- 1Department of Rehabilitation Sciences, MGH Institute of Health Professions, Boston, MA, United States
- 2Department of Speech and Hearing Bioscience and Technology, Harvard University, Boston, MA, United States
- 3Google LLC, Mountain View, CA, United States
Despite significant advancements in automatic speech recognition (ASR) technology, even the best performing ASR systems are inadequate for speakers with impaired speech. This inadequacy may be, in part, due to the challenges associated with acquiring a sufficiently diverse training sample of disordered speech. Speakers with dysarthria, which refers to a group of divergent speech disorders secondary to neurologic injury, exhibit highly variable speech patterns both within and across individuals. This diversity is currently poorly characterized and, consequently, difficult to adequately represent in disordered speech ASR corpora. In this article, we consider the variable expressions of dysarthria within the context of established clinical taxonomies (e.g., Darley, Aronson, and Brown dysarthria subtypes). We also briefly consider past and recent efforts to capture this diversity quantitatively using speech analytics. Understanding dysarthria diversity from the clinical perspective and how this diversity may impact ASR performance could aid in (1) optimizing data collection strategies for minimizing bias; (2) ensuring representative ASR training sets; and (3) improving generalization of ASR for difficult-to-recognize speakers. Our overarching goal is to facilitate the development of robust ASR systems for dysarthric speech using clinical knowledge.
Introduction
Dysarthria, or impaired speech due to motoric deficits, can have a detrimental impact on functional communication, often leading to significantly reduced quality of life (Hartelius et al., 2008). For individuals with speech impairments, automatic speech recognition (ASR) systems can enhance accessibility and interpersonal communication. However, inadequate acoustic models continue to impede the widespread success of ASR for disordered speech (Gupta et al., 2016; Moore et al., 2018). The limits of disordered speech ASR may be, in part, a byproduct of the significant variety of abnormal speech patterns across individuals (Duffy, 2013) and their underrepresentation in training corpora (Gupta et al., 2016). Nevertheless, studies on ASR for dysarthria have rarely considered this diversity (Blaney and Wilson, 2000; Benzeghiba et al., 2007; Gupta et al., 2016; Keshet, 2018; Moore et al., 2018). In this perspective article, we examine how speech impairment diversity has been characterized based on clinical models and how this diversity may impact ASR performance.
ASR can be broadly classified into (1) speaker-independent (SI) systems, which are typically trained on large multispeaker datasets, and (2) personalized systems, which can be trained either by adapting an existing SI model to a target speaker (i.e., speaker-adaptive [SA]) or by solely using the target speaker's speech data (i.e., speaker-dependent [SD]). Although commercially developed SI ASR systems have demonstrated low word error rates (WER) for healthy speakers, these systems perform considerably worse with impaired speech (Moore et al., 2018). An increasing amount of work has thus investigated the use of personalized systems for speakers with speech impairments, demonstrating significantly stronger performance compared to that of SI systems (Mengistu and Rudzicz, 2011; Kim et al., 2013; Mustafa et al., 2014; Xiong et al., 2019; Takashima et al., 2020; Green et al., 2021). Green et al. (2021), for example, recently demonstrated that the recognition accuracy of short phrases using end-to-end (E2E) ASR models was 4.6% for personalized models compared to 31% for SI models.
While personalized systems have promising utility for recognizing impaired speech, they require training data from the speaker, which may be impractical for some applications and can be cumbersome for individuals with neurodegenerative diseases who are prone to fatigue. Thus, a more efficient and effective approach would be to improve the recognition accuracy for existing SI systems for dysarthric speech. For example, prior work has shown improvements in performance of SI systems when training sets include dysarthric speakers, thereby providing more variability on which to train (Mengistu and Rudzicz, 2011; Mustafa et al., 2014). However, even the highest performing SI ASR models are inadequate for impaired speakers.
Although poor performance has largely been attributed to the shortage of disordered speech training datasets, closing the performance gap is likely to require not only more data but also sufficiently diverse corpora. Indeed, solely adding speakers to the training corpora in attempts to improve ASR performance is inefficient, expensive, and possibly unachievable. Ensuring dysarthric speech diversity requires conceptual schemes for identifying salient atypical speech variables and their expressed ranges across individuals. In this article, we consider several conceptual schemes used by speech-language pathologists to clinically characterize dysarthria diversity often for the purpose of speech diagnosis. An improved understanding of the diversity inherent to dysarthria and its potential impact on ASR performance could lead to (1) optimized data collection strategies for minimizing bias; (2) sufficiently representative ASR training sets; and (3) more widespread generalization across ASR users and, in turn, stronger performance for difficult-to recognize speakers. We consider the following questions:
1. What types of diversity need to be represented in dysarthria ASR training corpora?
2. What phonemic patterns are present in dysarthric speech and therefore might impact dysarthria ASR performance?
3. What can be done to adequately represent the different sources of variability in dysarthria ASR training corpora?
Characterizing Dysarthria Diversity
What Types of Diversity Need to Be Represented in Dysarthria ASR Training Corpora?
