- 1CSIRO Oceans and Atmosphere, Hobart, TAS, Australia
- 2Centre for Marine Socioecology, University of Tasmania, Hobart, TAS, Australia
Coral reefs are extremely vulnerable to climate change and their recent degradation will continue unless we can instigate strong global climate action with effective regional interventions. Many types of intervention have been proposed and some aspects of their deployment are now being tested. However, their long-term efficacy under climate change can only be evaluated using complex biophysical models applied over a range of plausible socio-economic pathways. The associated uncertainties in climate trajectories, ecological responses, and the mitigating effects of interventions, necessitate the use of a risk-based approach to evaluating model results. I show that ensemble modeling can be used to develop rigorous risk assessments suitable for comparing intervention strategies. A major strength of this approach is that all the key elements required for risk assessment (exposure, sensitivity, adaptive capacity, and impacts) can be generated by the model in a dynamically consistent form. This is a major advance on semi-quantitative climate change vulnerability risk assessments that estimate these quantities independently and then combine them under additional assumptions. Applying ensemble modeling risk assessment to the Great Barrier Reef (GBR) suggests that regional intervention strategies, such as solar radiation management (SRM) and control of coral predators, can slow the increase in risk and potentially avoid extreme risks predicted for the second half of the century. Model results further suggest that deployments focused within the northern and central GBR will be most effective due to underlying patterns of reef connectivity.
Introduction
Most ecological systems are multi-dimensional, non-linear, and non-stationary, with potential to undergo major regime shifts (Schaffer and Kot, 1986; Hagstrom and Levin, 2017). Under these circumstances statistical inferences based on historical data are unreliable over multi-generational timescales. Climate impact projections are therefore usually based on dynamical models, which themselves have large structural and parameter uncertainties associated with both climate forcing (Zhang and Chen, 2021) and associated ecological responses (Fulton et al., 2003). Many of these uncertainties are essentially irreducible over the timeframes still available for making critical management decisions (<10 years) and therefore must be incorporated into the decision-making process itself (Lewandowsky et al., 2015; Adger et al., 2018). While this points to the need for a risk-based approach to managing climate change, significant methodological challenges remain (Jurgilevich et al., 2017; Weaver et al., 2017; Sutton, 2019; Viner et al., 2020).
Dynamical ensemble modeling (whereby models are run many times to encompass model structures and parameter values that are consistent with current process understanding and empirical data) is the only method currently available to estimate the uncertainties associated with climate projections. Results from ensemble global climate models have traditionally been reported as trajectories of key indicators with associated confidence intervals, and a similar approach has been adopted across many sectors to evaluate the efficacy of intervention and adaptation strategies (Logan et al., 2014; van Hooidonk et al., 2016; Condie et al., 2021). While this approach supports many of the needs of climate scientists, managers, and policy-makers often have different requirements focused more on understanding how intervention options reduce risks to managed assets (Sutton, 2019; Kause et al., 2020).
The Great Barrier Reef (GBR) is one of the world's most vulnerable regional ecosystems (Lenton et al., 2019), with the increasing frequency of mass bleaching events under climate change (De'ath et al., 2012; Hughes et al., 2017) combining with the impacts of tropical cyclones and outbreaks of the coral-eating crown-of-thorns starfish (CoTS) (Pratchett et al., 2014) to degrade reef health and reduce associated ecosystem services (Cinner et al., 2018; Stoeckl et al., 2021). Intervention and adaptation measures aimed at preserving the GBR will require major investment decisions that need to be based on rigorous assessments of risk. We have used modeled climate projections for the health of the GBR to demonstrate the translation of ensemble scenario results into risk assessments in the form of probability distributions, quantitative risk matrices, and risk trajectories, that can be readily utilized by managers and policy makers. This approach effectively translates climate model outputs into a form that can be used within risk assessment procedures currently used to manage the GBR (GBRMPA, 2017).
Methods
Reef ecosystem projections
Risks of coral loss on the GBR were estimated using previously reported reef ecosystem model projections generated using the Coral Community Network (CoCoNet) model (Condie et al., 2018, 2021). While other modeled reef health indicators (e.g., coral diversity) could have been assessed, most indicators tended to be highly correlated at the GBR-scale and available data allowed coral cover to be most directly validated (Condie et al., 2021).
Details of the CoCoNet model have been reported previously (Condie et al., 2021), including its key assumptions (their Table 2), equations (their Table 3), and parameter values (their Tables 4 and 5). Briefly, it is a meta-community model that includes communities of corals and age-structured populations of their main predator, the CoTS. These predator-prey communities were distributed across a network of 3,753 reefs, with each reef resolved down to the scale of 8 ha sites. Reefs were exposed to environmental forcing in the forms of tropical cyclones, impacts from flood plumes, heat waves, and ocean acidification, with cumulative influences on coral growth and coral mortality. For example, tropical cyclones increased coral mortality over the impact area through wave damage and heatwaves increased coral mortality over the impact area through bleaching processes.
CoCoNet has been calibrated for coral cover and CoTS densities against the Australian Institute of Marine Science (AIMS) Long Term Monitoring Program (LTMP) dataset (Sweatman et al., 2011) at both the individual reef scale (Morello et al., 2014; Plaganyi et al., 2014) and reef network scale (Condie et al., 2018, 2021). It has successfully reproduced historical trajectories of coral cover and CoTS outbreak densities (Condie et al., 2021), as well as emergent system responses such as CoTS outbreaks and coral recovery at close to their observed periodicity (Condie et al., 2018).
Future projections were generated using a combination of estimated environmental exposure (van Hooidonk et al., 2016) and ecological sensitivities based on empirical data (Condie et al., 2018, 2021). The most important environmental changes were associated with marine heat waves, characterized by persistent high sea surface temperatures often extending over scales of hundreds of kilometers. The strength of these events can be characterized by the cumulative heating in units of degree heating weeks (DHWs), where one DHW is equivalent to 1 week of sea surface temperature 1°C above the maximum of the monthly mean based on a specified historical period. Cumulative heating of 4 DHW is associated with mild bleaching, whereas exceeding 8 DHW usually results in severe bleaching and widespread coral mortality (Kayanne, 2017; Fordyce et al., 2019).
Severe bleaching was observed on the GBR in 1998, 2002, 2016, 2017, and 2020, with events over the last decade impacting 30–65% of all reefs (Pratchett et al., 2021b). Climate projections suggest that heatwaves will continue to become more frequent and intense as global temperatures rise (Oliver et al., 2019; Grose et al., 2020). Changes in coral cover have therefore been explored under Representative Concentration Pathways RCP 2.6, RCP 4.5, and RCP 8.5 (noting that indices for ocean temperature extremes in the GBR region are similar in the last two phases of the Climate Model Intercomparison Experiments, CMIP5 and CMIP6, Grose et al., 2020).
For each climate scenario, 100-member ensemble model runs covered the period 1950–2070. Where previous fitting to data could only constrain model parameters within a specified range (Morello et al., 2014), parameter values were varied randomly (i.e., flat distribution) over that range throughout each run (Condie et al., 2021). Historical cyclones and marine heatwaves were applied as stochastic mortality events following observed spatial patterns for 1950–2019. From 2020 to 2070, they switched to random events described by probabilistic frequency and intensity distributions based on modeled projections (van Hooidonk et al., 2016; Condie et al., 2021). For each RCP, maximum DHW was represented by a sigmoid curve describing regional DHW envelopes from the Hadley Center Global Environmental Model version 2 (HadGEM2-ES) Earth system model using the long-term monthly mean sea surface temperature field from the period 1985–2000 (Kwiatkowski et al., 2015). A marine heatwave could occur in any year with the maximum geographical extent of the bleaching event increasing with the maximum annual DHW. The ratio of these two quantities was set to ensure that the average proportion of locations bleached on the GBR per annum aligned with corresponding empirical estimates from the Australasian region (Hughes et al., 2018) and longer-term projections downscaled from an ensemble of CMIP5 models (Taylor et al., 2012; van Hooidonk et al., 2016). While the timing and intensity of individual events changed with each run within the ensemble, the underlying probability distributions were consistent across all runs for a given RCP scenario.
