- 1College of Biotechnology and Pharmaceutical Engineering, Nanjing Technology University, Nanjing, China
- 2Institute of Microbiology, Xinjiang Academy of Agricultural Sciences, Urumqi, China
- 3School of Food Science and Pharmaceutical Engineering, Nanjing Normal University, Nanjing, China
Geosmin is one of the most common earthy-musty odor compounds, which is mainly produced by Streptomyces. Streptomyces radiopugnans was screened in radiation-polluted soil, which has the potential to overproduce geosmin. However, due to the complex cellular metabolism and regulation mechanism, the phenotypes of S. radiopugnans were hard to investigate. A genome-scale metabolic model of S. radiopugnans named iZDZ767 was constructed. Model iZDZ767 involved 1,411 reactions, 1,399 metabolites, and 767 genes; its gene coverage was 14.1%. Model iZDZ767 could grow on 23 carbon sources and five nitrogen sources, which achieved 82.1% and 83.3% prediction accuracy, respectively. For the essential gene prediction, the accuracy was 97.6%. According to the simulation of model iZDZ767, D-glucose and urea were the best for geosmin fermentation. The culture condition optimization experiments proved that with D-glucose as the carbon source and urea as the nitrogen source (4 g/L), geosmin production could reach 581.6 ng/L. Using the OptForce algorithm, 29 genes were identified as the targets of metabolic engineering modification. With the help of model iZDZ767, the phenotypes of S. radiopugnans could be well resolved. The key targets for geosmin overproduction could also be identified efficiently.
Introduction
Geosmin (trans-1,10-dimethyl-trans-9-decalol) is an irregular sesquiterpenoid of various actinomycetes and fungi, which has a distinct earthy or musty odor (Jiang et al., 2007). Geosmin is associated with the flavors in drinking water, wine, fish, and other foodstuffs. Several microorganisms, such as most Streptomyces (Jiang et al., 2006; Becher et al., 2020) and several species of cyanobacteria (Jiang et al., 2006; Giglio et al., 2008), myxobacteria (Dickschat et al., 2005), and fungi (Liato and Aider, 2017) can produce geosmin. Streptomyces radiopugnans belongs to the genus of Streptomyces and has been isolated from radiation-polluted soil from the Xinjiang Province in China (Mao et al., 2007). The genome of S. radiopugnans was sequenced in 2016 and can be accessed from the NCBI database. However, limited by the lack of experimental data, the regulation mechanism of geosmin biosynthesis was not clear in S. radiopugnans.
The genome-scale metabolic model (GSMM) is a mathematical model, which presents the gene-protein-reaction relationship. GSMM has been being developed for more than 20 years, since the first published GSMM of Haemophilus influenzae in 1999. Over 2,000 GSMMs have been published for over 1,000 organisms (Ye et al., 2022). With the increase of experimental data, some published GSMMs are continuously being improved. Some typical organisms, such as Escherichia coli K12 (6 GSMMs) (Ye et al., 2022), Saccharomyces cerevisiae S288c (12 GSMMs) (Ye et al., 2022), and Yarrowia lipolytica CLIB 122 (6 GSMMs) (Loira et al., 2012; Pan and Hua, 2012; Kavscek et al., 2015; Kerkhoven et al., 2016; Wei et al., 2017; Mishra et al., 2018) have more than one GSMM. Combined with different algorithms, GSMMs have been widely applied in network properties analysis, cellular phenotype prediction, metabolic engineering guidance, model-driven discovery, evolutionary process exploration, and interspecies interaction identification (Gu et al., 2019; Jansma and El Aidy, 2021; Patra et al., 2021).
