- 1Department of Agricultural Economics and Agribusiness Management, Faculty of Agriculture, Egerton University, Njoro, Kenya
- 2Department of Economics and Management, University of Pavia, Pavia, Italy
In Kenya’s dairy sector, climate change mitigation focuses on sustainable milk production. However, dairy producers often overlook emission reduction, creating a gap between national policies and local practices. This paper aims to identify the factors, including smallholder entrepreneurial orientation, socio-economic characteristics, and institutional influences, that drive the adoption and uptake intensity of on-farm greenhouse gas reduction measures in the dairy sector, particularly among smallholder producers in Nyandarua County, Kenya. The study uses a fractional response model to examine factors influencing greenhouse gas reduction at the farm level based on survey data from 385 dairy farmers. Greenhouse gas reduction was measured using a composite index, with proxies drawn from the literature. Key factors influencing greenhouse gas reduction include education, social capital, entrepreneurial orientation, awareness, and risk perception. The study recommends that the national and county governments promote and support the adoption of climate-smart dairy strategies that increase milk production while simultaneously reducing greenhouse gas emissions. This support could include technical assistance, financial support and educational programs to encourage complementary adoption by dairy farmers.
1 Introduction
Dairy production faces a critical challenge: balancing the need to increase output to meet rising milk demand with the imperative to reduce greenhouse gas (GHG) emissions (O'Hara, 2023). Meeting this growing demand has advantages and drawbacks (Graham et al., 2022). On the positive side, increasing production ensures an adequate supply of dairy products, supporting food security and contributing to economic growth for dairy farmers. However, it also risks exacerbating climate change through higher GHG emissions (Vernooij et al., 2024). Globally, agriculture contributes approximately 17% of total greenhouse gas emissions, with the livestock sector responsible for nearly two-thirds of these emissions. The dominant greenhouse gas emissions in the dairy sector are methane, accounting for 96%, nitrous oxide at 3%, and carbon dioxide at 1% (FAO, 2017). A significant portion of these emissions, particularly in African agriculture, arises from enteric fermentation, which accounts for half of the sector’s GHG emissions (Ntinyari and Gweyi-Onyango, 2020). This issue has prompted a growing body of literature on low-emission development strategies in developing countries (Kihoro et al., 2021; Vernooij et al., 2024). Factors such as breed composition, poor-quality feeds, and inadequate manure management significantly influence the amount of GHG emissions (Rotz, 2018; Pinto et al., 2020a, 2020b). However, discrepancies remain in the reported emissions by smallholder farmers. While some studies argue that smallholding agricultural production is a major contributor to climate change (Vernooij et al., 2024), others contend that their emissions per capita are negligible (Mwaura et al., 2024).
Despite the dairy sector’s contribution to GHG emissions, it is also heavily affected by climate change. Challenges such as rising temperatures, water scarcity, declining feed quality and quantity, and the emergence of new pests and diseases threaten its sustainability (Graham et al., 2022).
Recognising this twofold challenge, numerous Sub-Saharan African nations have incorporated climate change policies into their national frameworks by participating in the United Nations Framework Convention on Climate Change and the Paris Climate Agreement (Mwaura et al., 2024). Many countries mention livestock in their Nationally Determined Contributions and/or Adaptation Plans or outline large-scale low-emissions development initiatives (Graham et al., 2022).
The literature focused on the dairy sector suggests that changes should be made to production practices at the farm level to reduce GHG emissions. These changes include implementing improved feeding strategies, better management of manure and herds, conserving feed, and treating crop residues (Maindi et al., 2020; Kihoro et al., 2021). Studies have used life cycle assessment to measure GHG reduction at the farm level (Zhao et al., 2017; Xu et al., 2023). However, using this approach is complicated, resource-intensive, and time-consuming, with limitations that can affect its feasibility and effectiveness. To this purpose, Mwaura et al. (2024) recommend simpler and easily monitored techniques to tackle the current emission trend among smallholder farmers. Collectively, these studies have provided insights into how various climate-smart dairy strategies (CSDS) reduce GHG emissions. The available literature lacks an empirical model to assess how farm and farmer characteristics influence the uptake intensity of GHG emissions reduction strategies. Addressing this gap is crucial because this information provides critical insights for designing targeted, effective interventions.
The present paper addresses these aspects using the Fractional Response Model (FRM) to assess the factors influencing the adoption of on-farm GHG reduction measures in the dairy sector. Employing a different approach to studying the determinants of GHG emissions reduction contributes to enhancing the depth, breadth, and robustness of the analysis conducted in the literature. This information is essential for tailoring policies, programs, and technologies to specific contexts, thereby increasing the feasibility and scalability of GHG reduction initiatives. Furthermore, such a model could help bridge the gap between high-level theoretical frameworks and on-the-ground implementation, ensuring that proposed solutions are practical and impactful.
Building on the work of Kihoro et al. (2021) and Vernooij et al. (2024), this study introduces a novel approach by utilising proxies as indicators of GHG reduction to compute a comprehensive index. The selected CSDS are grounded in robust evidence from the literature, which demonstrates their effectiveness in reducing GHG emissions (Ericksen and Crane, 2018; Wilkes et al., 2020; Kihoro et al., 2021; Mburu et al., 2024; Vernooij et al., 2024).
What sets this study apart is the integration of smallholder entrepreneurial orientation alongside socio-economic and institutional factors to investigate the drivers of adopting various GHG reduction measures among farmers. This multidimensional approach provides added value by offering a more comprehensive and nuanced understanding of the decision-making processes and constraints at the farm level. Unlike previous studies, which primarily focus on the technical efficacy of CSDS, this research delves into the interplay of individual, economic, and institutional influences, shedding light on the conditions necessary for successful adoption.
This research is particularly important for informing policies and interventions tailored to regions facing similar climate-related challenges, ensuring that agricultural practices are both productive and environmentally sustainable. This study, therefore, assesses the factors that influence the reduction of greenhouse gas emissions at the farm level. The study hypothesised that farmer demographic, socio-economic, institutional, entrepreneurial, and behavioral factors significantly influence the level of greenhouse gas emissions reduction.
