Applications of Biological and Physiological Signals in Commercial Video Gaming and Game Research: A Review
- Multimodal Interaction Group, Music Technology Group, ETIC, Universitat Pompeu Fabra, Barcelona, Spain
Video gaming is now available as a fully immersive experience that creates responsive inputs and outputs concerning the user, and some experimental developers have integrated the use of the voice, brain, or muscles as input controls. The use of physiological signal equipment can provide valuable information regarding the emotion of a player or patient during gameplay. In this article, we discuss five of the most common biosignals that are used in gaming research, and their function and devices that may be used for measurement. We break down those individual signals and present examples of research studies that implement them. We also discuss the usage of biological signals within commercial gaming and conclude with some possible future directions for the use of biological signals in gaming and game research.
Introduction
Game companies and researchers have been exploring the benefits of using physiological sensing devices within their video games and their research. They have experimented with brain-controlled gaming for stress control and used biosignals to retrieve emotional and interaction information from users during gameplay. Researchers have also integrated biosensors into games, to aid in physical rehabilitation as well as in the treatment of some attention and neurological disorders. In many of these studies, games have been implemented along with biosensors, to study the biological data of the participants during play as well as to make these games adaptive using the data from their biosignals.
A biosignal is any signal from living beings that can be continually measured and monitored. Although the term is often used to refer to bioelectrical signals, it may indeed refer to both electrical and nonelectrical signals. Biosignals can come from different parts of the body, such as the skin, brain, heart, muscles, or eyes. Among the bioelectrical signals commonly used in research, as well as being integrated into some commercial devices, are electroencephalography (EEG), electrocardiography (ECG), electromyography (EMG), electrooculography (EOG), and electrodermal activity (EDA).
Within a game, these biosignals can be used for different applications. They may be used as inputs to control certain aspects or objects within a game. Biosignals that can act as controllers or data input typically include (but are not limited to): eye movements and gaze, muscle activity, skin conductance level, heart rate variation, and brain activity. In this article, we will present examples of the use of biosignals and how they have been used within gaming research to aid persons with disabilities. These are also used often for therapeutic and rehabilitation purposes, namely EMG, which has been used to train persons who are learning to use prostheses.
The rest of this article is structured as follows. In Overview of Biosignals we introduce each of the most commonly used biosignals and physiological signals within medicinal and gaming research, and in Applications of Biosignals in Gaming, we describe their applications within commercial and serious games. In the final section, we discuss the possibilities for future implementations.
Overview of Biosignals
In this section, we introduce the five electrical biosignals that will be discussed in this article, namely electromyography (EMG), electroencephalography (EEG), electrodermal activity (EDA), eye tracking, and electrooculography (EOG), and electrocardiography (ECG). Widely used in research, they can all be accessed today in noninvasive means and through consumer devices, which makes them easily retrievable, also for non-researchers. New scientific and consumer hardware, along with their functionality and purpose, will also be introduced.
Electromyography
Electromyography (EMG) is a technique used to measure and record the electrical activity generated by the muscles (Athavale and Krishnan, 2017). EMG has been widely used for rehabilitation and for control of prosthetic limbs, and also as input control for gaming, primarily by developers and researchers. A popular commercial EMG device, the MYO armband (Figure 1A), has been used for research purposes and has also been a hacker’s favorite in finding novel uses for EMG in nonmedical fields.
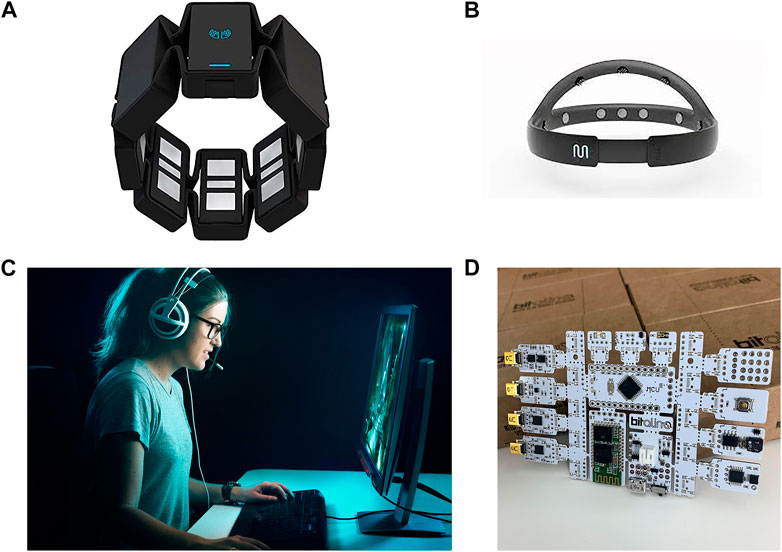
FIGURE 1. (A) Thalmic Labs MYO armband. Thalmic Labs 2013–2018. (B) Imec EEG unit. The unit uses dry rubber electrodes for conductance. The unit can easily be integrated to be worn with a head-mounted device for monitoring brain signals while experiencing Virtual Reality. (C) “Gamer using a Tobii Eye Tracker mounted on a monitor” Tobii AB. (D) “Bitalino Board Kit. Input for multiple biosignals including EDA and ECG.” PLUX Wireless Biosignals S.A.
Electroencephalography
Electroencephalography (EEG) is the measuring of electrical signals in the brain, through the use of electrodes that are placed on certain points on the skull, to assess the activity of specific regions of the brain. Brain–computer interfaces (BCIs) are EEG devices that communicate between the neural pathways and a computer. These have been developed to decode brain signals and translate them into a computer program (Abdulkader et al., 2015). Researchers are able to perform tests using EEG, to understand how the brain responds to certain stimuli, such as music, or how the brain behaves in a person experiencing sleep disturbances or epilepsy. These devices have made the process of retrieving the brain’s electrical signals into a less complex process so that even non-researchers can now use them to interact with their brainwaves.
