- 1Department of Electrical Engineering, National Tsing Hua University, Hsinchu, Taiwan
- 2MOST Joint Research Center for AI Technology and All Vista Healthcare, Taipei, Taiwan
Research in advancing speech emotion recognition (SER) has attracted a lot of attention due to its critical role for better human behaviors understanding scientifically and comprehensive applications commercially. Conventionally, performing SER highly relies on hand-crafted acoustic features. The recent progress in deep learning has attempted to model emotion directly from raw waveform in an end-to-end learning scheme; however, this particular approach remains to be generally a sub-optimal approach. An alternative direction has been proposed to enhance and augment the knowledge-based acoustic representation with affect-related representation derived directly from raw waveform. Here, we propose a complimentary waveform-feature dual branch learning network, termed as Dual-Complementary Acoustic Embedding Network (DCaEN), to effectively integrate psychoacoustic knowledge and raw waveform embedding within an augmented feature space learning approach. DCaEN contains an acoustic feature embedding network and a raw waveform network, that is learned by integrating negative cosine distance constraint in the loss function. The experiment results show that DCaEN can achieve 59.31 an 46.73% unweighted average recall (UAR) in the USC IEMOCAP and the MSP-IMPROV speech emotion databases, which improves the performance compared to modeling either acoustic hand-crafted features or raw waveform only and without this particular loss constraint. Further analysis illustrates a reverse mirroring pattern in the learned latent space demonstrating the complementary nature of DCaEN feature space learning.
1. Introduction
Human's internal affective states influence our high-level cognitive processing that is reflected both in our daily decision making and manifested in our behaviors. Enabling machines to assess human emotion has become an important research direction that impacts the current proliferation of human-centered technology. The ability to perform emotion recognition from speech has shown to be beneficial across a wide range of current speech-based interfacing applications, e.g., intelligent commercial dialog system (Callejas et al., 2011), personalized recommendation system (Tkalcic et al., 2011), and health care service (Pentland, 2004; Tokuno et al., 2011; Mano et al., 2016). Research in algorithmic development for speech emotion recognition (SER) has started off by computing hand-crafted engineered features, such as mel-frequency cepstral coefficients (MFCCs), perceptual linear prediction (PLP) coefficients, and supra-segmental features like prosodic descriptors. These acoustic descriptors are designed based on decades of research in auditory production and speech perception, and they have been consistently applied for many paralinguistic recognition tasks such as the series of ComParE (Schuller et al., 2013) and AVEC (Ringeval et al., 2015) challenges. Furthermore, prior to deep learning, researchers utilized supervised models such as hidden markov model (HMM) (Nwe et al., 2003; Mao et al., 2009), support vector machine (SVM) (Lin and Wei, 2005; Chavhan et al., 2010), and Gaussian mixture model (GMM) (Neiberg et al., 2006; Hu et al., 2007), to perform emotion recognition.
Much of the recent improvement in the SER system comes from the advancement of the deep learning based recognition frameworks, i.e., replacing the classifier portion with different neural network architectures. For example, neural network based recognition methods such as feedforward neural network (Li et al., 2013; Han et al., 2014), 1-D and 2-D convolutional neural networks (Huang et al., 2014; Badshah et al., 2017; Zhao et al., 2019), recurrent neural network (RNN) (Lee and Tashev, 2015; Mirsamadi et al., 2017) and hybrid models combining different network architectures (Lim et al., 2016) have all been shown to exceed performances of traditional supervised machine learning methods. Even though there are already a number of effective deep learning frameworks proposed, most of these architectures still heavily rely on the hand-crafted acoustic features as front-end input (Huang et al., 2018; Jiang et al., 2019; Shahin et al., 2019), which continue to require extensive knowledge for emotion-related acoustic feature design and engineering (El Ayadi et al., 2011).
Deep learning based methods provides not only an improved recognition framework but more importantly an ability to automatically learn representation directly from the raw data. Researchers have also started to investigate end-to-end approaches for task of SER, i.e., learning to classify emotion states directly from time-domain speech waveform. For example, 1-D time domain raw waveform is modeled by multiplying parallel 1-D convolution layers and pooling layers using a variety of filter parameter design within each layer and finally feeds the learned layer representation to a CNN-LSTM architecture for recognition (Latif et al., 2019). A similar end-to-end approach is also proposed by using a different choice of convolutional kernels in terms of overlapping ratio, kernel size and pooling size (Tzirakis et al., 2018). Another research uses time-delay neural network (TDNN) layers and unidirectional recurrent long short term memory (LSTM) layer with time restricted attention layers to tackle the issue of long temporal context for raw speech emotion identification task (Sarma et al., 2018).
While several of these works have invested extensive effort in the design of sophisticated network architectures in order to perform end-to-end emotion recognition, performances of using raw waveform can still hardly surpass most of those past works on using hand-crafted acoustic features or those that based on using mel-frequency spectrograms as input (Yenigalla et al., 2018; Badshah et al., 2019; Tripathi et al., 2019); furthermore, modeling raw waveform tends to require extensive network parameter tuning and non-trivial techniques in dealing with the complexity (frequency-phase interaction) in time domain. An alternative recent direction has emerged that leverages the complementary information between knowledge-inspired acoustic descriptors and end-to-end acoustic representation (either time domain-derived or mel-frequency domain-derived), specifically, augmenting these two acoustic representations has been found to effectively enhance the accuracy of emotion recognition. For example, Lakomkin et al. propose a progressively trained neural network that transfer knowledge of automatic speech recognition to emotion recognition by augmenting feature space of spectrogram jointly with MFCC and pitch (Lakomkin et al., 2017). Yang et al. uses convolutional neural network (CNN) to model raw waveform and spectrogram separately and fuse these two different representation streams with a bidirectional long short-term memory (BLSTM) (Yang and Hirschberg, 2018). Guo et al. (2018) designs a CNN-based representation learning approach to extract both amplitude and phase information and demonstrates that the joint representation can outperforms other conventional methods. Most recently, Guo et al. further proposes a dynamic fusion network using kernel extreme learning machine (KELM) to consider both spectrogram and knowledge-inspired acoustic features (Guo et al., 2019).
These recent works demonstrate that end-to-end acoustic representation indeed complement hand-crafted acoustic features, and by fusing these two inputs together, SER performances can be further improved compared to either end-to-end only or knowledge-inspired acoustic representation only. However, all of these previously-proposed frameworks have merely stacked the separately-learned network layers, e.g., one from raw waveform and another from knowledge-inspired features, as the fusion scheme. This type of method ignores the naturally-existed redundancy between the two representations and create a potential sub-optimal solution for SER, i.e., not dealing explicitly with the complex interaction between different types of feature inputs in a fine-grained manner. Hence, in our previous work (Huang et al., 2019), we propose an waveform-feature dual branch acoustic network learning framework called Dual Complementary Acoustic Embedding Network (DCaEN) to learn an augmented acoustic representation space that embeds both knowledge based acoustic features and the extra complementary cues derived from raw waveform to improve the emotion recognition accuracy on the USC IEMOCAP database (Busso et al., 2008). The learning of complementary information depends on the introduction of a negative cosine constraint loss term between the hand-crafted features and raw waveform and further jointly optimizes with respect to the emotion discriminative loss. In this work, we further extend our proposed DCaEN framework with the following specific contribution:
• Evaluate and analyze the DCaEN on two different large scale SER corpus, i.e., the USC IEMOCAP and the MSP IMPROV (Busso et al., 2016), in order to further demonstrate the effectiveness and robustness of our framework.