While the method can be applied at any geographical scale, assessments presented here were all undertaken at the whole GBR-scale. This approach reflects the strong underlying interconnectedness of the GBR system through processes such as larval dispersal (Hock et al., 2014, 2019). It also aligned with the scale at which modeled projection data were available (Condie et al., 2021) and reduced the sensitivity of assessments to uncertainties in the finer-scale spatial structure of climate projections (Grose et al., 2020).
Reef interventions
Coral reef interventions aimed at retaining and restoring coral cover include actions such as restoring adjacent catchments and wetlands to improve water quality, introducing more resilient corals, reducing coral predators, and shading corals to reduce heat stress. However, effective deployment across large reef systems remains a challenge (Morrison et al., 2020). We are concerned here with deployments on scales ranging from the major zones of the GBR (northern, central, and southern) to the entire GBR, which we refer to as regional interventions to differentiate them from global climate action. Two types of regional intervention were used to demonstrate the risk-based approach, chosen due to their high modeled efficacy relative to other options (Condie et al., 2021). These were CoTS control and solar radiation management (SRM). The analysis also focused on RCP 4.5, usually regarded as the closest pathway to current Nationally Determined Contribution (NDC) commitments (Gallo et al., 2017).
Modeling of CoTS control closely followed the approach used by control vessels currently operating on the GBR (Condie et al., 2021). Briefly, control vessels deployed divers to kill adult CoTS using lethal injection. Scenarios assumed either five control vessels, corresponding to the 2012–18 deployment on the GBR, or a more aspirational ten control vessels (Table 1). Control targeted priority reefs before moving onto other reefs as allowed by vessel capacity. Each vessel carried 8 divers and conducted 20 voyages per year, each lasting 10 days over which 36 dive sessions were completed for a total of 5,760 individual dives per vessel per year. Crown-of-thorns starfish were killed sequentially at each dive site (corresponding to 8 ha of coral habitat) until numbers fell below an ecological threshold that increased with coral cover (Condie et al., 2021). It was also assumed that younger CoTS were harder to detect, thereby leaving a smaller proportion of them controlled (Pratchett et al., 2021a).
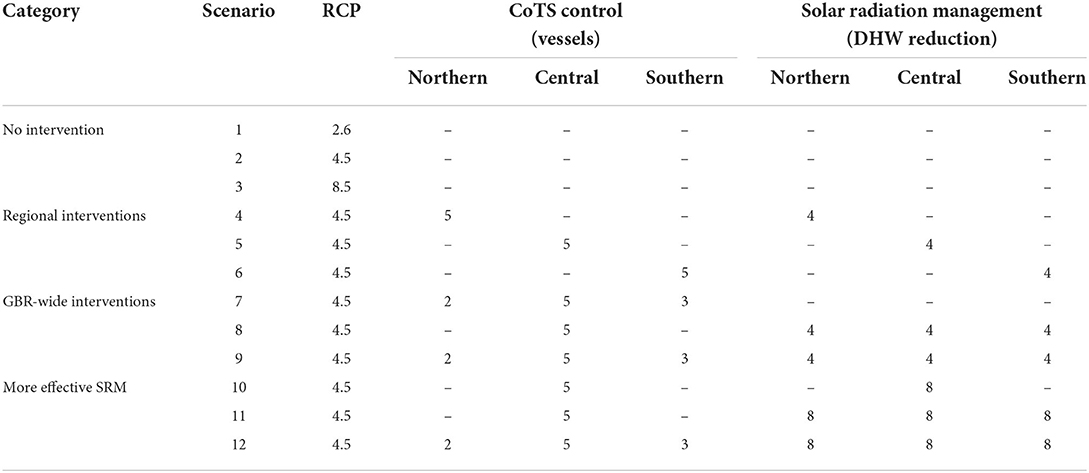
Table 1. Definition of model scenarios in terms of climate forcing and the distribution of interventions across the GBR.
Solar radiation management modeled the reduction in solar radiation and ocean heating due to cloud-brightening—a process involving spraying ultra-fine droplets of seawater into the atmosphere that are subsequently uplifted into stratocumulus clouds where residual salt crystals provide nucleation sites to enhance cloud condensation. The net effect of SRM in the model was specified as a fixed reduction in DHW exposure of corals within the target SRM area (Condie et al., 2021). Scenarios assumed a reduction of either 4 or 8 DHW (Table 1). Previous modeling of the effect of radiative forcing on ocean temperatures over GBR reefs indicates that a 30% increase in low-level cloud albedo (corresponding to a 6.5% increase in average albedo) would have reduced heat stress by 7.5 ± 3.5 DHW over the summer of 2015–2016 and 8.3 ± 3.7 DHW over the summer of 2016–2017 (Harrison et al., 2019). While still uncertain, the two DHW values adopted for the scenarios cover the range that current trials of cloud-brightening technologies suggest might be feasible.
While assessments were applied at the GBR-scale, interventions were mostly deployed at regional-scale (northern, central, or southern GBR, Figure 1A) to reflect expected cost and logistical constraints. However, because the GBR is strongly interconnected by larval dispersal for both corals and CoTS, intervention benefits often extended well-beyond the deployment region. Scenarios explored different geographical distributions of CoTS control starting in 2020 and SRM starting in 2025 (Table 1). While CoTS control has already been widely deployed on the GBR (Babcock et al., 2020; Pratchett et al., 2021a), the delayed start of SRM reflects the need for further development of technologies and likely represents the earliest possible time that deployment at scale could be feasible.
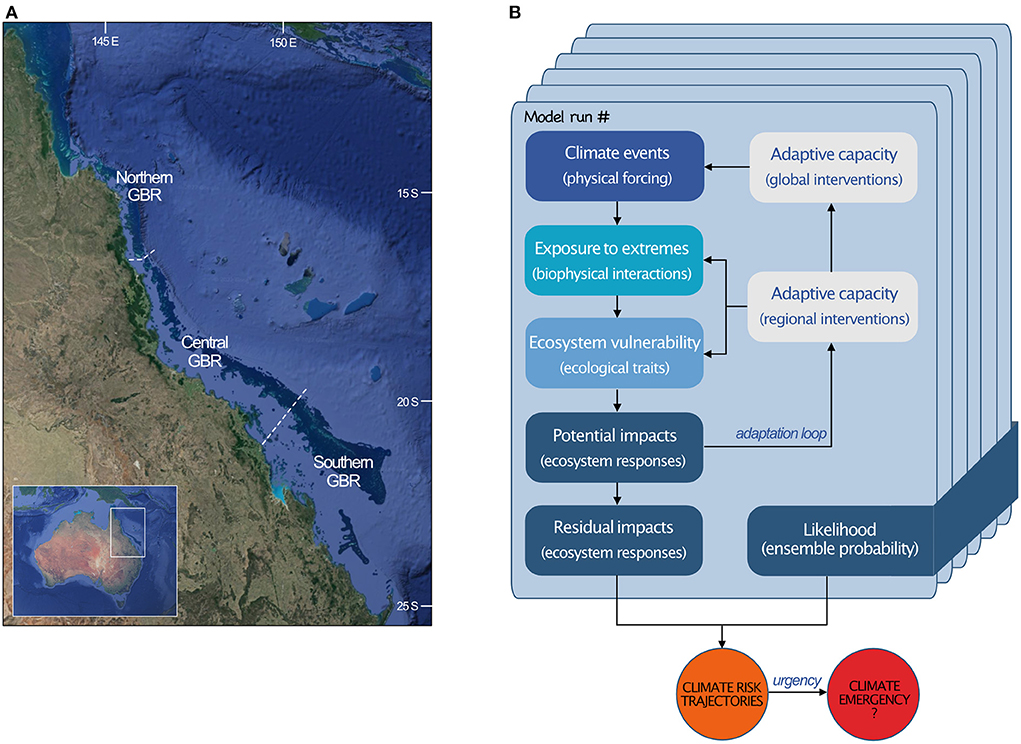
Figure 1. (A) Map of the Great Barrier Reef (GBR) indicating major zonal regions. (B) Components of the climate risk assessment utilizing ensemble modeling. Adaptive capacity can be in the form of either regional interventions (deployments on the GBR) or global interventions (emission reductions to modify the RCP), both of which were varied to generate the series of climate risk trajectories. The time required to transition from an unacceptable trajectory to an acceptable trajectory is influenced by technological and socio-economic factors. Where that transition cannot be completed before the risk level become unacceptable, a climate emergency is said to exist.
