Rewiring of Microbiota Networks in Erosive Inflammation of the Stomach and Small Bowel
- 1Department of Gastroenterology, Shanghai Jiao Tong University Affiliated Sixth People’s Hospital, Shanghai, China
- 2Aginome-XMU Joint Laboratory, Xiamen University, Xiamen, China
- 3Clinical Research Center, Shanghai Jiao Tong University Affiliated Sixth People’s Hospital, Shanghai, China
The development of non-invasive, inexpensive, and effective early diagnosis tests for gastric and small-bowel lesions is an urgent requirement. The introduction of magnetically guided capsule endoscopy (MGCE) has aided examination of the small bowel for diagnoses. However, the distribution of the fecal microbiome in abnormal erosions of the stomach and small bowel remains unclear. Herein, alternations in the fecal microbiome in three groups [normal, small-bowel inflammation, and chronic gastritis (CG)] were analyzed by metagenomics and our well-developed method [individual-specific edge-network analysis (iENA)]. In addition to the dominant microbiota identified by the conventional differential analysis, iENA could recognize novel network biomarkers of microbiome communities, such as the genus Bacteroide in CG and small-bowel inflammation. Combined with differential network analysis, the network-hub microbiota within rewired microbiota networks revealed high-ranked iENA microbiota markers, which were disease specific and had particular pathogenic functions. Our findings illuminate the components of the fecal microbiome and the importance of specific bacteria in CG and small-bowel erosions, and could be employed to develop preventive and non-invasive therapeutic strategies.
Introduction
Choung and colleagues reported that in the United States, 338 out of 31,255 adult residents were diagnosed with diseases of the digestive system (Choung et al., 2017). In particular, chronic gastritis (CG) and small-bowel erosion account for >60% of cases diagnosed by magnetically guided capsule endoscopy (MGCE) in China (Ding et al., 2019) and South Korea (Lim et al., 2015). Introduction of MGCE has expanded clinical diagnosis of gastrointestinal diseases, including CG and small-bowel erosion (Aktas and Mensink, 2012). However, the unbiopsied state, high cost, and time consumption of MGCE limit its application in diagnoses (Costamagna et al., 2002; Niv and Niv, 2005). Development of non-invasive, effective detection in small-bowel lesions is needed urgently.
Metagenomics using next-generation sequencing (mNGS) is becoming a promising approach for identifying the microbiome community in human diseases (Wilson et al., 2019). Some studies have shown an increased ratio of Basidiomycota:Ascomycota, increased proportion of Candida albicans, and a decreased abundance of Saccharomyces cerevisiae in inflammatory bowel disease (IBD) (Sokol et al., 2017). Significant differences in levels of bacterial genera have been used to detect atrophic gastritis/intestinal metaplasia, and gastrointestinal tumors (Coker et al., 2017; Zhang et al., 2019). mNGS can also be used to distinguish the functions of the gut microbiome in IBD and irritable bowel syndrome (IBS) (Vich Vila et al., 2018). Small-bowel microbiota not only regulate assimilation of the adaptive responses to lipids in germ-free mice (Martinez-Guryn et al., 2018) but also act by assessing the small-bowel damage induced by non-steroidal anti-inflammatory drugs (Otani et al., 2017). However, use of the fecal microbiome for identification of gastric and small-bowel abnormalities has not been done.
An accurate clinical diagnosis can enable monitoring, quantification, and progression of a disease (Zeng et al., 2014; Yu et al., 2015), and can be realized using sample-specific biomarkers (Zeng et al., 2016). Previously, we proposed an individual-specific edge-network analysis (iENA) to detect the early warning signals or pre-disease state before disease onset (Yu et al., 2017). Also, we carried out proof-of-concept research on the rewiring community of intestinal ecosystems by an adjusted iENA method on the basis of 16S rRNA data (Wang et al., 2018; Yu et al., 2019).
Here, we used the method of computational systems biology that we had developed to analyze the dominant microbiota and network on the basis of fecal metagenomics data. We identified specific bacteria that had key roles in the clinical classification of erosive lesions of the small bowel and CG that might offer prevention and non-invasive treatment strategies.
Materials and Methods
Ethical Approval of the Study Protocol
The study protocol was approved by the Ethics Committee of Shanghai Sixth People’s Hospital, which is affiliated with Shanghai Jiao Tong University (Shanghai, China). Written informed consent was obtained from all individuals. Personal data were anonymized and omitted.
Study Enrollment
The study ran at Shanghai Sixth People’s Hospital from May 1, 2017 to September 1, 2018. The individuals who agreed to complete examinations of MGCE (Ankon Medical Technologies, Shanghai, China) and mNGS examination of their stools (n = 15) were recruited. The procedures of enrollment, fecal mNGS, and MGCE classification were completed independently by different investigators who were blinded to the results of each other’s examinations. Fisher’s exact test was used for evaluation of statistical difference in comparisons between three groups. p < 0.05 was considered statistically significant.
MGCE and Stool Collection
At least three stool samples were collected from each eligible individual and stored at −80°C. All patients underwent intestinal preparation with an electrolyte solution of polyethylene glycol, fasted all night, and completed MGCE in the morning.
Healthy individuals (H group) were characterized by an absence of lesions in the stomach and small bowel through MGCE. Gastric inflammation (G group) was identified based on the Updated Sydney System (Dixon et al., 1996). Inflammation located in one out of three parts of the small bowel (duodenum, jejunum, and ileum) but not in the stomach was defined as “small intestinal inflammation” (I group).
We recorded (i) mucosal lesions, such as erosions; (ii) capillary lesions (angiodysplasias, petechiae); (iii) mucosal changes (erythema, edema, prominent mucosal folds); (iv) changes in villi (flat mucosa, coarsened villi); (v) lymphangiectasias/lymphocellular infiltrates.
DNA Sampling
Samples of total DNA from fecal samples were extracted using a Fast DNA SPIN extraction kit (MP Biomedicals, Santa Ana, CA, United States) and stored at −20°C. The quality and quantity of isolated DNA were assessed by agarose gel electrophoresis and a NanoDropTM ND-1000 spectrophotometer (Thermo Fisher Scientific, Waltham, MA, United States).
mNGS
DNA extracted from fecal samples was used for mNGS. Metagenomic libraries were constructed with a TruSeqTM DNA Sample Preparation kit (Illumina, San Diego, CA, United States) and sequenced at Shanghai Personal Biotechnology (Shanghai, China) on an Illumina HiSeq system with a 150-bp paired-end protocol. Reads from each sample were retained and then merged by megahit (Li et al., 2015) and, if not, matched to human genome sequences (hg19) using Bowtie 2 (Langmead and Salzberg, 2012). The remaining results of merging were contigs of length ≥500 bp. Gene prediction was carried out based on MetaGeneMark (Noguchi et al., 2006) and then combined into a gene set. The protein sequences of genes were clustered to remove redundancy using cd-hit (Li and Godzik, 2006), with an identity cutoff of 90%, which resulted in unique gene sets. Reads from each sample were mapped to obtain their unique gene set. “Gene abundance” in each sample was the number of reads mapped to each gene sequence divided by the gene length. The percentage of gene abundance in the whole gene catalog was called the “relative abundance.” Diamond (Buchfink et al., 2015) was introduced to gene alignment and gene annotation in the National Center for Biotechnology Information-NR database with an e-value cutoff of 10-fold of the minimum value. Based on the alignment results, the algorithm of nearest common ancestors was taken into account for species annotation on genes. We annotated genes to species with in-house Perl scripts. The abundance of species in each sample was defined as the sum of gene abundance annotated to the same species. Functional classification was carried out by mapping to the Kyoto Encyclopedia of Genes and Genomes (KEGG) protein database and Clusters of Orthologous Groups of Proteins (COG) database (Tatusov et al., 2000) using KEGG Orthology-Based Annotation System (KOBAS) (Xie et al., 2011) and Diamond, respectively. Kruskal–Wallis analysis (Segata et al., 2011) was applied for classification and analyses of differentially expressed genes among dissimilar groups (p < 0.05, fdr < 0.05). In addition, principal component analysis (PCA) was used to analyze and visualize the sample distribution and discrimination among different disease groups.