2 Methodology
2.1 Description of the study area
This study was conducted in Nyandarua County, located between Mount Kenya and Aberdare areas in Central Kenya. The County between latitude 0°8’ North and 0°50’ South and between longitude 35°13’ East and 36°42’ West (Figure 1). The area receives long rains in March and May, with an annual rainfall of 1700 mm. Short rains are recorded from September to December, with an annual rainfall of 700 mm. The temperatures are considered moderate, ranging between 120\u00B0C and 250\u00B0C. Agriculture is the primary economic activity in the region, contributing approximately 3.9% to the national gross domestic product. The major agricultural produce includes dairy, poultry, Irish potatoes, floriculture, cabbages, carrots, peas, pyrethrum, sugar beet, and cereals. However, the county has experienced decreased agricultural productivity due to its increasingly semi-arid conditions, which have led to frequent food and water shortages for both households and livestock. In response, the County, in collaboration with the World Bank, has implemented climate-smart agriculture interventions to improve livelihoods while simultaneously reducing GHG emissions (County Government of Nyandarua, 2023).
The proposed study in this area is crucial as it addresses the dual challenge of adapting to climate change while promoting sustainable agricultural practices. Focusing on Nyandarua County provides valuable insights into how smallholder farmers can adopt climate-smart strategies to mitigate the impacts of climate change and reduce emissions.
2.2 Data and sampling procedure
The study employed a cross-sectional research design, utilizing a multi-stage sampling technique to select Kipipiri and Kinangop Sub-Counties of Nyandarua County based on their favourable conditions for milk production and climatic conditions that favour agriculture. In this area, six wards namely Engineer, Gathara, Njabini, Kipipiri, Wanjohi, and Githioro, were selected because their relevance to the dairy sector and their vulnerability to climate impacts make them ideal for investigating the adoption of CSDS. In Kenya, a ward is a smaller administrative unit within a sub-county, typically representing a community or village. Given the known target population size of the study area, it used the Yamane (1967) formula to estimate a minimum sample size of 384 dairy farmers from a population of 9,049 to achieve a 5% margin of error. Smallholder dairy farmers were randomly selected from the six wards considered using a systematic random sampling at intervals of five from the list of dairy producers. To distribute the sample across the wards, the study used the target population data at the ward level provided by the Ministry of Agriculture Livestock and Fisheries, County Government of Nyandarua (2023). The proportion of the target population was calculated in each ward relative to the total target population and then used these proportions to allocate the sample accordingly (Table 1).
This study used primary data collected through a semi-structured questionnaire with a mix of open and closed-ended questions chosen based on relevant literature and validated through focus group discussion and key informant interviews. Altogether, 8 dairy farmers engaged in the focus group discussion, encompassing 2 youths, 2 females, and 4 males. Likewise, 8 experts were interviewed for the key informant interviews, representing a diverse range of perspectives: 2 Sub-County dairy board members, 2 dairy cooperative leaders, 2 extension officers, and 2 officers from the Kenya Climate Smart Agriculture Project with each interview taking about forty minutes. In addition, a questionnaire pre-test was carried out to assess the questions’ effectiveness, sufficiency, and suitability in obtaining the necessary data. The pilot study also evaluated the time needed to complete the questionnaire and the respondents’ sensitivity to the questions. The pilot study involved respondents representing 10% of the actual sample size (Abegunde et al., 2020). Data was collected through face-to-face interviews conducted by a team of properly trained enumerators chosen for their data collection skills and knowledge of the local language.
2.3 Outcome variable selection
The outcome variable in this study is the Composite Greenhouse Gas Reduction Index (CGHGRI), which was developed using the following steps:
i. Identification of GHG reduction strategies: Based on a review of relevant literature, the study identified strategies that reduce GHG emissions (methane, nitrous oxide and carbon dioxide) to form the basis of the index.
ii. Data collection using yes/no questions: the study designed a series of yes/no questions to assess whether smallholder dairy producers in our sample implemented these strategies.
iii. Scoring and aggregation: The responses to these questions were aggregated to calculate a score for each CGHG reduction indicator, contributing to the final CGHG reduction index.
The GHG reduction strategies considered are listed in Table 2 with the supporting literature.
Given each indicator’s varying number of attribute levels, the study normalised them to ensure comparability. The literature suggests normalising variables within a range of 0 to 1 (Kumar et al., 2016; Sendhil et al., 2018; Balaganesh et al., 2020). Accordingly, the study employed minimum-maximum normalisation, a straightforward method commonly used to standardise various indicators before amalgamating them into a single index (Shahbaz et al., 2023).
After getting the normalised values for each indicator, the next step was to assign weights to indicators. There are various ways of assigning weights to indicators, including equal weights, expert opinion, and principal component analysis. The equal weights method removes the influence of certain variables but may oversimplify the index, while expert opinion can be subjective depending on the expert’s knowledge (Kumar et al., 2016; Dabkiene et al., 2021). Principal Component Analysis (PCA) assumes a linear relationship among variables and uses their correlations, but it may assign low weights to important indicators with weak correlations, leading to potentially invalid results. Additionally, PCA requires a sufficient number of indicators with a moderate correlation to be effective (Greco et al., 2019).
To avoid the above biases, the following formula was employed to assign weights to indicators as suggested by Shahbaz et al. (2023). First, the study normalised each indicator of the GHG emissions reduction as shown in Equation 1:
where represents the normalised value of the indicator for household represents its actual score, is the lowest score in the sample and the maximum score. The weights were computed as shown in Equation 2:
where represents the weight of the indicator; denotes the sum of normalised values of four indicators for household . This weight estimation formula offers the advantage of distributing weight to each indicator according to its contributing share. According to the results, animal health management, improved breeding, feeding and manure management received the highest weights, respectively.
Finally, a combined GHG emissions reduction index was computed using indicators and their respective weights as per Shahbaz et al. (2023). For each of the N = 4 indicators in Table 2, the study computed the weighted index (Index) as follows:
Afterwards, the study computed the GHGRI as a sum of the indices computed with Equation 3 as shown in Equation 4:
Where denotes composite greenhouse gas emissions reduction index for the four indicators. Higher values of indicate a reduction of GHG emissions by a household. As suggested by the literature, the study utilised a set of characteristics of farms and farmers to explain the dependent variable.
