- 1Department of Sports Medicine, Honghui Hospital, Xi’an Jiaotong University, Xi’an, China
- 2Department of Foot and Ankle Surgery, Honghui Hospital, Xi’an Jiaotong University, Xi’an, China
The COVID-19 pandemic caused by the severe acute coronavirus disease 2 (SARS-CoV-2) virus represents an ongoing threat to human health and well-being. Notably, many COVID-19 patients suffer from complications consistent with osteoporosis (OP) following disease resolution yet the mechanistic links between SARS-CoV-2 infection and OP remain to be clarified. The present study was thus developed to explore the potential basis for this link by employing transcriptomic analyses to identify signaling pathways and biomarkers associated with OP and SARS-CoV-2. Specifically, a previously published RNA-sequencing dataset (GSE152418) from Gene Expression Omnibus (GEO) was used to identify the differentially expressed genes (DEGs) in OP patients and individuals infected with SARS-CoV-2 as a means of exploring the underlying molecular mechanisms linking these two conditions. In total, 2,885 DEGs were identified by analyzing the COVID-19 patient dataset, with shared DEGs then being identified by comparison of these DEGs with those derived from an OP patient dataset. Hub genes were identified through a series of bioinformatics approaches and protein-protein interaction analyses. Predictive analyses of transcription factor/gene interactions, protein/drug interactions, and DEG/miRNA networks associated with these DEGs were also conducted. Together, these data highlight promising candidate drugs with the potential to treat both COVID-19 and OP.
Introduction
The COVID-19 (coronavirus disease 2019) pandemic, caused by the single-stranded RNA severe acute respiratory syndrome coronavirus 2 (SARS-CoV-2) virus, has caused extensive death and suffering throughout the world (Chen et al., 2022; Shapira et al., 2022; Swets et al., 2022). Notably, many older adults that recover from SARS-CoV-2 infection have been reported to develop new-onset or aggravated osteoporosis (OP). As a systemic bone disease, OP is associated with reductions in bone density, increased bone fragility, and the degradation of the bone microstructure (Xu et al., 2022). Several potential mechanisms may explain the observed link between COVID-19 and OP development. For one, a range of antiviral drugs are employed in the treatment of COVID-19 patients, including corticosteroids, which can contribute to OP onset or aggravation (Xiong et al., 2020). Infected patients also exhibit abnormal increases in miR-4485 expression in their bone marrow, and this microRNA (miRNA) has been reported to target TLR for and to interfere with appropriate osteogenic remodeling (Mi et al., 2021). COVID-19 can also interfere with patient exercise and medication use, both of which can contribute to higher OP rates in elderly individuals (Salvio et al., 2022). There is thus a clear need to better clarify the mechanisms linking SARS-CoV-2 infection and OP development.
The angiotensin-converting enzyme 2 (ACE2) receptor has been confirmed to play a key role as a receptor that SARS-CoV-2 utilizes to enter into and infect target cells (Casciola-Rosen et al., 2022; Popescu et al., 2022). Bone tissue, however, exhibits only low levels of ACE2 expression, and whether COVID-19-related bone damage is the result of direct infection or is secondary to pathological changes in other organs thus remains to be conclusively established. While certain bone marrow cells do exhibit ACE2 receptor expression, a biological link between SARS-CoV-2 and bone remodeling has yet to be demonstrated.
The present study was developed to explore the potential mechanisms whereby SARS-CoV-2 infection may influence OP development or severity. To that end, extant RNA-sequencing (RNA-seq) data were utilized to conduct transcriptomic analyses of signaling pathways and biomarkers associated with SARS-CoV-2 infection and OP. Differentially expressed genes (DEGs) expressed in OP patients infected with SARS-CoV-2 were identified using the GSE152418 dataset from the GEO database, and the resultant gene list was used to explore relevant signaling pathways as well as candidate drugs with the potential to aid in COVID-19 treatment. Hub regulatory genes in this pathological context were further identified through a series of bioinformatics analyses and through the construction of a protein-protein interaction (PPI) network. Moreover, efforts to clarify the biological link between SARS-CoV-2 infection and OP incidence were made by using the identified DEGs to conduct predictive transcription factor/gene interaction, protein/drug interaction, and DEG/miRNA network analyses with the goal of elucidating the underlying molecular mechanism.
Results
Data Search and DEG Identification
Initially, the GSE152418 dataset was downloaded from the GEO database. This dataset, consisting of 17 COVID-19 patients and 17 healthy controls, was analyzed, revealing 2,885 DEGs between these two groups (2,701 upregulated, 184 downregulated in COVID-19) (Figure 1A). The GSE100609 dataset consisting of 4 OP patients and 4 healthy controls was subsequently retrieved from the GEO database and analyzed, leading to the identification of 494 DEGs, of which 296 and 198 were respectively upregulated and downregulated in OP patients (Figure 1B).
