- 1Grantham Institute, Imperial College London, London, United Kingdom
- 2RAND Corporation, Santa Monica, CA, United States
Our plans to tackle climate change could be thrown off-track by shocks such as the coronavirus pandemic, the energy supply crisis driven by the Russian invasion of Ukraine, financial crises and other such disruptions. We should therefore identify plans which are as resilient as possible to future risks, by systematically understanding the range of risks to which mitigation plans are vulnerable and how best to reduce such vulnerabilities. Here, we use electricity system decarbonization as a focus area, to highlight the different types of technological solutions, the different risks that may be associated with them, and the approaches, situated in a decision-making under deep uncertainty (DMDU) paradigm, that would allow the identification and enhanced resilience of mitigation pathways.
Introduction
A variety of “good news” narratives tell us that the world is getting better according to multiple statistics, including around health, conflict, education and wealth (Pinker, 2018; Rosling et al., 2018). By contrast, the world has been hit by severe shocks, including the emergence of the coronavirus pandemic and global economic slowdown that followed in 2020, a burst of inflation, as well as the Russia-Ukraine crisis of 2022 and its impact on food and energy availability. In addition, longer-term problems, often exacerbated by such shocks, including the pressure on healthcare systems across the world as populations age (The Economist, 2023), all demonstrate that there remain serious threats to society, which may be exacerbated by the connectivity that has increased wellbeing, but that also increases the potential for complex and cascading risks (Simpson et al., 2021).
One societal risk that looms large is anthropogenic climate change. Indeed, the World Economic Forum's Global Risks Report 2023 survey of 1,200 experts across the globe found that most of the severe risks judged to be facing society over the next 10 years were all directly or indirectly related to climate change (World Economic Forum, 2023).
Overwhelming evidence makes clear that it is imperative that climate change mitigation does not fail (Masson-Delmotte et al., 2021; IPCC, 2022). Mitigation refers to the reduction of carbon dioxide and other greenhouse gas emissions through a combination of technological and behavioral changes. Technological changes consist primarily of using low-carbon technologies (such as solar photovoltaics, wind turbines, and other renewables) to replace incumbent technologies reliant on the combustion of fossil fuels, as well as technologies that use less energy. Behavioral changes consist of lowering demand for industrial manufactured goods, for transportation technologies and for building energy uses such as heating, cooling and appliance use, such that we become less wasteful and more conserving of energy, whilst still improving our welfare and development prospects, particularly in emerging, less-developed economies.
Over recent years, an increasing number of governments and private sector organizations have laid out policies and plans to reduce climate risk by charting pathways to a low-carbon future, in many cases through the achievement of net-zero targets around the middle of the 21st century. By mid-2022, 19 of the G20 countries had announced net-zero targets, with national governments representing more than 90% of global GDP having net-zero targets (Net Zero Tracker, 2022).
These targets have been developed using a least-cost analysis, which charts the path from our current configuration to a future net-zero target that incurs the lowest economic cost. For instance, integrated assessment models (IAMs) have formed the bedrock of analytical tools to help formulate pathways to low-carbon futures, including contributing heavily to the emergence of the requirement for net-zero targets by or around mid-century (IPCC, 2018). Such models overwhelmingly take a least-cost approach to calculating technological and behavioral strategies toward climate change mitigation (Wilson et al., 2021). However, as readily demonstrated by recent disruptions to societies and economies, climate change is just one of a number of risks. Considering how mitigation interplays with these other risks, what appears to be a least-cost pathway to net-zero emissions could in reality become neither least-cost nor net-zero.
We therefore propose that mitigation planning employ a least-risk, as opposed to least-cost, approach. The severe global disruptions of the last few years remind us how consequential the current focus on the latter might become. For example, the Russia-Ukraine conflict has revised coal- and oil-based power generation by significantly increasing gas prices in Asia and Europe (IEA, 2022). Meanwhile, severe and continuing heat waves in Europe (as well as across the world) during 2022 contributed to water shortages affecting the output of nuclear power plants in France (Plackett, 2022) and of hydro power plants throughout Europe (The Economist, 2022). Following a least-cost electricity system pathway, built on technologies that fail to perform in the face of climate impacts, would prove a costly mistake.