A risk-based approach
Nearly all quantitative risk analyses are based on the premise that risk to an asset is the product of the probability (or likelihood) that an event will occur at the asset location (exposure) and the adverse impact (or consequence) of that event on the asset (Figure 1B). The impact is in turn dependent on the vulnerability of the asset. If the risk is managed through an intervention, then risk may be reduced where the asset has the ability to adapt to change through its adaptive capacity (Cinner et al., 2018). This is the underlying framework recommended by the Intergovernmental Panel on Climate Change (IPCC) for managing the risks associated climate change (i.e., climate risk) and identifying adaptive pathways (Lavell et al., 2012). It is also consistent with risk assessment procedures currently used on the GBR (GBRMPA, 2017).
A key objective of the current study is to demonstrate how results from stochastic ensemble modeling can be integrated into the traditional risk assessment framework (Figure 1B). The advantage of using ensemble modeling is that the key elements of exposure, vulnerability, adaptive capacity, and impact are all explicitly included in the model and therefore dynamically consistent. This obviates the need to estimate them independently and then combine them under further assumptions, as is the case for semi-quantitative climate change vulnerability risk assessments that typically utilize rankings based on literature and expert knowledge (Chin et al., 2010; Hobday et al., 2011). Using large ensembles of model runs incorporating stochastic aspects of exposure and response also provides a rigorous approach for capturing uncertainty and estimating probabilistic likelihood, which are both fundamental to the estimation of risk (Reckhow, 1999).
Risk matrices
The risk-based approach required dynamically consistent estimates of consequence (impact) and likelihood (probability) derived from the model ensemble results. For the reef system, consequence was defined in terms of exceeding thresholds in coral cover. The likelihood of exceeding such thresholds was assumed to equal the proportion of the ensemble runs that exceeded these thresholds. Different risk levels could then be defined by combinations of likelihood and consequence mapped onto a risk matrix. Specific combinations have been selected here to demonstrate the approach (Table 2). However, for sector specific applications, definitions should be based on relevant management objectives.
The efficacy of combinations of CoTS control and SRM was measured in terms of forecast projections for average coral cover across the GBR reef network. For each run within an ensemble, impacts on average coral cover were calculated relative to 2020 levels from the same run (which ranged from 10 to 22%). Combining all 100 members of the ensemble then provided exceedance probabilities for any level of loss in any year. These distributions were transformed into risk matrices and risk trajectories by focusing on three loss thresholds corresponding to a decline (>0 pp), a major decline (>5 pp), or a catastrophic decline (>10 pp), where pp refers to the percentage points of coral cover lost since 2020 (Table 2). These thresholds generally provide good coverage of modeled losses (Figure 3A). For example, runs with the ensemble average coral cover of 16% in 2020 (Figure 2) had thresholds of 16%, 11%, and 6%, respectively. These levels were selected to illustrate the approach and should ultimately be defined by management objectives. However, given that prior to the year 2000 coral cover was typically within the range 21–26%, it is safe to assume that the levels chosen here will be of significant concern to most stakeholders.
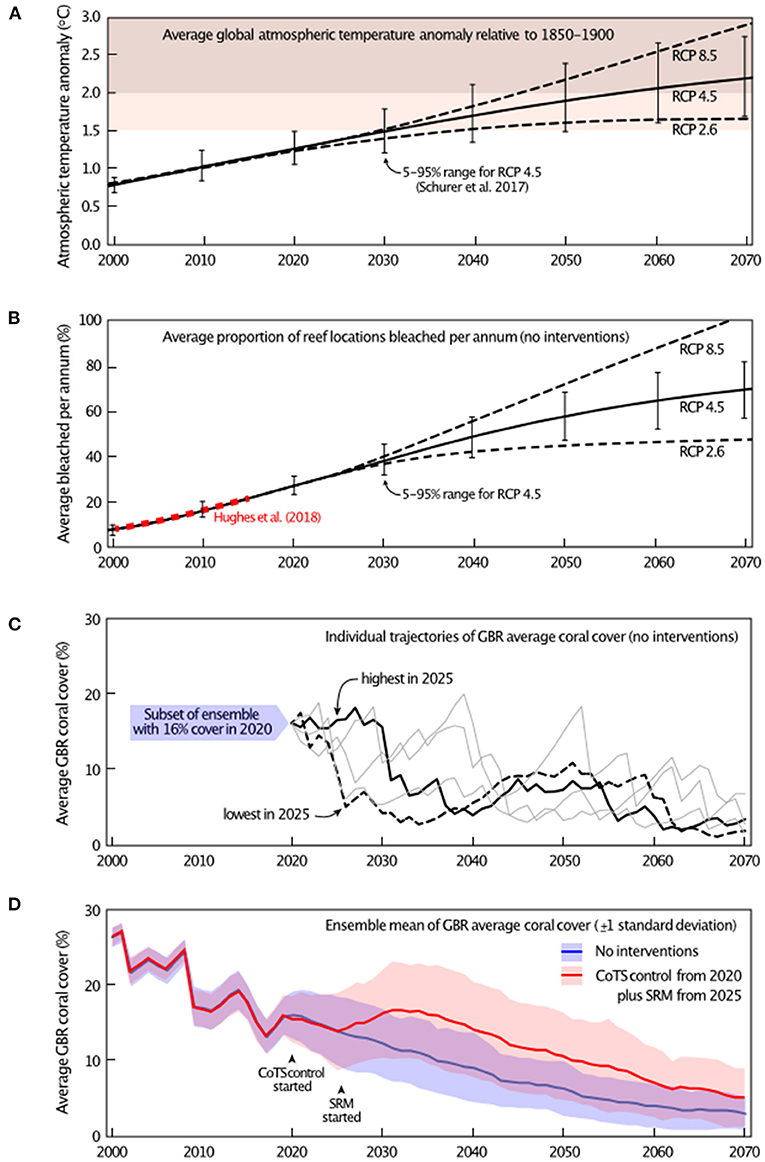
Figure 2. Modeled projections of climate conditions and GBR responses. (A) Multi-model-mean global atmospheric temperature anomaly relative to 1850–1900 under three RCP scenarios (Schurer et al., 2017). (B) Average proportion of locations bleached per annum under three RCP scenarios, assuming that the past linear relation between trends in mean atmospheric temperature and DHWs continues (Lough et al., 2018), and corresponding estimates from empirical data for 1980–2016 (Hughes et al., 2018). The long-term values are also consistent with the frequency of mass bleaching (>8 DHW) estimated from climate model projections for RCP 2.6 (0.35–0.45) and RCP 4.5 (0.55–0.75) (Frieler et al., 2013; Kwiatkowski et al., 2015), as well as forecasts of annual bleaching across nearly all of the GBR by 2070 under RCP 8.5 (van Hooidonk et al., 2016). (C) A sample of five individual trajectories selected from a 100-member model ensemble under RCP 4.5 with no interventions. Trajectories were selected on the basis that they all had close to 16% average coral cover in 2020 equal to the ensemble average in that year and consistent with GBR observations (https://www.aims.gov.au/reef-monitoring/gbr-condition-summary-2019-2020). The solid black line shows the trajectory with the highest average coral cover in 2025, the dashed black line shows the trajectory with the lowest average coral cover in 2025, and the three gray lines show trajectories that had intermediate levels of coral cover in 2025. (D) Ensemble mean (±1 standard deviation) under RCP 4.5 without interventions (blue, scenario 2) and with deployment in the central GBR of five CoTS control vessels from 2020 and SRM of 8 DHW from 2025 (red, scenario 10). Note the strong overlap of the two trajectory ranges (mauve).
