Dynamics of Green and Blue Water Supply Stress Index Across Major Global Cropland Basins
- 1Arctic Slope Regional Corporation Federal Data Solutions, Contractor to the U.S. Geological Survey Earth Resources Observation and Science Center, Sioux Falls, SD, United States
- 2U.S. Geological Survey Earth Resources Observation and Science Center, North Central Climate Adaptation Science Center, Boulder, CO, United States
- 3Innovate! Inc., Contractor to the U.S. Geological Survey Earth Resources Observation and Science Center, Sioux Falls, SD, United States
Global food and water insecurity could be serious problems in the upcoming decades with growing demands from the increasing global population and more frequent effect of climatic extremes. As the available water resources are diminishing and facing continuous stress, it is crucial to monitor water demand and water availability to understand the associated water stresses. This study assessed the water stress by applying the water supply stress index (WaSSI) in relation to green (WaSSIG) and blue (WaSSIB) water resources across six major cropland basins including the Mississippi (North America), San Francisco (South America), Nile (Africa), Danube (Europe), Ganges-Brahmaputra (Asia), and Murray-Darling (Australia) for the past 17-years (2003–2019). The WaSSIG and WaSSIB results indicated that the Murray-Darling Basin experienced the most severe (maximum WaSSIG and WaSSIB anomalies) green and blue water stresses and the Mississippi Basin had the least. All basins had both green and blue water stresses for at least 35% (6 out of 17 years) of the study period. The interannual variations in green water stress were driven by both crop water demand and green water supply, whereas the blue water stress variations were primarily driven by blue water supply. The WaSSIG and WaSSIB provided a better understanding of water stress (blue or green) and their drivers (demand or supply driven) across cropland basins. This information can be useful for basin-specific resource mobilization and interventions to ensure food and water security.
Introduction
Water resources are critical for providing human needs of water, energy, and food, and preserving healthy ecosystems (Bhaduri et al., 2016; Vanham, 2016). Increasing water demands from the growing population and shifting lifestyles are increasing competition within and among water use sectors (Molden, 2007). Additionally, climate change is deteriorating water resources (Scanlon et al., 2007) and fueling more stress on water resources (Hanjra and Qureshi, 2010; Siegfried et al., 2012), resulting in conflicts during water shortages (Eriksen and Lind, 2009; Theisen et al., 2012; Tang et al., 2018). Water stress assessments at local to regional scale are increasingly crucial to understand the vulnerability and resiliency of water resources. In particular, assessing water stress associated with crop water use (or evapotranspiration) and its relationship with green water (precipitation) or blue water (surface water and groundwater) sources provides integrated information on food and water status.
Global water supply is declining but the water demand has tripled since the 1950s (Gleick, 2003). Approximately 1.4 billion people live in river basins where water use is larger than water recharge rates (UNDP, 2006) with the projection that more than half of the global population will live in areas that suffer water scarcity at least a month each year by 2050 (WWAP, 2018). Several river basins and regions with large cropland areas that are heavily dependent on blue water irrigation could face critical challenges for food and water security because irrigation is the first sector to lose water when the water scarcity increases (Molden, 2007). For example, the Ganges-Brahmaputra, the largest basin in Asia with ~32% of its total area as irrigated croplands (Thenkabail et al., 2016), may face reductions in blue water availability for irrigation due to increasing competition among the water use sectors (Flörke et al., 2018). The southern High Plains of the United States (U.S.), a heavy blue water-dependent irrigated region, is diminishing due to unsustainable groundwater withdrawal (Scanlon et al., 2012). The continuous depletion of groundwater level would result in more than a third of the region being unable to support irrigation within the next 30 years (Scanlon et al., 2012). With the continuous effects of frequent and severe droughts in agriculture and its projected effects in the future (Li et al., 2009; van Asten et al., 2011; Howitt et al., 2014), the irrigated croplands (~20% of the global cropland area) may undergo severe water stress due to blue water shortages and could cause significant reduction to food production (Siebert and Döll, 2010; Leng and Hall, 2019).
Conversely, rainfed agriculture (~80% of the global cropland) is dependent on green water, which contributes to more than half of the global food production (Rosegrant et al., 2002). Food production in some regions is completely dependent on green water-supplied rainfed agriculture such as in Sub-Saharan Africa (Alexandratos, 1995). River basins with large portion as rainfed croplands are also under food and water security threat due to climate change-induced variabilities in precipitation (Kang et al., 2009). For example, the largest basins in Europe—the Danube Basin has almost two-third (~64%) of its area as rainfed croplands and several sub-regions could face severe water stress due to the shortage of green water availability from the projected reduction in precipitation (Bisselink et al., 2018; ICPDR, 2018). For such green water-dependent cropland basins, indicators for monitoring the crop water use and green water availability provide useful information to detect the associated water stress. Similarly, for the basins with blue water-dependent cropland, indicators that monitor blue water use and availability are more useful than other water stress indicators for better decision making. Thus, the stress indicators that integrate the water use and water availability information relating to the type of water resources (i.e., blue and green) will help to better characterize water stresses.
Water stress at a basin scale is commonly quantified as a ratio of water demand (or water use) to water availability (Falkenmark et al., 2007; Sun et al., 2008; Richey et al., 2015). One of the several water stress indices to detect water stress at basin scale is the water supply stress index (WaSSI) (Sun et al., 2008), originally developed to simulate water stress based on water demand and water supply on an annual time-step (Sun et al., 2015). The WaSSI has been applied to model and predict water stress caused by increasing human population, land-use change, and climate change (Sun et al., 2008, 2015; Caldwell et al., 2012; Duan et al., 2019) across several basins worldwide (Ji et al., 2012; Eldardiry et al., 2016; McNulty et al., 2016; Tang et al., 2018; Zhang et al., 2018). However, only a few studies have applied the WaSSI to understand the contribution of different water use sectors to basin water stress. Averyt et al. (2013) assessed the contribution of different water use sectors across the 8-digit Hydrological Unit Code (HUC8) (Seaber et al., 1987) scale basins in the U.S. The study reported agriculture as the main sector contributing to water stress at about two-thirds of the water-stressed HUC8 basins in the U.S. Larger contributions to water stress from the agricultural sector were observed across HUC8 basins in the western U.S., where there are fewer surface water resources compared to the eastern U.S. (Averyt et al., 2013). Similar studies with further investigation of green and blue water use and availability across agricultural regions would help to understand the vulnerability and resiliency of those regions for food and water security.
This study aims to assess water stress of six major cropland-dominated basins from six continents (Mississippi in North America, Sao Francisco in South America, Danube in Europe, Nile in Africa, Ganges-Brahmaputra in Asia, and Murray-Darling in Australia) by applying the WaSSI and by associating the index with green (WaSSIG) and blue (WaSSIB) water resources. The WaSSIG and WaSSIB were generated for the time period from 2003 to 2019 to investigate the significance of green or blue water resources and associated water stresses. The water stress information with integration to green and blue water resources is useful for resource mobilization and interventions to improve and ensure food and water security for human use and the environment.
Materials and Methods
Study Site
The six major river basins from six continents were selected for this study (Figure 1). The selection of these basins was based on being one of the major basins in each continent that has large cropland areas facing challenges on water availability for food production. The basin sizes are between 52.0 × 104 km2 (Sao Francisco Basin in South America) and 344.7 × 104 km2 (Mississippi Basin in North America). These basins have croplands (rainfed and irrigated) varying between ~20% (Nile Basin in Africa) and ~72% (Danube Basin in Europe) of their basin area (Table 1). All basins have larger rainfed cropland areas than irrigated cropland areas except the Ganges-Brahmaputra in Asia, which has ~30% of basin area irrigated and ~22% rainfed. The study basins cover wide variations in climate from warm temperate humid in southeastern regions of the Mississippi Basin to arid desert in northern regions of the Nile Basin (Kottek et al., 2006). Among the basins, the Murray-Darling (Australia) had the lowest average annual precipitation of 436 mm/year and the Ganges-Brahmaputra had the highest average annual precipitation of 1,268 mm/year during the study period from 2003 to 2019 (Table 1).