This study uses a FRM to assess the factors influencing the adoption of GHG emission reduction levels. Since the FRM effectively captures nonlinear relationships, it is particularly suitable for our analysis where the dependent variable, the CGHGRI, is an index ranging between 0 and 1. As noted by Wamuyu et al. (2023), FRM provides reliable estimates of regression coefficients regardless of the dependent variable’s distribution. Additionally, it accounts for nonlinearity arising from data censoring without the need for transformations or corrections for observations at the data’s upper or lower bounds. Under the assumptions of a Generalized Linear Model, FRM is both robust and efficient (Wamuyu et al., 2023). The specification equation is as follows:
where:
• : The dependent variable and is its expected value given the independent variables ( )
• : A set of independent variables
• : A vector of coefficients that measure the effect of each independent variable.
• : The inverse of a link function which ensures the predicted stays between 0 and 1.
3 Results
3.1 Farm and farm producers’ characteristics
Table 3 presents the descriptive statistics of the variables used in the analysis. The average age of the farmers was 51 years. A majority (56.6%) of the households were male-headed. The mean household size was about 5 members, indicating moderate household sizes among the sampled farmers.
In terms of socio-economic characteristics, respondents had an average of 9 years of formal education, suggesting that most had completed at least primary schooling, with others progressing to secondary level. The average farm size allocated to dairy production was 1.3 acres, and the mean herd size was about 2 cows. The land allocated to dairy emphasizes the significance of dairy farming as a primary income source for a majority of households, considering smallholder dairy production. Approximately 44% of the respondents reported having off-farm income sources.
Regarding social capital and institutional factors, the survey findings indicated that the sampled farmers had extensive experience in dairy production, with many having more than 15 years of experience in the field. Group membership was relatively common, with 62% of farmers participating in groups and 35% being members of cooperatives. Access to extension services was reported by 43% of respondents. On average, farmers accessed KES 15,496.1 in credit and lived 2.5 km from the nearest market. The average number of training sessions attended was 1.6.
In terms of entrepreneurial and behavioral factors, the average entrepreneurial orientation score was 64.5. Risk perception had a mean score of 3.6, while awareness and perceived behavioral control had average scores of 2.9 and 2.8, respectively. The average behavioral intention score was 3.9. On average, milk yield per cow per year was 2,724.1 litres. The mean greenhouse gas (GHG) reduction index was 0.7, reflecting moderate adoption of GHG-reducing practices among dairy producers.
3.2 Diagnostic tests
Before estimating the probit model, diagnostic states were conducted to determine multicollinearity in the explanatory variables, heteroscedasticity, and normality of the residuals. The results indicated that the Variance Inflation Factor (VIF) was 1.31, below the recommended threshold of 5 (Table 4). The Breusch-Pagan test (p-value = 0.000) showed that the variance of the error terms is not constant across the observations thus heteroscedasticity was a problem. This study accounted for heteroscedasticity by running robust standard errors. Finally, the Shapiro–Wilk test (p-value = 0.037) depicted that the residuals were normally distributed.
When choosing between the logit and probit models for the fractional response model, the study evaluated key model fit statistics, including Akaike Information Criteria (AIC), Bayesian Information Criteria (BIC), and log-likelihood (Table 5). The logit model had a slightly higher log-likelihood compared to the probit model, which suggested that the logit model fitted the data reasonably well. Additionally, the logit model exhibited lower AIC and BIC values compared to the probit model’s AIC and BIC values. Since lower AIC and BIC values indicate a better model, the logit model emerged as the most suitable model for this analysis.
3.3 Determinants of greenhouse gas emissions reduction level among smallholder dairy farmers
Table 6 shows the factors influencing the GHG reduction level among smallholder farmers. A total of 19 explanatory variables were included in the model. The model’s strong statistical significance (Prob>χ2 = 0.0000) and the pseudolikelihood value (269.3741) indicate robust model performance, implying that at least a subset of the explanatory variables has non-zero effects. The results highlight several key determinants across demographic, socio-economic, social capital, institutional, entrepreneurial, and behavioral factors. Specifically, nine variables (education, off-farm income, proportion of cows milked, farmer group and cooperative membership, entrepreneurial orientation, perceived behavioral control, awareness, behavioral intention and risk perception) were statistically significant in explaining GHG reduction levels among farmers. Specifically, education level, proportion of cows milked, group and cooperative membership, and perceived behavioral control were positive and statistically significant at the 1% level, indicating a strong association with GHG reduction levels. Entrepreneurial orientation, awareness, and risk perception were all significant at the 5% level, while behavioral control was negative and statistically significant at the 10% level.