In this study, based on the genome sequence of S. radiopugnans, a GSMM named iZDZ767 was constructed. Model iZDZ767 contained 1,411 reactions, 1,399 metabolites, and 767 genes. Compared with experimental data, iZDZ767 could achieve 82.1% and 83.3% accuracy for the utilization of different carbon sources and nitrogen sources. In addition, the prediction accuracy of essential genes was 97.6%, compared with the DEG database (Luo et al., 2021). Then, based on iZDZ767, D-glucose and urea were identified as the most suitable carbon source and nitrogen source, respectively. The experiments proved that when urea was used as nitrogen and controlled at 4 g/L, geosmin production could reach 581.6 ng/L. Finally, 29 genes (seven upregulation, six downregulation, and 16 knockout targets) were identified as potential targets, which could improve the geosmin synthesis rate using the OptForce algorithm (Ranganathan et al., 2010). This study provides new insights that could be used to investigate the phenotype of S. radiopugnans and identify the metabolic engineering targets for geosmin overproduction.
Materials and methods
Strain
The S. radiopugnans R97T strain was screened from the contaminated radiation-contaminated area in Xinjiang, China (Mao et al., 2007).
Genome sequence
The genome sequence of S. radiopugnans was downloaded from the NCBI database (https://www.ncbi.nlm.nih.gov/genome/49825?genome_assembly_id=1862392). The protein sequences of S. radiopugnans were downloaded from the UniProt database (https://www.uniprot.org/taxonomy/403935).
Culture medium
The fermentation medium (1 L) contained 10 g/L glucose, 4 g/L yeast tract, 4 g/L K2HPO4, 4 g/L KH2PO4, and 0.5 g/L MgSO4. Before cultivation, the medium pH was adjusted to 7.2 using NH4OH (25%, v/v).
Culture condition
For shake-flask cultivation, the S. radiopugnans strain was first cultured on an agar plate. Then, a single colony was selected to be cultured in 50 mL fresh medium until the OD600 reached a value of 0.8. Finally, the strain was transferred into a 500 mL shake-flask containing 50 mL fermentation medium and cultivated at 30°C for 240 h with shaking at 200 rpm.
Determining the growth rate and glucose consumption rate
The optical density (OD) was first measured at 600 nm with a spectrophotometer. The cell dry weight was then calculated by multiplying OD600 by 0.36 g/L (Supplementary Figure S1) (Fischer and Sawers, 2013). The growth curve was fitted using the Logistic function of Origin software. Finally, the cell growth rate was calculated with differential values of cell dry weight (Supplementary Figure S2). Similarly, the glucose consumption rate was also calculated using Origin software, based on the experimental data (Supplementary Figure S3).
Geosmin extraction and analysis
The extraction and analysis of geosmin were followed by (Shen et al., 2021).
Prediction of optimized fermentation conditions
The robustness analysis [(controlFlux, objFlux) = robustnessAnalysis (model, controlRxn, nPoints, plotResFlag, objRxn, objType)] program was run in MATLAB to simulate the effect of the urea uptake rate on the synthesis rate of geosmin.
Hardware and software used for model construction and analysis
Detailed information is listed in Supplementary Table S1.
Results
Model construction and characteristics analysis
To construct the genome-scale metabolic model of S. radiopugnans, several steps were carried out. First, ModelSEED (Henry et al., 2010) and CarveME (Machado et al., 2018) were used to construct the draft model of S. radiopugnans. Then, based on the KAAS annotation (Moriya et al., 2007) results, gaps were fixed by referring to KEGG pathway maps, manually. In addition, the biomass composition of S. radiopugnans was identified through literature mining, which includes proteins, RNA, DNA, lipids, cell wall, and small molecules (Supplementary Material S1). Finally, the defined model was mathematized with COBRA toolbox 3.0 (Heirendt et al., 2019). The model of S. radiopugnans consisted of 1,411 reactions, 1,399 metabolites, and 767 genes, and was named iZDZ767 (Table 1; Supplementary Material S1). Of these reactions, 88.0% were gene associated. According to the KEGG pathway maps, these reactions can be classified into 10 subsystems. Lipid metabolism, carbohydrate metabolism, and amino acid metabolism were the most common, accounting for 25.3%, 18.1%, and 16.3%, respectively (Figure 1). The gene coverage of model iZDZ767 was 14.1%, which was close to the newest model iAA1259 of Streptomyces coelicolor (15.1%) (Amara et al., 2018). Cytoscape software was used to analyze the network characteristics of model iZDZ767. There were 3,577 nodes and 8,706 edges in model iZDZ767.