There are several consumer-level EEG headsets on the market such as the Muse headband,1 the Emotiv Insight,2 the EPOC,3 the Imec EEG4 headset (Figure 1B), or the Galea by Open BCI5 (to be released in 2022). These headsets tend to come with proprietary software, which allows the user to calibrate the device easily, provides artifact filtering, and data readouts for separate brainwaves, brain regions, and emotions. In counterpart, due to this proprietary nature, headsets such as Emotiv and Muse cannot be used with external software, without much revision and hacking into the company software. Devices such as the IMEC headset can be mounted within a VR headset, and use flexible, polymer dry electrodes. Although researchers prefer to use headsets that use wet electrodes (electrodes that are placed with conductive paste for better signal transmission), an analysis of the electrodes used in the IMEC headset showed no viable differences in signal when tested against conventional wet electrodes (Chen et al., 2014).
BCIs use two main methods for measuring responses in brain activity. Using the method of steady-state-evoked visual potentials (SSEVP), a visual stimulus occurs at a different constant frequency (Allison, et al., 2008). A stimulus that is presented at a frequency of 6–8 Hz is more likely to elicit an SSEVP (Müller and Hillyard, 2000). Through the use of a BCI that uses SSEVP, the EEG activity is measured at a certain frequency to measure the intent of the user. Examples of steady-state methods can be seen in the studies mentioned in this article.
An event-related response is when small electrical changes result from a stimulus change (Blackwood and Muir, 1990). The stimulus could be cognitive, sensory, or motor (Abdulkader et al., 2015). Examples of event-related response can be seen in examples in which the researchers monitor biological changes from specific changes in the stimuli, such as a change in the tension or music.
Eye Tracking and Electrooculography
Electrooculography (EOG) and eye tracking are two methods for measuring the point of gaze or the motion of an eye relative to the head. EOG tracks ocular activity through the use of sensors that are placed around the eyes, in a similar manner as EMG electrodes. These sensors record the corneo-retinal potential difference between the positive and negative sides of the eyeball (Bulling et al., 2009). Alternatively, eye tracking uses a camera and image analysis for measuring the point of gaze or the motion of the eye. Eye tracking has become integrated into consumer devices such as the VIVE Pro Eye headset6 and Alienware laptops, and companies such as Vive and Tobii7 (Figure 1C) market eye tracking to companies as a tool for gathering analytical information to use for their businesses. Eye tracking can record where a potential customer may look or keep her attention during an advertisement or shopping trip, or how immersed a person may be during a task such as playing a game. Researchers, on their side, have implemented eye tracking to study many problems, including people with gambling (Murch et al., 2019) and gaming disorders.
Electrodermal Activity
Electrodermal activity (EDA) is a measure of the electrical activity or resistance level of the skin. It is a physiological response that is activated by the sympathetic system (Dawson et al., 2017). The skin’s electrical resistance typically changes during moments of arousal or physical activity, such as exercise, nervousness, or fear response. While we notice when our heart is beating quickly, or we may even perceive at moments of panic or nervousness an elevated amount of sweat in the palms, small changes in our sweat or skin conductance are not noticeable to us. Minute changes in skin conductance require the use of EDA sensors. One advantage of EDA is that it is less sensitive to noise in comparison with EMG and EEG, which receive a lot of noise artifacts from muscle and body movements (Kivikangas et al., 2011).
Electrocardiography
ECG is the measure of the electrical activity generated by the heart. Similar to EMG or EOG, the heart rate is measured using three electrodes that are stuck onto the skin of the chest over the heart. Heart rate changes during exercise and physical activity, during times of emotional duress, and bodily fatigue (Chęć et al., 2015). ECG can be used to measure stress and ECG changes in a player during gameplay (Porter et al., 2019). When measuring emotions and behavioral responses, ECG is often coupled with another sensor, usually EDA or EEG.
Applications of Biosignals in Gaming
In this section, we discuss the applications of each of the aforementioned biosignals in gaming. While much of this section addresses the uses of biosignals in game-based research, commercial applications will also be considered. We introduce some commercially available devices, as well as the challenges and possibilities of these technologies reaching a wider audience, through the release of developer-friendly software and hardware. In Table 1, we provide a reference table of the games that have been reviewed in researching this article. This table includes serious games, commercial games, and games that were modified for use within a study. It lists for each game, the type of biosignal monitored and its usage, and summarizes most of the information contained in this section. Additionally, in Table 2, we present a taxonomy of the biosignals discussed in this article, broken down into sections by their usages and the devices available.
Applications of EMG in Gaming
In some studies, researchers have used devices with biosensors such as EEG or EMG connected to a game, to aid persons with learning how to gain use of muscles or to test anticipation of movement for those with muscle impairment (Armiger and Vogelstein, 2008; Norman et al., 2016; Rincon et al., 2016; Prahm et al., 2017). In other uses, a researcher can, for instance, monitor in real time how players react to a game by measuring their attention levels or immersion level (Nacke and Lindley, 2008), stress (Karthikeyan et al., 2011), or panic levels (Russoniello et al., 2009; Wang et al., 2014).
