- 1Grassland Management, Department of Grassland and Forage Research, Agricultural Centre for Cattle, Grassland, Dairy, Game and Fishery of Baden-Württemberg, Aulendorf, Germany
- 2Grassland Science, Department of Crop Science, University of Göttingen, Göttingen, Germany
- 3Geo-Laboratory, Department of Geography, University of Vechta, Vechta, Germany
- 4Centre for Biodiversity and Sustainable Land Use University of Göttingen, Göttingen, Germany
In pasture-based dairy farming, animal behavior data can improve data-driven pasture management. Information on the grazing behavior of dairy cows can be retrieved from sensor-based data. However, this approach generally requires sophisticated sensor equipment and involves labor-intensive animal observations. As an alternative, the use of data from simple and commonly used collar-mounted accelerometers and global navigation system services (GNSS) receivers was investigated. In our on-farm study, cows grazed in a rotational or in a continuous grazing system, with a higher sward or a lower sward height, respectively. As an indicator of grazing activity, the overall dynamic body acceleration (ODBA) was calculated from the accelerometer data. After differentiating the grazing process (forage uptake) into grazing with grazing steps (i.e., moving to the next feeding station) and grazing without grazing steps (i.e., true standing) from the GNSS data, only a negligible effect of grazing steps on ODBA was found. The ODBA was higher in short swards (3.47 m s−2) than in tall swards (2.88 m s−2). The ODBA was also affected by the time of the day, with major grazing activity around dusk. These findings show the potential of simple accelerometers on collars in research on grazing patterns and cattle monitoring and for use in pasture management. The ODBA can be calculated from any three-dimensional accelerometer also from existing commercial technology, which allows a wide in-field application.
1 Introduction
For the behavior classification of cattle, collar-mounted sensors, which are usually based on accelerometers, are widely used in precision livestock farming. The aim is to monitor animal health and welfare and the production parameters of the individual animal (Halachmi et al., 2019). The monitoring of the grazing process as the uptake of forage of cows is more complex, and research studies have often involved the use of noseband or jaw sensors, which are uncommon in commercial farming (Chapa et al., 2020). For an on-farm setting, a simpler approach with collar-mounted sensors could be a solution as such sensors are already established in many dairy farms. Especially with the increasing interest in sensor-based virtual fencing technology, an extension of data-driven pasture management with animal data could be of high value (Hamidi et al., 2023). The grazing behavior of cattle is influenced by a number of factors including the sward height, the time of the day, and grazing bouts (Taweel et al., 2004). The sward height is considered a major influence on the grazing behavior of cattle: a number of studies on noseband and jaw sensors have shown that the bite rate and the head and jaw movement increase with decreasing sward heights (Gibb et al., 1997; Shafiullah et al., 2019; Rombach et al., 2022). The challenge, however, is that an isolated analysis of the jaw or head movement seems hardly feasible using a collar-based approach. Nevertheless, the study of Guo et al. (2018) indicated the potential of movement-measuring devices at the neck of sheep to discriminate grazing in different sward heights. The overall dynamic body acceleration (ODBA) extracted from three-dimensional acceleration measurements is a frequently used proxy for activity (Wilson et al., 2006; Miwa et al., 2015). The magnitude of the ODBA can be affected by head movements and by spatial movements such as walking. As the sward height affects the step number and the walking distance of grazing dairy cows, a differentiation of movement in non-spatial and spatial acceleration is necessary for an exact assessment of the ODBA. Spatial movement can be tracked using step sensors or by spatial tracking with global navigation system services (GNSS). The step number is higher and the walking distance is greater in short swards [“Kurzrasen,” continuous grazing (CG)] than in higher swards [strip or rotational grazing (RG)] (Hoekstra et al., 2019; Obermeyer and Kayser, 2023). During the grazing process (uptake of forage) itself, the cow is at one place (static) or it makes a very short move to the next feeding spot. The spatial movement during the grazing process is characterized by grazing steps, which are defined as a single or a few steps of the cow moving from one feeding station to the next (Rook et al., 2004; Menegazzi et al., 2021). The GNSS technology is widely used in grazing research on topics such as habitat use (McGranahan et al., 2018), animal relations (Keshavarzi et al., 2022), and behavior classification (Williams et al., 2022). However, most of the commercially available technologies for animal monitoring operate only with inertial motion units (IMUs; accelerometer and gyroscope), with the major exception of virtual fencing technology (Goliński et al., 2023). This means that the monitoring of grazing with only IMUs would be more practical in most cases.
Our overall objective was to assess, in an on-farm setting, whether it is possible to use information based on the ODBA derived from commonly used collar-mounted accelerometers to describe the grazing process. This was performed in a case study with two different grazing systems (CG and RG) mainly characterized by different sward heights. The hypotheses were:
1. The ODBA is affected by spatial movement (grazing steps) within the grazing process.
2. IMU is sufficient to discriminate grazing with and without spatial movement.
3. Cow activity during the grazing process indicated by the ODBA is higher in CG compared with the RG grazing system and is equally influenced by different grazing bouts.
2 Materials and methods
The experiment was conducted in 2022 on a conventional pasture-based dairy farm located in the “Bergische Land,” North Rhine Westphalia, Germany (51.1460, 7.5060; World Geodetic System 1984), at an altitude of 385 m a.s.l. The long-term annual precipitation (1991–2020) was 1,163.7 mm, while the long-term daily mean temperature was 8.8°C. The grazing block of the farm covers 42.3 ha. The grassland can be classified as Lolio Cynosuretum dominated by Lolium perenne, Poa pratensis, and Trifolium repens. The pasture was managed in two ways: the majority of the grazing block (38.1 ha) was managed as CG targeting a constant compressed sward height (CSH) of 6 cm. This area was split into two paddocks, which were grazed at alternate days. The remaining 4.2 ha were managed as RG with a pre-grazing target CSH of 10 cm and a post-grazing target CSH of 5 cm. The RG area was split into four paddocks of approximately 1 ha, with each paddock allocated in turn as a 24-h grazing. The 24-h grazings started after the morning milking, were interrupted by the afternoon milking, and ended with the following morning milking. However, during the last run in August, the RG paddocks were allocated for only 12 h, either day or night, as a drought led to an acute shortage of available feed. The CSH was recorded with a Jenquip EC20 (Fielding, New Zealand) rising plate meter with at least 150 measurements per paddock before and after the cow accessed a paddock (Table 1).