Model verification
Based on the minimal culture medium, model iZDZ767 was used to simulate whether 28 carbon sources and 6 nitrogen sources could be utilized. For carbon sources, model iZDZ767 achieved 82.1% (23/28) correction. For the nitrogen sources, there was 83.3% (5/6) agreement with the experimental results, which could not grow with L-Cysteine as the sole nitrogen source (Table 2). In addition, the simulated maximum growth rate (μmax) was 0.131 h−1, which was only 4.4% lower than the measured growth rate (0.137 h−1, Supplementary Figure S2). The essentialities of individual genes of S. radiopugnans were analyzed under minimal glucose medium conditions using iZDZ767 by deleting each gene in turn. The genes were categorized into three classes: essential genes, partially essential genes, and non-essential genes. There were 84 genes identified as essential genes. These genes were further compared with the DEG database (Luo et al., 2021), and 97.6% of the predicted essential genes could be matched by sequence blast (identity ≥30%, e-value ≤ 1e-6, Supplementary Material S2). These results proved that model iZDZ767 could predict the phenotype of S. radiopugnans well.
The optimization of culture condition with iZDZ767
The carbon source was a key factor for cell growth and product synthesis. Using model iZDZ767, the effect of different carbon sources, such as D-glucose, D-Fructose, Mannose, L-Rhamnose, and D-xylose was predicted. Of these selected carbon sources, D-glucose was the most suitable, the GPR was 2.04 mmol/gDW/h (Figure 2A). The experimental results show that when D-glucose was used as a carbon source, the yield of geosmin was 317.5 ng/L, which was higher than others (Figure 2B). Similarly, three types of nitrogen sources were used for simulation. The model predicted that when urea was used as a nitrogen source, the GPR was 3.03 mmol/gDW/h, which was 48.9% and 50.7% higher than NH4+ and NO3− (Figure 3A). Compared with the experiments, the geosmin yield was 435.1 ng/L, which agreed with the simulation (Figure 3B). In addition, a robustness analysis algorithm was used to analyze the effect of the urea uptake rate on the geosmin production rate. The simulated results showed that with the increase in the urea uptake rate, the GPR would first increase to the maximum value, then remain stable until the urea uptake rate was over 500 mmol/gDW/h. Finally, the GPR would decrease to 0, which means that the suitable urea uptake rate should be 88.38 mmol/gDW/h (Figure 3C). When we controlled the addition of urea at different levels, the experiment results proved that 4 g/L urea could achieve a maximum geosmin production of 581.6 ng/L (Figure 3D).
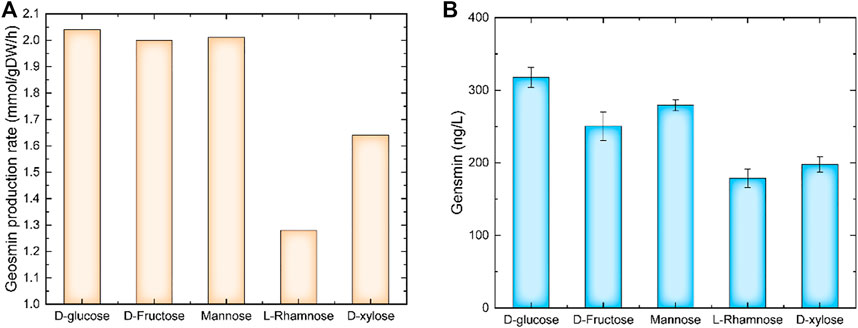
FIGURE 2. The effect of carbon sources on geosmin production. (A) Simulation results of different carbon sources. (B) Experimental results of different carbon sources.
