- 1Potsdam Institute for Climate Impact Research (PIK), Potsdam, Germany
- 2Global Data Institute, International Organization for Migration, Berlin, Germany
Many studies now provide evidence of weather and climate effects on human migration, but only few have attempted to project the impact of future climate change, or attribute the impact of past climate change, on global migration patterns. Here we compare the existing projections, and find that for international migration from African countries, they differ by about two orders of magnitude, while for internal migration even the sign is uncertain. None of the various models used have been shown to explain historical migration changes, limiting the confidence one may have in their projections. We then discuss prospects for two types of models. Econometric models have been used to identify the marginal effects of climate on migration. Their utility for projections is limited, but they may lend themselves to specific questions of attributing current migration patterns to climate change, which has rarely been done so far. On the other hand, models of total migration can better account for the complex dynamics likely important for long-term projections, but constraining them is a challenge given the current understanding of these dynamics. Improvements may come from closer investigation of potential nonlinearities in the response to increasingly extreme climatic conditions.
Introduction
Model-based estimates are an important ingredient for designing evidence-based migration policies (Willekens, 2018). They can aid policymakers in understanding past and current migration patterns by integrating, complementing, and interpreting data; and in anticipating potential changes in migration due to policy interventions, internal dynamics of migration systems, or external shocks or trends. A particular area of interest is the effect of climate change on global migration. Ample evidence shows that climatic conditions and weather events can affect migration (Cattaneo et al., 2019; Hoffmann et al., 2021; Moore and Wesselbaum, 2023; Sedova et al., 2021), and the Intergovernmental Panel on Climate Change (IPCC) concluded that “climatic conditions, events and variability are important drivers of migration and displacement (high confidence), with migration responses to specific climate hazards being strongly influenced by economic, social, political and demographic processes (high confidence)” (Cissé et al., 2022).
Given this strong evidence of weather and climate effects on migration, the amount of climate change induced by human activities thus far, and further into the future, may also induce significant changes in global migration patterns. However, the question to what extent future climate change will affect human migration, within and across national borders, is still largely unresolved. Again citing the recent IPCC sixth assessment report (AR6), “only a very small number of studies have attempted to make systematic projections of future regional or global migration and displacement numbers under climate change” (Cissé et al., 2022). And curiously, even less is known about the effects of recent climate change to date: according to the IPCC, “the contribution of long-term changes in climate-related systems [i.e., climate change] to observed human displacement or migration patterns has not been quantified so far” (O’Neill et al., 2022). Such attribution studies, usually involving the comparison between a factual and a counterfactual, no-climate-change scenario, are gaining traction in other fields as the impacts of climate change are becoming more evident (Perkins-Kirkpatrick et al., 2024).
Although some new research has appeared since the AR6, by and large these statements still hold. In this article, we address the following questions with respect to global or continental scale studies: (1) Do the few existing studies projecting migration under climate change agree with each other on the magnitude of the climate change contribution? (2) What are the challenges of turning current knowledge about the effects of climate and weather on migration into credible future projections? (3) What can be learned about the magnitude of climate migration using existing models in the context of attribution?
This article adds to recent reviews and perspectives on climate migration modeling.1 Our selection of projection studies is partly based on the study by Schewel et al. (2024), who reviewed 30 papers “forecasting” climate-related migration and asked how well these can “predict climate migration.” They identified four common limitations: Underrepresentation of non-climate related migration drivers, such as social networks; underrepresentation of fast-onset events and combinations of multiple types of events; neglect of immobility as a potential outcome; and the failure to account for future thresholds in either climate or society. In our paper, we add to this “inside” perspective on the modeling assumptions by providing an “outside” perspective on the numbers produced by different projection studies, which Schewel et al. (2024) did not discuss. Similarly, Cattaneo et al. (2019) reviewed the projections available at the time but, given these mostly focused on different, individual countries, did not engage with or even compare their quantitative results. We offer a like-for-like comparison of global-and continental-scale projections. Beyer et al. (2023) discussed a range of different modeling approaches used for causal inference or projections of climate-related migration. They highlighted how different studies sometimes arrive at contradictory conclusions on climate-migration relationships, and provide a number of recommendations to improve model-based studies, in terms of methods and data used as well as how model results are evaluated and interpreted. In our paper, we address the relationships between causal inference, projection, and attribution; and highlight some of the pitfalls in, and opportunities for, extrapolating insights from causal inference (the identification of climate effects on migration using econometric regression models) into the future or, for attribution, into counterfactual historical worlds (Figure 1).