• Compare the accuracy obtained when using different hand-crafted acoustic features sets, i.e., the 88 dimensional eGeMAPS (Eyben et al., 2016) and the 1,536 dimensional EmoBase2010 (Schuller et al., 2010).
• Perform additional analysis in understanding the acoustic properties of the learned complementary embedding from raw waveform.
In this work, our proposed DCaEN, i.e., trained jointly with end-to-end and hand-crafted acoustics features using complementary constraint, obtains the highest emotion recognition rates as compared to using single feature representation and/or augmented representations without the complementary constraint. Specifically, we obtain 59.31 and 59.00% unweighted average recall (UAR) in a four-class emotion classification tasks when using the eGeMAPS and the EmoBase2010 feature sets on the IEMOCAP database, it also obtains the highest accuracy of 44.42 and 46.73% on the MSP IMPROV dataset using the eGeMAPS and the EmoBase2010. We further present two different analyses on the effect of imposing this complementary constraint on the two corpora, and both analyses results show a similar trend, i.e., learning a more negatively-correlated (i.e., not simply orthogonal) representations between the two feature spaces tend to result in a higher accuracy rates, and the two learned representations also show to occupy a mirroring space with respect to each other. Furthermore, we report a correlation analysis to demonstrate the potential properties of the learned complementary embedding from the raw waveform.
2. Methods
2.1. Emotion Database
We evaluate our framework on two different databases, the USC IEMOCAP (Busso et al., 2008) database and the MSP-IMPROV database (Busso et al., 2016). We will briefly describe each database below.
2.1.1. The IEMOCAP Database
We use the USC Interactive Emotional Dyadic Motion Capture (IEMOCAP) database (Busso et al., 2008) to evaluate our proposed framework. The database consists of 5 sessions acted by 10 different actors (including 5 males and 5 females). The database has approximately 12 h of data segmented manually into utterances. Each utterance is annotated by at least three annotators on 10 categorical emotion labels. In our experiment, we follow the exact same experimental setting used in a previous work (Fayek et al., 2017), i.e., using 4 emotion classes as the targeted labels: sadness, happiness (include excitement), anger, and neutral with a total of 5,531 utterances. The proportion distribution of these emotion classes is shown in Figure 1.
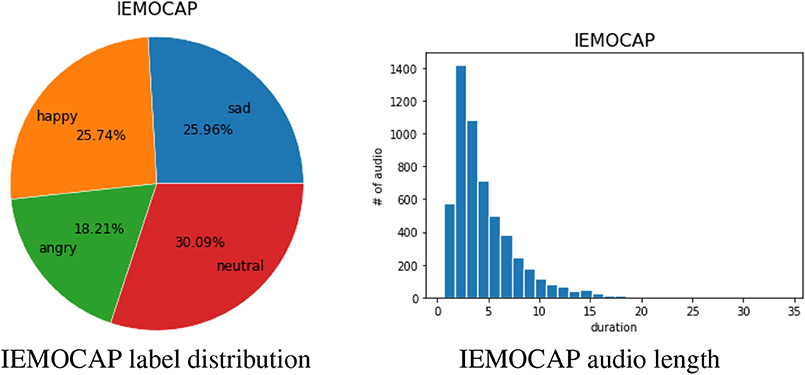
Figure 1. The 4-class emotion label distribution and the audio length distribution of IEMOCAP database.
2.1.2. The MSP-IMPROV Database
We also test our proposed model on the MSP-IMPROV database (Busso et al., 2016). It contains utterances of 12 speakers from a total of 6 sessions (each with a male and female pair). The speaker in the MSP-IMPROV database is asked to speak a specific sentence (target sentence) in each session in order to carry out a constrained improvisational dialog. In addition, the corpus is post-processed and split into four sub-sets: (i) target-improvised: target sentences during improvised sessions (ii) target-read: a read speech version of the target sentences, (iii) other-improvised: utterances in the improvisation dialogs other than the target sentences, and (iv) natural interaction: utterances made during the breaks between each improvisation session (i.e., when the actors are not acting). To be consistent with the IEMOCAP database, we use 7,798 utterances that are labeled as anger, happiness, neutrality, and sadness (792 angry, 2,644 happy, 3,477 neutral, and 885 sad). The proportion of each emotion category is shown below Figure 2. We can see that the distribution of emotion categories is more unbalance compared to the IEMOCAP. In addition, the distribution of duration of utterances is also shown blow Figure 2; the average duration of an utterance is 4.07 s.
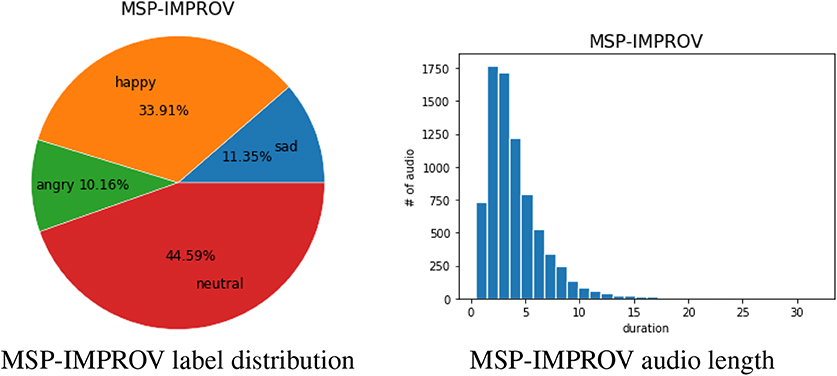
Figure 2. The 4-class emotion label distribution and the audio length distribution of MSP-IMPROV database.
2.2. Acoustic Features
2.2.1. Hand-Crafted Features
We utilize two different acoustic feature sets in this work including eGeMAPS and EmoBase2010; each will be described briefly in the following sections.
2.2.1.1. eGeMAPS
The extended Geneva Minimalistic Acoustic Parameter Set (eGeMAPS) (Eyben et al., 2016) has been developed as a minimal set of knowledge-inspired acoustic parameter set (88 dimensional functional features) that has been shown to be robust for SER across different databases (Aldeneh and Provost, 2017; Neumann and Vu, 2017; Han et al., 2018). The eGeMAPS acoustic feature set contains energy, spectral and frequency based low-level descriptors associated with functional set implemented with arithmetic mean, coefficient of variation, 20, 50, 80th percentile of pitch and loudness, mean and standard deviation of the slope of rising/falling signal parts. We extract the eGeMAPS feature set using openSMILE toolbox (Eyben et al., 2013) with 20ms frame size and 10ms step size.
2.2.1.2. EmoBase2010
We further use the openSMILE (Eyben et al., 2013) to extract a much higher dimension (1536 dimensional) functional acoustic feature, termed as the EmoBase2010. This 1536 dimensional acoustic feature set has been proposed in the Interspeech 2010 Paralinguistic Challenge (Schuller et al., 2010). The high dimensional feature set is composed by using a suite of functional operators on a larger (34) low level descriptors including 12 dimensional Mel-Frequency Cepstral Coefficients, fundamental frequency (F0), loudness, voice probability, zero crossing rate and the first and second derivatives of MFCCs. The above extracted low level descriptors are further combined with 21 different functionals in order to derive a high dimensional acoustic feature vector. This set of acoustic parameters have been applied successfully in various emotion recognition works when the number of feature dimensions do not need to be constrained nor minimal (Toledo-Ronen and Sorin, 2013; Chen et al., 2014; Sahu et al., 2018).