Differential Function of Expressed Genes
We compared any two sets of samples from H, G, and I groups by the relative abundance of genes. In each comparison, we removed the genes detected in less than five samples and continued analysis of abundance for the remaining genes using the Wilcoxon rank sum test, and the genes with test significance p < 0.05 were selected (Supplementary Table S1).
For additional analysis of differential function, the genes annotated in KEGG and COG databases were used for functional categories. For example, we used the differential genes of the G and H groups for analysis of hypergeometric distribution with unique genes as the background. Also, function annotations with p < 0.05 and fdr < 0.05 were enriched functions. Conversely, we counted the number of genes annotated in KEGG and COG functional categories and compared such functional categories between any two groups of samples by the Wilcoxon rank sum test. A function with p < 0.05 and fdr < 0.05 was determined to be a significantly differential function (Supplementary Table S2).
Analysis of Dominant Microbiota and Networks in Bowel Inflammation and CG
Previously, we proposed an advanced computational framework (i.e., iENA) to provide a powerful network-analysis tool to quantify disease progression in an individual patient. Recently, we implemented an adjusted iENA using samples from healthy individuals as a network reference due to a limited number of individual samples and applied it in a proof-of-concept study on microbiota dynamics (Yu et al., 2019). Here, we used our approach to analyze the dominant microbiota and network to quantify different disease states using metagenomics data in three steps.
Constructing a Microbiota Edge-Network by iENA
After selecting reference samples, we constructed a co-expression network for one sample with our single-sample measurement of the Pearson correlation coefficient (sPCC) (Yu et al., 2017). Because of the absence of a background network for microbial communities, the top-ranked edges (i.e., one pair of species/genus) with strong relationships were selected as the background “nodes” for constructing the subsequent edge-network, which could consist of a conventional node-network or microbiota community (Wang et al., 2016; Sung et al., 2017). Furthermore, we continued quantification of the fourth-order correlation coefficient for each edge-pair (i.e., two species/genus pairs) by sPCC (Yu et al., 2017) for each single sample. Similarly, we only computed the correlations between the pre-selected high-ranked relations (edges) so that we could reduce unnecessary computations drastically. Finally, we obtained the microbiota-pair community/network corresponding to each sample from different disease states.
Recognizing and Quantifying Individual-Specific Microbiota Biomarkers
We selected top-ranked edge-pairs as edge-biomarkers, which have strong high-order compositional correlations and can be viewed as feature candidates represented as a set called “Markers.” In theory, each individual-specific biomarker is related to the clinical phenotype to some extent because the closely contacted candidates are identified in a certain disease state. As a warning signal (Chen et al., 2012; Zeng et al., 2013), we extended the dynamic network biomarker model in a manner of a single sample with its quantification criterion [i.e., composite index (sCI)] to quantify the disease state of each sample:
where is the average absolute value of PCC of a species/genus in the Marker group in one sample; is the average absolute value of PCC of a species/genus between the Marker group and the other in one sample; is the average standard deviation in the Marker group. “Marker” was the set of dominant species/genus identified by iENA. Then, the sCI of individual markers could be used to indicate the possible disease signals when its value was sufficiently large.
Comparing Disease-Specific Markers and Their Discrimination for Disease
We could obtain the different individual-specific biomarkers to indicate disease-specific signals. Microbiota features that always present in the same disease group are more robust and representative in terms of disease specificity (Supplementary Table S3). Thus, we used the union set of microbiota features from patients with CG and small-bowel inflammation to characterize the varying microbiota community corresponding to disease states. In expectation of disease discrimination, we re-obtained the sCI value for each subject in different disease groups using such union markers, which indicated different etiologic mechanisms.
Results and Discussion
Study Populations
From May 1, 2017 to September 1, 2018, 38 people with symptoms such as chronic abdominal pain, abdominal distention, and diarrhea participated in this study. They were screened across the Department of Gastroenterology of Shanghai Sixth People’s Hospital (Figure 1A).
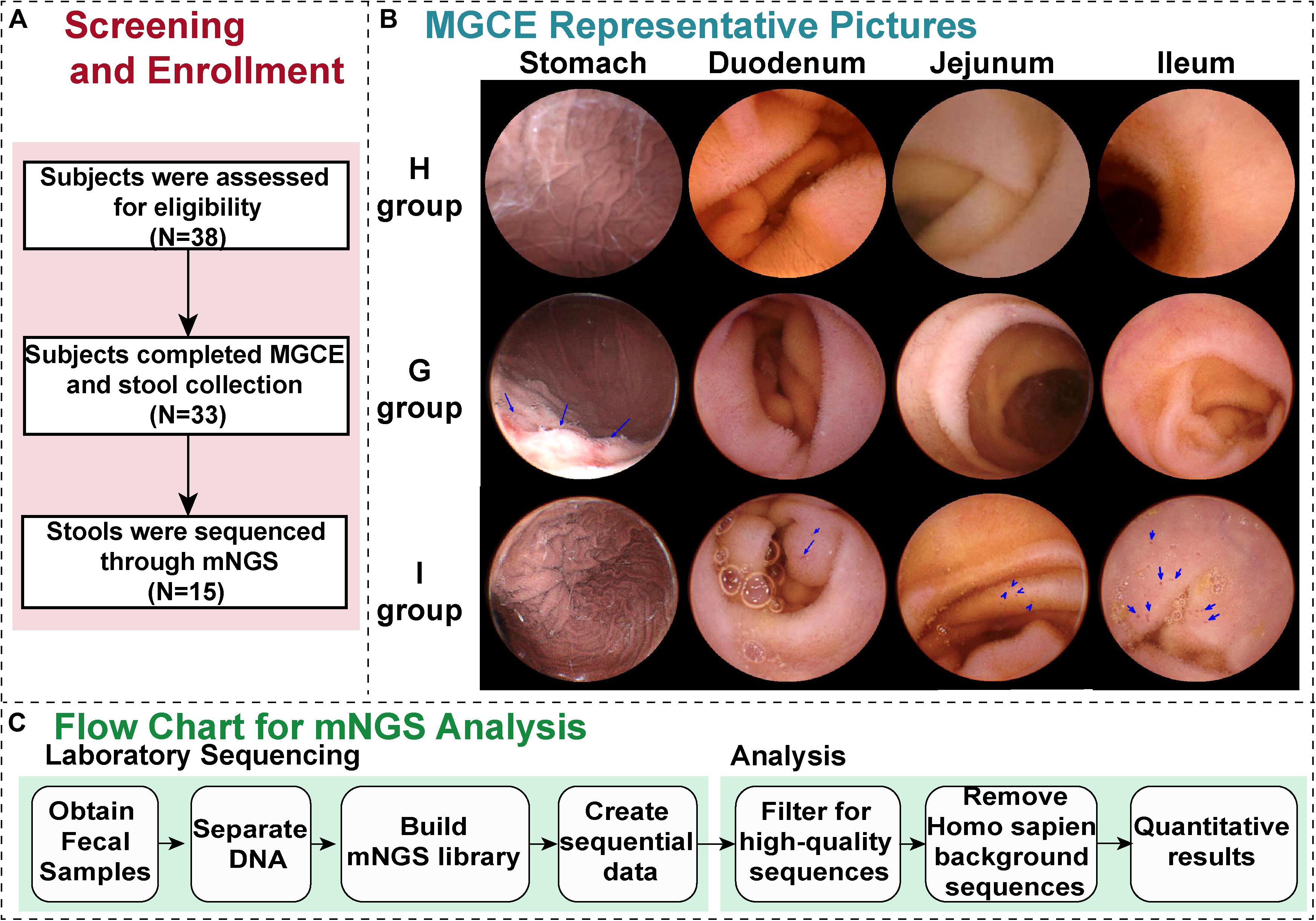
Figure 1. Generalization of the study. (A) The screening and enrollment of patients through the study. (B) Representative magnetically guided capsule endoscopy (MGCE) images (stomach, duodenum, jejunum, and ileum) of the H group (health group), G group (chronic gastritis), and I group (small bowel inflammation). (C) The protocol for the metagenomic next-generation sequencing (mNGS) assay. After samples of stool are received in the hospital, DNA is isolated, followed by construction of a metagenomic NGS library and sequencing.