Mitigation pathways designed to minimize risks, rather than purely to minimize expected costs, could help address these challenges. Such least-risk pathways should be grounded in a decision-making under deep uncertainty (DMDU) (Kwakkel and Haasnoot, 2019) framework. DMDU contrasts with traditional analytical approaches grounded in making best-estimate point or probabilistic forecasts of future outcomes and then developing a plan around those forecasts. Applied to decarbonization, DMDU involves stress-testing alternative pathways over a wide range of plausible futures, in order to identify the vulnerabilities of each pathway, that is, the future conditions in which it would fail to meet societal goals, including decarbonization, cost, and equity (Lempert and Trujillo, 2018). The DMDU analysis then uses this information on vulnerabilities to identify new or augmented pathways with less vulnerability—and thus greater resilience—to a range of plausible risks. Here we focus on examples concerning decarbonizing electricity systems, which form a central pillar of practically all low-carbon transition pathways (Byers et al., 2022; IPCC, 2022).
Current mitigation pathways for electricity generation
There are many potential pathways to a low-carbon electricity system as part of energy system decarborization pathways consistent with the Paris Agreement goal of limiting global temperature increase to 1.5°C. Selected pathways for different integrated assessment models, all achieving a 1.5°C temperature target, have different mixes of electricity generation technologies as shown in Figure 1, indicating that a number of electricity technology portfolios could be deployed to meet this target. For instance, the fraction of electricity generated in 2050 by solar and wind in these pathways ranges from about 40 to 70%. Each portfolio is in effect a different strategy, which could have different vulnerabilities to a range of societal risks. Only a small subset of possible strategies is shown—for example there are approximately 100 different 1.5°C pathways in the latest IPCC sixth assessment report database of scenarios (Byers et al., 2022), themselves just a small subset of the future possibility space.
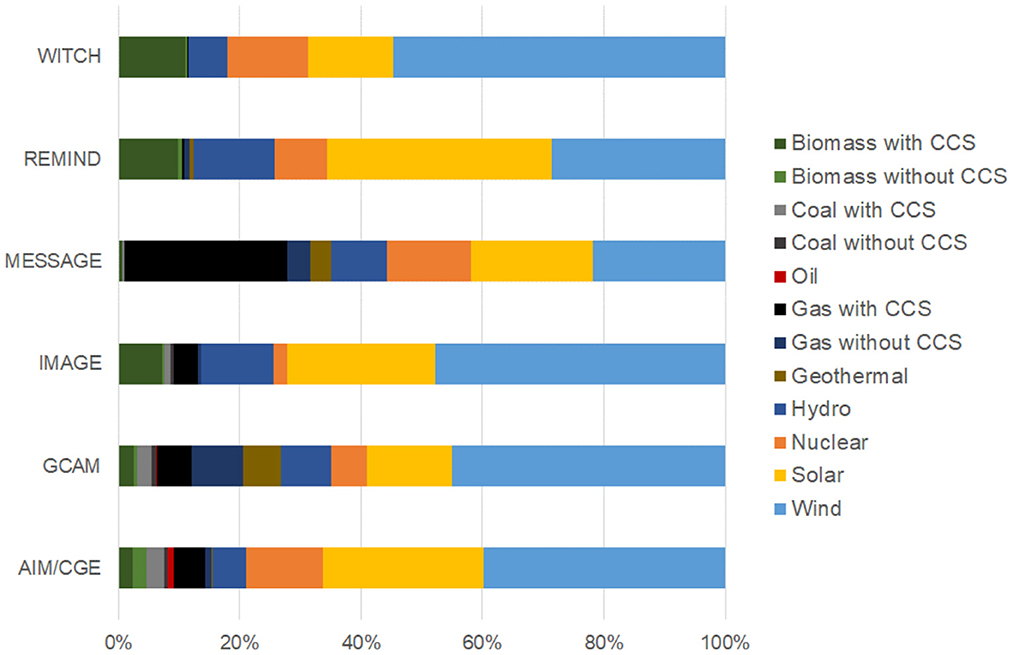
Figure 1. Share of 2050 electricity generation by model type in SSP1-1.9 scenario for OECD region. SSP1 is a socio-economic storyline emphasizing a “green growth” paradigm (Van Vuuren et al., 2017), whilst SSP1-1.9 is a set of scenarios that achieve an approximate 1.9 W/m2 radiative forcing by 2100, in line with the achievement of a 1.5°C limit to global warming (Rogelj et al., 2018). Source: SSP database (Riahi et al., 2017).