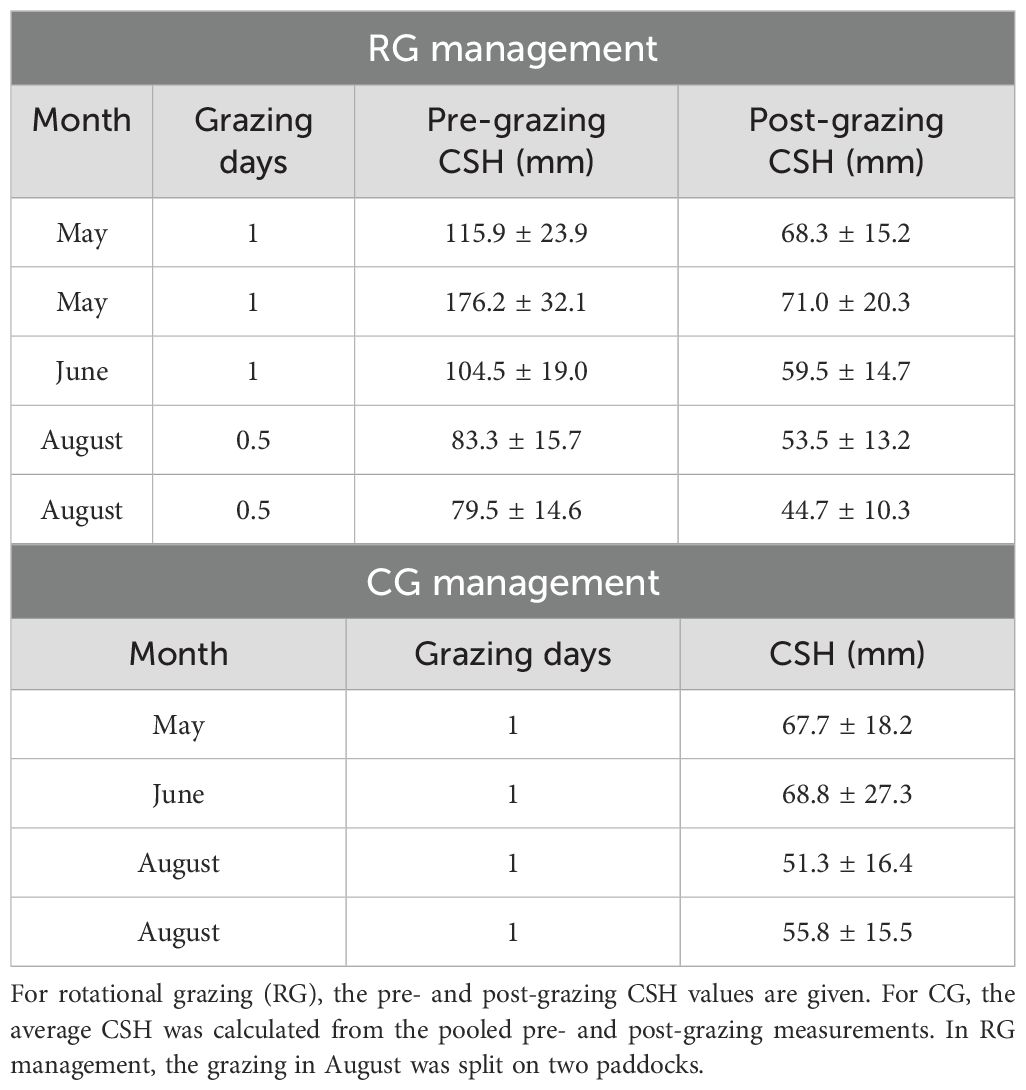
Table 1. Average ± standard deviation of the compressed sward height (CSH) of the eight grazing days.
The herd of the farm consisted of 93 crossbreed cows (Jersey × Friesian, New Zealand Strain) with a milk yield of 7,057.7 ± 1,294.6 kg (average ± SD) energy-corrected milk in 2022 for the standard 305-day lactation. The farm practiced seasonal calving beginning in January. During the grazing season (April–November), the cows were grazed without any further feed except for minerals.
Cow activity and position were tracked in three runs. The three runs had a duration of 2 or 3 days, which summed up to a total of eight tracking days equally divided between the two pasture management strategies, RG and CG (Table 1). On average, 23 cows, which were randomly selected and stratified by age, were tracked. Self-assembled and programmed cow trackers based on the Arduino environment and constructed from breakout boards obtained from Adafruit (New York, NY, USA) were used for the tracking (Obermeyer and Kayser, 2023). The tracker recorded the spatial position and the time at a frequency of 0.5 Hz. The circular error probable was calculated from 509 measurements under field conditions comparing the GNSS measurements against a reference point defined with an RTK GNSS device (ArduSimple, Andorra la Vella, Andorra) (Kalafus and Chin, 1986). The circular errors for 10%, 50%, and 90% were 2.11, 3.92, and 8.54 m, respectively. The acceleration and rotation in three dimensions was read at a frequency of 20 Hz. The accelerometer and the gyroscope range were 4 g and 250 deg s−1, respectively. The tracking of the animals was calibrated against visual observations. The observations were based on an ethogram including the following behaviors: grazing, ruminating, walking, resting, and drinking. The behavior grazing was defined as feed intake on pasture, the grazing process, including mastication with an elevated head and single steps (Delagarde and Lamberton, 2015; Barwick et al., 2018). For further details on the herd, the cow tracker, and the visual observation procedure, see Obermeyer and Kayser (2023).
The classification of the cow behaviors such as grazing, ruminating, walking, resting, and drinking followed the approach suggested by Riaboff et al. (2022). A detailed description of the classification procedure for the presented data is given in Obermeyer and Kayser (2023). In brief, the first step consisted of the extraction of further axes from the raw recordings of the accelerometer, gyroscope, and the GNSS module. The second step involved the segmentation of all axes in windows of 10-s width with an overlap of 75%. Subsequently, each axis was summarized in every window by multiple summary features, resulting in a temporal feature resolution of 2.5 s. Among others, these features included the mean, variance, median, quartiles, and range. In the third step, the pre-processed data retrieved from the cow trackers were merged with the visual behavior observations by time stamp and animal identity. The fourth and final step was the machine learning procedure with training and subsequent validation of a random forest algorithm for behavior classification.