Risk trajectories
Because the likelihood of any consequence level evolves through time, future time periods can be mapped onto the risk matrix. The current analysis averaged over 5-year blocks, which is a timescale that adequately resolves climate driven trends while remaining relevant to environmental decision-making. The earliest 5-year period that each level of risk (low to extreme) was reached could then be read directly from this temporal form of the risk matrix. Mapping risk level as a function of the earliest period that the level was reached, then provided a single risk trajectory. Trajectories from multiple scenarios were compared by combining them into a single matrix with columns corresponding to the 5-year time blocks and rows to scenarios.
Results
Future projections
Uncertainty in the future health of the GBR arises from uncertainties in both future emissions (Figure 2A) and the regional ecological response of the GBR to associated changes in climate (Figure 2B). Comparisons of spatially-averaged coral cover from ensemble model runs reveal large variability of individual trajectories through time and large differences between individual trajectories (up to a factor of 5) even through the underlying forcing by cyclones and heat waves were sampled from the same probabilistic frequency and intensity distributions (Figure 2C). Such non-linear responses place fundamental limitations on our ability to predict the future state of the GBR and underline the need for risk estimates that reflect these uncertainties.
Large uncertainties in future projections also impact on our ability to evaluate the efficacy of interventions. While combinations of interventions have potential to delay declines in ensemble averaged coral cover, strong overlap in the uncertainties associated with intervention options (Figure 2D) can confound decision-making aimed at reducing risk. An alternative approach is to use ensemble model results to estimate probabilities of different levels of climate impact, which can then be visualized within a risk framework.
The decline of coral communities on the GBR has been modeled and tracked in terms of the percentage point (pp) decrease in coral cover averaged over all GBR reefs. Without intervention, the probability distribution for coral loss in 2030 is similar for all climate scenarios (Figure 3A), with the median (50% probability) loss being 4–5 pp (Figure 3A). However, by 2050 scenario responses had diverged with median losses of 9 pp under RCP 2.6, 10 pp under RCP 4.5, and 12 pp under RCP 8.5. By 2070, median losses grew to 11, 14, and 14 pp, respectively (Figure 3A). Focusing on specific loss thresholds revealed that the probability of a net decline in coral cover grew quite sharply from around 50% in the early 2020s to more than 90% by the 2040s for all RCPs (Figure 3B). The probability of a major decline (>5 pp) grew from <20% in the early 2020s to 90% by the 2050s. The probability of a catastrophic coral cover decline (>10 pp) grew from <10% in the late 2020s to more than 50% by the early 2050s. Prior to 2040, probabilities showed no clear ranking with RCP, whereas after 2040, probabilities always ranked exactly with RCP level indicating statistically significant differences in the probability trajectories (Figure 3B).
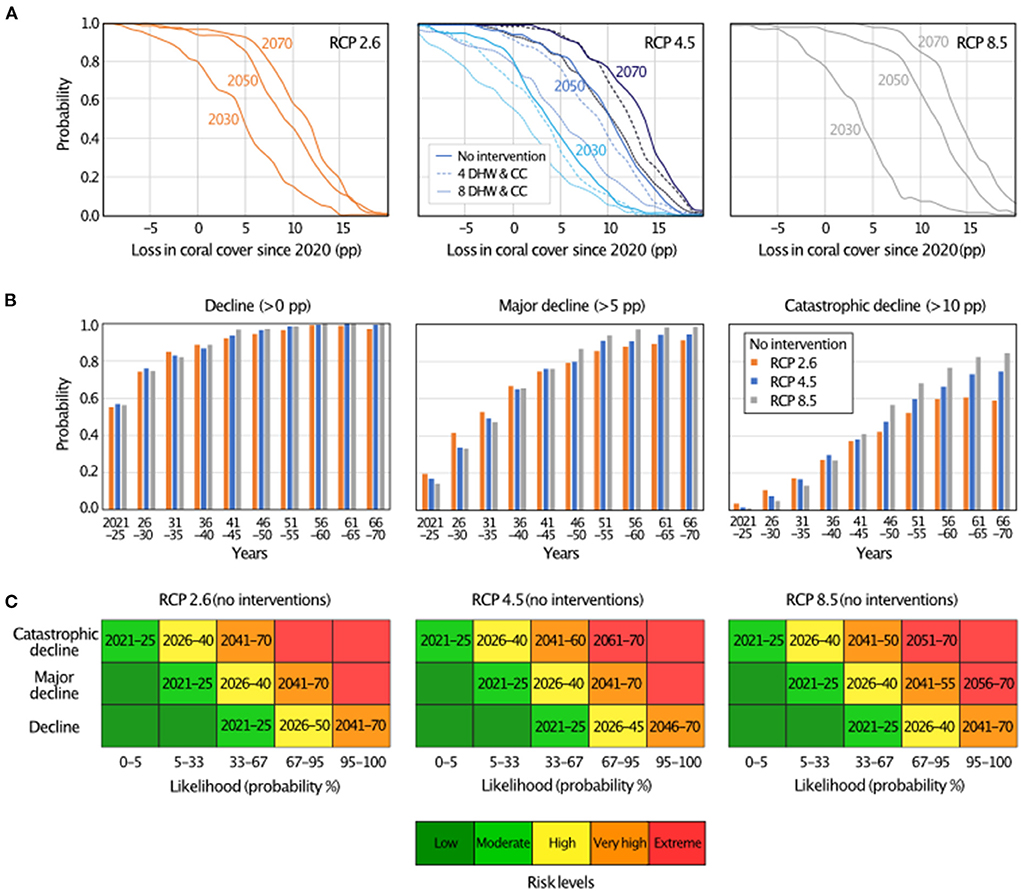
Figure 3. Comparisons of risk to coral cover (percentage of available reef habitat averaged over all reefs on the GBR) for RCP 2.6, RCP 4.5, and RCP 8.5 (scenarios 1–3, respectively) based on 100-run ensembles. (A) Probability of coral losses relative to 2020 in years 2030, 2050, and 2070. Dashed lines (RCP 4.5 only) include interventions in the form of CoTS control on the central GBR and two levels of SRM (4 DHW for scenario 8 and 8 DHW for scenario 11) applied across the GBR. (B) Probability of a net decline (>0 pp, left), a major decline (>5 pp, center), and a catastrophic decline (>10 pp, right). (C) Risk matrices indicating the likelihood (probability) of each level of coral cover impact (decline) over time measured in 5-year blocks over the period 2021–2070. The selected risk level descriptors (and colors) are only illustrative and can be modified to align with the objectives of any risk assessment.
Risk matrices
By defining risk level thresholds for impacts (declines) and likelihoods (probabilities), data from Figure 3A was transformed directly into quantitative risk matrices showing when each risk level was reached (Figure 3C). This revealed a rapid escalation to high risk levels in less than a decade under all RCPs, and then to very high risk in the 2040s. RCP 2.6 then plateaued at very high risk, whereas RCP 4.5 and RCP 8.5 both escalated to extreme risk by the 2060s and 2050s, respectively. There was a lag between the divergence of warming scenarios (Figures 2A,B) and the divergence of coral community responses (Figure 3C), reflecting gradual accumulation of impacts from multiple stressors.
A similar approach has been used to compare the residual risks following interventions in the form of CoTS control and SRM. Focusing first on regional differences, combined SRM (4 DHW) and CoTS control (5 control vessels) was most effective when focused in the northern or central GBR, where the transition to very high risk was delayed and extreme risks avoided out to at least 2070 (Figures 3A, 4A). Enhancing CoTS control across the GBR (10 vessels) without SRM delayed the risk of catastrophic decline in the second half of the century (cf. Figures 3B, 4B). When SRM was deployed throughout the GBR, reductions in risk were evident earlier (from around 2040), and were similar for both levels of CoTS control. Assuming more effective SRM (8 DHW) was successful in delaying very-high risk levels by an extra decade to the mid 2050s and avoiding extreme risks (Figure 4C). Importantly, these benefits were realized even when both CoTS control and SRM were restricted to the central GBR.