Among them, 33 patients completed MGCE and stool collection, and the feces of 15 patients were processed through mNGS (Figure 1). The cohort primarily comprised, as defined by MGCE, individuals with gastric inflammation (G group), small bowel inflammation (I group), and a healthy population (H group), with five people in each group (Figure 1B and Table 1). The median age of the 15 patients was 53.0 years (Table 1). The proportion of male in these individuals was 40.0%, which was significantly high in patients with I group (26.7%) compared to H group (Supplementary Table S4). Recent studies observed no evidence for associations between gastrointestinal inflammation and gender (Khalili et al., 2020). The median value of alanine aminotransferase and total bile acid was 18 U/L and 3.35 μmol/L, respectively. Fourteen patients did have negative fecal occult blood in their feces (Table 1).
Analyses of Microbiota Composition to Distinguish Between Small-Bowel Inflammation and CG
Using mNGS, we demonstrated the quality of sequencing and obtained the microbe abundance through a standard pipeline. There were 52,820 differential genes between the G group and H group; 22,537 genes between the I group and H group; 17,670 genes between the G group and I group. We obtained two important results (Figure 2).
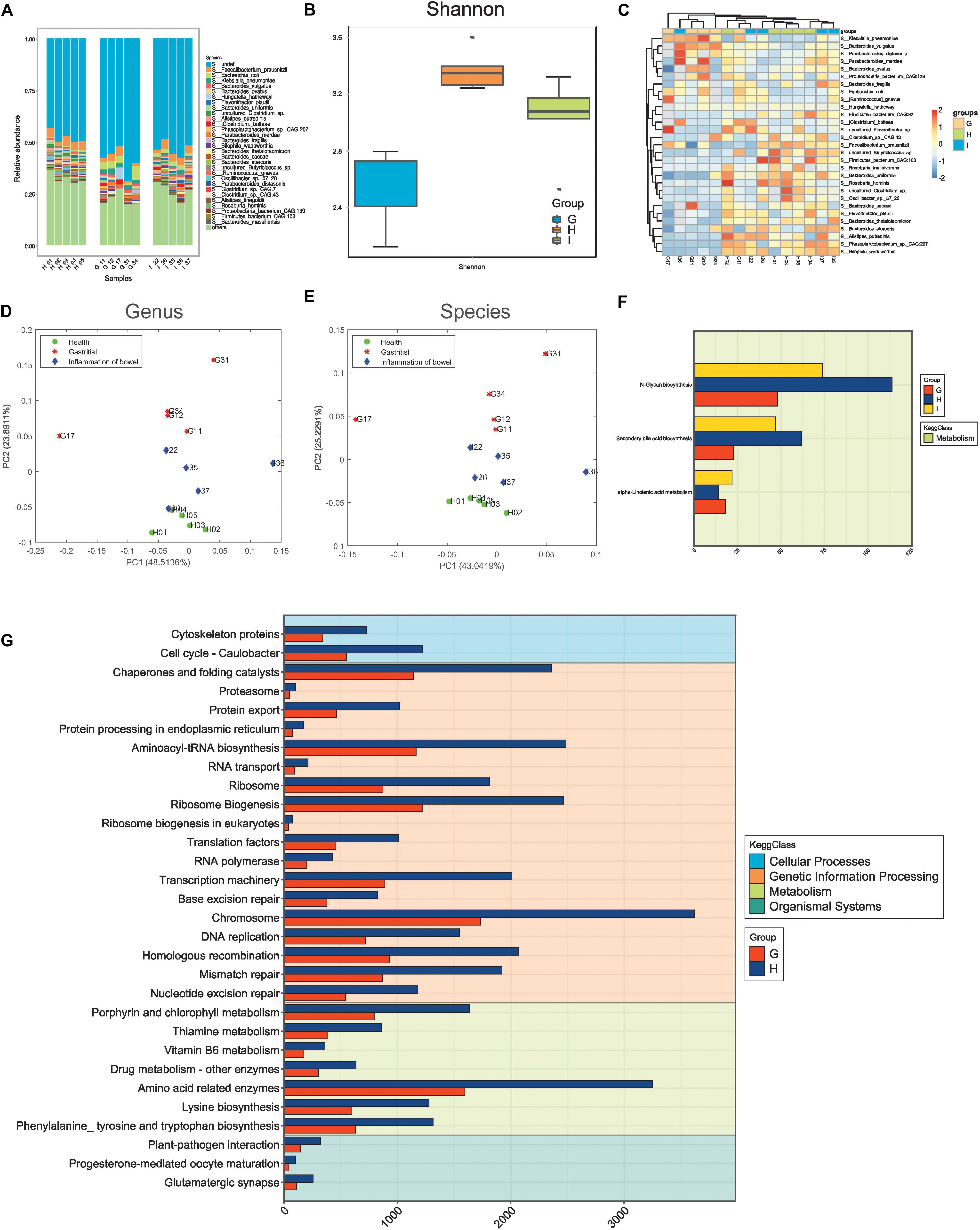
Figure 2. Microbiota composition distinguishing small-bowel inflammation and chronic gastritis. (A) The distribution of microbes in different samples. (B) The alpha-diversity of samples in different groups. (C) The abundance heatmap of differential microbes. (D) Principal component analysis (PCA) of samples with genus features. (E) PCA of samples with species features. (F) The differential functions between groups G (chronic gastritis), I (small bowel inflammation), and H (health group). (G) The differential functions between groups G and H.
First, the distribution of different species in samples indicated the varying microbiota compositions. Also, the samples in the same group/state tended to have similar microbiota compositions (Figure 2A). The different species diversities displayed consistent measurements, and the microbiota compositions in healthy states were more diverse than those in the disease state (Figure 2B and Supplementary Figure S1), thereby suggesting remarkable disruption of the microbiota community when diseases occurred. The microbiota compositions in small-bowel inflammation were more diverse than those in CG (Figure 2B), which suggested that CG might be more serious than small-bowel inflammation at the microbiota level. A few microbes with different abundance between different groups were detected, although they seemed to not have effective discrimination together (Figure 2C).
Second, PCA supported the observation mentioned above that the microbiota community in healthy samples was consistent and similar, but had varying degrees of alterations in small-bowel inflammation or CG samples at the level of genus (Figure 2D) or species (Figure 2E). Functional analysis using metagenomics revealed that, compared with samples from healthy people, samples from people with small-bowel inflammation had dysfunction of the microbiome on metabolism (Figure 2F) (e.g., N-Glycan biosynthesis, biosynthesis of secondary bile acids, and metabolism of -Linolenic acid). Recent study has revealed that patients with IBD have a higher abundance of large-size glycans, as well as lower levels of galactosylation and fucosylation (Clerc et al., 2018). Those data are contrary to studies showing that levels of core fucosylation are increased in T cells in patients with IBD with an inflamed mucosa and in mice with colitis (Fujii et al., 2016). Moreover, supplements of related metabolites (e.g., diets of sage oil or hydrolyzed protein) can be efficacious treatment of chronic enteropathy. Rats with IBD or colitis have much lower mRNA levels of pro-inflammatory factors in the colon, resulting in a lower inflammatory response, significantly less colonic damage, and enhanced histological repair after administration of sage-oil diets (Reifen et al., 2015). Secondary bile acids consist of lithocholic acid and deoxycholic acid. A diet of hydrolyzed protein increases can lead to growth inhibition of Escherichia coli and Clostridium perfringens in rats suffering from chronic enteropathy. Moreover, the bile-acid producers C. hiranonis and C. scindens are related to diet-induced remission in rats with dextran sulfate sodium-induced colitis and children with IBD (Wang et al., 2019). CG samples showed greater changes in diverse KEGG functions (e.g., skeletal proteins in cellular processes, amino acid-related enzymes in metabolism, and glutamatergic synapses in organismal systems) (Figure 2G). The chromosome 1-related dominant trait is linked to resistance in mice to the autoimmune gastritis (Fujii et al., 2014). Besides, high levels of aneuploidy in chromosomes 4, 8, 20, and 17 (p53) have been detected in gastritis, dysplasia, intestinal metaplasia, and cancer samples (Williams et al., 2005).