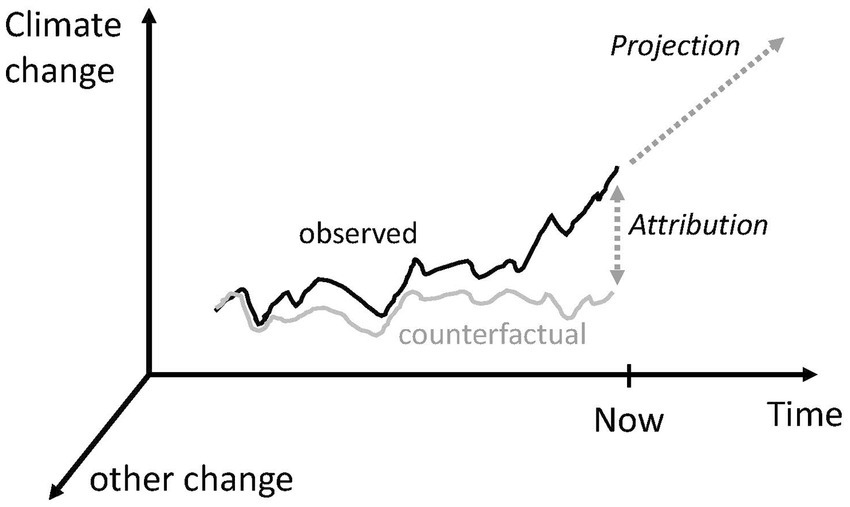
Figure 1. Schematic illustration of projection and attribution. Over time, the world (black line) progresses along the climate change axis (while being subject to natural climate variability), as well as along the other change axis representing, e.g., economic, demographic, or political developments.
Cross-comparison of migration projections
We identified seven different models that have been employed to project climate-related migration at a continental or global scale (Table 1, top). Four of them are what we call “system-wide” models—models of migration flows or stocks, that in principle aim to describe these variables completely, as potentially complex functions of both climate-related variables and non-climate variables . Three other models are “marginal-effects” models: regression models designed to identify the marginal effect of one or more observed (climate-related) variables on the outcome variable, while controlling for other, unobserved variables . Such a model makes a number of simplifications in order to estimate the parameters of interest without knowing the full system. As a stylized yet typical example,
The first equality corresponds to a system-wide model. The second equality simplifies this by assuming that there is no interaction between observed and unobserved variables, and hence, and are separable. The unknown function is then approximated through a set of constant factors (or simple functions of time) . Finally, is often specified as a simple power function, which allows applying linear regression techniques to estimate the coefficient of interest , after taking the logarithm on both sides: , where are a set of fixed or random effects or time trends. Below we will return to the implications of these assumptions when such a model is used to project future changes in as a function of .
We compare projections for Africa around the year 2050. This choice maximizes the number of studies for comparison—only four models are global, while three are limited to Africa—but is also relevant in itself given the particular vulnerability of many African regions to climate change impacts (Donatti et al., 2024). We extract approximate cumulative numbers of additional migrant movements due to climate change, originating in African countries, under a medium-high or high greenhouse gas (GHG) concentration scenario, separately for international (including both within and outside of Africa, unless otherwise mentioned) and internal migration where possible. While details, such as the precise GHG scenario chosen, differ between studies, these details are not expected to affect the order of magnitude of projection results. In terms of international migration, projections from system-wide models (n = 3) range between about 0.02–0.4 million and 3–9 million additional movements (Table 1). In terms of internal migration, projections (n = 3) range from about 7 million fewer movements to about 110 million additional movements. Some of this variation may be explained by differences in the climate measures and pathways considered: A model considering only the effect of gradual temperature change on countries’ GDP as a mechanism for climate migration (Rikani et al., 2022) projects much smaller changes in international migration than a model considering a larger number of climate effects on local economic productivity (Burzyński et al., 2022); and a model limited to drought-induced migration (Smirnov et al., 2022) produces smaller projections of internal climate migration than a model considering multiple slow-onset hazards as well as floods (Amakrane et al., 2023). However, given other, conceptual differences between the models, it is difficult to attribute differences in projections to any particular modeling choice.