Diversity in Speech Severity
To date, the most frequently used metric for distinguishing variation in a dysarthria research cohort is overall speech impairment severity (Duffy, 2013). Severity is a multidimensional construct that refers to the speaker's overall impairment and includes a range of components, including naturalness, intelligibility, and subsystem abnormalities (see section Diversity in Speech Subsystems Impairment) (Duffy, 2013). Severity is often indexed by trained listeners, such as speech-language pathologists, who use adjectival descriptors (e.g., mild, moderate, severe, and profound). Alternatively, severity can be assessed using human transcription intelligibility, which indicates a listener's ability to understand the speaker based on the speech signal alone (Yorkston et al., 2007). While a functional metric, intelligibility is just one component of severity and does not necessarily account for all the different fluctuations in speech that are influenced by severity (e.g., changes in voice and resonance), especially for more mild speech impairment (Rong et al., 2015).
Including the full range of speech severities in ASR training sets is essential because good recognition accuracy for mild speech is unlikely to generalize to more severely affected speech (Moore et al., 2018). Thus, sufficient representation of speakers with severe dysarthria, in addition to those with mild and moderate impairments, in the training dataset could provide a more sustainable approach for enabling models that generalize to speakers across the severity continuum. Representing diversity only with severity, however, fails to address the substantial variety of aberrant speech features that characterize clinically distinct dysarthria variants. Other sources of diversity in dysarthric speech must, therefore, be considered to develop inclusive and sufficiently representative datasets.
Diversity in Dysarthria Type
One of the most established clinical taxonomies for speech motor disorders was developed over 50 years ago by Darley, Aronson, and Brown (DAB) (Darley et al., 1969). The DAB labeling system distinguishes 38 atypical speech features that are rated on a 7-point scale and groups dysarthria types based on speech feature profiles. For the development of the DAB model, the authors stratified dysarthric speakers based on clusters of speech features associated with lesions in specific regions of the central and peripheral nervous systems. These clusters are associated with at least five subtypes of dysarthria: flaccid, spastic, ataxic, hypokinetic, and hyperkinetic (see Figure 1). In many cases, patients exhibit a combination of the five subtypes (i.e., mixed dysarthria) (Darley et al., 1969). In addition to its clinical and neurological implications, the DAB model can serve as a basic heuristic in developing comprehensive and representative ASR corpora.
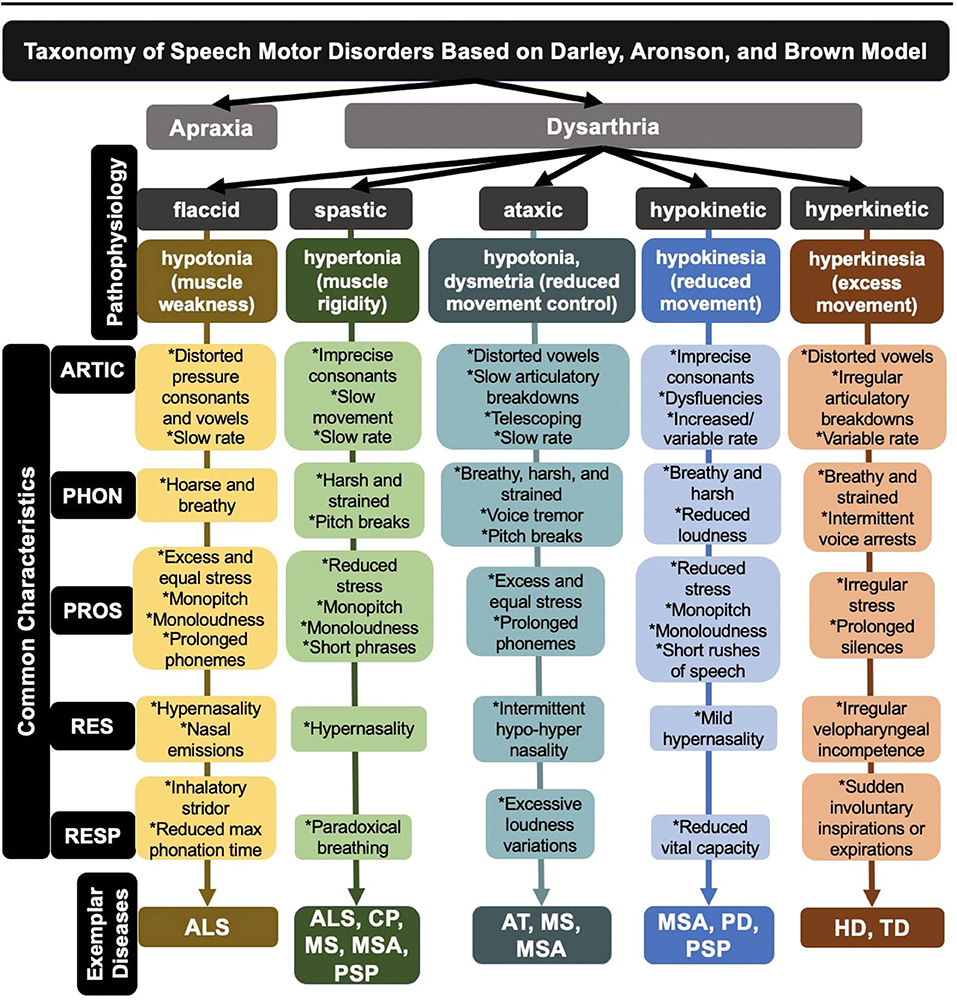
Figure 1. Breakdown of dysarthria subtypes within a widely used taxonomy of speech motor disorders. ALS, amyotrophic lateral sclerosis; CP, cerebral palsy; AT, ataxia; HD, Huntington's disease; MS, multiple sclerosis; MSA, multiple systems atrophy; PD, Parkinson's disease; PSP, progressive supranuclear palsy; TD, tardive dyskinesia; ARTIC, articulation; PHON, phonation; PROS, prosody; RES, resonance; RESP, respiration.