2.2.2. Raw Waveform
We pre-process the raw waveform by splitting each utterance audio recording into 6 s fix length segments. For those utterances shorter than 6 sections, we perform zero-padding. Each 6 s segment consists of 96,000 sample points (16 kHz sampling rate). We use a 640 dimensional input vector, i.e., gathered every 150 time step units, as input to our proposed DCaEN.
2.3. Dual Complementary Acoustic Embedding Network
The complete architecture is shown in Figure 3. Our proposed DCaEN framework comprises two sub-networks: Feature Network for hand-crafted features (Stage 1) and Complementary Learning Network for learning representation from raw waveform (Stage 2). We train the Feature Network using a stacked fully connected layer neural network in the first stage and then freeze it as a feature extractor. In the second stage, the output of the Complementary Learning Network that models raw waveform is learned with additional loss using a negative cosine distance to the output of Feature Network. Finally, the output of two networks learned in above stages are concatenated as an joint representation for emotion classification layers.
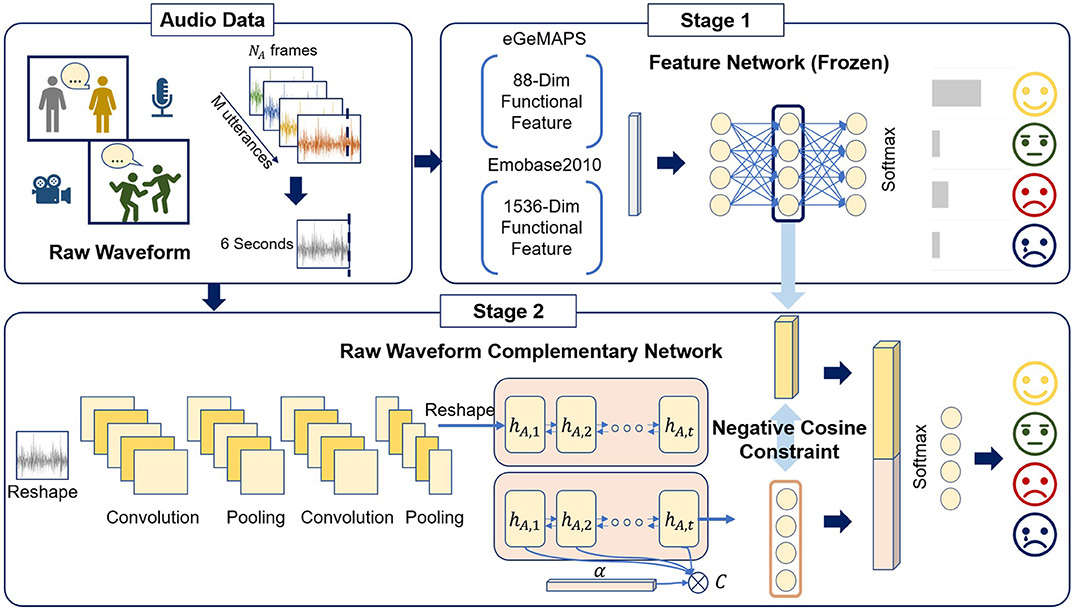
Figure 3. This is an overall schematic of our proposed Dual Complementary Acoustic Embedding Model (DCaEN) framework. The training process contains two stages: the first stage is learning the Feature Network and freezing the network; the second stage is building an end-to-end architecture to learn complementary embedding from raw waveform based on negative cosine loss constraint to the learned frozen Feature Network embedding. We finally concatenate both embeddings to perform the final emotion recognition.
2.3.1. Feature Network (Stage 1)
We implement the feature network using fully connected layers that embed information from hand-crafted acoustic features as input and end with softmax recognition layer. In this stage, the loss function is specified as categorical cross entropy. The embedding layer learns emotion related characteristics in terms of expert-designed psychoacoustic features. That is, by extracting the embedding output, we can obtain the knowledge-based summarization of acoustically emotion relevant information. We then freeze the learned Feature Network to provide an embedding that represents expert knowledge of acoustic manifestation of emotion in order to facilitate the complementary learning from raw waveform in stage 2.
2.3.2. Raw Waveform Complementary Network (Stage 2)
We build a CNN-LSTM neural network with two stacked 1-D convolutional layers followed by two LSTM layers with attention mechanism to learn representation from the raw waveform. The network is further connected to a complementary embedding layer and a final softmax dense layer for classification. The core idea in Raw Waveform Complementary Network is that raw waveform encompasses all aspects of acoustic information involving both human physical and psychological characteristics. Hence, our idea is to introduce a constraint to enforce the learning of the representation to be both emotionally relevant, and at the same time complementary to the expert-designed acoustic feature in order to mitigate the issue that the network converges to a representation that is affect irrelevant or redundant.
Specifically, we use negative cosine similarity as the complementary constraint (Equation 1) on the embedding layer to force the raw waveform embedding becoming negatively correlated to the extracted representation from the Feature Network. The constraint can be written as a loss function to be optimized shown as below:
where x1 and x2 represent the extracted embedding from Feature Network and the complementary embedding from Raw Waveform Complementary Network, respectively. The cosine complementary loss is added to the cross entropy loss and is jointly optimized in the learning of the whole Raw Waveform Complementary Network:
where w is a loss weighting term and the categorical cross entropy loss is written as:
In this complementary design of the DCaEN, the joint optimization of complementary loss and classification loss enables learning a latent complementary (with respect to hand-crafted feature) representation in order to enhance the emotional discriminative power as compared to simply learning directly from the waveform (without complementary loss). In our complete recognition framework, the two representations (Feature Network and Raw Waveform Complementary Network) are concatenated to form the final feature input, i.e., combining both expert knowledge and automatically derived raw waveform information, to the recognition layer. Note that with this particular optimization approach, the cosine similarity would tend to converge to value close to −1; however, in order to satisfy the classification loss, this complementary constraint would not directly equals to −1 as it will lead to an identical representation as the Feature Network embedding, which is a complete redundant representation deteriorating recognition performances. Detailed analysis on the effect of complementary loss value is presented in section 4.1.
3. Experiment
3.1. Experimental Setting
In our experiment, we use leave one dyad out cross validation scheme, i.e., to resemble the true application scenario where the two interacting interlocutors are both unseen in our training set, with unweighted average recall (UAR) as the evaluation metric for both databases. In both Feature Network and Raw Waveform Complimentary Network, we utilize Adam optimizer with learning rate set as 10−3 and train 20 epochs with mini-batch size of 32. The hand-crafted features are z-normalized for each dimension to zero mean and unit variance for each speaker. The Feature Network has a 64 nodes fully-connected layer with dropout.
For the Raw Waveform Complementary Network, each frame is convolved with 40 filters with kernel size of 20 to extract features from high sampling rate time domain signal, and then we downsample to 8 kHz by pooling each filter output with a pool size 2. To extract long-term characteristics of the speech and roughness of the speech signal, we convolve the pooled frame by kernel size 40 in each filter followed by max-pooling layer across the channel domain with a pool size of 10. Then, we use 2 stacked LSTMs each with 256 cells with attention mechanism to learn the sequential relation. Afterwards, a fully connected layer with 64 nodes is used to derive the representation from raw waveform data. The two constrained fully-connected layers from the frozen Feature Network and the Raw Waveform Complementary Network, are concatenated to form the final augmented representation (128 dimensional vector) for each sample. The weight of the complete loss function (Equation 2) is specified as w = 0.6 using grid-search.