Marginal-effects models are conceptually more similar, but the related projections in our sample are more difficult to compare in terms of the outputs they provide. They exclusively project international migration. One (Cottier, 2024) projects about 0.1 to 1.2 million additional migrants between African countries; it does not consider migration to other continents. The second (Cattaneo et al., 2024) projects about 100 to 200 million additional movements globally (excluding rich origin countries) compared to a scenario with population change but no climate change. The study does not allow extracting separate figures for Africa, though it does show larger impacts of climate change in Africa than in other regions. Given the proportions of within-African migration to total emigration from African countries (roughly 2/3), and of migration from African countries relative to global migration today [roughly 1/8; both derived from Abel and Cohen (2022)], the projections by Cottier (2024) and Cattaneo et al. (2024) may be consistent with about 0.2 to 1.9 million, and about 15 to 30 million, additional cumulative movements from or within the continent, respectively. A third, older study projects about 3 to 25 million additional net migrants from African countries annually, by the end of the 21st century (Marchiori et al., 2012), which despite the more distant time horizon would likely correspond to cumulative migrant numbers by mid-century of dozens to hundreds of millions.2
Thus, from the studies that allow extracting comparable information, there is little agreement on the order of magnitude of migration related to climate change. Projections for international migration from African countries span approximately three orders of magnitude (not including the range of projections within each study). This spread is reduced to about two orders of magnitude when one excludes the highest and lowest figures—produced, respectively, more than 10 years ago (Marchiori et al., 2012) or considering only indirect effects through GDP per capita (Rikani et al., 2022). For internal migration, fewer projections are available, and they range from negative values to hundreds of millions. For the global studies, global figures are spread out similarly as figures for Africa (Supplementary Table S1). In fact, being derived independently of each other and using rigorous analytical methods, the projections we consider diverge more strongly than the early predictions of global environmental migration reviewed by Gemenne (2011). Notably, our review does not support the assumption that climate migration will largely play out internally: of three studies projecting both internal and international migration, internal flows are larger than international flows in one study (Marchiori et al., 2012), smaller in another (Burzyński et al., 2022), and of comparable magnitude in the third (Smirnov et al., 2022). This is consistent with country-level evidence (Hoffmann et al., 2020) and may be because some of the recent models include indirect effects—e.g. through large-scale economic impacts—not evident in early assessments (Jäger et al., 2009).
Two of the models have also been applied to attribute past changes in migration to recent climate change (Table 1, bottom). One study concludes that climate change, by slowing down economic growth in poor countries, has mainly inhibited migration from African countries, resulting in tens of thousands fewer international movements over a 30-year period than without climate change (Rikani et al., 2023). The other concludes that climate change has induced about 5 million additional net international migration movements, and about 170 million additional internal (rural–urban) movements, over a 40-year period (Marchiori et al., 2012). These divergent estimates are not necessarily in contradiction, since the first study only considered the indirect effect of climate change on migration through GDP per capita, whereas the second study estimated the marginal effect of climate change on migration regardless of the underlying mechanism. Clearly though, these two studies are not sufficient to draw any firm conclusions on the attribution of climate migration.
Given these large differences between models in terms of quantitative results, can any of them be considered more realistic or plausible than others? An objective, though partial answer to this question could come from empirical validation. However, only for two of the models we evaluated could we find any comparison against past migration trends. Neither of these models is able to reproduce the temporal variability of migration flows found in the observational estimates used for calibration, as evidenced by visual comparison of modeled and observed country-level (Rikani and Schewe, 2021) or bilateral flows (Cottier, 2024) and by calculating R2 on each bilateral flow corridor (Cottier, 2024). Thus, the outputs of the projection models in Table 1 either were not compared to observed past migration changes, or they were and that comparison yielded a negative result. Future projection efforts will benefit from more routinely testing a model’s ability to explain, in historical data, the equivalent phenomena that it is supposed to predict, to the extent the available data allows this; for instance, by calculating R2 on individual flow timeseries (Beyer et al., 2023; Cottier, 2024).