For the data presented here, only the behavior “grazing” was of interest. Accordingly, the behavior categories were simplified into two classes: “grazing” and “other,” with “other” including walking, ruminating, resting, and drinking. The timeline of behavior was smoothed by a centered running window with a width of 1 min counting the occurrence of the two behavior classes. The more frequent behavior was subsequently used as the defining behavior for each minute. The aim of the smoothing was to decrease the influence of a single misclassification and of the short expressions of other behaviors during the grazing process.
Grazing bouts were defined for the individual cow by intervals of 7 min for the intra- and inter-meal duration (Pérez-Ramírez et al., 2008; Werner et al., 2018). This means that a grazing bout needs to have at least a duration of 7 min without interruption in order to be identified as a grazing bout. If within another 7 min after the end of the present grazing bout another grazing bout emerged, these bouts were merged. The major diurnal grazing bouts were defined by the overall herd behavior, as some degree of synchronization among cows is to be expected (Rook and Huckle, 1995). Following this, the occurrence of a major grazing bout was defined by the fact that ≥50% of the tracked cows grazed simultaneously. For further analysis, the full dataset was than subset to the herd grazing bouts by removing data when less than 50% of the herd was grazing. By doing this, a potential excessive influence of a few grazing animals during longer periods of non-grazing of the majority of the herd could be avoided. The class “morning bout” (MB) contained grazing bouts from 1 h before to 5 h after dawn, “evening bout” (EB) contained the same intervals at dusk, the class “day bout” (DB) contained grazing bouts between MB and EB, and the “night bout” (NB) contained the bouts between EB and MB (Barrett et al., 2001; Gregorini, 2012). Apart from the factor grazing bout, the factor grazing management with the two levels—CG and RG—was considered. During the grazing process, the spatial movement (i.e., the grazing steps from one feeding station to the next) needs to be addressed. The walking derived from the GNSS described the spatial movement of the cows while grazing based on the distance traveled in each 2.5-s segment. However, the raw distance contains the grazing steps, therefore not only the actual spatial movement between feeding stations but also the noise from non-sufficient GNSS precision. The behavior grazing was therefore classified into grazing activity with spatial movement (i.e., grazing steps between feeding stations) and grazing without any spatial movement (i.e., true standing) for every individual cow. This discrimination was based on finding a threshold in the distance traveled per 2.5-s segment. This was performed by calculating a density function of the walking speed medians across all cows and subsequent visual inspections. The density function had a bimodal shape in the interval of the highest density from 0 to 4 m min−1. The local minimum in this interval was calculated for every individual cow and was used as a threshold (Figure 1).
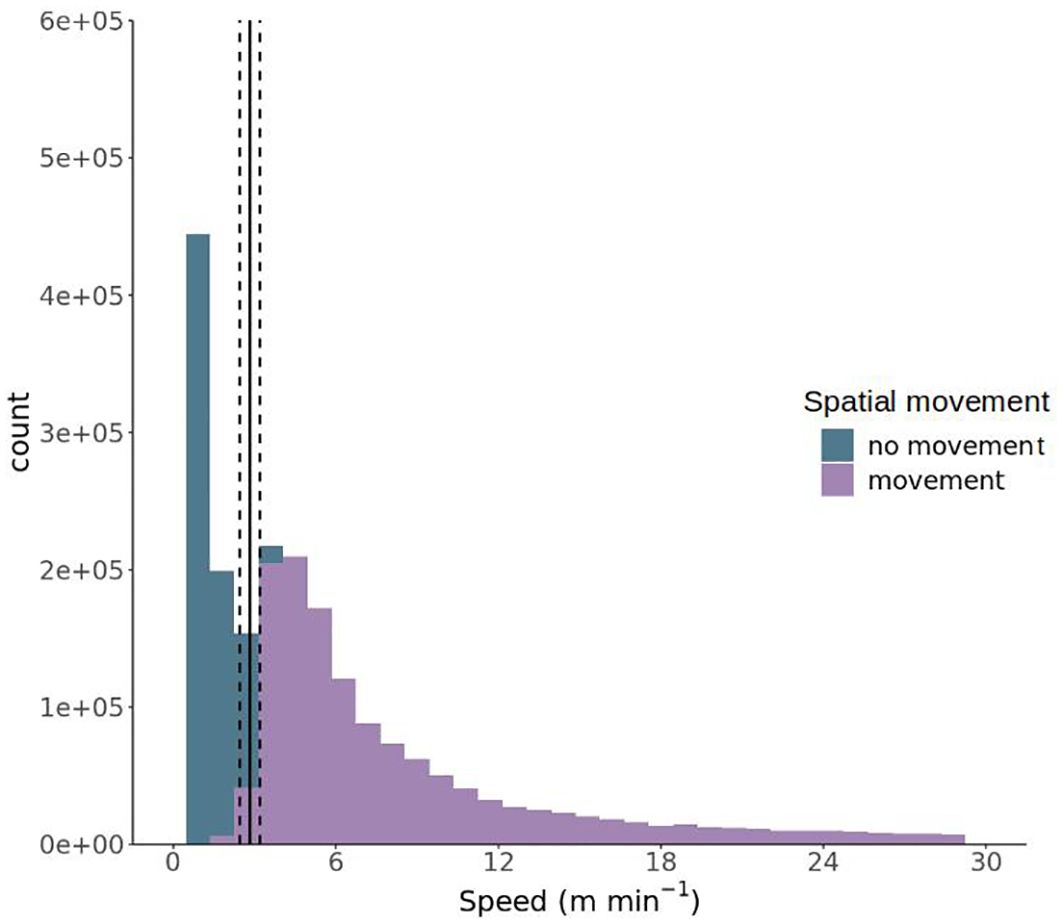
Figure 1. Histogram of the median walking speed (in meters per minute) differentiated in the two classes “no spatial movement” and “spatial movement” during the grazing process. The solid line indicates the average threshold for the differentiation of the two movement classes across individual cows. The dashed lines indicate the standard deviation of the thresholds. The bin width of the histogram is 0.9 m min−1, which was chosen not only as a compromise between sufficient details but also to allow a clear visual assessment and to avoid an overfitting appearance.