3.1.1. Comparison of Front-End Models
The following front-end convolutional neural networks are compared as baseline for raw waveform modeling. We keep Raw Waveform Complimentary Network only without the cosine constraint while replacing the convolutional neural network with different well-known architectures. We implement VGG-like and Inception-like networks following the original design while reduce to 6 layers since deep layer suffer from serious overfitting problem in our context. Moreover, we examine the results based on a sequence-to-sequence architecture implemented with the software auDeep (Freitag et al., 2017), which has been used in the series of ComparE challenges for paralinguistics recognition from speech.
• P: The architecture proposed in a recent work (Tzirakis et al., 2018) that works directly with raw waveform for SER
• VGG: A VGG-like network with 6 convolutional layers
• Inception: A Inception-like network with 6 convolutional layers
• auDeep: An unsupervised acoustic sequence-to-sequence feature extractor (Freitag et al., 2017)
• Raw: Raw Waveform Complimentary Network without the cosine constraint.
3.1.2. Comparison of Framework Design
We also compare our framework with different components in our model in the following experiments in the IEMOCAP and the MSP-IMPROV databases using either eGeMAPS or EmoBase2010 as the hand-crafted feature sets. The comparison baselines are listed as below:
• Raw: Raw Waveform Complimentary Network only without the cosine constraint
• Ftr: Feature Network in stage 1
• nC: Dual network architecture with the same structure as our proposed DCaEN but learning without the cosine constraint
• CF: DCaEN with cosine similarity constraint applied directly on the hand-crafted features without the Feature Network embedding learning
• uF: DCaEN with unfrozen (adaptable) Feature Network that updates parameters simultaneously with the optimization of Raw Waveform Complementary Network in stage 2
• C0: DCaEN with targeted cosine similarity constraint converge close to 0 instead of −1
• R_C: Raw waveform network with cosine similarity constraint but without concatenating the embedding from the Feature Network.
• MSE: DCaEN minimizing mean square error constraint instead of cosine similarity.
3.2. Results
3.2.1. Recognition on the IEMOCAP
The results in comparing raw waveform models for the IEMOCAP are demonstrated in the left part of Table 1. Our proposed architecture achieves 52.82% unweighted average recall which is a 17.25, 16.60, 12.34, and 8.26% relative improvement compared to P, VGG, Inception, auDeep network structures. The architecture is designed specifically to model raw waveform, i.e., capturing spatio-temporal characteristics of raw waveform for emotion recognition, while most of these compared architecture are designed for other purposes (mostly object recognition tasks). Even for the structure of P, which is specialized in modeling raw waveform for SER, they assess only on the IEMOCAP database, where we have shown that the accuracy does not generalize to the MSP-IMPROV database. A complete experimental result summarizing different baselines for the IEMOCAP is shown in Table 2. The upper part of the table shows the results of using the eGeMAPS feature set in the Feature Network, and the lower part of the table provides the results using the Emobase2010 feature set. The details of features are described in sections 2.1, 2.2. The confusion matrix of the best performed results are shown in Figure 4.
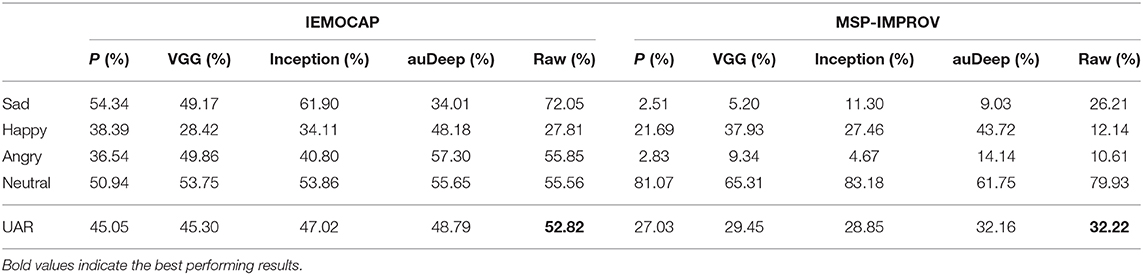
Table 1. Comparing emotion recognition performances of different front-end networks on the IEMOCAP and the MSP-IMPROV databases.
3.2.1.1. eGeMAPS
We obtain 59.31% UAR with our proposed DCaEN, which outperforms all other baselines. In the baseline model, Raw obtains 52.82% UAR that is lower than Ftr, which achieves 57.36%. This result reinforces past literature in indicating better discriminative power can be achieved by using the knowledge-based acoustic features compared to learning directly from the raw waveform. The recall of Raw for happiness category suffers from a significant drop from Ftr, and the recall of neutral class is also slightly worse than Ftr while the sadness category performs better than Ftr. The difference indicates that models with raw waveform and hand-crafted features possess distinct characteristics in terms of capturing different emotion classes, which further supports the idea to model these two feature streams within a complementary feature augmentation paradigm. When comparing to our proposed DCaEN, Raw and Ftr demonstrate 6.49 and 1.95% relative deterioration in accuracy rates.
To further investigate the effect in the network designs of DCaEN, we compare nC to examine the effect of our use of complementary constraint. nC model can be regard as a naive concatenation of both hand-crafted feature embedding and raw-waveform embedding, and the results barely improves (56.99%) likely due to highly redundant acoustic information exists in both embeddings. On the other hand, CF obtains 56.57% UAR that is also inferior than our proposed model. The 88-dimensional eGeMAPS features without Feature Network learning do not possess enough emotion discriminative information, and it may not serve as an adequate targeted frozen representation when carrying out complementary embedding learning. This demonstrates that by using hand-crafted feature directly for the complementary learning is not ideal. When examining the accuracy obtained using uF, i.e., the non-frozen Feature Network as embedding, it still performs better than using hand-crafted feature directly (CF) but lacks behind as compared to our proposed DCaEN, which requires the Feature Network to be frozen once learned. This result further suggests that the frozen Feature Network enforce the complementary learning to be robust without tuning excessive number of parameters.
Furthermore, in contrast to limit the complementary cosine similarity loss close to −1 (DCaEN), we compare to the results of a model with orthogonal cosine constraint (C0, i.e., limit the cosine similarity loss to be close to zero). The DCaEN obtains a 3.98% relative superior performance over C0 suggesting that orthogonal constraint may lead to learning a converged embedding space that is irrelevant to emotion, i.e., only different from the Feature Network embedding but not complementary. To verify the effective of complementary loss constraint, we use R_C which is derived based on learning representation from waveform with constraint, but the recognition layer does not use the waveform-feature augmentation. In this model, we observe an increase of 0.81% compared to using Raw only. Furthermore, we explore mean square error as loss function denoted as MSE which declines 2.08% compared to DCaEN. Finally, we compare our proposed DCaEN with recent studies within the same experimental setup, which achieves a slightly worse recognition rates (58%) on 4 class emotion recognition task (Lakomkin et al., 2017). The better performance of DCaEN corroborates the capability and the potential of the model.
3.2.1.2. EmoBase2010
We conduct similar experiments using Emobase2010, which is a much higher dimensional feature set capturing exhaustive granular and statistical functions of the acoustic stream, as an input to the Feature Network to train our DCaEN. The results are shown in the lower part of Table 2, all of the baseline models are the same as the ones when using eGeMAPS feature set. Several observations can be made, the approach of using hand-crafted feature directly, i.e., CF, damages the recognition rates severely (7.90% relative drop), which is likely due to the even higher dimensional features of Emobase2010 that contains emotionally irrelevant information. We also see a similar result occurs for the uF model, which achieves an UAR of 53.71% only; the higher dimensional representation of Emobase2010 when combining with an adaptable (non-frozen) Feature Network representation would result in an excessive number of parameter learning causing an degradation in the overall network's emotion recognition ability. Similar trend is also observed when examining accuracy obtained using C0, i.e., orthogonal constraint, and R_C, i.e., using complementary constraint-enhanced raw waveform embedding, as described in section 3.2.1.1. MSE brings 1.94% relative improvement over Ftr though there is a minor gap (0.37%) compared to DCaEN.