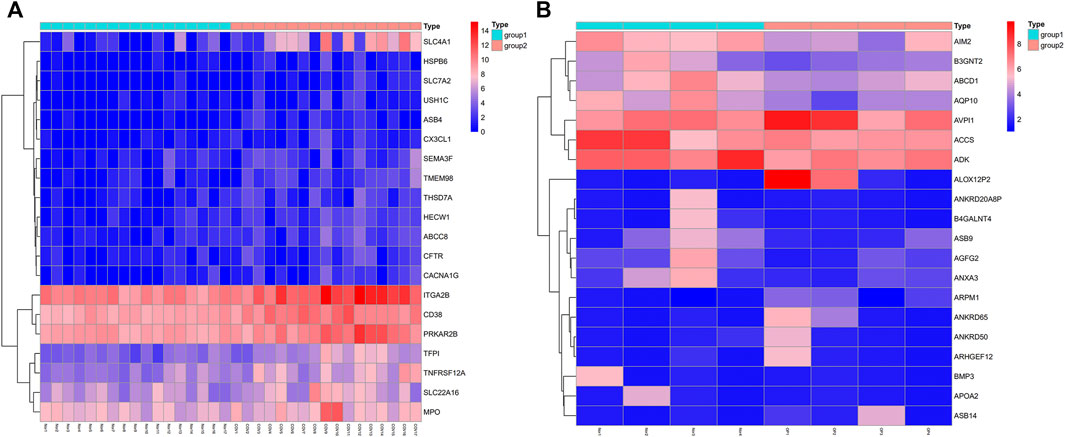
FIGURE 1. Identification of COVID-19 and OP-related DEGs. (A) GSE152418: COVID-19 patients vs. controls. (B) GSE100609: OP patients vs. controls.
Identification of DEGs Shared Between COVID-19 and OP
The JVenn program was next used to identify 73 DEGs shared between these COVID-19 and OP datasets, suggesting that they may be associated with the biological link between these two conditions (Figure 2). Given this overlap, it is possible that drugs capable of treating OP may offer value in the treatment of COVID-19.
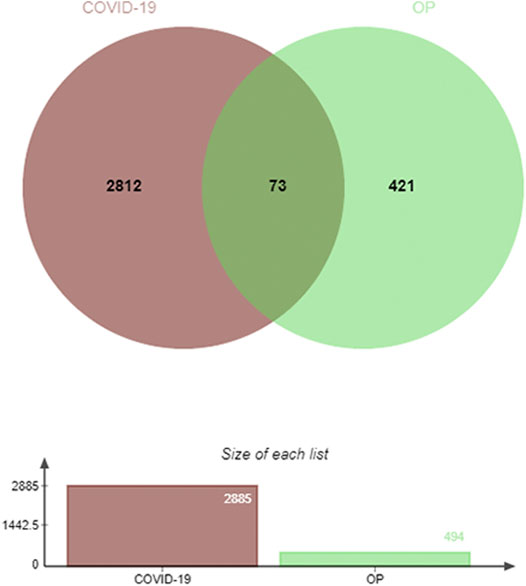
FIGURE 2. Identification of DEGs shared between COVID-19 and OP. In total, 73 shared DEGs were identified.
Gene Enrichment Analyses of Shared COVID-19- and OP-Related DEGs
Next, GO and KEGG enrichment analyses of these shared DEGs were conducted using the Enrichr application. These DEGs were significantly enriched in biological process GO terms including regulated exocytosis, platelet degranulation, cytokine-mediated signaling pathway, regulation of macrophage-derived foam cell differentiation, and positive regulation of tau-protein kinase activity. They were also enriched for cellular component GO terms including platelet alpha granule, platelet alpha granule lumen, muscle myosin complex, platelet alpha granule membrane, high-density, and lipoprotein particle. With respect to molecular function GO terms, these shared DEGs were enriched in the calcium-dependent phospholipid binding, CXCR chemokine receptor binding, glutamate receptor activity, ligand-gated channel activity, and ligand-gated ion channel activity terms (Figure 3; Table 1). Common DEG-enriched pathways were further explored using the KEGG, Reactome, WikiPathway, and BioCarta databases, with the top 10 enriched terms being compiled in Figure 4 and Table 2.
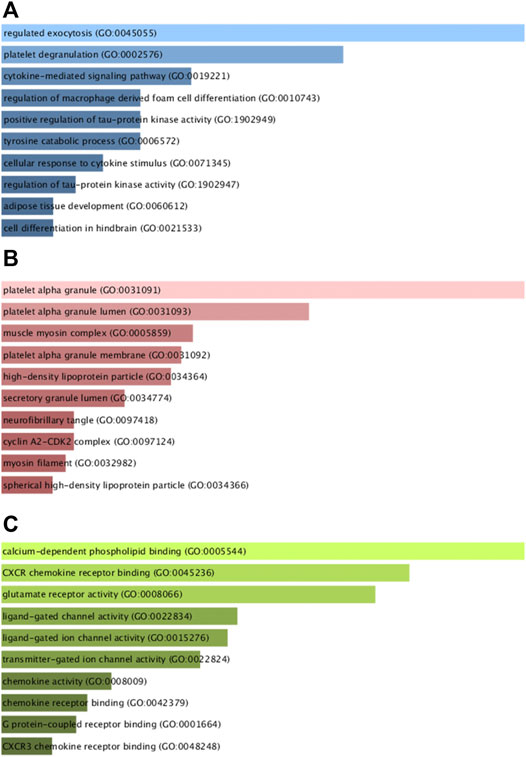
FIGURE 3. GO analysis of mutual DEGs associated with COVID-19 and OP. (A) biological process, (B) cellular component, and (C) molecular function GO term enrichment results.