The gap between causal inference and projections
Generally, log-linear regression models (used in the marginal-effects modeling studies here, as well as more broadly in migration studies) have been shown to explain differences across countries or regions, but not changes in migration over time (Beyer et al., 2022). This limitation applies not only to the context of climate change, but to migration research as a whole, and indicates that, generally, we have trouble understanding why migration flows changed over time in the ways they did according to available data; either because those data are flawed, or because the factors and processes inducing the changes are more complex or idiosyncratic than can be captured with existing models and theories (Arango, 2000; Beyer et al., 2023; de Sherbinin et al., 2022).
This lack of understanding of migration changes is a problem for projection studies aimed at estimating such changes in the future. It is less of a problem when one is only interested in the marginal effects of climate variables on migration in an (assumed) stationary context. This explains why there is ample evidence on climate and weather effects on migration, but it is difficult to turn this evidence into estimates of climate change effects (see the contrasting IPCC quotes above): The evidence on climate and weather effects rests in large parts on marginal-effects models, which have been successful at extracting some signals of climate and weather from limited and noisy migration data (Hoffmann et al., 2021). They have done so by purposefully ignoring much of the (known and unknown) complexity of migration in order to apply simple and powerful statistical methods. For instance, a model of international migration using country-level fixed effects ignores any changes over time in unobserved, slowly-varying country-level variables and their potential interactions with the observed (climate-related) variables, in order to isolate the average effects of the latter. The log-linear form of most marginal-effects models (Equation (1)) is another strong assumption that however makes it easy to estimate the model parameters. More complex models, such as some of the system-wide models in Table 1, are more difficult to calibrate given the available data.
Attribution as a near-term opportunity
What are the prospects for better constraining the magnitude of climate migration? The problem can be split into questions of projection—how many people will migrate in the future under a certain climate change scenario?—and questions of attribution—how many more (or fewer) people have already migrated due to climate change? We argue that marginal-effects models are inherently more limited than system-wide models when it comes to projections, but they may be useful in certain types of attribution contexts; and such attribution studies may be a feasible near-term goal, in parallel with improving system-wide models.
As useful as the simplifications made in marginal-effects models are when testing for climate signals in migration data in a stationary context (such as a relatively short historical period or a relatively stable climate), they limit the extent to which the findings can be extrapolated outside of that context—either into a future with different climatic and socio-economic conditions, or into a counterfactual, no-climate change scenario for attribution of historical changes. In particular, the separability assumption in Equation (1) neglects interactions between climatic and non-climatic variables; and the ceteris paribus assumption [constant ] neglects changes in non-climatic drivers of migration, including feedbacks of migration on itself. To illustrate, assume climate change increases emigration from a given origin country. This will diminish the number of remaining potential migrants in the origin, lowering flows in subsequent periods, all else equal. At the same time, the additional emigrants, once arrived in their destination countries, may contribute to lowering migration costs for further migrants from the same origin (known as network or diaspora effects), acting to increase flows in subsequent periods. Even when projections are only meant to estimate the additional migration due to climate change in a world where nothing else changes, ignoring such feedback mechanisms may induce biases especially on long timescales, though at least in principle they can be quantified and accounted for through “book-keeping” of migrant and non-migrant populations (Supplementary material).