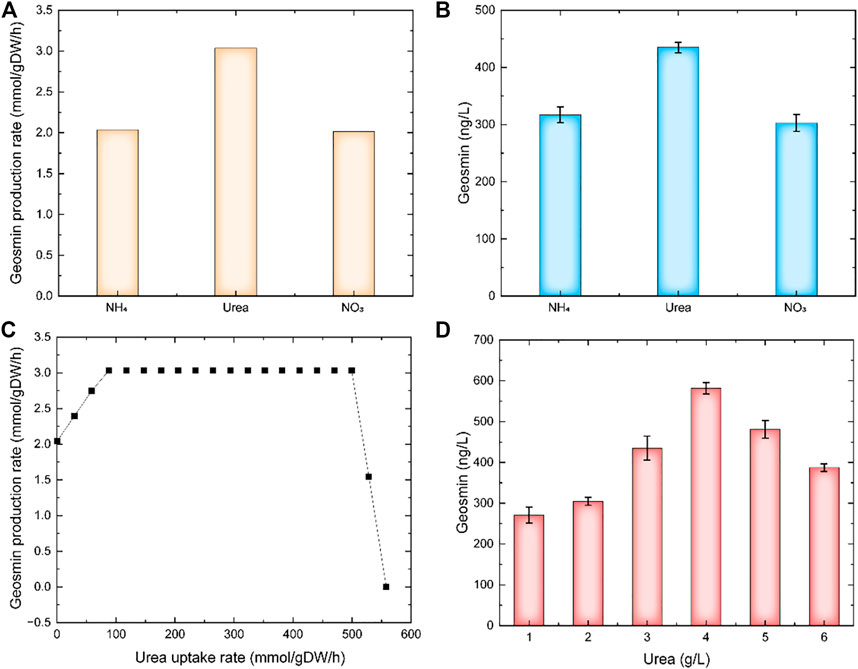
FIGURE 3. The effect of nitrogen sources on geosmin production. (A) simulation results of different nitrogen sources. (B) Experimental results of different nitrogen sources. (C) Robustness analysis results of urea uptake rate. (D) Effect of urea concentration on geosmin production.
Identification of the potential geosmin overproduction targets with iZDZ767
To identify potential targets for the improvement of geosmin production, the OptForce algorithm was used (Ranganathan et al., 2010). According to the predicted results, a total of 29 genes were identified as the targets, including seven upregulation, six downregulation, and 16 knockout targets (Supplementary Material S3). According to the function of each gene, these targets can be classified into four types: precursor accumulation, geosmin biosynthesis, by-product elimination, and energy supplement. For the geosmin synthesis pathway, the geoA gene, which encodes geosmin synthase, catalyzing the synthesis of geosmin from farnesyl diphosphate, should be upregulated (Shen et al., 2021). For by-product elimination, to accumulate more geosmin, the acnA gene (Aconitate hydratase A) should be downregulated to decrease the carbon flux of the TCA cycle (Figure 4). Similarly, the fabD gene [(acyl-carrier-protein) S-malonyltransferase] should also be downregulated to limit the flux of fatty acids synthesis. For energy supplements, the nuo gene (NADH-quinone oxidoreductase) was predicted to be knocked out so that more NADH could be supplied for geosmin synthesis.
Discussion
Geosmin is a common pollutant and is widely recognized by the public, but this is not the case when studying some biological systems and organisms. Toxicological studies have shown that a certain concentration of geosmin could inhibit the growth of Salmonella typhimurium and sea urchin embryos (Dionigi et al., 1993; Nakajima et al., 1996). This provides a new direction for the study of how to inhibit pathogens. At the same time, researchers have also found that geosmin has a potential effect on genotoxicity. Geosmin is only mildly toxic at extremely high concentrations, far exceeding the actual level in the environment (Silva et al., 2015). Some researchers have found that geosmin, at a concentration of 50–5000 ng/L, can increase the body length and change the growth-related genes of zebrafish (Zhou et al., 2020).
S. radiopugnans can be screened from radiation-contaminated soil and, although not widely studied, are capable of producing geosmin in large quantities. However, phenotypes of S. radiopugnans are difficult to study due to their complex cellular metabolism and regulatory mechanisms. Therefore, by manually refining the first genome-scale metabolic network model (iZDZ767) of S. radiopugnans, we analyzed the synthesis mechanism of geosmin and identified the key targets of geosmin synthesis based on the model, which provided a basis for further study of the synthesis of geosmin and the internal mechanism of S. radiopugnans.