One disadvantage of the taxonomy, however, is that it relies entirely on subjective observations, which requires expert clinical training and may be too coarse and unreliable for capturing the range of diversity in dysarthria (Kent, 1996). To address this limitation, researchers have been exploring the diagnostic utility of a wide variety of speech analytic approaches for identifying variants of disordered speech (Rusz et al., 2018; Rowe et al., 2020)—an effort referred to as quantitative or digital phenotyping.
Diversity in Speech Subsystems Impairment
Regardless of dysarthria subtype, disordered speech is the byproduct of impairments in neural control over one or more of the five speech subsystems (i.e., respiration, phonation, resonance, prosody, and articulation) (see Figure 1). Objective characterizations of dysarthria through quantitative and digital phenotyping have allowed for more precise measures of speech, which has further illuminated the diversity in subsystem functioning. Indeed, deficits in each subsystem can engender specific aberrant speech features, many of which can be detected in the acoustic signal. For example, respiratory deficits in ataxic dysarthria can lead to excessive loudness variations, quantified acoustically using amplitude modulation (MacDonald et al., 2021); similarly, phonatory deficits in flaccid dysarthria can lead to a breathy vocal quality, quantified acoustically using cepstral peak prominence (Heman-Ackah et al., 2002).
While phonatory, resonatory, respiratory, and prosodic deficits can significantly limit communicative capacity, articulatory subsystem impairments have the greatest impact on speech intelligibility (Lee et al., 2014; Rong et al., 2015). Given the strong association between intelligibility and ASR performance (McHenry and LaConte, 2010; Tu et al., 2016; Jacks et al., 2019), it is possible that (1) articulatory motor impairments may be a major contributor to degraded ASR performance and (2) representing the range of articulatory motor impairments seen in dysarthria may maximize ASR accuracy and generalizability.
Considering the potential value of articulatory features and the need for objective and reliable measures of speech function, our group conducted a scoping review of the dysarthria literature to summarize the variety of acoustic techniques used to characterize articulatory impairments in neurodegenerative diseases (Rowe et al., under review). Across the 89 articles that met our inclusion criteria, we identified 24 different articulatory impairment features. To summarize the findings, we stratified the acoustic features into five aspects of articulatory motor control: Coordination, Consistency, Speed, Precision, and Rate (Rowe and Green, 2019). The findings demonstrated variable manifestation of articulatory impairments (1) across diseases [e.g., speakers with ataxia (AT) exhibited greater impairments in features associated with Rate than did speakers with Parkinson's disease (PD)] and (2) across articulatory components within each disease [e.g., speakers with Huntington's disease (HD) demonstrated greater impairments in Consistency than in Rate] (see Figure 2) (Rowe et al., under review).