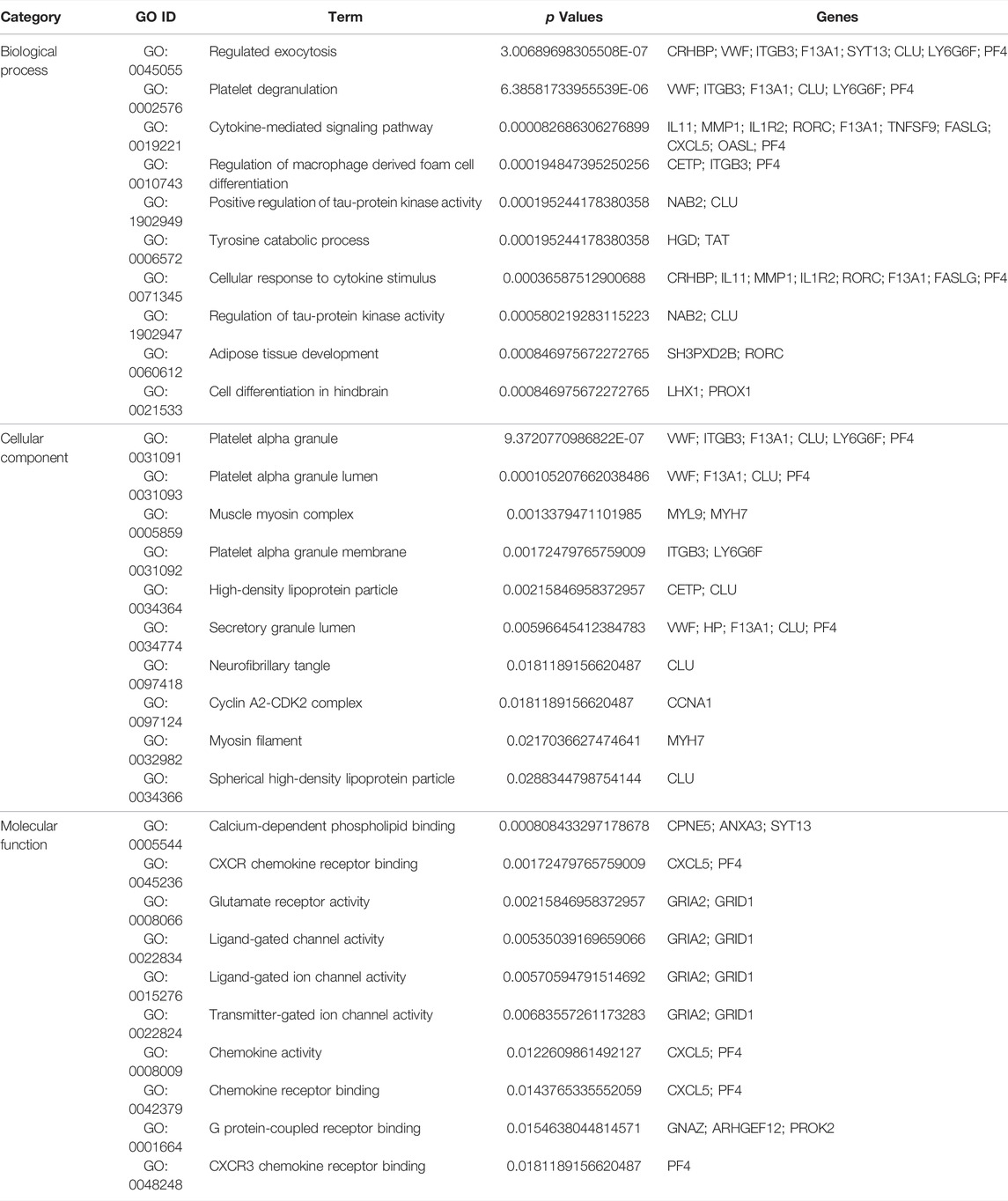
TABLE 1. GO analysis of common DEGs beween COVID-19 and osteoporosis (Top 10 terms of each category are listed).
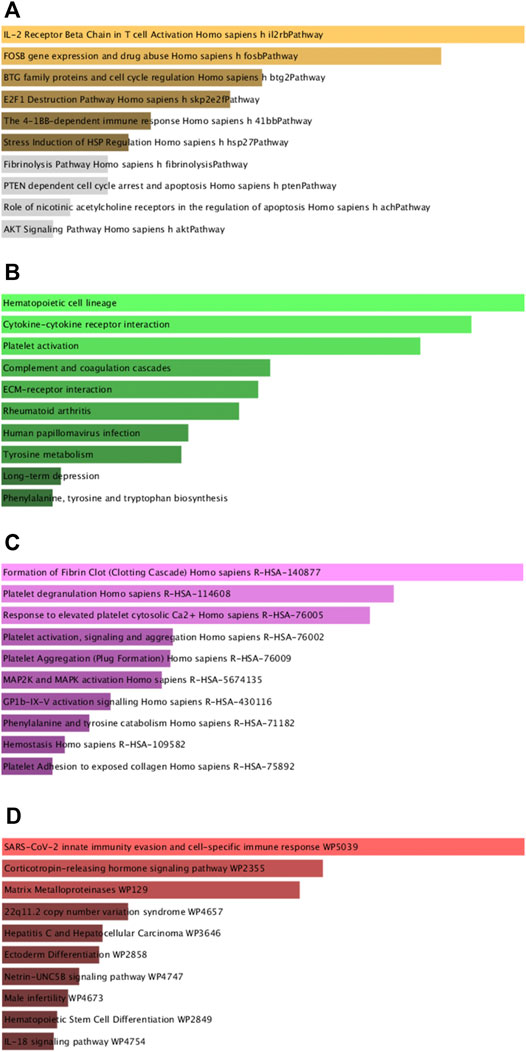
FIGURE 4. Pathway enrichment analyses of mutual DEGs associated with COVID-19 and OP. (A). BioCarta, (B). KEGG, (C). Reactome, (D). WikiPathway.