The ODBA median of the 2.5-s segments was used for the exploration of activity. The ODBA was calculated against the background of the 10-s sliding window as follows:
where a is the magnitude of acceleration and ā is the acceleration of the 10-s sliding window (usually close to the magnitude of gravitation). x, y, and z represent the three raw acceleration axes in three dimensions. The extracted ODBA axis was favored as it combines the raw axes. Therefore, the extracted axis can be considered as independent from the sensor position and robust against differences in the sensor mounts between and within animals.
For the statistical analysis, mixed linear models were used. Some animals were tracked more than once within the experiment. To consider this, the identity of the cow and the identity of the cow nested in the run were used as random effects. All statistical models were fitted with the package “glmmTMB” (Brooks et al., 2017) and subsequently validated by inspection of the residual plots with the package “DAHRMa” (Hartig, 2022). P-values were produced with the marginal Wald test. The package “emmeans” was used for the post-hoc calculation of means with confidence intervals (Lenth, 2021).
The differentiation between grazing with and without spatial movement was obtained from the GNSS data, as described above. We further attempted to replicate this classification from the IMU data only including the three-dimensional accelerometer and the gyroscope data. A random forest (package “ranger”) (Wright and Ziegler, 2017) was trained on the summary statistics of the 2.5-s segments of the raw accelerometer and gyroscope axes and on the summary features of the axes (i.e., vectorial dynamic body acceleration, ODBA, signal magnitude, signal magnitude area, and rotational activity) to classify the grazing segments with and without spatial movement. For details on the summary statistics and summary features, see Obermeyer and Kayser (2023). For validation, 15% of the IMU data of individual cows were retained, with the remaining data used for algorithm training and tuning. The hyperparameter tuning was performed in a grid search and 10-fold cross-validation in the package “caret” (Kuhn, 2021).
The proportions of the 2.5-s grazing segments with and without spatial movement were modeled by the interaction of the presence of a grazing step and the grazing management with a generalized linear mixed effects model (referred to as the grazing step model) with an underlying beta distribution including the random effects as described above. A total of n = 272 observations were used in the model. The model CG vs. RG evaluated the effect of the interaction of grazing management and grazing bout and the additive effect of spatial movement on the ODBA of the 2.5-s segments. The dataset used for the first model included 1,523,748 ODBA observations collected in 68 tracks from 43 individual animals in three runs. This equals to 1,058 h of grazing observation.
All data processing was conducted in R 4.2.2 (R Core Team, 2022).
3 Results
The differentiation of the grazing process with and without spatial movement, i.e., grazing steps or standing, from GNSS resulted in a threshold for the 2.5-s segments of 2.83 ± 0.37 m min−1 (mean ± SD). In CG, proportions of 0.666 (95%CI = 0.645; 0.687) and 0.334 (95%CI = 0.313; 0355) were allocated to grazing with and without grazing steps, respectively, calculated from the threshold of 2.83 m min−1. Compared with CG, there were fewer grazing steps in RG, where the proportion of grazing with grazing steps was 0.546 (95%CI = 0.524; 0.568) and that without grazing was 0.454 (95%CI = 0.432; 0.476; all p < 0.0001) (Table 2).
Our second approach, predicting the spatial movement in the grazing process, i.e., the grazing steps, only with the IMU data was not successful. The validation of the random forest with the independent test dataset had an accuracy of 0.668 (95%CI = 0.666; 0.669) and a Cohen’s kappa of 0.2796. When treating the class “without spatial movement” as a positive class, the sensitivity was 0.487 and the specificity was 0.784. Similarly, when modeling the ODBA in different grazing bouts, grazing management, and grazing steps, the grazing steps had only a negligible effect on the ODBA. The difference in the ODBA between grazing with and without spatial movement was small, with mean ODBAs of 3.20 m s−2 (95%CI = 3.01; 3.38) and 3.15 m s−2 (95%CI = 2.96; 3.33), respectively (p < 0.0001), indicating that grazing with or without spatial movement could not be differentiated by IMU.
The ODBA was affected by the interaction of the factors grazing management and grazing bout (p < 0.0001, model RMSE = 0.66 m s−2) (Table 2). The CSH measurements clearly showed that the swards were shorter in the CG grazing management and taller in RG. The ODBA was generally higher in CG (3.47 m s−2, 95%CI = 3.28; 3.65) than in RG (2.88 m s−2, 95%CI = 2.69; 3.07). However, this finding needs to be interpreted in the interaction with the grazing bout (Figure 2, Table 2). CG management showed ODBA values of 3.54 m s−2 (95%CI = 3.31; 3.76) in the MB, 3.53 m s−2 (95%CI = 3.3; 3.76) in the DB, 3.75 m s−2 (95%CI = 3.52; 3.98) in the EB, and 3.05 m s−2 (95%CI = 2.82; 3.27) in the NB. In RG management, the ODBA values were 3.02 m s−2 (95%CI = 2.8; 3.25) in the MB, 2.82 m s−2 (95%CI = 2.6; 3.05) in the DB, 3.05 m s−2 (95%CI = 2.82; 3.28) in the EB, and 2.62 m s−2 (95%CI = 2.4; 2.85) in the NB.