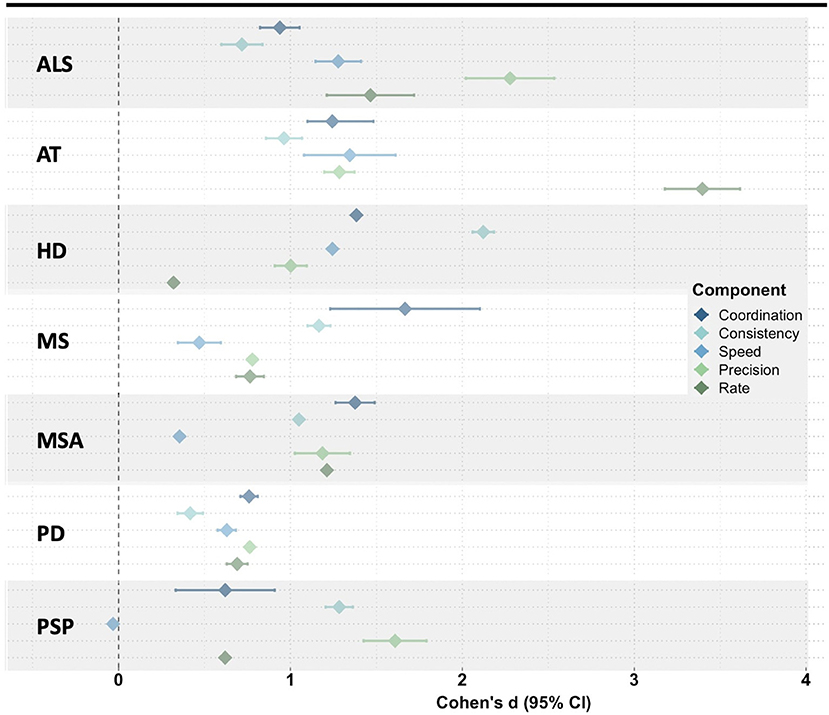
Figure 2. Meta-analysis of the mean effect size (disease group compared to healthy controls) for all acoustic features within each articulatory component. ALS, amyotrophic lateral sclerosis; AT, ataxia; HD, Huntington's disease; MS, multiple sclerosis; MSA, multiple systems atrophy; PD, Parkinson's disease; PSP, progressive supranuclear palsy.
Within-Speaker Variability Due to Motor Disease Type, Disease Progression, Fatigue, and Medication Use
Our discussion thus far has focused on between-speaker differences in severity, dysarthria type, and subsystem involvement. However, there is also a significant amount of within-speaker variability that should be considered in ASR corpora development. For example, some dysarthria types, such as ataxic dysarthria, exhibit inconsistent motor patterns of limb and speech muscles (Darley et al., 1969), which can result in significant variability even across repetitions of the same utterance. Furthermore, across all dysarthria types, changes in disease progression, fatigue, and medication use can lead to rapid and transitory fluctuations in speech. Indeed, progressive diseases, such as ALS or PD, can result in declines in speech performance over several months or even weeks. Daily fluctuations in speech patterns can occur in patient populations who are prone to fatigue (Abraham and Drory, 2012). Lastly, medication use can result in dramatic changes—both positive and negative—in speech output. For example, levodopa has been related to improvements in voice quality, pitch variation, and articulatory function in patients with PD (Wolfe et al., 1975), while antipsychotic medication has been related to excess word stress and increased timing deficits in patients with HD (Rusz et al., 2014). To mitigate the detrimental effects of within-speaker variability on ASR performance, training datasets may need to include multiple instances of the same utterances recorded at different timepoints in individuals experiencing frequent speech changes (due to motor disease type, disease progression, fatigue, and/or medication use).
What Phonemic Patterns Are Present in Dysarthric Speech and Therefore Might Impact ASR Performance?
The influence of dysarthria diversity on phonemes is complex and not fully understood, as phoneme production involves intricate interactions between speech subsystems. Nevertheless, while modern E2E ASR systems often operate at the word or subword level (Kochenderfer, 2015) and employ a strong language model, sound-level distortions may still have a substantial negative impact on the recognition accuracy. In these cases, it may be necessary to compensate for acoustic distortions by increasing their representation in the training data or adjusting the encoder. Previous research has used methods such as phoneme confusion matrices to identify phonetic error patterns and create pronunciation models. For instance, Caballero-Morales and Trujillo-Romero (2014) examined substitution errors made by an ASR system1 for a speaker with severe dysarthria. They noted that phonemes /r/, /s/, /sh/, and /th/ and phonemes /k/, /m/, and /p/ were consistently substituted by /f/ and /t/, respectively. The authors suggested that an improved system could use these error patterns to estimate /k/, /m/, or /p/ from a recognized /t/ (Caballero-Morales and Trujillo-Romero, 2014). However, most of the dysarthria ASR literature is based on datasets that combine subtypes of dysarthria and, therefore, do not specify which phonemes are misrecognized for each subtype. We propose that a heterogeneous corpus of disordered speech based on known error patterns may improve phoneme recognition. Below, we describe a subset of such error patterns in individuals with dysarthria. A more detailed and extensive list of these patterns can be found in Duffy (2013).