When comparing the effectiveness between the two hand-crafted Feature Network, eGeMAPS obtains 57.36% whereas Emobase2010 obtains 57.66%, for the IEMOCAP database. The slightly higher accuracy of Emobase2010 implies that a higher dimension of acoustic feature is in favor of the recognition performance. However, for IEMOCAP database, under the complementary learning scheme, this brute-force design of hand-crafted feature with thousands of dimensions limits the complementary learning capacity, e.g., DCaEN performs better with the 88 dimensional eGeMAPS feature than the 1,536 dimensional Emobase2010 feature. Moreover, the lower recognition performance of R_C with Emobase2010 (49.49%) than eGeMAPS (53.63%) is another clue that the additional useful complementary information extracted from high dimensional feature space is less than those extracted from a compact and robust minimal feature set; another plausible reason is that when eGeMAPS are first proposed in the literature, IEMOCAP is one of its evaluation dataset.
3.2.2. Recognition on the MSP-IMPROV
We also explore DCaEN on the MSP-IMPROV database by using these two different feature sets. The front-end network architectures comparison results are shown in the right half part of Table 1. Our proposed architecture attains 32.22% which is relatively superior than P, VGG, Inception, and auDeep architectures with 19.20, 9.41, 11.68, and 0.19%, respectively.
3.2.2.1. eGeMAPS
The results in the upper part of Table 3 shows that our proposed DCaEN achieves 44.42% UAR, which is 37.86 and 6.60% relatively higher than the Raw and the Ftr when applying the eGeMAPS feature set in Feature Network. The confusion matrix of DCaEN result is shown in Figure 4C. The better predicted emotion classes in Raw and Ftr are distinct, e.g., happiness and angry classes performs better in Ftr while Raw obtains recognition performance in sadness and neutral classes. All of the baseline model comparisons are the same as in the IEMOCAP database. The naive concatenation model, i.e., CF, without complementary loss learning obtains 42.31%, which still has a 4.99% performance gap beneath DCeAN. CF causes a drop in accuracy suggesting a non-linear dense layer used in Feature Network is essential in condensing emotionally-relevant acoustic information from the hand-crafted features. In the MSP-IMPROV experiment with eGeMAPS, using an augmented representation is crucial to obtain an improvement in the recognition, e.g., by examining R_C, it attains minor improvement over Raw but only with 33.65% UAR. On the other hand, MSE can improve the UAR to 42.79% and C0 obtains 43.48% which are both lower than DCaEN. This is another clue to show essentialness of learning with our specific complementary constraint.
3.2.2.2. EmoBase2010
The experimental results using EmoBase2010 feature set are demonstrated at the lower part of Table 3, which also includes the comparison among different baselines. The DCaEN model with 46.73% UAR outperforms not only Raw with 32.22% UAR but also Ftr with 45.21% UAR. The confusion matrix of DCaEN result is shown in Figure 4D. The Ftr using EmoBase2010 feature set obtains higher performance in happiness and angry classes, which is identical to the trend with the eGeMAPS feature set. The naive concatenation of both Feature Network embedding and raw waveform complementary embedding (nC) has slightly better performance (46.71%) than using either one type of embeddings separately. Both uF and C0 results are in lower UAR than Ftr. Noted that uF and C0 using eGeMAPS feature obtain better results than Ftr, this may due to the increasing complexity in optimizing higher dimensional input feature when the Feature Network is not frozen or the cosine constraint is specified to be zero. When observing the model of R_C, by discarding Feature Network and using only raw waveform learned complementary embedding for recognition, the minority class suffers from imbalance class distribution issue, i.e., 2.15% in sadness and 14.14% in angry. Meanwhile, DCaEN is robust against issue of minority classes recognition.
4. Discussions
4.1. Analysis on Levels of Complementary Constraint
In this session, we attempt to understand the effect on the levels of complementary loss constraint of our proposed DCaEN model has on the final accuracy obtained. To evaluate this effect experimentally, we train the DCaEN with a modified threshold-ed negative cosine similarity loss function,
which can limit the value of cosine complementary constraint to the specified threshold when training the DCaEN.
4.1.1. Analysis on the IEMOCAP
Table 4 reports the results obtained by enforcing different targeted threshold values in the DCaEN framework. The trend that we observe is that the performance improves as the threshold tending toward more negative values. The phenomena has an underlying interpretation to do with the intensity of constraint during training, i.e., orthogonal cosine constraint has limited strength to confine complementary learning in an emotionally relevant space (the network could potentially just converge to a random space that is orthogonal but without any affect-related information); we observe indeed that the recognition capacity gradually enhances as cosine similarity constraint tend toward more negative.
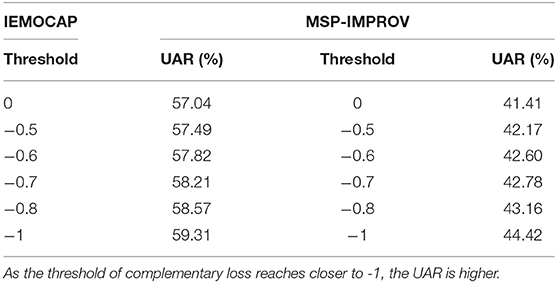
Table 4. The results of different threshold on cosine similarity in both IEMOCAP and MSP-IMPROV databases.
Besides presenting recognition rates, we further provide the visualization results to illuminate the learned latent embeddings of both Feature Network and Complementary Raw Waveform Network in our proposed DCaEN. We use t-distributed Stochastic Neighbor Embedding (t-SNE) for visualization. From Figure 5, we can observe that the raw waveform embedding and hand-crafted feature embedding form a more separated and opposite pattern as the threshold becomes closer to −1. Additionally, the more negative correlation imposed on the two representations the more hand-crafted feature embedding distributed similarly to the raw waveform embedding. These two space seem to become reverse mirroring to each other, and it becomes intuitive as we augment these two representations to improve the overall recognition rates.
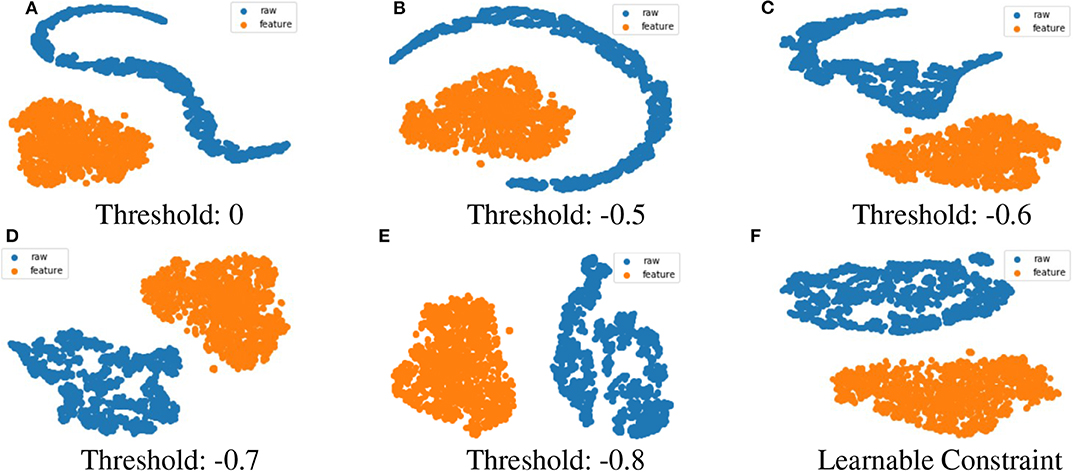
Figure 5. The visualization of raw waveform complementary embedding and Feature Network embedding using eGeMAPS feature set in DCaEN are projected on two dimensional feature space. The visualization figure from (A–F) shows the increasing specified constraint values and the distribution patterns of two embeddings become similar while in a reverse direction.