PPI Network Construction and Hub Gene Identification
Following the importation of the 73 shared DEGs into the STRING database, a PPI network consisting of 73 nodes and 235 edges was generated (Figure 5A), with the Cytoscape 3.7.2 tool being used to reorganize this network (Figure 5B) The Cytohubba plugin was then used to identify the 10 genes with the highest degree value in this network, with these genes (CXCL5, MMP1, PF4, VWF, IL11, ITGB3, HP, IL1R2, FASLG, MMP10) being identified as hub genes (Figure 5C).
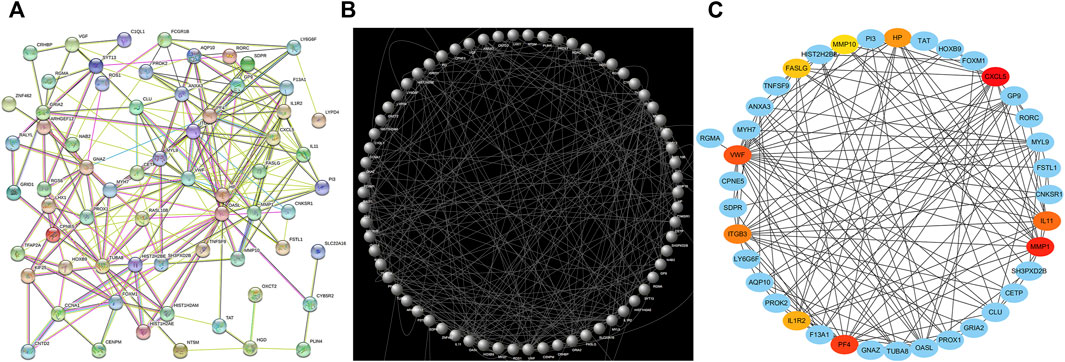
FIGURE 5. PPI network and hub genes analyses. (A) STRING was used to generate a PPI network. (B) Cytoscape was used for PPI network reorganization. (C) The Cytohubba plugin was used for hub gene identification.
Identification of Transcription Factors and miRNAs Associated With Shared DEGs
To more fully explore the potential regulation of the identified hub DEGs at the transcriptional level, the NetworkAnalyst tool was used to identify shared transcription factors and miRNAs associated with these DEGs that may regulate their expression (Figure 6).
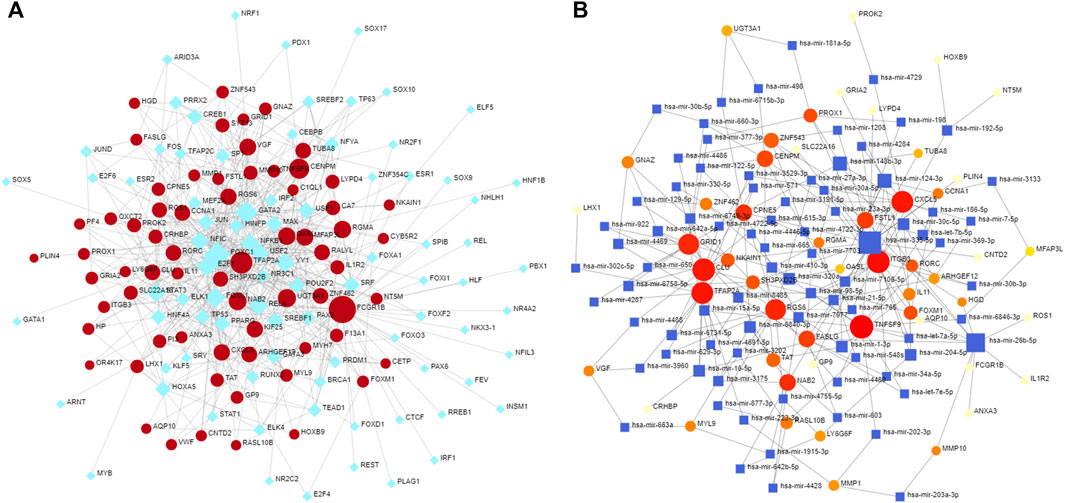
FIGURE 6. Transcription factors and miRNAs associated with mutual DEGs. (A) Regulatory DEG-transcription factor interactions, with transcription factors and gene symbols being shown in blue and red, respectively. (B) Interconnected DEG/miRNA regulatory network, with miRNAs and gene symbols being shown in blue and reg, respectively.