Researchers have used EMG for designing experiences to train individuals with physical disabilities, to aid in their recovery (Armiger and Vogelstein, 2008; Converse et al., 2013; Hayes et al., 2013). One study explored the feasibility of using an EMG device built with commercial off-the-shelf sensors and placed into an arm sleeve, for physical rehabilitation (Visconti et al., 2018; Converse et al., 2013; Hayes et al., 2013) (Table 1, row 11). The surface sensors were placed over the forearm just below the elbow. Two simple games were created for the experiment: a tower defense game8 and a Pong-style game. EMG is also being used to build and test assistive technology for those in need of prostheses. One group of researchers modified a Guitar Hero controller with EMG sensors to allow amputees to play the game (Armiger and Vogelstein, 2008) (Table 1, row 4). Another group looked to broaden the use of a prosthetic limb to have more input options to a game (Vujaklija et al., 2017) (Table 1, rows 57). For this, they used a prosthetic forearm and hand called the Ottobock Myoboy.9 The aim of the study was to use gaming as a method of teaching users how to properly control a new prosthesis and stay motivated. The outcome showed that this method of training prosthesis users was efficient in training separate muscle movements and endurance. Studies such as these demonstrate methods of keeping those in rehabilitation interested and motivated while improving their physical state.
One related example of commercial use of an EMG device was produced in 2019 by the French company Perifit,10 which released a device that aids new mothers in strengthening their pelvic muscles in postnatal recovery, as well as women who suffer from bladder control issues. To motivate these users to do the exercises and to do them correctly, the company provides several games with their applications, where the user interacts with them by using the device as a controller (Table 1, row 12). These games change exercises at each level, depending on which recovery program the user has chosen.
EMG can also act as a noninvasive and reliable signal for measuring emotions. Soares et al. (2017) employed a combination of EMG and EDA signals for reading emotions during a playing experience (Table 1, rows 6, 27). They placed EMG sensors on the facial muscles for tracking facial expressions to pinpoint positive or negative valence. For sensing EMG and EDA, these researchers used a Bitalino. The Bitalino11 (Figure 1D), from the Portuguese company Plux, is a small board that fitted with the corresponding sensors, can be used for measuring multiple simultaneous biosignals, including EMG, EDA, EEG, and ECG. It has become popular among researchers and hobbyists, due to its open-source platform, its affordability, and its reliability.
EEG in Gaming Applications
Whereas the aforementioned use of EMG for measuring emotions is still scarce, the use of EEG for the same purpose is much more widespread. Numerous studies have been done in monitoring EEG signals during gameplay, to analyze the emotional mindset of a player during game interaction. One direct benefit of measuring emotions during gameplay is to use the biological data to change the difficulty or experience of the game (Carofiglio et al., 2019).
These devices show great possibilities, not just for analysis of emotional states and adaptive gaming, but can also provide opportunities for health-related research and for severely disabled persons to have a means of interacting with video games. Researchers have studied applications of using BCIs in games to search for new interventions for people with Autism and Autism Spectrum Disorder (Friedrich et al., 2014). One study used a BCI to test the possibility of removing attention impairments from children with autism (Mercado et al., 2019). In this study, the use of a BCI with a video game improved the attention level of the children with two showing no attention issues post-study. While researchers are using BCIs to aid those with an emotional disorder or other disabilities, there still remains a gap in testing the possibilities in BCI gaming with persons with severe physical disabilities.
Relaxation and meditation has become a popular topic in research as well as at a commercial level. In a seminal experiment, Hjelm and Browall (2000) created a game using a BCI to test the alleviation of stress (Table 1, row 2). This experiment involved two gamers “competing” to become relaxed by moving a metal ball with their alpha and beta signals. More recently, Ahn et al. (2014) targeted the brain’s alpha signals for an adapted version of World of Worldcraft (Table 1, row 19). In this experiment, the user could control the game through conventional input as well as affect changes in the game through their alpha brain signals. The company Neurosky,12 which produces BCIs, launched a game entitled Neuroboy (Table 1, row 17), to accompany their headset in 2007. As of 2020, the company has released numerous applications and games either for free or purchase, such as Neuro Tower Defense (Table 1, row 18), a strategy game that also tests the brain to remain calm during the task of defense. Neurosky is not the only commercially available headset that provides its own applications to go along with its BCIs. Other headsets such as Muse are in fact marketed with the main purpose of reducing the stress of the user.
Electrooculography and Eye-Tracking Applications in Gaming
Widely used in commercial and marketing studies, eye gaze is still not as commonly employed in gaming as other biosignals. It is mostly used as an implementation for hands-free gaze-based interaction, which has clear applications in Virtual Reality (VR), as well as for allowing those with severe motor disabilities to interact with video games and other applications. In the case of using VR, the game VRailSurfer was developed and participants played it by controlling the direction of the character and avoiding obstacles through gaze and eye movement (Kumar and Sharma, 2016) (Table 1, row 23). The technique called dwell-time-based selection (Isokoski et al., 2009) uses visual fixation for allowing the eyes to act as a “mouse emulator.” One group tested a Guitar Hero style game, evaluating where the eyes fixated (Vickers et al., 2010) (Table 1, row 25). The Eyeharp is a software-based musical instrument that permits people with disabilities to play music with gaze or with head movements, allowing them to play melodies simply by looking at the notes on the screen (Vamvakousis and Ramirez, 2016). On a different line, Balasubramanian and Adalarasu (2007) combined EOG with EEG for measuring the onset of fatigue while users played a driving game (Table 1, row 24).
