- 1School of Natural Sciences, College of Science and Engineering, University of Galway, Galway, Ireland
- 2Ryan Institute, University of Galway, Galway, Ireland
- 3School of Biological and Chemical Sciences, College of Science and Engineering, University of Galway, Galway, Ireland
Anthropogenic changes to our Earth’s climate system are amongst the most pressing challenges facing humankind. Advances in satellite systems for earth observation are revolutionizing our ability to monitor and assess environmental changes, manage natural resources and respond to global challenges, including climate change and disaster management. Here we review the potential for satellite Earth Observation and artificial intelligence solutions to accelerate climate action at scale. The satellite industry already has extensive expertise in emergency communication and is a critical element of any comprehensive global emergency warning and messaging infrastructure to support unserved populations in remote and rural regions. We review the literature demonstrating how current technologies and methodologies that have been developed to leverage satellite-based information and the critical role that satellite data plays to support vulnerable populations in Low-and middle-income countries. Whilst previous reviews focus on isolated climate indicators, our systematic review demonstrates how the fusion of remote sensing, AI-driven analytics and geospatial data can provide a more comprehensive, real-time assessment of climate vulnerabilities and adaptive capacities. We situate our review within established global frameworks, such as: the Sustainable Development Goals (SDGs) addressing goals related to climate action (SDG 13) and zero hunger (SDG 2). The Sendai Framework for Disaster Risk Reduction, the Paris Agreement: supporting Nationally Determined Contributions (NDCs) with data-driven strategies and the delivery of the Early Warnings for All initiative which calls for the implementation of early warning systems to protect all global populations by 2027.
1 Introduction
Climate change encompasses multiple environmental and climatic shifts, temperature alterations, precipitation patterns, sea level rise, and the frequency and intensity of extreme weather. “The economic consequences are substantial, affecting industries such as agriculture, fisheries, and tourism, and contributing to global inequality as vulnerable communities bear the brunt of the impacts. Mitigating these effects requires urgent, coordinated efforts to reduce GHGE, adapt to current changes, and foster resilience in vulnerable regions” (Jha and Dev, 2024). Populations living in poverty are most vulnerable and, typically, most at risk. Peer-reviewed studies are the cornerstone of evidence for climate change action. This review examines how climate resilience is measured in Low- and Middle-Income Countries (LMICs) using Advanced Analytical Techniques and Satellite Data. By establishing a scalable and replicable framework, the review both advances the scientific discourse on climate resilience and also generates a multiplier effect—enabling policymakers, researchers, and development agencies to apply these methodologies across diverse geographic and socioeconomic contexts. The review facilitates the integration of research findings into actionable policies. This approach fosters more precise, data-driven interventions, accelerating climate action from local initiatives to global strategies.
The conceptualization of climate resilience in LMICs extends beyond technical frameworks to encompass socio-economic and political realities and requires a shift from reactive measures to proactive, systemic approaches that address underlying vulnerabilities and promote sustainable development (Krichen and Abdalzaher, 2024). A people-centered approach is particularly crucial in the challenging context of conflict and post-conflict situations, where “natural hazards can precipitate or intensify other hazards and societal crises, making the need for a multi-hazard EWS approach even more critical” (GFDRR/The World Bank, 2024). In 2024, extreme weather events—including tropical cyclones, floods, droughts, and other hazards—resulted in the highest displacement of people recorded in the past 16 years. These events also exacerbated food crises and inflicted substantial economic losses. By integrating preparedness, adaptive capacity, and transformation, LMICs can build resilience that not only mitigates climate risks but also empowers communities to thrive in a changing world. Ultimately, fostering climate resilience in these contexts is not just an environmental imperative but a developmental necessity to achieve global equity and sustainability.
Advances in satellite technologies and remote sensing missions for Earth Observation (EO) have significantly improved our ability to monitor and understand our planet’s complex biophysical systems, including weather and climate change. These advancements are largely driven by innovations in AI capabilities and data processing speed. Additionally, improved GPS-enabled and Internet of Things (IoT) devices have increased the volume of EO data available from both public and commercial remote sensing constellations. A novel IoT system-based Earthquake early-warning system “using a deep learning model integrating AE and CNN has been utilized to rapidly calculate EQ parameters … [with] minimal error between the predicted EQ parameters and their manual solution to the same events that represent the most accurate calculation done by the expert seismologists. Moreover, the proposed approach is designed to be integrated with an IoT system” (Abdalzaher et al., 2021). However, the volume of data being generated often outpaces analytical capacities due to storage limitation, computational power, real-time processing capabilities and human expertise. Addressing these bottlenecks will require investment in technology, infrastructure and capacity-building, particularly in regions where EO data has the most potential for significant impact.
Merging satellite imagery with Deep Learning techniques, such as Convolutional Neural Networks (CNNs), Recurrent Neural Networks (RNNs), Generative Adversarial Networks (GANs), and transformers, can unlock the full potential of satellite imagery. These models provide powerful tools for image analysis, time-series prediction and data segmentation and a major opportunity to effectively interpret and extract meaningful insights from satellite imagery and hence inform and measure climate mitigation and adaptation (Schneider et al., 2023). To strengthen international cooperation and development of these space-related technologies the EU Agency for the Space Programme (EUSPA) and the ‘UN Office for Outer Space Affairs’ (UNOOSA) formalized their commitment to advance the implementation of the “Space2030” Agenda. Adopted by the UN General Assembly, the strategy provides a “guide for policymakers and legislators in emerging economies entering the space sector or advancing their space activities” (UNOOSA, 2024).
Satellite systems currently provide reliable emergency messaging across all geolocations on the planet and a robust service during disaster situations if traditional communications are rendered inoperable. They cover more than 99% of the world’s population, providing vulnerable communities in LMICs with tools and resources to improve resilience against environmental and socio-economic pressures. As the WEF note, “By 2030, the economic opportunity afforded by EEO insights is projected to surpass $700 billion while directly contributing to the abatement of 2 gigatons of greenhouse gases annually, marking an era of unprecedented potential in monitoring and analyzing Earth from space” (WEF, 2023). However, the ability of EO to generate value is closely tied to regional technological readiness. As outlined in Figure 1, the projected growth of these industries within each region offers a global perspective on the future potential of Earth data particularly in LMICs. “While North America and Europe are currently global leaders in EO adoption, future growth in these regions is projected to remain steady, while regions with comparatively lower adoption are projected to experience approximately threefold expansion from 2023 to 2030. As outlined in the figure the Asia-Pacific region is poised to capture the largest share of EO’s value in this period, reaching a potential value of $315 billion, while Africa and South America are positioned to realize the largest percentage growth” (WEF, 2024). These findings highlight the critical need to address foundational challenges associated with scaling efforts.
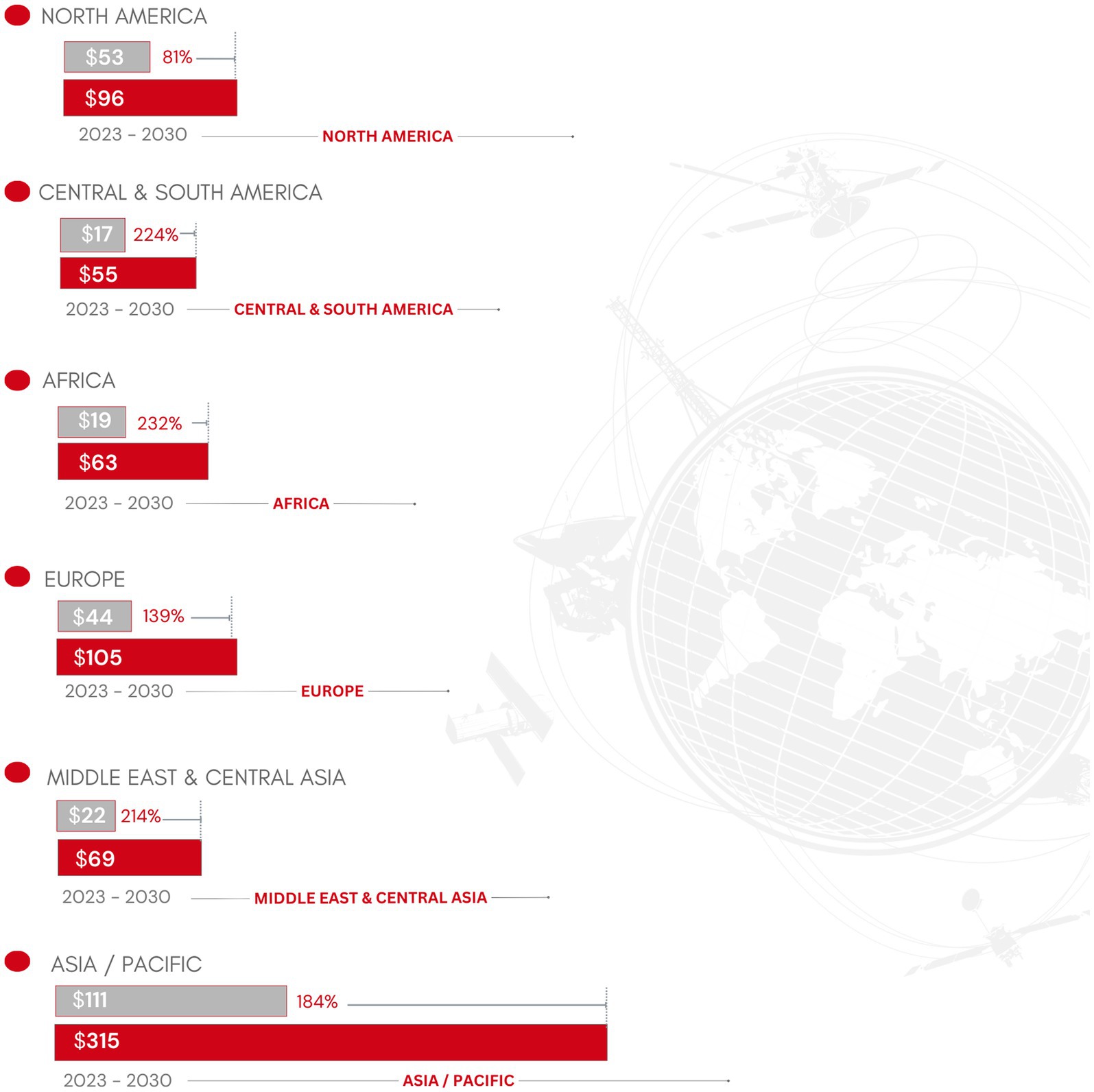
Figure 1. Earth observation: Projected regional growth from 2023 to 2030, based on WEF data (WEF, 2024).
For example, the ECV Inventory is an open resource to explore existing and planned data records from space agency-sponsored activities and provides a unique source of information on CDRs available internationally. Access links to the data are provided within the inventory, alongside details of the data’s provenance, integrity and application to climate monitoring. A summary table comparing satellite systems, their applications, and relevance for LMICs can be found in Supplementary material (Annex B), comparing over 1250 data sources and their associated applications, all of which are open source.
Established global frameworks such as Early Warnings for All (EW4ALL) call for shared action to implement early warning systems to protect the global population by 2027. The EW4ALL co-leads are the World Meteorological Organization (WMO) and the United Nations Office for Disaster Risk Reduction (UNDRR) (Disaster Risk Reduction, 2023). These organizations stress the importance of EO for collaboration to combat climate shocks. An exploration and mapping of MHEWS-relevant programs and initiatives per country and region is provided (Annex C). However, the resources needed for analysis and interpretation of satellite imagery and machine learning applications pose challenges in cost, access and implementation in low resource settings: The current lack of infrastructure, access and inclusiveness hinders LMICs from effectively harnessing satellite remote sensing to inform decisions and develop evidence-based policies. Digital technology-driven solutions from the satellite industry, connected to terrestrial (mobile wireless industry, device manufacturers) could transform the provision of critical information and advance both climate action and MHEWS generally. However, greater use of EO data raises concerns regarding data privacy, equity in access, and potential risks of reliance on foreign satellite operators or third-party data providers resulting in vendor “lock in” and disruptions in service, particularly in LMICs (Kuffer et al., 2020). This overreliance could limit the autonomy of LMICs in managing their own environmental, agricultural, and disaster-related challenges, as they may lack the capacity to independently collect, analyze, and interpret EO data. Privacy safeguards must be integrated into EO technologies to prevent unintended consequences. The digital divide amplifies these challenges, as poor internet infrastructure in LMICs hampers downloading and analyzing large satellite datasets. Additionally, the high costs of advanced EO products and software limit LMIC governments’ ability to engage with these data. Addressing these issues requires policy interventions and technical innovations: international cooperation is essential to ensure equitable access to EO data via affordable or free access to LMICs. Capacity-building initiatives in these regions are needed to bridge knowledge gaps and enable local stakeholders to effectively use EO data. Open data platforms and improved data-literacy in LMICs, including among policymakers, are critical for equitable global data access.