Few studies, to our knowledge, have examined the association between dysarthria subtype and ASR phonemic error patterns. Shor et al. (2019) examined the WER of an ASR model fine-tuned to speakers with ALS, a neurodegenerative disease characterized by mixed flaccid-spastic dysarthria. The study found that (1) /p/, /k/, /f/, and /zh/ were among five phonemes that accounted for the highest likelihood of deletion and (2) /m/ and /n/ accounted for 17% of substitution/insertion errors in the ASR response (Shor et al., 2019). The authors' first finding is consistent with the muscular weakness and low muscle tone characteristic of flaccid dysarthria, which frequently leads to deficits in sounds that require a buildup of pressure (i.e., pressure consonants) (Darley et al., 1969). Furthermore, flaccidity may lead to hypernasality due to air escape from the nasal cavity (i.e., velopharyngeal insufficiency). As a result, speakers often incorrectly insert nasal consonants such as /m/ and /n/ during speech (Duffy, 2013), which is consistent with Shor et al. (2019)'s latter finding. Increased severity in speakers with ALS also affects phonetic features, including stop-nasal (e.g., “no” for “toe”) and glottal-null (e.g., “high” for “eye”) contrasts (Kent et al., 1989). Additionally, abnormal lingual displacement and coupling in ALS has been associated with reduced vowel distinctiveness (Rong et al., 2021).
Spastic dysarthria is characterized by muscle stiffness and rigidity (Darley et al., 1969). Prior work on speakers with cerebral palsy (CP), who often exhibit pure spastic dysarthria, demonstrated that the predominant phonemic errors occurred on fricatives (Platt et al., 1980), suggesting that spasticity impairs oral constriction. A more recent study corroborated this finding by highlighting abnormalities in the fricative /s/ in speakers with CP (Chen and Stevens, 2001). Another etiology of spastic dysarthria—traumatic brain injury—can result in phonetic contrast errors between glottal-null (e.g., “hall”/“all”), voiced-voiceless (e.g., “bit”/“pit”), alveolar-palatal (e.g., “shy”/“sigh”), and nasal-stop (e.g., “meat”/“beat”) sounds (Roy et al., 2001).
Ataxic dysarthria is characterized by muscle weakness and incoordination (Darley et al., 1969). Seminal acoustic work has described the impact of voice onset time (VOT) disturbances on voicing contrasts (Ackermann and Hertrich, 1997). Later research revealed similar findings, demonstrating that VOT abnormalities in speakers with Friedreich's ataxia (FA) resulted in voicing contrast errors (e.g., /d/ vs. /t/ or /s/ vs. /z/) (Blaney and Hewlett, 2007).
Hypokinetic dysarthria is characterized by reduced range and speed of movement (Darley et al., 1969). Acoustically, speakers with hypokinetic dysarthria secondary to PD tend to replace a stop gap with low-intensity noise due to incomplete plosive closure, a process known as spirantization, which often occurs on voiceless phonemes, such as /p/, /t/, or /k/ (Canter, 1965). Reduced range of motion characteristic of hypokinesia also leads to articulatory undershoot and is reflected in features such as reduced second formant (F2) slope and restricted vowel space (Kim et al., 2009), which can lead to vowel centralization (e.g., /uh/ for /i/).
Lastly, hyperkinetic dysarthria, which is characterized by excess movement, encompasses a diverse range of speech characteristics (Darley et al., 1969). The phonemic errors in speakers with hyperkinesia are often influenced by the associated movement disorder. For example, hyperkinesia associated with HD may lead to variability in VOT and incomplete closure of pressure consonants, which could result in voicing substitutions (e.g., /t/ for /d/) and manner substitutions (e.g., /z/ for /d/), respectively (Hertrich and Ackermann, 1994). However, hyperkinesia associated with tardive dyskinesia, an antipsychotic medication side effect, may lead to excessive formant fluctuations and distorted vowels during sustained phonation (e.g., sustained /ah/) (Gerratt et al., 1984).
Overall, understanding the phonemic patterns specific to different dysarthria types can provide insight into which words or subwords (e.g., those that include pressure consonants or nasal sounds) may need to be disproportionately represented in the training data.
Considerations for Improving ASR Corpora
What Can Be Done to Adequately Represent the Different Sources of Diversity in Dysarthria ASR Training Corpora?
Deploying E2E machine learning models may preclude the need to understand the underlying pathophysiological phenomena given a sufficiently diverse training set. However, the current ineffectiveness of ASR approaches for dysarthric speech suggests that the limits of E2E models are presently defined by the lack of training sample diversity, which in this case is the wide variety and variability of dysarthric speech patterns both between and within speakers. Attempting to capture this diversity solely by adding more speakers is a costly endeavor that would likely be insufficient. The domain knowledge that we have discussed in this article is likely to help optimize participant selection strategies in large cohort studies on speech disordered ASR.