Exploration of Potential Therapeutic Drugs and Gene-Disease Associations
Lastly, the DSigDB database was used to identify candidate drugs associated with these shared DEGs, working under the assumption that these genes may represent promising therapeutic targets associated with both COVID-19 and OP. The top 10 retrieved drug compounds were ARSENIC CTD 00005442, MS-275 PC3 UP, camptothecin PC3 UP, fluoride CTD 00005982, 1,4-chrysenequinone PC3 UP, azacitidine PC3 UP, sanguinarine HL60 UP, benzo [a]pyrene CTD 00005488, ellipticine PC3 UP, and CP-690334-01 PC3 UP (Figure 7; Table 3). Gene-disease association analyses conducted with Network-Analyst further revealed the Hypersensitivity, Prostatic Neoplasms, Atherosclerosis, Diabetic Angiopathies, Anemia, Myocardial Infarction, Schizophrenia, Hemorrhage, Unipolar Depression, and Major Depressive Disorder disease states to be most associated with the identified COVID-19/OP-related hub genes (Figure 8).
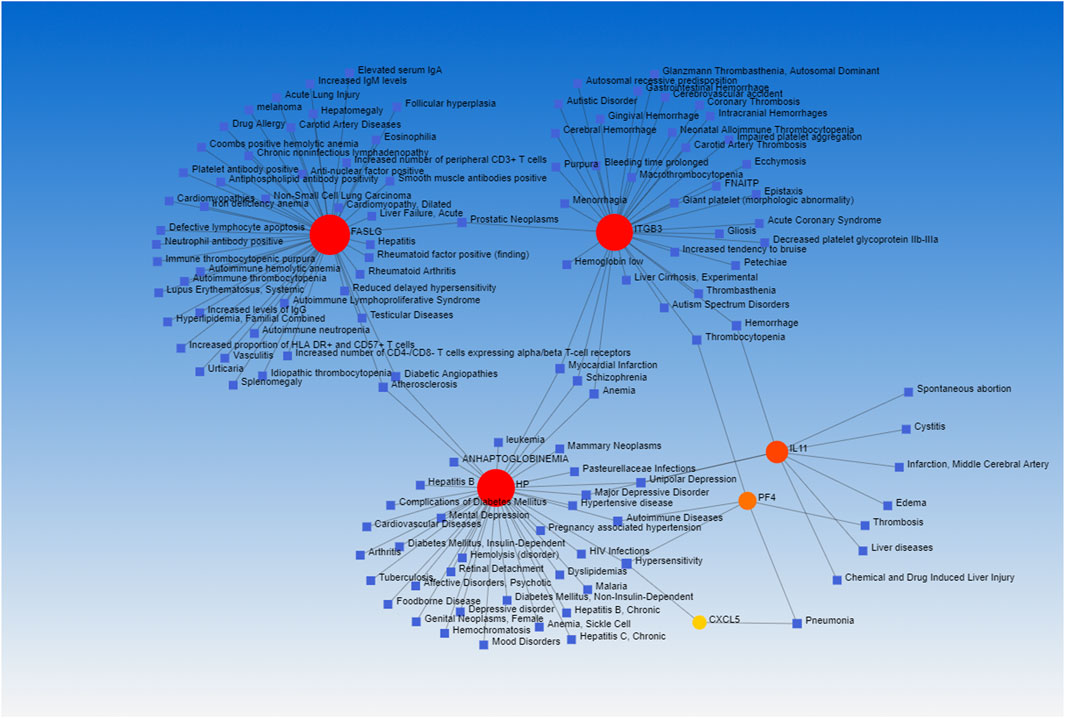
FIGURE 8. Hub gene-disease association network. Disorders and hub genes are respectively represented in blue and red.
Discussion
OP is a systemic bone disease that results in reductions in bone density, increased bone fragility, and the degradation of the bone microstructure. Recent research suggests that there may be an important link between COVID-19 and the onset or aggravation of OP among older adults. The present study was thus conducted to identify potential molecular biomarkers that may be shared between COVID-19 and OP in an effort to clarify the link between these two conditions and to guide future therapeutic efforts. High-throughput gene expression profiling is commonly used to identify molecular biomarkers associated with a range of disease states (Mahmud et al., 2021). In the present study, 73 DEGs shared between SARS-CoV-2-infected and OP patients were identified through transcriptomic analyses, and these genes were then subject to further enrichment analyses aimed at better exploring the link between OP and COVID-19.
GO analyses enable the assessment of the regulatory relationships for particular genes based on theoretical models of associated genes and internal relationships (Nashiry et al., 2021), with these predictive enrichment analyses being based on a growing base of knowledge regarding gene function and associated ontological classes (Wang et al., 2022). GO analyses broadly classify gene characteristics based on associated biological process (BP), cellular component (CC), and molecular function (MF) terms (Cao et al., 2022). The shared DEGs identified in this study were enriched in BP terms including regulated exocytosis (8 genes) and the platelet degranulation signaling pathway (6 genes) are among the top GO terms. Exocytosis is closely tied to the survival and function of osteoblasts (Oppert et al., 2022), and the regulation of osteoblast exocytosis by vitamin D3 is reportedly significant in the context of bone remodeling (Chen et al., 2021). The shared DEGs were also highly enriched in the platelet alpha granule (6 genes) and platelet alpha granule lumen (4 genes) CC terms. Platelet alpha granules impact endothelial exocytosis and OP development, and platelets play a well-documented role in regulating bone remodeling and vascular function (Li et al., 2021; Salamanna et al., 2021). The top MF terms in which these shared DEGs were enriched included calcium-dependent phospholipid binding (3 genes) and CXCR chemokine receptor binding (2 genes). Notably, calcium-dependent phospholipid binding plays a key role in viral replication (Schleiss et al., 2021), while the CXCR chemokine receptor family can recruit stem cells to facilitate subsequent osteogenic differentiation (Khokhar et al., 2022).