The 2030 Agenda for Sustainable Development has increased the need for common standard indicators that effectively measure global progress. Satellite networks can baseline SDG indicators, while satellite surveillance and AI offer datasets that complement traditional surveys and national reporting. If multilateral frameworks for using sensing indicators can be agreed between governments, satellite remote sensing data could be integrated with multilateral policy processes, e.g., the Conferences of the Parties (COPs) to the United Nations Framework Convention on Climate Change (UNFCCC), the Convention on Biological Diversity (CBD), and the United Nations Convention to Combat Desertification (UNCCD). Such indicators could also be integrated into the Lima-Paris Action Agenda (LPAA) or Marrakech Partnership for Global Climate Action (MPGCA). There are also potential collaboration with initiatives led by or involving non-state actors such as the Oil and Gas Climate Initiative (OGCI), Sustainable Energy for All (SE4ALL), and the Resilient Cities Acceleration Initiative.
2 Methodology
Our review focuses specifically on the long-term feasibility and sustainability of satellite remote sensing systems, as well as identifying gaps in the existing evidence base in the literature. It seeks to offer a unique contribution to the field by synthesizing current research, identifying gaps, and proposing actionable recommendations, thereby facilitating a cohesive understanding of this complex topic. Integration of the Preferred Reporting Items for Systematic Reviews and Meta-Analyses (PRISMA) framework ensured methodological rigor, transparency, and reproducibility during the review process. The PRISMA Flow Diagram (Figure 2) is a Graphical Abstract (GA) that depicts the sequential process of information through these phases and outlines the inclusion criteria. A comprehensive search strategy was systematically applied across multiple databases and literature sources. The screening phase documented study retrieval, inclusion, and exclusion, with justifications based on predefined criteria. Full-text reviews minimized selection bias, and independent reviewers cross-validated selections, resolving discrepancies through a third reviewer.
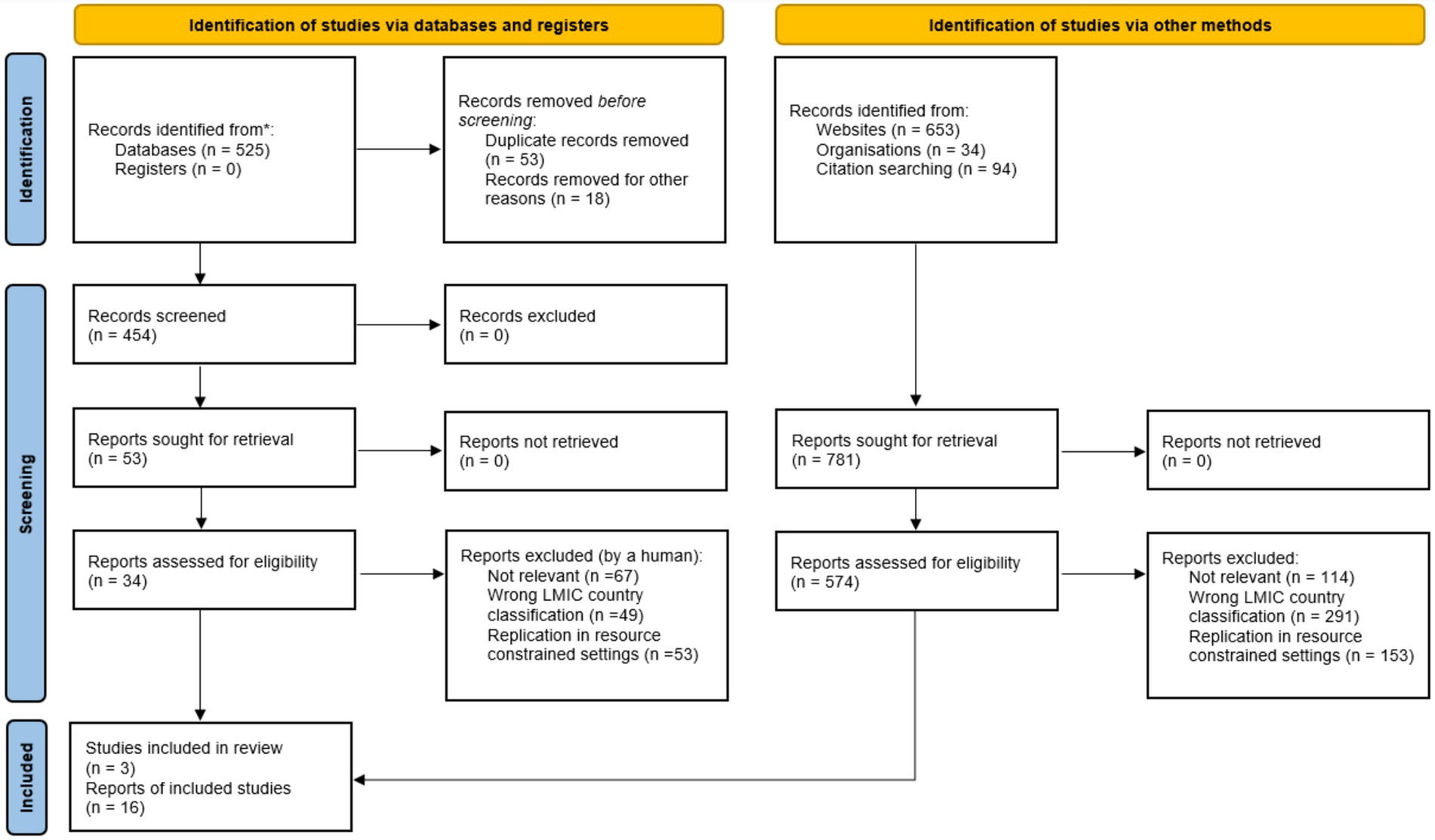
Figure 2. Data extraction process using the PRISMA 2020 flow diagram (Page et al., 2021).
2.1 Development of analytical frameworks and applications of satellite data
We critically evaluate current techniques employed in measuring climate resilience, including machine learning, geospatial modeling, and econometric analyses. By assessing their strengths, limitations, and applicability in LMIC contexts, we refine our understanding of the methodological robustness required for accurate resilience assessment, and hence the standardization needed to enable comparability and scalability. We also identify cutting-edge applications of satellite data, critique its limitations, and highlight emerging tools for resolution and accessibility. Ultimately, we aim to help democratize the use of satellite data by recommending cost-effective, open-source solutions.
2.2 Context-specific challenges and identification of research gaps
LMICs face unique challenges such as limited ground-truth data, socio-economic vulnerabilities, and diverse climate risks. We identify how analytical techniques and satellite data can be tailored to these specific contexts, underscoring the importance of integrating local knowledge, stakeholder engagement, and interdisciplinary approaches for more effective resilience measurement. Research gaps include the underrepresentation of socio-cultural dimensions of resilience and the lack of longitudinal studies tracking resilience over time. This provides a roadmap for innovations in data integration, policy alignment, and multi-scale analyses. In conclusion, we aim to provide a catalyst for climate resilience measurement in LMICs and facilitate informed decision-making and resource allocation, empowering policymakers, researchers, and practitioners to collaboratively address climate challenges in vulnerable regions.
2.3 The PRISMA process and search strategy
Our systematic review employed internationally recognized literature search methodologies and includes a balanced, comprehensive, and critical view of the research area, following the PRISMA checklist (Page et al., 2021). The review was segmented into five categories: “literature search, inclusion criteria, methodological quality assessment, data extraction, and data analysis” (Page et al., 2021). The quality of each included study was evaluated, and findings critically appraised. The process comprised: identifying relevant records, selecting eligible studies, assessing the risk of bias, extracting data, and conducting a qualitative synthesis of the included studies. A meta-analysis was performed to integrate data from all pertinent studies to provide a more precise estimate than individual study-derived estimates. Results and conclusions were then summarized. Pertinent studies were drawn from peer-reviewed literature concerning interventions that utilize environmental informatics and satellite remote sensing applications (see search terms and strings; Table 1). Scopus, Web of Knowledge and IEEE Xplore were searched for EO interventions in LMICs; all studies underwent independent evaluation by the primary author, [PC]. Any issues regarding eligibility were addressed through discussions with a secondary reviewer [MG]. The search covered published studies in English (whilst English remains the predominant language for international scientific collaboration, many agencies do publish in their national languages with translation to facilitate multilingual access to satellite data and reports). Further sources were independently gathered by the primary author to identify studies that fulfilled the inclusion criteria in Table 1. Information from verified literature beyond traditional academic publishing was also scanned to gain region-specific information. Final searches were performed on January 24, 2025.
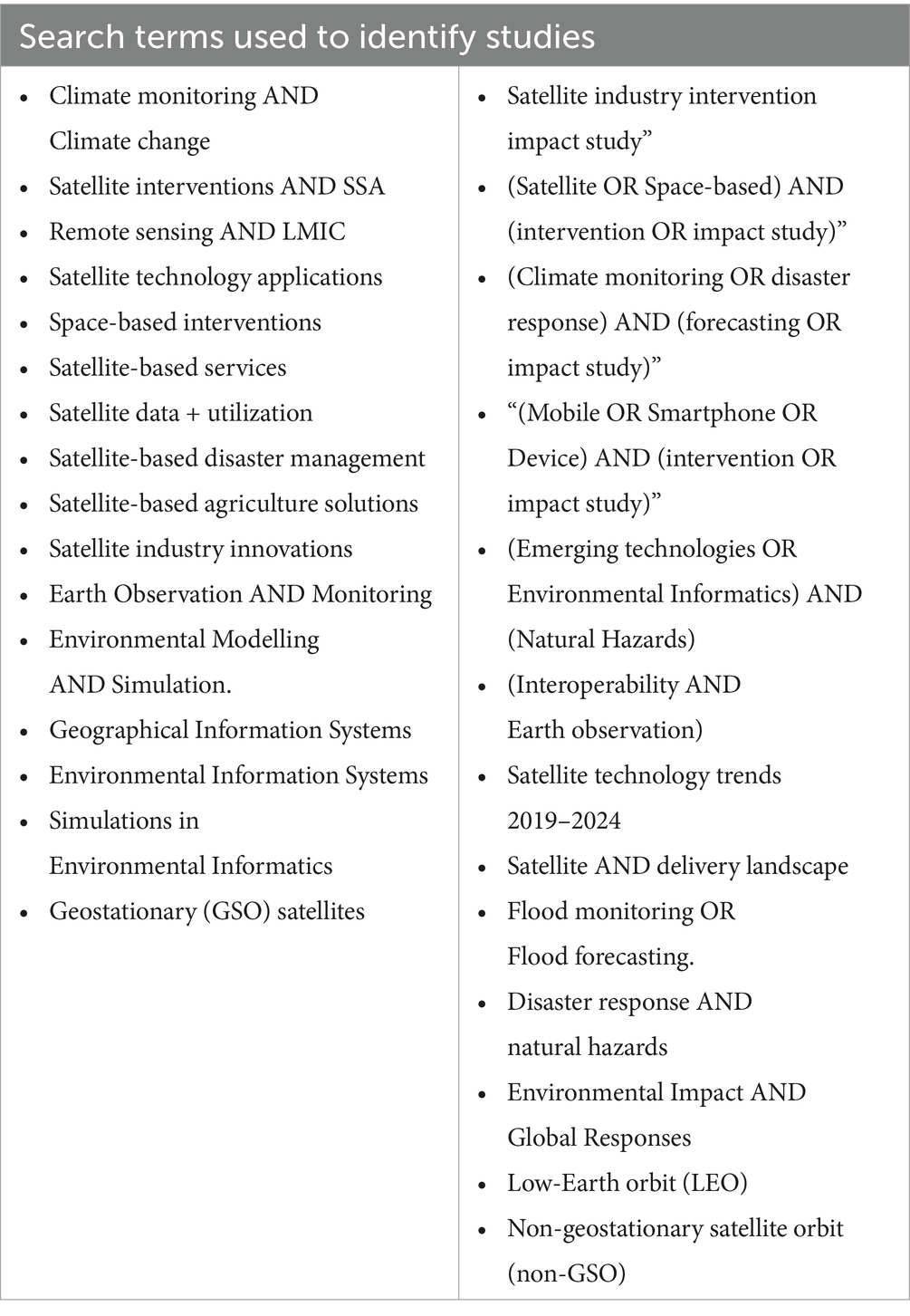
Table 1. Search terms used to identify studies of interventions using satellite and EO technologies.
