 2019), and (iii) provision of biomass that supplements and recycles nutrients for crops from tree residuals or deeper soil layers (Mafongoya and Dzowela, 1999; Partey et al., 2011; Singh and Singh, 2016). These mechanisms represent the most significant ways in which trees influence crop production by altering the balance of resource availabilities to the crop (Ong and Kho, 2015).
There are currently no clear approaches to integrate agroforestry tree-crop interactions in process-based crop simulation models to understand the large-scale and long-term benefits of agroforestry systems such as multi-purpose trees at national level. This is because many crop models do not explicitly represent crop-tree interactions (Smethurst et al., 2017; Dilla et al., 2018; Holzworth et al., 2020). This restricts quantitative assessment of agroforestry potential; and hampers further understanding of its potential role and limits, especially in smallholder farming systems. Realizing this, the process-based crop model Agricultural Production System sIMulator Next Generation (APSIM2020) implemented individual tree simulation of agroforestry (Holzworth et al., 2020). Current simulation of agroforestry, however, is limited in numbers and arrangement of trees in fields and cannot therefore be used for modeling multi-purpose agroforestry systems.
We, therefore, developed an approach for analyzing multi-purpose trees agroforestry in a dynamic crop simulation model by representing the three ways of tree-crop interactions in agroforestry. We implemented agroforestry in APSIM in three ways: (i) through representation of radiation interception, (ii) microclimate temperature regulation, and (iii) the fertilization effect of biomass from agroforestry trees to the maize field. Overall, the aim of this study was to evaluate the potential of multi-purpose trees agroforestry to stabilize maize yields under climate change in Ethiopia, and examine if these effects are the same across different geographic areas. The study results will provide a framework for multi-purpose trees agroforestry representation in process-based crop models while providing spatially-disaggregated quantitative assessments for the potential contributions of agroforestry in maize production systems in Ethiopia. The later can further be used in national adaptation planning and in designing resilient agricultural systems under climate change risks without the risk of maladaptation.
Materials and Methods
Study Zones and Data
We focused this study on simulating maize production in Ethiopia. Maize is among the most important cereals in Ethiopia as it ranks first in total national production (~3.3 million tons) and yield per hectare (2.95 t/ha) and second after teff in area dedicated to crops (Taffesse et al., 2012; CSA, 2015). Observed maize yield data is obtained from Annual Agricultural Sample Surveys (2006 to 2008; and 2010 to 2016) by the Central Statistical Agency (CSA) of Ethiopia. Although maize is one of the staple crops in Ethiopia growing in many parts of the country, we found only 14 administrative zones (equivalent to districts) out of more than 85 zones to have sufficient data on maize yields and farm management to necessary for the modeling exercise. These are Asosa Kemashi, and Metekel zones (from Benishangul-Gumuz region), Guraghe, Hadiya, and Sidama zones (from the Southern Nations, Nationalities, and Peoples—SNNP hereafter), Illubabor, and Jimma zones (from Oromia region), North Gonder, South Gonder, North Wollo, West Gojjam, and North Shewa zones (from Amhara region) and Western Tigray zone (from Tigray region) (Supplementary Material 1). The selected administrative zones not only represent the four main agricultural regions of Ethiopia (Tigray, Amhara, Oromia, and SNNP) but also the four main agro-ecological zones (cereal-based moisture-reliable highlands, enset-based moisture reliable highlands, drought-prone highlands, moisture-reliable humid-lowlands). The fifth agro-ecological zone which is pastoralist agro-ecological zone is not represented as there is no significant maize production (Supplementary Material 2). Besides, in this study, we only focused and simulated the Mehr season (long rains) maize yield as it contributes over 90–95% percent of the nation's total cereals output (Cochrane and Bekele, 2018).
APSIM Model
The Agricultural Production System sIMulator (APSIM) (Keating et al., 2003; Holzworth et al., 2014) is used to simulate current and future maize yields in the study zones. APSIM is a crop model with a proven track record in modeling the performance of diverse cropping systems, rotations, intercropping, fallowing, crop and environmental dynamics by representing essential resource dynamics. It is especially distinctive in being able to capture crop resource demands as influenced by weather and management, and how these demands affect the soil after every successive cropping, and therefore skillful for both short and long term time scales (Gaydon et al., 2017). In APSIM model, there is a central interface engine that allows individual modules to be used and that controls communication among biological, environmental, and management modules. The generic crop growth model simulates growth based on the physiological determinants framework for growth and development of cereals and simulates growth of individual organs based on the supply-demand balance for available resources (carbon, water and nitrogen etc) within the crop (Soufizadeh et al., 2018). In particular, The APSIM-Maize module simulates several key underpinning physiological processes and operates on a daily time step in response to daily inputs of weather data, soil characteristics and crop management practices. In particular, the maize module has been shown to adequately simulate a number of processes, including phenological development, biomass accumulation, and yield formation, soil moisture and nutrient status, and agricultural management practices (Sun et al., 2016; Soufizadeh et al., 2018). Consequently, APSIM is widely used for climate change impact studies at site (Zhang et al., 2013; Hailesilassie, 2015; Pembleton et al., 2016; Deihimfard et al., 2018) and landscape (Tachie-Obeng et al., 2013; Liu et al., 2018) scales. APSIM version 7.10 was run under R environment with the apsimr (Stanfill, 2015) and the APSIM (Fainges, 2017) libraries.
Model Calibration
Daily Weather Data
The minimum data requirements for APSIM are daily rainfall, maximum temperature, minimum temperature and solar radiation. The Climate Hazards Group InfraRed Precipitation with Station data (CHIRPS) rainfall data at 0.05 degrees (Funk et al., 2015) is used to derive daily precipitation data from 2006 to 2016 for Ethiopia. The gridded daily precipitation values were summarized over croplands for each zone using a crop mask from the Global GCE 1 km Cropland Dominance for the year 2015 developed with satellite data (Teluguntla et al., 2015). This was done to ensure that the rainfall values corresponded with cultivation areas where maize is grown. Daily maximum and minimum temperature was obtained from the WFDEI Near Surface Temperature data for the same period at 0.5 degrees resolution (Weedon et al., 2014). Since temperature values are not highly variable over space, the resolution was considered reasonable for temperature data. Radiation values were derived from the maximum and minimum temperature values, latitude, day of the year and derived temperature amplitude values using the sirad package of R (Bojanowski et al., 2016) using the method developed by Mahmood and Hubbard (2002).
Soil Data
For soil profiles, we used the International Soil Reference and Information Center's (ISRIC) gridded soil data (Han et al., 2015; Hengl et al., 2017). This database has soil properties such as bulk density, organic carbon, percentage of clay and silt, soil pH and cation exchange capacity while other properties were calculated by us using pedo-transfer functions (Han et al., 2015, 2019). The soil parameters were then averaged over the zone to produce a representative soil profile for croplands of the zones after ensuring that the values were reasonable by cross checking with local profiles available in literature (Supplementary Material 3). To ensure that the soil profiles corresponded with crop lands, only profiles whose center coordinates corresponded to the Global GCE 1 km Cropland Dominance crop mask were used. The key parameters for the soil profiles are given in (Supplementary Material 4).
Crop Management Data
Fertilizer rates, planting windows, maize varieties and planting density were the key management inputs that varied for each zone. Data on fertilizer application rates for each zone was obtained with the crop yield data (Supplementary Material 5). This data was on amount on total amount of fertilizer used and area fertilized, pesticides quantities and area, organic fertilizer quantities and area applied for each zone. Each year, the simulated crop was planted after sufficient first rains in a planting window, which was determined by the climatic profile of each respective zone. A maize-fallow system was employed in all simulations. Information on tillage, planting windows, planting density, residues, growing season and other parameters was collected from published papers, national and regional research reports, and other secondary databases with agronomic information on maize production in Ethiopia (Global Yield Gaps Atlas, 2013; Araya et al., 2015; Dimes et al., 2015; Kassie et al., 2015; Smethurst et al., 2017; Seyoum et al., 2018). Local and regional maize varieties were calibrated and used for each zone (see section Model input data). The final model parameters that remained constant across all zones and all years are listed in Table 1.
Model Input Data
A model calibration was conducted because some model parameters are not available as directly measured or observed values (e.g., split between top dressing and basal fertilizer) or are difficult to assign at zone level (e.g., crop varieties). Therefore, the best initial estimates for those input parameters were calibrated for each zone based on the agreement with the observed yield. Two input parameters were of calibration interest. These are fertilizer split and maize varieties. To ensure that the calibration was independent, a leave-one-out cross validation where the model was iteratively calibrated with 9 years of data and evaluated on the 10th data point iteratively was used to produce the validation metrics (see also section Model evaluation).