Vulnerability of electricity decarbonisation technologies and strategies
To assess the vulnerability of different electricity decarbonization strategies, it would first be useful to systematically categorize risks on scales relating to their potential likelihood of occurrence and impact if realized. Many risk taxonomies have been proposed, including by source (whether natural or anthropogenic), likelihood and/or severity of impact (from imperceptible to terminal), and scope (from personal to cosmic) (Bostrom, 2013). Major (potentially catastrophic and even existential) risks emanating from such taxonomies include natural risks such as asteroid strikes, earthquakes, solar (geomagnetic) storms, supervolcano eruptions, and naturally evolved pandemics. Anthropogenic risks include climate change itself, terrorism, cyber-attacks, and geopolitical conflicts affecting mineral and fuel availability. Each of these could potentially be applied to electricity decarbonization technologies and strategies.
For example, one system-level risk associated with decarbonization of electricity systems is their increasingly “smart” nature, with interconnected meters and appliances gaining the capability to respond to fluctuations in power prices, so as to manage the variability of generation from weather-dependent renewables. Advanced Metering Infrastructures (AMIs) consisting of smart meters, communication networks and data management systems are susceptible to cyber-attacks, calling for focused attention on security measures (Goel and Hong, 2015; Otuoze et al., 2018). There is unlikely to be a risk-free strategy to decarbonization, which is why it is important to identify each strategy's level of vulnerability as well as its cost.
Visualization and scenario discovery methods can help to highlight those mitigation measures and overall mitigation plans that are most and least vulnerable to multiple risks. Such approaches can be applied to both the consideration of individual electricity system technologies, as well as whole electricity systems, which may bring additional system-level risks that are not relevant to individual technologies alone. Figure 2 provides an example of how different technologies, and portfolios of technologies constituting whole electricity systems, can be compared across a number of example risk categories (which is clearly not a comprehensive set of risks). The greatest value of this approach lies in comparing a large number of different electricity decarbonization systems which would meet our climate change goals. Plans to develop these systems could then be compared against each other to identify those which perform best in the face of the realization of multiple risks.
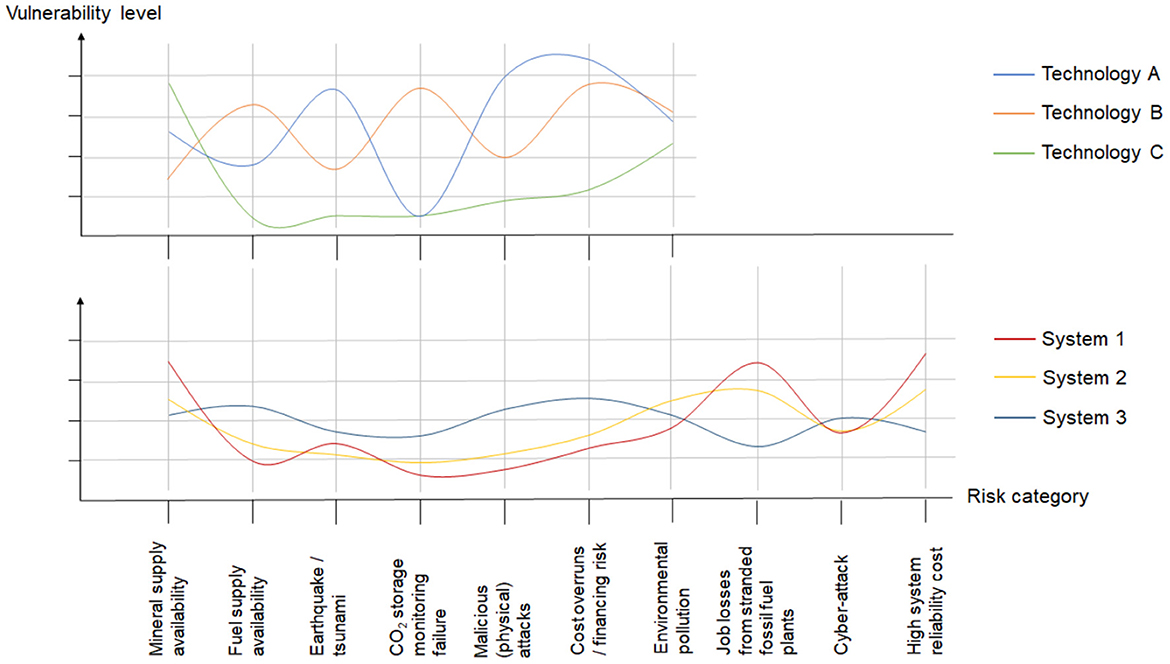
Figure 2. Example mapping of electricity technologies and system vulnerabilities to different risks. Source: Authors, based on ref. Popper (2019). Vulnerability assessment levels are subjective and illustrative only, to demonstrate the process rather than precise outcome of the assessment.
Comparing responses