2.4 Regional selection and geographical focus
This systematic review aims to investigate good practices and successful examples of satellite remote sensing projects to deliver actionable climate and weather data, specific to Low and Middle-Income Countries (LMICs) where this information is most essential but currently is not widely accessible: “According to this year’s report on the Global Status of Multi-Hazard Early Warning Systems, the world is at its highest levels of early warning coverage since 2015. That said, progress remains uneven. Half of the countries in Africa and only 40 per cent of countries in the Americas and the Caribbean have reported the existence of Multi-Hazard Early Warning Systems” (United Nations Office for Disaster Risk Reduction and World Meteorological Organization, 2024). The LMIC classification system, utilized by international organizations such as the World Bank, categorizes nations based on their Gross National Income (GNI) per capita. Designated LMICs have a GNI per capita ranging from US$ 1,046 to US$ 4,095.
2.5 Review inclusion, exclusion and limitations
The criteria for inclusion and exclusion in the systematic review comprised of control conditions, study methodology, acceptability and effective outcomes. Predefined criteria were:
a) The identification of remote sensing climate interventions reporting positive outcomes and potential for future replication in resource constrained settings.
b) A focus on vulnerable communities in LMICs where satellite information is needed but often not widely provided due to political or technical capacity.
The review identified that while the literature contains numerous examples, the implementation of satellite observations is rarely conducted in a systematic manner due to the uncertainty of lasting continuity of EO missions. This may lead to underreporting within the existing literature. In addition, an additional constraint arises from the rapidly advancing field of satellite remote sensing. The technology and tools that support many of these projects are under continuous development, making project evaluation reflective only of past performance resulting in significant differences from estimated performance data. This underscores the necessity for timely strategic and impact analyses. The exclusion criteria for this review were designed to ensure the relevance and quality of the included studies (Figure 2). Studies lacking empirical data or those focused solely on conceptual models were excluded, as they do not provide actionable insights for measuring climate resilience. Research on high-income countries was excluded unless it involved comparative analyses with low-and middle-income countries, to maintain focus on the target context. Duplicate studies were removed to avoid redundancy, and non-English publications were excluded unless translation was available, as language barriers could limit the study’s accessibility. These criteria ensured a rigorous selection process. It is important to note that the scope of this paper does not permit an exhaustive account of all space agencies and their missions: each country has its own unique aims in the space sector, and many programmes, particularly from emerging spacefaring nations, may not gain widespread attention.
2.6 Systematic review goal and objectives
We used an item checklist, and a four-phase workflow process as outlined in the PRISMA flow diagram, where Figure 2 illustrates the selection method whereby titles and abstracts were retrieved. The following specific objectives guided the review process:
a) Assessing the integration of satellite systems and EO data for multi-hazard early warning systems in LMIC settings.
b) Identification of global capabilities in Earth Observation for climate mitigation and adaptation reporting positive outcomes.
c) Monitoring standards, guidance and good practice for implementation of Earth Observation (EO) data.
d) Enabling advanced analytical techniques and satellite data interpretation for LMICs.
Much of the literature that was screened in this review demonstrates that investment in space and services components form the foundation for effective implementation of the four essential pillars of the “Multi-Hazard Early Warning Systems (MHEWS); Pillar 1: Disaster risk knowledge. Pillar 2: Detection, observation, monitoring, analysis and forecasting of hazards. Pillar 3: Warning dissemination and communication. Pillar 4: Preparedness and response. It is acknowledged that this is a crucial decade for the future of geospatial technologies, observation, and geo-information services” (WMO, 2023). However, we found that evidence of sharing scientific models and data resources is lacking: North–South, South–South and triangular regional and international cooperation in infrastructure and utilization of these technologies for actionable data and environmental knowledge exchange is needed at a global level.
3 Results and discussion
The review’s innovative contribution to the specialized scientific literature on climate resilience promotes the use of precise, data-driven approaches to enhance the effectiveness of climate resilience strategies. The review results underscore the need for policymakers, researchers, and development agencies to apply real time satellite data, earth observation, metrological and environmental methodologies to deal with the impact of climate change. The review findings support the acceleration of climate action by presenting both local initiatives and global strategies, ensuring that interventions are both context-specific and scalable.
3.1 The need and opportunity for satellite systems and EO data for multi-hazard early warning system (MHEWS) in LMICs
Those adversely impacted by climate-related disasters, especially in LMICS, and associated economic losses, are increasing. Experience gained from implementing people-centric, early warning, alert and response systems is essential to mitigate harm to individuals, assets, and livelihoods. By initiating early action plans that are well prepared and rigorously tested, such systems minimize disaster impacts and are among the most effective and economically viable methods for risk reduction and climate adaptation. As many climate hazards transcend national borders, disaster response measures must reflect this. Enhancing early warning systems to address the needs of both current and future generations necessitates international cooperation and just transition principles to protect those who have contributed least to the climate crisis. Despite their proven efficacy, as of 2024, only “half of all countries worldwide have adequate multi-hazard early warning systems” (WMO, 2025). Such protective coverage is even more limited in developing nations, with less than half of the Least Developed Countries and only one-third of Small Island Developing States equipped with such systems. The integration of satellite systems and EO data holds significant promise in advancing risk management strategies and capabilities, particularly in LMICs.
By integrating satellite data with AI and Deep Learning, early warning systems can deliver critical insights into environmental hazards, allowing proactive risk management (Jones and Brown, 2018). Additionally, the use of satellite imagery enables real-time disaster event monitoring, aiding swift response efforts (Wilson and Patel, 2020). The wide scope of examples identified in the peer reviewed and grey literature is indicative of the potential to apply a range of satellite applications to effectively deliver risk mitigation (Table 2). While existing reviews were informative, it was found that despite the implementation of projects within low resource settings, there is a lack of “comprehensive data for evidence-based research and decision-making is crucial but scarce” (Barteit et al., 2023; Table 2). It is necessary to provide evidence beyond technological integration to encompass stakeholder coordination and innovation fostering multi-sectoral collaboration to maximize the impact of early warning systems (Albris et al., 2020). Such collaborative approaches can promote knowledge-sharing and capacity-building among diverse stakeholders, including government agencies, NGOs, and local communities (Luna, 2017).
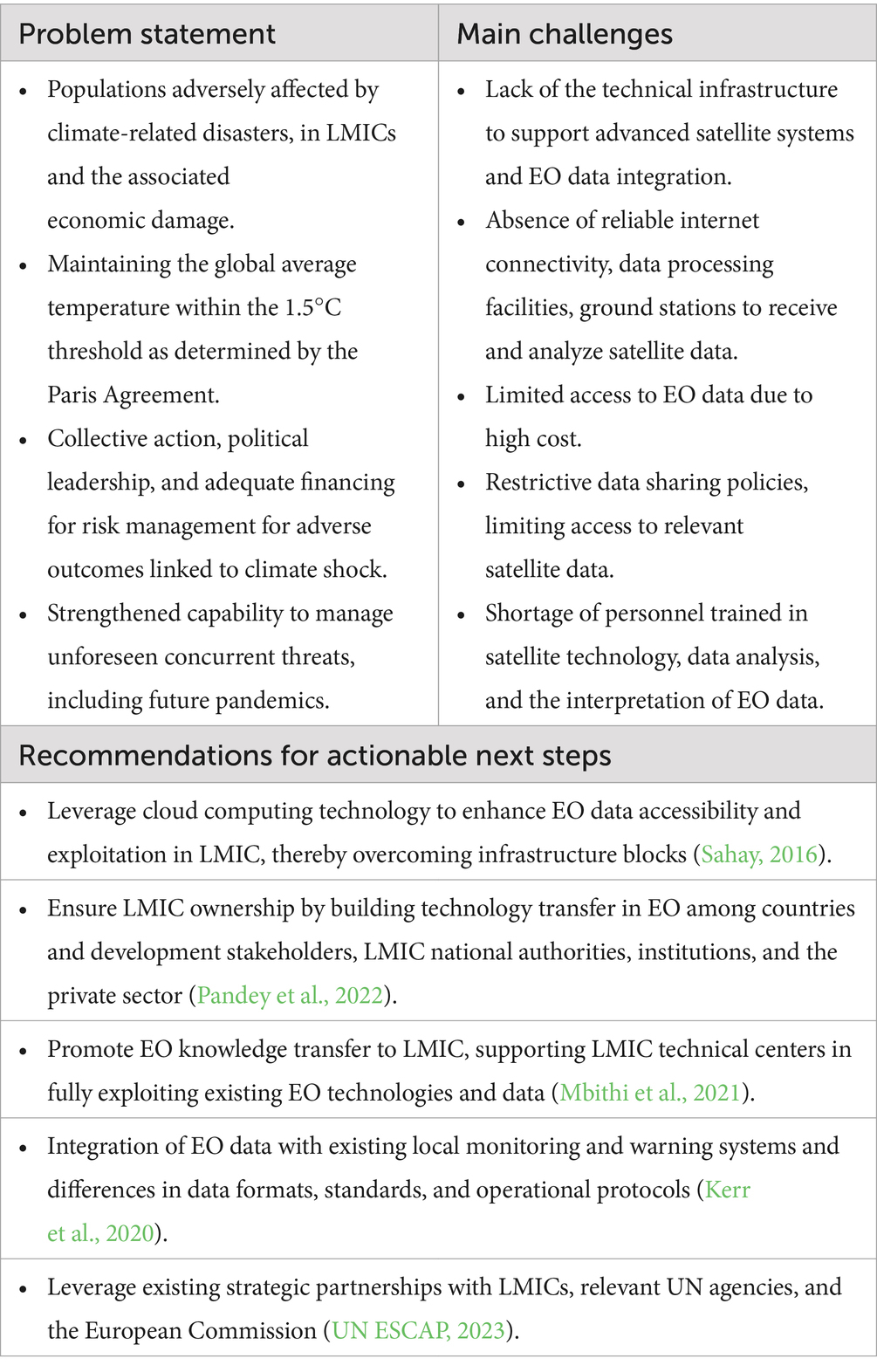
Table 2. Assessing the integration of satellite systems and EO data for multi-hazard early warning systems in LMIC settings.
Remotely-sensed data for drought and food insecurity assessments includes the use of rainfall, temperature, and satellite images for anomaly assessment and early warning (Senay et al., 2015; Sudianto et al., 2023; Yildirim et al., 2022). Existing assessments have used various indices derived from remote sensing data in combination with precipitation and temperature data to explore environmental anomalies and indicate disasters. These indices include Precipitation Anomaly Percentage (PAP), Temperate Condition Index (TCI), Palmer Drought Severity Index (PDSI), Normalized Temperature Anomaly Index (NTAI), Soil Water Deficit Index (SWDI), Vegetation Condition Index (VCI), Normalized Vegetation Anomaly Index (NVAI) and Normalized Difference Vegetation Index (NDVI). For instance, remote sensing-derived indices such as NDVI were used as an indicator of vegetation/crop health, exploring their relationships with environmental variables including soil moisture, precipitation, and temperature anomalies (Ghazaryan et al., 2020). The integration of remote sensing data with weather data allows for the exploration of climatic stress and implications on crop productivity, forest fire, and rangeland availability that enable early warning (Antonelli et al., 2023; Krishnamurthy et al., 2020; van Ginkel and Biradar, 2021).
The availability of open-access data has significantly enhanced the utilization of remote sensing technologies for Early Warning Systems. Initiatives such as the European Space Agency’s EO AFRICA initiative promotes the sustainable integration of Earth Observation (EO) and associated space technologies across Africa through its collaborative African-European research and development (R&D) partnership. It focuses on capacity building and offers customized, domain-specific training programs. Scientific collaboration and research capacity is co-designed and executed by African and European research teams. The “Famine Early Warning Systems Network” (FEWSNET) originally created by the United States Agency for International Development (USAID) during the severe famines of the 1980s in East and West Africa, serves as a key tool for early drought warning, providing timely data and analysis through remote sensing. It has been instrumental in identifying areas vulnerable to humanitarian crises, such as droughts, floods, and natural disasters in LMICs, a challenge often difficult to address through traditional ground surveys. Cross-disciplinary consortia and citizen scientists also enhance data-driven decision-making processes, enabling alert responses to global food security challenges. The NASA Harvest consortium connects NASA Earth observations to support a wide range of stakeholders, including leading researchers, humanitarian organizations, economists, policymakers, and other decision-makers. By providing actionable information, NASA Harvest facilitates preparedness and response to events such as food price volatility, weather-induced food shortages and other agricultural disruptions (NASA, 2024).