This eye-tracking technology has already been implemented commercially by companies such as Tobii and HTC Vive. The gaming giant Ubisoft worked with the Tobii-tracking software and hardware (Figure 1C) to include this capability into the PC versions of more than 130 of their games. Eye tracking is also used in a process called foveated rendering, which tracks the eye and renders images in greater quality where the eye gazes. This allows for lower machine latency as the image can maintain optimum frame rate inside the focal area while leaving the area outside of view at lower quality when not being tracked. The VR headset HTC VIVE Pro Eye, released in 2019, has eye-tracking capabilities within the headset. Vive has also released an SDK for developers to easily integrate this into their game.
Electrodermal Activity in Gaming
Several studies have shown that as the level of challenge or speed of a game increases, the skin conductance level also augments (Parsons and Reinebold, 2012) (Table 1, row 13). EDA correlates with the levels of nervousness or fear, the integration of this biosignal into gaming brings possibilities for adaptive games, such as introducing more challenges in a level depending on the user’s state of calm Chanel et al. (2008) used EDA (along with other biosignals) to measure the level of excitement, boredom, and anxiety in participants during gameplay of Tetris. The company Valve used skin response to measure players’ sweat levels during testing phases of the game Left 4 Dead 2 (Polygon, 2020).13 This allowed them to measure stress levels and study and adjust the time that the objective should take to finish. EDA has also shown possibilities in therapeutic games, for instance in the treatment of phobias Kritikos et al. (2021) use EDA with users with arachnophobia in a Virtual Reality environment. The users were exposed to an environment with spiders and their skin response was measured and the intensity of the experience was controlled in real time. Researchers are also using physiological signals to solve issues in experiences, such as Virtual Reality sickness (Martin et al., 2018). In this study, EDA was used along with a measurement of cardiac activity to test a detection method for users who become motion sick within VR.
ECG in Gaming Applications
ECG is another biosignal often used in gaming for measuring emotional states during gameplay. Vachiratamporn et al. (2015) used EEG and ECG to monitor players’ emotional states during a horror game (Table 1, row 20). They reported that ECG was an effective means of predicting the user’s affect during pre-scary and post-scary moments. ECG and skin conductance were also used during an experiment in which players played the game Half Life 2 (Dekker and Champion, 2007) (Table 1, row 21). In this experiment, the researchers modified the game in several ways to react and change, depending on the readings, such as sound volume, shader effects (color changes), and rewards. Nonplayable characters would try to scare the player if they were reading too calmly. In 2016, the game company Flying Mollusk released a commercial game entitled Nevermind (Table 1, row 26), which is usable with consumer level heart rate monitors. The developers created the horror genre game with the purpose of creating a more frightening experience for the player, based upon their biological signals.
ECG monitoring during gameplay can also note the varying engagement levels of players during a game (Giakoumis et al., 2011). By monitoring the engagement level of players, game developers can adjust game difficulty (Dynamic Difficulty Adjustment) and algorithms to keep players interested (Kivikangas et al., 2011; Xue et al., 2019). The company Valve has long been researching gameplay using biosignals (Ambinder, 2011), and has even patented a method for capturing player biosignals in real time to affect gameplay (Bond and Ambinder, 2009).
Using ECG during gameplay can also be used for training of breath and staying calm during stressful situations. An experiment was done using members of the Canadian Forces with this purpose (Bouchard et al., 2012) (Table 1, row 22). The participants were given refresher training on Stress Management, which included breathing practices. The participants played an adapted version of a game while ECG and skin conductance were monitored.
Biosignal Use for Affective Gaming and Research
Biosignal data can help researchers understand how a person interacts with a game—a technique that major companies have also employed to test game design and experience. Researchers can harness the biosignals of a player to provide real-time interaction and feedback in response to the player’s emotional state. This implementation in which the users’ behavior or emotional state directly affects gameplay is known as affective gaming (Kotsia et al., 2013). Affective gaming is a branch of affective computing, a type of computing that influences, arises from, or relates to human emotion (Picard, 1995). An affective program can receive input from gestures and biological signals. This is particularly helpful in gathering information in regard to learning in games (Cowley et al., 2013; Cowley and Ravaja, 2014), as well as understanding how emotions correlate to stimuli such as music (Bo et al., 2017; Suto and Oniga, 2018) or visuals (Suhaimi et al., 2018). By designing a game to be affective, a developer or an artist can tailor the experience to the person playing the game (Liu et al., 2009; Nacke et al., 2011).
Biosignals in Commercial Gaming
The largest challenge for using biosignals on a massive scale in a game environment, be it for control, monitoring, affective gaming, or for providing some sort of biofeedback, is indeed the hardware equipment. In order for biosignals to be employed on a mainstream level, the leading companies such as Sony, Valve, or Microsoft would need to develop controllers with biosensors unobtrusive and easy to use, and game developers would need to create games that would integrate these sensors and make the games affective or provide some type of biofeedback.
As we have reviewed, the present level of market penetration varies considerably among the different biosignals, depending on several factors such as the potential applications and benefits that these biosignals can bring, and the cost, reliability, or unobtrusiveness of the related sensors. Eye-tracking technologies, for example, which offer interesting possibilities for gaze control, with clear applications in VR and for providing control mechanisms to people with severe motor disabilities, have already been implemented commercially by companies such as Tobii and HTC Vive.
Concerning EEG, although consumer level and noninvasive headsets are being produced by companies such as Muse or Emotiv, this still constitutes a niche market, mostly targeting mindfulness and relaxation. If EEG sensing and interpretation will probably have to wait some more years for offering more reliable and useful possibilities at the consumer level, EMG, EDA, and ECG on their hand, which provide physiological information much simpler to measure and interpret, would seem to be ready for affective gaming.