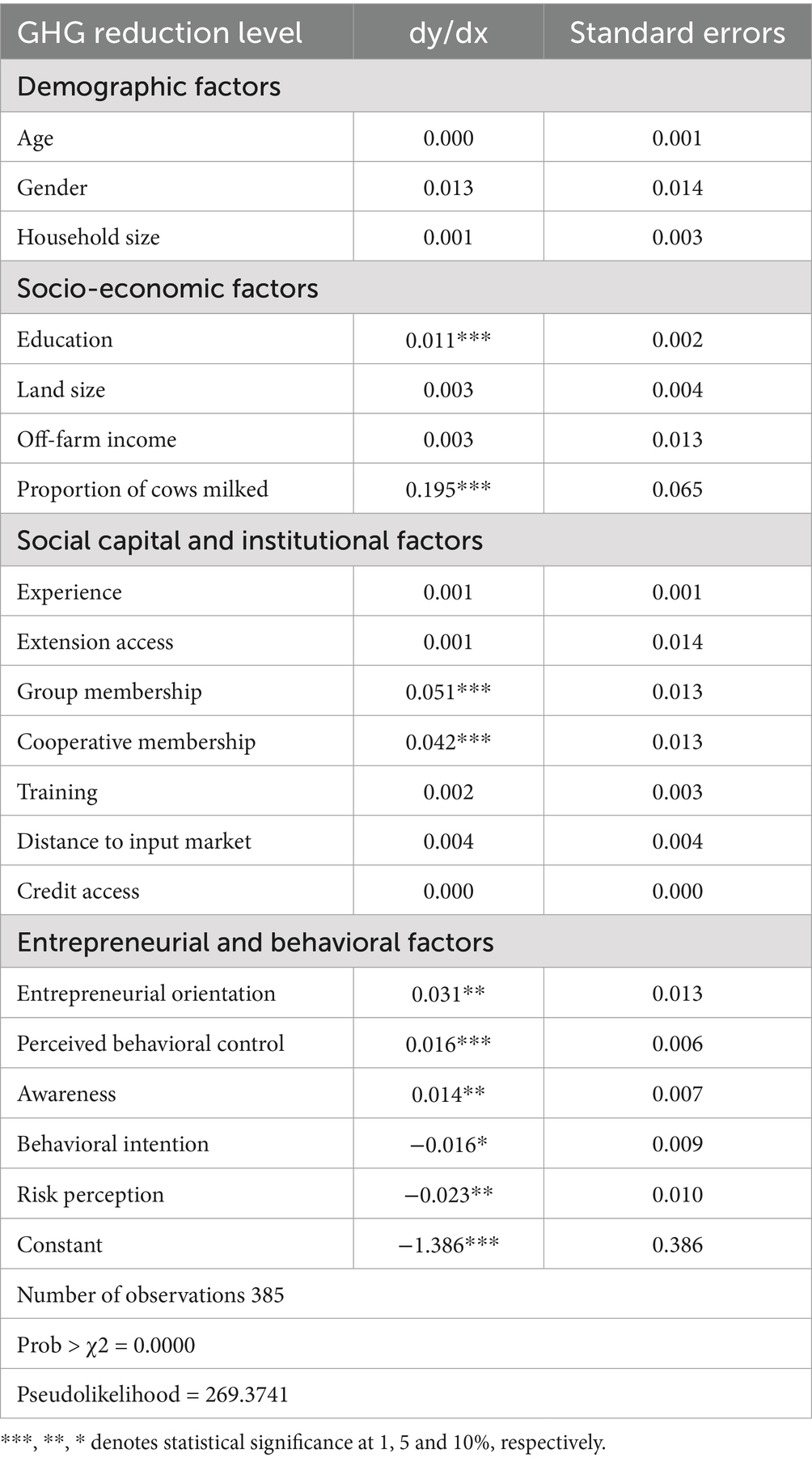
Table 6. Analytical results for the drivers of GHG emissions reduction using fractional response model.
4 Discussion
Among the socio-economic factors, education exhibited a positive and highly statistically significant effect, indicating that higher educational attainment is strongly associated with increased GHG reduction levels. The knowledge gained through education increases the likelihood of adopting climate CSDS since individual farmers can fully understand the benefits of the specific strategies. This finding aligns with the conclusions drawn by Brody and Ryu (2006), Ongare et al. (2016), Alsayed and Malik (2020) and Bohvalovs et al. (2023). Additionally, higher levels of education may enhance farmers’ ability to comprehend the synergies between various mitigation strategies, thereby facilitating the adoption of multiple GHG-reducing practices, as supported by Gebre et al. (2023), Korir et al. (2023) and Asante et al. (2024).
The proportion of dairy animals milked was positively associated with reduced GHG emissions, confirming the studies by Zehetmeier et al. (2012) and Kashangaki and Ericksen (2018). Milking a higher proportion of dairy animals is associated with lowering GHG emissions due to several climate-smart strategies. These include improved feed efficiency, where lactating cows are provided with nutrient-dense feed, resulting in higher milk production (Ericksen and Crane, 2018). Further, there might be an element of efficiency where if a larger proportion of the herd is productive, the overall emissions per unit of milk produced could be lower (Vernooij et al., 2024). These results suggest conducting further studies to determine whether this relationship holds in other regions or with different livestock types. Selective breeding and enhanced animal health contribute to fewer emissions, as healthier, more productive animals emit less methane per unit of milk (Kihoro et al., 2021). Additionally, proper manure management and the adoption of renewable energy, such as bio-digesters, reduce methane and nitrous oxide emissions (Kandulu et al., 2024).
Cooperative and other farmers’ group membership had a significant positive effect on the GHG reduction level. Agricultural groups play a crucial role in disseminating knowledge, and by actively engaging in these groups, smallholder farmers gain a better understanding of the benefits of adopting multiple GHG-reducing strategies. Participation in social and institutional networks, such as group and cooperative memberships, has been shown to enhance the adoption of climate-smart practices (Nganga et al., 2019). This observation aligns with Akzar et al. (2023), who emphasised the importance of cooperative membership in embracing complementary dairy feed technologies. This pattern is also consistent with the findings of Bacon et al. (2012), Pinto et al. (2020a, 2020b), Chong et al. (2023), and Guo et al. (2023), who discovered that substantial social capital promotes the adoption of key climate-smart strategies, including improved breeds, fodder production, irrigation, and livestock manure management. The literature also suggests that agricultural cooperatives can play a significant role in promoting the adoption of green technologies among farmers. Cooperatives can facilitate the diffusion of green technologies through technical training, breaking down the knowledge barriers that may hinder farmers from adopting these practices (Chong et al., 2023). Additionally, cooperatives can help establish market-based incentives for the adoption of green technologies, as the social benefits generated by their use can be transformed into tangible benefits for the adopters through market transactions (Guo et al., 2023).
Entrepreneurial orientation was positively and significantly associated with GHG reduction, highlighting the importance of proactive and informed decision-making. This finding implies that farmers with higher entrepreneurial orientation were slightly more likely to adopt GHG-reducing strategies. This tendency is supported by research emphasizing the role of entrepreneurial spirit in embracing sustainable agricultural technologies, as reported by Barzola Iza and Dentoni (2020), Daneluz et al. (2021), and Wang et al. (2023). Entrepreneurial orientation constructs such as risk-taking, innovativeness, and proactiveness enable farmers to implement innovative climate-smart practices (Kangogo et al., 2021).
Perceived behavioral control was positively associated with a reduction in GHG emissions. Perceived behavioral control reflects farmers’ confidence in their ability to perform specific behaviors. When farmers believe they have the necessary skills and resources, they are more likely to adopt GHG-reducing practices. This sense of control can stem from access to information, extension services, and supportive networks. According to Ngigi et al. (2018) and Kirungi et al. (2023), farmers require support such as technical training and resources from the government and other actors to implement climate-smart agricultural technologies. This finding also aligns with Li et al. (2020) and Elahi et al. (2021), who demonstrated a correlation between farmers who perceive themselves as possessing adequate skills and their increased propensity to adopt sustainable production practices in cattle production.