When other things besides climate change, too, projections of climate change-induced migration may additionally be biased by omitting interactions. These arise, for example, if climate effects depend on income levels, and income levels change (over time or between factual and counterfactual). A model extrapolating only the marginal effect of climate given present income levels would entail a bias, the magnitude of which depends on the strength of the interaction and the amount of change in the unobserved variable. The first is generally unknown. The second can be expected to increase with the time over which the model is extrapolated; as a well measurable example, GDP is projected to increase by a factor of 3 to 8 globally until 2100, with large variation between countries (SSP Scenario Explorer, 2024), meaning omitted interactions may induce serious biases in long-term projections.3
In attribution, on the other hand, the model may be applied to the same time period as it was calibrated on, so the question changes to how much difference there might be in unobserved variables between the factual and the counterfactual scenario. Commonly, the counterfactual scenario represents a world identical to the real world except for the absence of anthropogenic climate change (Perkins-Kirkpatrick et al., 2024). This does not necessarily mean all non-climatic variables have identical values in factual and counterfactual, because those variables may have been influenced by climate change: For instance, economic inequality, in terms of per-capita GDP, is larger today than it would be if it wasn’t for the accumulated effects of climate change (Diffenbaugh and Burke, 2019); with likely implications for migration.
For a complete attribution of (direct and indirect) climate change effects on migration, it is important to account for such accumulated, indirect effects just as in projections; although given the relatively small magnitude of climate change up until the most recent decades, the accumulated historical effects may be smaller than those going forward into the future. Studies addressing such research questions may benefit from scrutinizing the feedbacks and interactions that may be relevant in their particular context, and gauging their potential magnitudes. On the other hand, some interesting and policy-relevant insights may also be gained from asking “ahistoric” attribution questions—i.e., what part of current migration is due to the current, human-induced climate anomaly? (Or, how would migration change if climate were reset to pre-industrial conditions instantly?) While neglecting indirect and accumulated effects, addressing such questions could already tell us something about the extent to which migration patterns have been altered by climate change; and it may require little more than connecting causal inference models with plausible counterfactual climate scenarios (e.g., Gillett et al., 2016; Mengel et al., 2021; Treu et al., 2024).
Conclusion
A first step toward improved climate migration projections might be for future studies to provide as much detail about their projection results as possible, to facilitate comparison with other studies; and to validate models on historical data where feasible. Besides improving our understanding of past migration dynamics, however, it is also important to recognize that these dynamics may change in the future as climatic conditions become more extreme. A system-wide perspective will be required to account for the interactions between climate change, population dynamics, and other economic and social factors and processes, as well as for the potential nonlinearities both inherent in those processes and induced by their interactions (McLeman, 2018; Lenton et al., 2023). For instance, a closer investigation of in-situ adaptation options and their limits (Midgley et al., 2023) may help identify potential thresholds in the climate-migration association that cannot be derived just from past observations. On the other hand, data-driven, predictive modeling approaches from machine learning may help overcome the current impasse of explanatory models when it comes to reproducing observed trends (Shmueli, 2010).
Meanwhile, useful and policy-relevant insights could be gained by extending marginal-effects models from establishing the existence of a causal effect, to quantifying the magnitude of migration change resulting from the already observed climatic changes. For instance, if a temperature increase, or a flood event, of a given magnitude is found to be associated with a certain change in migration, then by how much has climate change already altered migration patterns through its impacts on average temperatures or flood frequencies? Such attribution studies should, however, strive to (i) demonstrate a significant effect of climate variables over time within a unit of observation (e.g., a country), as opposed to an effect between units which would not necessarily represent the response of a given unit to a change in climate (Bell et al., 2019); and (ii) assess the potential role of feedbacks and interactions with unobserved variables in their particular context. Finally, studies will only be able to speak to the overall scale of climate migration—present or future—if they identify the most influential climate-related variables, and account for their combined effects.
Author contributions
JS: Conceptualization, Investigation, Writing – original draft, Writing – review & editing. RB: Writing – review & editing.
Funding
The author(s) declare that no financial support was received for the research and/or publication of this article.
Conflict of interest
The authors declare that the research was conducted in the absence of any commercial or financial relationships that could be construed as a potential conflict of interest.
Generative AI statement
The author(s) declare that no Gen AI was used in the creation of this manuscript.
Publisher’s note
All claims expressed in this article are solely those of the authors and do not necessarily represent those of their affiliated organizations, or those of the publisher, the editors and the reviewers. Any product that may be evaluated in this article, or claim that may be made by its manufacturer, is not guaranteed or endorsed by the publisher.