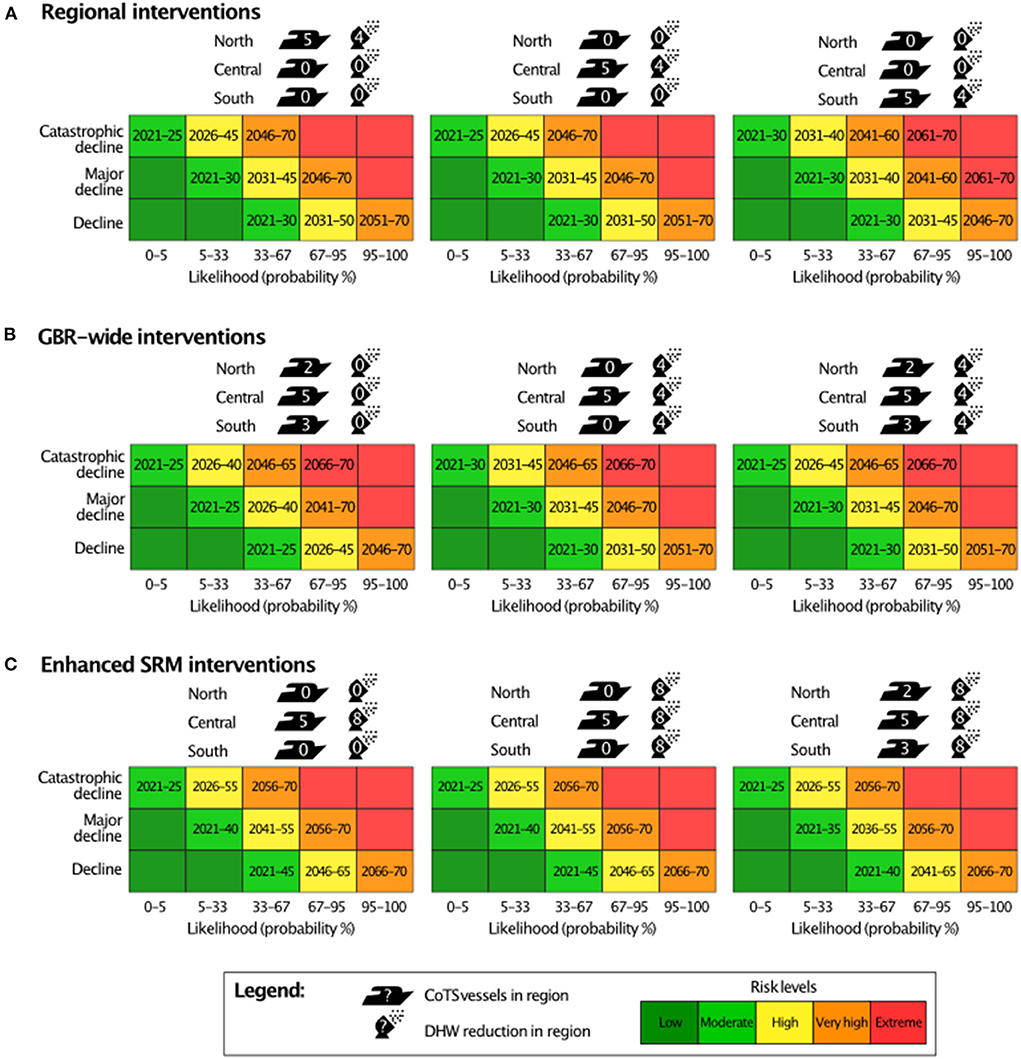
Figure 4. Comparisons of risk to coral cover (percentage of available reef habitat averaged over all reefs on the GBR and 5-year blocks over the period 2021–2070) under a range of global climate action and regional intervention scenarios, each based on 100-run ensembles. In each case, the vessel icon indicates the number of CoTS control vessels operating and the cloud generator icon indicates the reduction in DHW within each region. (A) Risk matrices for interventions focusing exclusively in the northern, central, or southern parts of the GBR (scenarios 4–6). (B) Risk matrices for interventions with zero or modest levels of SRM (4 DHW) distributed across the entire GBR and two levels of CoTS control (scenarios 7–9). (C) Risk matrices for interventions with enhanced levels of SRM (8 DHW) and two levels of CoTS control (scenarios 10–12). The selected risk level descriptors (and colors) are only illustrative and can be modified to align with the objectives of any risk assessment.
Risk trajectories
Levels of risk reduction associated with intervention options can be more easily compared by transforming risk matrices (Figures 3C, 4A–C) into risk trajectories (Figure 5). The risk trajectories clearly demonstrate the benefits of combining a strong SRM program with sustained CoTS control in the central GBR. For example, 5 control vessels with a 4 DHW SRM program focused in the central GBR could provide moderate reductions in risk over the medium to long-term. Increasing the efficacy of SRM to 8 DHW provided further reductions, particularly around the middle of the century (Figure 5), with most of the benefit achievable through intervention in the central GBR.
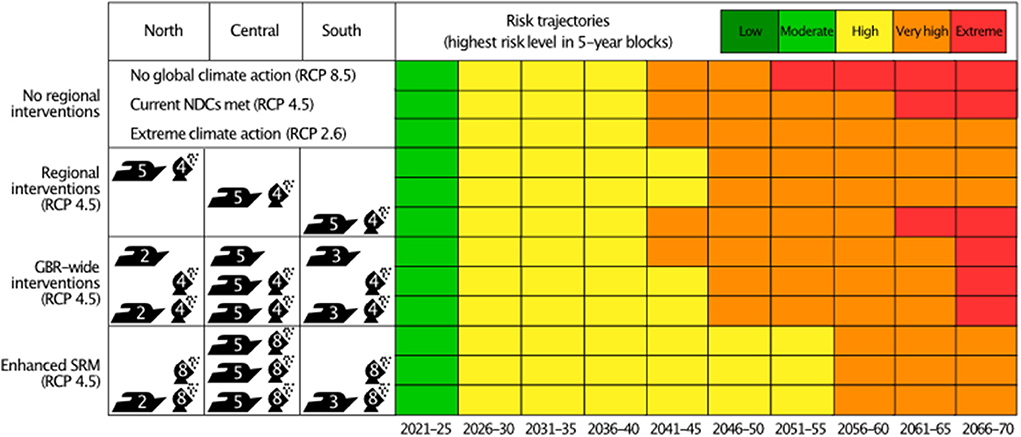
Figure 5. Risk trajectories based on the highest risk level for each 5-year time block over the period 2021–2070 read directly from the risk matrices in Figure 3C (no regional interventions) and Figures 4A–C (regional interventions). The selected risk level descriptors (and colors) are only illustrative and can be modified to align with the objectives of any risk assessment.
Discussion
Any ambiguity in the assessment of the risks of climate change can increase the scope for both untoward influence by vested interests and post-hoc justification of decisions (Frank, 2019; Morrison et al., 2019). Disconnects between climate scenario modeling and more traditional risk assessment methods have hampered effective characterization and communication of climate change risks (Jurgilevich et al., 2017; Weaver et al., 2017; Adger et al., 2018; Sutton, 2019; Viner et al., 2020). While integrated assessments have been undertaken for hazardous events (King et al., 2015) and agricultural impacts (Cobon et al., 2016), such analyses have largely been restricted to direct impacts of climate change estimated at large spatial and temporal scales. The approach described here for translating ensemble model results into risk trajectories is transferable to any sector where quantitative climate impact modeling has been undertaken with estimates of uncertainty. Importantly, this approach eliminates the need for independent estimates of exposure, sensitivity, impacts, and likelihood, all of which can be dynamically linked through ensemble modeling of the system (Figure 1B). Additional local socio-ecological knowledge (Ekstrom et al., 2013) and other lines of evidence (Sherwood et al., 2020) can also be incorporated when setting and interpreting risk categories and thresholds (Brown, 2018).