Varietal Allocation
There are many maize varieties planted in Ethiopia. However, only a few varieties dominate mainly determined by altitude. Since no information about the dominant varieties is available on zone level, the information on maize varieties was obtained through iterative allocation using five local and regional varieties in Ethiopia. The dominant maize varieties are BH540, BH660, Katumeni, Melkasa-2 and MH19 whose genetic coefficients were already inbuilt in APSIM or were created into the model from literature (given in Table 2 and Supplementary Material 6).
Fertilizer Split
The actual fertilizer applications for the period were available and used in the modeling. However, the information on zone level fertilizer applied to maize systems did not distinguish between sowing and top dressing fertilizers. Yet, the APSIM requires these separately. To determine the split for each zone, four split ratios (20:80, 30:70, 40:60, and 50:50) between sowing basal and top dressing fertilizer were evaluated and the rates with the best match to observed yields were selected for that zone. The amounts were converted to equivalent per unit area for DAP (basal with 18%N and 46% P2O5) and Urea (top dressing with 46%N) applications with the later applied from 36 days after planting. After identifying the split ratio with the best accuracy for each zone, the splits were allocated for each zone accordingly.
Model Evaluation
Zone level data (2006–2016) were used to calibrate the models as well as validate the model. The final parameterized and calibrated model was applied to simulate independently the maize yield. The correlation coefficient (r) and coefficient of determination (R2) was used to assess the goodness of fit between simulated and observed zone level yields for individual zones, regions and all data. In addition, mean absolute error (MAE, Equation 1), root mean square error (RMSE, Equation 2), percent bias [pBias (%), Equation 3] and index of agreement (d, Equation 4) were used to assess the model performance. For MAE, RMSE, and pBias values of 0 indicate a perfect fit between observed and simulated values. MAE is the average of the absolute values of the differences between simulated and observed values. RMSE is one of the commonly used error index statistics and the lower the RMSE the better the model performance. pBias measures the average tendency of the simulated yields to be larger or smaller than their observed counterparts and positive values indicate scale of overestimation whereas negative values indicate scale of model underestimation. d measures the additive and proportional differences in the observed and simulated means and variances for long term data and a d of 0.5 is considered satisfactory model performance (Araya et al., 2015).
Where for all cases n is the number of data points, yi and ŷi denote the observed and simulated yield, and is the mean of the observed yield (Moriasi et al., 2007).
Climate Change Impact Assessment
The impacts of climate change on maize for each zone were determined by simulating maize growth under both the baseline and future climates. This was done after the model was satisfactorily setup for the period 2006–2016. The baseline period for was 1997 to 2016 while the 2050s was represented by projected climate data from 2041–2060 for each of the 14 zones from three different general circulation models (GCMs). The daily climate data from the Inter-Sectoral Impact Model Inter-comparison Project (ISIMIP) was used for the study. ISIMIP provided bias adjusted climate projections following the method of Lange (2016) and Weedon et al. (2014) where the model data is adjusted to EWEMBI data. This approach applies quantile mapping to adjust target distributions as empirically estimated from source distribution in the form of cumulative distribution functions. We choose three available GCMs for our climate projections. The three GCMs are GFDL-ESM2M (Dunne et al., 2013), HadGEM-ES2 (Jones et al., 2011), and MIROC-ESM-CHEM (Watanabe et al., 2011) were used. For future projections, two representative concentration pathways (RCP)–RCP2.6 and RCP8.5—were used as emission scenarios. The RCPs were chosen to represent the “well below 2 °C” target (of the Paris Agreement) and a scenario without climate policy, respectively, to capture the possible future range of climatic possibilities. Climate change impacts are then calculated as the difference between the future simulated maize yields and historical simulation for each zone following a method suggested by Lobell (2014).
Emulating Agroforestry Systems
We sought to simulate the general farm effect of multi-purpose trees agroforestry system in which farms have various multipurpose tree species. The system is based on at least 12 mature multiple-species trees per hectare with an average radius of influence of 10 m scattered haphazardly or according to some systematic patterns within fields, on bunds, terraces or plot/field boundaries. The multi-purpose trees agroforestry system is the most common agroforestry system in smallholder farms in Ethiopia, and it has multiple functions of production of various tree products and ecosystem services. We derived biomass production and N mineralization from 27 common trees (17 N-fixing) as agroforestry tree species vary greatly between fields and farms, and across the landscape. To represent multi-purpose trees agroforestry effects on maize yield, 3 aspects were concurrently implemented in the model.
Shading and Microclimate Regulation Agroforestry Effect
Shading was implemented by reducing the radiation parameters in the weather files by 10 and 20% as done by Dilla et al. (2018). To represent microclimate regulation of agroforestry, we modeled, for each zone, the corresponding effects of reducing daily radiation values by 10 and 20% in the weather file on maximum and minimum temperature. Some approaches to model effect of shading on temperature are available, for e.g., Dumas et al. (2015), Ziter et al. (2019), Speak et al. (2020), and Sida et al. (2018). These approaches are problematic for crop agroforestry simulation because they are (i) only for effects of tree shading average daily temperature, which is not directly translatable to effects on daily maximum and minimum temperature, (ii) tree species specific for general agroforestry application, and (iii) mostly from urban surfaces whose thermal responses are different from agricultural soils. Therefore, we developed a machine learning random forest regression model between radiation and temperature parameters in unmodified data that we then applied on the reduced radiation values (Supplementary Material 7). This is because we considered that there is a non-linear cooling effect of radiation interception on minimum and maximum temperature (Figure 1). This approach is more robust because an individual model is developed for each study area dealing with problems from differential radiation reception cause by, for example, altitude. It is also tree species agnostic as the effects of 10 and 20% shading can be achieved with any species depending on planting arrangements. Finally, it can also be applied for projected climatic data.
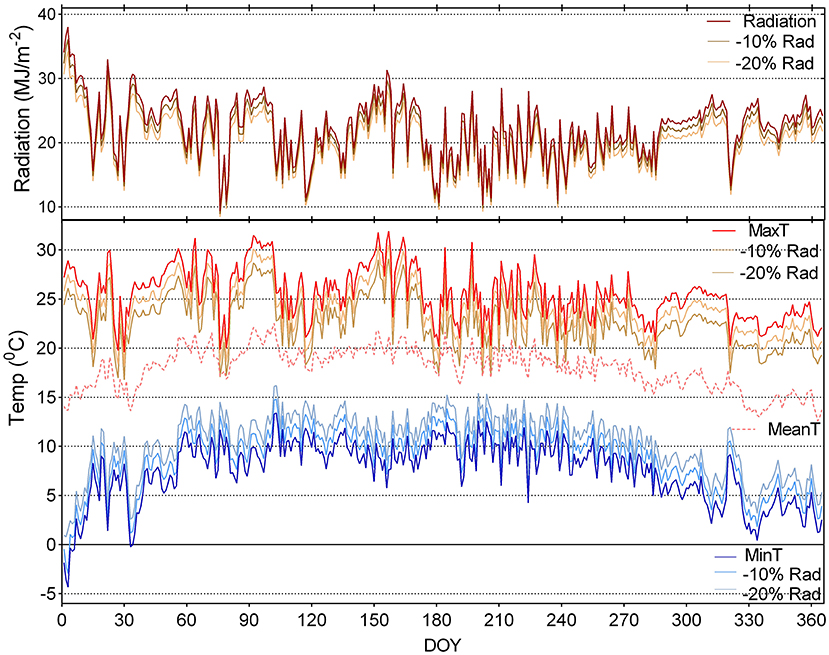
Figure 1. Emulation of agroforestry radiation interceptions and its effects on understory temperature of maize. The base year is 2006.
Nutrient Cycling and Fertilization Effect of Agroforestry
To implement the fertilization effects of multi-purpose trees agroforestry system, we reviewed literature on average plant biomass addition and the resultant N contribution. A mature agroforestry tree (older than 6 years) that provides 10% shading produces an average ~500 kg/ha-1/year of litter biomass, with 20% shading producing ~1,000 kg/ha-1/year (Palm, 1995; Mafongoya and Dzowela, 1999; Dagar and Tewari, 2016; Nair and Toth, 2016). Accordingly, biomass was added as manure input at planting (60%) and 45 days after planting (40%) with the manure application module with an average C:N ratio of 16, which is the average of multiple purposes agroforestry trees in smallholder farming systems (Mafongoya et al., 1998). We considered that the agroforestry trees would not have significant competitive water demand with the maize crop as they have matured with sufficiently deeper rooting system, and thus do not rely on rainfall but are able to access deeper water reserves (Ong et al., 2000; Lott et al., 2003, 2009; Smethurst et al., 2017).