Awareness of CSDS was another significant factor in explaining the uptake of GHG reduction strategies. Access to information on the benefits of CSDS such as increased milk production, and reduced cost of production increased the uptake of GHG reduction measures. Maina et al. (2020), Li et al. (2023), and Mburu et al. (2024) suggest that a comprehensive understanding of CSDS encourages the adoption of a wide range of practices, particularly when farmers recognise the potential for increased production and reducing GHG emissions.
Behavioral intention and risk perception negatively affected GHG reduction supporting studies such as (Gebre et al., 2023). Although farmers recognise the risks of climate change, factors like uncertainty about the effectiveness of new practices, fear of potential losses, and lack of immediate benefits can lead to inaction (Wossen et al., 2019). This finding potentially reflects hesitation or aversion to adopting mitigation practices due to perceived risks or uncertainties. A possible explanation for this evidence is that farmers tend to prioritise strategies that enhance milk production, often overlooking those that do not contribute directly to increased output (Kogo et al., 2022). This finding appears to contradict Amamou et al. (2018), who found that climate change risks such as new diseases, reduced animal fertility, decreased milk production, reduced longevity, and feed unavailability increased the likelihood of adopting climate-smart practices (Gebre et al., 2023). Likewise, the intention to adopt GHG reduction measures was negatively related to GHG emissions reduction. A possible explanation could be that factors such as limited resources, lack of immediate benefits, and socio-cultural barriers can impede the realisation of intentions. For instance, a study by Gikunda et al. (2022) reported that communication barriers with extension agents reduced the intentions to adopt climate-smart practices. Similar findings were reported by Kashangaki and Ericksen (2018). This study’s finding contradicts the result of Kirungi et al. (2023), who found that intention to adopt significantly influenced the uptake of climate-smart technologies among farmers. These results highlight the complexity of the decision-making process in adopting sustainable dairy practices.
This study relied on the self-reported adoption status of the surveyed households. The self-reporting method may introduce response bias, as respondents may try to present certain images of themselves to the researcher. Literature indicates that self-reported data can be prone to misclassification, leading to biased estimates of adoption rates and associated outcomes (Wossen et al., 2019). To overcome response distortion, this study explained to the respondents the importance of the exercise and its contents in informing policy decisions. Future studies could consider cross-validating self-reported data with observational methods to address measurement errors in the self-reported adoption status. Moreover, a detailed analysis of external factors influencing adoption beyond farmers’ characteristics would enhance the understanding of the drivers of GHG reduction measures.
5 Conclusion
This study reveals several critical determinants of GHG reduction practices among farmers, encompassing demographic, socio-economic, social capital, institutional, entrepreneurial, and behavioral factors. These findings offer significant insights into areas where targeted policies and interventions could promote climate-smart agricultural practices. Policies should prioritise enhancing farmers’ knowledge through educational initiatives, as higher educational attainment strongly correlates with adopting GHG-reducing strategies. Promoting advanced livestock management practices can also play a significant role in climate change mitigation. Strengthening social capital by supporting agricultural groups and networks is essential for facilitating knowledge sharing, peer learning, and resource access, as well as encouraging sustainable practices. Addressing behavioral barriers such as risk aversion and resource constraints through targeted interventions, including risk mitigation strategies and financial support, is critical for the broader adoption of climate-smart agriculture.
To translate these insights into action, several stakeholders must play a role. Agricultural extension officers should focus on delivering localized training on GHG-reducing practices, particularly those tied to improved livestock feeding, manure management, and animal health management. Regular on-farm demonstrations and follow-up visits can enhance practical adoption and confidence among farmers, especially those with limited formal education.
Dairy cooperatives are well-positioned to drive peer learning by facilitating farmer field schools and incentivizing members who adopt GHG-reducing practices through preferential access to markets and feed subsidies. Cooperatives can also partner with service providers to offer bundled climate advisory services alongside input delivery.
Local governments should integrate climate-smart agriculture into county-level agricultural extension plans and budgets. This includes financing training programs for extension staff on climate resilience, creating ward-level climate response plans, and supporting the establishment of innovation platforms that bring together farmers, researchers, and private sector actors. Local governments can also provide targeted subsidies, or financial support to smallholder farmers adopting GHG-reducing technologies.
An integrated policy approach combining education, social capital enhancement, technical training, and financial incentives is necessary to drive the implementation of GHG-reducing practices. However, the potential impact of these policy interventions requires careful evaluation to ensure their effectiveness and adaptability to diverse agricultural contexts, such as wards. Additionally, further research is needed to understand the nuanced relationship between risk perception, resource availability, and adoption behavior, enabling the design of more effective and evidence-based interventions.
Data availability statement
The datasets presented in this study can be found in online repositories. The names of the repository/repositories and accession number(s) can be found below: https://github.com/Naomi-Chebi/Naomi-Chebiwot-Chelang-a/commit/4f7798ff7fa6c0306a8f77a5d6490484ef3fb6b9.
Ethics statement
The studies involving humans were approved by National Commission for Science Technology and Innovation. The studies were conducted in accordance with the local legislation and institutional requirements. The participants provided their written informed consent to participate in this study. Written informed consent was obtained from the individual(s) for the publication of any potentially identifiable images or data included in this article.
Author contributions
NC: Writing – original draft, Formal analysis, Resources, Visualization, Writing – review & editing, Project administration, Methodology, Validation, Investigation, Conceptualization, Data curation, Software. MM: Supervision, Writing – review & editing, Investigation, Conceptualization, Methodology. DO: Writing – review & editing, Conceptualization, Supervision. MS: Writing – review & editing, Supervision, Writing – original draft, Conceptualization.
Funding
The author(s) declare that no financial support was received for the research and/or publication of this article.
Conflict of interest
The authors declare that the research was conducted in the absence of any commercial or financial relationships that could be construed as a potential conflict of interest.