We propose that developing diverse corpora may involve a principled method of creating datasets for highly heterogeneous data, which may best be achieved through a three-pronged approach: (1) clinical phenotyping (i.e., characterizations of speech based on perceptual features); (2) quantitative phenotyping (i.e., characterizations of speech based on objective features); and (3) data-driven clustering (unsupervised groupings of speakers). Clinical phenotyping will require domain experts, such as speech-language pathologists, to guide the inclusion criteria for ensuring adequate representation of atypical speech characteristics (e.g., speech severity, articulatory deficits, etc.). Ultimately, with large datasets and validated quantitative measures of speech, data-driven clustering of dysarthric speech characteristics may become feasible. Upon the development of larger and more diverse datasets, quantifying heterogeneity with a diversity metric may be the next step toward ensuring that the training samples are sufficiently diverse. Such a metric will only be possible with a deeper understanding of the potential variables to consider in dysarthria and their impact on speech changes.
Of course, all these approaches will need to be supported by large-scale data collection efforts that will require partnerships with speech-language pathology clinics, private foundations, and medical institutions (MacDonald et al., 2021). This effort will be greatly facilitated by the development of secure but accessible electronic medical record systems and mHealth platforms (i.e., the use of mobile technologies that improve health outcomes), which will, in turn, aid in identifying and collecting speech recordings from individuals with diverse etiologies and speech impairments (Ramanarayanan et al., 2022).
Conclusions
Improving ASR accuracy for dysarthric speech may have significant implications for communication and quality of life. This article outlined the sources of diversity inherent to speech motor disorders, their potential impact on ASR performance, and the importance of their representation in training sets. Representing dysarthric speech variability in ASR corpora may be an important step for improving disordered speech ASR and is consistent with the call to action in the artificial intelligence community to reduce bias in the training data by increasing diversity.
Data Availability Statement
The original contributions presented in the study are included in the article/supplementary materials, further inquiries can be directed to the corresponding author/s.
Author Contributions
All authors listed have made a substantial, direct, and intellectual contribution to the work and approved it forpublication.
Funding
This work was supported by NIH-NIDCD under Grants K24DC016312, F31DC019556, F31DC019016, and F31DC020108.
Conflict of Interest
KT was an employee of Google LLC.
The remaining authors declare that the research was conducted in the absence of any commercial or financial relationships that could be construed as a potential conflict of interest.
Publisher's Note
All claims expressed in this article are solely those of the authors and do not necessarily represent those of their affiliated organizations, or those of the publisher, the editors and the reviewers. Any product that may be evaluated in this article, or claim that may be made by its manufacturer, is not guaranteed or endorsed by the publisher.
Footnotes
1. ^Note that the adapted ASR system did not employ an e2e deep learning model. Thus, because the system did not possess a strong language model, phoneme confusion could be measured in a meaningful way.
References
Abraham, A., and Drory, V. E. (2012). Fatigue in motor neuron diseases. Neuromuscul. Disord. 22, S198–S202. doi: 10.1016/j.nmd.2012.10.013
Ackermann, H., and Hertrich, I. (1997). Voice onset time in ataxic dysarthria. Brain Lang. 56, 321–333. doi: 10.1006/brln.1997.1740
Benzeghiba, M., De Mori, R., Deroo, O., Dupont, S., Erbes, T., Jouvet, D., et al. (2007). Automatic speech recognition and speech variability: a review. Speech Commun. 49, 763–786. doi: 10.1016/j.specom.2007.02.006
Blaney, B., and Hewlett, N. (2007). Dysarthria and Friedreich's ataxia: what can intelligibility assessment tell us? Int. J. Lang. Commun. Disord. 42, 19–37. doi: 10.1080/13682820600690993
Blaney, B., and Wilson, J. (2000). Acoustic variability in dysarthria and computer speech recognition. Clin. Linguist. Phon. 14, 307–327. doi: 10.1080/02699200050024001
Caballero-Morales, S. O., and Trujillo-Romero, F. (2014). Evolutionary approach for integration of multiple pronunciation patterns for enhancement of dysarthric speech recognition. Expert Syst. Appl. 41, 841–852. doi: 10.1016/j.eswa.2013.08.014
Canter, G. J. (1965). Speech characteristics of patients with Parkinson's disease: articulation, diadochokinesis, and overall speech adequacy. J. Speech Hear. Disord. 30, 217–224. doi: 10.1044/jshd.3003.217
Chen, H., and Stevens, K. N. (2001). An acoustical study of the fricative /s/ in the speech of individuals with dysarthria. J. Speech Lang. Hear. Res. 44, 1300–1314. doi: 10.1044/1092-4388(2001/101)
Darley, F. L., Aronson, A. E., and Brown, J. R. (1969). Differential diagnostic patterns of dysarthria. J. Speech Lang. Hear. Res. 12, 246–269. doi: 10.1044/jshr.1202.246
Duffy, J. R. (2013). Motor Speech Disorders: Substrates, Differential Diagnosis, and Management, 3rd Edn. Saint Louis, MO: Elsevier Mosby.