KEGG enrichment analyses were additionally used to explore shared pathways associated with these 73 common DEGs evident in the analyzed COVID-19 and OP datasets. The top 10 KEGG pathways associated with these DEGs included the hematopoietic cell lineage, cytokine-cytokine receptor interaction, platelet activation, complement and coagulation cascades, ECM-receptor interaction, rheumatoid arthritis, human papillomavirus infection, tyrosine metabolism, long-term depression, and phenylalanine, tyrosine and tryptophan biosynthesis pathways. A PPI network was further constructed based on the identified DEGs, with the hub proteins in this network (CXCL5, MMP1, PF4, VWF, IL11, ITGB3, HP, IL1R2, FASLG, MMP10) being regarded as the most critical shared regulators of OP and SARS-CoV-2 infection. CXCL5 signaling is associated with cellular proliferation and differentiation (Kawagoe et al., 2020), and the aberrant expression of this chemokine can lead to a range of dysfunctional outcomes, potentially contributing to the incidence of infection and/or OP development (Zhang et al., 2020).
Transcription factors and miRNAs that may function as upstream regulators of these DEGs were additionally identified in an effort to gain more insight into the pathological basis of these disease states. Many drugs have been tested for the treatment of COVID-19, including favipiravir, which has been shown to exhibit promising antiviral efficacy against COVID-19 (Cai et al., 2020). In this study, the DSigDB database was used to identify drugs with the potential to regulate target hub genes shared in COVID-19 and OP patient datasets. The top 10 of these candidate pharmacological agents were ARSENIC CTD 00005442, MS-275 PC3 UP, camptothecin PC3 UP, fluoride CTD 00005982, 1,4-chrysenequinone PC3 UP, azacitidine PC3 UP, sanguinarine HL60 UP, benzo [a]pyrene CTD 00005488, ellipticine PC3 UP, CP-690334-01 PC3 UP. A further understanding of the biological relationships between SARS-CoV-2 infection and OP development may help to mitigate the risk of OP onset following COVID-19 resolution. It is reported that some OP drugs are associated with reduced risk of pneumonia (Sing et al., 2020). Many older adults suffering from COVID-19 also exhibit comorbid OP. The identification of pathogenic factors shared by these two diseases may enable the simultaneous administration of antiviral and anti-OP agents with the potential to provide immense clinical benefit to this patient population.
There are some limitations of this study. Firstly, we didn’t perform any in vivo or in vitro experiments to validate our bioinformatics result, because it is a preliminary study. Validations will be carried out in our further research. Secondly, the number of cases in GSE100609 is relatively small. In addition, for clinical traslation of our findings, it is really a long way from the bench side to clinical use. Osteoporosis is one of the most common disorders in the elderly, who are also relatively vulnerable to COVID-19. The drugs identified in this study are promising to improve both osteoporosis and COVID-19, providing references for further studies.
Materials and Methods
Data Search and DEG Identification
The GEO (Gene Expression Omnibus, https://www.ncbi.nlm.nih.gov/geo/) database repository, which compiles MIAME-compliant data submissions, was searched to identify RNA-seq data corresponding to COVID-19 and OP patients. Genes differentially expressed between COVID-19 or OP patients and healthy controls were identified with the R limma package and DESeq2 using the following criteria: p < 0.05 and |logFC| > 1.0. A heatmap of the identified DEGs was constructed with the R pheatmap package applied to detect significant DEGs. The heatmap was drawn with the pheatmap package in R.
Identification of Shared DEGs Between COVID-19 and OP Patients
All DEGs identified in the initial comparison of COVID-19 and OP patient datasets were imported into the online JVenn tool (http://jvenn.toulouse.inra.fr/app/example.html), which was used to identify mutual DEGs shared between these two datasets. The shared DEGs were then represented in a pie chart generated by JVenn.
Functional Enrichment Analyses of Shared DEGs
GO (Gene ontology) and KEGG (Kyoto Encyclopedia of Genes and Genomes) pathway analyses enable researchers to gauge the potential functional roles of particular genes of interest. The Enricher tool is a web-based platform that enables these functional enrichment analyses following the importation of gene lists of interest. For the present study, Enrichr analyses of mutual DEGs were conducted using four databases (KEGG, Reactome, WikiPathways, and BioCarta), with the results being compiled in the form of histograms.
Protein-Protein Interaction Network Analysis
The STRING (https://string-db.org/) database was used to construct a PPI network incorporating the identified mutual DEGs, with interactions exhibiting a combined score >0.5 being incorporated into the generated network. The open-source Cytoscape (v 3.7.2) platform was used to construct and visualize the resultant network.
Hub Gene Identification
The Cytohubba plugin for Cytoscape was used to extract network features using an MMC (Maximal Clique Centrality) approach, with the top 10 genes identified within the PPI network being selected as hub genes for further analysis.