In 2020, Sony released a patent for the Dualshock 5 controller (Andall and Hogarth, 2018), which incorporates the use of physiological signal technology. The controller design includes EDA sensors and an input for possible integration of other biosignals such as heart rate. Will this convey the massive use of biosignals in commercial controllers, and bring affective gameplay to the mainstream? Even in the case of not making gaming affective, game companies would likely find it useful to have the possibility of collecting users’ biodata during gameplay to adapt gameplay in updates or future releases. Perhaps the proposed commercial use of biofeedback from skin conductance from Sony will integrate this feature of bio-adaptability into Playstation 5 games in the future. If implemented, this would be an advance for biofeedback as well as the first popular commercial release of this technology.
Future Uses and Possibilities and Conclusion
We have presented an overview of the past and present uses of biological signals in research utilizing video games, as well as their uses in current commercial gaming and their potential for the near future. Many of the examples we have commented come from the fields of medical and psychological research, but there still remain many possibilities for the use of biological and physiological signals in tandem with video games. With Virtual Reality now an accessible and commonplace technology, more researchers are integrating it into treatment for anxiety disorders. The ability to monitor a patient’s emotional state is valuable, and being able to have a controlled environment, such as a game, allows a researcher or practitioner, the opportunity of coaxing a response from a patient using visual and audio stimuli. The investigation that has been done using immersive gaming has been promising; however, more integration of biosignal observation and usage for affective experiences is needed. New devices, such as several that have been discussed in this article, will make this integration easier and will assist with issues that arise from movement artifacts.
Utilizing games for research can be an effective means of studying emotional and physical states. It is clear that gaming is not waning in popularity and developers will continue to integrate more methods of interaction, and adaptability into video games and controllers.
Author Contributions
All authors contributed to manuscript revision, read, and approved the submitted version.
Funding
This research has been partially supported by the project Musical AI - PID2019-111403GB-I00/AEI/10.13039/501100011033 funded by the Spanish Ministerio de Ciencia, Innovación y Universidades (MCIU) and the Agencia Estatal de Investigación (AEI).
Conflict of Interest
The authors declare that the research was conducted in the absence of any commercial or financial relationships that could be construed as a potential conflict of interest.
Footnotes
1Muse EEG Headset https://choosemuse.com/?utm_source=google&utm_medium=cpc&gclid=Cj0KCQiAkKnyBRDwARIsALtxe7jBThM4p04pea4W-YIvNy-OaeEW5aTIQAzy8kYYZjBIn2vAxiam08YaAtjXEALw_wcB
2Emotiv Insight, https://www.emotiv.com/product/emotiv-insight-5-channel-mobile-eeg/?gclid=Cj0KCQiAkKnyBRDwARIsALtxe7jJ9pVcLRQ2zoyxcoDoNJeGX4plsd_kz21Uqw6BWCaKJgP8u1VBxOcaAufUEALw_wcB
3Emotiv EPOC https://www.emotiv.com/product/emotiv-epoc-14-channel-mobile-eeg/
4Imec Wearable Neurotechnology https://www.imec-int.com/en/connected-health-solutions/neurotechnology
5Open BCI https://shop.openbci.com/collections/all/eeg
6Vive Pro Eye, https://www.vive.com/eu/product/vive-pro-eye/
7Tobii Eye Tracker 4C, https://gaming.tobii.com/tobii-eye-tracker-4c/
8Tower defense (TD) is a subgenre of strategy video game where the goal is to defend a player’s territories or possessions by obstructing the enemy attackers or by stopping enemies from reaching the exits, usually achieved by placing defensive structures on or along their path of attack. https://en.wikipedia.org/wiki/Tower_defense
9Ottobock Myoboy, https://shop.ottobock.us/Prosthetics/Upper-Limb-Prosthetics/Myo-Hands-and-Components/Myo-Software/MyoBoy/p/757M11∼5X-CHANGE
10Perifit, https://perifit.co/pages/perifit-benefits-faster-postnatal-recovery
11Bitalino https://bitalino.com/en/hardware
12NeuroSky, https://store.neurosky.com/collections/apps/games
13(https://www.polygon.com/2013/5/7/4307692/valve-experimenting-with-sweat-based-left-4-dead-and-eye-controlled)
References
Abdulkader, S. N., Atia, A., and Mostafa, M-S. M. (2015). Brain Computer Interfacing: Applications and Challenges. Egypt. Inform. J. 16 (2), 213–230. doi:10.1016/j.eij.2015.06.002
Ahn, M., Lee, M., Choi, J., and Jun, S. (2014). A Review of Brain-Computer Interface Games and an Opinion Survey from Researchers, Developers and Users. Sensors 14 (8), 14601–14633. doi:10.3390/s140814601
Alchalcabi, A. E., Eddin, A. N., and Shirmohammadi, S. (2017). “More Attention, Less Deficit: Wearable EEG-Based Serious Game for Focus Improvement,” 2017 IEEE 5th International Conference on Serious Games and Applications for Health (SeGAH), Perth, WA, Australia, 1–8. doi:10.1109/SeGAH.2017.7939288
Allison, B. Z., McFarland, D. J., Schalk, G., Zheng, S. D., Jackson, M. M., and Wolpaw, J. R. (2008). Towards an Independent Brain-Computer Interface Using Steady State Visual Evoked Potentials. Clin. Neurophysiol. 119 (2), 399–408. doi:10.1016/j.clinph.2007.09.121
Ambinder, M. (2011). “Biofeedback in Gameplay: How Valve Measures Physiology to Enhance Gaming Experience,” Game Developers Conference 2011 Proceedings, San Francisco, CA, USA, 28 February–4 March, Available at: http://www.gdcvault.com/play/1014510/Biofeedback-in-Gameplay-How-Valve (Accessed March 6, 2021).