Analyses of Microbiota Communities to Distinguish Small-Bowel Inflammation and CG Using iENA
The data shown above indicated that the microbe abundance reflected the distribution of groups of samples (even though a simple combination of microbes could not be used to distinguish between disease states). Thus, the variance in microbe abundance was expected to supply more discriminative information, which could be detected by our proposed iENA approaches. Indeed, using one CI score with iENA-identified marker microbes, each sample could be assigned to a suitable group correctly. In accordance with the analysis stated above, CG samples had the highest scores, indicating the greatest variation/alteration in the microbiota community, whereas samples from healthy people had the lowest scores (which represented the stable microbiota community in individuals) (Figure 3A). More importantly, as reported in our previous study (Yu et al., 2019), the abundance of microbes could not be used to group samples with different phenotypes directly (Figure 3B). However, the co-expressed abundances of two microbes (e.g., edge makers detected using iENA) had the power to distinguish samples within dissimilar groups (Figures 3C,D). Such variation of the microbiota community can be used to quantify rewiring of microbiota networks. Hence, we reconstructed the correlation network of microbes corresponding to healthy samples, CG samples, and samples of small-bowel inflammation, respectively. In particular, the network-hub microbes in the different networks were the key microbiota involved in pathogenic processes (Supplementary Table S5). For example, CG-specific network-hub microbes tended to interact with many other microbes in the CG state but not in healthy or small-bowel-inflammation states (Figures 3E–G). Similarly, the small bowel inflammation-specific network-hub microbes tended to interact with many other microbes in the small-bowel-inflammation state, but not in the healthy or CG states (Figures 3E–G).
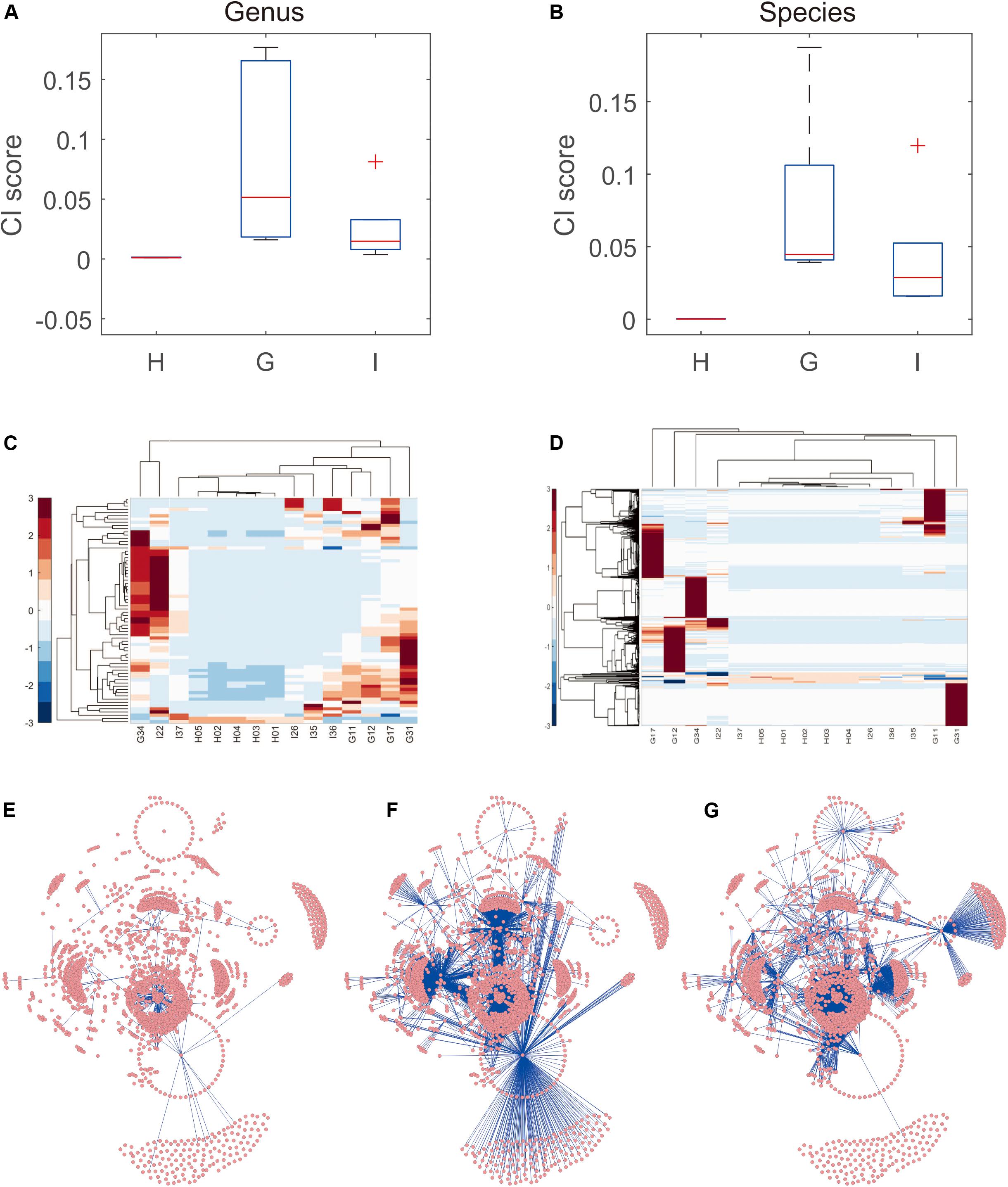
Figure 3. Microbiota community distinguishing small-bowel inflammation and chronic gastritis. (A) The distribution of CI scores distinguishes different groups on genus level. (B) The distribution of CI scores distinguishes different groups on species level. (C) The abundance heatmap of microbes in iENA markers. (D) The sPCC heatmap of microbe-pairs involved in iENA markers. (E) The microbe association network characterizing healthy state. (F) The microbe association network characterizing chronic gastritis state. (G) The microbe association network characterizing small bowel-inflammation state.
Key Microbiota and Networks Revealed the Specific Pathogenesis Underlying Small-Bowel Inflammation and CG
Three important trends were observed in this part of our study.
First, for the key microbiota with differential abundance identified by conventional analysis, some well-known pathogenic microbes were discovered.
Microbes from the genera Roseburia and Enterobacter were related to Helicobacter pylori (HP) in gastritis and Clostridioides difficile infection (CDI) in IBD. The absence of Roseburia has been observed in HP-positive samples (Zhao et al., 2019), whereas the genus Enterobacter is dominant in HP-free patients (Hsieh et al., 2018).
Microbes from the genera Blautia, Roseburia, and Flavonifractor assist in the clinical classification and prognosis assessment of intestinal diseases, including IBS, IBD, and colorectal cancer (CRC) (Labus et al., 2017; Machiels et al., 2017; Ai et al., 2019; Gupta et al., 2019). The genera Blautia and Flavonifractor contribute to discrimination of IBS or CRC from controls (Labus et al., 2017; Ai et al., 2019). The decreased abundance of Roseburia and Blautia in feces specimens of patients with ulcerative colitis (UC) indicates a higher risk of pouchitis after ileal–anal pull-through surgery (Machiels et al., 2017). In particular, patients with IBD or CDI have lower levels of Blautia than those without CDI (Sokol et al., 2018). Also, the genus Blautia is enriched after 26 weeks of quadruple treatment with bismuth in patients with asymptomatic HP-related gastritis (He et al., 2019). Also, the abundance of Roseburia increases significantly after 1-week administration of vitamin D in Crohn’s disease (CD) cases (Schäffler et al., 2018).