Traditional model construction methods are mainly divided into automatic construction and manual construction. Among them, automatic construction automatically obtains the GSMM of the target strain or plant by uploading the genomic data to the existing tools. To date, researchers have developed many tools, such as ModelSEED (Flowers et al., 2018; Seaver et al., 2021), COBRA (Dal’molin et al., 2014; King et al., 2015), and RAVEN (Wang et al., 2018), for the automatic construction of models. The advantage of this construction method is that the model can be built in a short time, but the accuracy of the model is low and its applicability is not strong. The manual construction of this method mainly depends on the results of genome annotation, combined with the metabolic pathway of KEGG, it collates information, such as the genes and metabolites of each reaction, and manually adds it to the model. Considering the problems of traditional modeling methods, we used a semi-automatic modeling method. This method integrates the first two methods, first obtaining a coarse model through the automated construction tool, then manually refining the model so that a more accurate model can be obtained. Based on the automatic construction of the ModelSEED database and CarveME (Machado et al., 2018), combined with the complete metabolic pathway in KEGG, we added the missing reaction to the model and added the known metabolic pathway of geosmin to the model and, thus, manually refined a genome-scale metabolic network model of S. radiopugnans. Based on model iZDZ767, a series of strategies for improving geosmin were proposed. Although S. radiopugnans can make good use of microbial fermentation to produce geosmin, its metabolic network is complex, and the fermentation experiment period is long. It depends on repeated experiments to increase the production of geosmin, and the economic cost is high. Model iZDZ767 can predict the effects of carbon and nitrogen sources on the synthesis rate of geosmin well and provide directions for optimizing the culture conditions of S. radiopugnans. At the same time, the model is used to analyze the algorithm to predict the key targets for improving the geosmin synthesis rate. Through the analysis of these key targets, we found that the key reactions affecting the synthesis of geosmin are mainly divided into two types. One type of reaction is related to the synthesis of geosmin itself, while the other is related to the growth and reproduction of S. radiopugnans. No matter which type of reaction is upregulated, downregulated, or knocked out, the synthesis of geosmin can be effectively improved. Although the effectiveness of these regulation methods has not been proven, they provide the opportunity to study geosmin production by fermentation. In summary, model iZDZ767 is a powerful tool for analyzing and predicting the metabolic pathways and yields of various products in S. radiopugnans, which provides convenient conditions for us to study S. radiopugnans in the field of systems biology.
Data availability statement
Publicly available datasets were analyzed in this study. This data can be found here: https://www.uniprot.org/taxonomy/403935.
Author contributions
ZZ constructed the model and wrote this manuscript. QG analyzed data and drew figures. JQ answered the reviewers' questions. CY and HH proof-read the manuscript. All authors have read and approved the final manuscript.
Funding
This work was supported by the National Natural Science Foundation of China (32060004), the Xinjiang Academy of Agricultural Sciences Science and technology innovation key cultivation project (xjkcpy-2021002, xjkcpy-2022004), the Third Xinjiang Scientific Expedition Program (2022xjkk1200), and the “Outstanding Youth Fund” of Xinjiang Natural Science Foundation (2022D01E19).
Conflict of interest
The authors declare that the research was conducted in the absence of any commercial or financial relationships that could be construed as a potential conflict of interest.
Publisher’s note
All claims expressed in this article are solely those of the authors and do not necessarily represent those of their affiliated organizations, or those of the publisher, the editors and the reviewers. Any product that may be evaluated in this article, or claim that may be made by its manufacturer, is not guaranteed or endorsed by the publisher.