Supplementary material
The Supplementary material for this article can be found online at: https://www.frontiersin.org/articles/10.3389/fclim.2025.1570995/full#supplementary-material
Footnotes
1. ^“Climate migration” is used here as a shorthand for any changes in migration flows due to climate change; irrespective of whether or not climatic factors are the most important determinants of any individual’s decision to move or stay. In a quantitative sense, climate migration may also be negative, meaning climate change leads to a decrease in migration, which at the individual or community level can be related to what is often referred to as “trapped populations” (Adger et al., 2015).
2. ^This study also uses an early, 1980–1999 reference period; all other studies present additional migration relative to a year or period within the range 2008–2017. See Supplementary Data for details on projections and derivation of figures cited in the main text.
3. ^If the interactions between climate and non-climate variables are known, they can be included explicitly in the model, such as the interaction between weather anomalies and agricultural dependence in Marchiori et al. (2012). The problem is with knowing, and measuring, all the relevant interactions. Projections of the economic impacts of climate change have been facing similar challenges (O’Neill et al., 2022; Rising et al., 2022).
References
Abel, G. J. (2018). Estimates of global bilateral migration flows by gender between 1960 and 2015 1. Int. Migr. Rev. 52, 809–852. doi: 10.1177/0197918318781842
Abel, G. J., and Cohen, J. E. (2019). Bilateral international migration flow estimates for 200 countries. Scientific Data 6:82. doi: 10.1038/s41597-019-0089-3
Abel, G. J., and Cohen, J. E. (2022). Bilateral international migration flow estimates updated and refined by sex. Scientific Data 9:173. doi: 10.1038/s41597-022-01271-z
Adger, W. N., Arnell, N. W., Black, R., Dercon, S., Geddes, A., and Thomas, D. S. G. (2015). Focus on environmental risks and migration: causes and consequences. Environ. Res. Lett. 10:060201. doi: 10.1088/1748-9326/10/6/060201
Amakrane, K., Rosengaertner, S., Simpson, N. P., de Sherbinin, A., Linekar, J., Horwood, C., et al. (2023). African shifts: The Africa climate mobility report, addressing climate-forced migration & displacement, 242.
Arango, J. (2000). Explaining migration: a critical view. Int. Soc. Sci. J. 52, 283–296. doi: 10.1111/1468-2451.00259
Bell, A., Fairbrother, M., and Jones, K. (2019). Fixed and random effects models: making an informed choice. Qual. Quant. 53, 1051–1074. doi: 10.1007/s11135-018-0802-x
Beyer, R. M., Schewe, J., and Abel, G. J. (2023). Modeling climate migration: dead ends and new avenues. Front. Climate 5, 1–10. doi: 10.3389/fclim.2023.1212649
Beyer, R. M., Schewe, J., and Lotze-Campen, H. (2022). Gravity models do not explain, and cannot predict, international migration dynamics. Human. Soc. Sci. Commun. 9:56. doi: 10.1057/s41599-022-01067-x
Burzyński, M., Deuster, C., Docquier, F., and de Melo, J. (2022). Climate change, inequality, and human migration. J. Eur. Econ. Assoc. 20, 1145–1197. doi: 10.1093/jeea/jvab054
Cattaneo, C., Beine, M., Fröhlich, C. J., Kniveton, D., Martinez-Zarzoso, I., Mastrorillo, M., et al. (2019). Human migration in the era of climate change. Rev. Environ. Econ. Policy 13, 189–206. doi: 10.1093/reep/rez008
Cattaneo, C., Massetti, E., Farinosi, F., and Dasgupta, S. (2024). Climate variability and worldwide migration: current evidence and future projections. Environ. Res. Lett. 19:124083. doi: 10.1088/1748-9326/ad91cb
Cissé, G., McLeman, R., Adams, H., Aldunce, P., Bowen, K., Campbell-Lendrum, D., et al. (2022). “2022: health, wellbeing, and the changing structure of communities” in Climate change 2022 – Impacts, adaptation and vulnerability. Contribution of working group II to the sixth assessment report of the intergovernmental panel on climate change. Eds. H.-O. Pörtner, D. C. Roberts, M. Tignor, E. S. Poloczanska, K. Mintenbeck, A. Alegría, et al. UK and New York, NY, USA: Press, Cambridge. pp. 1041–1170.