In addition, E. cloacae is associated significantly with CD patients without antibodies to Saccharomyces cerevisiae (Kansal et al., 2019). Also, many microbes are associated with the immune response and therapeutic results in gut inflammation. For example, oral administration of Citrobacter koseri JCM1658 aggravates systemic allergic reactions and reduced numbers of intestinal T-helper-17 cells (Matsui et al., 2019).
Second, for the key microbiota with differential abundance identified by iENA, some were indeed candidate pathogenic microbes, though they did not have significantly different abundances.
Microbes from the family Erysipelotrichaceae and genus Klebsiella are strongly related to inflammation in the stomach and intestine. The family Erysipelotrichaceae is overgrown in mice with basal colitis (Chen et al., 2017) or HP infection (Pan et al., 2016). Conversely, the genus Klebsiella has not only been identified in case reports of acute phlegmonous gastritis (Kim et al., 2011; Matsuura et al., 2018) but is also the most familiar strain isolated from the small intestine of patients with small-intestinal bacterial overgrowth or UC (Pyleris et al., 2012; Tanaka et al., 2019). In particular, K. pneumoniae has been detected in the vast majority of patients with autoimmune gastritis (Furuta et al., 2018). Also, K. oxytoca is regarded as a pathogen that induces colitis (Högenauer et al., 2006), and its levels are increased in patients with active IBD compared with those in controls (Sánchez et al., 2013).
Third, for the key microbiota with intensive interactions with other microbes recognized by network-hub ranking, there is also considerable evidence of their pathogenic roles in gastroenteric inflammation or tumor.
Microbes are associated with inflammation in the gut, including E. coli-induced infectious ileitis (Alvarado et al., 2019) and the effect of K. oxytoca on gastritis and colitis, as mentioned above. Acinetobacter lwoffii has been isolated in a gastric-tissue culture in a case of acute phlegmonous gastritis (Kim et al., 2011). Non-HP organisms can induce gastritis, particularly inflammation or lwoffi (Rathinavelu et al., 2003) and Listeria monocytogenes in mice (Park et al., 2004).
Besides, the genera Bacteroides and Bacillus are strongly associated with patients with CG accompanied with a yellow tongue coating (Ye et al., 2016) or Barrett’s esophagus (Gutiérrez-Escobar et al., 2014). Moreover, different dietary strategies can alter the Bacteroides composition post-intervention in patients with superficial CG, such as a high-fat diet with increased Bacteroides (Wan et al., 2019) and wheat peptides/fucoidan with increased B. intestinalis (Kan et al., 2019). Some microbes are used in the treatment of diarrhea-predominant IBS and IBD, including B. coagulans MTCC 5856 (Majeed et al., 2016; Shinde et al., 2019) and E. coli Nissle 1917 (Sassone-Corsi et al., 2016).
In particular, microbe co-occurrence can have a combined effect during disease. The genera Acinetobacter and Bacteroides are increased significantly in IBD patients during the active phase (Andoh et al., 2012; Tang et al., 2015), though a massive amount of Dialister invisus has been identified in the state of inactivity or dormancy in the gut of patients with IBD (Schirmer et al., 2018). Also, the genus Tyzzerella and a greater abundance of Dorea and Bacteroides have shown good performance in distinguishing between patients with gastric cancer (Thomas et al., 2016; Qi et al., 2019) and rectal cancer (Dong et al., 2019). Also, B. fragilis has been identified to contribute to CRC development (Kwong et al., 2018).
Conclusion
Recent studies have shown a high prevalence of small-bowel diseases (66% in Korea and 65.6% in China) in MGCE-examined subjects according to the data from multiple medical centers (Lim et al., 2015; Ding et al., 2019). Thus, increasing numbers of studies have focused on multiple microbiome compositions and their clinical applications through NGS, including CG, IBD, and IBS (Coker et al., 2017; Sokol et al., 2017; Vich Vila et al., 2018). However, the distribution of the fecal microbiome for explaining non-specific, mild inflammation (e.g., erosions) is largely unclear. We revealed the distribution of different species compositions, important alterations in microbiota, and metabolic dysfunction of the microbiome in fecal mNGS samples from patients with bowel inflammation or CG using a standard procedure. The metabolic dysfunctions in intestinal inflammation have been confirmed by other studies. Indeed, supplements of related metabolites can result in efficacious treatment of IBD, including diets of sage oil (rich in α-linolenic acid) and hydrolyzed protein (increased levels of secondary bile acids) (Reifen et al., 2015; Wang et al., 2019).
We used our own iENA approaches and discovered that two network-hub microbes with co-expression abundances were in ability to recognize samples in multiple clinical groups. In particular, the pivotal microorganisms in different networks were the key microbiota involved in pathogenesis. Recent reports have shown the pathogenic roles of microbes in bowel inflammation or CG, such as the genera Acinetobacter and Bacteroides in patients with IBD (Andoh et al., 2012; Tang et al., 2015).
The network-hub microbiota, significant rewiring of microbiota networks, and differential network analysis demonstrated that high-ranked iENA microbiota markers were disease specific and had particular pathogenic functions. Using mNGS combined with iENA represents a potential non-invasive step in the discrimination between gastrointestinal inflammation and healthy individuals. This approach could guide more targeted therapies against pathogens, assist in identification of disease phenotypes, and accelerate prognosis assessment of intestinal inflammation. Nevertheless, the pathogenesis, preferred timing of treatment, and patient population for clinical mNGS testing must be elucidated fully through further research.
Data Availability Statement
The raw data generated for this study can be found in The National Omics Data Encyclopedia (NODE), project ID OEP000779.
Ethics Statement
The studies involving human participants were reviewed and approved by the Ethics Committee of Shanghai Jiao Tong University Affiliated Sixth People’s Hospital, Shanghai, China. The patients/participants provided their written informed consent to participate in this study. Written informed consent was obtained from the individual(s) for the publication of any potentially identifiable images or data included in this manuscript.
Author Contributions
J-SZ, JZ, and X-TY supervised the study. X-TY, X-YC, and JZ designed the experiments and analyzed the data. X-YC, H-NF, and H-WQ carried out the experiments. X-TY, X-YC, H-KZ, and LS undertook the data analysis. X-YC and X-TY prepared the manuscript. X-TY and X-YC revised the manuscript.
Funding
This study was supported by grants from the National Natural Science Foundation of China (81873143 and 61803360), Double-Hundred Talent Plan of Shanghai Jiao Tong University School of Medicine (20191831), Shanghai Science and Technology Commission Western Medicine Guide project (17411966500), and Shanghai Jiao Tong University School of Medicine Transformation Medicine and Innovation Center Research Project (TM201723).
Conflict of Interest
The authors declare that the research was conducted in the absence of any commercial or financial relationships that could be construed as a potential conflict of interest.
Supplementary Material
The Supplementary Material for this article can be found online at: https://www.frontiersin.org/articles/10.3389/fbioe.2020.00299/full#supplementary-material
FIGURE S1 | Different diversities of samples.
TABLE S1 | List of differential microbes.
TABLE S2 | List of differential functions.
TABLE S3 | List of markers from iENA.
TABLE S4 | Baseline characteristics and distribution of participants.
TABLE S5 | List of network-hub microbes.