Gerratt, B. R., Goetz, C. G., and Fisher, H. B. (1984). Speech abnormalities in tardive dyskinesia. Arch. Neurol. 41, 273–276. doi: 10.1001/archneur.1984.04050150051016
Green, J. R., MacDonald, B., Jiang, P.-P., Cattiau, J., Heywood, R., Cave, R., et al. (2021). “Automatic speech recognition of disordered speech: personalized models outperforming human listeners on short phrases,” in Proceedings of Interspeech (Brno), 4778-4782.
Gupta, R., Chaspari, T., Kim, J., Kumar, N., Bone, D., and Narayanan, S. (2016). “Pathological speech processing: state-of-the-art, current challenges, and future directions,” in Proceedings of 2016 IEEE International Conference on Acoustics, Speech and Signal Processing (ICASSP) (Shanghai), 6470–6474.
Hartelius, L., Elmberg, M., Holm, R., Loovberg, A. S., and Nikolaidis, S. (2008). Living with dysarthria: evaluation of a self-report questionnaire. Folia Phoniatr. Logop. 60, 11–19. doi: 10.1159/000111799
Heman-Ackah, Y. D., Michael, D. D., and Goding, G. S. (2002). The relationship between cepstral peak prominence and selected parameters of dysphonia. J. Voice 16, 20–27. doi: 10.1016/S0892-1997(02)00067-X
Hertrich, I., and Ackermann, H. (1994). Acoustic analysis of speech timing in Huntington's disease. Brain Lang. 47, 182–196. doi: 10.1006/brln.1994.1048
Jacks, A., Haley, K. L., Bishop, G., and Harmon, T. G. (2019). Automated speech recognition in adult stroke survivors: comparing human and computer transcriptions. Folia Phoniatr. Logop. 71, 286–296. doi: 10.1159/000499156
Kent, R. D. (1996). Hearing and believing: some limits to the auditory perceptual assessment of speech and voice disorders. Am. J. Speech Lang. Pathol. 5, 7–23. doi: 10.1044/1058-0360.0503.07
Kent, R. D., Weismer, G., Kent, J. F., and Rosenberg, J. C. (1989). Toward phonetic intelligibility testing in dysarthria. J. Speech Hear. Disord. 54, 482–499. doi: 10.1044/jshd.5404.482
Keshet, J. (2018). Automatic speech recognition: a primer for speech language pathology researchers. Int. J. Speech Lang. Pathol. 20, 599–609. doi: 10.1080/17549507.2018.1510033
Kim, M. J., Yoo, J., and Kim, H. (2013). “Dysarthric speech recognition using dysarthria-severity-dependent and speaker-adaptive models,” in Proceedings of Interspeech (Lyon), 3622–3626.
Kim, Y., Weismer, G., Kent, R. D., and Duffy, J. R. (2009). Statistical models of F2 slope in relation to severity of dysarthria. Folia Phoniatr. Logop. 61, 329–335. doi: 10.1159/000252849
Lee, J., Hustad, K. C., and Weismer, G. (2014). Predicting speech intelligibility with a multiple speech subsystems approach in children with cerebral palsy. J. Speech Lang. Hear. Res. 57, 1666–1678. doi: 10.1044/2014_JSLHR-S-13-0292
MacDonald, R. L., Jiang, P.-P., Cattiau, J., Heywood, R., Cave, R., Seaver, K., et al. (2021). “Disordered speech data collection: lessons learned at 1 million utterances from Project Euphonia,” in Proceedings of Interspeech (Brno), 4833–4837.
McHenry, M. A., and LaConte, S. M. (2010). Computer speech recognition as an objective measure of intelligibility. J. Med. Speech. Lang. Pathol. 18, 99–103.
Mengistu, K. T., and Rudzicz, F. (2011). “Adapting acoustic and lexical models to dysarthric speech,” in Proceedings of 2011 IEEE International Conference on Acoustics, Speech and Signal Processing (ICASSP) (Prague), 4924–4927.
Moore, M., Venkateswara, H., and Panchanathan, S. (2018). “Whistle blowing ASRs: evaluating the need for more inclusive speech recognition systems,” in Proceedings of Interspeech (Hyderabad), 466–470. doi: 10.21437/Interspeech.2018-2391
Mustafa, M. B., Salim, S. S., Mohamed, N., Al-Qatab, B., and Siong, C. E. (2014). Severity-based adaptation with limited data for ASR to aid dysarthric speakers. PLoS One 9, e86285. doi: 10.1371/journal.pone.0086285
Platt, L. J., Andrews, G., and Howie, P. M. (1980). Dysarthria of adult cerebral palsy: II. Phonemic analysis of articulation errors. J. Speech Lang. Hear. Res. 23, 51–55. doi: 10.1044/jshr.2301.41
Ramanarayanan, V., Lammert, A. C., Rowe, H. P., Quatieri, T. F., and Green, J. R. (2022). Speech as a biomarker: opportunities, interpretability, and challenges. ASHA Persp. Speech Sci. 7, 276–283.