Once vulnerabilities have been identified, the next step is to use this information to identify new pathways with reduced vulnerabilities. DMDU methods aim to craft such robust, risk-minimizing pathways through combinations of “low regret” options and adaptive strategies designed to evolve over time in response to new information (Haasnoot et al., 2013). Having identified the vulnerabilities of several alternative pathways, decision-makers might come to recognize that the alternatives have complementary risks. For example, a national or regional decarbonization pathway dependent on high shares of wind power might perform well in futures in which pathways dependent on nuclear or carbon capture are vulnerable, perhaps because of a history of cost overruns. On the other hand, a pathway in which cost-effective nuclear, geothermal or other firm power is available might perform well where options to cost-effectively manage power system variability with high penetrations of wind are relatively limited. The least-risk pathway might begin with one pathway, while making preparations that would make it possible to shift in the future to alternative pathways. The least-risk strategy would also monitor for signposts that would indicate the need to shift pathways.
It is important to realize that many (though not all) risks can be mitigated, which should be taken into account when scoring individual mitigation plans' vulnerability to different risks. In some cases risks may require tailored responses, but in other cases appropriate actions may help mitigate many risks. This could happen through particular response and preparedness actions, such as (in the case of electricity sector decarbonization) effective monitoring and regulations of CO2 storage or nuclear fuel waste sites, or through regular stress-tests of electricity systems to perceived risks in order to identify weaknesses and strengthen them, as well as build in back-up and redundancy features.
Moving beyond least-cost
There have already been efforts to explore risks for different electricity system technologies and configurations. For example, the University of Geneva's “RISKMETER” project allows an exploration of different European electricity system technology portfolios across multiple risk-related criteria, including climate change, local air pollution, land use, electricity cost and employment1. In addition, one exercise has used spatially detailed modeling of central European electricity systems to highlight trade-offs between least-cost, maximum equity and maximum renewables shares in the system portfolios, thereby demonstrating how different goals beyond pure cost-minimization can be explored (Sasse and Trutnevyte, 2020).
But why hasn't such an approach already been mainstreamed? We propose that there are three principal reasons. First, there is an inherent logic to least-cost thinking. Why wouldn't societies at national, regional, or indeed global levels, not want to achieve an important objective at the lowest possible cost, given other competing public priorities such as poverty alleviation, improved healthcare and economic recoveries from slowdowns and recessions? Although the distributional consequences—essentially the winners and losers—from a societally least cost approach might be deeply inequitable, in theory corrective redistribution could help to achieve a superior outcome for all (Barr and Barr, 2020), compared to other decarbonization pathways. Notwithstanding the power structures that would in many cases hamper such redistributive efforts, there is thus an attraction to pursuing, and communicating to the public, a least-cost approach.