We used the 10 and 20% reduced solar radiation and fertilization effect representation in APSIM as the effects can be achieved by different species or tree mixes (Figure 1). Our multi-purpose trees agroforestry emulation allows covering a realistic representation of agroforestry systems in a crop model for evaluating its role and contribution to a resilient agricultural system in the face of climate change.
Statistical Analysis
We performed a sensitivity analysis to separate the yield effect of photo active radiation (PAR) and fertilization of multi-purpose trees agroforestry shading systems. This was is necessary because fertilizer use in Ethiopia is comparatively low compared that of other significant maize producing countries especially in some zones. Some studies have shown that while majority of the farmers use some fertilizers, the rates are far below the recommended or the required rates (Tefera et al., 2020). To separate the influence of added nitrogen (N) fertilization on agroforestry, we did a Pearson correlation between the yield effect of agroforestry under current and projected climate scenarios and the average fertilizer applied in each zone. We hypothesized that if the correlation is negative and significant, then, it means that zones with current low levels of fertilizer application will have higher response from fertilization effect compared to those that are currently having relatively high levels of fertilizer use. This correlation shows if the yield change is caused by N supply since this depends on current levels of N supply for the zone. If a positive yield effect of agroforestry is mainly the effect of N, there are other ways to supply N directly.
Results
Distribution and Tends Observed Maize Yield in Calibrated Zones in Ethiopia
The temporal (year-to-year) and spatial (zone-to-zone) variability in maize grain yield anomalies from 2006 to 2016 is shown in Figure 6. The data shows that there is significant year-to-year variation in maize yield, with magnitude of change being different across zones. There is also potential for a higher production as the highest mean yield (West Gojjam, 3.3 t/ha) is twice as high as the lowest mean yield (North Wollo, 1.7 t/ha) for the period (Figure 2). Generally, most of the zones are producing approximately the national average yield (2.8 t/ha), with West Gojjam having consistently high maize yield (its minimum yield of 2.6 t/ha) is higher than the average of other five zones). The similarity in maize yields between zones and years is shown in Supplementary Material 2. These zones with high inter-annual variability in maize yield (e.g., Kemashi, Western Tigray, Illubabor, Hadiya and Guraghe) represent areas of high volatility and production risk under current climatic conditions (Figure 2).
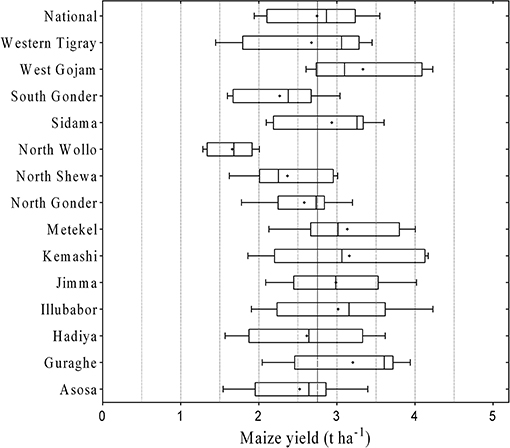
Figure 2. Similarity analysis of observed maize yield anomalies in Ethiopia between 2006 and 2016. The solid line represents the national mean yield.
Varietal Allocation and Fertilizer Split
The calibration process allocated the maize varieties to each of the zones depending on the fit. The most allocated maize variety was MH19 (6 (43%) zones) followed by BH660 that was allocated to four (29%) zones. The regional maize variety Katumani was allocated to only two zones (Jimma and Metekel) while BH540 (Guraghe) and Melkasa-2 (North Gonder) had one zone each. The allocation of the maize varieties showed that the BH660 variety fit the yield trends in three zones in the south central Ethiopian highlands while Katumani was distributed in the zones in the drought prone areas (see more in Supplementary Material 8). In terms of fertilizer split calibration, the 40:60 and 50:50 splits were most common calibrated split ratio for fertilizers in the zones.
Statistical Model Evaluation
The model performance varied among zones and the relationship between the observed and simulated maize yield for all the zones are shown in Table 3 with a overall d of 0.64, and RMSE of 0.53. The RMSE values for all zones was acceptable with a slightly over-estimated maize yield. This overall match between the long-term observed and simulated maize yields is shown on the probability of exceedance plot which confirm this slight overestimation of the model for lower maize yields compared to yields above 3.5t/ha (Figure 3).
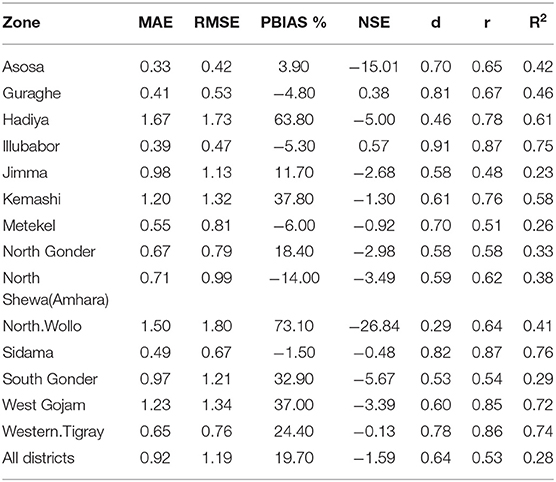
Table 3. Model performance evaluation for each zone from the observed versus simulated maize grain yield from 2006 to 2016 with APSIM.
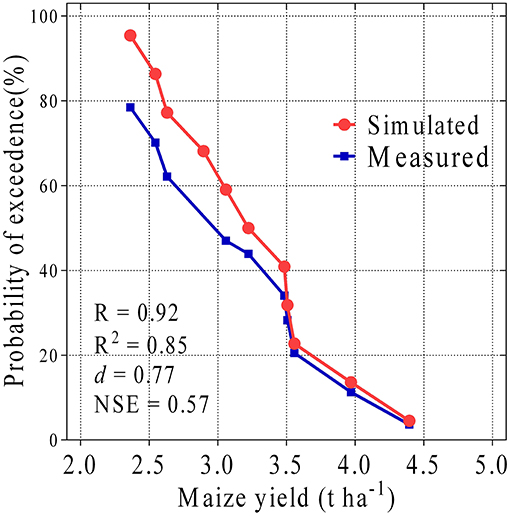
Figure 3. Probability of exceedance for the observed versus simulated maize yield in study zones in Ethiopia using the calibrated model for 2006 to 2016. Note that observed data had one missing data point.
Table 3 shows the model performance for each zone. Zone-wise model performance showed that four zones (Illubabor, Sidama, West Gojjam, and Western Tigray) had excellent model performance (d > 0.8 and R2 > 0.7). The least performance in terms of accuracy was observed for simulation of maize yields in South Gonder and Jimma (R2 < 0.3) (Table 3). Metekel had a low overall fit (R2 = 0.26) but the results show that the model was able to capture the yields trends as shown by the high index of agreement (d = 0.70), indicating that there is a specific year that the model was not able to simulate correctly while the other years were well-simulated (Table 3). There was therefore confidence to apply the model for climate impact assessment and adaptation evaluation as it was able to capture the current production levels and trends (Table 3).
Projected Maize Yields Under Climate Change
The climate change-attributable yield changes are shown in Figures 4, 5. To assess climate change impacts on maize yield, the weather variables for each of the sample zones were replaced by the projected climate data for the 2050s under RCP2.6 (Figure 4A) and RCP8.5 (Figure 4B). For all of the zones, we project a mean maize yield increase of 7.5% under RCP2.6 and 3.1% under RCP8.5 in Ethiopia. This national level increase is manly driven by simulated maize yield increases in six zones under climate change which include West Gojjam, South Gonder, Sidama, Jimma, Hadiya, and Asosa) (Figures 4, 5). Five zones have projected maize yield losses with the highest changes projected for Western Tigray (25.7% under RCP2.6 and 22.1% for RCP8.5).