Risks to the GBR
Without regional interventions, model results suggest that risks to the GBR will transition from high to very high in the early 2040s, irrespective of the climate scenario (Figure 5). This escalates to extreme risk in the early 2060s for RCP 4.5 and early 2050s for RCP 8.5. The latter is a decade earlier than the “ecological disruption” (defined as more than 20% of coral species exceeding their historical ocean temperature maximum for five consecutive years) predicted under RCP 8.5 for the even more susceptible Coral Triangle region (Trisos et al., 2020). While the approaches used to model risk are different (estimating distributions of coral species based on their exposure to annually-averaged surface ocean temperatures verses dynamic modeling of coral cover exposed to extreme events), the earlier impacts implied by our study may reflect the additional risks posed by cumulative short-term regional events that are resolved by the downscaled model (Condie et al., 2021), but not by global projections used to define environmental niches (Trisos et al., 2020). In any case, the risk trajectories emphasize that the slow pace of climate action has already committed us to increasing risks, which could plateau around the middle of the century with strong global climate action, or continue to deteriorate to extreme levels of risk even under current NDC commitments (Figure 5).
Reducing risk through regional interventions
While the current assessments have focused on GBR-wide benefits, deployment of interventions within some regions has been found to have higher efficacy than others. For example, marked reductions in future risks to coral cover may be achieved by maximizing SRM and focusing CoTS control in the northern or central GBR (Figure 5). Indeed, applying these strategies under RCP 4.5 resulted in lower risk trajectories than RCP 2.6 without intervention (Figure 3A or cf. Figures 3C, 5). The higher efficacy associated with northern and central interventions is likely due to the cascading effect of coral and CoTS larvae dispersing across the GBR under prevailing current patterns (Hock et al., 2017, 2019). Expanding SRM more broadly across the GBR reduced the risk of catastrophic decline (compare central column of risk matrices in Figure 4), although improvements in the overall risk trajectory required enhanced rates of SRM (8 DHW).
Risk trajectories can directly support management and policy objectives that are formulated from a risk perspective. For example, if the objective is to avoid extreme risk levels over the long term under RCP 4.5, then some form of regional intervention will be essential. Northern or central GBR interventions combining CoTS control and moderate SRM, or any of the interventions with enhanced SRM, will satisfy this objective provided the transition occurs before 2040 when the RCP 4.5 trajectory with no regional interventions diverges from the regional intervention trajectories (Figure 5). For the more ambitious objective of avoiding very high risks, then even enhanced SRM can only achieve this to the mid 2050s. Meeting this objective over the longer term will likely require better optimization of deployments, new technologies that are yet to be explored using the model, and possibly a further reduction in global emissions (below RCP 4.5).
Model uncertainty
Large uncertainties remain in modeling both environmental change at regional scales and the ecological response to those changes (van Hooidonk et al., 2016). For example, while natural adaptation of corals would be expected to delay risk trajectories, it was excluded from the formal risk analysis due to uncertainties in rates of both thermal adaptation in coral species (Hughes et al., 2003) and shuffling of their algal symbiont populations (Sampayo et al., 2008). Explorative runs of the CoCoNet model using “plausible” rates of natural adaption based on past bleaching patterns (Logan et al., 2014; Sully et al., 2019) suggest that transitions to higher risk levels may be delayed by up to 10-years without intervention and up to 15-years with large-scale interventions (Condie et al., 2021). These gains correspond to 2–3 of the 5-year time blocks in Figure 5, which is comparable to the difference between RCP 4.5 and RCP 2.6. However, without further empirical data to constrain adaptation rates, these estimates remain speculative.
While the underlying models will continue to improve in terms of both environmental forcing (CMIP6 and beyond) and parameterization of ecological responses (new empirical data), the current study provides a first estimate of the reduction in risk associated with intervention options and supports the assertion that regional action can help manage climate risk on the GBR within limits. For example, to maintain the current moderate level of risk, CoTS control efforts will need to be maintained and high-efficacy SRM deployed by 2030, with even more effective interventions needing to be identified and deployed by 2035 (Figure 5). These are very short timelines for the development of new technologies, which underlines the need for urgency in protecting vulnerable ecosystems such as the GBR.
The urgency of climate action has previously been defined as the required reaction time divided by the time left to avoid a bad outcome (Lenton et al., 2019). When the urgency exceeds one, control has effectively been lost and conditions are described as a climate emergency (McHugh et al., 2021). This definition can be recast in terms of our risk trajectories as the time to transition from the current risk trajectory to an acceptable risk trajectory, divided by the time before an unacceptable risk level on the current trajectory (Figure 1B). The acceptability of risk to the GBR is dependent on a diverse range of social and economic values (Stoeckl et al., 2021) and its determination is beyond the scope of the current study. However, for a society that accepts no more than a moderate level of risk (as defined in Table 2), then a GBR climate emergency appears almost inevitable before 2030. If a high level of risk was somehow acceptable, then the climate emergency might be delayed for 15–30 years depending on the effectiveness of interventions (Figure 5). In any case, urgent climate emissions mitigation and local interventions to improve adaptive capacity will continue to be required to avoid very high to extreme levels of risk after 2040.
Data availability statement
Publicly available datasets were analyzed in this study. This data can be found here: Dryad: https://doi.org/10.5061/dryad.zkh18937s.
Author contributions
SC conceived the study, conducted the analysis, and wrote the manuscript.
Funding
The Reef Restoration and Adaptation Program (RRAP, https://gbrrestoration.org) contributed funding to the study.
Acknowledgments
The Reef Restoration and Adaptation Program (RRAP, https://gbrrestoration.org) contributed to the development of the CoCoNet model. Thanks also to Dr. Keith Hayes whose expert advice on risk assessment approaches improved the manuscript.
Conflict of interest
The author declares that the research was conducted in the absence of any commercial or financial relationships that could be construed as a potential conflict of interest.
Publisher's note
All claims expressed in this article are solely those of the authors and do not necessarily represent those of their affiliated organizations, or those of the publisher, the editors and the reviewers. Any product that may be evaluated in this article, or claim that may be made by its manufacturer, is not guaranteed or endorsed by the publisher.