Secondly, least-cost modeling is relatively easy to embed in modeling tools. The operational research challenge of goal-seeking a least-cost solution, whether through linear programming tools and solvers, or through repeated sampling of possible pathways until a least-cost pathway is identified, is computationally straightforward. This compares to the much messier process of identification of least risk, least regrets or most societally preferred scenarios, accounting for multiple uncertainties around risks (some of which may in fact be unquantifiable uncertainties and unknowns) and multiple stakeholder preferences. There is a deep legacy of modeling tools such as IAMs, which—although being developed in new directions at rapid pace—nevertheless still predominantly stem from least-cost optimization roots (Wilson et al., 2021). Recent approaches such as stochastic optimization (Nikas et al., 2019; Grant et al., 2021) or use of multiple criteria are allowing the exploration of least regrets pathways as well as those that simultaneously fulfill multiple criteria, such as employment increases (van de Ven et al., 2022), reduced inequality (Ferrari et al., 2022), or the achievement of sustainable development goals (van Soest et al., 2019; Fujimori et al., 2020). But it seems very possible that—given the ease with which least-cost pathways still get produced—they could continue to dominate the scenario space.
Thirdly, the policy audience has come to expect least-cost analysis as the way in which they should receive information from model-based policy analyses. Least-cost is also often built into regulatory requirements such as those informed by the U.S. government estimates of the social cost of carbon. As one example, a recent, large-scale DMDU study of Costa Rica's National Decarbonization Plan was generally communicated to senior decision makers and the public in cost terms because this was the language they were most comfortable hearing (Groves et al., 2020).
Nevertheless, with a concerted re-orientation toward stakeholder co-creation of scenarios, as well as the increasing realization that least cost pathways may lead to regret, and actually greater cost, in the long run, there is an opportunity to shift the paradigm. What data and actions would be required? First, less exclusive use of single modeling types like IAMs is to be encouraged. Supplementing IAMs with other models and tools, as well as adding non-IAM pathways to scenario databases, is of critical importance (Gambhir et al., 2022). Such exercises might also require coupling of models at multiple scales, such as a suite of system models now being used for DMDU analyses of the climate resilience of electric grids (Ralston Fonseca et al., 2021), or the structured interplay of simulation models and human red-teaming to identify non-modeled system shocks (Lempert et al., 2002). Such exercises need time, methodological development, and sufficient funding to employ more lengthy stakeholder consultation, scenario discovery and deliberation techniques.
Secondly, better data is required on the potential risks of low-carbon technologies and pathways. In this paper we introduce the concepts around multiple possible risks, but a much more systematic undertaking is required, with reference to historical analysis of what went “wrong” in the past and the use of futures thinking techniques (including gaming, scanning, surveys, and SWOT analysis) (Popper, 2008) to envisage what might go wrong in the future. Again, time and funding will be critical.
Third, the rapid development of new scenario production and exploration methodologies is required, including techniques to produce large scenario ensembles from different models, as well as analyze these ensembles to extract critical trends. Statistical methods including classification and regression tree (CART) and principal component analysis (PCA) would be useful bases, with the application of machine learning to derive robust insights from large datasets of scenario inputs and outputs. Such techniques could help identify that, for example, the contribution of a particular power sector technology to electricity decarbonization is much more, or less, sensitive than others under a range of future scenarios around cost overruns, material supply bottlenecks or adverse climate conditions.
Fourth and finally, the analytic community has to help the audience for policy analysis to expect, appreciate, and demand least-risk information.
Concluding thoughts
In setting out a process to assess climate change mitigation plans in the context of multiple risks, we do not necessarily claim that climate change is the most significant risk facing humanity, nor that every other risk should be seen only through its lens. Rather, we assert that, as a recognized major societal risk which could profoundly affect our future welfare and prosperity, we must address climate change not only with urgency and cost-efficiency, but also in a way that is actively cognizant of multiple other risks which might disrupt our mitigation plans.