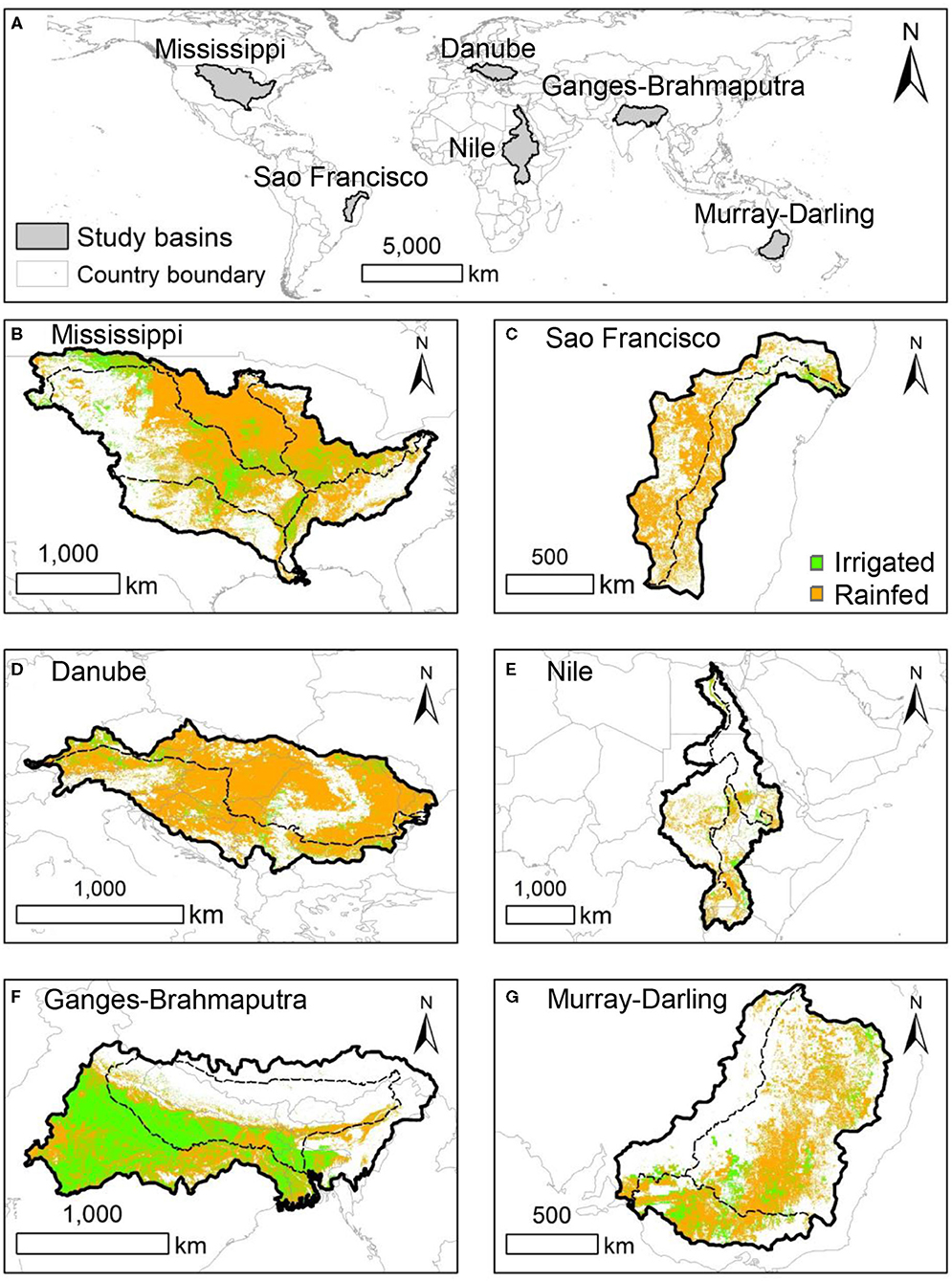
Figure 1. The global map showing the location of six study basins (A), including Mississippi (B), Sao Francisco (C), Danube (D), Nile (E), Ganges-Brahmaputra (F), and Murray-Darling (G). The dashed black color line inside the basins represents the major rivers. The croplands map is from the Global Food Security Support Analysis Data (https://lpdaac.usgs.gov/products/gfsad1kcmv001/) and basin boundaries are from World Resources Institute (https://www.wri.org/).
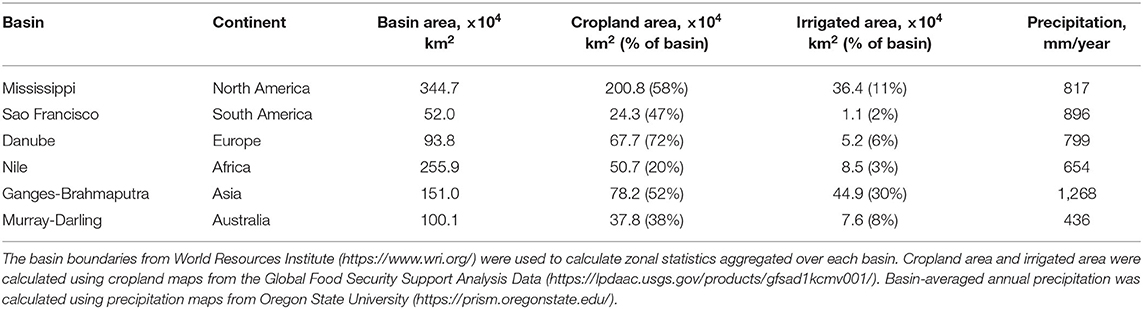
Table 1. Study basins with respective continents, basin area, cropland area, irrigated area, and average (2003–2019) annual precipitation.
Green and Blue WaSSI
Water demands from different water use sectors such as agriculture, industry, public supply, and others are primarily supplied by precipitation (green water) and surface water/groundwater (blue water). In this study, the water supply stress index (WaSSI), a ratio of water use to water available, was generated for croplands to evaluate the water stress associated with water use and water availability. The WaSSI was partitioned into green WaSSI (WaSSIG) and blue WaSSI (WaSSIB) based on the supply source for either green or blue water in the study basins. Because the green water is applied to all croplands regardless of rainfed or irrigated, all cropland area was used for computing WaSSIG. But this could create an exaggerated WaSSIG over irrigated areas because part of the crop water use (or actual evapotranspiration, ETa) is met by irrigation. However, due to the difficulty of quantifying the partial contributions of precipitation and irrigation to the index, the inter-basin differences are driven by the supply source (precipitation or runoff) to meet the ETa, representing the total crop water demand that is met. In all basins, the relative proportion of rainfed areas is much larger than irrigated area except for the Ganges-Brahmaputra Basin (Table 1), thus the basin-scale estimates are in proportion to the relative area under rainfed or irrigation for WaSSIG. For computing WaSSIB, only ETa from the irrigated cropland area was used as the blue water is supplied to irrigated croplands only. Similar to WaSSIG, the total ETa over the irrigated areas was used for WaSSIB, but the supply is attributed to the runoff instead of precipitation. The WaSSIG and WaSSIB for each basin and year were computed as:
where n is the number cropland pixels at each basin, and ETa is the actual evapotranspiration.
The water used by crops or actual evapotranspiration (ETa) is driven by crop water demand and availability of supply. Thus, ETa represents the crop water demand that was met by available water from green and blue water sources. The separation of ETa for representing green and blue water components requires running a water balance model (including additional data) which will introduce additional uncertainties. Thus, the total ETa (both green and blue water), estimated using a surface energy balance model (detailed in the following section Data Preparation), from the cropland and irrigated areas was assumed to represent the green and blue water demands to compute WaSSIG and WaSSIB, respectively. Precipitation only over the cropland area (not for basin) was considered as the available green water to compute WaSSIG. Not all precipitation is expected to have been available for crop water use due to various losses (e.g., canopy interception and runoff); however, to develop the index, total precipitation was used to minimize uncertainties associated to determining effective precipitation for soil moisture and ETa. The total runoff from the basin was assumed as the available blue water for irrigated area to compute WaSSIB. The use of runoff as blue water instead of surface water and groundwater is due to the limitation of obtaining reliable data at the study basins, specifically for the groundwater that is declining in many places, and quality data on remaining volume and drawdown rates are limited (Reilly et al., 2008). The use of runoff as the blue water availability assumes that there is a strong hydraulic linkage between surface water and groundwater at basin scales.
The evaluation of WaSSIG and WaSSIB was made at an annual scale. The values of the indices vary from zero to infinity. The values closer to zero indicate lower stress and the larger values indicate higher water stress. Previous studies (Ji et al., 2012; Averyt et al., 2013) have applied a threshold of one (1) to represent no-stress (<1) and stress (>1) including the water demand from several water use sectors. However, as this study was focused on the water demand for croplands, anomalies (deviation from the average value) of WaSSIG and WaSSIB were used to detect the severity (magnitude) and duration of green and blue water stresses. The WaSSIG and WaSSIB anomalies larger than their average (2003–2019) values were considered stress and the anomalies equal to or smaller than their average values were considered no-stress.
where i in WaSSIG, i and WaSSIB, i represents the study years from 2003 to 2019. The WaSSIG, avg. and WaSSIB, avg. are the basin average (2003–2019) WaSSIG and WaSSIB, respectively. The responses of WaSSIG and WaSSIB were also evaluated during dry (lowest basin precipitation) and wet (highest basin precipitation) years both at pixel [following Equations (1, 2) for each pixel] and basin scales.