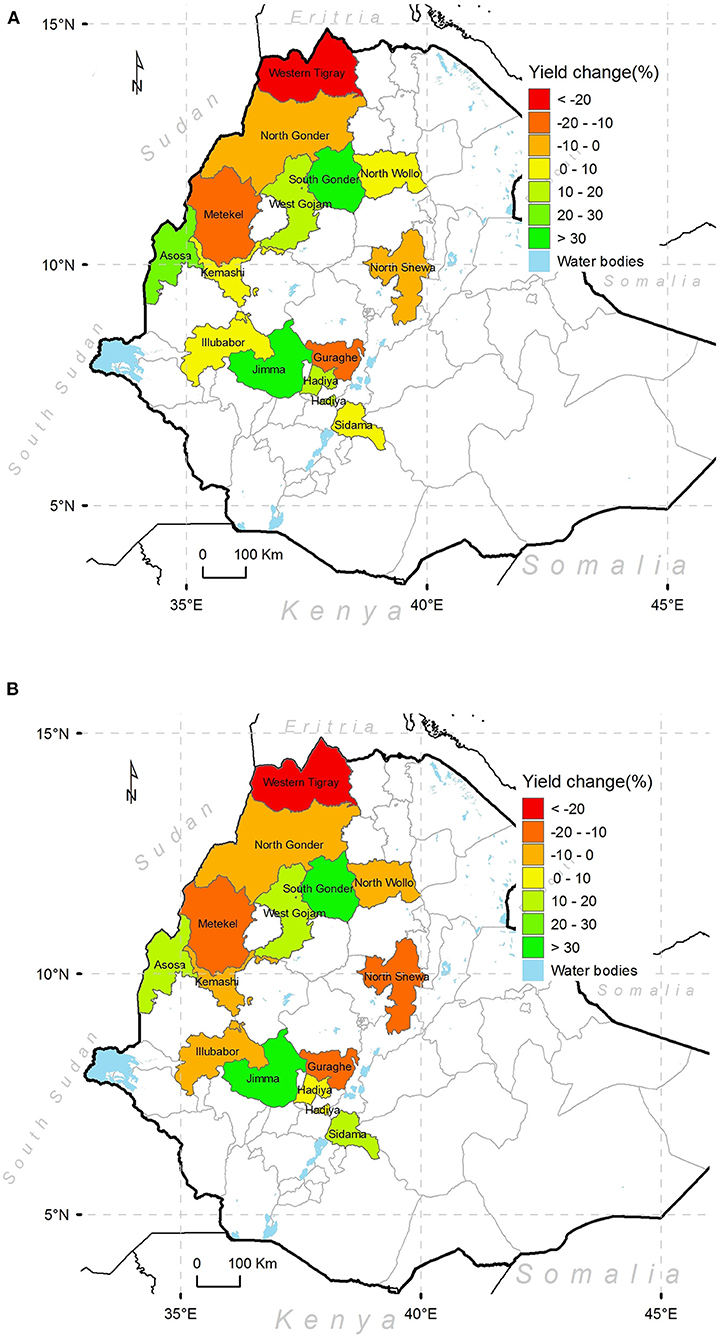
Figure 4. Projected maize yield changes in Ethiopia under climate change scenarios of (A) RCP2.6 and (B) RCP8.5.
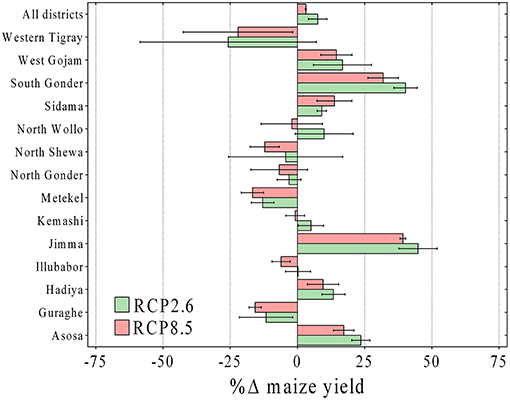
Figure 5. Projected climate change impacts on maize yield in Ethiopia by 2050 under RCP2.6 and RCP8.5 climate change scenarios.
While higher yield losses are projected under RCP8.5 than under RCP2.6 for most zones, positive effects of climate change on maize yield were higher for RCP2.6 (Figure 5). The results also show only small changes with no clear positive or negative signal of maize yield changes in North Wollo, Kemashi, and Illubabor zones. Overall, the result show that the impact of climate change on maize yields in Ethiopia to depend on the geography (zones in our case), the climate model (GCMs), and the climate scenario (RCPs). For instance, the MIROC5 GCM projected more severe impacts of climate change compared to the other two models.
Effect of Agroforestry Systems on Maize Yield Changes
We further evaluated the impact of our multi-purpose trees agroforestry in APSIM on maize yield in the study zones under current and projected climatic conditions. The results show that implementing agroforestry did not increase maize yield in most of the zones under current climate conditions. However, when the contribution of the agroforestry was assessed under projected climatic conditions, the results showed that the effect was positive for eight zones, and resulting in small changes (<5%) for a further three zones under both RCP2.6 and RCP8.5 (Figure 6). The adaptation benefits were especially higher under RCP8.5 and when 20% shade was applied. Positive multi-purpose trees agroforestry contribution was notable for zones that were projected to have maize grain yield losses as those projected to have yield gains had negative response of agroforestry shading. 10% agroforestry shade will reduce maize yield losses by 6.9% (RCP2.6) and 4.2 % (RCP8.5) while 20% shade will reduce maize yield losses by 11.5% (RCP2.6) and 11% (RCP8.5) for projected loss zones. Overall, the results indicate that changing the crop micro-climate alone may not provide yield increases but can reduce the yield losses by ensuring that yields remain stable in the face of changing climate conditions (Figure 7, Supplementary Material 9).
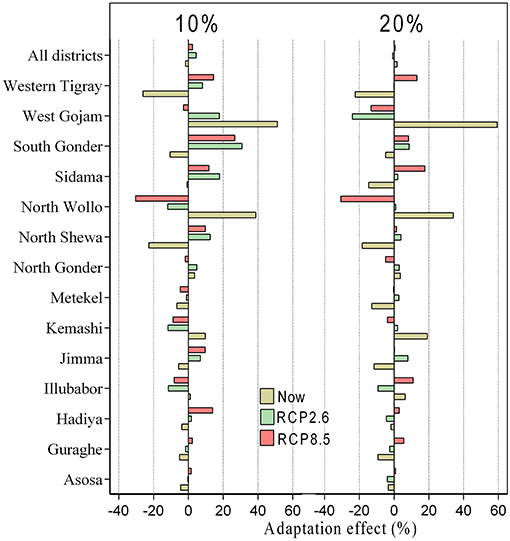
Figure 6. Adaptation effect of simulated multi-purpose trees agroforestry shading on maize yield changes in Ethiopia. The current is the yield change while the scenario is the difference between the projected changes with agroforestry and current condition with agroforestry.
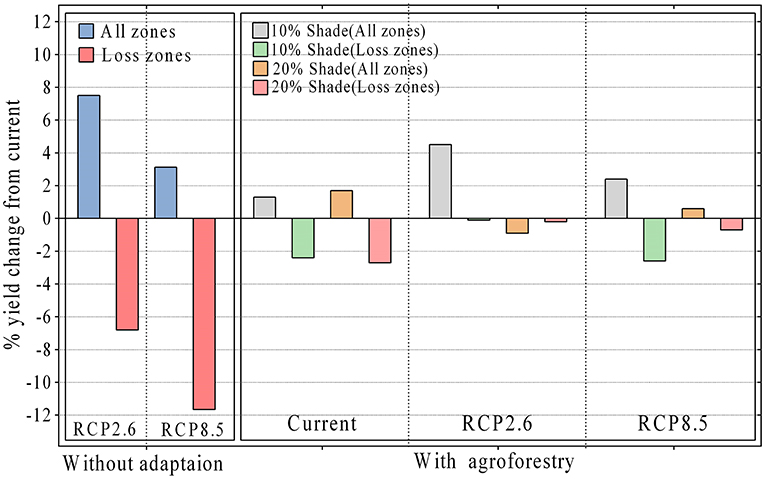
Figure 7. Effect of climate change on maize yields and with climate change and multi-purpose trees agroforestry as adaptation measure on national level in Ethiopia.
The sensitivity test showed that there was no association between current levels of fertilization and response to agroforestry systems for both 10 and 20% shading and climate scenarios (Table 4). This shows that the combined fertilization and climate regulation effect of agroforestry are responsible for climate change resilience, especially, under expected severe climate conditions such as under RCP8.5.