Retrieval of Transcription Factors and miRNAs That Interact With Mutual DEGs
Transcription factors regulate the expression of specific target genes, and identifying shared transcriptional regulators of particular genes of interest can thus offer valuable molecular insight. The web-based NetworkAnalyst (http://www.networkanalyst.ca) tool was used with the JASPAR database to identify transcription factors with the potential to bind to the shared DEGs associated with OP and COVID-19 in the present study. In addition, miRNAs with the potential to regulate these DEGs were identified through the use of the Tarbase and mirTarbase databases. Cytoscape 3.7.2 was then used to visualize the identified transcription factor/gene and miRNA/gene interaction networks produced through these analyses.
Exploration of Potential Therapeutic Drugs and Gene-Disease Associations
The gene set enrichment analysis-based DSigDB database was used with EnrichR to conduct a protein/drug interaction analysis with the goal of identifying pharmacological compounds with the potential to regulate these target DEGs. In total, DSigDB incorporates 22,527 gene sets and 17,389 unique compounds associated with 19,531 genes. In addition, DEG/disease relationships were assessed with DisGeNTET via NetworkAnalyst.
Data Availability Statement
Publicly available datasets were analyzed in this study. This data can be found here: GEO.
Author Contributions
HZ conceived and designed the study; XK and XW performed bioinformatics analysis; JL and LL analyzed the data; YZ and QW provided advice and technical assistance; XK and XW wrote the manuscript. All authors approved the final manuscript.
Conflict of Interest
The authors declare that the research was conducted in the absence of any commercial or financial relationships that could be construed as a potential conflict of interest.
Publisher’s Note
All claims expressed in this article are solely those of the authors and do not necessarily represent those of their affiliated organizations, or those of the publisher, the editors and the reviewers. Any product that may be evaluated in this article, or claim that may be made by its manufacturer, is not guaranteed or endorsed by the publisher.
Acknowledgments
The authors would like to thank all the reviewers who participated in the review and MJEditor (www.mjeditor.com) for its linguistic assistance during the preparation of this manuscript.
References
Cai, Q., Yang, M., Liu, D., Chen, J., Shu, D., Xia, J., et al. (2020). Experimental Treatment with Favipiravir for COVID-19: An Open-Label Control Study. Engineering 6 (10), 1192–1198. doi:10.1016/j.eng.2020.03.007
Cao, X., Liang, Y., Liu, R., Zao, X., Zhang, J., Chen, G., et al. (2022). Uncovering the Pharmacological Mechanisms of Gexia-Zhuyu Formula (GXZY) in Treating Liver Cirrhosis by an Integrative Pharmacology Strategy. Front. Pharmacol. 13, 793888. doi:10.3389/fphar.2022.793888
Casciola-Rosen, L., Thiemann, D. R., Andrade, F., Trejo-Zambrano, M. I., Leonard, E. K., Spangler, J. B., et al. (2022). IgM Anti-ACE2 Autoantibodies in Severe COVID-19 Activate Complement and Perturb Vascular Endothelial Function. JCI Insight. doi:10.1172/jci.insight.158362
Chen, X., Zhang, J., Liu, P., Wei, Y., Wang, X. e., Xiao, J., et al. (2021). Proteolytic Processing of Secretory Pathway Kinase Fam20C by Site-1 Protease Promotes Biomineralization. Proc. Natl. Acad. Sci. U.S.A. 118 (32). e2100133118. doi:10.1073/pnas.2100133118
Chen, Z., Azman, A. S., Chen, X., Zou, J., Tian, Y., Sun, R., et al. (2022). Global Landscape of SARS-CoV-2 Genomic Surveillance and Data Sharing. Nat. Genet. 54, 499–507. doi:10.1038/s41588-022-01033-y
Kawagoe, Y., Kawashima, I., Sato, Y., Okamoto, N., Matsubara, K., and Kawamura, K. (2020). CXCL5‐CXCR2 Signaling Is a Senescence‐associated Secretory Phenotype in Preimplantation Embryos. Aging Cell 19 (10), e13240. doi:10.1111/acel.13240
Khokhar, M., Tomo, S., and Purohit, P. (2022). MicroRNAs Based Regulation of Cytokine Regulating Immune Expressed Genes and Their Transcription Factors in COVID-19. Meta Gene 31, 100990. doi:10.1016/j.mgene.2021.100990
Li, J., Zhao, M., Xiang, X., He, Q., and Gui, R. (2021). A Novel Biomimetic Nanomedicine System with Anti-inflammatory and Anti-osteoporosis Effects Improves the Therapy Efficacy of Steroid-Resistant Nephrotic Syndrome. J. Nanobiotechnol 19 (1), 417. doi:10.1186/s12951-021-01165-z