A key question not so far addressed is who should undertake this risk assessment of different mitigation plans, in order that they can be compared so as to identify the most resilient plans? We propose that this process will require a number of different stakeholders who are able to draw on their own knowledge of risks and their potential consequences. This includes the analysts and modelers who construct the mitigation pathways around which plans and policies are designed. Crucially, these pathways, and the resulting plans, should be “red teamed” by others not involved in creating them, with the explicit purpose of identifying and assessing the risks that could affect them, including their likelihood, impact if realized and ease of risk mitigation. Here there is a critical role for policy makers who may be well placed to balance multiple public policy priorities so as to think outside of the climate change mitigation box.
It is unclear what other organization(s) would be most appropriate to conduct such red team analyses. For national pathways planning, the task might be taken up by a government or other agency dedicated to the purpose, such as a national climate change committee. Of course, individual businesses and organizations would also be well-advised to consider their own strategies in such a way. In addition, scientific assessment organizations, such as the IPCC (which, although primarily a reviewer of the scientific evidence, also endeavors to place levels of confidence and likelihood on different findings) might also address potential vulnerabilities of alternative mitigation plans at global or regional levels.
Ultimately the most effective method of embedding risk considerations into mitigation planning will be through establishing iterative, deliberative processes that allow assessment, discussion, revision and ultimately agreement around different plans' levels of risk, as well as policy makers', businesses', and societies' preferences around the most resilient plans identified. This is not an easy, nor rapid, task, but as recent crises demonstrate, it is an essential undertaking.
Data availability statement
Publicly available datasets were analyzed in this study. This data can be found here: https://tntcat.iiasa.ac.at/SspDb/dsd?Action=htmlpage&page=10.
Author contributions
AG and RL authors contributed equally to all aspects of this manuscript, including conceptualization, research, and writing. Both authors contributed to the article and approved the submitted version.
Funding
AG thanks UK Research and Innovation, Project 10045455, as part of the Horizon Europe European Commission Project IAM COMPACT, Grant No. 101056306, for supporting this work. RL was funded by the RAND Pardee Center for Longer Range Global Policy and the Future Human Condition.
Conflict of interest
RL is affiliated with the RAND Corporation, a non-profit public policy research organization.
The remaining author declares that the research was conducted in the absence of any commercial or financial relationships that could be construed as a potential conflict of interest.
Publisher's note
All claims expressed in this article are solely those of the authors and do not necessarily represent those of their affiliated organizations, or those of the publisher, the editors and the reviewers. Any product that may be evaluated in this article, or claim that may be made by its manufacturer, is not guaranteed or endorsed by the publisher.
Footnotes
1. ^UNIGE. RISKMETER. Available online at: www.riskmeter.ch
References
Barr, N., and Barr, N. (2020). The Economics of the Welfare State. (Oxford, UK: Oxford University Press).
Bostrom, N. (2013). Existential risk prevention as global priority. Glob. Policy 4, 15–31. doi: 10.1111/1758-5899.12002
Byers, E., Krey, V., Kriegler, E., Riahi, K., Schaeffer, R., Kikstra, J., et al. (2022). “AR6 Scenarios Database,” in International Institute for Applied Systems Analysis.
Ferrari, L., Carlino, A., Gazzotti, P., Tavoni, M., and Castelletti, A. (2022). From optimal to robust climate strategies: expanding integrated assessment model ensembles to manage economic, social, and environmental objectives. Environ. Res. Lett. 17, 084029. doi: 10.1088/1748-9326/ac843b
Fujimori, S., Hasegawa, T., Takahashi, K., Dai, H., Liu, J. Y., Ohashi, H., et al. (2020). Measuring the sustainable development implications of climate change mitigation. Environ. Res. Lett. 15, 085004. doi: 10.1088/1748-9326/ab9966
Gambhir, A., Ganguly, G., and Mittal, S. (2022). Climate change mitigation scenario databases should incorporate more non-IAM pathways. Joule 6, 2663–2667. doi: 10.1016/j.joule.2022.11.007
Goel, S., and Hong, Y. (2015). “Security Challenges in Smart Grid Implementation,” in Smart Grid Security (London: Springer) 1–39. doi: 10.1007/978-1-4471-6663-4_1
Grant, N., Hawkes, A., Mittal, S., and Gambhir, A. (2021). Confronting mitigation deterrence in low-carbon scenarios. Environ. Res. Lett. 16, 064099. doi: 10.1088/1748-9326/ac0749
Groves, D. G., Syme, J. C., Molina-Perez, E., Calvo-Hernandez, C., Víctor-Gallardo, L. F., Godinez-Zamora, G., et al. (2020). The Benefits and Costs of Decarbonizing Costa Rica's Economy: Informing the Implementation of Costa Rica's National Decarbonization Plan Under Uncertainty. Arlington, VA: RAND. doi: 10.7249/RRA633-1
Haasnoot, M., Kwakkel, J. H., Walker, W. E., and ter Maat, J. (2013). Dynamic adaptive policy pathways: A method for crafting robust decisions for a deeply uncertain world. Glob. Environ. Change 23, 485–498. doi: 10.1016/j.gloenvcha.2012.12.006
IEA (2022). Gas Market Report, Q4-2022, 1–80. Available online at: https://www.iea.org/reports/gas-market-report-q4–2022 (accessed March 01, 2023).