References
Ai, D., Pan, H., Li, X., Gao, Y., Liu, G., and Xia, L. C. (2019). Identifying gut microbiota associated with colorectal cancer using a zero-inflated lognormal model. Front. Microbiol. 10:826. doi: 10.3389/fmicb.2019.00826
Aktas, H., and Mensink, P. B. (2012). Small bowel diagnostics: current place of small bowel endoscopy. Best Pract. Res. Clin. Gastroenterol. 26, 209–220. doi: 10.1016/j.bpg.2012.03.007
Alvarado, D. M., Chen, B., Iticovici, M., Thaker, A. I., Dai, N., VanDussen, K. L., et al. (2019). Epithelial Indoleamine 2,3-dioxygenase 1 modulates aryl hydrocarbon receptor and notch signaling to increase differentiation of secretory cells and alter mucus-associated microbiota. Gastroenterology 157, 1093–1108.e11. doi: 10.1053/j.gastro.2019.07.013.
Andoh, A., Kuzuoka, H., Tsujikawa, T., Nakamura, S., Hirai, F., Suzuki, Y., et al. (2012). Multicenter analysis of fecal microbiota profiles in Japanese patients with Crohn’s disease. J. Gastroenterol. 47, 1298–1307. doi: 10.1007/s00535-012-0605-0
Buchfink, B., Xie, C., and Huson, D. H. (2015). Fast and sensitive protein alignment using DIAMOND. Nat. Methods 12, 59–60. doi: 10.1038/nmeth.3176
Chen, L., Liu, R., Liu, Z.-P., Li, M., and Aihara, K. (2012). Detecting early-warning signals for sudden deterioration of complex diseases by dynamical network biomarkers. Sci. Rep. 2:342. doi: 10.1038/srep00342
Chen, L., Wilson, J. E., Koenigsknecht, M. J., Chou, W.-C., Montgomery, S. A., Truax, A. D., et al. (2017). NLRP12 attenuates colon inflammation by maintaining colonic microbial diversity and promoting protective commensal bacterial growth. Nat. Immunol. 18, 541–551. doi: 10.1038/ni.3690
Choung, R. S., Larson, S. A., Khaleghi, S., Rubio-Tapia, A., Ovsyannikova, I. G., King, K. S., et al. (2017). Prevalence and morbidity of undiagnosed celiac disease from a community-based study. Gastroenterology 152, 830–839.e5. doi: 10.1053/j.gastro.2016.11.043
Clerc, F., Novokmet, M., Dotz, V., Reiding, K. R., de Haan, N., Kammeijer, G. S. M., et al. (2018). Plasma N-Glycan signatures are associated with features of inflammatory bowel diseases. Gastroenterology 155, 829–843. doi: 10.1053/j.gastro.2018.05.030
Coker, O. O., Dai, Z., Nie, Y., Zhao, G., Cao, L., Nakatsu, G., et al. (2017). Mucosal microbiome dysbiosis in gastric carcinogenesis. Gut 67, 1024–1032. doi: 10.1136/gutjnl-2017-314281
Costamagna, G., Shah, S. K., Riccioni, M. E., Foschia, F., Mutignani, M., Perri, V., et al. (2002). A prospective trial comparing small bowel radiographs and video capsule endoscopy for suspected small bowel disease. Gastroenterology 123, 999–1005. doi: 10.1053/gast.2002.35988
Ding, Z., Shi, H., Zhang, H., Meng, L., Fan, M., Han, C., et al. (2019). Gastroenterologist-level identification of small-bowel diseases and normal variants by capsule endoscopy using a deep-learning model. Gastroenterology 157, 1044–1054. doi: 10.1053/j.gastro.2019.06.025.e5
Dixon, M. F., Genta, R. M., Yardley, J. H., and Correa, P. (1996). Classification and grading of gastritis. The updated Sydney system. International Workshop on the histopathology of gastritis, Houston 1994. Am. J. Surg. Pathol. 20, 1161–1181. doi: 10.1097/00000478-199610000-00001
Dong, Z., Chen, B., Pan, H., Wang, D., Liu, M., Yang, Y., et al. (2019). Detection of microbial 16S rRNA gene in the serum of patients with gastric cancer. Front. Oncol. 9:608. doi: 10.3389/fonc.2019.00608
Fujii, H., Shinzaki, S., Iijima, H., Wakamatsu, K., Iwamoto, C., Sobajima, T., et al. (2016). Core fucosylation on T cells, required for activation of T-cell receptor signaling and induction of colitis in mice, is increased in patients with inflammatory bowel disease. Gastroenterology 150, 1620–1632. doi: 10.1053/j.gastro.2016.03.002
Fujii, M., Suzuki, K., Suenaga, S., Wakatsuki, M., Kushida, Y., Touma, M., et al. (2014). Dominant trait linked to chromosome 1 in DBA/2 mice for the resistance to autoimmune gastritis appears in bone marrow cells. Exp. Anim. 63, 155–167. doi: 10.1538/expanim.63.155
Furuta, T., Baba, S., Yamade, M., Uotani, T., Kagami, T., Suzuki, T., et al. (2018). High incidence of autoimmune gastritis in patients misdiagnosed with two or more failures of H. pylori eradication. Aliment. Pharmacol. Ther. 48, 370–377. doi: 10.1111/apt.14849
Gupta, A., Dhakan, D. B., Maji, A., Saxena, R., Vishnu Prasoodanan, P. K., Mahajan, S., et al. (2019). Association of flavonifractor plautii, a flavonoid-degrading bacterium, with the gut microbiome of colorectal cancer patients in India. mSystems 4:e438-19.
Gutiérrez-Escobar, A. J., Bayona-Rojas, M., Barragan-Vidal, C., Rojas-Lara, S., and Oliveros, R. (2014). Metagenomic analysis of the gastric microbiota cultivable from a patient with gastritis concomitant with Barrett’s esophagus. Rev. Gastroenterol. Peru 34, 229–235.
He, C., Peng, C., Wang, H., Ouyang, Y., Zhu, Z., Shu, X., et al. (2019). The eradication of Helicobacter pylori restores rather than disturbs the gastrointestinal microbiota in asymptomatic young adults. Helicobacter 24:e12590. doi: 10.1111/hel.12590
Högenauer, C., Langner, C., Beubler, E., Lippe, I. T., Schicho, R., Gorkiewicz, G., et al. (2006). Klebsiella oxytoca as a causative organism of antibiotic-associated hemorrhagic colitis. N. Engl. J. Med. 355, 2418–2426. doi: 10.1056/NEJMoa054765
Hsieh, Y.-Y., Tung, S.-Y., Pan, H.-Y., Yen, C.-W., Xu, H.-W., Lin, Y.-J., et al. (2018). Increased abundance of clostridium and fusobacterium in gastric microbiota of patients with gastric cancer in Taiwan. Sci. Rep. 8:158.
Kan, J., Cheng, J., Xu, L., Hood, M., Zhong, D., Cheng, M., et al. (2019). The combination of wheat peptides and fucoidan protects against chronic superficial gastritis and alters gut microbiota: a double-blinded, placebo-controlled study. Eur. J. Nutr. [Epub ahead of print].
Kansal, S., Catto-Smith, A. G., Boniface, K., Thomas, S., Cameron, D. J., Oliver, M., et al. (2019). Variation of Gut mucosal microbiome with ASCA status in pediatric Crohn’s disease. J. Pediatr. Gastroenterol. Nutr. [Epub ahead of print].
Khalili, H., Burke, K. E., Roelstraete, B., Sachs, M. C., Olén, O., and Ludvigsson, J. F. (2020). Microscopic colitis and risk of inflammatory bowel disease in a nationwide cohort study. Gastroenterology [Epub ahead of print].