Data Preparation
For generating the WaSSIG and WaSSIB, four primary datasets were used: actual evapotranspiration (ETa), runoff, precipitation, and land cover. The ETa data were generated from the Operational Simplified Surface Energy Balance (SSEBop) model (Senay et al., 2013; Senay, 2018) using the Moderate Resolution Imaging Spectroradiometer (MODIS) imagery and global gridded weather datasets. The SSEBop model uses the surface energy balance approach to estimate daily ETa for the satellite overpass dates. The MODIS-scale (daily, 1-km) ETa maps were summed to generate annual ETa maps for the study basins from 2003 to 2019. These ETa datasets were validated with 12 flux tower sites across the six continents. The data are applied by the U.S. Geological Survey (USGS) Famine Early Warning Systems Network (FEWS NET) for drought monitoring and early warning purposes (Senay et al., 2020). The ETa data are freely available for download from the USGS FEWS NET Data Portal (https://earlywarning.usgs.gov/fews).
Runoff datasets were generated using the VegET water balance model (Senay, 2008). The VegET is a root-zone water balance model driven by precipitation and remotely sensed land surface phenology (Senay, 2008). Modeled runoff was used instead of observed runoff due to limitation of complete runoff observations for the study basins during the study period (2003–2019). For the calibration purpose, in the first step, the modeled runoff was compared with an observation-based global gridded runoff (GRUN) dataset (Ghiggi et al., 2019). In the second step, when the modeled runoff data was not within ±10% of the GRUN runoff, the modeled runoff was adjusted (by percentage) with the global composite runoff data (Fekete et al., 2002) from the global runoff data center (GRDC). The GRUN runoff was applied as reference data to filter the basins for the calibration of modeled runoff with GRDC runoff. Except for the Ganges-Brahmaputra Basin, where the modeled runoff was within ±10% of GRUN runoff, modeled runoff for all other basins was adjusted with the GRDC data.
Precipitation data were obtained from the Climate Hazards Group Infrared Precipitation with Stations (CHIRPS; https://www.chc.ucsb.edu/data/chirps). The CHIRPS rainfall data (0.05° × 0.05° spatial resolution) are generated by integrating infrared imagery, climatology, and observed rainfall records, and have global coverage (50°S−50°N, 180°E−180°W) ranging from 1981 to near-present (Funk et al., 2015). These rainfall data have been applied to support the drought monitoring efforts by FEWS NET, especially in areas where observed rainfall data are sparse. The land cover map to distinguish irrigated and rainfed croplands was obtained from the Global Food Security Support Analysis Data Crop Mask Global 1-km dataset (GFSAD1KCM; https://lpdaac.usgs.gov/products/gfsad1kcmv001/). The GFSAD1KCM map provides irrigated (major and minor) and rainfed (rainfed, minor fragments, and very minor fragments) croplands for the nominal year of 2010 (Teluguntla et al., 2015). All other globally consistent irrigated and rainfed maps were limited for all study years; thus, the GFSAD1KCM map was applied in this study.
Results
Dynamics of Green and Blue WaSSI
The interannual variation of WaSSIG and WaSSIB of the six study basins is shown in Figure 2. Overall, WaSSIG was greater than WaSSIB for all basins except the Murray-Darling Basin indicating more water stresses associated with green water (precipitation) compared to blue water (runoff in this study). The average (2003–2019) WaSSIG values were less than one (1) for all basins (Table 1). The WaSSIG is the largest for Sao Francisco (0.91), followed by Nile (0.78), Mississippi (0.71), Murray-Darling (0.69), Ganges-Brahmaputra (0.69), and Danube (0.50) (Table 2). The larger interannual variation of WaSSIG (max. WaSSIG–min. WaSSIG) was observed for the Murray-Darling Basin (Figure 2), indicating vulnerability to green water stress compared to other basins. In contrast, the smallest interannual variation of WaSSIG was for the Mississippi Basin reflecting resiliency to green water stress.
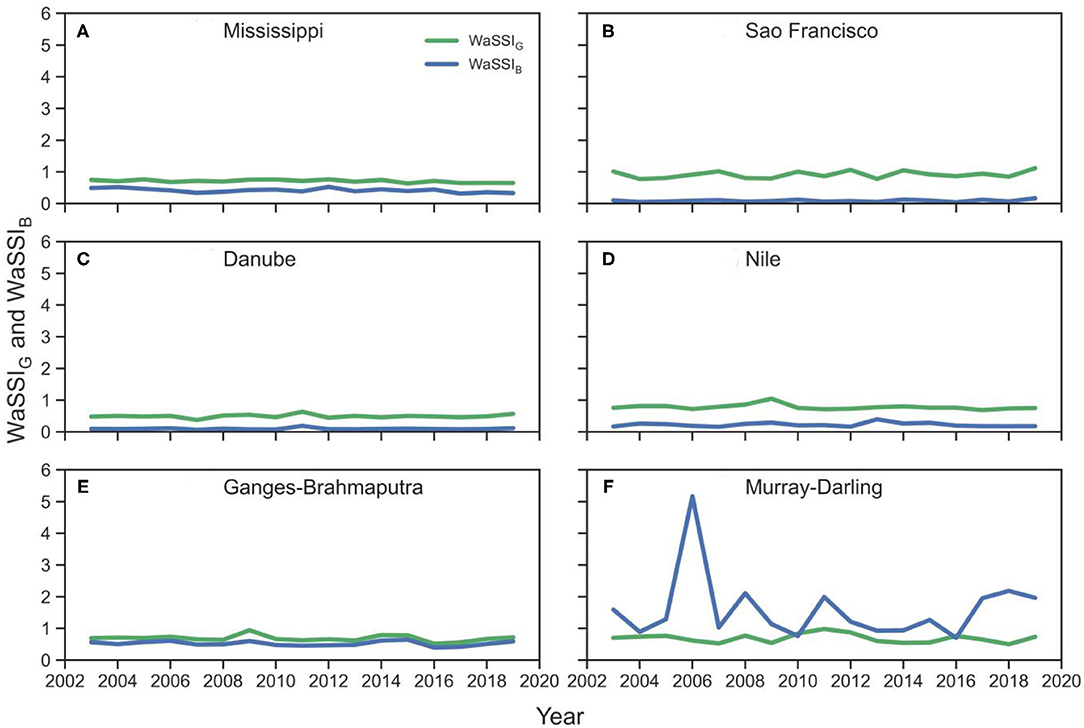
Figure 2. Interannual variations of green (WaSSIG) and blue (WaSSIB) water supply stress indices across study basins including Mississippi (A), Sao Francisco (B), Danube (C), Nile (D), Ganges-Brahmaputra (E), and Murray-Darling (F) from 2003 to 2019.
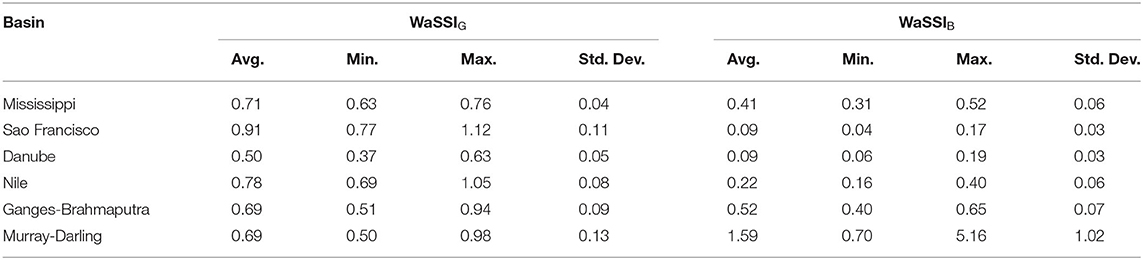
Table 2. The average, minimum, maximum, and standard deviation of green (WaSSIG) and blue (WaSSIB) water supply stress indices of study basins from 2003 to 2019.