4.1.2. Analysis on the MSP-IMPROV
As shown in Table 4, a similar trend is also observed for the MSP-IMPROV, i.e., the UAR performance improves as the threshold becomes more negative. We further use t-SNE to further reduce the dimension of both Feature Network embedding and raw waveform complementary embedding to provide a 2D visualization. From Figure 6, when the threshold is closer to 0, i.e., learning to become orthogonal between two embedding, the resulting distribution of the two embedding becomes very dissimilar, e.g., as shown in Figures 6A,B, the embedding of hand-crafted feature (indicated in blue) has a clustered shape, but the embedding of raw waveform shows an extremely irregular form. When the thresholds becomes closer to -1, the raw waveform embedding and the hand-crafted feature embedding form an opposite mirroring pattern. Specifically, if we compare between threshold set at 0 and closer to −1, as the threshold becomes more negative, the representation learned from raw waveform seems to converge to a similar shape as the hand-crafted feature embedding just at a 180 degree mirroring reverse.
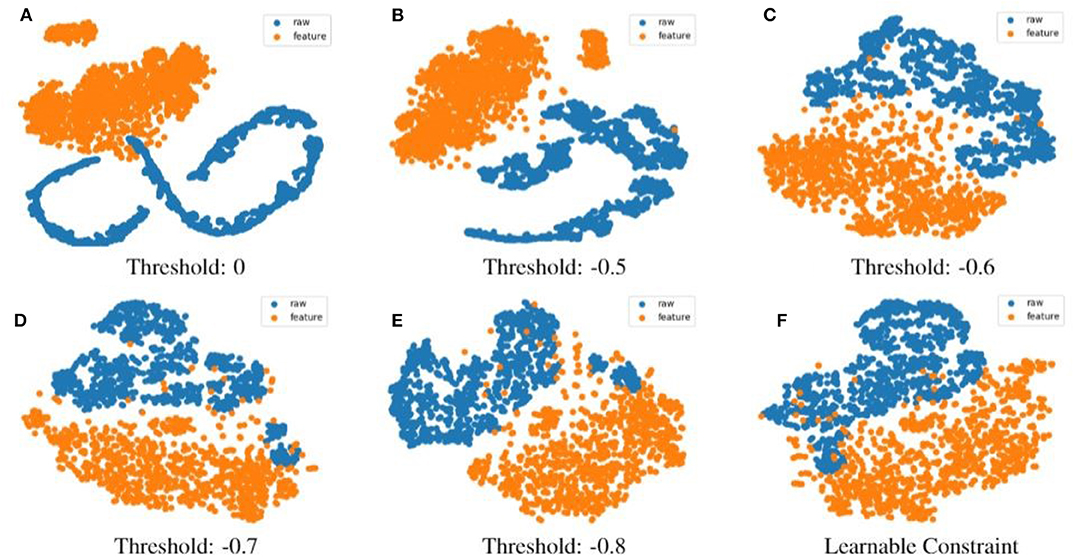
Figure 6. The visualization results of Feature Network embedding using eGeMAPS feature set and complementary embedding on MSP-IMPROV database. The visualization figure from (A–F) shows change of distributions as the specified constraint value increases.
Furthermore, we can explore the differences between two corpora evaluated in this work. Comparing the Figure 5 with the Figure 6, we can see that the distance between the learned raw waveform and the feature embedding in the Figure 5 is larger than the Figure 6. This result confirms our experimental result indicating that the recognition UARs of our DCaEN is lower for the MSP-IMPROV than for the IEMOCAP; the MSP-IMPROV database is a more recent corpus including more diverse and complex emotion elicitation than the well-known IEMOCAP. This is also demonstrated in the more overlapping representations in the MSP-IMPROV as compared to the IEMOCAP.
4.2. Analysis on the Complementary Representation
To examine the characteristics of the learned latent complementary embedding from raw-waveform, we report the averaged correlation between the principal component and different types of acoustic feature sets. Specifically, we utilize the first principal component from principal component analysis (PCA) for further examine of the most relevant feature type extracted from raw-waveform embedding. We compute Pearson correlation between this principal vector and each dimension of eGeMAPS and EmoBase2010 feature sets. Then, we report the averaged absolute correlation over the all functional features within each major acoustic feature categories, i.e., energy, frequency, spectral related parameter sets, and others; “others” contains features not belonging the conventional three categories like mean length of unvoiced region, number of continuous voiced region, or equivalent sound level.
As results demonstrated in Table 5, we see that a clear indication that the first component of the complementary embedding correlate with energy and spectral related features the most, while frequency related features tend to be less correlated. This correlation may also contribute to the fact the raw waveform embedding is better at capturing sad and angry (arousal dimension) where the feature network is better at recognizing happy (valence dimension). The results are slightly different between eGeMAPS and EmoBase2010 feature sets, e.g., correlation effect to different types of Emobase2010 acoustic feature types in the IEMOCAP is more uniform. The IEMOCAP and the MSP-IMPROV databases also exhibit minor differences likely due to idiosyncratic characteristics of each of these databases. The overall correlational effect is not very strong, which suggests that the learned complementary latent space do encompass partial known acoustic properties of hand-crafted features and further captures additional information beyond these feature sets from the raw-waveform.
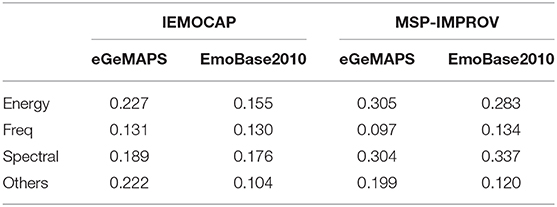
Table 5. The averaged correlation between the first principal component vector and different types of acoustic feature categories.
5. Conclusion
In this work, we present an evaluation on the recently proposed Dual Complementary Acoustic Embedding Network (DCaEN). It contains two sub-structures: a Feature Network that uses expert knowledge-driven acoustic parameters and a Raw Waveform Complementary Network that uses raw waveform with complementary learning constraint. DCaEN utilizes a negative cosine complementary constraint in order to leverage the hand-crafted features to enhance and extract discriminative characteristics from raw waveform, and by concatenating these two representations, we demonstrate that the robustness of our framework across database. Specifically, it can improve 4-class emotion recognition rates to 59.13% on the IEMOCAP dataset with eGeMAPS and 46.22% on the MSP-IMPROV dataset with Emobase2010.
In the work, the core idea of DCaEN can be extended to other state and trait recognition tasks from speech or other behavior modalities, which at the moment still relies heavily on prior expert knowledge in the feature design. Specifically, aside from constraining on eGeMAPS and EmoBase2010, we can explore other domain expert knowledge as auxiliary information in better achieving high-performing end-to-end SER. Technically, deep metric learning can be included to learn an even more constrained feature space in the complementary learning process; further investigation on exactly what particular aspect of speech acoustics is being extracted additional from the raw waveform will be an interesting scientific direction to pursue next. In this way, the model can facilitate human knowledge and machine capability to be integrated together for precise understanding of acoustic manifestation of affect state and robust emotion sensing technology.