IPCC (2022). Climate change 2022: Mitigation of climate change. Working Group III Contribution to the IPCC Sixth Assessment Report. (Cambridge University Press, Cambridge, United Kingdom and New York, NY, USA).
Kwakkel, J. H., and Haasnoot, M. (2019). “Supporting DMDU: A Taxonomy of Approaches and Tools,” in Decision Making under Deep Uncertainty: From Theory to Practice, eds. V. A. W. J., Marchau, W. E., Walker, P. J. T. M., Bloemen, and S. W., Popper (Springer International Publishing) 355–374. doi: 10.1007/978-3-030-05252-2_15
Lempert, R., Popper, S., and Bankes, S. (2002). Confronting surprise. Soc. Sci. Comput. Rev. 20, 420–440. doi: 10.1177/089443902237320
Lempert, R. J., and Trujillo, H. R. (2018). Deep Decarbonization as a Risk Management Challenge. Arlington, VA: RAND. doi: 10.7249/PE303
Masson-Delmotte, V., Zhai, P., Pirani, A., Connors, S. L., Péan, C., Berger, S., et al. (2021). “Climate change 2021: the physical science basis,” in Contribution of working group I to the sixth assessment report of the intergovernmental panel on climate change, 2. (Cambridge University Press, IPCC).
Net Zero Tracker (2022). Net Zero Stocktake 2022, 1–52. Available online at: https://ca1-nzt.edcdn.com/Net-Zero-Tracker/Net-Zero-Stocktake-Report-2022.pdf?v=1655074300 (accessed March 01, 2023).
Nikas, A., Doukas, H., and Papandreou, A. (2019). “A detailed overview and consistent classification of climate-economy models,” in Understanding Risks and Uncertainties in Energy and Climate Policy: Multidisciplinary Methods and Tools for a Low Carbon Society, eds. H., Doukas, A., Flamos and J., Lieu (Springer International Publishing) 1–54. doi: 10.1007/978-3-030-03152-7_1
Otuoze, A. O., Mustafa, M. W., and Larik, R. M. (2018). Smart grids security challenges: Classification by sources of threats. J. Electr. Syst. Inf. Technol. 5, 468–483. doi: 10.1016/j.jesit.2018.01.001
Pinker, S. (2018). Enlightenment Now: The Case for Reason, Science, Humanism, and Progress. New York, NY: Viking, an imprint of Penguin Random House LLC.