Andall, , and Hogarth, (2018). inventor: Sony Interactive Entertainment Europe Limited., Assignee. Sensing Apparatus And Method. United States Patent US 20200054940.
Armiger, R., and Vogelstein, R. (2008). “Air-Guitar Hero: a Real-Time Video Game Interface for Training and Evaluation of Dexterous Upper-Extremity Neuroprosthetic Control Algorithms,” 2008 IEEE-BIOCAS Biomedical Circuits and Systems Conference, Baltimore, MD, 1, 121–124.
Athavale, Y., and Krishnan, S. (2017). Biosignal Monitoring Using Wearables: Observations and Opportunities. Biomed. Signal Process. Control. 38, 22–33. doi:10.1016/j.bspc.2017.03.011
Balasubramanian, V., and Adalarasu, K. (2007). EMG-based Analysis of Change in Muscle Activity during Simulated Driving. J. Bodywork Mov. Therapies 11, 151–158. doi:10.1016/j.jbmt.2006.12.005
Blackwood, D. H., and Muir, W. J. (1990). Cognitive Brain Potentials and Their Application. Br. J. Psychiatry 9, 96–101. doi:10.1192/s0007125000291897
Bo, H., Ma, H., and Li, H. (2017). Music-evoked Emotion Classification Using EEG Correlation-Based Information. Annu Int Conf IEEE Eng Med Biol Soc. 2017, 3348–3351. doi:10.1109/EMBC.2017.8037573
Bond, S., and Ambinder, M. (2009). inventor Corporation. Player Biofeedback for Dynamically Controlling a Video Game State. United States Patent Office. US 9511289B2.VALVE.
Bouchard, S., Bernier, F., Boivin, E., Morin, B., and Robillard, G. (2012). Using Biofeedback while Immersed in a Stressful Videogame Increases the Effectiveness of Stress Management Skills in Soldiers. PloS one 7 (4), e36169. doi:10.1371/journal.pone.0036169
Bulling, A., Roggen, D., and Tröster, G. (2009). Wearable EOG Goggles: Seamless Sensing and Context-Awareness in Everyday Environments. J. Ambient Intelligence Smart Environments 1 (2), 157–171. doi:10.3233/ais-2009-0020
Carofiglio, V., de Carolis, B., and D’Errico, F. (2019). “A BCI-Based Assessment of a Player’s State of Mind for Game Adaptation,” Proceedings of 3rd Workshop on Games-Human Interaction (GHItaly19). Padova, Italy, September 23, 2019.
Chanel, G., Rebetez, C., Bétrancourt, M., and Pun, T. (2008). “Boredom, Engagement and Anxiety as Indicators for Adaptation to Difficulty in Games,” MindTrek '08: Proceedings of the 12th international conference on Entertainment and media in the ubiquitous era, Tampere, Finland, 1, 13–17. doi:10.1145/1457199.1457203
Chęć, A., Olczak, D., Fernandes, T., and Ferreira, H. (2015). Physiological Computing Gaming-Use of Electrocardiogram as an Input for Video Gaming,” in International Conference on Physiological Computing Systems, Loire Valley, France, (SCITEPRESS) 2, 157–163.
Chen, Y-H., de Beeck, M., Vanderheyden, L., Carrette, E., Mihajlović, V., Vanstreels, K., et al. (2014). Soft, Comfortable Polymer Dry Electrodes for High Quality ECG and EEG Recording. Sensors 14 (12), 23758–23780. doi:10.3390/s141223758
Converse, H., Ferraro, T., Jean, D., Jones, L., Mendhiratta, V., Naviasky, E., et al. (2013). “An EMG Biofeedback Device for Video Game Use in Forearm Physiotherapy,” Proceedings of IEEE Sensors, Baltimore, MD, USA, Nov 3-6, 2013, 1–4.
Cowley, B., and Ravaja, N. (2014). Learning in Balance: Using Oscillatory EEG Biomarkers of Attention, Motivation and Vigilance to Interpret Game-Based Learning. Cogent Edu. 1 (1), 1–23. doi:10.1080/2331186x.2014.962236
Cowley, B., Ravaja, N., and Heikura, T. (2013). Cardiovascular Physiology Predicts Learning Effects in a Serious Game Activity. Comput. Edu. 60, 299–309. doi:10.1016/j.compedu.2012.07.014
Dawson, M. E., Schell, A. M., and Filion, D. L. (2017). “The Electrodermal System”. Cambridge Handbooks in Psychology.in Handbook of Psychophysiology. Editors J. T. Cacioppo, L. G. Tassinary, and G. G. Berntson (Cambridge University Press), 217–243.
De Luca, C. J. (1984). Myoelectrical Manifestations of Localized Muscular Fatigue in Humans. Crit. Rev. Biomed. Eng. 11 (4), 251–279.
Dekker, A., and Champion, E. (2007). “Please Biofeed the Zombies: Enhancing the Gameplay and Display of a Horror Game Using Biofeedback,” 3rd Digital Games Research Association International Conference: Situated Play, DiGRA, 550–558.
Friedrich, E. V. C., Suttie, N., Sivanathan, A., Lim, T., Louchart, S., and Pineda, J. A. (2014). Brainâ€"computer Interface Game Applications for Combined Neurofeedback and Biofeedback Treatment for Children on the Autism Spectrum. Front. Neuroeng. 7, 21. doi:10.3389/fneng.2014.00021
Giakoumis, D., Tzovaras, D., Moustakas, K., and Hassapis, G. (2011). Automatic Recognition of Boredom in Video Games Using Novel Biosignal Moment-Based Features. IEEE Trans. Affective Comput. 2 (3), 119–133. doi:10.1109/t-affc.2011.4
Gorzkowski, S., and Sarwas, G. (2019). “Exploitation of EMG Signals for Video Game Control,” 20th International Carpathian Control Conference (ICCC), Krakow-Wieliczka, Poland, May 26–29, 2019, 1–6.