Data Availability Statement
Publicly available datasets were analyzed in this study. This data can be found here: https://sail.usc.edu/iemocap/, https://ecs.utdallas.edu/research/researchlabs/msp-lab/MSP-Improv.html.
Author Contributions
J-LL is responsible for conceptualization, algorithmic development, data curation, formal analysis, investigation, validation, visualization, and writing—original draft. T-YH is responsible for algorithmic validation, data curation. C-MC oversees the development as well as investigation. C-CL supervises the entire work and contributes to idea conceptualization, manuscript revision, and approval the submission.
Funding
This work was supported by Ministry of Science and Technology, Taiwan (Grant 108-2634-F-007-005) for research funding, publication fee, and other relevant research expenses.
Conflict of Interest
The authors declare that the research was conducted in the absence of any commercial or financial relationships that could be construed as a potential conflict of interest.
References
Aldeneh, Z., and Provost, E. M. (2017). “Using regional saliency for speech emotion recognition,” in ICASSP (New Orleans, LA), 2741–2745. doi: 10.1109/ICASSP.2017.7952655
Badshah, A. M., Ahmad, J., Rahim, N., and Baik, S. W. (2017). “Speech emotion recognition from spectrograms with deep convolutional neural network,” in 2017 International Conference on Platform Technology and Service (PlatCon) (Busan: IEEE), 1–5. doi: 10.1109/PlatCon.2017.7883728
Badshah, A. M., Rahim, N., Ullah, N., Ahmad, J., Muhammad, K., Lee, M. Y., et al. (2019). Deep features-based speech emotion recognition for smart affective services. Multimedia Tools Appl. 78, 5571–5589. doi: 10.1007/s11042-017-5292-7
Busso, C., Bulut, M., Lee, C.-C., et al. (2008). IEMOCAP: Interactive emotional dyadic motion capture database. Lang. Resour. Eval. 42:335. doi: 10.1007/s10579-008-9076-6
Busso, C., Parthasarathy, S., Burmania, A., AbdelWahab, M., Sadoughi, N., and Provost, E. M. (2016). MSP-IMPROV: an acted corpus of dyadic interactions to study emotion perception. IEEE Trans. Affect. Comput. 8, 67–80. doi: 10.1109/TAFFC.2016.2515617
Callejas, Z., Griol, D., and López-Cózar, R. (2011). Predicting user mental states in spoken dialogue systems. EURASIP J. Adv. Signal Process. 2011:6. doi: 10.1186/1687-6180-2011-6
Chavhan, Y., Dhore, M., and Yesaware, P. (2010). Speech emotion recognition using support vector machine. Int. J. Comput. Appl. 1, 6–9. doi: 10.5120/431-636
Chen, S., Jin, Q., Li, X., Yang, G., and Xu, J. (2014). “Speech emotion classification using acoustic features,” in The 9th International Symposium on Chinese Spoken Language Processing (Singapore: IEEE), 579–583. doi: 10.1109/ISCSLP.2014.6936664
El Ayadi, M., Kamel, M. S., and Karray, F. (2011). Survey on speech emotion recognition: features, classification schemes, and databases. Pattern Recogn. 44, 572–587. doi: 10.1016/j.patcog.2010.09.020
Eyben, F., Scherer, K. R., Schuller, B. W., et al. (2016). The Geneva minimalistic acoustic parameter set (gemaps) for voice research and affective computing. IEEE Trans. Affect. Comput. 7, 190–202. doi: 10.1109/TAFFC.2015.2457417
Eyben, F., Weninger, F., Gross, F., and Schuller, B. (2013). “Recent developments in opensmile, the Munich open-source multimedia feature extractor,” in Proceedings of the 21st ACM International Conference on Multimedia (Barcelona: ACM), 835–838. doi: 10.1145/2502081.2502224
Fayek, H. M., Lech, M., and Cavedon, L. (2017). Evaluating deep learning architectures for speech emotion recognition. Neural Netw. 92, 60–68. doi: 10.1016/j.neunet.2017.02.013
Freitag, M., Amiriparian, S., Pugachevskiy, S., Cummins, N., and Schuller, B. (2017). auDeep: unsupervised learning of representations from audio with deep recurrent neural networks. J. Mach. Learn. Res. 18, 6340–6344. doi: 10.5555/3122009.3242030
Guo, L., Wang, L., Dang, J., Liu, Z., and Guan, H. (2019). Exploration of complementary features for speech emotion recognition based on kernel extreme learning machine. IEEE Access 7, 75798–75809. doi: 10.1109/ACCESS.2019.2921390
Guo, L., Wang, L., Dang, J., Zhang, L., Guan, H., and Li, X. (2018). “Speech emotion recognition by combining amplitude and phase information using convolutional neural network,” in Proc. Interspeech 2018 (Hyderabad), 1611–1615. doi: 10.21437/Interspeech.2018-2156
Han, J., Zhang, Z., Keren, G., and Schuller, B. (2018). “Emotion recognition in speech with latent discriminative representations learning,” in Acta Acustica United with Acustica, Vol. 104 (S. Hirzel Verlag), 737–740. doi: 10.3813/AAA.919214
Han, K., Yu, D., and Tashev, I. (2014). “Speech emotion recognition using deep neural network and extreme learning machine,” in Fifteenth Annual Conference of the International Speech Communication Association (Singapore).
Hu, H., Xu, M.-X., and Wu, W. (2007). “GMM supervector based SVM with spectral features for speech emotion recognition,” in 2007 IEEE International Conference on Acoustics, Speech and Signal Processing-ICASSP'07, Vol. 4 (Honolulu, HI: IEEE), 4–413. doi: 10.1109/ICASSP.2007.366937
Huang, J., Li, Y., Tao, J., Lian, Z., Niu, M., and Yi, J. (2018). “Speech emotion recognition using semi-supervised learning with ladder networks,” in 2018 First Asian Conference on Affective Computing and Intelligent Interaction (ACII Asia) (Beijing: IEEE), 1–5. doi: 10.1109/ACIIAsia.2018.8470363
Huang, T.-Y., Li, J.-L., Chang, C.-M., and Lee, C.-C. (2019). “A dual-complementary acoustic embedding network learned from raw waveform for speech emotion recognition,” in Proceedings of Affective Computing Intelligent Interaction (ACII) (Cambridge: Association for the Advancement of Affective Computing). doi: 10.1109/ACII.2019.8925496
Huang, Z., Dong, M., Mao, Q., and Zhan, Y. (2014). “Speech emotion recognition using CNN,” in Proceedings of the 22nd ACM International Conference on Multimedia (Orlando, FL: ACM), 801–804. doi: 10.1145/2647868.2654984
Jiang, W., Wang, Z., Jin, J. S., Han, X., and Li, C. (2019). Speech emotion recognition with heterogeneous feature unification of deep neural network. Sensors 19:2730. doi: 10.3390/s19122730
Lakomkin, E., Weber, C., Magg, S., and Wermter, S. (2017). “Reusing neural speech representations for auditory emotion recognition,” in Proceedings of the Eighth International Joint Conference on Natural Language Processing, Vol. 1 (Taipei).
Latif, S., Rana, R., Khalifa, S., Jurdak, R., and Epps, J. (2019). Direct modelling of speech emotion from raw speech. arXiv preprint arXiv:1904.03833. doi: 10.21437/Interspeech.2019-3252
Lee, J., and Tashev, I. (2015). “High-level feature representation using recurrent neural network for speech emotion recognition,” in Sixteenth Annual Conference of the International Speech Communication Association (Dresden).