Our review presents a novel synthesis of satellite-based climate resilience measures in low-and middle-income countries, integrating advanced analytical techniques and artificial intelligence (AI) to enhance decision-making and policy implementation. Research and development partnerships, with facilitation of sustainable adoption of EO and related space technologies, could expedite the integration of small satellites utilizing standardized technologies, allowing populations with limited research budgets or technological capacity to access and benefit from space technology advances. The review identified key factors that could enable implementation and scale-up by deploying small, cost-effective satellites. Thus, facilitating greater participation of LMICs in EO missions as they can operate efficiently and affordably, enabling effective utilization of data and facilitating the development of application-driven missions and space system management. It is predicted that over the next decade, advancements in resolution and reductions in the cost per image will enable (small) satellite-based remote sensing to expand to previously unobtainable scales.
3.2 Global capabilities in earth observation presenting challenges and opportunities specific to LMICs
While many high-income countries worldwide continue to advance their infrastructure and capabilities for EO through satellite remote sensing, this progress creates both challenges and opportunities for collaboration in the adoption and application of these technologies tailored to low-and middle-income countries (LMICs). Over 40% of the world’s natural disaster events occur in Asia-Pacific, where international cooperation in EO is key to mitigating these disasters and ensuring “preparedness for future calamities” (Kaku, 2023). The China National Space Administration (CNSA) advances EO technologies for disaster risk reduction in China and beyond. CNSA’s satellite missions, like Gaofen, provide critical data for earthquake monitoring, landslide detection, and urban planning (Chen et al., 2022). The Indian Space Research Organization (ISRO) plays a vital role in EO for disaster management in India and neighboring South-East Asia regions. ISRO’s satellites provide critical data for cyclone tracking, drought monitoring, and urban planning to mitigate disaster risk (Khatri et al., 2022). The Japan Aerospace Exploration Agency (JAXA) utilizes satellite technology for disaster response and recovery efforts in Japan and the pacific. JAXA’s ALOS satellites contribute to land-use mapping, earthquake monitoring, and forest fire detection, supporting disaster resilience initiatives (Harvey, 2023). The Korea Aerospace Research Institute (KARI) develops EO capabilities for disaster risk reduction in South Korea. KARI’s satellites monitor typhoons, landslides, and forest fires, enhancing national resilience to natural hazards (Park et al., 2024).
The United Arab Emirates Space Agency (UAESA) and Mohammed Bin Rashid Space Center (MBRSC) advance EO technologies for disaster management in the Middle East region (Al Rashedi et al., 2020). UAE’s satellites provide critical data for desertification monitoring, coastal zone mapping, and disaster response (Al Hosani et al., 2021). The Egyptian Space Agency (EgSA) is one of the leading space players in Africa and is working towards greater independence in satellite manufacturing and space technology in the region. Its EgyptSat-3 (Planned for 2025–2026) will enhance Earth observation, agriculture monitoring and environmental tracking and aims to reduce reliance on foreign-built satellites.
South American agencies include the Comisión Nacional de Actividades Espaciales (CONAE), Argentina contributing to disaster risk reduction, leveraging satellite missions and EO applications like SAOCOM for flood monitoring and agricultural assessment. CONAE collaborates with regional partners to enhance disaster resilience in South America (Sahade, 1992). The Bolivarian Agency for Space Activities (ABAE), supports EO initiatives for disaster risk reduction in Venezuela, focusing on satellite applications for flood mapping and agriculture monitoring. ABAE contributes to regional collaborations to strengthen disaster preparedness. Instituto Nacional de Pesquisas Espaciais (INPE), Brazil INPE plays a critical role in EO for disaster monitoring in Brazil’s Amazon rainforest and coastal regions. INPE’s satellite data supports deforestation monitoring, fire detection, and biodiversity conservation efforts (Dantas, 2020).
Similarly, the European Union Space Programme components, specifically Galileo, EGNOS, Copernicus and GOVSATCOM, cover more than a third of the global data processing market and provides a diversity of specialist products and services offered by the geo-information sector. A notable milestone has been the “constellation of satellites (Sentinel family) and contributing missions (existing commercial and public satellites) providing datasets for Copernicus services” (ESA, 2023). These satellites are key to operational environmental information services, providing unprecedented volumes of data with assured continuity (Table 3). The launch of the “EarthCARE (Earth Clouds, Aerosols and Radiation Explorer) satellite a joint European/Japanese satellite will specifically address climate change and rising temperatures” (Eisinger et al., 2024). The European Organization for the Exploitation of Meteorological Satellites (EUMETSAT) maintains services for meteorological satellites in disaster risk management, e.g., severe weather monitoring, tropical cyclone tracking and climate studies. The German Aerospace Center (DLR) contributes to EO research and applications for disaster management, focusing on innovative satellite technologies. DLR’s TerraSAR-X and TanDEM-X missions support disaster response efforts through high-resolution radar imaging. In addition, France’s CNES plays pivotal roles in EO for disaster risk reduction, focusing on high-resolution imagery and radar satellite missions. CNES contributes to international collaborations, such as the International Charter on Space and Major Disasters, facilitating rapid access to satellite data during emergencies (Deniel and Tabary, 2023). SRON Netherlands Institute for Space Research (SRON) specializes in developing advanced technology in astrophysical research, Earth observation and design of satellite instruments for space research missions conducted by the European Space Agency (ESA), NASA and other space agencies. The UK Space Agency (UKSA) supports EO applications for disaster risk management through collaborations with European and international partners. UKSA-funded missions, such as Sentinel satellites, contribute to disaster response and environmental monitoring (Lappa et al., 2024). Private sector companies such as UK based Disaster Monitoring Constellation International Imaging Ltd. (DMCii) operate a constellation of EO satellites for disaster monitoring and response at the International Charter for Space and Major Disasters. Its rapid imaging capabilities enable timely assessments of floods, wildfires, and humanitarian crises worldwide. It also sells satellite imaging services and manages the operations of spacecraft such as UK-DMC1 and UK-DMC2.
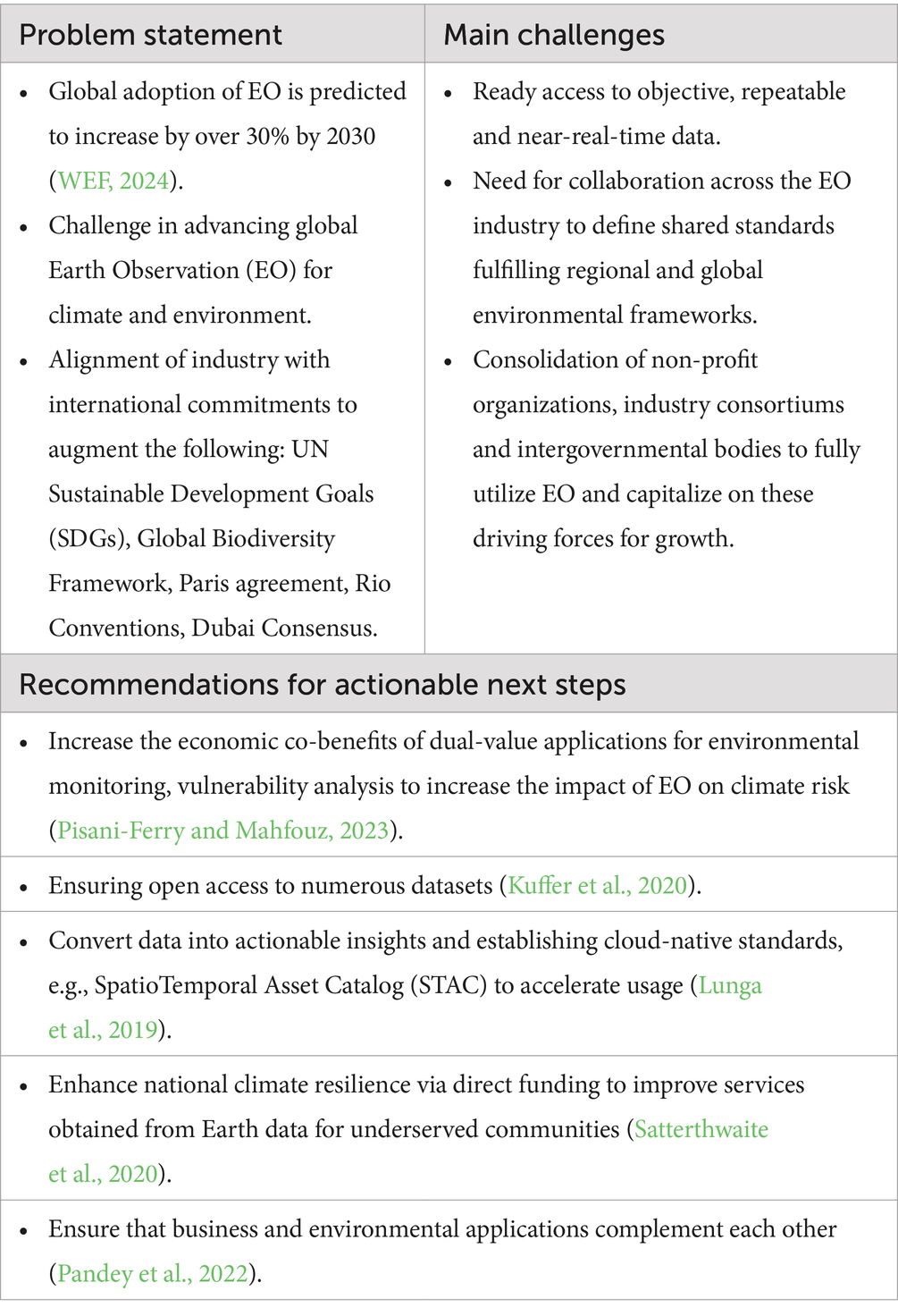
Table 3. Global capabilities in earth observation for climate mitigation reporting positive outcomes.
The State Space Corporation (ROSCOSMOS) of Russia also contributes to global EO efforts through its satellite missions, providing data for disaster response and environmental monitoring. ROSCOSMOS collaborates with international partners to enhance disaster resilience (Kartamyshev et al., 2021) and methods for “forming multi-dimensional data in the information financial and economic system at the enterprise of state space corporation” (Gumenyuk, 2023).
In North America, the US National Oceanic and Atmospheric Administration (NOAA) is instrumental in disaster risk assessment, offering a wealth of environmental datasets through its satellite systems. NOAA’s geostationary and polar-orbiting satellites monitor weather patterns, hurricanes, and ocean conditions, aid in disaster forecasting and response. “Radiances and Environmental Data Records (EDR) from the Joint Polar Satellite System (JPSS) are critical for weather prediction. A variety of EDRs such as fire detection, retrievals of aerosols and water vapor are used by forecasters to provide warnings and alerts during disasters. Several JPSS products also provide continuity of essential climate variables such as upper atmospheric temperature and ozone concentration for climate change monitoring″ (Kalluri et al., 2021). The United States Geological Survey (USGS) is a key provider of EO data for disaster risk assessment, particularly through its Landsat programme. USGS satellites monitor land cover changes, volcanic activity, and coastal erosion, supporting disaster preparedness in the United States and globally (Teodoro and Duarte, 2022). In addition, the Canadian Space Agency (CSA) supports EO applications for disaster management through its RADARSAT program. RADARSAT satellites provide all-weather, day-and-night imaging capabilities for monitoring floods, landslides, and ice hazards in Canada and globally (Garneau, 2005).