Plackett, B. (2022). Why France's nuclear industry faces uncertainty. Nature 75, 102008. doi: 10.1038/d41586-022-02817-2
Popper, R. (2008). “Foresight methodology,” in The Handbook of Technology Foresight: Concepts and Practice, ed. L. Georghiou (Edward Elgar Publishing). doi: 10.4337/9781781008768.00012
Popper, S. W. (2019). Robust decision making and scenario discovery in the absence of formal models. Futur. Foresight Sci. 1, e22. doi: 10.1002/ffo2.22
Ralston Fonseca, F., Craig, M., Jaramillo, P., Bergés, M., Severnini, E., Loew, A., et al. (2021). Climate-induced tradeoffs in planning and operating costs of a regional electricity system. Environ. Sci. Technol. 55, 11204–11215. doi: 10.1021/acs.est.1c01334
Riahi, K., Van Vuuren, D. P., Kriegler, E., Edmonds, J., O'neill, B. C., Fujimori, S., et al. (2017). The Shared Socioeconomic Pathways and their energy, land use, and greenhouse gas emissions implications: An overview. Glob. Environ. Change 42, 153–168. doi: 10.1016/j.gloenvcha.2016.05.009
Rogelj, J., Popp, A., Calvin, K. V., Luderer, G., Emmerling, J., Gernaat, D., et al. (2018). Scenarios towards limiting global mean temperature increase below 1.5 °C. Nat. Clim. Change 8, 325–332. doi: 10.1038/s41558-018-0091-3
Rosling, H., Rosling, O., and Rönnlund, A. R. (2018). Factfulness: Ten Reasons We're Wrong About the World - And Why Things are Better Than You Think, 1st Edn. New York, NY: Flatiron Books.
Sasse, J. P., and Trutnevyte, E. (2020). Regional impacts of electricity system transition in Central Europe until 2035. Nat. Commun. 11, 4972. doi: 10.1038/s41467-020-18812-y
Simpson, N. P., Mach, K. J., Constable, A., Hess, J., Hogarth, R., Howden, M., et al. (2021). A framework for complex climate change risk assessment. One Earth 4, 489–501. doi: 10.1016/j.oneear.2021.03.005
van de Ven, D. J., Nikas, A., Koasidis, K., Forouli, A., Cassetti, G., Chiodi, A., et al. (2022). COVID-19 recovery packages can benefit climate targets and clean energy jobs, but scale of impacts and optimal investment portfolios differ among major economies. One Earth 5, 1042–1054. doi: 10.1016/j.oneear.2022.08.008
van Soest, H. L., van Vuuren, D. P., Hilaire, J., Minx, J. C., Harmsen, M. J., Krey, V., et al. (2019). Analysing interactions among sustainable development goals with integrated assessment models. Glob. Transit. 1, 210–225. doi: 10.1016/j.glt.2019.10.004
Van Vuuren, D. P., Stehfest, E., Gernaat, D. E., Doelman, J. C., Van den Berg, M., Harmsen, M., et al. (2017). Energy, land-use and greenhouse gas emissions trajectories under a green growth paradigm. Glob. Environ. Change 42, 237–250. doi: 10.1016/j.gloenvcha.2016.05.008
Wilson, C., Guivarch, C., Kriegler, E., Van Ruijven, B., Van Vuuren, D. P., Krey, V., et al. (2021). Evaluating process-based integrated assessment models of climate change mitigation. Clim. Change 166, 3. doi: 10.1007/s10584-021-03099-9
World Economic Forum (2023). Global Risks Report 2023. Available online at: https://www.weforum.org/reports/global-risks-report-2023/ (accessed March 01, 2023).
Keywords: climate change mitigation, decision making under deep uncertainty, low-carbon electricity, global risks, integrated assessment models (IAMs), climate risk management
Citation: Gambhir A and Lempert R (2023) From least cost to least risk: Producing climate change mitigation plans that are resilient to multiple risks. Front. Clim. 5:1149309. doi: 10.3389/fclim.2023.1149309
Received: 21 January 2023; Accepted: 15 March 2023;
Published: 17 April 2023.
Edited by:
Adrian Gault, London School of Economics and Political Science, United KingdomReviewed by:
Paulina Jaramillo, Carnegie Mellon University, United StatesCopyright © 2023 Gambhir and Lempert. This is an open-access article distributed under the terms of the Creative Commons Attribution License (CC BY). The use, distribution or reproduction in other forums is permitted, provided the original author(s) and the copyright owner(s) are credited and that the original publication in this journal is cited, in accordance with accepted academic practice. No use, distribution or reproduction is permitted which does not comply with these terms.
*Correspondence: Ajay Gambhir, YS5nYW1iaGlyQGltcGVyaWFsLmFjLnVr