Similar to the WaSSIG values, the average WaSSIB values were also less than one (1) for all basins with the exception for the Murray-Darling Basin (Table 1). The largest WaSSIB was for Murray-Darling (1.59), followed by Ganges-Brahmaputra (0.52), Mississippi (0.41), Nile (0.22), Danube (0.09), and Sao Francisco (0.09). The largest interannual variation of WaSSIB was at the Murray-Darling Basin. This basin also had the largest WaSSIG variation, indicating greater vulnerability to both green and blue water stresses compared to other study basins. The smallest variation of WaSSIB was at the Sao Francisco Basin showing more resilience to blue water stress.
The plots of WaSSIG and WaSSIB anomalies (percent deviation from the average value) show the blue and green water stress at study basins from 2003 to 2019 (Figure 3). In general, the WaSSIG anomalies were within ±43% of their 2003–2019 average values for all basins. In contrast, WaSSIB anomalies are relatively larger within ±103% of their average values except for a maximum of 224% for the year 2006, which was the driest year for the Murray-Darling Basin. During the study period, the most severe (maximum anomaly) green water stress was observed at the Murray-Darling Basin (average positive WaSSIG anomaly of +16%) and the least severe (minimum anomaly) green water stress at the Mississippi Basin (average positive WaSSIG anomaly of +5%). For the blue water stress, the most severe was also at the Murray-Darling Basin (+61%) and the least was at the Mississippi Basin (+12%). Thus, the most severe blue and green water stresses were observed at the Murray-Darling Basins and the least severe at the Mississippi Basin.
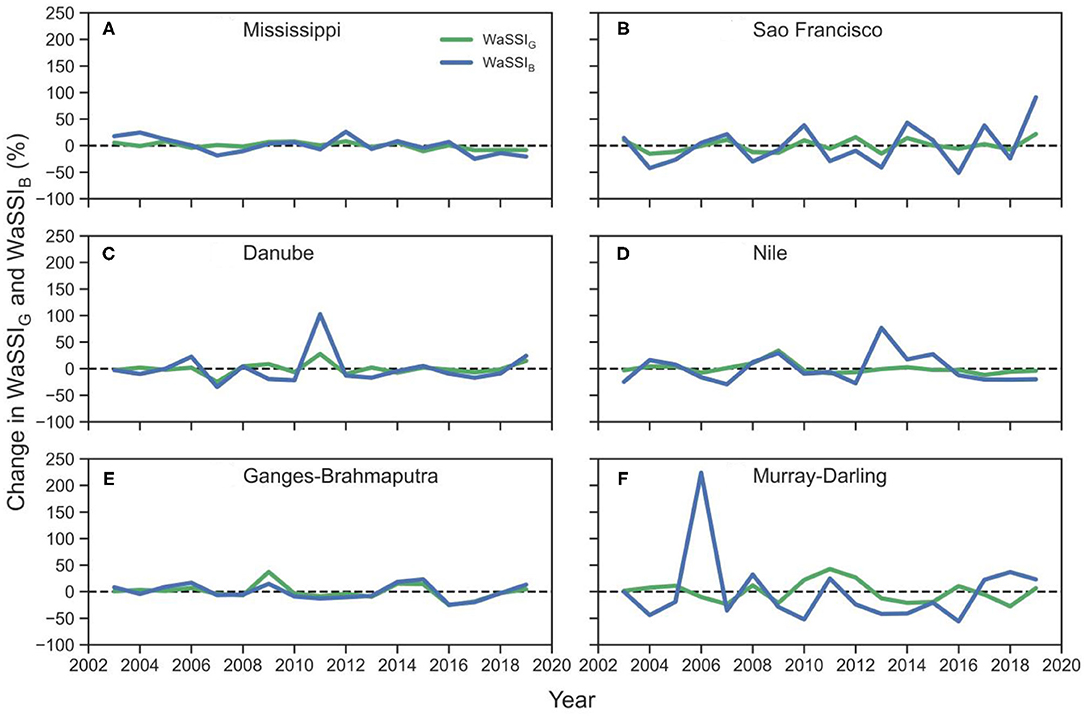
Figure 3. Anomalies (percent deviation from average value) of green (WaSSIG) and blue (WaSSIB) water supply stress indices across study basins including Mississippi (A), Sao Francisco (B), Danube (C), Nile (D), Ganges-Brahmaputra (E), and Murray-Darling (F) from 2003 to 2019.
During the 17-year study period, the WaSSIG and WaSSIB anomalies were greater than zero (0) for at least six (6) years and >5% for at least two (2) years for all basins. In other words, these basins faced both green and blue water stresses for at least 35% of the study years from 2003 to 2019. The maximum number of years with WaSSIB anomalies greater than zero was observed for the Mississippi (9 years) and Murray-Darling (9 years) basins. Similarly, the maximum number of years with WaSSIG anomalies greater than zero was observed at the Mississippi Basin (9 years). These results indicate that the Mississippi Basin had the longest period (>50% of study years) of both green and blue water stresses. However, this basin had the least severe blue and green water stresses compared to other basins (Figure 3).
Responses of Green and Blue WaSSI During Dry and Wet Years
The WaSSIG and WaSSIB showed green and blue water stresses during the dry years (Figure 4; Table 3). The intensity of green and blue water stresses during dry years varied within and across the study basins. For example, the western regions of the Mississippi Basin faced more green and blue stresses compared to the eastern regions of the basin (Figure 4) in the dry year of 2012. The central region of the Sao Francisco Basin had more stress than the northern or southern regions of the basin in the dry year 2012. Similarly, the central and eastern regions of Danube, southern region of the Nile Basin, central region of the Ganges-Brahmaputra Basin, and eastern region of the Murry-Darling Basin faced maximum stress during dry years (Figure 4). The spatially distributed maps of WaSSIG are visually clearer than WaSSIB maps showing several green water stress regions during the respective dry and wet years for all basins. In contrast, blue water stress indicated by WaSSIB maps for Sao Francisco, Danube, and Nile Basins is less evident due to the lower irrigated area (<6%) compared to other basins.
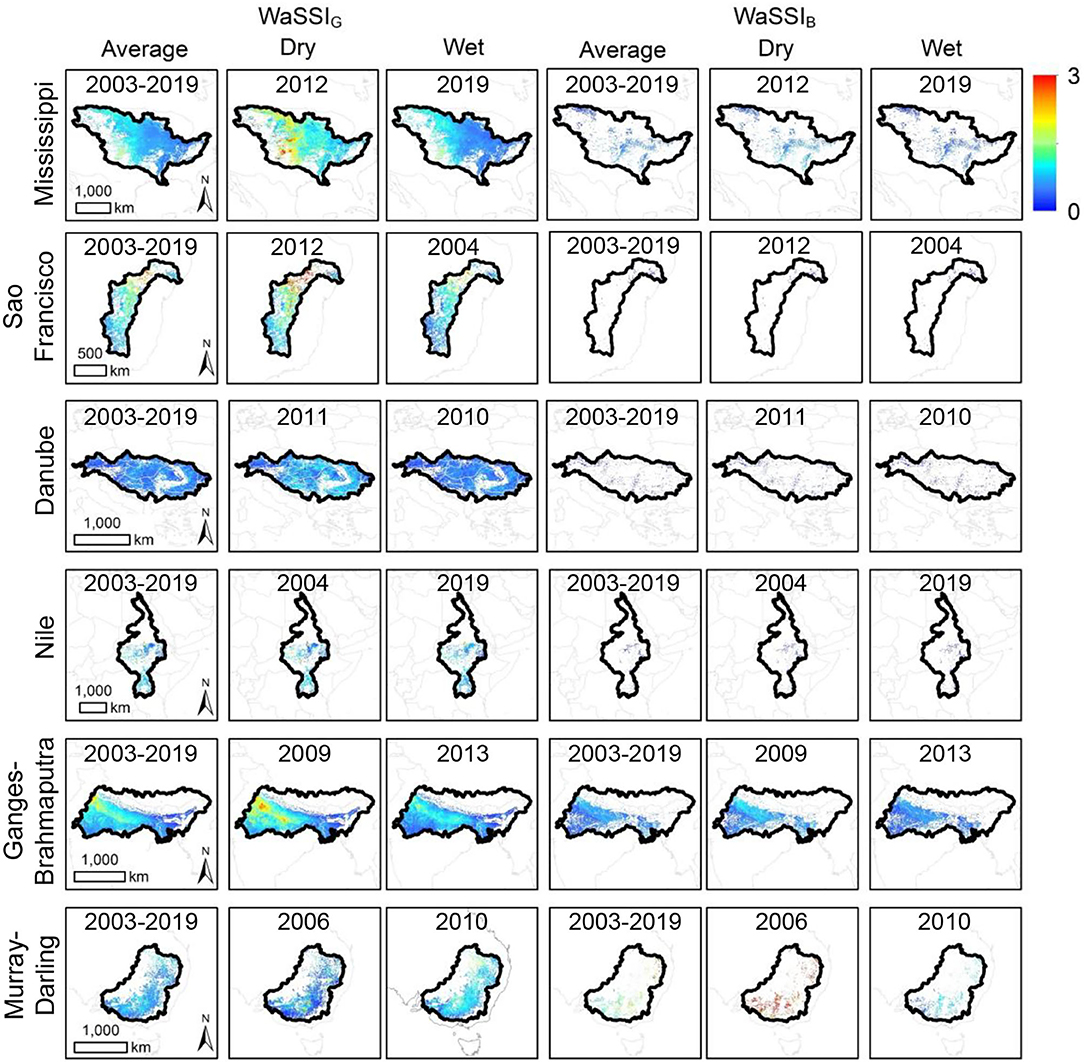
Figure 4. Spatial variation of green (WaSSIG) and blue (WaSSIB) water supply stress indices during dry- and wet-years across study basins.