Kim, N. Y., Park, J. S., Lee, K. J., Yun, H. K., and Kim, J. S. (2011). A case of acute phlegmonous gastritis causing gastroparesis and cured with medical treatment alone. Korean J. Gastroenterol. 57, 309–314. doi: 10.4166/kjg.2011.57.5.309
Kwong, T. N. Y., Wang, X., Nakatsu, G., Chow, T. C., Tipoe, T., Dai, R. Z. W., et al. (2018). Association between bacteremia from specific microbes and subsequent diagnosis of colorectal cancer. Gastroenterology 155, 383–390.e8. doi: 10.1053/j.gastro.2018.04.028
Labus, J. S., Hollister, E. B., Jacobs, J., Kirbach, K., Oezguen, N., Gupta, A., et al. (2017). Differences in gut microbial composition correlate with regional brain volumes in irritable bowel syndrome. Microbiome 5:49. doi: 10.1186/s40168-017-0260-z
Langmead, B., and Salzberg, S. L. (2012). Fast gapped-read alignment with Bowtie 2. Nat. Methods 9, 357–359. doi: 10.1038/nmeth.1923
Li, D., Liu, C.-M., Luo, R., Sadakane, K., and Lam, T.-W. (2015). MEGAHIT: an ultra-fast single-node solution for large and complex metagenomics assembly via succinct de Bruijn graph. Bioinformatics 31, 1674–1676. doi: 10.1093/bioinformatics/btv033
Li, W., and Godzik, A. (2006). Cd-hit: a fast program for clustering and comparing large sets of protein or nucleotide sequences. Bioinformatics 22, 1658–1659. doi: 10.1093/bioinformatics/btl158
Lim, Y. J., Lee, O. Y., Jeen, Y. T., Lim, C. Y., Cheung, D. Y., Cheon, J. H., et al. (2015). Indications for detection, completion, and retention rates of small bowel capsule endoscopy based on the 10-year data from the korean capsule endoscopy registry. Clin. Endosc. 48, 399–404. doi: 10.5946/ce.2015.48.5.399
Machiels, K., Sabino, J., Vandermosten, L., Joossens, M., Arijs, I., de Bruyn, M., et al. (2017). Specific members of the predominant gut microbiota predict pouchitis following colectomy and IPAA in UC. Gut 66, 79–88. doi: 10.1136/gutjnl-2015-309398
Majeed, M., Nagabhushanam, K., Natarajan, S., Sivakumar, A., Ali, F., Pande, A., et al. (2016). Bacillus coagulans MTCC 5856 supplementation in the management of diarrhea predominant Irritable Bowel Syndrome: a double blind randomized placebo controlled pilot clinical study. Nutr. J. 15:21.
Martinez-Guryn, K., Hubert, N., Frazier, K., Urlass, S., Musch, M. W., Ojeda, P., et al. (2018). Small intestine microbiota regulate host digestive and absorptive adaptive responses to dietary lipids. Cell Host Microbe 23, 458–469.e5. doi: 10.1016/j.chom.2018.03.011
Matsui, S., Kataoka, H., Tanaka, J.-I., Kikuchi, M., Fukamachi, H., Morisaki, H., et al. (2019). Dysregulation of intestinal microbiota elicited by food allergy induces IgA-mediated oral dysbiosis. Infect. Immun. 88:e741-19.
Matsuura, K., Hiramatsu, S., Taketani, R., Ishibashi, K., Uraoka, M., Baba, S., et al. (2018). Medical treatment of postendoscopic submucosal dissection phlegmonous gastritis in an elderly diabetic woman with myelodysplastic syndrome. Case Rep. Gastrointest. Med. 2018, 8046817. doi: 10.1155/2018/8046817
Niv, Y., and Niv, G. (2005). Capsule endoscopy examination-preliminary review by a nurse. Digest. Dis. Sci. 50, 2121–2124. doi: 10.1007/s10620-005-3017-7
Noguchi, H., Park, J., and Takagi, T. (2006). MetaGene: prokaryotic gene finding from environmental genome shotgun sequences. Nucleic Acids Res. 34, 5623–5630. doi: 10.1093/nar/gkl723
Otani, K., Tanigawa, T., Watanabe, T., Shimada, S., Nadatani, Y., Nagami, Y., et al. (2017). Microbiota plays a key role in non-steroidal anti-inflammatory drug-induced small intestinal damage. Digestion 95, 22–28. doi: 10.1159/000452356
Pan, M., Wan, C., Xie, Q., Huang, R., Tao, X., Shah, N. P., et al. (2016). Changes in gastric microbiota induced by Helicobacter pylori infection and preventive effects of Lactobacillus plantarum ZDY 2013 against such infection. J. Dairy Sci. 99, 970–981. doi: 10.3168/jds.2015-10510
Park, J.-H., Kim, D.-J., Park, Y.-H., Seok, S.-H., Cho, S.-A., Baek, M.-W., et al. (2004). Characteristics of the gastritis induced by Listeria monocytogenes in mice: microbiology, histopathology, and mRNA expression of inflammatory mediators with time course of infection. Microb. Pathog. 37, 87–94. doi: 10.1016/j.micpath.2004.05.002
Pyleris, E., Giamarellos-Bourboulis, E. J., Tzivras, D., Koussoulas, V., Barbatzas, C., and Pimentel, M. (2012). The prevalence of overgrowth by aerobic bacteria in the small intestine by small bowel culture: relationship with irritable bowel syndrome. Dig. Dis. Sci. 57, 1321–1329. doi: 10.1007/s10620-012-2033-7
Qi, Y.-F., Sun, J.-N., Ren, L.-F., Cao, X.-L., Dong, J.-H., Tao, K., et al. (2019). Intestinal microbiota is altered in patients with gastric cancer from shanxi province, China. Dig. Dis. Sci. 64, 1193–1203. doi: 10.1007/s10620-018-5411-y
Rathinavelu, S., Zavros, Y., and Merchant, J. L. (2003). Acinetobacter lwoffii infection and gastritis. Microbes Infect. 5, 651–657. doi: 10.1016/s1286-4579(03)00099-6
Reifen, R., Karlinsky, A., Stark, A. H., Berkovich, Z., and Nyska, A. (2015). α-Linolenic acid (ALA) is an anti-inflammatory agent in inflammatory bowel disease. J. Nutr. Biochem. 26, 1632–1640. doi: 10.1016/j.jnutbio.2015.08.006
Sánchez, E., Donat, E., Ribes-Koninckx, C., Fernández-Murga, M. L., and Sanz, Y. (2013). Duodenal-mucosal bacteria associated with celiac disease in children. Appl. Environ. Microbiol. 79, 5472–5479. doi: 10.1128/aem.00869-13
Sassone-Corsi, M., Nuccio, S.-P., Liu, H., Hernandez, D., Vu, C. T., Takahashi, A. A., et al. (2016). Microcins mediate competition among Enterobacteriaceae in the inflamed gut. Nature 540, 280–283. doi: 10.1038/nature20557
Schäffler, H., Herlemann, D. P., Klinitzke, P., Berlin, P., Kreikemeyer, B., Jaster, R., et al. (2018). Vitamin D administration leads to a shift of the intestinal bacterial composition in Crohn’s disease patients, but not in healthy controls. J. Dig Dis. 19, 225–234. doi: 10.1111/1751-2980.12591
Schirmer, M., Franzosa, E. A., Lloyd-Price, J., McIver, L. J., Schwager, R., Poon, T. W., et al. (2018). Dynamics of metatranscription in the inflammatory bowel disease gut microbiome. Nat. Microbiol. 3, 337–346. doi: 10.1038/s41564-017-0089-z
Shinde, T., Perera, A. P., Vemuri, R., Gondalia, S. V., Karpe, A. V., Beale, D. J., et al. (2019). Synbiotic supplementation containing whole plant sugar cane fibre and probiotic spores potentiates protective synergistic effects in mouse model of IBD. Nutrients 11:E818. doi: 10.3390/nu11040818
Sokol, H., Jegou, S., McQuitty, C., Straub, M., Leducq, V., Landman, C., et al. (2018). Specificities of the intestinal microbiota in patients with inflammatory bowel disease and Clostridium difficile infection. Gut Microbes 9, 55–60. doi: 10.1080/19490976.2017.1361092
Segata, N., Izard, J., Waldron, L., Gevers, D., Miropolsky, L., Garrett, W. S., et al. (2011). Metagenomic biomarker discovery and explanation. Genome Biol. 12:R60. doi: 10.1186/gb-2011-12-6-r60.