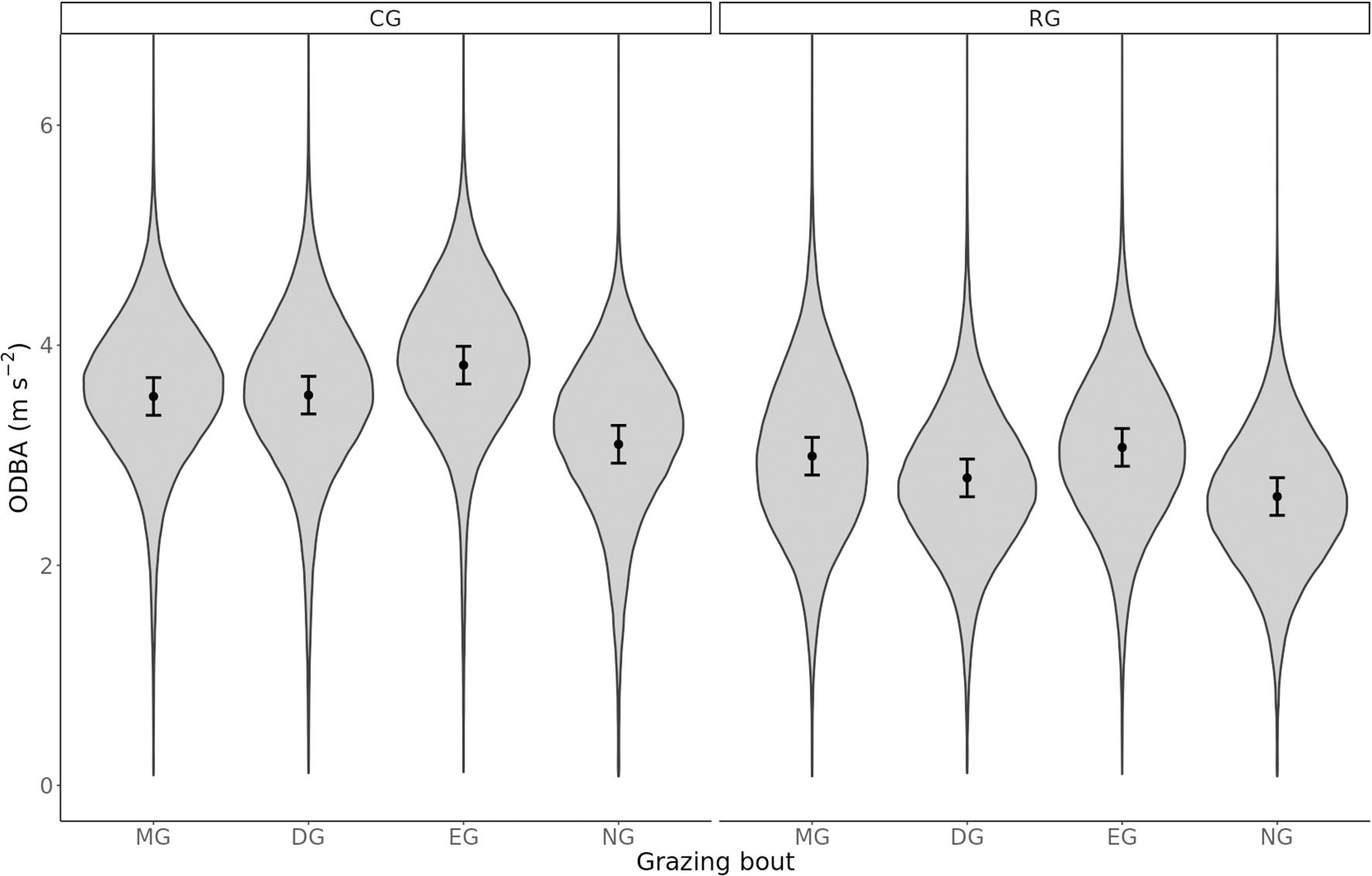
Figure 2. Violin plots showing the overall dynamic body acceleration (ODBA) for the two grazing management strategies—continuous grazing (CG) and rotational grazing (RG)—and for the grazing bouts: morning grazing (MG), day grazing (DG), evening grazing (EG), and night grazing (NG). The dots represent the estimate of the model with 95% confidence interval. The plot is truncated at ODBA 6.5 m s−2 for better readability.
4 Discussion
Precision livestock farming technology is well established in many commercial dairy farms. While most of these systems focus on dairy cows in confinement, monitoring grazing behavior is gaining increasing attention, particularly with the growing interest in virtual fencing and the collars used there (Goliński et al., 2023). The behavior classification of commercial ear tags and collars showed promising results with regard to behavior classification on pasture (Werner et al., 2019; Leso et al., 2021). Up to now, pasture-specific measurements, especially for describing the behavior grazing as the uptake of forage, have only been conducted with noseband or jaw sensors (Gibb et al., 1997; Shafiullah et al., 2019; Hart et al., 2022). Our approach aimed at taking simple IMUs such as on commonly used collars to monitor grazing-specific behavior in an on-farm environment with a simple metric, the ODBA. The ODBA can be calculated from three-axis accelerometers that record data in three dimensions.
The ODBA is a well-established measure of movement activity and can be related to the energy expenditure of an animal (Qasem et al., 2012). A higher ODBA indicates higher energy expenditure (Wilson et al., 2006). Miwa et al. (2015) found a correlation between ODBA and heart rate in grazing cattle and concluded that the ODBA is the best single metric from IMUs to predict physiological parameters. Our experiment was an approach to implement the findings of Miwa et al. (2015) in an on-farm grazing research. We assumed that the ODBA is affected not only by head and neck movements but also by spatial movement. In the case of behavior grazing (grass intake and short movement to the next feeding station), the spatial movement is caused by grazing steps as opposed to true standing.
The threshold of 2.83 m min−1 found in this study is close to the threshold of 2.47 m min−1 Rook et al. (2004) found when observing the grazing process including manually measuring the grazing steps of heifers. A typical residence time at a feeding station is approximately 10 s, which allows for the assumption that the temporal resolution of 2.5 s used in this study is sufficient for the differentiation of grazing with and without grazing steps (Rook et al., 2004). Hoekstra et al. (2019) found more steps per grazing hour in a CG system compared to a strip grazing system with higher swards (1,732 vs. 1,588 steps per hour) in a study using step sensors, which is similar to our findings. This difference can be explained by a more frequent relocation to new feeding stations in CG with less feed-on-offer per feeding station (Menegazzi et al., 2021). It needs to be considered that our approach of differentiating grazing steps by GNSS was not compared to “gold standard” methods such as step sensors or visual observations (Ungar et al., 2018). The circular error probable of our device, which is similar to other studies, suggests some uncertainty with regard to the threshold of movement during grazing (Zhao et al., 2024). Tracking with real-time or post-processing kinematics GNSS, as shown by Keshavarzi et al. (2021), allows spatial positioning with errors smaller than 10 cm, but increases the complexity and costs of the tracker setup. However, this could improve small-scale GNSS applications such as that in our study, whereas larger errors can be neglected when observing livestock on habitat (Riaboff et al., 2020) or even landscape level (Brennan et al., 2021). In comparison to the differentiation with GNSS, the approach using only machine learning and IMU data was not successful. We conclude that the ODBA from a neck-mounted accelerometer is primarily influenced by head and neck movements rather than spatial movement, as grazing steps cannot be detected without GNSS-based spatial differentiation.