Generative AI statement
The author(s) declare that no Gen AI was used in the creation of this manuscript.
Publisher’s note
All claims expressed in this article are solely those of the authors and do not necessarily represent those of their affiliated organizations, or those of the publisher, the editors and the reviewers. Any product that may be evaluated in this article, or claim that may be made by its manufacturer, is not guaranteed or endorsed by the publisher.
References
Abegunde, V. O., Sibanda, M., and Obi, A. (2020). Determinants of the adoption of climate-smart agricultural practices by small-scale farming households in king Cetshwayo district municipality, South Africa. Sustain. For. 12:195. doi: 10.3390/su12010195
Aguirre-Villegas, H. A., Larson, R. A., Rakobitsch, N., Rakobitsch, N., Wattiaux, M. A., and Silva, E. (2022). Farm level environmental assessment of organic dairy systems in the US. J. Clean. Prod. 363:132390. doi: 10.1016/j.jclepro.2022.132390
Akzar, R., Peralta, A., and Umberger, W. (2023). Adoption of dairy feed technology bundles improves smallholder dairy farmers’ milk production. J. Agribus. Dev. Emerg. Econ. 15, 269–287. doi: 10.1108/JADEE-12-2022-0267
Alsayed, A. R. M., and Malik, A. (2020). Detecting the environmental Kuznets curve in African countries. Stud. Econ. Econ. 44, 35–44. doi: 10.1080/10800379.2020.12097355
Amamou, H., Sassi, M. B., Aouadi, H., Amamou, H., Sassi, M. B., Aouadi, H., et al. (2018). Climate change-related risks and adaptation strategies as perceived in dairy cattle farming systems in Tunisia. Clim. Risk Manag. 20, 38–49. doi: 10.1016/j.crm.2018.03.004
Asante, B. O., Ma, W., Prah, S., and Temoso, O. (2024). Farmers’ adoption of multiple climate-smart agricultural technologies in Ghana: determinants and impacts on maize yields and net farm income. Mitig Adapt Starat Gl 29, 1–23. doi: 10.1007/s11027-024-10114-8
Bacon, C. M., Getz, C., Kraus, S., Montenegro, M., and Holland, K. (2012). The social dimensions of sustainability and change in diversified farming systems. Ecol. Soc. 17, 41–60. doi: 10.5751/es-05226-170441
Balaganesh, G., Malhotra, R., Sendhil, R., Sirohi, S., Maiti, S., Ponnusamy, K., et al. (2020). Development of composite vulnerability index and district level mapping of climate change-induced drought in Tamil Nadu, India. Ecol Indic 113:106197. doi: 10.1016/j.ecolind.2020.106197
Barzola Iza, C. L., and Dentoni, D. (2020). How entrepreneurial orientation drives farmers’ innovation differential in Ugandan coffee multi-stakeholder platforms. J. Agribus. Dev. Emerg. Econ. 10, 629–650. doi: 10.1108/JADEE-01-2020-0007
Bohvalovs, G., Kalnbaļķīte, A., and Pakere, I. (2023). Driving sustainable practices in vocational education infrastructure: a case study from Latvia. Sustain. For. 15:10998. doi: 10.3390/su151410998
Brody, S. D., and Ryu, H. (2006). Measuring the educational impacts of a graduate course on sustainable development. Environ. Educ. Res. 12, 179–199. doi: 10.1080/13504620600688955
Chand, P., Sirohi, S., and Sirohi, S. K. (2015). Development and application of an integrated sustainability index for smallholder dairy farms in Rajasthan, India. Ecol. Indic. 56, 23–30. doi: 10.1016/j.ecolind.2015.03.020
Chong, D., Wang, H., Long, W., Ma, J., Ma, J., and Cui, Y. (2023). Can agricultural cooperatives promote Chinese farmers’ adoption of green technologies? Int. J. Environ. Res. Public Health 20, 40–51. doi: 10.3390/ijerph20054051
County Government of Nyandarua. (2023). Nyandarua county integrated development plan (CIDP3) draft 1. Nyandarua, Kenya. Available online at: http://www.nyandarua.go.ke/wp-content/uploads/2023/01/CIDP3_DRAFT.pdf
Dabkiene, V., Balezentis, T., and Streimikiene, D. (2021). Development of Agri-environmental footprint indicator using the FADN data: tracking development of sustainable agricultural development in Eastern Europe. Sustain. Prod. Consump. 27, 2121–2133. doi: 10.1016/j.spc.2021.05.017
Daneluz, M., Canever, M. D., Lima, H. G. D., Bermudes, R. F., and Menezes, G. (2021). Linking entrepreneurial orientation and managerial capacity to performance in dairy farms. Rev. Econ. Sociol. Rural. 60:e229910. doi: 10.1590/1806-9479.2021.229910
Elahi, E., Zhang, H., Lirong, X., Khalid, Z., and Xu, H. (2021). Understanding cognitive and socio-psychological factors determining farmers’ intentions to use improved grassland: implications of land use policy for sustainable pasture production. Land Use Policy 102:105250. doi: 10.1016/j.landusepol.2020.105250
Ericksen, P. J., and Crane, T. A. (2018). The feasibility of low emissions development interventions for the east African livestock sector: Lessons from Kenya and Ethiopia. ILRI Research Report. Nairobi, Kenya. Available online at: https://ccafs.cgiar.org/donors
FAO (2016) Reducing enteric fermentation for improving food security and livelihoods, Rome, Italy. Available online at: https://www.fao.org/3/i5902e/i5902e.pdf
FAO. (2017). Livestock solutions for climate change. Rome, Italy. Available online at: https://www.fao.org/3/i8098e/i8098e.pdf
FAO. (2019). Options for low-emission development in the Tanzania dairy sector: Reducing enteric methane for food security and livelihoods, Rome, Italy. Available online at: https://www.fao.org/documents/card/ru/c/CA3215EN
Gebre, G. G., Amekawa, Y., and Fikadu, A. A. (2023). Farmers′ use of climate change adaptation strategies and their impacts on food security in Kenya. Clim. Risk Manag. 40:100495. doi: 10.1016/j.crm.2023.100495