Rong, P., Usler, E., Rowe, L. M., Allison, K., Woo, J., El Fakhri, G., et al. (2021). Speech intelligibility loss due to amyotrophic lateral sclerosis: the effect of tongue movement reduction on vowel and consonant acoustic features. Clin. Linguist. Phon. 11, 1–22. doi: 10.1080/02699206.2020.1868021
Rong, R., Yunusova, Y., Wang, J., and Green, J. R. (2015). Predicting early bulbar decline in amyotrophic lateral sclerosis: a speech subsystem approach. Behav. Neurol. 2015, 1–11. doi: 10.1155/2015/183027
Rowe, H. P, Shellikeri, S., Yunusova, Y., Chenausky, K., and Green, J. R. (under review). Quantifying articulatory impairments in neurodegenerative motor diseases: a scoping review meta-analysis of hypothesis-driven acoustic features.
Rowe, H. P., and Green, J. R. (2019). “Profiling speech motor impairments in persons with amyotrophic lateral sclerosis: an acoustic-based approach,” in Proceedings of Interspeech (Graz), 4509–4513.
Rowe, H. P., Gutz, S. E., Maffei, M., and Green, J. R. (2020). “Acoustic based articulatory phenotypes of amyotrophic lateral sclerosis and Parkinson's disease: towards an interpretable, hypothesis-driven framework of motor control,” in Proceedings of Interspeech (Shanghai), 4816–4820.
Roy, N., Leeper, H. A., Blomgren, M., and Cameron, R.M. (2001). A description of phonetic, acoustic, and physiological changes associated with improved intelligibility in a speaker with spastic dysarthria. Am. J. Speech Lang. Pathol. 10, 274–288. doi: 10.1044/1058-0360(2001/025)
Rusz, J., Benova, B., Ruzickova, H., Novotny, M., Tykalova, T., Hlavnicka, J., et al. (2018). Characteristics of motor speech phenotypes in multiple sclerosis. Mult. Scler. Relat. 19, 62–69. doi: 10.1016/j.msard.2017.11.007
Rusz, J., Klempir, J., Tykalova, T., Barborova, E., Cmejla, R., Ruzicka, E., et al. (2014). Characteristics and occurrence of speech impairment in Huntington's disease: possible influence of antipsychotic medication. J. Neural Transm. 121, 1529–1539. doi: 10.1007/s00702-014-1229-8
Shor, J., Emanuel, D., Lang, O., Tuval, O., Brenner, M., Cattiau, J., et al. (2019). “Personalizing ASR for dysarthric and accented speech with limited data,” in Proceedings of Interspeech (Graz), 784–788.
Takashima, R., Takiguchi, T., and Ariki, Y. (2020). “Two-step acoustic model adaptation for dysarthric speech recognition,” in Proccedings of ICASSP 2020 - 2020 IEEE International Conference on Acoustics, Speech and Signal Processing (ICASSP) (Barcelona), 6104–6108.
Tu, M., Wisler, A., Berisha, V., and Liss, J. M. (2016). The relationship between perceptual disturbances in dysarthric speech and automatic speech recognition performance. J. Acoust. Soc. Am. 140, 416–422. doi: 10.1121/1.4967208
Wolfe, V. I., Garvin, J. S., Bacon, M., and Waldrop, W. (1975). Speech changes in Parkinson's disease during treatment with L-dopa. J. Commun. Disord. 8, 271–279. doi: 10.1016/0021-9924(75)90019-2
Xiong, F., Barker, J., and Christensen, H. (2019). “Phonetic analysis of dysarthric speech tempo and applications to robust personalized dysarthric speech recognition,” in Proceedings of ICASSP 2019 - 2019 IEEE International Conference on Acoustics, Speech and Signal Processing (ICASSP) (Brighton), 5836–5840.
Keywords: training corpora, dysarthric speech, automatic speech recognition, acoustic analysis of speech, clinical framework, diversity and inclusion
Citation: Rowe HP, Gutz SE, Maffei MF, Tomanek K and Green JR (2022) Characterizing Dysarthria Diversity for Automatic Speech Recognition: A Tutorial From the Clinical Perspective. Front. Comput. Sci. 4:770210. doi: 10.3389/fcomp.2022.770210
Received: 03 September 2021; Accepted: 14 March 2022;
Published: 12 April 2022.
Edited by:
Anton Nijholt, University of Twente, NetherlandsReviewed by:
Stuart Cunningham, The University of Sheffield, United KingdomCopyright © 2022 Rowe, Gutz, Maffei, Tomanek and Green. This is an open-access article distributed under the terms of the Creative Commons Attribution License (CC BY). The use, distribution or reproduction in other forums is permitted, provided the original author(s) and the copyright owner(s) are credited and that the original publication in this journal is cited, in accordance with accepted academic practice. No use, distribution or reproduction is permitted which does not comply with these terms.
*Correspondence: Jordan R. Green, jgreen2@mghihp.edu