Grazing management and the grazing bout had an effect on ODBA. This is partly an effect of the differences in the grazing process. The feed intake of cows on pasture includes different types of bites such as prehension (gathering and ingestion of herbage), manipulative (mastication), and rumination. The head and neck movement can be mainly related to prehension bites in the grazing process as the manipulation and mastication of ingested herbage mainly cause jaw, but not neck, movement (Laca et al., 1992; Shafiullah et al., 2019; Rombach et al., 2022). Thus, we assumed that mainly the prehension bites affect the ODBA in our collar-based approach. The bite rate (prehension bites) is influenced by factors such as the sward height, feed quality, and rumen fill. With a decreased CSH, the proportion of prehension bites increases and the proportion of manipulative bites decreases; in tall swards, more herbage per bite increases the need for manipulative bites (Gibb et al., 1997). This can explain the higher ODBA in CG compared with that in RG in our study. Furthermore, the time of the day and the allocated grazing bouts further influenced the grazing behavior of cattle. Typically, the major grazing bouts occur at dawn or after the morning milking (when milking twice a day, in the afternoon and at dusk: Rook and Huckle, 1995; Gibb et al., 1998; Taweel et al., 2004). Earlier studies found an increased grazing activity and a higher bite frequency in the dusk grazing bout and explained this as the cows trying to have a high feed intake before nighttime; during nighttime, the grazing activity is usually low (Gibb et al., 1998; Taweel et al., 2004). In addition, the higher feed quality around dusk contributes to high feed intake rates (Gibb et al., 1998; Taweel et al., 2004; Gregorini et al., 2008; Gregorini, 2012). In the NB, the grazing activity was low, as reported in multiple other studies (e.g., Taweel et al., 2004; Gregorini, 2012). The allocation of fresh pasture in the afternoon usually leads to a more intensive grazing than an allocation in the morning (Gregorini, 2012). This might explain the grazing pattern in RG: in four out of five RG pasture allocations, the cows received fresh pasture in the early evening after milking (Supplementary Figure 1). This resulted in a high ODBA in the EB. The MB, as expected, had a slightly lower ODBA, but the distinctly lower values of ODBA were visible in DB. An increase of the ODBA would be expected with decreasing CSH in RG from the start to the end of grazing in one paddock. However, additional effects need consideration: Barrett et al. (2001) found increasing stem/leaf proportions and decreasing CSH in RG from the start to the end of grazing a paddock as the cows favored and selected leafy material. While the overall bite rate (prehension and chewing) only slightly decreased during the grazing period of the RG paddocks, the intake rate declined strongly. This means that the increased need for chewing in swards with a high stem/leaf proportion leads to a smaller number of prehension bites, hence a lower ODBA. In CG, the difference in the ODBA between MB and DB was less pronounced. In continuous grazing, sward characteristics such as CSH and stem/leaf proportion are not affected by the allocation time or cow selection. However, also in CG, the sward was not of even height, but there was a pattern of somewhat taller, less frequently grazed patches and short, frequently grazed patches; this can have a non-observed effect on the grazing process (Gibb et al., 1997). The findings in the CG management in our study appear to be similar to those of Taweel et al. (2004), who found a linear increase in the prehension bites during the day from dawn until dusk in continuous grazing. We conclude that the grazing activity in RG management as described by the ODBA in our study is influenced by the combined effects of pasture allocation, declining CSH, increasing stem/leaf proportion over the grazing time, and the diurnal pattern; on the other hand, in CG, the sward characteristics are more stable and the major influence on ODBA is by the diurnal pattern of grazing and fluctuations in pasture quality.
5 Conclusion
Our findings extend the use of collar-based accelerometers in a grazing context. We were able to reproduce well-researched grazing patterns from the ODBA; however, there is still a need for a validation against “gold standard” methods. The negligible influence of spatial movement on the ODBA allows observing grazing patterns even without GNSS-supported data, while the GNSS data can add substantial value in answering other research questions. The widespread use of accelerometer sensors in dairy farming allows on-farm research on grazing management without additional expensive equipment and, moving further, an animal-centered pasture management approach. Future research could include the use of ODBA for a detailed differentiation of grazing in different settings (i.e., height, botanical composition, and livestock type), as well as to better gain insights into grazing practices in commercial farms.
Data availability statement
The raw data supporting the conclusions of this article will be made available by the authors, without undue reservation.
Ethics statement
The animal studies were approved by Animal Ethics Commissioner of the University of Vechta. The studies were conducted in accordance with the local legislation and institutional requirements. Written informed consent was obtained from the owners for the participation of their animals in this study.
Author contributions
KO: Conceptualization, Data curation, Formal analysis, Investigation, Methodology, Software, Validation, Visualization, Writing – original draft. MK: Conceptualization, Funding acquisition, Project administration, Resources, Supervision, Writing – review & editing. JI: Conceptualization, Resources, Supervision, Writing – review & editing.
Funding
The author(s) declare that financial support was received for the research and/or publication of this article. This work (Model- and Demonstration Project for animal welfare) is financially supported by the Federal Ministry of Food and Agriculture based on a decision of the Parliament of the Federal Republic of Germany, granted by the Federal Office for Agriculture and Food. The project grant number is 2819MDT100.
Acknowledgments
We would like to thank both the families and staff of the Apelt Farm and Bartels Farm for their willing participation and support for our study. We would further like to thank Christina Behrendt, Lena Weber and Thore Heß for their help with animal observations and the accompanying field work.
Conflict of interest
The authors declare that the research was conducted in the absence of any commercial or financial relationships that could be construed as a potential conflict of interest.
Generative AI statement
The author(s) declare that no Generative AI was used in the creation of this manuscript.
Publisher’s note
All claims expressed in this article are solely those of the authors and do not necessarily represent those of their affiliated organizations, or those of the publisher, the editors and the reviewers. Any product that may be evaluated in this article, or claim that may be made by its manufacturer, is not guaranteed or endorsed by the publisher.
Supplementary material
The Supplementary Material for this article can be found online at: https://www.frontiersin.org/articles/10.3389/fanim.2025.1517570/full#supplementary-material
Supplementary Figure 1 | Grazing bouts for all grazing days of the study time with marking lines for dusk and dawn. The breaks in the plot (white) show the time off paddock i.e. milking time. Grazing in rotational grazing management is abbreviated as RG, in continuous grazing as CG. The abbreviated months also outline the run. Grazing 7 describes the grazing of two RG paddocks.