Gikunda, R., Lawver, D., and Magogo, J. (2022). Culture as a predictor of effective adoption of climate-smart agriculture in Mbeere North, Kenya. Adv. Agric. Dev. 3, 48–61. doi: 10.37433/aad.v3i2.203
Graham, M. W., du Butterbach-Bahl, K., and Toit, C. L. (2022). Research progress on greenhouse gas emissions from livestock in sub-Saharan Africa falls short of national inventory ambitions. Front. Soil Sci. 2:927452. doi: 10.3389/fsoil.2022.927452
Greco, S., Ishizaka, A., Tasiou, M., and Torrisi, G. (2019). On the methodological framework of composite indices: a review of the issues of weighting, aggregation, and robustness. Soc. Indic. Res. 141, 61–94. doi: 10.1007/s11205-017-1832-9
Guo, Y., Cui, M., and Xu, Z. (2023). Spatial characteristics of transfer plots and conservation tillage technology adoption: evidence from a survey of four provinces in China. Agriculture 13:1601. doi: 10.3390/agriculture13081601
Hawkins, J. W., Komarek, A. M., Kihoro, E. M., Nicholson, C. F., Omore, A. O., Yesuf, G. U., et al. (2022). High-yield dairy cattle breeds improve farmer incomes, curtail greenhouse gas emissions, and reduce dairy import dependency in Tanzania. Nat. Food 3, 957–967. doi: 10.1038/s43016-022-00633-5
Herrero, M., Henderson, B., and Havlí, P. (2016). Greenhouse gas mitigation potentials in the livestock sector. Nat. Clim. Chang. 6, 452–461. doi: 10.1038/nclimate2925
Ibidhi, R., and Calsamiglia, S. (2020). Carbon footprint assessment of z dairy cattle farms: effectiveness of dietary and farm management practices as a mitigation strategy. Animals 10:2083. doi: 10.3390/ani10112083
Kandulu, J. M., Zuo, A., Wheeler, S., Dusingizimana, T., and Chagunda, M. G. (2024). Influence of climate-smart technologies on the success of livestock donation programs for smallholder farmers in Rwanda. Mitig. Adapt. Strat. Gl. 29, 1–27. doi: 10.1007/s11027-024-10120-w
Kangogo, D., Dentoni, D., and Bijman, J. (2021). Adoption of climate-smart agriculture among smallholder farmers: does farmer entrepreneurship matter? Land Use Policy 109:105666. doi: 10.1016/j.landusepol.2021.105666
Kashangaki, J., and Ericksen, P. (2018). Cost-benefit analysis of fodder production as a low emissions development strategy for the Kenyan dairy sector. Available online at: https://cgspace.cgiar.org/handle/10568/97426
Kihoro, E. M., Schoneveld, G. C., and Crane, T. A. (2021). Pathways toward inclusive low-emission dairy development in Tanzania: producer heterogeneity and implications for intervention design. Agric. Syst. 190:103073. doi: 10.1016/j.agsy.2021.103073
Kirungi, D., Senyange, B., Wesana, J., Sseguya, H., Gellynck, X., and De Steur, H. (2023). Entrepreneurial and attitudinal determinants for adoption of climate-smart agriculture technologies in Uganda. Cogent Food Agric. 9:2282236. doi: 10.1080/23311932.2023.2282236
Kogo, B. K., Kumar, L., and Koech, R. (2022). Response to climate change in a rain-fed crop production system: insights from maize farmers of western Kenya. Mitig. Adapt. Strat. Gl. 27:50. doi: 10.1007/s11027-022-10023-8
Korir, L., Manning, L., and Moore, H. L. (2023). Adoption of dairy technologies in smallholder dairy farms in Ethiopia. Front. Sustain. Food Syst. 7:1070349. doi: 10.3389/fsufs.2023.1070349
Kumar, S., Raizada, A., Biswas, S., and Mondal, B. (2016). Application of indicators for identifying climate change vulnerable areas in semi-arid regions of India. Ecol. Indic. 70, 507–517. doi: 10.1016/j.ecolind.2016.06.041
Li, J., Feng, S., Luo, T., and Guan, Z. (2020). What drives the adoption of sustainable production technology? Evidence from the large scale farming sector in East China. J. Clean. Prod. 257:120611. doi: 10.1016/j.jclepro.2020.120611
Li, J., Liu, G., Chen, Y., and Li, R. (2023). Study on the influence mechanism of adoption of smart agriculture technology behavior. Sci. Rep. 13:8554. doi: 10.1038/s41598-023-35091-x
Maina, K. W., Ritho, C. N., Lukuyu, B. A., and Rao, E. J. O. (2020). Socio-economic determinants and impact of adopting climate-smart Brachiaria grass among dairy farmers in eastern and Western regions of Kenya. Heliyon 6:e04335. doi: 10.1016/j.heliyon.2020.e04335
Maindi, N. C., Osuga, I. M., and Gicheha, M. G. (2020). Advancing climate smart agriculture: adoption potential of multiple on-farm dairy production strategies among farmers in Murang’a county, Kenya, Livestock Res. Rural Dev. 32. Available online at: https://www.researchgate.net/profile
Mburu, M., Mburu, J., Nyikal, R., Mugera, A., and Ndambi, A. (2024). Role of agricultural extension in learning for uptake and intensification of less-practiced dairy climate-smart practices in Kenya. Cogent Food Agric. 10:2330182. doi: 10.1080/23311932.2024.2330182
Mwaura, F. M., Ngigi, M. W., and Obare, G. (2024). Do smallholders have a role to play in atmospheric greenhouse gas removal? Insights from western Kenya. Sci. Afric. 24:e02206. doi: 10.1016/j.sciaf.2024.e02206
Nganga, S. K., Jalango, D. A., and Girvetz, E. (2019). Adoption of technologies that enhance soil carbon sequestration in East Africa. What influence farmers’ decision? Int. Soil Water Conserv. Res. 8, 90–101. doi: 10.1016/j.iswcr.2019.11.001
Ngigi, M. W., Müller, U. K., and Birner, R. (2018). Farmers’ intrinsic values for adopting climate-smart practices in Kenya: empirical evidence from a means-end chain analysis. Clim. Dev. 10, 614–624. doi: 10.1080/17565529.2018.1442786