Clement, V., Rigaud, K. K., de Sherbinin, A., Jones, B., Adamo, S., Schewe, J., et al. (2021). Groundswell part 2: acting on internal climate migration. World Bank. Available at: http://hdl.handle.net/10986/36248
Cottier, F. (2024). Projecting future migration with Bayesian hierarchical gravity models of migration: an application to Africa. Front. Climate 6, 1–13. doi: 10.3389/fclim.2024.1384295
de Sherbinin, A., Grace, K., McDermid, S., van der Geest, K., Puma, M. J., and Bell, A. (2022). Migration theory in climate mobility research. Front. Climate 4, 1–16. doi: 10.3389/fclim.2022.882343
Diffenbaugh, N. S., and Burke, M. (2019). Global warming has increased global economic inequality. Proc. Natl. Acad. Sci. 116, 9808–9813. doi: 10.1073/pnas.1816020116
Donatti, C. I., Nicholas, K., Fedele, G., Delforge, D., Speybroeck, N., Moraga, P., et al. (2024). Global hotspots of climate-related disasters. Int. J. Disaster Risk Reduction 108:104488. doi: 10.1016/j.ijdrr.2024.104488
Gemenne, F. (2011). Why the numbers don’t add up: a review of estimates and predictions of people displaced by environmental changes. Glob. Environ. Chang. 21, S41–S49. doi: 10.1016/j.gloenvcha.2011.09.005
Gillett, N. P., Shiogama, H., Funke, B., Hegerl, G., Knutti, R., Matthes, K., et al. (2016). The detection and attribution model Intercomparison project (DAMIP v1.0) contribution to CMIP6. Geosci. Model Dev. 9, 3685–3697. doi: 10.5194/gmd-9-3685-2016
Hoffmann, R., Dimitrova, A., Muttarak, R., Crespo Cuaresma, J., and Peisker, J. (2020). A meta-analysis of country-level studies on environmental change and migration. Nat. Clim. Chang. 10, 904–912. doi: 10.1038/s41558-020-0898-6
Hoffmann, R., Šedová, B., and Vinke, K. (2021). Improving the evidence base: a methodological review of the quantitative climate migration literature. Glob. Environ. Chang. 71:102367. doi: 10.1016/j.gloenvcha.2021.102367
Jäger, J., Frühmann, J., Grünberger, S., and Vag, A. (2009). EACH-FOR: Environmental change and forced migration scenarios: Synthesis report. EU project, environmental change and forced migration. Budapest, Hungary: Atlas lnnoglobe for the EACH-FOR Project.
Lenton, T. M., Armstrong McKay, D. I., Loriani, S., Abrams, J. F., and Lade, S. J. (2023). The global tipping points report 2023. Exeter, UK: University of Exeter.
Marchiori, L., Maystadt, J.-F., and Schumacher, I. (2012). The impact of weather anomalies on migration in sub-Saharan Africa. J. Environ. Econ. Manag. 63, 355–374. doi: 10.1016/j.jeem.2012.02.001
McLeman, R. (2018). Thresholds in climate migration. Popul. Environ. 39, 319–338. doi: 10.1007/s11111-017-0290-2
Mengel, M., Treu, S., Lange, S., and Frieler, K. (2021). ATTRICI v1.1 – counterfactual climate for impact attribution. Geosci. Model Dev. 14, 5269–5284. doi: 10.5194/gmd-14-5269-2021
Midgley, G. F., Chapman, R. A., and Araujo, J. (2023). Potential tipping points for climate change adaptation costs. Clim. Dev. 15, 717–722. doi: 10.1080/17565529.2022.2151306
Moore, M., and Wesselbaum, D. (2023). Climatic factors as drivers of migration: a review. Environ. Dev. Sustain. 25, 2955–2975. doi: 10.1007/s10668-022-02191-z
O’Neill, B., van Aalst, M., Ibrahim, Z. Z., Ford, L. B., and Bhadwal, S. (2022). “Key risks across sectors and regions” in Climate change 2022: Impacts, adaptation and vulnerability. Contribution of working group II to the sixth assessment report of the intergovernmental panel on climate change (pp. 20–22). eds. H.-O. Pörtner, D. C. Roberts, M. Tignor, E. S. Poloczanska, and K. Mintenbeck (US: Cambridge University Press).