Mahmud, S. M. H., Al-Mustanjid, M., Akter, F., Rahman, M. S., Ahmed, K., Rahman, M. H., et al. (2021). Bioinformatics and System Biology Approach to Identify the Influences of SARS-CoV-2 Infections to Idiopathic Pulmonary Fibrosis and Chronic Obstructive Pulmonary Disease Patients. Brief. Bioinform 22 (5). bbab115. doi:10.1093/bib/bbab115
Mi, B., Xiong, Y., Zhang, C., Zhou, W., Chen, L., Cao, F., et al. (2021). SARS-CoV-2-induced Overexpression of miR-4485 Suppresses Osteogenic Differentiation and Impairs Fracture Healing. Int. J. Biol. Sci. 17 (5), 1277–1288. doi:10.7150/ijbs.56657
Nashiry, A., Sarmin Sumi, S., Islam, S., Quinn, J. M. W., and Moni, M. A. (2021). Bioinformatics and System Biology Approach to Identify the Influences of COVID-19 on Cardiovascular and Hypertensive Comorbidities. Brief. Bioinform 22 (2), 1387–1401. doi:10.1093/bib/bbaa426
Oppert, B., Muszewska, A., Steczkiewicz, K., Šatović-Vukšić, E., Plohl, M., Fabrick, J., et al. (2022). The Genome of Rhyzopertha dominica (Fab.) (Coleoptera: Bostrichidae): Adaptation for Success. Genes 13 (3), 446. doi:10.3390/genes13030446
Popescu, I., Snyder, M. E., Iasella, C. J., Hannan, S. J., Koshy, R., Burke, R., et al. (2022). CD4+ T Cell Dysfunction in Severe COVID-19 Disease Is TNFα/TNFRI-dependent. Am. J. Respir. Crit. Care Med. doi:10.1164/rccm.202111-2493OC
Salamanna, F., Maglio, M., Borsari, V., Landini, M. P., and Fini, M. (2021). Blood Factors as Biomarkers in Osteoporosis: Points from the COVID-19 Era. Trends Endocrinol. Metabolism 32 (9), 672–679. doi:10.1016/j.tem.2021.05.005
Salvio, G., Gianfelice, C., Firmani, F., Lunetti, S., Ferroni, R., Balercia, G., et al. (2022). Remote Management of Osteoporosis in the First Wave of the COVID-19 Pandemic. Arch. Osteoporos. 17 (1), 37. doi:10.1007/s11657-022-01069-x
Schleiss, M. R. (2021). Letermovir and HCT: Too Much of a Good Thing? Blood 138 (1), 1–2. doi:10.1182/blood.2021011459
Shapira, T., Monreal, I. A., Dion, S. P., Buchholz, D. W., Imbiakha, B., Olmstead, A. D., et al. (2022). A TMPRSS2 Inhibitor Acts as a Pan-SARS-CoV-2 Prophylactic and Therapeutic. Nature. doi:10.1038/s41586-022-04661-w
Sing, C. W., Kiel, D. P., Hubbard, R. B., Lau, W. C., Li, G. H., Kung, A. W., et al. (2020). Nitrogen‐Containing Bisphosphonates Are Associated with Reduced Risk of Pneumonia in Patients with Hip Fracture. J. Bone Min. Res. 35 (9), 1676–1684. doi:10.1002/jbmr.4030
Swets, M. C., Russell, C. D., Harrison, E. M., Docherty, A. B., Lone, N., Girvan, M., et al. (2022). SARS-CoV-2 Co-infection with Influenza Viruses, Respiratory Syncytial Virus, or Adenoviruses. Lancet. 399. 1463. doi:10.1016/S0140-6736(22)00383-X
Wang, Y., Song, H., Miao, Q., Wang, Y., Qi, J., Xu, X., et al. (2022). PLA2G6 Silencing Suppresses Melanoma Progression and Affects Ferroptosis Revealed by Quantitative Proteomics. Front. Oncol. 12, 819235. doi:10.3389/fonc.2022.819235
Xiong, Y., Chen, L., Lin, Z., Panayi, A. C., Mi, B., and Liu, G. (2020). Orthopaedic Guidelines for the COVID-19 Post-Outbreak Period. J. Bone Jt. Surg. Am. 102 (15), e87. doi:10.2106/JBJS.20.00773
Xu, W., Wu, W., Yang, S., Chen, T., Teng, X., Gao, D., et al. (2022). Risk of Osteoporosis and Fracture after Hysterectomies without Oophorectomies: a Systematic Review and Pooled Analysis. Osteoporos. Int. doi:10.1007/s00198-022-06383-1
Keywords: COVID-19, infection, biological interaction, bioinformatics, drug
Citation: Kang X, Wen X, Liang J, Liu L, Zhang Y, Wang Q and Zhao H (2022) The Biological Interaction of SARS-CoV-2 Infection and Osteoporosis: A Preliminary Study. Front. Cell Dev. Biol. 10:917907. doi: 10.3389/fcell.2022.917907
Received: 11 April 2022; Accepted: 27 April 2022;
Published: 11 May 2022.
Edited by:
Yuan Xiong, Huazhong University of Science and Technology, ChinaReviewed by:
Xiangyu Chu, Huazhong University of Science and Technology, ChinaRuiyin Zeng, Huazhong University of Science and Technology, China
Copyright © 2022 Kang, Wen, Liang, Liu, Zhang, Wang and Zhao. This is an open-access article distributed under the terms of the Creative Commons Attribution License (CC BY). The use, distribution or reproduction in other forums is permitted, provided the original author(s) and the copyright owner(s) are credited and that the original publication in this journal is cited, in accordance with accepted academic practice. No use, distribution or reproduction is permitted which does not comply with these terms.
*Correspondence: Hongmou Zhao, zhaohongmou@xiyi.edu.cn
†These authors have contributed equally to this work