References
Barrett P. D., Laidlaw A.S., Mayne C.S., Christie H. (2001). Pattern of herbage intake rate and bite dimensions of rotationally grazed dairy cows as sward height declines. Grass Forage Sci. 56, 362–373. doi: 10.1046/j.1365-2494.2001.00286.x
Barwick J., Lamb D.W., Dobos R., Welch M., Trotter M. (2018). Categorising sheep activity using a tri-axial accelerometer. Comput. Electron. Agric. 145, 289–297. doi: 10.1016/j.compag.2018.01.007
Brennan J., Johnson P., Olson K. (2021). Classifying season long livestock grazing behavior with the use of a low-cost GPS and accelerometer. Comput. Electron. Agric. 181, 105957. doi: 10.1016/j.compag.2020.105957
Brooks M. E., Kristensen K., van Benthem K. J., Magnusson A., Berg C. W., Nielsen A., et al. (2017). glmmTMB balances speed and flexibility among packages for zero-inflated generalized linear mixed modeling. R J. 9, 378–400. doi: 10.32614/RJ-2017-066
Chapa J. M., Maschat K., Iwersen M., Baumgartner J., Drillich M. (2020). Accelerometer systems as tools for health and welfare assessment in cattle and pigs – A review. Behav. Processes 181, 104262. doi: 10.1016/j.beproc.2020.104262
Delagarde R., Lamberton P. (2015). Daily grazing time of dairy cows is recorded accurately using the Lifecorder Plus device. Appl. Anim. Behav. Sci. 165, 25–32. doi: 10.1016/j.applanim.2015.01.014
Gibb M. J., Huckle C. A., Nuthall R., Rook A. J. (1997). Effect of sward surface height on intake and grazing behaviour by lactating Holstein Friesian cows. Grass Forage Sci. 52, 309–321. doi: 10.1111/j.1365-2494.1997.tb02361.x
Gibb M. J., Huckle C. A., Nuthall R. (1998). Effect of time of day on grazing behaviour by lactating dairy cows. Grass Forage Sci. 53, 41–46. doi: 10.1046/j.1365-2494.1998.00102.x
Goliński P., Sobolewska P., Stefańska B., Golińska B. (2023). Virtual fencing technology for cattle management in the pasture feeding system—A review. Agriculture 13, 91. doi: 10.3390/agriculture13010091
Gregorini P. (2012). Diurnal grazing pattern: its physiological basis and strategic management. Anim. Production Sci. 52, 416–430. doi: 10.1071/AN11250
Gregorini P., Gunter S. A., Beck P. A. (2008). Matching plant and animal processes to alter nutrient supply in strip-grazed cattle: Timing of herbage and fasting allocation1. J. Anim. Sci. 86, 1006–1020. doi: 10.2527/jas.2007-0432
Guo L., Welch M., Dobos R., Kwan P., Wang W. (2018). Comparison of grazing behaviour of sheep on pasture with different sward surface heights using an inertial measurement unit sensor. Comput. Electron. Agric. 150, 394–401. doi: 10.1016/j.compag.2018.05.004
Halachmi I., Guarino M., Bewley J., Pastell M. (2019). Smart animal agriculture: application of real-time sensors to improve animal well-being and production. Annu. Rev. Anim. Biosci. 7, 403–425. doi: 10.1146/annurev-animal-020518-114851
Hamidi D., Hütt C., Komainda M., Grinnell N.A., Horn J., Riesch F., et al. (2023). Grid grazing: A case study on the potential of combining virtual fencing and remote sensing for innovative grazing management on a grid base. Livestock Sci. 278, 105373. doi: 10.1016/j.livsci.2023.105373
Hart L., Dickhoefer U., Paulenz E., Umstaetter C. (2022). Evaluation of a binary classification approach to detect herbage scarcity based on behavioral responses of grazing dairy cows. Sensors 22, 968. doi: 10.3390/s22030968
Hartig F. (2022). DHARMa: Residual diagnostics for hierarchical (multi-level / mixed) regression models. manual. Available online at: https://CRAN.R-project.org/package=DHARMa (Accessed February 3, 2023).
Hoekstra N., Holshof G., Schils R., Philipsen B., van Reenen K., van Houwelingen K., et al. (2019). The effect of kurzrasen and strip-grazing on grassland performance and soil quality of a peat meadow. Sustainability 11, 6283. doi: 10.3390/su11226283
Kalafus R. M., Chin G. Y. (1986). “Measures of accuracy in the navstar/GPS: 2drms vs. CEP,” in Proceedings of the 1986 National Technical Meeting of The Institute of Navigation, National Technical Meeting of The Institute of Navigation; Long Beach. 49–54.
Keshavarzi H., Lee C., Johnson M., Abbott D., Ni W., Campbell D. L. M. (2021). Validation of real-time kinematic (RTK) devices on sheep to detect grazing movement leaders and social networks in merino ewes. Sensors 21, 924. doi: 10.3390/s21030924
Keshavarzi H., Lee C., Dyall T., Johnson M., Abbott D., Belson S., et al. (2022). Using real-time kinematic (RTK) devices to show a limited effect of boldness and fearfulness on social proximity in sheep grazing at pasture. Front. Anim. Sci. 3. doi: 10.3389/fanim.2022.872132
Kuhn M. (2021). caret: classification and regression training. Available online at: https://CRAN.R-project.org/package=caret (Accessed February 9, 2023).
Laca E. A., Ungar E. D., Seligman N., Demment M. W. (1992). Effects of sward height and bulk density on bite dimensions of cattle grazing homogeneous swards. Grass Forage Sci. 47, 91–102. doi: 10.1111/j.1365-2494.1992.tb02251.x
Lenth R. V. (2021). emmeans: Estimated Marginal Means, aka Least-Squares Means. Available online at: https://CRAN.R-project.org/package=emmeans (Accessed February 11, 2022).