Hayes, C., Teressa, F., Daniel, J., Jones, L., Mendhiratta, V., Naviasky, E. P., et al. (2013). An EMG biofeedback device for video game use in forearm physiotherapy. Proc. IEEE Sens., 1–4. doi:10.1109/ICSENS.2013.6688474
Hjelm, S., and Browall, C. (2000). “Brainball-Using Brain Activity for Cool Competition,” Proceedings NordiCHI 2000, 177.
Isokoski, P., Joos, M., Špakov, O., and Martin, B. (2009). Gaze Controlled Games. Univ. Access Inf. Soc. 8, 323–337. doi:10.1007/s10209-009-0146-3
Jiang, L., Guan, C., Zhang, H., Wang, C., and Jiang, B. (2011). “Brain Computer Interface Based 3D Game for Attention Training and Rehabilitation,” Proceedings of the 2011 6th IEEE Conference on Industrial Electronics and Applications ICIEA, Beijing, China, 124–127.
Karthikeyan, P., Murugappan, M., and Yaacob, S. (2011). “A Review on Stress Inducement Stimuli for Assessing Human Stress Using Physiological Signals,” Proceedings–2011 IEEE 7th International Colloquium on Signal Processing and its ApplicationsCSPA, 420–425.
Kivikangas, J. M., Chanel, G., Cowley, B., Ekman, I., Salminen, M., Järvelä, S., et al. (2011). A Review of the Use of Psychophysiological Methods in Game Research. journal gaming virtual worlds 3, 181–199. doi:10.1386/jgvw.3.3.181_1
Kotsia, I., Zafeiriou, S., and Fotopoulos, S. (2013). “Affective Gaming: A Comprehensive Survey,” IEEE Computer Society Conference on Computer Vision and Pattern Recognition Workshops, 663–670. doi:10.1109/CVPRW.2013.100
Kritikos, J., Alevizopoulos, G., and Koutsouris, D. (2021). Personalized Virtual Reality Human-Computer Interaction for Psychiatric and Neurological Illnesses: A Dynamically Adaptive Virtual Reality Environment that Changes According to Real-Time Feedback from Electrophysiological Signal Responses. Front. Hum. Neurosci. 15, 596980. doi:10.3389/fnhum.2021.596980
Kumar, D., and Sharma, A. (2016). Electrooculogram-based Virtual Reality Game Control Using Blink Detection and Gaze Calibration. International Conference on Advances Computing, Communications Inform (ICACCI) Jaipur, India. 2358–2362.
Lim, S., Yeo, M., and Yoon, G. (2019). Comparison between Concentration and Immersion Based on EEG Analysis. Sensors 19, 1669. doi:10.3390/s19071669
Liu, C., Agrawal, P., Sarkar, N., and Chen, S. (2009). Dynamic Difficulty Adjustment in Computer Games through Real-Time Anxiety-Based Affective Feedback. Int. J. Human-Computer Interaction 25 (6), 506–529. doi:10.1080/10447310902963944506-529
Martin, N., Mathieu, N., Pallamin, N., Ragot, M., and Diverrez, J. (2018). Automatic Recognition of Virtual Reality Sickness Based on Physiological Signals. Amsterdam, Netherlands: IBC.
Mercado, J., Espinosa-Curiel, I., and Escobedo, L. (2019). Developing and evaluating a BCI video game for neurofeedback training: the case of autism. Multimed Tools Appl. 78, 13675–13712. doi:10.1007/s11042-018-6916-2
Müller, M. M., and Hillyard, S. (2000). Concurrent Recording of Steady-State and Transient Event-Related Potentials as Indices of Visual-Spatial Selective Attention. Clin. Neurophysiol. 111 (9), 1544–1552. doi:10.1016/s1388-2457(00)00371-0
Murch, W. S., Limbrick‐Oldfield, E. H., Ferrari, M. A., MacDonald, K. I., Fooken, J., Cherkasova, M. V., et al. (2020). Zoned in or Zoned Out? Investigating Immersion in Slot Machine Gambling Using Mobile Eye‐tracking. Addiction 115 (6), 1127–1138. doi:10.1111/add.14899
Nacke, L., and Lindley, C. A. (2008). “Flow and Immersion in First-Person Shooters,” Proceedings of the 2008 Conference on Future Play: Research, Play. share, Toronto, Canada. 1. 81–88. doi:10.1145/1496984.1496998
Nacke, L., Kalyn, M., Lough, C., and Mandryk, R. (2011). Biofeedback Game Design: Using Direct and Indirect Physiological Control to Enhance Game Interaction. Conference on Hum. Factors Comput. Syst. Vancouver, Canada 1, 103–113.