Sokol, H., Leducq, V., Aschard, H., Pham, H.-P., Jegou, S., Landman, C., et al. (2017). Fungal microbiota dysbiosis in IBD. Gut 66, 1039–1048. doi: 10.1136/gutjnl-2015-310746
Sung, J., Kim, S., Cabatbat, J. J. T., Jang, S., Jin, Y.-S., Jung, G. Y., et al. (2017). Global metabolic interaction network of the human gut microbiota for context-specific community-scale analysis. Nat. Commun. 8:15393. doi: 10.1038/ncomms15393
Tanaka, H., Watanabe, T., Nagai, T., Minaga, K., Kamata, K., Komeda, Y., et al. (2019). Hepatic portal venous gas associated with Klebsiella oxytoca infection in the absence of preceding antibiotic treatment. Clin. J. Gastroenterol. 12, 316–319. doi: 10.1007/s12328-019-00947-1
Tang, M. S., Poles, J., Leung, J. M., Wolff, M. J., Davenport, M., Lee, S. C., et al. (2015). Inferred metagenomic comparison of mucosal and fecal microbiota from individuals undergoing routine screening colonoscopy reveals similar differences observed during active inflammation. Gut Microbes 6, 48–56. doi: 10.1080/19490976.2014.1000080
Tatusov, R. L., Galperin, M. Y., Natale, D. A., and Koonin, E. V. (2000). The COG database: a tool for genome-scale analysis of protein functions and evolution. Nucleic Acids Res. 28, 33–36. doi: 10.1093/nar/28.1.33
Thomas, A. M., Jesus, E. C., Lopes, A., Aguiar, S., Begnami, M. D., Rocha, R. M., et al. (2016). Tissue-associated bacterial alterations in rectal carcinoma patients revealed by 16S rRNA community profiling. Front. Cell Infect. Microbiol. 6:179. doi: 10.3389/fcimb.2016.00179
Vich Vila, A., Imhann, F., Collij, V., Jankipersadsing, S. A., Gurry, T., Mujagic, Z., et al. (2018). Gut microbiota composition and functional changes in inflammatory bowel disease and irritable bowel syndrome. Sci. Transl. Med. 10:eaa8914. doi: 10.1126/scitranslmed.aap8914
Wan, Y., Wang, F., Yuan, J., Li, J., Jiang, D., Zhang, J., et al. (2019). Effects of dietary fat on gut microbiota and faecal metabolites, and their relationship with cardiometabolic risk factors: a 6-month randomised controlled-feeding trial. Gut 68, 1417–1429. doi: 10.1136/gutjnl-2018-317609
Wang, H., Li, Y., Feng, X., Li, Y., Wang, W., Qiu, C., et al. (2016). Dysfunctional gut microbiota and relative co-abundance network in infantile eczema. Gut Pathog. 8:36.
Wang, L., Yu, X., Zhang, C., and Zeng, T. (2018). Detecting personalized determinants during drug treatment from omics big data. Curr. Pharm. Des. 24, 3727–3738. doi: 10.2174/1381612824666181106102111
Wang, S., Martins, R., Sullivan, M. C., Friedman, E. S., Misic, A. M., El-Fahmawi, A., et al. (2019). Diet-induced remission in chronic enteropathy is associated with altered microbial community structure and synthesis of secondary bile acids. Microbiome 7:126.
Williams, L., Jenkins, G. J. S., Doak, S. H., Fowler, P., Parry, E. M., Brown, T. H., et al. (2005). Fluorescence in situ hybridisation analysis of chromosomal aberrations in gastric tissue: the potential involvement of Helicobacter pylori. Br. J. Cancer 92, 1759–1766. doi: 10.1038/sj.bjc.6602533
Wilson, M. R., Sample, H. A., Zorn, K. C., Arevalo, S., Yu, G., Neuhaus, J., et al. (2019). Clinical metagenomic sequencing for diagnosis of meningitis and encephalitis. N. Engl. J. Med. 380, 2327–2340. doi: 10.1056/NEJMoa1803396
Xie, C., Mao, X., Huang, J., Ding, Y., Wu, J., Dong, S., et al. (2011). KOBAS 2.0: a web server for annotation and identification of enriched pathways and diseases. Nucleic Acids Res. 39, W316–W322. doi: 10.1093/nar/gkr483
Ye, J., Cai, X., Yang, J., Sun, X., Hu, C., Xia, J., et al. (2016). Bacillus as a potential diagnostic marker for yellow tongue coating. Sci. Rep. 6:32496. doi: 10.1038/srep32496
Yu, X., Chen, X., and Wang, Z. (2019). Characterizing the personalized microbiota dynamics for disease classification by individual-specific edge-network analysis. Front. Genet. 10:283. doi: 10.3389/fgene.2019.00283
Yu, X., Zeng, T., Wang, X., Li, G., and Chen, L. (2015). Unravelling personalized dysfunctional gene network of complex diseases based on differential network model. J. Transl. Med. 13:189.
Yu, X., Zhang, J., Sun, S., Zhou, X., Zeng, T., and Chen, L. (2017). Individual-specific edge-network analysis for disease prediction. Nucleic Acids Res. 45:e170. doi: 10.1093/nar/gkx787
Zeng, T., Sun, S.-Y., Wang, Y., Zhu, H., and Chen, L. (2013). Network biomarkers reveal dysfunctional gene regulations during disease progression. FEBS J. 280, 5682–5695. doi: 10.1111/febs.12536
Zeng, T., Wang, D. C., Wang, X., Xu, F., and Chen, L. (2014). Prediction of dynamical drug sensitivity and resistance by module network rewiring-analysis based on transcriptional profiling. Drug Resist. Update 17, 64–76. doi: 10.1016/j.drup.2014.08.002
Zeng, T., Zhang, W., Yu, X., Liu, X., Li, M., and Chen, L. (2016). Big-data-based edge biomarkers: study on dynamical drug sensitivity and resistance in individuals. Brief. Bioinform. 17, 576–592. doi: 10.1093/bib/bbv078
Zhang, B., Xu, S., Xu, W., Chen, Q., Chen, Z., Yan, C., et al. (2019). Leveraging fecal bacterial survey data to predict colorectal tumors. Front. Genet. 10:447. doi: 10.3389/fgene.2019.00447
Keywords: magnetically guided capsule endoscopy, metagenomics, edge-network analysis, chronic gastritis, small bowel erosion
Citation: Chen X-Y, Fan H-N, Zhang H-K, Qin H-W, Shen L, Yu X-T, Zhang J and Zhu J-S (2020) Rewiring of Microbiota Networks in Erosive Inflammation of the Stomach and Small Bowel. Front. Bioeng. Biotechnol. 8:299. doi: 10.3389/fbioe.2020.00299
Received: 15 January 2020; Accepted: 20 March 2020;
Published: 13 May 2020.
Edited by:
Wen Zhang, Huazhong Agricultural University, ChinaReviewed by:
Jingmin Ou, Xinhua Hospital, Shanghai Jiao Tong University, ChinaZheng Wang, Albert Einstein College of Medicine, United States
Copyright © 2020 Chen, Fan, Zhang, Qin, Shen, Yu, Zhang and Zhu. This is an open-access article distributed under the terms of the Creative Commons Attribution License (CC BY). The use, distribution or reproduction in other forums is permitted, provided the original author(s) and the copyright owner(s) are credited and that the original publication in this journal is cited, in accordance with accepted academic practice. No use, distribution or reproduction is permitted which does not comply with these terms.
*Correspondence: Xiang-Tian Yu, graceyu1985@163.com; Jing Zhang, jing5522724@163.com; Jin-Shui Zhu, zhujs1803@163.com
†These authors have contributed equally to this work