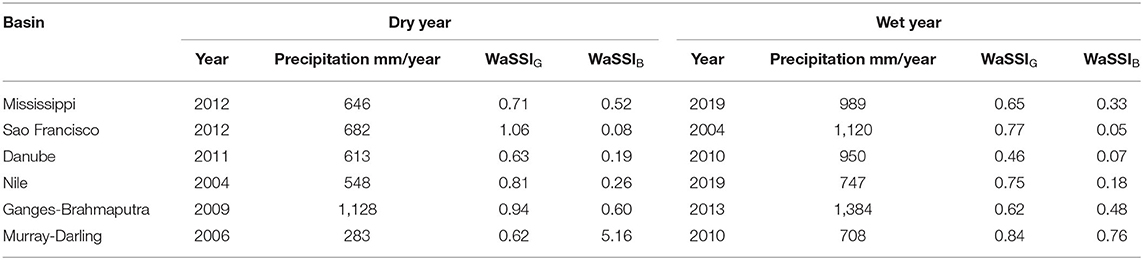
Table 3. Summary of green (WaSSIG) and blue (WaSSIB) water supply stress indices during dry and wet years across study basins.
During the dry years across all basins, the largest green water stress (28% above average) was observed at the Ganges-Brahmaputra Basin for the dry year 2009 when the basin precipitation was 11% below the average precipitation. Similarly, the largest blue water stress (224% above average) was at the Murray-Darling Basin for the dry year 2006 when the precipitation was 35% below average. For wet years, the least green water stress (15% below average) was at the Sao Francisco Basin when the precipitation was 24% above average and the least blue water stress (52% below average) was for the Murray-Darling Basin when the precipitation was 35% above average. These results show that WaSSIG and WaSSIB variations followed the trends of basin precipitation; however, the magnitudes of green and blue water stresses varied across and within basins. Thus, WaSSIG and WaSSIB indices can be applied for monitoring green and blue water stresses at basin scales. For basins with the potential spatial disconnect between the available water (runoff and precipitation) and the point of water use (irrigation), pixel-scale applications of WaSSIG and WaSSIB may benefit from additional in-situ information before decisions can be made.
Discussion
Drivers of Green and Blue Water Stresses
The interannual plots of WaSSIG and WaSSIB indicate the large variations of green and blue water stresses within and across the study basins (Figures 2, 3). These variations are primarily driven by either demand or supply of green and blue water at the basins. Based on the water demand and water available for crops applied in this study, the interannual variations of green water stresses were driven by both the demand (ETa) and supply (precipitation) for most of the basins. The differences of the percent coefficient of variation (CV) between the green water demand and the green water supply were within ±7% for all basins except for the Murray-Darling Basin (Supplementary Table 1). The Murray-Darling Basin is the basin with the largest interannual variations in green water stress (Figure 3). The basin had the percent CV of green water demand that was 16% greater than that of green water supply, indicating the larger influence of water demand for green water stress variations. For the blue water stress, the percent CV of blue water supply (runoff) was larger than the percent CV of demand (ETa) for all basins (Supplementary Table 1). The smallest difference between the percent CVs of water demand and supply was for the Ganges-Brahmaputra Basin (4% larger CV for supply) and the largest difference was for the Murry-Darling basin (29% larger CV for supply). Thus, the variation in blue water stress was driven more by water supply than by water demand.
The cropland area within a basin can affect green and blue water stresses. Basins with large cropland areas tend to have higher green and blue (when irrigated) water demands and therefore are more prone to water stress when the water supply is limited. Among the study basins, the Danube Basin had the largest cropland (~72%); however, the maximum magnitude of water stress (both green and blue water) was observed at the Murray Darling Basin. Similarly, the Nile Basin had the smallest cropland (~20%) but the minimum water stress magnitude was observed at the Mississippi Basin. Although the Mississippi Basin had the least water stress magnitude, this basin had the longest duration of both green and blue water stresses (9 out of 17 years). These results indicate the percent area of cropland is not the primary factor to drive green and blue water stresses (magnitude and duration) at these studied basins. However, the spatial distribution of cropland within the basins can affect the green and blue water stresses. For example, irrigated cropland with easy access to available water may not suffer more blue water stress during droughts compared to cropland that is far from the available water within a basin. Other factors such as climate, crop types (high or low water demand), infrastructure development for irrigation systems (high or low water use efficiency), and local and regional water management policies can influence variations in green and blue water stresses across the basins.
Limited studies have applied the WaSSI approach for assessing water stress across basins considered in this study. A study by Averyt et al. (2013) in the U.S. reported about 9% (193 out of 2103) of the HUC8 basins were stressed in 2013. The agricultural sector was the main contributor to the water stress, mostly in the lower Mississippi Basin and most of the western U.S. Although our study was further extended to link with green and blue water resources, the spatial distribution of water stress regions in the Mississippi Basin (Figure 4) is consistent with Averyt et al. (2013). Several other studies have accounted blue and green water availability and consumption (Schuol et al., 2008; Wada et al., 2011; Hoekstra et al., 2012), and the potential factors that can affect water availability for croplands (Rost et al., 2008; Liu and Yang, 2010). For example, Ferrarini et al. (2020) reported the potential expansion of irrigated areas in the upper and middle regions of Sao Francisco Basin due to water availability. Our study shows that the blue water stress at the Sao Francisco Basin is among the lowest (Table 2); however, expansion of irrigated areas may add stress on blue water resources in the basin. The larger blue water stress in the Ganges-Brahmaputra Basin and Murray-Darling Basins (Table 2) indicates the higher vulnerability of croplands in the basin to extreme water stress, especially during the dry seasons/years, that may lead to complete desiccation and substantial economic disruption (Hoekstra et al., 2012).
Model Parameter Estimation Uncertainties
Three primary parameters (precipitation, ETa, and runoff) were applied to assess green and blue water stresses across the study basins. The precipitation data were obtained from the gauge-adjusted CHIRPS datasets. The CHIRPS precipitation data have been widely validated across the globe (Paredes-Trejo et al., 2017; Prakash, 2019; Tarek et al., 2020). A recent global-scale evaluation of CHIRPS monthly precipitation from 2000 to 2016 with the Global Precipitation Climatology Center gauge-based precipitation data reported error (including random and bias components) within ±2.5% across Europe, Africa, Australia, United States, and South America (Shen et al., 2020). The Southeast China region had a relatively larger error at 5.6% (Shen et al., 2020); however, this region does not cover the large cropland areas included in this study. Additionally, these errors are at a monthly scale and the annual scale error is smaller at −0.06% (Shen et al., 2020).
ETa data were from the surface energy balance based SSEBop model. The SSEBop ETa products have been applied across different climates and land covers for monitoring water use from a field to regional scales (Singh et al., 2014; Alemayehu et al., 2017; da Motta Paca et al., 2019; Schauer and Senay, 2019). The continental-scale validated ETa maps across several land covers (Senay et al., 2020) were applied in this study which captured seasonal and interannual variations when compared with flux tower observations. For croplands, the SSEBop tends to underestimate ETa at a monthly scale in North America and Europe (up to −33%) compared to flux tower observations without energy balance closure (Senay et al., 2020). Considering the energy balance closure issue with flux towers, which often lag in the order of 20% (Wilson et al., 2002), errors from SSEBop ETa would be lower when aggregated to longer temporal scales such as monthly and annual scales (Senay et al., 2016). Further, green and blue water stresses in this study are evaluated at a basin-scale (unlike a few 100 m in the flux tower footprint) and therefore these ETa-related errors are expected to be lower for monitoring interannual water stress variations at basin scales.