Perkins-Kirkpatrick, S. E., Alexander, L. V., King, A. D., and Kew, S. F. (2024). Frontiers in attributing climate extremes and associated impacts. Front. Climate 6, 1–24. doi: 10.3389/fclim.2024.1455023
Rigaud, K. K., Sherbinin, A. D., Jones, B., and Bergmann, J., (2018). Groundswell: Preparing for Internal Climate Migration. Available online at: https://openknowledge.worldbank.org/handle/10986/29461 (Accessed May 9, 2025).
Rikani, A., Frieler, K., and Schewe, J. (2022). Climate change and international migration: exploring the macroeconomic channel. PLoS One 17:e0276764. doi: 10.1371/journal.pone.0276764
Rikani, A., Otto, C., Levermann, A., and Schewe, J. (2023). More people too poor to move: divergent effects of climate change on global migration patterns. Environ. Res. Lett. 18:024006. doi: 10.1088/1748-9326/aca6fe
Rikani, A., and Schewe, J. (2021). Global bilateral migration projections accounting for diasporas, transit and return flows, and poverty constraints. Demogr. Res. 45, 87–140. doi: 10.4054/DemRes.2021.45.4
Rising, J., Tedesco, M., Piontek, F., and Stainforth, D. A. (2022). The missing risks of climate change. Nature 610, 643–651. doi: 10.1038/s41586-022-05243-6
Schewel, K., Dickerson, S., Madson, B., and Nagle Alverio, G. (2024). How well can we predict climate migration? A review of forecasting models. Front. Climate 5, 1–17. doi: 10.3389/fclim.2023.1189125
Sedova, B., Cizmaziova, L., and Cook, A. (2021). A meta-analysis of climate migration literature. CEPA Discussion Papers 29:83. doi: 10.25932/publishup-49982
Smirnov, O., Lahav, G., Orbell, J., Zhang, M., and Xiao, T. (2022). Climate change, drought, and potential environmental migration flows under different policy scenarios. Int. Migr. Rev. 57, 36–67. doi: 10.1177/01979183221079850
SSP Scenario Explorer. SSP 3.0. (2024). Available online at: https://data.ece.iiasa.ac.at/ssp/#/about (Accessed on January 20, 2025)
Treu, S., Muis, S., Dangendorf, S., Wahl, T., Oelsmann, J., Heinicke, S., et al. (2024). Reconstruction of hourly coastal water levels and counterfactuals without sea level rise for impact attribution. Earth System Sci. Data 16, 1121–1136. doi: 10.5194/essd-16-1121-2024
Keywords: econometric modeling, model intercomparison, validation, future scenarios, nonlinear migration dynamics
Citation: Schewe J and Beyer R (2025) The magnitude of climate change-induced migration: an overview of projections and a case for attribution. Front. Clim. 7:1570995. doi: 10.3389/fclim.2025.1570995
Edited by:
Gabriele Standardi, University of Milan, ItalyReviewed by:
Amsalu Woldie Yalew, Ca’ Foscari University of Venice, ItalyWoi Sok Oh, The Ohio State University, United States
Copyright © 2025 Schewe and Beyer. This is an open-access article distributed under the terms of the Creative Commons Attribution License (CC BY). The use, distribution or reproduction in other forums is permitted, provided the original author(s) and the copyright owner(s) are credited and that the original publication in this journal is cited, in accordance with accepted academic practice. No use, distribution or reproduction is permitted which does not comply with these terms.
*Correspondence: Jacob Schewe, amFjb2Iuc2NoZXdlQHBpay1wb3RzZGFtLmRl