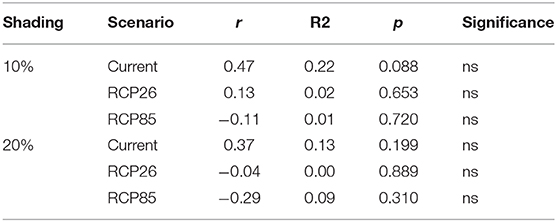
Table 4. Correlation between multi-purpose trees agroforestry response and baseline average zone level fertilizer use for the sample zones in Ethiopia.
Discussion
We observed significant year-to-year variability in maize production and also variation between the zones in Ethiopia in terms of maize yield levels. These results also reveal that there is a large risk of maize production to projected climate change as yield levels are generally very low and also dependent on annual weather patterns (Abate et al., 2015; Alemu and Mengistu, 2019) reflecting the country's heavy reliance on rain-fed maize production (Lemi, 2005; Bewket, 2009; Alemayehu and Bewket, 2016) with low level of bio-technology adoption and low fertilizer use (Abdulkadir et al., 2017). Both of these reduce productivity gains from crop variety developments over the years (Abate et al., 2015).
The key findings of this study are that the impacts of climate change on maize production in Ethiopia are uneven across geographic areas. The maize yield are projected to increase in some areas but decrease in others. The findings at country level that maize yield will increase under climate change corroborate with previous studies (Thornton et al., 2009; Araya et al., 2015; Muluneh et al., 2015; Alemayehu and Bewket, 2016; Thomas et al., 2019). We showed these positive climate change effects on maize yields are explained by strong increases in rainfall in parts of the country, which will increase the agronomic conditions for maize cultivation compared to current levels, especially in the highlands. In contrast, some area specific case studies in the country have projected decreases in maize yield of Ethiopia such as in Bako (Mamo et al., 2011), Melkassa (Abera et al., 2018) and the Central Rift Valley (Kassie et al., 2015). The previous studies are all indicative of the impacts of climate change on maize in yields in Ethiopia driven especially by limited water for the crops from higher evaporative demand with climate change (Kassie et al., 2015) and also increased rainfall variability for some areas particularly in the mehr season (Brown et al., 2017). The projected impacts of climate change on maize yields in Ethiopia pose risks in food availability in future for some regions, with its related impacts on livelihoods, health and general well-being. In this study, we provide spatially disaggregated impacts of climate change which show some areas as increasing in yields while others will become marginal, substantiating the findings of studies at national that show increases in yields and those local levels that show decreases in some areas. Therefore, the study results are relevant for national-level adaptation planning as adaptation measures such as cropping systems, irrigation, improved varieties and extension services and farmers' training can be proactively prepared for zones expecting yield losses. Concurrently, strengthening maize market value chains and transfer mechanisms between gain and loss zones can be planned to ensure that those zones projected to have increased yield will benefit economically by trading surplus while lose impacted will remain food secure by receiving from those projected to have positive effects. Whether and how the households in the impacted zones will afford to buy from the other gain zones requires further consideration beyond the scope of this study.
The study further provides quantitative assessments on the role of multi-purpose trees agroforestry to underpin the resilience of agricultural systems under climate change in Ethiopia. The results also show that agroforestry contribution to yield increases is surpassed by its ability to stabilize yields under limiting climatic conditions is significant in designing resilient agricultural systems in Ethiopia. Significant yield stabilization (as indicator for system resilience) of up to 30% is observed for Sidama, Jimma, and South Gonder in which climate change-related yield losses in the future have been projected. We, therefore, conclude that radiation interception and microclimatic temperature stabilization is more important that additional nitrogen from agroforestry for climate resilience. This implies that various agroforestry species which are planted or from natural regeneration are able to provide crop yield benefits under climate change, although leguminous nitrogen fixing trees provide additional nutrient benefits. Interestingly, we find that multi-purpose trees agroforestry implementation may reduce maize yield under current climate change but increase under projected climate conditions for some zones. This is explained by the fact that reducing radiation in high yield zones will obviously result in reduced maize biomass and yields especially with higher levels of shading (Dilla et al., 2018). Given the projected warming, the PAR reduction may maintain the crop production potential at required levels as radiation is not a limiting factor for tropical areas such as Ethiopia. It is therefore imperative that the agroforestry systems be designed according to not over-shade the crops and result in reduce yields as observed in some areas where the measure had a negative effect.
The results of multi-purpose trees agroforestry on maize yields show spatially variable effects in some zones, meaning that recommendations for climate change adaptation should be region sensitive. For example, our results indicate that 10% shading agroforestry system will increase yield in North Wollo by 39% under current climatic conditions but reduce it under climate change by 30% under RCP8.5 while for North Shewa it will decrease yields by 23% under current conditions and increase maize yield potential under both scenarios. While these regional disparities are directly explained by differences in altitude and resultant radiation levels, we demonstrate that multi-purpose trees agroforestry contribution under climate change may not be positive for all areas in Ethiopia at the modeling density of our study. Therefore, recommendations on effectiveness of agroforestry systems in climate buffering should not all be based on site-specific experimental studies as effects may be different elsewhere. Therefore, agroforestry systems in terms of choice and density of species should be carefully planned taking into consideration local conditions and responses. Otherwise, they may have maladaptive outcomes. Thus, the study findings adds on recommendations outlined by Alemu and Mengistu (2019), Ong and Kho (2015), and Nair and Toth (2016) to consider the potential drawbacks of agroforestry systems in sustainable rural development and food security. However, it is also important to mention that multi-purpose trees agroforestry has an effect on the entire agro-ecological system and that it has a high potential to provide added economic co-benefits from the use of the trees and tree products. Besides, agroforestry has non-pecuniary positive environmental externalities which contribute to the overall sustainable development of a given area. It is also imperative to note that agroforestry is not a panacea to climate change problems in maize systems but can be part of a basket of options that include adjustment of sowing dates, conservation agriculture, supplementary irrigation among others (Araya et al., 2015; Kassie et al., 2015; Muluneh et al., 2017a).
We report satisfactory model agreement between the observed and simulated maize yields for the sample zones, which is comparable to those obtained from experimental sites (see, for e.g., Smethurst et al., 2017). In addition, with a time series data of 10 years, we consider our modeling approach to be very skillful for assessing both climate change impacts and adaptation measures. Due to lack of data, only grain yield was considered for the model calibration and validation. Model calibration and evaluation would benefit from more data on phenology and biomass growth. The lack of calibration of model for simulating accurately the phenology and biomass growth before grain yield under current conditions could likely affects the growth response and thus yield predictions under future climate conditions. While our focus was not on crop physiological responses to multi-purpose trees agroforestry and climate change, we believe that warming and elevated CO2 conditions may result in more rapid accumulation of biomass and enhanced water use that generally lead to earlier onset of stress and likely reduced yield and WUE. This implication becomes stronger when considering crop-tree interactions under future climate and yet is not captured in this modeling. In addition, our agroforestry emulation and impacts assessment is based on the assumption that if the model is correctly parameterized and able to reproduce trends and ranges of maize yields, and yet its skill remains uncertain when non-calibration parameters are changed. This is largely true but the model performance was not consistent among the modeled zones which introduces levels of uncertainty that need to be considered in interpretation of the results.
Conclusions
We find that it is possible to simulate maize yields at zone level in Ethiopia with sufficient confidence for climate change impact assessment and adaptation evaluation. Three main conclusions can be drawn from the study results. First, the impacts of climate change are dependent on the zone (some losses and gains) with a projected national maize yield increase by 2050s. Second, multi-purpose trees agroforestry has huge potential in building resilience in maize systems threatened by climate change although its benefits are not even across the country. Third, a multi-purpose trees agroforestry emulation protocol such as the one presented in this study, is important in providing quantitative evaluation of the contribution to climate change resilience of agroforestry in agricultural systems. Therefore, the appraisal of agroforestry as adaptation measure to climate change, shall take into account region-specific environmental conditions and the potential of other adaptation measures.
Data Availability Statement
The original contributions presented in the study are included in the article/Supplementary Material, further inquiries can be directed to the corresponding author/s.
Author Contributions
AC and CG designed the research. AC, AY, and CG planned the research activities, reviewed, and fine-tuned the manuscript. AC and AY collected, analyzed, and curated data. AC ran the models and wrote draft manuscript. All authors contributed to the article and approved the submitted version.