Runoff estimations from the water balance VegET model were calibrated with the observation-based GRUN and the climatology runoff data from GRDC. Although the VegET model was calibrated when the differences in annual runoff values were >±10%, the runoff estimation uncertainty may still exist when applying the model for evaluating blue waters stress for basins with relatively low percent cover of irrigated croplands (for example, the Sao Francisco and Nile Basins). However, the evaluation of green and blue water stresses severity (magnitude) and duration with the baseline of 17-year (2003–2019) average values, rather than the absolute values of WaSSIG and WaSSIB for each year, would minimize the potential uncertainties from the model parameter estimations.
Advantages and Limitations of Partitioning Green and Blue Water Stresses
The idea of partitioning water consumed by crops into green and blue water sources is a fairly new approach (Falkenmark and Rockström, 2006; Rost et al., 2008; Liu and Yang, 2010; Velpuri and Senay, 2017). The assessment of green and blue water use at varying scales helps to identify the areas to efficiently manage and use the available water. This study demonstrated an approach for monitoring green and blue water stresses for croplands, which is directly associated with food and water status. The current study of green and blue water stresses across the six major cropland basins provides an insight on how green and blue water stresses vary (demand vs. supply driven) with time and space, and identified the basins that need to address the associated water stresses. For example, most basins had green water stress larger than the blue water stresses highlighting the uncertainty in food production as most of the global food production comes from green water-dependent rainfed systems. Similarly, the Murray-Darling Basin had the most severe (maximum WaSSIG and WaSSIB anomalies) green and blue water stress, which indicates that adoption of measures to improve the overall water use efficiency for sustaining food production may be beneficial. Proven methods and technologies can help to efficiently manage the available water. For example, Chukalla et al. (2015) reported that change in irrigation and mulching strategies in irrigated agriculture can reduce the green and blue water footprint up to 28%. Identification of regions or basins with green and blue water stresses could be useful for specific resource allocation and potential infrastructure development for improving green water and blue water use efficiency. Additionally, while blue water-based policies have been focused in the past (Sulser et al., 2010), the basin-specific green and blue water integrative plans and polices would help to minimize water stress and increase food production.
Besides the advantages of partitioning green and blue water resources, limitations exist when implementing the partitioning approach in this study. Our study is primarily focused on identifying an index to account for both green and blue water stress and their variations across time and space, rather than to accurately quantify green and blue water use as explored in previous studies (Siebert and Döll, 2010; Hoekstra, 2019). Thus, the equations used to compute WaSSIG and WaSSIB and assumptions made in this study must be considered before making decisions. For example, effective precipitation would have applied to compute WaSSIG for an ideal condition. However, due to additional data required for partitioning precipitation to canopy interception, runoff, soil moisture, and effective precipitation, and additional uncertainties associated with these estimations, total precipitation was applied to compute WaSSIG. The use of total precipitation may have exaggerated WaSSIG, especially for irrigated areas due to the contribution of blue water and reached the basin-scale WaSSIG more than one (1). Basin-scale WaSSIG values were less than 1 for most basins but a year (2009) in the Nile Basin and 6 years (2003, 2007, 2010, 2012, 2014, and 2019) in the Sao Francisco Basin. The frequent WaSSIG values of more than 1 in the Sao Francisco Basin may indicate a larger contribution of blue water across irrigated croplands. For computing WaSSIB, reliable groundwater data was not available. For this reason, surface runoff was considered available blue water with the assumption of interconnections between the surface water and groundwater at a basin-scale analysis. This assumption may add bias to the blue water stress estimations. For example, WaSSIB for the dry year 2006 in the Murray-Darling Basin was relatively high, compared to other years (Figure 2), primarily due to a substantial reduction (87% below average) in the modeled runoff. The exclusion of groundwater may have exaggerated the blue water stress for the dry year in this basin. However, the calculated blue water stress anomalies are based on the 17-year (2003–2019) average, and therefore, the biases on the anomalies were not influenced primarily by the unavailability of groundwater data. The green and blue water stress anomalies are presented at an annual scale to capture water used by all crops including main crops grown during the growing season and other crops (e.g., cover crops, secondary crops) grown during the non-growing season. Thus, the annual scale analysis does not reflect monthly or seasonal stresses. The potential water transfer (blue water) from a year to the following year that is stored in reservoirs is not accounted for by WaSSI indices. Another limitation included the unavailability of consistent land cover data for all years during the study period. Therefore, this study used the cropland data for the nominal year 2010 based on several studies from 2007 to 2012 (Thenkabail et al., 2016). The cropland area may have changed in the other years, which could change the water stresses. However, the water stresses are generalized at a basin-scale and may have minimal effects on the overall outcome of this study. Additionally, previous studies have suggested refinement of green and blue water estimation in croplands such as by computing soil water balance components (Siebert and Döll, 2010), which could improve the spatiotemporal accuracy and further enhancement of similar green and blue water stress indices. With the availability of consistent finer scale land cover data, groundwater data, and associated dataset, the WaSSIG and WaSSIB indices can be improved and implemented for monitoring water stress and have potential applications for creating and implementing basin-specific adaptive decision support systems to ensure food and water security.
Conclusion
An approach to assess the water stress across croplands based on green and blue water resources is demonstrated by applying the water supply stress index across six large cropland basins across the globe. The results from the 17-year (2003–2019) study show that all basins had both green and blue water stresses for at least 35% (6 out of 17 years) of the study period. The most severe (maximum WaSSIG and WaSSIB anomalies) green and blue water stresses were observed for the Murray-Darling Basin in Australia and the least severe (minimum WaSSIG and WaSSIB anomalies) stress for the Mississippi Basin in North America. The interannual variations in green water stress were driven by both crop water demand and green water supply, whereas the blue water stress variations were primarily driven by blue water supply. This study identified the basins and regions that may benefit from basin-specific adaptive measures and policies for the efficient use and management of available water. Similar studies can be implemented to monitor the green and blue water stresses at varying scales for developing decision support systems to ensure food and water security.
Data Availability Statement
The datasets presented in this study can be found in online repositories. The names of the repository/repositories are provided in the methods section of the article.
Author Contributions
GS and KK: conceptualization. KK, GS, SK, and GP: methodology, formal analysis, and writing—review and editing. SK and GP: software. KK: writing—original draft preparation. GS: project administration. All authors contributed to the article and approved the submitted version.
Funding
This research was funded by the USGS Land Change Science program.
Conflict of Interest
KK and SK were employed by company ASRC Federal Data Solutions. GP was employed by Innovate! Inc. The affiliations listed as Innovate! Inc. and ASRC Federal Data Solutions are contractors for the USGS EROS Center.
The remaining authors declare that the research was conducted in the absence of any commercial or financial relationships that could be construed as a potential conflict of interest.
Publisher's Note
All claims expressed in this article are solely those of the authors and do not necessarily represent those of their affiliated organizations, or those of the publisher, the editors and the reviewers. Any product that may be evaluated in this article, or claim that may be made by its manufacturer, is not guaranteed or endorsed by the publisher.
Acknowledgments
The authors would like to acknowledge the USGS Land Change Science program and FEWS NET for supporting remote sensing-based ET research and applications. Any use of trade, firm, or product names is for descriptive purposes only and does not imply endorsement by the U.S. Government.