Private sector Earth Observation (EO) suppliers are also pivotal in advancing global geospatial intelligence and remote sensing. Companies such as Maxar Technologies, Planet Labs, and Airbus Defence and Space are leading providers of satellite imagery and related data services. These leverage technological advancements in high-resolution imaging, and AI-driven data analysis to offer an array of products and services across various sectors, including agriculture, urban planning, environmental monitoring, and disaster management. The rise of private sector EO suppliers may present challenges, particularly in terms of data ownership, privacy, and regulatory oversight. Unlike publicly funded EO programs, private companies operate within a competitive, profit-driven environment, raising concerns about equitable access to data, especially in regions with limited economic investment or political constraints. Furthermore, use of EO data for commercial gain brings additional complexity to data security and ethical considerations, particularly in sensitive fields such as surveillance and national security. However, international collaboration can address shared global challenges while ensuring equitable access to EO technologies. This collaboration not only empowers LMICs to build resilience and improve decision-making but also fosters innovation and strengthens global partnerships in sustainable development. Ultimately, inclusive approaches to advancing EO capabilities have the potential to benefit all stakeholders, contributing to a more connected and informed world.
3.3 Monitoring standards, guidance and good practice for implementation of earth observation (EO) data specific to LMICs
Satellite observations provide a wealth of data on a range of Essential Climate Variables (ECVs), which are critical for climate research, policymaking, and climate adaptation planning, particularly in LMICs that are most vulnerable to climate change (GCOS, 2016). ECV variables include land and sea surface temperature, precipitation, and CO2 levels. Despite these challenges, there are immense opportunities for collaboration between high-income countries and LMICs to bridge these gaps. Knowledge-sharing initiatives, technology transfers, and partnerships focused on developing affordable, context-specific solutions can enable LMICs to harness the potential of EO technologies. International frameworks and programs, such as those spearheaded by the UN, play crucial roles in such collaborations.
Established standards, guidance, and good practices are vital for generating, sustaining and curating reliable global datasets to meet the needs of LMICs (Table 4). Monitoring standards for ECVs are often outdated, inadequate or absent. Addressing these issues will improve climate observations, predictions, and warnings, and ultimately climate adaptation planning in LMICs. The Global Climate Observing System (GCOS, 2016) has outlined standards and best practices for many ECVs to ensure data consistency, interoperability and reliability across different regions and time periods. Inconsistent data can lead to poor climate predictions and inadequate early warning systems (UNEP, 2023) and exacerbate LMICs’ vulnerability to climate-related hazards. For example, accurate precipitation data is essential for managing water resources and agricultural practices under rain-fed agriculture. Inconsistencies can result in unreliable hydrological models, poor water management decisions and increased vulnerability to droughts and floods. Similarly, inadequate or incorrect monitoring of temperature extremes can affect public health responses to heatwaves.
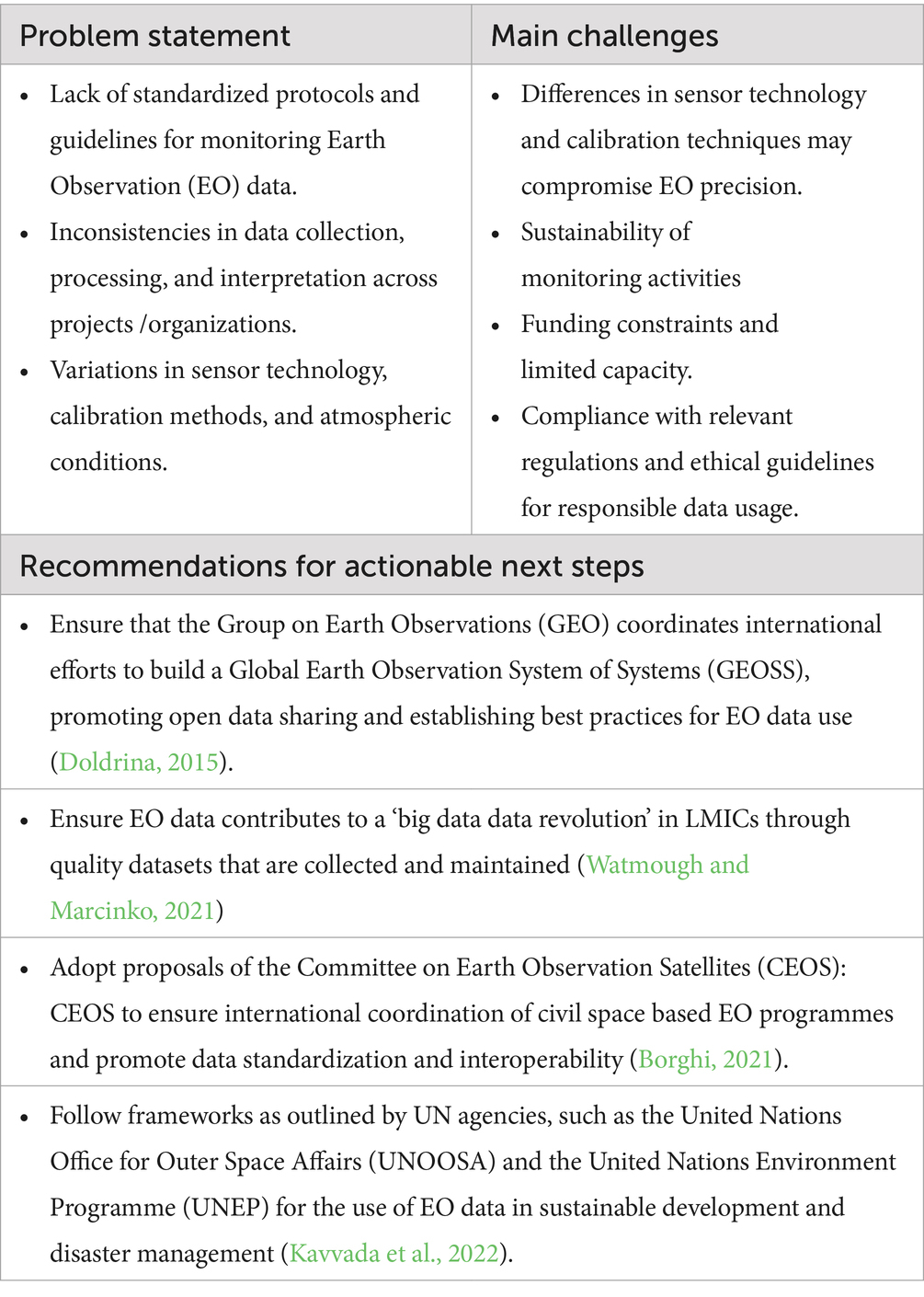
Table 4. Monitoring standards, guidance and best practices for implementation of Earth Observation (EO) data.
Capacity development for harnessing earth observation data from satellite remote sensing is essential, training of local scientists and technicians, establishment of necessary technical infrastructure and the creation of sustainable funding mechanisms are key to deliver impact. This involves both technology transfer in the use of EO technologies and developing capabilities for data interpretation and application (e.g., training on software tools, data processing techniques, and the integration of EO data with other data sources). Additionally, capacity development initiatives need to be tailored to the specific country need and contexts to ensure they are relevant and effective.
Improving the global consistency of EO observations is a critical goal. A concerted effort to harmonize data collection, data formats and reporting practices globally can only be achieved through international cooperation and establishment of centralized data repositories that maintain standardized protocols and curate data effectively. “Reliable data and effective disaster risk reduction policies are crucial to saving lives. Disaggregated data – showing the frequency, triggers and sequence of displacement – can help response and development planners mitigate impacts on displaced people and host communities” (WMO, 2024). Such repositories (ideally open access) would greatly facilitate data sharing, interoperability and integration, enhancing the overall quality and usability of global climate datasets. To address the risks associated with data discontinuity for LMICs, several strategies could be implemented at the national, regional, and international levels. Active collaboration is necessary between space agencies, research institutions and international organizations to advocate for the continuation of critical satellite missions and the development of follow-on missions (GEO, 2021). By engaging in partnerships and knowledge exchange, LMICs can better leverage existing expertise and resources to mitigate f data discontinuity impacts on climate monitoring. Investment in capacity building and technology transfer programs would enhance in-country ability to develop and operate satellite missions independently (UNESCO, 2019) and avoid vendor “lock in”. By building local technical expertise and infrastructure, LMICs can reduce their dependence on external sources of satellite data and ensure the continuity of climate observations over the long term.
For example, the European Commission is proposing an African-European partnership for digital transformation of the African continent. In this context, Earth Observation by satellite remote sensing is identified as essential digital technology providing information for evidence-based policy and decision making (ESA, 2024). The European Copernicus program and its Sentinel satellites offer open and free data of up to 1.2 petabytes per year across the African continent. The Sentinel-1 and -2 missions can now provide geospatial satellite remote sensing data that can strengthen climate resilience and disaster preparedness across African countries and subregions (ESA, 2024). Additionally, LMICs can combine EU data with alternative and complementary streams of climate data, such as ground-based monitoring networks, citizen science initiatives, and unmanned aerial vehicles (UAVs), to complement satellite observations and fill data gaps (Conrad and Hilchey, 2011).
Governments, NGOs, and companies can facilitate seamless data sharing by implementing mechanisms that are user-friendly, anonymous, and add value. In particular, the major cloud computing providers are well positioned to play a more significant role in reducing redundancy in imagery, streamlining processing costs, and reduce overall costs per data point (Lee et al., 2023). It can be anticipated that the cost of satellite imagery will continue to decrease to a level comparable with alternative imaging solutions.
Satellite-based measurements offer a cost-effective means of monitoring large and remote areas, providing critical data that may be difficult or very expensive to obtain through ground-based observations alone (Zhao et al., 2020). Integrating satellite data with ground-based measurements can also improve the reliability and comprehensiveness of datasets. By strengthening local capabilities, LMICs can contribute more effectively to global climate monitoring efforts and benefit from improved climate predictions and early warning systems to inform their Nationally Determine Contributions (NDCs) and National Adaptation Plans (UNEP, 2023).
3.4 Enabling equity in access to advanced analytical techniques and satellite data interpretation for LMICs
The rapid advancement of analytical methods and AI technologies is revolutionizing the interpretation of satellite data, holding significant potential where access to timely and accurate data is crucial to address natural resource management and disaster risk reduction. International collaborations and partnerships can play a significant role in capacity development in EO and analytical techniques for LMICs. The combined resources of these agencies and technologies globally have unlimited capacity to deliver large-scale environmental monitoring services to terrestrial populations particularly in resource-constrained settings. These existing missions are all fully operational and highlight that the international satellite remote sensing community has the capacity to provide financial assistance and technical support to LMICs to strengthen their climate monitoring capabilities and resilience (UNFCCC, 2015). The Green Climate Fund, the Global Environment Facility and international funding mechanisms can allocate resources to LMICs for the procurement of satellite data, the development of climate information systems and implementation of capacity building initiatives (The World Bank Group, 2020). Likewise, international agreements and conventions can promote collaboration and knowledge sharing among countries to address common challenges related to climate monitoring. The Group on Earth Observations (GEO) and the African Space Policy and Strategy provide frameworks for cooperation, enabling knowledge transfer and resource sharing (GEO, 2021). It is critical to ensure the reliability and longevity of the satellites, developing appropriate data processing and analysis tools, and integrating satellite data with other sources of climate information (Choubey et al., 2020). International collaborations and partnerships can support the development and deployment of satellite cubes, leveraging shared expertise and resources.
Partnerships to develop CubeSats, or small satellites, presents a promising avenue for bolstering climate mitigation efforts in LMICs. These compact and cost-effective satellites offer the capacity to collect high-resolution data essential for monitoring various climate variables, thus supporting actions to mitigate and adapt to climate change. CubeSats hold particular significance for LMICs, providing a more accessible and affordable means of acquiring Earth Observation (EO) data and deliver crucial insights for climate mitigation strategies, monitoring deforestation patterns, tracking urban heat islands and coastal erosion dynamics, “advances in artificial intelligence and deep learning underpin all these technologies by enabling insights to be drawn from increasingly large data volumes” (Cavanaugh et al., 2024). The European Space Agency’s (ESA) new Φsat-2 mission, launched in August 2024, will push the boundaries of AI for Earth observation – demonstrating the transformative potential of AI for space technology. The Φsat-2 is a dedicated AI mission to explore the capabilities of utilizing extended onboard processing and further demonstrate the benefits of using AI for innovative EO. “With six AI applications running onboard, the satellite is designed to turn images into maps, detect clouds in the images, classify them and provide insight into cloud distribution, detect and classify vessels, compress images on board and reconstruct them in the ground reducing the download time, spot anomalies in marine ecosystems and detect wildfires” (ESA, 2024).