Funding
This work was funded by the German Ministry for Economic Cooperation and Development (BMZ) in close collaboration with the Deutsche Gesellschaft für Internationale Zusammenarbeit (GIZ) GmbH under the country study in Ethiopia as part of the project AGRICA–Climate risk analyses for identifying and weighing adaptation strategies in sub-Saharan Africa.
Conflict of Interest
The authors declare that the research was conducted in the absence of any commercial or financial relationships that could be construed as a potential conflict of interest.
Acknowledgments
We are grateful to Lisa Murken for project management, and to Ponraj Arumugum and Stephanie Gleixner for climate data support.
Supplementary Material
The Supplementary Material for this article can be found online at: https://www.frontiersin.org/articles/10.3389/fagro.2021.609536/full#supplementary-material
References
Abate, T., Shiferaw, B., Menkir, A., Wegary, D., Kebede, Y., Tesfaye, K., et al. (2015). Factors that transformed maize productivity in Ethiopia. Food Secur. 7, 965–981. doi: 10.1007/s12571-015-0488-z
Abdulkadir, B., Kassa, S., Desalegn, T., Tadesse, K., Haileselassie, M., Fana, G., et al. (2017). Crop Response to Fertilizer Application in Ethiopia: A Review, eds L. Tamene, T. Amede, J. Kihara, D. Tibebe, S. Schulz (Addiss Ababa: CIAT/ICRISAT).
Abera, K., Crespo, O., Seid, J., and Mequanent, F. (2018). Simulating the impact of climate change on maize production in Ethiopia, East Africa. Environ. Syst. Res. 7:4. doi: 10.1186/s40068-018-0107-z
Alemayehu, A., and Bewket, W. (2016). Local climate variability and crop production in the central highlands of Ethiopia. Environ. Dev. 19, 36–48. doi: 10.1016/j.envdev.2016.06.002
Alemu, T., and Mengistu, A. (2019). “Impacts of climate change on food security in Ethiopia: adaptation and mitigation options: a review,” in Climate Change-Resilient Agriculture and Agroforestry, eds Castro P., Azul A., Leal Filho W., and Azeiteiro U (Cham: Springer), 397–412.
Araya, A., Hoogenboom, G., Luedeling, E., Hadgu, K. M., Kisekka, I., and Martorano, L. G. (2015). Assessment of maize growth and yield using crop models under present and future climate in southwestern Ethiopia. Agric. Forest Meteorol. 214, 252–265. doi: 10.1016/j.agrformet.2015.08.259
Araya, A., Kisekka, I., Girma, A., Hadgu, K., Tegebu, F., Kassa, A., et al. (2017). The challenges and opportunities for wheat production under future climate in Northern Ethiopia. J. Agric. Sci. 155:379. doi: 10.1017/S0021859616000460
Bedeke, S. B., Vanhove, W., Wordofa, M. G., Natarajan, K., and Van Damme, P. (2020). Vulnerability to climate change among maize-dependent smallholders in three districts of Ethiopia. Environ. Dev. Sust. 22, 693–718. doi: 10.1007/s10668-018-0215-y
Bewket, W. (2009). “Rainfall variability and crop production in Ethiopia: case study in the Amhara region”, in: Proceedings of the 16th International Conference of Ethiopian Studies (Trondheim: Norwegian University of Science and Technology Trondheim), 823–836.
Brown, M. E., Funk, C., Pedreros, D., Korecha, D., Lemma, M., Rowland, J., et al. (2017). A climate trend analysis of Ethiopia: examining subseasonal climate impacts on crops and pasture conditions. Clim. Change 142, 169–182. doi: 10.1007/s10584-017-1948-6
Cannavo, P., Sansoulet, J., Harmand, J.-M., Siles, P., Dreyer, E., and Vaast, P. (2011). Agroforestry associating coffee and Inga densiflora results in complementarity for water uptake and decreases deep drainage in Costa Rica. Agric. Ecosyst. Environ. 140, 1–13. doi: 10.1016/j.agee.2010.11.005
Cerda, A., Rodrigo-Comino, J., Novara, A., Brevik, E. C., Vaezi, A. R., Pulido, M., et al. (2018). Long-term impact of rainfed agricultural land abandonment on soil erosion in the Western Mediterranean basin. Prog. Phys. Geogr. Earth Environ. 42, 202–219. doi: 10.1177/0309133318758521
Cochrane, L., and Bekele, Y. W. (2018). Average crop yield (2001–2017) in Ethiopia: trends at national, regional and zonal levels. Data Brief 16, 1025–1033. doi: 10.1016/j.dib.2017.12.039
Conway, D., and Schipper, E. L. F. (2011). Adaptation to climate change in Africa: challenges and opportunities identified from Ethiopia. Glob. Environ. Change 21, 227–237. doi: 10.1016/j.gloenvcha.2010.07.013
CSA (2015). “Central statistical authority, agricultural sample survey report on area and production for major crops (private peasant holdings Meher season) for 20014/15,” in Statistical Bulletin 278. (Addis Ababa: The Federal Democratic Republic of Ethiopia).
Dagar, J., and Tewari, J. (2016). Agroforestry Research Developments: Anecdotal to Modern Science. New York, NY: Nova Publishers.
de Sousa, K., van Zonneveld, M., Holmgren, M., Kindt, R., and Ordoñez, J. C. (2019). The future of coffee and cocoa agroforestry in a warmer Mesoamerica. Sci. Rep. 9:8828. doi: 10.1038/s41598-019-45491-7
Deihimfard, R., Eyni-Nargeseh, H., and Mokhtassi-Bidgoli, A. (2018). Effect of future climate change on wheat yield and water use efficiency under semi-arid conditions as predicted by APSIM-wheat model. Int. J. Plant Prod. 12, 115–125. doi: 10.1007/s42106-018-0012-4
Dilla, A., Smethurst, P. J., Barry, K., Parsons, D., and Denboba, M. (2018). Potential of the APSIM model to simulate impacts of shading on maize productivity. Agrofor. Syst. 92, 1699–1709. doi: 10.1007/s10457-017-0119-0
Dimes, J., Rodriguez, D., and Potgieter, A. (2015). “Raising productivity of maize-based cropping systems in eastern and southern Africa: step-wise intensification options,” in Crop Physiology, eds Sadras, V. O and Calderini, D. F. (London: Elsevier), 93–110. doi: 10.1016/B978-0-12-417104-6.00005-4
Dumas, A., Andrisani, A., Bonnici, M., Graditi, G., Leanza, G., Madonia, M., et al. (2015). A new correlation between global solar energy radiation and daily temperature variations. Solar Energy 116, 117–124. doi: 10.1016/j.solener.2015.04.002
Dunne, J. P., John, J. G., Shevliakova, E., Stouffer, R. J., Krasting, J. P., Malyshev, S. L., et al. (2013). GFDL's ESM2 global coupled climate–carbon earth system models. Part II: carbon system formulation and baseline simulation characteristics. J. Clim. 26, 2247–2267. doi: 10.1175/JCLI-D-12-00150.1
Fainges, J. (2017). APSIM R Package: General Utility Functions for the Agricultural Production Systems Simulator, Version: 0.9.2.
Funk, C., Peterson, P., Landsfeld, M., Pedreros, D., Verdin, J., Shukla, S., et al. (2015). The climate hazards infrared precipitation with stations-a new environmental record for monitoring extremes. Sci. Data 2, 1–21.
Gaydon, D., Wang, E., Poulton, P., Ahmad, B., Ahmed, F., Akhter, S., et al. (2017). Evaluation of the APSIM model in cropping systems of Asia. Field Crops Res. 204, 52–75. doi: 10.1016/j.fcr.2016.12.015
Global Yield Gaps Atlas (2013). Information From Collected Literature for Ethiopia to be Used for Crop Modelling for Ethiopia. University of Nebrasak Lincoln/Wageningen University/Water for Food. Available online at: http://www.yieldgap.org/gygamaps/pdf/Details%20on%20crop%20information%20to%20calibrate%20crop%20models%20for%20Ethiopia.pdf (accessed April 7, 2019).
Hailesilassie, W. T. (2015). “Climate change adaptation and mitigation through agroforestry systems in Wolaita zone, southern highland of Ethiopia,” in Changing Climates, Ecosystemsand Environments Within Arid Southern Africa and Adjoining Regions, ed J. Runge (London: Palaeoecology of Africa), 209–215.