Supplementary material
The Supplementary Material for this article can be found online at: https://www.frontiersin.org/articles/10.3389/fbioe.2023.1108412/full#supplementary-material
References
Amara, A., Takano, E., and Breitling, R. (2018). Development and validation of an updated computational model of Streptomyces coelicolor primary and secondary metabolism. BMC Genomics 19, 519. ARTN 519. doi:10.1186/s12864-018-4905-5
Becher, P. G., Verschut, V., Bibb, M. J., Bush, M. J., Molnar, B. P., Barane, E., et al. (2020). Developmentally regulated volatiles geosmin and 2-methylisoborneol attract a soil arthropod to Streptomyces bacteria promoting spore dispersal. Nat. Microbiol. 5 (6), 821–829. doi:10.1038/s41564-020-0697-x
Dal'molin, C. G. O., Quek, L-E., Palfreyman, R. W., and Nielsen, L. K. (2014). Plant genome-scale modeling and implementation. Methods Mol. Biol. Clift. NJ) 1090, 317–332. doi:10.1007/978-1-62703-688-7_19
Dhamodharan, D., Naine, S. J., Keziah, S. M., and Devi, C. S. (2019). Novel fibrinolytic protease producing Streptomyces radiopugnans VITSD8 from marine sponges. Mar. Drugs 17 (3), 164. ARTN 164. doi:10.3390/md17030164
Dickschat, J. S., Bode, H. B., Mahmud, T., Muller, R., and Schulz, S. (2005). A novel type of geosmin biosynthesis in myxobacteria. J. Org. Chem. 70 (13), 5174–5182. doi:10.1021/jo050449g
Dionigi, C. P., Lawlor, T. E., Mcfarland, J. E., and Johnsen, P. B. J. W. R. (1993). Evaluation of geosmin and 2-methylisoborneol on the histidine dependence of TA98 and TA100 Salmonella typhimurium tester strains. Water Res. 27 (11), 1615–1618. doi:10.1016/0043-1354(93)90125-2
Flowers, J. J., Richards, M. A., Baliga, N., Meyer, B., and Stahl, D. A. (2018). Constraint-based modelling captures the metabolic versatility of Desulfovibrio vulgaris. Environ. Microbiol. Rep. 10 (2), 190–201. doi:10.1111/1758-2229.12619
Giglio, S., Jiang, J. Y., Saint, C. P., Cane, D. E., and Monis, P. T. (2008). Isolation and characterization of the gene associated with geosmin production in cyanobacteria. Environ. Sci. Technol. 42 (21), 8027–8032. doi:10.1021/es801465w
Gu, C., Kim, G. B., Kim, W. J., Kim, H. U., and Lee, S. Y. (2019). Current status and applications of genome-scale metabolic models. Genome Biol. 20 (1), 121. doi:10.1186/s13059-019-1730-3
Heirendt, L., Arreckx, S., Pfau, T., Mendoza, S. N., Richelle, A., Heinken, A., et al. (2019). Creation and analysis of biochemical constraint-based models using the COBRA Toolbox v.3.0. Nat. Protoc. 14 (3), 639–702. doi:10.1038/s41596-018-0098-2
Henry, C. S., DeJongh, M., Best, A. A., Frybarger, P. M., Linsay, B., and Stevens, R. L. (2010). High-throughput generation, optimization and analysis of genome-scale metabolic models. Nat. Biotechnol. 28 (9), 977–982. doi:10.1038/nbt.1672
Jansma, J., and El Aidy, S. (2021). Understanding the host-microbe interactions using metabolic modeling. Microbiome 9 (1), 16. ARTN 16. doi:10.1186/s40168-020-00955-1
Jiang, J. Y., He, X. F., and Cane, D. E. (2007). Biosynthesis of the earthy odorant geosmin by a bifunctional Streptomyces coelicolor enzyme. Nat. Chem. Biol. 3 (11), 711–715. doi:10.1038/nchembio.2007.29
Jiang, J. Y., He, X. F., and Cane, D. E. (2006). Geosmin biosynthesis. Streptomyces coelicolor germacradienol/germacrene D synthase converts farnesyl diphosphate to geosmin. J. Am. Chem. Soc. 128 (25), 8128–8129. doi:10.1021/ja062669x