Building upon the foundation established by its predecessor, the African Regional Data Cube (ARDC), the Digital Earth Africa initiative secures support for a comprehensive, whole-of-government and multi-stakeholder approach to the utilization of Earth observation data. This collaboration is essential to promote the effective integration of these data into decision-making processes across various sectors and has helped countries such as Ghana, Kenya, Sierra Leone, Senegal, and Tanzania to address issues from agriculture and food security to deforestation and urbanization (Mubea et al., 2020). By facilitating access to EO data and fostering collaboration, initiatives like Digital Earth Africa can empower LMICs to monitor socioeconomic and biophysical conditions. Geographical coverage limitations, notably with geostationary satellites, do still exist, and stem from disparities in data availability across satellite agencies: collaboration is needed to address these challenges and ensure the reprocessing of data and their inclusion in climate data records.
In addition, assessing the accuracy and completeness of reliable model accuracy demands substantial investment in human resources, validation and ground truthing. This involves collecting field data to validate and calibrate satellite measurements, improving the accuracy and reliability of EO data. Manual data collection on the ground is indispensable for training analytics models and validating predictive imagery (Miller and Clark, 2020). Comprehensive ground-truthing data must encompass variables and observed events all geotagged to the observation location and compared against imagery from the same period (Robinson and White, 2023). However, the widespread acquisition of such data incurs significant costs.
Ground-truthing and validation are essential components of EO data implementation, ensuring that satellite observations accurately reflect on-the-ground conditions. In LMIC settings, ground-truthing is challenging due to financial, logistical and resource constraints. Ground-truthing efforts can be significantly impeded by remote and inaccessible areas, limited infrastructure, and scarcity of trained personnel (Smith et al., 2021; Johnson and Lee, 2023), challenges re particularly pronounced in LMICs, where logistical and resource constraints often limit the efficacy and accuracy of ground-based data validation (Garcia et al., 2022). Overcoming these challenges requires innovative approaches, including the use of citizen science and community-based monitoring. These reference-quality observations play a crucial role in monitoring climate system changes, bolstering confidence in assessments of future climate change and variability to facilitate the monitoring and quantification of the effectiveness of internationally agreed climate adaptation measures.
Enhancing accessibility and inclusivity in satellite remote sensing for the EO sector requires fostering equitable partnerships with local scientists and institutions in LMICs. This is essential to address data gaps and advance the sustainable development and utilization of satellite cubes by these countries (Table 5). These technical inputs could also be augmented through community-based approaches to make use of the resulting data, for example along the lines of the IDeAMapS approach proposed by Kuffer et al. (2020) with respect to LMIC urban areas. By establishing mutually beneficial partnerships to leverage local expertise, LMICs can build technical capacity and strengthen their ability to harness EO data for climate adaptation (Parkinson et al., 2016). Localisation is critical to ensure that satellite remote sensing contributes to local needs, and for long-term sustainability and impact in LMICs. Notably, few studies of community-based initiatives have been carried out for EO, in contrast to community-based and citizen-science approaches in biodiversity monitoring or hydrology (for example).
Advancements in machine learning, deep learning (DL) and big data analytics are transforming the landscape of satellite data interpretation, enabling more efficient, accurate, and scalable analysis. Machine learning algorithms, such as convolutional neural networks (CNNs) and recurrent neural networks (RNNs), can be used to extract complex patterns and features from satellite imagery (Ma et al., 2020). These algorithms can automate tasks such as land cover classification, vegetation monitoring, and disaster detection, significantly reducing the time and effort required for data analysis. Integration of satellite data with other sources of geospatial information, such as geographic information systems (GIS), crowd-sourced data, and social media feeds, has enabled the development of comprehensive analytical frameworks for understanding dynamic environmental processes and human-environment interactions (Qi and Hankey, 2021). These integrated approaches leverage the complementary strengths of different data sources to generate actionable insights holding immense potential to address key challenges faced by LMICs.
Disaster monitoring and response is a key arena where “timely and accurate information is critical for effective emergency management” (Sun, 2023). In this context, machine learning (ML) algorithms can synthesize satellite imagery to detect changes in land cover, identify affected areas, and assess the extent of damage following natural disasters such as floods, earthquakes, and wildfires (Feng et al., 2022). By providing real-time information to disaster response teams, satellite remote sensing technologies can improve the efficiency and effectiveness of relief efforts, saving lives and minimizing economic losses. Satellite remote sensing applications in agricultural monitoring and food security assessment are delivering on its potential, particularly in regions vulnerable to climate variability and extreme weather events. ML models trained on satellite data can analyze crop health, predict yields, and identify areas at risk of drought or pest infestation (Lobell et al., 2021). By providing EWS and decision support tools to farmers and policymakers, these technologies can enhance resilience to climate shocks and improve food security outcomes in LMICs.
Case studies evidencing the impact of this data on the prediction of future flood events are driven by the generation of extreme precipitation patterns. These patterns are produced by AI-driven weather generators, which are trained on historical satellite weather data and conditioned on climate change projections derived from global climate models. Techniques such as traditional Markov chain sampling (Steinschneider et al., 2015) or more advanced deep learning models, including variational autoencoders (Oliveira et al., 2022), have been shown to successfully generate synthetic weather data. This enables both the retrospective assessment of flood events and the forward-looking prediction of flood risks and damages, providing valuable insights into flood impact and future hazard preparedness (Annex A).
EO also supports natural resource management and biodiversity conservation. ML algorithms can use EO to detect deforestation, monitor changes in land use, and identify habitat loss hotspots, helping target interventions to mitigate environmental degradation. The widespread adoption of advanced analytical methods for satellite data interpretation may be constrained as “limited access to high-quality training data, inadequate technical infrastructure, and a shortage of skilled personnel are significant barriers to implementation” (Anderson et al., 2023). Data privacy, security, and equity challenges must be addressed to ensure that the benefits of these technologies are shared equitably across different socioeconomic groups and geographic regions. International collaborations, knowledge sharing initiatives, and capacity building programs can facilitate technology transfer and skill development, enabling LMICs to build local expertise and infrastructure for satellite data analysis (GEO, 2021). In theory, “public-private partnerships and innovative financing mechanisms can also support the development and deployment of tailored solutions that address the specific needs and priorities of LMICs” (UNEP, 2023).
3.5 How to realise the promise of satellite remote sensing earth observation for LMICs?
Much of the literature recommends harnessing the potential of satellite remote sensing to deliver innovative climate adaptation solutions and a range of significant benefits for LMICs. As evidenced in the review, the potential to apply satellite remote sensing applications to remotely gather EO data and information can enable multiple applications across different sectors. Advanced ML techniques could identify the most “effective methods for distinguishing EQ intensities, enhancing risk evaluation, and minimizing incorrect hazard assessments. Additionally, integration with IoT systems ensures reliable and swift alerts to decision-makers, crucial for effective EEWSs and evacuation plans” (Abdalzaher et al., 2024). Technological progression swiftly enhances the capacity of the satellite sector to monitor, measure, and disseminate information pertaining to various adverse climate events. The review found that 11.67% of the studies demonstrated improvements in disaster response using satellite data, whilst 37.22% highlighted gaps in data accessibility in LMICs. It also plays pivotal roles in forecasting, validating, and alleviating natural disaster impacts, “leveraging their distinctive capacity for remote monitoring of environmental conditions pre and post occurrences like droughts, wildfires, and floods. This capability holds significant utility for insurers and has the potential to facilitate parametric insurance schemes for the agriculture sector” (Born et al., 2019). Parametric insurance schemes, being detached from the underlying asset, can be linked to specific triggering events such as extreme weather conditions, averting the necessity for physical assessments.
The consideration of space science trends, market dynamics, policy landscapes, strategic frameworks, and regulatory frameworks, along with associated social, economic, and political challenges present detailed insights from emerging space-capable countries within these regions is crucial for establishing and enhancing efficient and mutually beneficial cooperation mechanisms within the realm of space technology to adapt to climate change shocks and disasters. Recent innovations bridging AI with EO infrastructure offer a range of platforms to access and process data at scale. Despite these trends, the current landscape of EO initiatives remains disjointed and is characterized by varying degrees of user engagement and impacts (Di Leo et al., 2023). Advances in AI applied to EO data platforms are facilitating a new generation of AI-based climate forecasting solutions to improve precision. However, access to computing power, large EO datasets, technology transfer resources and expertise all pose challenges for LMICs. Despite this, many LMICs are adopting impactful climate resilience strategies based on satellite data (Annex C). Examples include Pakistan, which has expanded EWS, reduced flood-related fatalities by over 90%, and built flood-resilient infrastructure, helping mitigate $30 billion of losses. Kenya promotes climate-smart agriculture, increasing crop yields by 30% while supporting 1 million farmers with drought-resistant practices and financial support. Bangladesh has pioneered floating schools and homes, providing education to thousands of children and strengthening coastal defenses to protect from rising sea levels and floods.
We have identified various gaps in research on this topic. While many of the studies presented satellite remote sensing projects and provided an immediate “snapshots ‘of project implementation, there was a lack of evaluation of the long-term impact of satellite remote sensing application in EO. Effective implementation of satellite remote sensing initiatives hinges on delivery of impact, which is achieved when driven by local or national needs, while adhering to monitoring standards, guidance, and best practices. International organizations play a pivotal role in establishing standardized protocols for data acquisition, processing and dissemination. By promoting data interoperability and harmonization, these initiatives facilitate cross-border collaboration and information sharing, thereby enhancing the scalability and replicability of satellite-based monitoring systems. Moreover, capacity-building efforts aimed at training local stakeholders in EO data analysis and interpretation will foster sustainable institutional capacities, ensuring the long-term viability of satellite remote sensing initiatives in LMICs.
4 Conclusion
Advanced analytical techniques and satellite data are pivotal in building robust climate resilience and operating multi-hazard disaster risk reduction systems. Integrating satellite data with in-situ observations and modelling techniques is found to enhance the accuracy and reliability of EWS, enabling authorities to issue timely alerts and mobilize response efforts to vulnerable communities. Additionally, the accessibility of satellite-derived information via online platforms and mobile applications facilitates community engagement and resilience-building initiatives, empowering populations to take proactive measures to mitigate the impacts of disasters. The integration of EO data into climate adaptation efforts has yielded positive outcomes globally. Satellite-based monitoring systems enable the quantification and verification to facilitate the implementation and monitoring of climate action initiatives, such as REDD+ (Reducing Emissions from Deforestation and Forest Degradation) and Nationally Determined Contributions (NDCs) reporting required of all governments under the Paris Agreement. Additionally, EO data provides essential inputs for climate modelling and forecasting, enabling policymakers to develop evidence-based adaptation and mitigation strategies in response to climate change. EO combined with AI analysis can contribute to more objective strategic planning, monitoring and impact analysis. Whilst holding promising potential, we consider that the deployment of generative AI applications in climate adaptation innovations warrants careful consideration to uphold the reliability and accuracy of AI-generated content. It should be acknowledged that whilst this review offers a comprehensive assessment of satellite-based solutions for climate resilience, certain limitations exist. The accuracy and granularity of analyses are influenced by the availability and quality of satellite-derived data, which can be particularly constrained in low- and middle-income countries. Additionally, although the integration of artificial intelligence in this field holds significant potential, it remains an evolving area with challenges related to data interpretation, algorithm development, and accessibility, particularly in resource-limited settings. Key areas for future research, such as improving data accessibility, advancing AI capabilities for climate modelling and exploring cross-sectoral partnerships and integration of satellite data will strengthen LMIC capabilities and lead to robust climate resilience strategies.
Investments in satellite remote sensing capabilities have increasingly become a cornerstone of both national and international frameworks for climate governance. These advanced technologies play a pivotal role in enhancing our understanding of environmental changes, supporting informed decision-making, and facilitating the monitoring of climate-related impacts. However, the integration of satellite-based data into effective governance strategies is not without challenges. A critical issue that has emerged is the pervasive digital divide that exists across stakeholder communities, particularly within the context of the food, land, and water nexus. Policymakers, investors, and innovators must collaborate to address the significant disparities in access to digital technologies, data, and infrastructure. These disparities disproportionately affect various regions, with vulnerable populations—particularly in the Global South—often being the most marginalized.
To address these inequities, the literature underscores the necessity for concerted, collaborative efforts aimed at developing inclusive and equitable strategies. Such strategies should be designed to promote digital inclusion, enabling underserved communities to fully participate in the digital economy and the benefits of remote sensing technologies. These recommendations highlight the importance of targeted investments in critical infrastructure that facilitates broader access to digital tools and data. Moreover, there is a pressing need for capacity-building initiatives that focus on enhancing digital literacy and skills at the local level. These initiatives will empower communities to effectively utilize remote sensing data and other digital resources, thus enabling them to contribute to and benefit from sustainable development initiatives.