Supplementary Material
The Supplementary Material for this article can be found online at: https://www.frontiersin.org/articles/10.3389/fclim.2021.663444/full#supplementary-material
References
Alemayehu, T., Griensven, A. V., Senay, G. B., and Bauwens, W. (2017). Evapotranspiration mapping in a heterogeneous landscape using remote sensing and global weather datasets: application to the Mara Basin, East Africa. Remote Sens. 9:390. doi: 10.3390/rs9040390
Averyt, K., Meldrum, J., Caldwell, P., Sun, G., McNulty, S., Huber-Lee, A., et al. (2013). Sectoral contributions to surface water stress in the coterminous United States. Environ. Res. Lett. 8:035046. doi: 10.1088/1748-9326/8/3/035046
Bhaduri, A., Bogardi, J., Siddiqi, A., Voigt, H., Vörösmarty, C., Pahl-Wostl, C., et al. (2016). Achieving sustainable development goals from a water perspective. Front. Environ. Sci. 4:64. doi: 10.3389/fenvs.2016.00064
Bisselink, B., De Roo, A., Bernhard, J., and Gelati, E. (2018). Future projections of water scarcity in the Danube River Basin due to land use, water demand and climate change. J. Environ. Geogr. 11, 25–36. doi: 10.2478/jengeo-2018-0010
Caldwell, P. V., Sun, G., McNulty, S. G., Cohen, E. C., and Myers, J. A. M. (2012). Impacts of impervious cover, water withdrawals, and climate change on river flows in the conterminous US. Hydrol. Earth Syst. Sci. 16, 2839–2857. doi: 10.5194/hess-16-2839-2012
Chukalla, A. D., Krol, M. S., and Hoekstra, A. Y. (2015). Green and blue water footprint reduction in irrigated agriculture: effect of irrigation techniques, irrigation strategies and mulching. Hydrol. Earth Syst. Sci. 19, 4877–4891. doi: 10.5194/hess-19-4877-2015
da Motta Paca, V. H., Espinoza-Dávalos, G. E., Hessels, T. M., Moreira, D. M., Comair, G. F., and Bastiaanssen, W. G. (2019). The spatial variability of actual evapotranspiration across the Amazon River Basin based on remote sensing products validated with flux towers. Ecol. Proces. 8, 1–20. doi: 10.1186/s13717-019-0158-8
Duan, K., Caldwell, P. V., Sun, G., McNulty, S. G., Zhang, Y., Shuster, E., et al. (2019). Understanding the role of regional water connectivity in mitigating climate change impacts on surface water supply stress in the United States. J. Hydrol. 570, 80–95. doi: 10.1016/j.jhydrol.2019.01.011
Eldardiry, H., Habib, E. H., and Borrok, D. M. (2016). Small-scale catchment analysis of water stress in wet regions of the US: an example from Louisiana. Environ. Res. Lett. 11:124031. doi: 10.1088/1748-9326/aa51dc
Eriksen, S., and Lind, J. (2009). Adaptation as a political process: adjusting to drought and conflict in Kenya's drylands. Environ. Manage. 43, 817–835. doi: 10.1007/s00267-008-9189-0
Falkenmark, M., Berntell, A., Jägerskog, A., Lundqvist, J., Matz, M., and Tropp, H. (2007). On the Verge of a New Water Scarcity: A Call for Good Governance and Hyman Ingenuity. Stockholm: Stockholm International Water Institute.
Falkenmark, M., and Rockström, J. (2006). The new blue and green water paradigm: breaking new ground for water resources planning and management. J. Water Resour. Plann. Manag. 132, 129–132. doi: 10.1061/(ASCE)0733-9496(2006)132:3(129)
Fekete, B. M., Vörösmarty, C. J., and Grabs, W. (2002). High-resolution fields of global runoff combining observed river discharge and simulated water balances. Global Biogeochem. Cycles 16, 15–11. doi: 10.1029/1999GB001254
Ferrarini, A. D. S. F., Ferreira Filho, J. B. D. S., Cuadra, S. V., and Victoria, D. D. C. (2020). Water demand prospects for irrigation in the São Francisco River: Brazilian public policy. Water Policy 22, 449–467. doi: 10.2166/wp.2020.215
Flörke, M., Schneider, C., and McDonald, R. I. (2018). Water competition between cities and agriculture driven by climate change and urban growth. Nat. Sustain. 1, 51–58. doi: 10.1038/s41893-017-0006-8
Funk, C., Peterson, P., Landsfeld, M., Pedreros, D., Verdin, J., Shukla, S., et al. (2015). The climate hazards infrared precipitation with stations—a new environmental record for monitoring extremes. Sci. Data 2, 1–21. doi: 10.1038/sdata.2015.66
Ghiggi, G., Humphrey, V., Seneviratne, S. I., and Gudmundsson, L. (2019). GRUN: an observation-based global gridded runoff dataset from 1902 to 2014. Earth Syst. Sci. Data 11, 1655–1674. doi: 10.5194/essd-11-1655-2019
Gleick, P. H. (2003). Water use. Annu. Rev. Environ. Resour. 28, 275–314. doi: 10.1146/annurev.energy.28.040202.122849
Hanjra, M. A., and Qureshi, M. E. (2010). Global water crisis and future food security in an era of climate change. Food Policy 35, 365–377. doi: 10.1016/j.foodpol.2010.05.006
Hoekstra, A. Y. (2019). Green-blue water accounting in a soil water balance. Adv. Water Resour. 129, 112–117. doi: 10.1016/j.advwatres.2019.05.012
Hoekstra, A. Y., Mekonnen, M. M., Chapagain, A. K., Mathews, R. E., and Richter, B. D. (2012). Global monthly water scarcity: blue water footprints versus blue water availability. PLoS ONE 7:e32688. doi: 10.1371/journal.pone.0032688
Howitt, R., Medellín-Azuara, J., Macewan, D., Lund, J. R., and Sumner, D. (2014). Economic Analysis of the 2014 Drought for California Agriculture. Davis, CA: Center for Watershed Sciences University of California. Available online at: https://watershed.ucdavis.edu/2014-497 drought-report (accessed March 19, 2021).
ICPDR (2018). Danube River Basin Climage Change Adaptation. Update of the Danube Study. International Commission for the Protection of the Danube River. Available online at: http://www.icpdr.org/main/resources/climate-change-adaptation-update-danube-study (accessed March 19, 2021).
Ji, Y., Chen, L., and Sun, R. (2012). Temporal and spatial variability of water supply stress in the Haihe River Basin, Northern China. J. Am. Water Resour. Assoc. 48, 999–1007. doi: 10.1111/j.1752-1688.2012.00671.x
Kang, Y., Khan, S., and Ma, X. (2009). Climate change impacts on crop yield, crop water productivity and food security–a review. Prog. Nat. Sci. 19, 1665–1674. doi: 10.1016/j.pnsc.2009.08.001
Kottek, M., Grieser, J., Beck, C., Rudolf, B., and Rubel, F. (2006). World map of the Köppen-Geiger climate classification updated. Meteorol. Zeitschrift 15, 259–263. doi: 10.1127/0941-2948/2006/0130
Leng, G., and Hall, J. (2019). Crop yield sensitivity of global major agricultural countries to droughts and the projected changes in the future. Sci. Total Environ. 654, 811–821. doi: 10.1016/j.scitotenv.2018.10.434
Li, Y., Ye, W., Wang, M., and Yan, X. (2009). Climate change and drought: a risk assessment of crop-yield impacts. Clim. Res. 39, 31–46. doi: 10.3354/cr00797
Liu, J., and Yang, H. (2010). Spatially explicit assessment of global consumptive water uses in cropland: green and blue water. J. Hydrol. 384, 187–197. doi: 10.1016/j.jhydrol.2009.11.024
McNulty, S., Mack, E. C., Sun, G., and Caldwell, P. (2016). “Hydrologic modeling for water resource assessment in a developing country: the Rwanda case study,” in Forest and the Water Cycle: Quantity, Quality, Management, eds P. Lachassagne and M. Lafforgue (Newcastle upon Tyne: Cambridge Scholars Publishing), 198–203.
Molden, D. (2007). Water responses to urbanization. Paddy Water Environ. 5, 207–209. doi: 10.1007/s10333-007-0084-8
Paredes-Trejo, F. J., Barbosa, H., and Kumar, T. L. (2017). Validating CHIRPS-based satellite precipitation estimates in Northeast Brazil. J. Arid Environ. 139, 26–40. doi: 10.1016/j.jaridenv.2016.12.009
Prakash, S. (2019). Performance assessment of CHIRPS, MSWEP, SM2RAIN-CCI, and TMPA precipitation products across India. J. Hydrol. 571, 50–59. doi: 10.1016/j.jhydrol.2019.01.036
Reilly, T. E., Dennehy, K. F., Alley, W. M., and Cunningham, W. L. (2008). Ground-Water Availability in the United States. Reston, VA: U.S. Geological Survey.
Richey, A. S., Thomas, B. F., Lo, M. H., Reager, J. T., Famiglietti, J. S., Voss, K., et al. (2015). Quantifying renewable groundwater stress with GRACE. Water Resour. Res. 51, 5217–5238. doi: 10.1002/2015WR017349
Rosegrant, M. W., Cai, X., Cline, S. A., and Nakagawa, N. (2002). The Role of Rainfed Agriculture in the Future of Global Food Production. Washington, DC: Food Policy Research Institute.