Kavscek, M., Bhutada, G., Madl, T., and Natter, K. (2015). Optimization of lipid production with a genome-scale model of Yarrowia lipolytica. BMC Syst. Biol. 9, 72. ARTN 72. doi:10.1186/s12918-015-0217-4
Kerkhoven, E. J., Pomraning, K. R., Baker, S. E., and Nielsen, J. (2016). Regulation of amino-acid metabolism controls flux to lipid accumulation in Yarrowia lipolytica. NPJ Syst. Biol. Appl. 2, 16005. ARTN 16005. doi:10.1038/npjsba.2016.5
King, Z. A., Lloyd, C. J., Feist, A. M., and Palsson, B. O. (2015). Next-generation genome-scale models for metabolic engineering. Curr. Opin. Biotechnol. 35, 23–29. doi:10.1016/j.copbio.2014.12.016
Liato, V., and Aider, M. (2017). Geosmin as a source of the earthy-musty smell in fruits, vegetables and water: Origins, impact on foods and water, and review of the removing techniques. Chemosphere 181, 9–18. doi:10.1016/j.chemosphere.2017.04.039
Loira, N., Dulermo, T., Nicaud, J. M., and Sherman, D. J. (2012). A genome-scale metabolic model of the lipid-accumulating yeast Yarrowia lipolytica. BMC Syst. Biol. 6 (1), 35. doi:10.1186/1752-0509-6-35
Luo, H., Lin, Y., Liu, T., Lai, F. L., Zhang, C. T., Gao, F., et al. (2021). DEG 15, an update of the Database of Essential Genes that includes built-in analysis tools. Nucleic Acids Res. 49 (D1), D677–D686. doi:10.1093/nar/gkaa917
Machado, D., Andrejev, S., Tramontano, M., and Patil, K. R. (2018). Fast automated reconstruction of genome-scale metabolic models for microbial species and communities. Nucleic Acids Res. 46 (15), 7542–7553. doi:10.1093/nar/gky537
Mao, J., Tang, Q. Y., Zhang, Z. D., Wang, W., Wei, D., Huang, Y., et al. (2007). Streptomyces radiopugnans sp nov., a radiation-resistant actinomycete isolated from radiation-polluted soil in China. Int. J. Syst. Evol. Microbiol. 57, 2578–2582. doi:10.1099/ijs.0.65027-0
Mishra, P., Lee, N. R., Lakshmanan, M., Kim, M., Kim, B. G., and Lee, D. Y. (2018). Genome-scale model-driven strain design for dicarboxylic acid production in Yarrowia lipolytica. BMC Syst. Biol. 12, 12. ARTN 12. doi:10.1186/s12918-018-0542-5
Moriya, Y., Itoh, M., Okuda, S., Yoshizawa, A. C., and Kanehisa, M. (2007). Kaas: An automatic genome annotation and pathway reconstruction server. Nucleic Acids Res. 35, W182–W185. doi:10.1093/nar/gkm321
Nakajima, M., Ogura, T., Kusama, Y., Iwabuchi, N., Imawaka, T., Araki, A., et al. (1996). Inhibitory effects of odor substances, geosmin and 2-methylisoborneol, on early development of sea urchins. early Dev. sea urchins 30 (10), 2508–2511. doi:10.1016/0043-1354(96)00104-2
Pan, P., and Hua, Q. (2012). Reconstruction and in silico analysis of metabolic network for an oleaginous yeast, Yarrowia lipolytica. Plos One 7 (12), e51535. doi:10.1371/journal.pone.0051535
Patra, P., Das, M., Kundu, P., and Ghosh, A. (2021). Recent advances in systems and synthetic biology approaches for developing novel cell-factories in non-conventional yeasts. Biotechnol. Adv. 47, 107695. ARTN 107695. doi:10.1016/j.biotechadv.2021.107695
Ranganathan, S., Suthers, P. F., and Maranas, C. D. (2010). OptForce: An optimization procedure for identifying all genetic manipulations leading to targeted overproductions. PLoS Comp. Biol. 6 (4), e1000744. ARTN e1000744. doi:10.1371/journal.pcbi.1000744