Li, L., Zhao, Y., Jiang, D., Zhang, Y., Wang, F., Gonzalez, I., et al. (2013). “Hybrid deep neural network-hidden Markov model (DNN-HMM) based speech emotion recognition,” in 2013 Humaine Association Conference on Affective Computing and Intelligent Interaction (Geneva: IEEE), 312–317. doi: 10.1109/ACII.2013.58
Lim, W., Jang, D., and Lee, T. (2016). “Speech emotion recognition using convolutional and recurrent neural networks,” in 2016 Asia-Pacific Signal and Information Processing Association Annual Summit and Conference (APSIPA) (Jeju: IEEE), 1–4. doi: 10.1109/APSIPA.2016.7820699
Lin, Y.-L., and Wei, G. (2005). “Speech emotion recognition based on HMM and SVM,” in 2005 International Conference on Machine Learning and Cybernetics, Vol. 8 (Guangzhou: IEEE), 4898–4901.
Mano, L. Y., Faical, B. S., Nakamura, L. H., Gomes, P. H., Libralon, G. L., Meneguete, R. I., et al. (2016). Exploiting IOT technologies for enhancing health smart homes through patient identification and emotion recognition. Comput. Commun. 89, 178–190. doi: 10.1016/j.comcom.2016.03.010
Mao, X., Chen, L., and Fu, L. (2009). “Multi-level speech emotion recognition based on HMM and ANN,” in 2009 WRI World Congress on Computer Science and Information Engineering (Los Angeles, CA: IEEE), Vol. 7, 225–229. doi: 10.1109/CSIE.2009.113
Mirsamadi, S., Barsoum, E., and Zhang, C. (2017). “Automatic speech emotion recognition using recurrent neural networks with local attention,” in 2017 IEEE International Conference on Acoustics, Speech and Signal Processing (ICASSP) (New Orleans, LA: IEEE), 2227–2231. doi: 10.1109/ICASSP.2017.7952552
Neiberg, D., Elenius, K., and Laskowski, K. (2006). “Emotion recognition in spontaneous speech using GMMs,” in Ninth International Conference on Spoken Language Processing (Pittsburgh, PA).
Neumann, M., and Vu, N. T. (2017). Attentive convolutional neural network based speech emotion recognition: a study on the impact of input features, signal length, and acted speech. arXiv preprint. arXiv:1706.00612. doi: 10.21437/Interspeech.2017-917
Nwe, T. L., Foo, S. W., and De Silva, L. C. (2003). Speech emotion recognition using hidden Markov models. Speech Commun. 41, 603–623. doi: 10.1016/S0167-6393(03)00099-2
Pentland, A. (2004). Healthwear: medical technology becomes wearable. Computer. 37, 42–49 doi: 10.1109/MC.2004.1297238
Ringeval, F., Schuller, B., Valstar, M., Jaiswal, S., Marchi, E., Lalanne, D., et al. (2015). “AV+ EC 2015: The first affect recognition challenge bridging across audio, video, and physiological data,” in Proceedings of the 5th International Workshop on Audio/Visual Emotion Challenge (Brisbane, QLD: ACM), 3–8. doi: 10.1145/2808196.2811642
Sahu, S., Gupta, R. R., and Espy-Wilson, C. Y. (2018). “On enhancing speech emotion recognition using generative adversarial networks,” in Interspeech (Hyderabad). doi: 10.21437/Interspeech.2018-1883
Sarma, M., Ghahremani, P., Povey, D., Goel, N. K., Sarma, K. K., and Dehak, N. (2018). “Emotion identification from raw speech signals using DNNs,” in Interspeech 2018 - 19th Annual Conference of the International Speech Communication Association (Hyderabad). doi: 10.21437/Interspeech.2018-1353
Schuller, B., Steidl, S., Batliner, A., Burkhardt, F., Devillers, L., Müller, C., et al. (2010). “The interspeech 2010 paralinguistic challenge,” in Eleventh Annual Conference of the International Speech Communication Association (Makuhari, Chiba).
Schuller, B., Steidl, S., Batliner, A., Vinciarelli, A., Scherer, K., Ringeval, F., et al. (2013). “The interspeech 2013 computational paralinguistics challenge: social signals, conflict, emotion, autism,” in Proceedings INTERSPEECH 2013, 14th Annual Conference of the International Speech Communication Association (Lyon).
Shahin, I., Nassif, A. B., and Hamsa, S. (2019). Emotion recognition using hybrid Gaussian mixture model and deep neural network. IEEE Access 7, 26777–26787. doi: 10.1109/ACCESS.2019.2901352
Tkalcic, M., Kosir, A., and Tasic, J. (2011). Affective Recommender Systems: The Role of Emotions in Recommender Systems. Citeseer.
Tokuno, S., Tsumatori, G., Shono, S., Takei, E., Yamamoto, T., Suzuki, G., et al. (2011). “Usage of emotion recognition in military health care,” in 2011 Defense Science Research Conference and Expo (DSR) (Singapore: IEEE), 1–5. doi: 10.1109/DSR.2011.6026823
Toledo-Ronen, O., and Sorin, A. (2013). “Voice-based sadness and anger recognition with cross-corpora evaluation,” in 2013 IEEE International Conference on Acoustics, Speech and Signal Processing (Vancouver, BC: IEEE), 7517–7521. doi: 10.1109/ICASSP.2013.6639124
Tripathi, S., Kumar, A., Ramesh, A., Singh, C., and Yenigalla, P. (2019). Focal loss based residual convolutional neural network for speech emotion recognition. arXiv preprint arXiv:1906.05682.
Tzirakis, P., Zhang, J., and Schuller, B. W. (2018). “End-to-end speech emotion recognition using deep neural networks,” in 2018 IEEE International Conference on Acoustics, Speech and Signal Processing (ICASSP) (Calgary: IEEE), 5089–5093. doi: 10.1109/ICASSP.2018.8462677
Yang, Z., and Hirschberg, J. (2018). “Predicting arousal and valence from waveforms and spectrograms using deep neural networks,” in Interspeech (Hyderabad). doi: 10.21437/Interspeech.2018-2397
Yenigalla, P., Kumar, A., Tripathi, S., Singh, C., Kar, S., and Vepa, J. (2018). “Speech emotion recognition using spectrogram & phoneme embedding,” in Proc. Interspeech 2018 (Hyderabad), 3688–3692. doi: 10.21437/Interspeech.2018-1811
Keywords: speech emotion recognition, raw waveform, end-to-end, complementary learning, acoustic representation
Citation: Li J-L, Huang T-Y, Chang C-M and Lee C-C (2020) A Waveform-Feature Dual Branch Acoustic Embedding Network for Emotion Recognition. Front. Comput. Sci. 2:13. doi: 10.3389/fcomp.2020.00013
Received: 11 August 2019; Accepted: 16 April 2020;
Published: 15 May 2020.
Edited by:
Nicholas Cummins, University of Augsburg, GermanyReviewed by:
Saturnino Luz, University of Edinburgh, United KingdomTheodora Chaspari, Texas A&M University, United States
Copyright © 2020 Li, Huang, Chang and Lee. This is an open-access article distributed under the terms of the Creative Commons Attribution License (CC BY). The use, distribution or reproduction in other forums is permitted, provided the original author(s) and the copyright owner(s) are credited and that the original publication in this journal is cited, in accordance with accepted academic practice. No use, distribution or reproduction is permitted which does not comply with these terms.
*Correspondence: Chi-Chun Lee, Y2NsZWVAZWUubnRodS5lZHUudHc=