Rost, S., Gerten, D., Bondeau, A., Lucht, W., Rohwer, J., and Schaphoff, S. (2008). Agricultural green and blue water consumption and its influence on the global water system. Water Resour. Res. 44:W09405. doi: 10.1029/2007WR006331
Scanlon, B. R., Faunt, C. C., Longuevergne, L., Reedy, R. C., Alley, W. M., McGuire, V. L., et al. (2012). Groundwater depletion and sustainability of irrigation in the US High Plains and Central Valley. Proc. Natl. Acad. Sci. U.S.A. 109, 9320–9325. doi: 10.1073/pnas.1200311109
Scanlon, B. R., Jolly, I., Sophocleous, M., and Zhang, L. (2007). Global impacts of conversions from natural to agricultural ecosystems on water resources: quantity versus quality. Water Resour. Res. 43:W03437. doi: 10.1029/2006WR005486
Schauer, M., and Senay, G. B. (2019). Characterizing crop water use dynamics in the central valley of california using landsat-derived evapotranspiration. Remote Sens. 11:1782. doi: 10.3390/rs11151782
Schuol, J., Abbaspour, K. C., Yang, H., Srinivasan, R., and Zehnder, A. J. (2008). Modeling blue and green water availability in Africa. Water Resour. Res. 44:W07406. doi: 10.1029/2007WR006609
Seaber, P. R., Kapinos, F. P., and Knapp, G. L. (1987). “Hydrologic Unit Maps”. U. S. Geological Survey Water Supply Paper 229. Reston, VA: U.S. Geological Survey.
Senay, G. B. (2008). Modeling landscape evapotranspiration by integrating land surface phenology and a water balance algorithm. Algorithms 1, 52–68. doi: 10.3390/a1020052
Senay, G. B. (2018). Satellite psychrometric formulation of the operational Simplified Surface Energy Balance (SSEBop) model for quantifying and mapping evapotranspiration. Appl. Eng. Agirc. 43, 555–566. doi: 10.13031/aea.12614
Senay, G. B., Bohms, S., Singh, R. K., Gowda, P. H., Velpuri, N. M., Alemu, H., et al. (2013). Operational evapotranspiration mapping using remote sensing and weather datasets: a new parameterization for the SSEB approach. JAWRA J. Am. Water Resour. Assoc. 49, 577–591. doi: 10.1111/jawr.12057
Senay, G. B., Friedrichs, M., Singh, R. K., and Velpuri, N. M. (2016). Evaluating Landsat 8 evapotranspiration for water use mapping in the Colorado River Basin. Remote Sens. Environ. 185, 171–185. doi: 10.1016/j.rse.2015.12.043
Senay, G. B., Kagone, S., and Velpuri, N. M. (2020). Operational global actual evapotranspiration: development, evaluation, and dissemination. Sensors 20:1915. doi: 10.3390/s20071915
Shen, Z., Yong, B., Gourley, J. J., Qi, W., Lu, D., Liu, J., et al. (2020). Recent global performance of the Climate Hazards group Infrared Precipitation (CHIRP) with Stations (CHIRPS). J. Hydrol. 591:125284. doi: 10.1016/j.jhydrol.2020.125284
Siebert, S., and Döll, P. (2010). Quantifying blue and green virtual water contents in global crop production as well as potential production losses without irrigation. J. Hydrol. 384, 198–217. doi: 10.1016/j.jhydrol.2009.07.031
Siegfried, T., Bernauer, T., Guiennet, R., Sellars, S., Robertson, A. W., Mankin, J., et al. (2012). Will climate change exacerbate water stress in Central Asia? Clim. Change 112, 881–899. doi: 10.1007/s10584-011-0253-z
Singh, R., Senay, G., Velpuri, N., Bohms, S., Scott, R., and Verdin, J. (2014). Actual evapotranspiration (water use) assessment of the Colorado River Basin at the Landsat resolution using the Operational Simplified Surface Energy Balance Model. Remote Sens. 6, 233–256. doi: 10.3390/rs6010233
Sulser, T. B., Ringler, C., Zhu, T., Msangi, S., Bryan, E., and Rosegrant, M. W. (2010). Green and blue water accounting in the Ganges and Nile basins: implications for food and agricultural policy. J. Hydrol. 384, 276–291. doi: 10.1016/j.jhydrol.2009.10.003
Sun, G., McNulty, S. G., Moore Myers, J. A., and Cohen, E. C. (2008). Impacts of multiple stresses on water demand and supply across the Southeastern United States 1. JAWRA J. Am. Water Resour. Assoc. 44, 1441–1457. doi: 10.1111/j.1752-1688.2008.00250.x
Sun, S., Sun, G., Caldwell, P., McNulty, S. G., Cohen, E., Xiao, J., et al. (2015). Drought impacts on ecosystem functions of the US National Forests and Grasslands: part I evaluation of a water and carbon balance model. For. Ecol. Manage. 353, 260–268. doi: 10.1016/j.foreco.2015.03.054
Tang, L.-L., Cai, X.-B., Gong, W.-S., Lu, J.-Z., Chen, X.-L., Lei, Q., et al. (2018). Increased vegetation greenness aggravates water conflicts during lasting and intensifying drought in the Poyang Lake watershed, China. Forests 9:24. doi: 10.3390/f9010024
Tarek, M., Brissette, F. P., and Arsenault, R. (2020). Large-scale analysis of global gridded precipitation and temperature datasets for climate change impact studies. J. Hydrometeorol. 21, 2623–2640. doi: 10.1175/JHM-D-20-0100.1
Teluguntla, P., Thenkabail, P., Xiong, J., Gumma, M. K., Giri, C., Milesi, C., et al. (2015). Global Food Security Support Analysis Data (GFSAD) at Nominal 1 km (GCAD) Derived From Remote Sensing in Support of Food Security in the Twenty-First Century: Current Achievements and Future Possibilities. Boca Raton, FL: CRC Press.
Theisen, O. M., Holtermann, H., and Buhaug, H. (2012). Climate wars? assessing the claim that drought breeds conflict. Int. Secur. 36, 79–106. doi: 10.1162/ISEC_a_00065
Thenkabail, P., Knox, J., Ozdogan, M., Gumma, M., Congalton, R., Wu, Z., et al. (2016). NASA Making Earth System Data Records for Use in Research Environments (MEaSUREs) Global Food Security Support Analysis Data (GFSAD) CROP Dominance 2010 Global 1 km V001. Reston, VA: U.S. Geological Survey.
UNDP (2006). Beyond Scarcity: Power, Poverty, and the Global Water Crisis. London: Palgrave Macmillan.
van Asten, P. J., Fermont, A., and Taulya, G. (2011). Drought is a major yield loss factor for rainfed East African highland banana. Agric. Water Manag. 98, 541–552. doi: 10.1016/j.agwat.2010.10.005
Vanham, D. (2016). Does the water footprint concept provide relevant information to address the water–food–energy–ecosystem nexus? Ecosyst. Serv. 17, 298–307. doi: 10.1016/j.ecoser.2015.08.003
Velpuri, N. M., and Senay, G. B. (2017). Partitioning evapotranspiration into green and blue water sources in the conterminous United States. Sci. Rep. 7, 1–12. doi: 10.1038/s41598-017-06359-w
Wada, Y., Van Beek, L., and Bierkens, M. F. (2011). Modelling global water stress of the recent past: on the relative importance of trends in water demand and climate variability. Hydrol. Earth Syst. Sci. 15, 3785–3808. doi: 10.5194/hess-15-3785-2011
Wilson, K., Goldstein, A., Falge, E., Aubinet, M., Baldocchi, D., Berbigier, P., et al. (2002). Energy balance closure at FLUXNET sites. Agric. For. Meteorol. 113, 223–243. doi: 10.1016/S0168-1923(02)00109-0
WWAP (2018). The United Nations World Water Development Report 2018: Nature-Based Solutions for Water. Paris: UNESCO.
Keywords: evapotranspiration, green and blue water, water stress, drought, food security
Citation: Khand K, Senay GB, Kagone S and Parrish GEL (2021) Dynamics of Green and Blue Water Supply Stress Index Across Major Global Cropland Basins. Front. Clim. 3:663444. doi: 10.3389/fclim.2021.663444
Received: 02 February 2021; Accepted: 15 July 2021;
Published: 09 August 2021.
Edited by:
Andrew Hoell, Earth System Research Laboratory (NOAA), United StatesReviewed by:
Nicholas Christopher Mbangiwa, University of Botswana, BotswanaTamuka Magadzire, University of California Santa Barbara, United States
Copyright © 2021 Khand, Senay, Kagone and Parrish. This is an open-access article distributed under the terms of the Creative Commons Attribution License (CC BY). The use, distribution or reproduction in other forums is permitted, provided the original author(s) and the copyright owner(s) are credited and that the original publication in this journal is cited, in accordance with accepted academic practice. No use, distribution or reproduction is permitted which does not comply with these terms.
*Correspondence: Kul Khand, kkhand@contractor.usgs.gov