Norman, S., Dennison, M., Wolbrecht, E., Cramer, S., Srinivasan, R., Srinivasan, R., et al. (2016). Movement Anticipation and EEG: Implications for BCI-Contingent Robot Therapy. IEEE Trans. on Neural Syst. Rehabil. Engineering 24 (8), 911–919. doi:10.1109/tnsre.2016.2528167
Parsons, T. D., and Reinebold, J. L. (2012). Adaptive virtual environments for neuropsychological assessment in serious games. IEEE Trans. Consumer Electron. 2, 197–204. doi:10.1109/TCE.2012.6227413
Plass-Oude Bos, D., Reuderink, B., Laar, B., Gürkök, H., Mühl, C., Poel, M., et al. (2010). Brain-Computer Interfacing and Games. doi:10.1007/978-1-84996-272-8_10
Porter, A. M., and Goolkasian, P. (2019). Video games and stress: how stress appraisals and game content affect cardiovascular and emotion outcomes. Front. psycho. 10, 967. doi:10.3389/fpsyg.2019.00967
Polygon. (2020). Valve Experimenting with Sweat-Based Left 4 Dead And Eye-Controlled Portal 2. Available at: https://www.polygon.com/2013/5/7/4307692/valve-experimenting-with-sweat-based-left-4-dead-and-eye-controlled (Accessed March 11, 2020).
Prahm, C., Kayali, F., Vujaklija, I., Sturma, A., and Aszmann, O. (2017). “Increasing Motivation, Effort and Performance through Game-Based Rehabilitation for Upper Limb Myoelectric Prosthesis Control,” International Conference on Virtual Rehabilitation (ICVR), Montreal, QC, Canada, 1–6. doi:10.1109/ICVR.2017.8007517
Rincon, A. L., Yamasaki, H., and Shimoda, S. (2016). “Design of a Video Game for Rehabilitation Using Motion Capture, EMG Analysis and Virtual Reality,” International Conference on Electronics, Communications and Computers, CONIELECOMP 2016, 198–204. doi:10.1109/CONIELECOMP.2016.7438575
Russoniello, C., O’Brien, K., and Parks, J. (2009). The Effectiveness of Casual Video Games in Improving Mood and Decreasing Stress. J. Cyber Ther. Rehabil. 2, 53–66.
Sakurazawa, S., Yoshida, N., and Munekata, N. (2004). “Entertainment feature of a game using skin conductance response”. in Proceedings of the 2004 ACM SIGCHI International Conference on Advances in Computer Entertainment Technology, Singapore, June 3–5, 2004. 181–186. doi:10.1145/1067343.1067365
Soares, R., Siqueira, E., Miura, M., Silva, T., Jacobi, R., and Castanho, C. (2017). Biofeedback Sensors in Electronic Games: A Practical Evaluation. 56–65. doi:10.1109/SBGames.2017.00015
Suhaimi, N., Yuan, C., Teo, J., and Mountstephens, J. (2018). “Modeling the Affective Space of 360 Virtual Reality Videos Based on Arousal and Valence for Wearable EEG-Based VR Emotion Classification,” Proceedings–2018 IEEE 14th International Colloquium on Signal Processing and its Application, 167–172.
Suto, J., and Oniga, S. (2018). Music Stimuli Recognition in Electroencephalogram Signal. ElAEE 24, 68–71. doi:10.5755/j01.eie.24.4.21482
Vachiratamporn, V., Legaspi, R., Moriyama, K., Fukui, K-I., and Numao, M. (2015). An Analysis of Player Affect Transitions in Survival Horror Games. J. Multimodal User Inter. 9, 43–54. doi:10.1007/s12193-014-0153-4
Vamvakousis, Z., and Ramirez, R. (2016). The EyeHarp: A Gaze-Controlled Digital Musical Instrument. Front. Psychol. 7. doi:10.3389/fpsyg.2016.00906
Vickers, S., Istance, H., and Smalley, M. (2010). “EyeGuitar: Making Rhythm Based Music Video Games Accessible Using Only Eye Movements,” Proceedings of the 7th International Conference on Advances in Computer Entertainment Technology (ACE '10), New York, NY, USA, Association for Computing Machinery, 36–39.
Visconti, P., Gaetani, F., Zappatore, G. A., and Primiceri, P. (2018). Technical Features and Functionalities of Myo Armband: An Overview on Related Literature and Advanced Applications of Myoelectric Armbands Mainly Focused on Arm Prostheses. Int. J. Smart Sensing Intell. Syst. 11, 1–25. doi:10.21307/ijssis-2018-005
Vujaklija, I., Prahm, C., Agnes, S., Aszmann, O., and Kayali, F. (2017). “Increasing Motivation, Effort and Performance through Game-Based Rehabilitation for Upper Limb Myoelectric Prosthesis Control,” International Conference on Virtual Rehabilitation, ICVR, 1–6. doi:10.1109/ICVR.2017.8007517
Wang, Q., Wang, H., and Hu, F. (2014). “Combining EEG and VR Technology to Assess Fear of Heights,” Proceedings–9th International Conference on Information Technology in Medicine and Education, ITME 2018, 110–114.
Keywords: EMG, video games, EEG, biofeedback, eye tracking, ECG
Citation: Hughes A and Jorda S (2021) Applications of Biological and Physiological Signals in Commercial Video Gaming and Game Research: A Review. Front. Comput. Sci. 3:557608. doi: 10.3389/fcomp.2021.557608
Received: 30 April 2020; Accepted: 16 April 2021;
Published: 11 May 2021.
Edited by:
Hugo Plácido da Silva, Institute of Telecommunications (IT), PortugalReviewed by:
Benjamin Cowley, University of Helsinki, FinlandIvan Miguel Pires, Universidade da Beira Interior, Portugal
Copyright © 2021 Hughes and Jorda. This is an open-access article distributed under the terms of the Creative Commons Attribution License (CC BY). The use, distribution or reproduction in other forums is permitted, provided the original author(s) and the copyright owner(s) are credited and that the original publication in this journal is cited, in accordance with accepted academic practice. No use, distribution or reproduction is permitted which does not comply with these terms.
*Correspondence: Alayna Hughes, alayna.hughes@upf.edu; Sergi Jorda, sergi.jorda@upf.edu