Santhanam, R., Okoro, C. K., Rong, X. Y., Huang, Y., Bull, A. T., Weon, H. Y., et al. (2012). Streptomyces atacamensis sp nov., isolated from an extreme hyper-arid soil of the Atacama Desert, Chile. Int. J. Syst. Evol. Microbiol. 62, 2680–2684. doi:10.1099/ijs.0.038463-0
Seaver, S. M. D., Liu, F., Zhang, Q. Z., Jeffryes, J., Faria, J. P., Edirisinghe, J. N., et al. (2021). The ModelSEED Biochemistry Database for the integration of metabolic annotations and the reconstruction, comparison and analysis of metabolic models for plants, fungi and microbes. Nucleic Acids Res. 49 (D1), D575–D588. doi:10.1093/nar/gkaa746
Shen, Q. Y., Shimizu, K., Miao, H. C., Tsukino, S., Utsumi, M., Lei, Z. F., et al. (2021). Effects of elevated nitrogen on the growth and geosmin productivity of Dolichospermum smithii. Environ. Sci. Pollut. R. 28 (1), 177–184. doi:10.1007/s11356-020-10429-4
Silva, A. F., Lehmann, M., and Dihl, R. R. (2015). Geosmin induces genomic instability in the mammalian cell microplate-based comet assay. Environ. Sci. Pollut. Res. 22 (21), 17244–17248. doi:10.1007/s11356-015-5381-y
Wang, H., Marcisauskas, S., Sanchez, B. J., Domenzain, I., Hermansson, D., Agren, R., et al. (2018). Raven 2.0: A versatile toolbox for metabolic network reconstruction and a case study on Streptomyces coelicolor. Plos Comput. Biol. 14 (10), e1006541. doi:10.1371/journal.pcbi.1006541
Wei, S. S., Jian, X. X., Chen, J., Zhang, C., and Hua, Q. (2017). Reconstruction of genome-scale metabolic model of Yarrowia lipolytica and its application in overproduction of triacylglycerol. Bioresour. Bioprocess 4, 51. ARTN 51. doi:10.1186/s40643-017-0180-6
Ye, C., Wei, X. Y., Shi, T. Q., Sun, X. M., Xu, N., Gao, C., et al. (2022). Genome-scale metabolic network models: From first-generation to next-generation. Appl. Microbiol. Biotechnol. 106 (13-16), 4907–4920. doi:10.1007/s00253-022-12066-y
Zhou, W. C., Wang, J. L., Zhang, J. L., Peng, C. R., Li, G. B., and Li, D. H. (2020). Environmentally relevant concentrations of geosmin affect the development, oxidative stress, apoptosis and endocrine disruption of embryo-larval zebrafish. Sci. Total Environ. 735, 139373. doi:10.1016/j.scitotenv.2020.139373
Keywords: geosmin, Streptomyces radiopugnans, genome-scale metabolic model, culture condition optimization, metabolic engineering
Citation: Zhang Z, Guo Q, Qian J, Ye C and Huang H (2023) Construction and application of the genome-scale metabolic model of Streptomyces radiopugnans. Front. Bioeng. Biotechnol. 11:1108412. doi: 10.3389/fbioe.2023.1108412
Received: 26 November 2022; Accepted: 07 February 2023;
Published: 17 February 2023.
Edited by:
Mingfeng Cao, Xiamen University, ChinaCopyright © 2023 Zhang, Guo, Qian, Ye and Huang. This is an open-access article distributed under the terms of the Creative Commons Attribution License (CC BY). The use, distribution or reproduction in other forums is permitted, provided the original author(s) and the copyright owner(s) are credited and that the original publication in this journal is cited, in accordance with accepted academic practice. No use, distribution or reproduction is permitted which does not comply with these terms.
*Correspondence: Chao Ye, Y2hhb3llMDlAbmpudS5lZHUuY24=; He Huang, aHVhbmdoQG5qbnUuZWR1LmNu
†These authors have contributed equally to this work