In addition, the implementation of policies that foster the affordability and accessibility of relevant technologies is paramount. Ensuring that digital tools, including satellite data, are within reach of underserved regions is essential for bridging the gap between high- and low-income areas. Addressing the digital divide in this manner not only ensures a more equitable distribution of resources but also unlocks transformative opportunities for innovation, efficiency improvements, and sustainable development. Such advancements can significantly contribute to the achievement of broader goals related to social and economic equity, particularly in the context of climate change adaptation and mitigation. However, despite these recommendations, significant challenges remain. Short-lived initiatives funded by high-income countries often conclude once the initial pilot funding period expires, leaving behind a legacy of limited impact. Even when satellite data is made freely available through open-source platforms, the lack of local capacity and expertise frequently undermines the sustainability of these projects. Without the necessary infrastructure, knowledge, and skills to ensure continuity, the potential of satellite remote sensing to drive long-term, meaningful change is compromised. Therefore, it is essential that efforts to promote digital inclusion and capacity-building are not viewed as temporary or philanthropic, but as long-term investments that provide lasting benefits to communities and contribute to global climate governance.
As EO technologies continue to evolve, addressing issues related to data privacy, equitable access, and technological dependency becomes increasingly critical. Ensuring that the benefits of EO data are shared fairly across nations, while safeguarding privacy and fostering local expertise, will be essential in creating a more just and sustainable global system. For LMICs, the path forward lies in strengthening capacity, promoting collaboration, and ensuring that they are not merely consumers of technological solutions but active participants in the creation and utilization of data for development. Through concerted efforts, it is possible to harness the full potential of Earth observation in a way that promotes global equity, protects privacy, and mitigates the risks associated with technological dependence.
As geopolitical tensions rise, fostering equitable partnerships in EO research and development between LMICs and high-income countries is imperative. Sustained investments, user-driven policies, and innovative financing mechanisms can prioritize high-impact EO topics while fostering capacity-building initiatives. Bridging global divides in satellite data access and utilization is not just a technical challenge but is essential to advance global resilience and unlock the full benefits of satellite remote sensing EO data for all.
Author contributions
PC: Conceptualization, Formal analysis, Investigation, Methodology, Project administration, Supervision, Visualization, Writing – review & editing. PCM: Writing – review & editing. DÓ: Writing – review & editing. JO’F: Writing – review & editing. YT: Writing – review & editing. CS: Writing – review & editing. PM: Writing – review & editing. MG: Formal analysis, Writing – review & editing. AG: Writing – review & editing.
Funding
The author(s) declare that financial support was received for the research and/or publication of this article. This study was supported by Taighde Éireann—Research Ireland (formerly Science Foundation Ireland) Future Innovator Prize AI for Societal Good Challenge for the Tracking Adaptation Progress in Agricultural Systems (TAPAS) project code19/FIP/AI/7515P co-led by CS and AG.
Conflict of interest
The authors declare that the research was conducted in the absence of any commercial or financial relationships that could be construed as a potential conflict of interest.
Generative AI statement
The authors declare that no Gen AI was used in the creation of this manuscript.
Publisher’s note
All claims expressed in this article are solely those of the authors and do not necessarily represent those of their affiliated organizations, or those of the publisher, the editors and the reviewers. Any product that may be evaluated in this article, or claim that may be made by its manufacturer, is not guaranteed or endorsed by the publisher.
Supplementary material
The Supplementary material for this article can be found online at: https://www.frontiersin.org/articles/10.3389/fclim.2025.1514423/full#supplementary-material
References
Abdalzaher, M. S., Soliman, M. S., El-Hady, S. M., Benslimane, A., and Elwekeil, M. (2021). A deep learning model for earthquake parameters observation in IoT system-based earthquake early warning. IEEE Internet Things J. 9, 8412–8424. doi: 10.1109/JIOT.2021.3114420
Abdalzaher, M. S., Soliman, M. S., Krichen, M., Alamro, M. A., and Fouda, M. M. (2024). Employing machine learning for seismic intensity estimation using a single station for earthquake early warning. Remote Sens. 16:2159. doi: 10.3390/rs16122159
Albris, K., Lauta, K. C., and Raju, E. (2020). Strengthening governance for disaster prevention: the enhancing risk management capabilities guidelines. Int. J. Disaster Risk Reduct. 47:101647. doi: 10.1016/j.ijdrr.2020.101647
Al Hosani, H., Al Rashedi, N., and Al Sharji, F. (2021). Empowering the UAE space sector through an attractive regulatory framework. Air Space Law 46, 329–350. doi: 10.54648/AILA2021019
Al Rashedi, N., Al Shamsi, F., and Al Hosani, H. (2020). “UAE approach to space and security” in Handbook of space security. (Cham: Springer), 621–652.
Anderson, K., Seto, K. C., and Peterson, P. M. (2023). Satellite-based methods for monitoring urbanization in low-and middle-income countries: a review. Remote Sens. Environ. 258:112343.
Antonelli, A., Dhanjal-Adams, K. L., and Silvestro, D. (2023). Integrating machine learning, remote sensing and citizen science to create an early warning system for biodiversity. Plants People Planet 5, 307–316. doi: 10.1002/ppp3.10337
Barteit, S., Sié, A., Zabré, P., Traoré, I., Ouédraogo, W. A., Boudo, V., et al. (2023). Widening the lens of population-based health research to climate change impacts and adaptation: the climate change and health evaluation and response system (CHEERS). Front. Public Health 11:1153559. doi: 10.3389/fpubh.2023.1153559
Borghi, M. (2021). Towards full and open access: challenges and opportunities for the legal interoperability of earth observation data. J. Space L. 45:142.
Born, L., Spillane, C., and Murray, U. (2019). Integrating gender into index-based agricultural insurance: a focus on South Africa. Dev. Pract. 29, 409–423. doi: 10.1080/09614524.2018.1556608
Burke, M., Driscoll, A., Lobell, D. B., and Ermon, S. (2021). Using satellite imagery to understand and promote sustainable development. Science 371:eabe8628. doi: 10.1126/science.abe8628
Cavanaugh, K. C., Bell, T. W., Aerni, K. E., Byrnes, J. E., McCammon, S., and Smith, M. M. (2024). New Technologies for Monitoring Coastal Ecosystem Dynamics. Annu. Rev. Mar. Sci. 17, 409–433. doi: 10.1146/annurev-marine-040523-020221
Chan, S., Hale, T., Deneault, A., Shrivastava, M., Mbeva, K., Chengo, V., et al. (2022). Assessing the effectiveness of orchestrated climate action from five years of summits. Nat. Clim. Chang. 12, 628–633. doi: 10.1038/s41558-022-01405-6
Chen, L., Letu, H., Fan, M., Shang, H., Tao, J., Wu, L., et al. (2022). An introduction to the Chinese high-resolution earth observation system: Gaofen-1~ 7 civilian satellites. J. Remote Sens. 2022, 9–12. doi: 10.34133/2022/9769536
Choubey, S., Kumar, S., and Mishra, M. (2020). “Cube sat design specification and its challenges” in Recent trends in communication, computing, and electronics. eds A. Khare, U. S. Tiwary, I. K. Sethi, and N. Singh (Singapore: Springer), 421–430.
Conrad, C. C., and Hilchey, K. G. (2011). A review of citizen science and community-based environmental monitoring: issues and opportunities. Environ. Monit. Assess. 176, 273–291. doi: 10.1007/s10661-010-1582-5
Dantas, C. C. (2020). Astrophysics Division Instituto Nacional de Pesquisas Espaciais (INPE), Brazil.
Deniel, C., and Tabary, P. (2023). “The role of the National Center for space studies (CNES, France)” in Satellites for atmospheric sciences 1: meteorology, climate and atmospheric composition. eds. T. Phulpin, D. Renaut, H. Roquet, and C. Camy-Peyret. (Wiley-ISTE) 141–162.
Di Leo, M., Minghini, M., Kona, A., Spadaro, N., Kotsev, A., Dusart, J., et al. (2023). Digital earth observation infrastructures and initiatives: a review framework based on open principles. Int. Arch. Photogramm. Remote. Sens. Spat. Inf. Sci. XLVIII-4/W7-2023, 33–40. doi: 10.5194/isprs-archives-XLVIII-4-W7-2023-33-2023
Disaster Risk Reduction. (2023) Policy-brief-disaster-risk-reduction-and-climate-change. Erişim Adresi (24.05. 2023): Available online at: https://www.undrr.org/publication/policy-brief-disaster-risk-reduction-and-climate-change (Accessed April 17, 2024).
Doldrina, C. (2015). Open data and earth observations: the case of opening up access to and use of earth observation data through the global earth observation system of systems. J. Intell. Prop. Info. Tech. Elec. Com. L. 6:73.
Eisinger, M., Marnas, F., Wallace, K., Kubota, T., Tomiyama, N., Ohno, Y., et al. (2024). The earth CARE mission: science data processing chain overview. Atmos. Meas. Tech. 17, 839–862. doi: 10.5194/amt-17-839-2024
ESA. (2023) Available online at: https://defence-industry-space.ec.europa.eu/system/files/2022-03/Copernicus%20General%20EN.pdf (Accessed May 15, 2024).
ESA. (2024) EO science for society. Available online at: https://eo4society.esa.int/eo-africa/overview/ (Accessed May 16, 2024).
Feng, Z., Zhang, Y., and Wang, J. (2022). A review of satellite-based methods for flood monitoring and assessment in low-and middle-income countries. J. Hydrol. 603:127344.
Garcia, M., Smith, J., and Robinson, L. (2022). Validation of satellite-derived insights: a key step towards enhancing agricultural decision-making. Remote Sens. 14:1001.
Garneau, M., (2005). Space in the service of society: a Canadian case study. In Proceedings of 2nd International Conference on Recent Advances in Space Technologies, 2005. RAST 2005. (pp. 1–6). IEEE.
GCOS (2016). The global observing system for climate: Implementation needs. Geneva: World Meteorological Organization.
GEO. (2021). Group on Earth Observations: Annual Report. Available online at: https://www.earthobservations.org/documents/geoar2021.pdf (Accessed February 22, 2024).
GFDRR/World Bank (2024). Early warning Systems in Fragile, conflict, and violence affected settings: Shielding communities from natural hazards amid compounded crises. Washington, DC: World Bank.
Ghazaryan, G., König, S., Rezaei, E. E., Siebert, S., and Dubovyk, O. (2020). Analysis of drought impact on croplands from global to regional scale: a remote sensing approach. Remote Sens. 12:4030. doi: 10.3390/rs12244030
Gumenyuk, I. V. (2023) About using space data from earth remote sensing devices during search and rescue operations (works). In AIP conference proceedings (Vol. 2549). AIP Publishing.
Harvey, B. (2023). “Technology, society and economy” in Japan in space: Past, present and future. eds. H. Kaufman and M. Maimone (Cham: Springer International Publishing), 65–120.
Index of Objects Launched into Outer Space, UNOOSA (2024). Available online at: https://www.unoosa.org/oosa (Accessed January 21, 2024).
Jha, M. K., and Dev, M. (2024). “Impacts of climate change” in Smart internet of things for environment and healthcare. eds. M. Azrour, J. Mabrouki, A. Guezzaz, A. Amounas, and F. Hedges (Cham: Springer), 139–159.
Johnson, P., and Lee, C. (2023). Overcoming infrastructure and personnel challenges in remote sensing. J. Geogr. Res. 58, 456–470.
Jones, P., and Brown, L. (2018). The role of earth observation data in early warning systems for climate-related risks in LMICs. J. Clim. Resilience 12, 45–58.
Kaku, K. (2023). A record of the international collaboration in space-based responses to the 2011 great East Japan earthquake. UK: Cambridge Scholars Publishing.
Kalluri, S., Zou, C. Z., and Flynn, L. E. (2021). Applications of joint polar satellite system data and products for severe weather events and climate monitoring. In 2021 IEEE international geoscience and remote sensing symposium IGARSS (pp. 695–698). IEEE.