Supplementary material
The Supplementary Material for this article can be found online at: https://www.frontiersin.org/articles/10.3389/fclim.2022.980035/full#supplementary-material
References
Adger, W. N., Brown, I., and Surminski, S. (2018). Advances in risk assessment for climate change adaptation policy. Philos. Trans. R. Soc. A 376:20180106. doi: 10.1098/rsta.2018.0106
Babcock, R. C., Plaganyi, E. E., Condie, S. A., Westcott, D. A., Fletcher, C. S., Bonin, M. C., et al. (2020). Suppressing the next crown-of-thorns outbreak on the Great Barrier Reef. Coral Reefs 39, 1233–1244. doi: 10.1007/s00338-020-01978-8
Brown, I. (2018). Assessing climate change risks to the natural environment to facilitate cross-sectoral adaptation policy. Philos. Trans. R. Soc. A 376:20170297. doi: 10.1098/rsta.2017.0297
Chin, A., Kyne, P. M., Walker, T. I., and McAuley, R. B. (2010). An integrated risk assessment for climate change: analysing the vulnerability of sharks and rays on Australia's Great Barrier Reef. Glob. Chang. Biol. 16, 1936–1953. doi: 10.1111/j.1365-2486.2009.02128.x
Cinner, J. E., Adger, W. N., Allison, E. H., Barnes, M. L., Brown, K., Cohen, P. J., et al. (2018). Building adaptive capacity to climate change in tropical coastal communities. Nat. Clim. Chang. 8, 117–123. doi: 10.1038/s41558-017-0065-x
Cobon, D. H., Williams, A. A. J., Power, B., McRae, D., and Davis, P. (2016). Risk matrix approach useful in adapting agriculture to climate change. Clim. Change 138, 173–189. doi: 10.1007/s10584-016-1732-z
Condie, S. A., Anthony, K. R. N., Babcock, R. C., Baird, M. E., Beeden, R., Fletcher, C. S., et al. (2021). Large-scale interventions may delay decline of the Great Barrier Reef. R. Soc. Open Sci. 8:201296. doi: 10.1098/rsos.201296
Condie, S. A., Plaganyi, E. E., Morello, E. B., Hock, K., and Beeden, R. (2018). Great Barrier Reef recovery through multiple interventions. Conserv. Biol. 32, 1356–1367. doi: 10.1111/cobi.13161
De'ath, G., Fabricius, K. E., Sweatman, H., and Puotinen, M. (2012). The 27-year decline of coral cover on the Great Barrier Reef and its causes. Proc. Natl. Acad. Sci. U.S.A. 109, 17995–17999. doi: 10.1073/pnas.1208909109
Ekstrom, M., Kuruppu, N., Wilby, R. L., Fowler, H. J., Chiew, F. H. S., Dessai, S., et al. (2013). Examination of climate risk using a modified uncertainty matrix framework - applications in the water sector. Glob. Environ. Change Hum. Policy Dimens. 23, 115–129. doi: 10.1016/j.gloenvcha.2012.11.003
Fordyce, A. J., Ainsworth, T. D., Heron, S. F., and Leggat, W. (2019). Marine heatwave hotspots in coral reef environments: physical drivers, ecophysiological outcomes, and impact upon structural complexity. Front. Mar. Sci. 6:498. doi: 10.3389/fmars.2019.00498
Frank, D. M. (2019). Ethics of the scientist qua policy advisor: inductive risk, uncertainty, and catastrophe in climate economics. Synthese 196, 3123–3138. doi: 10.1007/s11229-017-1617-3
Frieler, K., Meinshausen, M., Golly, A., Mengel, M., Lebek, K., Donner, S. D., et al. (2013). Limiting global warming to 2°C is unlikely to save most coral reefs. Nat. Clim. Chang. 3, 165–170. doi: 10.1038/nclimate1674
Fulton, E. A., Smith, A. D. M., and Johnson, C. R. (2003). Effect of complexity on marine ecosystem models. Mar. Ecol. Prog. Ser. 253, 1–16. doi: 10.3354/meps253001
Gallo, N. D., Victor, D. G., and Levin, L. A. (2017). Ocean commitments under the Paris Agreement. Nat. Clim. Change 7, 833–838. doi: 10.1038/nclimate3422
GBRMPA (2017). Risk Management Policy (Document No. 100427). Great Barrier Reef Marine Park Authority.
Grose, M. R., Narsey, S., Delage, F. P., Dowdy, A. J., Bador, M., Boschat, G., et al. (2020). Insights from CMIP6 for Australia's future climate. Earths Future 8:e2019EF001469. doi: 10.1029/2019EF001469
Hagstrom, G., and Levin, S. (2017). Marine ecosystems as complex adaptive systems: emergent patterns, critical transitions, and public goods. Ecosystems 20, 458–476. doi: 10.1007/s10021-017-0114-3
Harrison, D. P., Harrison, L., Baird, M., Utembe, S., Schofield, R., Escobar-Correa, R., et al. (2019). Environmental Modelling of Large Scale Solar Radiation Management. A Report Provided to the Australian Government from the Reef Restoration and Adaptation Program. Australian Instiute of Marine Science, Townsville, Australia.
Hobday, A. J., Smith, A. D. M., Stobutzki, I. C., Bulman, C., Daley, R., Dambacher, J. M., et al. (2011). Ecological risk assessment for the effects of fishing. Fish. Res. 108, 372–384. doi: 10.1016/j.fishres.2011.01.013
Hock, K., Doropoulos, C., Gorton, R., Condie, S. A., and Mumby, P. J. (2019). Split spawning increases robustness of coral larval supply and inter-reef connectivity. Nat. Commun. 10:3463. doi: 10.1038/s41467-019-11367-7
Hock, K., Wolff, N. H., Condie, S. A., Anthony, K. R. N., and Mumby, P. J. (2014). Connectivity networks reveal the risks of crown-of-thorns starfish outbreaks on the Great Barrier Reef. J. Appl. Ecol. 51, 1188–1196. doi: 10.1111/1365-2664.12320
Hock, K., Wolff, N. H., Ortiz, J. C., Condie, S. A., Anthony, K. R. N., Blackwell, P. G., et al. (2017). Connectivity and systemic resilience of the Great Barrier Reef. PLoS Biol. 15:e2003355. doi: 10.1371/journal.pbio.2003355
Hughes, T. P., Anderson, K. D., Connolly, S. R., Heron, S. F., Kerry, J. T., Lough, J. M., et al. (2018). Spatial and temporal patterns of mass bleaching of corals in the Anthropocene. Science 359, 80–83. doi: 10.1126/science.aan8048
Hughes, T. P., Baird, A. H., Bellwood, D. R., Card, M., Connolly, S. R., Folke, C., et al. (2003). Climate change, human impacts, and the resilience of coral reefs. Science 301, 929–933. doi: 10.1126/science.1085046
Hughes, T. P., Kerry, J. T., Alvarez-Noriega, M., Alvarez-Romero, J. G., Anderson, K. D., Baird, A. H., et al. (2017). Global warming and recurrent mass bleaching of corals. Nature 543, 373–377. doi: 10.1038/nature21707
Jurgilevich, A., Rasanen, A., Groundstroem, F., and Juhola, S. (2017). A systematic review of dynamics in climate risk and vulnerability assessments. Environ. Res. Lett. 12:013002. doi: 10.1088/1748-9326/aa5508
Kause, A., de Bruin, W. B., Fung, F., Taylor, A., and Lowe, J. (2020). Visualizations of projected rainfall change in the United Kingdom: an interview study about user perceptions. Sustainability 12, 2955. doi: 10.3390/su12072955
Kayanne, H. (2017). Validation of degree heating weeks as a coral bleaching index in the northwestern Pacific. Coral Reefs 36, 63–70. doi: 10.1007/s00338-016-1524-y
King, D., Schrag, D., Dadi, Z., Ye, Q., and Ghosh, A. (2015). Climate Change: A Risk Assessment. Cambridge UK: Centre for Science and Policy, University of Cambridge.