Han, E., Ines, A., and Koo, J. (2015). Global high-resolution soil profile database for crop modeling applications. Harvard Dataverse. 1, 1–37. doi: 10.7910/DVN/1PEEY0
Han, E., Ines, A. V., and Koo, J. (2019). Development of a 10-km resolution global soil profile dataset for crop modeling applications. Environ. Model. Softw. 119, 70–83. doi: 10.1016/j.envsoft.2019.05.012
Hengl, T., de Jesus, J. M., Heuvelink, G. B., Gonzalez, M. R., Kilibarda, M., Blagoti,ć, A., et al. (2017). SoilGrids250m: Global gridded soil information based on machine learning. PLoS ONE 12:e0169748. doi: 10.1371/journal.pone.0169748
Hoegh-Guldberg, O., Jacob, D., Bindi, M., Brown, S., Camilloni, I., Diedhiou, A., et al. (2018). Impacts of 1.5 C Global Warming on Natural and Human Systems. Global warming of 1.5° C. (Helsinki: IPCC).
Holzworth, D., Huth, N., Fainges, J., Herrmann, N., Zurcher, E., Brown, H., et al. (2020). “APSIM next generation: the final frontier?,” in Proceedings-21st International Congress on Modelling and Simulation, MODSIM 2015: Modelling and Simulation Society of Australia and New Zealand Inc. (MSSANZ), (Sydney), 347–353.
Holzworth, D. P., Huth, N. I., deVoil, P. G., Zurcher, E. J., Herrmann, N. I., McLean, G., et al. (2014). APSIM–evolution towards a new generation of agricultural systems simulation. Environ. Model. Softw. 62, 327–350. doi: 10.1016/j.envsoft.2014.07.009
Jones, C., Hughes, J., Bellouin, N., Hardiman, S., Jones, G., Knight, J., et al. (2011). The HadGEM2-ES implementation of CMIP5 centennial simulations. Geosci. Model Dev. 4, 543–570. doi: 10.5194/gmd-4-543-2011
Kassie, B. T., Asseng, S., Rotter, R. P., Hengsdijk, H., Ruane, A. C., and Van Ittersum, M. K. (2015). Exploring climate change impacts and adaptation options for maize production in the central Rift valley of Ethiopia using different climate change scenarios and crop models. Clim. Change 129, 145–158. doi: 10.1007/s10584-014-1322-x
Kassie, G. W. (2018). Agroforestry and farm income diversification: synergy or trade-off? The case of Ethiopia. Environ. Syst. Res. 6:8. doi: 10.1186/s40068-017-0085-6
Keating, B. A., Carberry, P. S., Hammer, G. L., Probert, M. E., Robertson, M. J., Holzworth, D., et al. (2003). An overview of APSIM, a model designed for farming systems simulation. Euro. J. Agron. 18, 267–288. doi: 10.1016/S1161-0301(02)00108-9
Keesstra, S. D., Bouma, J., Wallinga, J., Tittonell, P., Smith, P., Cerd,à, A., et al. (2016). The significance of soils and soil science towards realization of the United nations sustainable development goals. Soil 2, 111–128. doi: 10.5194/soil-2-111-2016
Lal, R., Kraybill, D., Hansen, D. O., Singh, B. R., Mosogoya, T., and Eik, L. O. (2016). Climate Change and Multi-Dimensional Sustainability in African Agriculture. Columbus: Springer.
Lange, S. (2016). “EartH2Observe, WFDEI and ERA-Interim Data Merged and Bias-corrected for ISIMIP (EWEMBI), GFZ Data Services”. Potsdam: ISIMIP.
Lemi, A. (2005). Rainfall probability and agricultural yield in Ethiopia. East. Afr. Soc. Sci. Res. Rev. 21, 57–96. doi: 10.1353/eas.2005.0004
Liu, Z., Yang, X., Lin, X., Gowda, P., Lv, S., and Wang, J. (2018). Climate zones determine where substantial increases of maize yields can be attained in Northeast China. Clim. Change 149, 473–487. doi: 10.1007/s10584-018-2243-x
Lobell, D. B. (2014). Climate change adaptation in crop production: beware of illusions. Glob. Food Secur. 3, 72–76. doi: 10.1016/j.gfs.2014.05.002
Lott, J., Khan, A., Black, C., and Ong, C. (2003). Water use in a Grevillea robusta–maize overstorey agroforestry system in semi-arid Kenya. For. Ecol. Manage. 180, 45–59. doi: 10.1016/S0378-1127(02)00603-5
Lott, J., Ong, C., and Black, C. (2009). Understorey microclimate and crop performance in a Grevillea robusta-based agroforestry system in semi-arid Kenya. Agric. For. Meteorol. 149, 1140–1151. doi: 10.1016/j.agrformet.2009.02.002
Luedeling, E., Kindt, R., Huth, N. I., and Koenig, K. (2014). Agroforestry systems in a changing climate—challenges in projecting future performance. Curr. Opin Environ. Sust. 6, 1–7. doi: 10.1016/j.cosust.2013.07.013
Mafongoya, P., and Dzowela, B. (1999). Biomass production of tree fallows and their residual effect on maize in Zimbabwe. Agrofor. Syst. 47, 139–151. doi: 10.1023/A:1006246928615
Mafongoya, P., Giller, K., and Palm, C. (1998). “Decomposition and nitrogen release patterns of tree prunings and litter,” in Directions in Tropical Agroforestry Research (Dordrecht: Springer), 77–97. doi: 10.1007/978-94-015-9008-2_3
Mahmood, R., and Hubbard, K. G. (2002). Effect of time of temperature observation and estimation of daily solar radiation for the Northern Great Plains, USA. Agron. J. 94, 723–733.
Mamo, G., Getachew, F., and Legesse, G. (2011). “The potential impacts of climate change–maize farming system complex in ethiopia: towards retrofitting adaptation and mitigation options,” in Meeting the Challenges of Global Climate Change and Food Security through Innovative Maize Research (Addis Ababa), 151.
Mbow, C., Van Noordwijk, M., Luedeling, E., Neufeldt, H., Minang, P. A., and Kowero, G. (2014). Agroforestry solutions to address food security and climate change challenges in Africa. Curr. Opin. Environ. Sust. 6, 61–67. doi: 10.1016/j.cosust.2013.10.014
Moreno, G., Aviron, S., Berg, S., Crous-Duran, J., Franca, A., de Jalón, S. G., et al. (2018). Agroforestry systems of high nature and cultural value in Europe: provision of commercial goods and other ecosystem services. Agrofor. Syst. 92, 877–891. doi: 10.1007/s10457-017-0126-1
Moriasi, D. N., Arnold, J. G., Van Liew, M. W., Bingner, R. L., Harmel, R. D., and Veith, T. L. (2007). Model evaluation guidelines for systematic quantification of accuracy in watershed simulations. Trans. ASABE 50, 885–900. doi: 10.13031/2013.23153
Muluneh, A., Biazin, B., Stroosnijder, L., Bewket, W., and Keesstra, S. (2015). Impact of predicted changes in rainfall and atmospheric carbon dioxide on maize and wheat yields in the central rift valley of Ethiopia. Reg. Environ. Change 15, 1105–1119. doi: 10.1007/s10113-014-0685-x
Muluneh, A., Stroosnijder, L., Keesstra, S., and Biazin, B. (2017a). Adapting to climate change for food security in the Rift Valley dry lands of Ethiopia: supplemental irrigation, plant density and sowing date. J. Agric. Sci. 155, 703–724. doi: 10.1017/S0021859616000897
Muluneh, A., van Loon, E., Bewket, W., Keesstra, S., Stroosnijder, L., and Burka, A. (2017b). Effects of long-term deforestation and remnant forests on rainfall and temperature in the central rift valley of Ethiopia. For. Ecosyst. 4:23. doi: 10.1186/s40663-017-0109-8
Nair, P. R., and Toth, G. G. (2016). “Measuring agricultural sustainability in agroforestry systems,” in Climate Change and Multi-Dimensional Sustainability in African Agriculture, eds Lal, R., Kraybill, D., Hansen, D., Singh, B., Mosogoya, T., and Eik, L. (London: Springer), 365–394. doi: 10.1007/978-3-319-41238-2_20
Ong, C., Black, C., Wallace, J., Khan, A., Lott, J., Jackson, N., et al. (2000). Productivity, microclimate and water use in Grevillea robusta-based agroforestry systems on hillslopes in semi-arid Kenya. Agric. Ecosyst. Environ. 80, 121–141. doi: 10.1016/S0167-8809(00)00144-4