Kartamyshev, A. S., Chernysh, B. A., and Murygin, A. V. (2021). Method for forming multi-dimensional data in the information financial and economic system at the enterprise of state space corporation “Roscosmos”. Siberian Aerosp. J. 22, 589–599. doi: 10.31772/2712-8970-2021-22-4-589-599
Kavvada, A., Ishida, C., Juárez, J., Ramage, S., Merodio, P., and Friedl, L. (2022). “EO4SDG: a GEO initiative on earth observations for sustainable development goals” in Earth observation applications and global policy frameworks. eds. A. Kavvada, D. Cripe, and L. Friedl, (American Geophysical Union), 145–157.
Kerr, M., Tonetti, S., Cornara, S., Bravo, J. I., Hinz, R., Latorre, A., et al. (2020). Eo-alert: a novel architecture for the next generation of earth observation satellites supporting rapid civil alert. 71st international Astronautical congress (IAC 2020),
Khatri, K., Kumari, B., and Shahana, K. (2022) ISRO’s capabilities in disaster management. In 3rd World Congress On Disaster Management (pp. 1–8).
Krichen, M., and Abdalzaher, M. S. (2024). Performance enhancement of artificial intelligence: a survey. J. Netw. Comput. Appl. 232:104034. doi: 10.1016/j.jnca.2024.104034
Krishnamurthy, R., Krishna, P., Fisher, J. B., Schimel, D. S., and Kareiva, P. M. (2020). Applying tipping point theory to remote sensing science to improve early warning drought signals for food security. Earths Future 8:e2019EF001456. doi: 10.1029/2019EF001456
Kuffer, M., Thomson, D. R., Boo, G., Mahabir, R., Grippa, T., Vanhuysse, S., et al. (2020). The role of earth observation in an integrated deprived area mapping “system” for low-to-middle income countries. Remote Sens. 12:982. doi: 10.3390/rs12060982
Lappa, M., Hamerton, I., Roberts, P. C., Kao, A., Domingos, M., Soorghali, H., et al. (2024) Why space? The opportunity for materials science and innovation.
Lee, S., Robinson, A., and Miller, B. (2023). Cloud computing and satellite imagery: enhancing validation efforts in agriculture. Remote Sens. Appl.: Soc. Environ. 5, 100–115.
Lobell, D. B., Di Tommaso, S., Burke, M., and Kilic, T. (2021). Twice is nice: the benefits of two ground measures for evaluating the accuracy of satellite-based sustainability estimates. Remote Sensing 13:3160.
Luna, E. M. (2017). “Education and training for disaster risk reduction including climate change adaptation” in The Routledge handbook of disaster risk Reduction including climate change adaptation. eds. I. Kelman, J. Mercer, and J. C. Gallard (London: Routledge), 238–251.
Lunga, D. D., Alemohammad, H., Liu, Y., Newsam, S., Pacifici, F., Santos-Villalobos, H., et al. (2019). The trillion pixel geo AI challenge workshop (no. ORNL/TM-2019/1442). Oak ridge National Lab.(ORNL), Oak ridge, TN (United States)
Ma, Z., Jia, G., Schaepman, M. E., and Zhao, H. (2020). Uncertainty analysis for topographic correction of hyperspectral remote sensing images. Remote Sensing 12:705.
Mbithi, P. M., Mbau, J. S., Muthama, N. J., Inyega, H., and Kalai, J. M. (2021). Higher education and skills development in Africa: an analytical paper on the role of higher learning institutions on sustainable development. J. Sustain. Environ. Peace 4, 58–73. doi: 10.53537/jsep.2021.08.001
Miller, E., and Clark, R. (2020). Addressing the challenges of model accuracy in satellite-based analytics. Int. J. Remote Sens. 33, 2130–2145.
Mubea, K., et al. (2020b). Leveraging data ecosystems: Opportunities to address development challenges in Africa : Global Partnership for Sustainable Development Data.
Mubea, K., Killough, B., Seidu, O., Kimani, J., Mugambi, B., and Kamara, S. (2020) Africa regional data cube (ARDC) is helping countries in Africa report on the sustainable development goals (SDGS). In IGARSS 2020–2020 IEEE international geoscience and remote sensing symposium (pp. 3379–3382). IEEE.
Murthi, K. R. (2022). Future Indian earth observation capabilities: policy challenges. New Space. 10, 68–78. doi: 10.1089/space.2021.0043
NASA. (2024). Global Space-based Intercalibration System (GSICS). Available online at: https://gsics.nesdis.noaa.gov (Accessed March 10, 2024).
Naser, K., Haq, Z., and Naughton, B. D. (2024). The impact of climate change on health services in low- and middle-income countries: a systematised review and thematic analysis. Int. J. Environ. Res. Public Health 21:434. doi: 10.3390/ijerph21040434
Okamura, Y., Ohlenburg, T., and Tesliuc, E. (2024). Scaling up social assistance where data is scarce: opportunities and limits of novel data and AI. Social Protection Discussion Papers and Notes (189993), doi: 10.1596/41553
Oliveira, D. A., Diaz, J. G., Zadrozny, B., Watson, C. D., and Zhu, X. X. (2022) Controlling weather field synthesis using variational autoencoders. In IGARSS 2022–2022 IEEE International Geoscience and Remote Sensing Symposium (pp. 5027–5030). IEEE.
Page, M. J., McKenzie, J. E., Bossuyt, P. M., Boutron, I., Hoffmann, T. C., Mulrow, C. D., et al. (2021). Updating guidance for reporting systematic reviews: development of the PRISMA 2020 statement. J. Clin. Epidemiol. 134, 103–112. doi: 10.1016/j.jclinepi.2021.02.003
Pandey, N., de Coninck, H., and Sagar, A. D. (2022). Beyond technology transfer: innovation cooperation to advance sustainable development in developing countries. Wiley Interdiscip. Rev.: Energy Environ. 11:e422. doi: 10.1002/wene.422
Parkinson, C. L., DiBattista, T., Valenti, S., and Larko, D. (2016). The future of earth observation from space. Bull. Am. Meteorol. Soc. 97, 43–48.
Park, J., Jung, H., and Park, J. H. (2024) Open collaborative strategic planning establishing Kari vision 2050 for the aerospace field in South Korea. Available online at SSRN 4749517.
Qi, M., and Hankey, S., (2021). Using street view imagery to predict street-level particulate air pollution. Environmental Science \u0026amp; Technology 55, 2695–2704.
Robinson, L., and White, D. (2023). Satellite imagery and ground-truthing in agricultural analytics: overcoming heterogeneity challenges. J. Agric. Resour. Econ. 29, 125–139.
Sahade, J. (1992). La comisión nacional de actividades espaciales (CONAE). Boletín de la Asociación Argentina de Astronomía.
Sahay, S. (2016). Big data and public health: challenges and opportunities for low-and middle-income countries. Commun. Assoc. Inf. Syst. 39, 419–438. doi: 10.17705/1CAIS.03920
Satterthwaite, D., Archer, D., Colenbrander, S., Dodman, D., Hardoy, J., Mitlin, D., et al. (2020). Building resilience to climate change in informal settlements. One Earth 2, 143–156. doi: 10.1016/j.oneear.2020.02.002
Schneider, T., Behera, S., Boccaletti, G., Deser, C., Emanuel, K., Ferrari, R., et al. (2023). Harnessing AI and computing to advance climate modelling and prediction. Nat. Clim. Chang. 13, 887–889. doi: 10.1038/s41558-023-01769-3
Senay, G. B., Velpuri, N. M., Bohms, S., Budde, M., Young, C., Rowland, J., et al. (2015). “Drought monitoring and assessment: remote sensing and modeling approaches for the famine early warning systems network” in Hydro-meteorological hazards, risks and disasters. eds. J. F. Shroder, P. Paron, and G. D. Baldassarre (Elsevier), 233–262.
Smith, A., Brown, C., and Patel, S. (2021). Addressing the barriers to effective ground Truthing in remote areas. Int. J. Remote Sens. 42, 2950–2965.
Steinschneider, S., Wi, S., and Brown, C. (2015). The integrated effects of climate and hydrologic uncertainty on future flood risk assessments. Hydrol. Process. 29, 2823–2839. doi: 10.1002/hyp.10409
Sudianto, S., Herdiyeni, Y., and Prasetyo, L. B. (2023). Early warning for sugarcane growth using phenology-based remote sensing by region. Int. J. Adv. Comput. Sci. Appl. 14, 502–503. doi: 10.14569/IJACSA.2023.0140259
Sun, Z. (2023). Actionable science of global environment change: from big data to practical research. Berlin: Springer Nature.
Teodoro, A. C., and Duarte, L. (2022) The role of satellite remote sensing in natural disaster management. In Nanotechnology-based smart remote sensing networks for disaster prevention 2022 (pp. 189-216). Elsevier
The World Bank Group (2020). Climate change and development report 2020. Washington, DC: World Bank.
The World Bank Group. (2024). Available online at: https://datahelpdesk.worldbank.org/knowledgebase/articles/906519-world-bank-country-and-lending-groups (Accessed May19, 2024).
UN ESCAP (2023) Regional Integrated Multi-Hazard Early Warning. "Compendium of multi-hazard early warning cooperation."
United Nations Environment Programme (2023). Adaptation gap report 2023: Underfinanced. Underprepared. Inadequate investment and planning on climate adaptation leaves world exposed. Nairobi: United Nations Environment Programme.
United Nations Office for Disaster Risk Reduction and World Meteorological Organization (2024). Global status of multi-Hazard early warning systems. Geneva: Switzerland.
UNOOSA. (2024). United Nations Register of Objects Launched into Outer Space. Available online at: https://www.unoosa.org/oosa/en/spaceobjectregister/index.html (Accessed May 14, 2024).
van Ginkel, M., and Biradar, C. (2021). Drought early warning in Agri-food systems. Climate 9:134. doi: 10.3390/cli9090134
Vijil, A., El-Serafy, Y., Adam, T., and Haßler, B. (2024). Data collection and visualization tools in the education sector in sub-Saharan Africa and South Asia : EdTech Hub.
Watmough, G. R., and Marcinko, C. L. (2021). “Towards achieving the UNs data revolution: combining earth observation and socioeconomic data for geographic targeting of resources for the sustainable development goals” in Data science applied to sustainability analysis. eds. J. Dunn and P. Balaprakash, (Elsevier), 229–254.
WEF (2023). Space applications in agriculture: Enhancing food and water security, improving climate action. Cologny: World Economic Forum.
Wilson, J., and Patel, S. (2020). Real-time monitoring of disaster events using satellite imagery: implications for LMICs. J. Disaster Sci. 17, 89–104.
WMO. (2023). Early warnings for all executive action plan. Available online at: https://library.wmo.int/doc_num.php?explnum_id=11426 (Accessed June 25, 2024).
WMO (2024). Global status of multi-Hazard early warning systems 2023. Geneva: World Meteorological Organization.
WMO. (2025). State of the global climate 2024. Available online at: https://wmo.int/sites/default/files/2025-03/WMO-1368-2024_en.pdf (Accessed March 19, 2025).
Yildirim, G., Rahman, A., and Singh, V. P. (2022). A bibliometric analysis of drought indices, risk, and forecast as components of drought early warning systems. Water 14:253. doi: 10.3390/w14020253
Keywords: environmental informatics, remote sensing, climate resilience, emergency preparedness, machine learning, satellite development, LMIC, climate action
Citation: Codyre P, Murphy PC, Ó Fionnagáin D, O’Farrell J, Tessema YM, Spillane C, McKeown PC, Geever M and Golden A (2025) Measuring climate resilience in low- and middle-income countries using advanced analytical techniques and satellite data: a systematic review. Front. Clim. 7:1514423. doi: 10.3389/fclim.2025.1514423
Edited by:
Maria Josefina Figueroa, Copenhagen Business School, DenmarkReviewed by:
Kirti Sundar Sahu, Canadian Red Cross, CanadaOtilia Manta, Romanian Academy, Romania
Mohamed S. Abdalzaher, National Research Institute of Astronomy and Geophysics, Egypt
Innocent Osoro Ngare, Kenyatta University, Kenya
Copyright © 2025 Codyre, Murphy, Ó Fionnagáin, O’Farrell, Tessema, Spillane, McKeown, Geever and Golden. This is an open-access article distributed under the terms of the Creative Commons Attribution License (CC BY). The use, distribution or reproduction in other forums is permitted, provided the original author(s) and the copyright owner(s) are credited and that the original publication in this journal is cited, in accordance with accepted academic practice. No use, distribution or reproduction is permitted which does not comply with these terms.
*Correspondence: Patricia Codyre, cGF0cmljaWEuY29keXJlQHVuaXZlcnNpdHlvZmdhbHdheS5pZQ==