Leso L., Becciolini V., Rossi G., Camiciottoli S., Barbari M. (2021). Validation of a commercial collar-based sensor for monitoring eating and ruminating behaviour of dairy cows. Animals 11, 2852. doi: 10.3390/ani11102852
McGranahan D. A., Geaumont B., Spiess J. W. (2018). Assessment of a livestock GPS collar based on an open-source datalogger informs best practices for logging intensity. Ecol. Evol. 8, 5649–5660. doi: 10.1002/ece3.4094
Menegazzi G., Giles P. Y., Oborsky M., Fast O., Mattiauda D. A., Genro T. C. M., et al. (2021). Effect of post-grazing sward height on ingestive behavior, dry matter intake, and milk production of holstein dairy cows. Front. Anim. Sci. 2. doi: 10.3389/fanim.2021.742685
Miwa M., Oishi K., Nakagawa Y., Maeno H., Anzai H., Kumagai H., et al. (2015). Application of overall dynamic body acceleration as a proxy for estimating the energy expenditure of grazing farm animals: relationship with heart rate. PloS One 10, e0128042. doi: 10.1371/journal.pone.0128042
Obermeyer K., Kayser M. (2023). On-farm assessment of grazing behaviour of dairy cows in two pasture management systems by low-cost and reliable cowtrackers. Smart Agric. Technol. 6, 100349. doi: 10.1016/j.atech.2023.100349
Pérez-Ramírez E., Delagarde R., Delaby L. (2008). Herbage intake and behavioural adaptation of grazing dairy cows by restricting time at pasture under two feeding regimes. Animal 2, 1384–1392. doi: 10.1017/S1751731108002486
Qasem L., Cardew A., Wilson A., Griffiths I., Halsey L.G., Shepard E.L.C., et al. (2012). Tri-axial dynamic acceleration as a proxy for animal energy expenditure; should we be summing values or calculating the vector? PloS One 7, e31187. doi: 10.1371/journal.pone.0031187
R Core Team (2022). R: A language and environment for statistical computing (Vienna, Austria: R Foundation for Statistical Computing). Available at: https://www.R-project.org/.
Riaboff L., Couvreur S., Madouasse A., Roig-Pons M., Aubin S., Massabie P., et al. (2020). Use of predicted behavior from accelerometer data combined with GPS data to explore the relationship between dairy cow behavior and pasture characteristics. Sensors 20, 4741. doi: 10.3390/s20174741
Riaboff L., Shalloo L., Smeaton A.F., Couvreur S., Madouasse A., Keane M.T. (2022). Predicting livestock behaviour using accelerometers: A systematic review of processing techniques for ruminant behaviour prediction from raw accelerometer data. Comput. Electron. Agric. 192, 106610. doi: 10.1016/j.compag.2021.106610
Rombach M., Südekum K.-H., Schori F. (2022). Influence of pre-grazing herbage mass on bite mass, eating behaviour, and dairy cow performance on pasture. J. Anim. Physiol. Anim. Nutr. 107 (5), 1–12. doi: 10.1111/jpn.13795
Rook A. J., Harvey A., Parsons A.J., Orr R.J., Rutter S.M. (2004). Bite dimensions and grazing movements by sheep and cattle grazing homogeneous perennial ryegrass swards. Appl. Anim. Behav. Sci. 88, 227–242. doi: 10.1016/j.applanim.2004.03.006
Rook A. J., Huckle C. A. (1995). Synchronization of ingestive behaviour by grazing dairy cows. Anim. Sci. 60, 25–30. doi: 10.1017/S1357729800008092
Shafiullah A. Z., Werner J., Kennedy E., Leso L., O’Brien B., Umstätter C. (2019). Machine learning based prediction of insufficient herbage allowance with automated feeding behaviour and activity data. Sensors 19, 4479. doi: 10.3390/s19204479
Taweel H. Z., Tas B.M., Dijkstra J., Tamminga S. (2004). Intake regulation and grazing behavior of dairy cows under continuous stocking. J. Dairy Sci. 87, 3417–3427. doi: 10.3168/jds.S0022-0302(04)73477-3
Ungar E. D., Nevo Y., Baram H., Arieli A. (2018). Evaluation of the IceTag leg sensor and its derivative models to predict behaviour, using beef cattle on rangeland. J. Neurosci. Methods 300, 127–137. doi: 10.1016/j.jneumeth.2017.06.001
Werner J., Leso L., Umstatter C., Niederhauser J., Kennedy E., Geoghegan A., et al. (2018). Evaluation of the RumiWatchSystem for measuring grazing behaviour of cows. J. Neurosci. Methods 300, 138–146. doi: 10.1016/j.jneumeth.2017.08.022
Werner J., Umstatter C., Leso L., Kennedy E., Geoghegan A., Shalloo L., et al. (2019). Evaluation and application potential of an accelerometer-based collar device for measuring grazing behavior of dairy cows. Animal 13, 2070–2079. doi: 10.1017/S1751731118003658
Williams T. M., Costa D.F.A., Wilson C.S., Chang A., Manning J., Swain D., et al. (2022). Sensor-based detection of parturition in beef cattle grazing in an extensive landscape: a case study using a commercial GNSS collar. Anim. Production Sci. doi: 10.1071/AN21528
Wilson R. P., White C.R., Quintana F., Halsey L.G., Liebsch N., Martin G.R., et al. (2006). Moving towards acceleration for estimates of activity-specific metabolic rate in free-living animals: the case of the cormorant. J. Anim. Ecol. 75, 1081–1090. doi: 10.1111/j.1365-2656.2006.01127.x
Wright M. N., Ziegler A. (2017). A fast implementation of random forests for high dimensional data in C++ and R. J. Stat. Software 77, 1–17. doi: 10.18637/jss.v077.i01
Keywords: dairy cow, pasture, sward height, behavior, sensors, rotational grazing system, continuous grazing system, global navigation satellite system (GNSS)
Citation: Obermeyer K, Kayser M and Isselstein J (2025) Observing grazing patterns with collar-mounted accelerometers and spatial data. Front. Anim. Sci. 6:1517570. doi: 10.3389/fanim.2025.1517570
Received: 26 October 2024; Accepted: 07 April 2025;
Published: 08 May 2025.
Edited by:
Bjørg Heringstad, Norwegian University of Life Sciences, NorwayReviewed by:
Riccardo Primi, University of Tuscia, ItalyNicolai Hermann Jørgensen, Norwegian University of Life Sciences, Norway
Copyright © 2025 Obermeyer, Kayser and Isselstein. This is an open-access article distributed under the terms of the Creative Commons Attribution License (CC BY). The use, distribution or reproduction in other forums is permitted, provided the original author(s) and the copyright owner(s) are credited and that the original publication in this journal is cited, in accordance with accepted academic practice. No use, distribution or reproduction is permitted which does not comply with these terms.
*Correspondence: Kilian Obermeyer, a2lsaWFuLm9iZXJtZXllckBsYXpidy5id2wuZGU=