Kwiatkowski, L., Cox, P., Halloran, P. R., Mumby, P. J., and Wiltshire, A. J. (2015). Coral bleaching under unconventional scenarios of climate warming and ocean acidification. Nat. Clim. Chang. 5, 777–781. doi: 10.1038/nclimate2655
Lavell, A., Oppenheimer, M., Diop, C., Hess, J., Lempert, R., Li, J., et al. (2012). “Climate change: new dimensions in disaster risk, exposure, vulnerability, and resilience,” in Managing the Risks of Extreme Events and Disasters to Advance Climate Change Adaptation. A Special Report of Working Groups I and II of the Intergovernmental Panel on Climate Change (IPCC), eds. C. B. Field, V. Barros, T. F. Stocker, D. Qin, D. J. Dokken, K. L. Ebi, M. D. Mastrandrea, K. J. Mach, G.-K. Plattner, S. K. Allen, M. Tignor, and P. M. Midgley (Cambridge, UK; New York, NY: Cambridge University Press), 25–64. doi: 10.1017/CBO9781139177245.004
Lenton, T. M., Rockstrom, J., Gaffney, O., Rahmstorf, S., Richardson, K., Steffen, W., et al. (2019). Climate tipping points - too risky to bet against. Nature 575, 592–595. doi: 10.1038/d41586-019-03595-0
Lewandowsky, S., Ballard, T., and Pancost, R. D. (2015). Uncertainty as knowledge. Philos. Trans. R. Soc. A 373:20140462. doi: 10.1098/rsta.2014.0462
Logan, C. A., Dunne, J. P., Eakin, C. M., and Donner, S. D. (2014). Incorporating adaptive responses into future projections of coral bleaching. Glob. Chang. Biol. 20, 125–139. doi: 10.1111/gcb.12390
Lough, J. M., Anderson, K. D., and Hughes, T. P. (2018). Increasing thermal stress for tropical coral reefs: 1871–2017. Sci. Rep. 8:6079. doi: 10.1038/s41598-018-24530-9
McHugh, L. H., Lemos, M. C., and Morrison, T. H. (2021). Risk? Crisis? Emergency? Implications of the new climate emergency framing for governance and policy. Wiley Interdiscipl. Rev. Clim. Change 12:e736. doi: 10.1002/wcc.736
Morello, E. B., Plaganyi, E. E., Babcock, R. C., Sweatman, H., Hillary, R., and Punt, A. E. (2014). Model to manage and reduce crown-of-thorns starfish outbreaks. Mar. Ecol. Prog. Ser. 512, 167–183. doi: 10.3354/meps10858
Morrison, T. H., Adger, N., Barnett, J., Brown, K., Possingham, H., and Hughes, T. (2020). Advancing coral reef governance into the anthropocene. One Earth 2, 64–74. doi: 10.1016/j.oneear.2019.12.014
Morrison, T. H., Hughes, T. P., Adger, W. N., Brown, K., Barnett, J., Lemos, M. C., et al. (2019). Save reefs to rescue all ecosystems. Nature 573, 333–336. doi: 10.1038/d41586-019-02737-8
Oliver, E. C. J., Burrows, M. T., Donat, M. G., Sen Gupta, A., Alexander, L. V., Perkins-Kirkpatrick, S. E., et al. (2019). Projected marine heatwaves in the 21st century and the potential for ecological impact. Front. Mar. Sci. 6:734. doi: 10.3389/fmars.2019.00734
Plaganyi, E. E., Ellis, N., Blamey, L. K., Morello, E. B., Norman-Lopez, A., Robinson, W., et al. (2014). Ecosystem modelling provides clues to understanding ecological tipping points. Mar. Ecol. Prog. Ser. 512, 99–113. doi: 10.3354/meps10909
Pratchett, M. S., Caballes, C. F., Cvitanovic, C., Raymundo, M. L., Babcock, R. C., Bonin, M. C., et al. (2021a). Knowledge gaps in the biology, ecology, and management of the pacific crown-of-thorns sea star, Acanthaster sp., on Australia's Great Barrier Reef. Biol. Bull. 241. doi: 10.1086/717026
Pratchett, M. S., Caballes, C. F., Rivera-Posada, J. A., and Sweatman, H. P. A. (2014). Limits to understanding and managing outbreaks of crown-of-thorns starfish (Acanthaster spp.). Oceanogr. Mar. Biol. 52, 133–199. doi: 10.1201/b17143-4
Pratchett, M. S., Heron, S. F., Mellin, C., and Cumming, G. S. (2021b). “Recurrent mass-bleaching and the potential for ecosystem collapse on Australia's Great Barrier Reef,” in Ecosystem Collapse and Climate Change. Ecological Studies, Vol. 241, eds J. G. Canadell and R. B. Jackson (Cham: Springer), 265–289. doi: 10.1007/978-3-030-71330-0_10
Reckhow, K. H. (1999). Lessons from risk assessment. Hum. Ecol. Risk Assess. 5, 245–253. doi: 10.1080/10807039991289392
Sampayo, E. M., Ridgway, T., Bongaerts, P., and Hoegh-Guldberg, O. (2008). Bleaching susceptibility and mortality of corals are determined by fine-scale differences in symbiont type. Proc. Natl. Acad. Sci. U.S.A. 105, 10444–10449. doi: 10.1073/pnas.0708049105
Schaffer, W. M., and Kot, M. (1986). Chaos in ecological-systems - the coals that Newcastle forgot. Trends Ecol. Evol. 1, 58–63. doi: 10.1016/0169-5347(86)90018-2
Schurer, A. P., Mann, M. E., Hawkins, E., Tett, S. F. B., and Hegerl, G. C. (2017). Importance of the pre-industrial baseline for likelihood of exceeding Paris goals. Nat. Clim. Chang. 7, 563–567. doi: 10.1038/nclimate3345
Sherwood, S. C., Webb, M. J., Annan, J. D., Armour, K. C., Forster, P. M., Hargreaves, J. C., et al. (2020). An assessment of earth's climate sensitivity using multiple lines of evidence. Rev. Geophys. 58,e2019RG000678. doi: 10.1029/2019RG000678
Stoeckl, N., Condie, S., and Anthony, K. (2021). Assessing changes to ecosystem service values at large geographic scale: a case study for Australia's Great Barrier Reef. Ecosyst. Serv. 51:101352. doi: 10.1016/j.ecoser.2021.101352
Sully, S., Burkepile, D. E., Donovan, M. K., Hodgson, G., and van Woesik, R. (2019). A global analysis of coral bleaching over the past two decades. Nat. Commun. 10:1264. doi: 10.1038/s41467-019-09238-2
Sutton, R. T. (2019). Climate science needs to take risk assessment much more seriously. Bull. Am. Meteorol. Soc. 100, 1637–1642. doi: 10.1175/BAMS-D-18-0280.1
Sweatman, H., Delean, S., and Syms, C. (2011). Assessing loss of coral cover on Australia's Great Barrier Reef over two decades, with implications for longer-term trends. Coral Reefs 30, 521–531. doi: 10.1007/s00338-010-0715-1
Taylor, K. E., Stouffer, R. J., and Meehl, G. A. (2012). An overview of CMIP5 and the experiment design. Bull. Am. Meteorol. Soc. 93, 485–498. doi: 10.1175/BAMS-D-11-00094.1
Trisos, C. H., Merow, C., and Pigot, A. L. (2020). The projected timing of abrupt ecological disruption from climate change. Nature 580, 496–501. doi: 10.1038/s41586-020-2189-9
van Hooidonk, R., Maynard, J., Tamelander, J., Gove, J., Ahmadia, G., Raymundo, L., et al. (2016). Local-scale projections of coral reef futures and implications of the Paris Agreement. Sci. Rep. 6, 39666. doi: 10.1038/srep39666
Viner, D., Ekstrom, M., Hulbert, M., Warner, N. K., Wreford, A., and Zommers, Z. (2020). Understanding the dynamic nature of risk in climate change assessments - a new starting point for discussion. Atmos. Sci. Lett. 21:e958. doi: 10.1002/asl.958
Weaver, C. P., Moss, R. H., Ebi, K. L., Gleick, P. H., Stern, P. C., Tebaldi, C., et al. (2017). Reframing climate change assessments around risk: recommendations for the US National Climate Assessment. Environ. Res. Lett. 12, 1–8. doi: 10.1088/1748-9326/aa7494
Keywords: coral reefs, climate risk, ensemble modeling, interventions, Great Barrier Reef
Citation: Condie SA (2022) Changing the climate risk trajectory for coral reefs. Front. Clim. 4:980035. doi: 10.3389/fclim.2022.980035
Received: 28 June 2022; Accepted: 20 October 2022;
Published: 04 November 2022.
Edited by:
Gustavo J. Nagy, Universidad de la República, UruguayReviewed by:
Lijing Cheng, Institute of Atmospheric Physics (CAS), ChinaKaoru Tachiiri, Japan Agency for Marine-Earth Science and Technology (JAMSTEC), Japan
Tiffany Morrison, James Cook University, Australia
Copyright © 2022 Condie. This is an open-access article distributed under the terms of the Creative Commons Attribution License (CC BY). The use, distribution or reproduction in other forums is permitted, provided the original author(s) and the copyright owner(s) are credited and that the original publication in this journal is cited, in accordance with accepted academic practice. No use, distribution or reproduction is permitted which does not comply with these terms.
*Correspondence: Scott A. Condie, c2NvdHQuY29uZGllQGNzaXJvLmF1