Ntinyari, W., and Gweyi-Onyango, J. P. (2020). “Greenhouse gases emissions in agricultural systems and climate change effects in sub-Saharan Africa” in African handbook of climate change adaptation (eds.) W. Leal Filho, N. Oguge, D. Ayal, L. Adeleke, and I. da Silva. (Cham: Springer International Publishing), 1–25. doi: 10.1007/978-3-030-42091-8_43-1
O’Hara, J. K. (2023). State-level trends in the greenhouse gas emission intensity of US milk production. J. Dairy Sci. 106, 5474–5484. doi: 10.3168/jds.2022-22741
Ongare, D., Macharia, A., Ndegwa, G. M., and Kidundo, M. (2016). Mainstreaming sustainability considerations into courses in a Kenyan university. Int. J. High. Educ. Sustain. 1, 119–132. doi: 10.1504/ijhes.2016.080777
Pinto, A. D., Cenacchi, N., Kwon, H., Koo, J., and Dunston, S. (2020a). Climate smart agriculture and global food-crop production. PLoS One 15:e0231764. doi: 10.1371/journal.pone.0231764
Pinto, A., Yin, T., Reichenbach, M., Malik, P. K., Schlecht, E., and König, S. (2020b). Enteric methane emissions of dairy cattle considering breed composition, pasture management, housing conditions and feeding characteristics along a rural-urban gradient in a rising megacity. Agriculture 10:628. doi: 10.3390/agriculture10120628
Rotz, C. A. (2018). Modeling greenhouse gas emissions from dairy farms. J. Dairy Sci. 101, 6675–6690. doi: 10.3168/jds.2017-13272
Sambrumo, G. M., Ngigi, M. W., and Mutai, B. K. (2022). Influence of agripreneurial orientation constructs on growth of cassava-based small and medium enterprises in Migori County, Kenya. Asian Res. J. Agric. 15, 45–55. doi: 10.9734/arja/2022/v15i430166
Sendhil, R., Jha, A., Kumar, A., and Singh, S. (2018). Extent of vulnerability in wheat producing agroecologies of India: tracking from indicators of cross-section and multi-dimension data. EcolIndic 89, 771–780. doi: 10.1016/j.ecolind.2018.02.053
Shahbaz, P., Haq, S. U., Abbas, A., Azadi, H., Boz, I., Yu, M., et al. (2023). Role of Farmers’ entrepreneurial orientation, Women’s participation, and information and communication technology use in responsible farm production: a step towards sustainable food production. Front. Sustain. Food Syst. 7:1248889. doi: 10.3389/fsufs.2023.1248889
Vernooij, V., Schoneveld, G., and Vellema, S. (2024). From fix to fitting: connecting low-emission development with multilevel smallholder dairy practices in Kenya. NJAS Impact Agric. Life Sci. 96:2334965. doi: 10.1080/27685241.2024.2334965
Wamuyu, S. N., Bett, H. K., Kariuki, I. M., and Cadot, J. (2023). Influence of climate-smart agricultural technologies and management practices on relative sustainability of smallholder potato farmers in Kenya. Rigorous J. Agric. Sci. 1, 34–47. doi: 10.70255/2022v1i1/205
Wang, S., Höhler, J., and Ang, F. (2023). Dutch dairy farmers’ adoption of climate mitigation measures. The role of socio-psychological and socio-demographical factors. J. Clean. Prod. 427:139187. doi: 10.1016/j.jclepro.2023.139187
Wilkes, A., Wassie, S., Fraval, S., and Dijk, S. (2020). Variation in the carbon footprint of milk production on smallholder dairy farms in Central Kenya. J. Clean. Prod. 265:121780. doi: 10.1016/j.jclepro.2020.121780
Wossen, T., Alene, A., Abdoulaye, T., Feleke, S., and Manyong, V. (2019). Agricultural technology adoption and household welfare: measurement and evidence. Food Policy 87:101742. doi: 10.1016/j.foodpol.2019.101742
Xu, J., Wang, J., Wang, T., and Li, C. (2023). Impact of industrial agglomeration on carbon emissions from dairy farming-empirical analysis based on life cycle assessments method and spatial Durbin model. J. Clean. Prod. 406:137081. doi: 10.1016/j.jclepro.2023.137081
Yamane, T. (1967). Statistics: An introductory analysis, 2nd edition. New York: Harper and Row. Available online at: http://www.sciepub.com/reference/180098
Zehetmeier, M., Baudracco, J., Hoffmann, H., and Heißenhuber, A. (2012). Does increase milk yield per cow reduce greenhouse gas emissions? A system approach. Animal 6, 154–166. doi: 10.1017/s1751731111001467
Keywords: smallholder farmers, adoption, climate-smart dairy strategies, greenhouse gas reduction, Kenya
Citation: Chelang’a NC, Mathenge M, Otieno DO and Sassi M (2025) The determinants of greenhouse gas reduction levels among smallholder farmers: insights from the adoption of climate-smart dairy strategies in Central Kenya. Front. Clim. 7:1593584. doi: 10.3389/fclim.2025.1593584
Edited by:
Titus Zindove, Lincoln University, New ZealandReviewed by:
Hasitha Priyashantha, Swedish University of Agricultural Sciences, SwedenMupenzi Mutimura, Rwanda Agriculture Board, Rwanda
Luxolo Qokweni, Department of Rural Development and Agrarian Reform, South Africa
Copyright © 2025 Chelang’a, Mathenge, Otieno and Sassi. This is an open-access article distributed under the terms of the Creative Commons Attribution License (CC BY). The use, distribution or reproduction in other forums is permitted, provided the original author(s) and the copyright owner(s) are credited and that the original publication in this journal is cited, in accordance with accepted academic practice. No use, distribution or reproduction is permitted which does not comply with these terms.
*Correspondence: Naomi Chebiwot Chelang’a, bmFvbWljaGViaTA5QGdtYWlsLmNvbQ==
†ORCID: Naomi Chebiwot Chelang’a, https://orcid.org/0000-0001-9590-0527
Mary Mathenge, https://orcid.org/0009-0008-1304-050X
Dickson Okello Otieno, https://orcid.org/0000-0002-7867-3753
Maria Sassi, https://orcid.org/0000-0002-6114-6826