Ong, C., and Kho, R. (2015). “A framework for quantifying the various effects of tree-crop interactions,” in Tree–Crop Interactions: Agroforestry in a Changing Climate (CABI), 1–23. doi: 10.1079/9781780645117.0001
Osbahr, H., Twyman, C., Adger, W. N., and Thomas, D. S. (2010). Evaluating successful livelihood adaptation to climate variability and change in southern Africa. Ecology and Society 15, 27–49. doi: 10.5751/ES-03388-150227
Palm, C. A. (1995). “Contribution of agroforestry trees to nutrient requirements of intercropped plants,” in Agroforestry: Science, Policy and Practice (Springer), 105–124. doi: 10.1007/978-94-017-0681-0_5
Pardon, P., Mertens, J., Reubens, B., Reheul, D., Coussement, T., Elsen, A., et al. (2019). Juglans regia (walnut) in temperate arable agroforestry systems: effects on soil characteristics, arthropod diversity and crop yield. Renew. Agric. Food Syst. 35, 535–549. doi: 10.1017/S1742170519000176
Pardon, P., Reubens, B., Mertens, J., Verheyen, K., De Frenne, P., De Smet, G., et al. (2018). Effects of temperate agroforestry on yield and quality of different arable intercrops. Agric. Syst. 166, 135–151. doi: 10.1016/j.agsy.2018.08.008
Partey, S., Quashie-Sam, S., Thevathasan, N., and Gordon, A. (2011). Decomposition and nutrient release patterns of the leaf biomass of the wild sunflower (Tithonia diversifolia): a comparative study with four leguminous agroforestry species. Agrofor. Syst. 81, 123–134. doi: 10.1007/s10457-010-9360-5
Pembleton, K., Cullen, B., Rawnsley, R., Harrison, M., and Ramilan, T. (2016). Modelling the resilience of forage crop production to future climate change in the dairy regions of Southeastern Australia using APSIM. J. Agric. Sci. 154, 1131–1152. doi: 10.1017/S0021859615001185
Qiao, X., Sai, L., Chen, X., Xue, L., and Lei, J. (2019). Impact of fruit-tree shade intensity on the growth, yield, and quality of intercropped wheat. PLoS ONE 14:e0203238. doi: 10.1371/journal.pone.0203238
Seyoum, S., Rachaputi, R., Chauhan, Y., Prasanna, B., and Fekybelu, S. (2018). Application of the APSIM model to exploit G × E × M interactions for maize improvement in Ethiopia. Field Crops Res. 217, 113–124. doi: 10.1016/j.fcr.2017.12.012
Sida, T. S., Baudron, F., Kim, H., and Giller, K. E. (2018). Climate-smart agroforestry: faidherbia albida trees buffer wheat against climatic extremes in the central rift valley of Ethiopia. Agric. For. Meteorol. 248, 339–347. doi: 10.1016/j.agrformet.2017.10.013
Singh, P., and Singh, B. (2016). Biomass and nitrogen dynamics of fine roots of poplar under differential N and P levels in an agroforestry system in Punjab. Trop. Ecol. 57, 143–152.
Smethurst, P. J., Huth, N. I., Masikati, P., Sileshi, G. W., Akinnifesi, F. K., Wilson, J., et al. (2017). Accurate crop yield predictions from modelling tree-crop interactions in gliricidia-maize agroforestry. Agric. Syst. 155, 70–77. doi: 10.1016/j.agsy.2017.04.008
Soufizadeh, S., Munaro, E., McLean, G., Massignam, A., van Oosterom, E., Chapman, S., et al. (2018). Modelling the nitrogen dynamics of maize crops–Enhancing the APSIM maize model. Euro. J. Agron. 100, 118–131. doi: 10.1016/j.eja.2017.12.007
Speak, A., Montagnani, L., Wellstein, C., and Zerbe, S. (2020). The influence of tree traits on urban ground surface shade cooling. Landsc. Urban Plan 197:103748. doi: 10.1016/j.landurbplan.2020.103748
Stanfill, B. (2015). Apsimr: Edit, Run, and Evaluate APSIM Simulations Easily Using R. R package Version 1.2.
Sun, H., Zhang, X., Wang, E., Chen, S., Shao, L., and Qin, W. (2016). Assessing the contribution of weather and management to the annual yield variation of summer maize using APSIM in the North China plain. Field Crops Res. 194, 94–102. doi: 10.1016/j.fcr.2016.05.007
Tachie-Obeng, E., Akponikpe, P., and Adiku, S. (2013). Considering effective adaptation options to impacts of climate change for maize production in Ghana. Environ. Dev. 5, 131–145. doi: 10.1016/j.envdev.2012.11.008
Taffesse, A. S., Dorosh, P., and Gemessa, S. A. (2012). Crop production in Ethiopia: regional patterns and trends. Food Agric. Ethiopia Prog. Policy Chall.0016, 53–83.
Tefera, T., Elias, E., and van Beek, C. (2020). Determinants of smallholder farmers' decisions on fertilizer use for cereal crops in the Ethiopian highlands. Exp. Agric. 1:11. doi: 10.1017/S001447972000023X
Teluguntla, P., Thenkabail, P., Xiong, J., Gumma, M., Giri, C., Milesi, C., et al. (2015). Global Food Security Support Analysis Data (GFSAD) at Nominal 1 Km (GCAD) Derived From Remote Sensing in Support of Food Security in the Twenty-First Century: Current Achievements and Future Possibilities, Hydrabad: ICRISAT.
Thomas, T. S., Dorosh, P. A., and Robertson, R. D. (2019). Climate change impacts on crop yields in Ethiopia. Int Food Policy Res. Inst. 130, 1–16. doi: 10.2499/9780896296916_04
Thornton, P. K., Jones, P. G., Alagarswamy, G., and Andresen, J. (2009). Spatial variation of crop yield response to climate change in East Africa. Glob. Environ. Change 19, 54–65. doi: 10.1016/j.gloenvcha.2008.08.005
Udawatta, R. P., Rankoth, L., and Jose, S. (2019). Agroforestry and biodiversity. Sustainability 11:2879. doi: 10.3390/su11102879
Watanabe, S., Hajima, T., Sudo, K., Nagashima, T., Takemura, T., Okajima, H., et al. (2011). MIROC-ESM 2010: Model description and basic results of CMIP5-20c3m experiments. Geosci. Model Dev. 4:845. doi: 10.5194/gmd-4-845-2011
Weedon, G. P., Balsamo, G., Bellouin, N., Gomes, S., Best, M. J., and Viterbo, P. (2014). The WFDEI meteorological forcing data set: WATCH forcing data methodology applied to ERA-interim reanalysis data. Water Resour. Res. 50, 7505–7514. doi: 10.1002/2014WR015638
Zhang, Y., Feng, L., Wang, J., Wang, E., and Xu, Y. (2013). Using APSIM to explore wheat yield response to climate change in the North China plain: the predicted adaptation of wheat cultivar types to vernalization. J. Agric. Sci. 151, 836–848. doi: 10.1017/S0021859612000883
Zhao, Y., Zhang, B., and Hill, R. (2012). Water use assessment in alley cropping systems within subtropical China. Agroforestry systems 84, 243–259. doi: 10.1007/s10457-011-9458-4
Keywords: agroforestry, process-based model, maize, climate change, Ethiopia
Citation: Chemura A, Yalew AW and Gornott C (2021) Quantifying Agroforestry Yield Buffering Potential Under Climate Change in the Smallholder Maize Farming Systems of Ethiopia. Front. Agron. 3:609536. doi: 10.3389/fagro.2021.609536
Received: 23 September 2020; Accepted: 13 January 2021;
Published: 01 February 2021.
Edited by:
Sudarshan Kumar Dutta, Mohammed VI Polytechnic University, MoroccoReviewed by:
Artemi Cerdà, University of Valencia, SpainMichelle M. Wander, University of Illinois at Urbana-Champaign, United States
Kokou Adambounou Amouzou, African Plant Nutrition Institute (APNI), Morocco
Copyright © 2021 Chemura, Yalew and Gornott. This is an open-access article distributed under the terms of the Creative Commons Attribution License (CC BY). The use, distribution or reproduction in other forums is permitted, provided the original author(s) and the copyright owner(s) are credited and that the original publication in this journal is cited, in accordance with accepted academic practice. No use, distribution or reproduction is permitted which does not comply with these terms.
*Correspondence: Abel Chemura, Y2hlbXVyYUBwaWstcG90c2RhbS5kZQ==