- 1School of Computing, University of Kent, Canterbury, United Kingdom
- 2Centre for Electronic Warfare, Information and Cyber, Cranfield University, Defence Academy of the United Kingdom, Shrivenham, United Kingdom
The perceived sensitivity of information is a crucial factor in both security and privacy concerns and the behaviors of individuals. Furthermore, such perceptions motivate how people disclose and share information with others. We study this topic by using an online questionnaire where a representative sample of 491 British citizens rated the sensitivity of different data items in a variety of scenarios. The sensitivity evaluations revealed in this study are compared to prior results from the US, Brazil and Germany, allowing us to examine the impact of culture. In addition to discovering similarities across cultures, we also identify new factors overlooked in the current research, including concerns about reactions from others, personal safety or mental health and finally, consequences of disclosure on others. We also highlight a difference between the regulatory perspective and the citizen perspective on information sensitivity. We then operationalized this understanding within several example use-cases exploring disclosures in the healthcare and finance industry, two areas where security is paramount. We explored the disclosures being made through two different interaction means: directly to a human or chatbot mediated (given that an increasing amount of personal data is shared with these agents in industry). We also explored the effect of anonymity in these contexts. Participants showed a significant reluctance to disclose information they considered “irrelevant” or “out of context” information disregarding other factors such as interaction means or anonymity. We also observed that chatbots proved detrimental to eliciting sensitive disclosures in the healthcare domain; however, within the finance domain, there was less effect. This article's findings provide new insights for those developing online systems intended to elicit sensitive personal information from users.
1. Introduction
The internet has enabled people throughout the world to connect with each other in ways that previously would have been considered unimaginable. To enable such interactions, individuals are often required to share various types of information and this can in turn lead to privacy concerns about how their personal information is stored, processed and disclosed to others.
From research, we know that a user's privacy concerns and their willingness to disclose information are affected by the perceived sensitivity of that information (Markos et al., 2018). However, it is vague and open to debate as to how “sensitive” information may be categorized. A risk-oriented definition is adopted by some studies in the literature as seen in the EU's General Data Protection Regulation (GDPR) (European Parliament, 2016) which defines sensitive information as follows:
Personal data which are, by their nature, particularly sensitive in relation to fundamental rights and freedoms merit specific protection as the context of their processing could create significant risks to the fundamental rights and freedoms.
However, several other dimensions are also introduced to explain how users perceive sensitivity including: perceived risk, possibility of harm or public availability of data can lead information to be perceived as sensitive (Ohm, 2014; Rumbold and Pierscionek, 2018). In addition to studies which explore the factors leading to a high perceived sensitivity, it is possible to report two other research themes in this area. Firstly, studies that report the perceived sensitivity of different data items at granular levels or in different usage contexts (Markos et al., 2017; Milne et al., 2017; Schomakers et al., 2019; Belen Sağlam et al., 2022). Secondly, studies which investigate the relationship between information sensitivity and disclosure (Treiblmaier and Chong, 2013; Bansal et al., 2016; Wadle et al., 2019; Aiello et al., 2020; Belen Sağlam and Nurse, 2020).
This research aims to provide a UK perspective on the research areas identified above, a problem that is missing in existing literature. To the best of our knowledge, there is also no study that synthesizes findings associated with the factors that lead certain information to be considered sensitive, sensitivity ratings of different personal data items and the comfort felt while disclosing them under different conditions. Therefore, we formulated our research question as follows: “What are the perspectives of British citizens regarding the sensitivity of the information and the impact of different factors on the disclosure of personal information?.” To answer this research question and provide key related insights into this issue, the following research objectives (RO) are defined:
• RO1: Identify the main factors that lead British citizens to regard certain information as sensitive.
• RO2: Explore the levels of sensitivity associated with the different personal data items
• RO3: Explore the impact of user factors on levels of sensitivity of the different personal data items.
• RO4: Explore if there is an international consensus on the level of sensitivity of the personal data items (comparing Germany, the US, Brazil and the UK).
• RO5: Determine the impact of context/situation (specifically finance or health domains) on an individual's level of comfort in disclosinginformation.
• RO6: Determine the impact of interaction means (human or chatbot) while sharing personal information on individual's level of comfort in disclosing information.
• RO7: Determine the impact of anonymity (identified or anonymous) on individual's level of comfort in disclosing information.
Through this research, we contribute to the literature on information sensitivity and disclosure in three novel ways:
1. We provide insights into the factors that lead to certain information being considered sensitive and provide a UK perspective on these debates.
2. We provide sensitivity ratings of different data items for UK citizens and explore the international consensus on data sensitivity. Those findings can further help to inform discussions on the process of cross-national data flows.
3. We empirically investigate the impact of demographic characteristics, anonymity, context (health and finance), and interaction means (human or chatbot) on information sensitivity and comfort to provide information.
Our findings, therefore, can also contribute to an understanding of how to design inclusive information systems when sensitive disclosures are required. The assumption we make in this study is that comfort is inversely related to sensitivity; i.e., the more comfortable an individual is in sharing some personal information, the less sensitive that information is perceived to be, this is consistent with prior work (e.g., Ackerman et al., 1999).
The remainder of this paper is structured as follows. The Literature Review section summarizes the literature relevant to our research question. We present our methodology in the Research Methodology section and following this, we present our descriptive results in Results section. We critically reflect on and consider our findings in the Discussions section, as well as highlighting the implications for research and practice. The paper closes with a discussion of the limitations of the research and future plans.
2. Literature Review
This section summarizes the relevant literature underpinning this research in following four sub-categories.
2.1. What Makes Information Sensitive?
A fundamental challenge for protecting personal information is first defining how it can be conceptualized and categorized. While there are several different opinions in the literature about how sensitive personal information may be defined, regulatory frameworks can provide a robust foundation. The European General Data Protection Regulation (GDPR) considers personal data sensitive if it reveals a racial or ethnic origin, political opinions, religious or philosophical beliefs, trade union membership, data concerning health, sex life and sexual orientation. In addition to these data types, genetic data and biometric data also fall into this category. The GDPR covers those data items in a special category defined as “data that requires specific protection as the context of their processing could create significant risks to an individual's fundamental rights and freedoms” (European Parliament, 2016).
One notable study on sensitive information, Ohm (2014) aimed to understand what makes information sensitive and focused on a list of categories of information that have been legally treated as sensitive, primarily from the United States. This list of sensitive categories was then employed to infer the characteristics of information types that result in it being considered sensitive. In brief, four factors were reported when assessing whether a given piece of information seems sensitive: the possibility of harm, probability of harm, presence of a confidential relationship, and whether the risk reflects majoritarian concerns.
A schema has been proposed for assessing data categories to guide the relative sensitivities of different types of personal information (Rumbold and Pierscionek, 2018). The paper explores several factors that influence the perception of personal data as sensitive, including the public availability of data, the context of the data use and its potential to identify individuals. Contrary to popular belief, researchers stated that data publicly observable is not necessarily non-sensitive data (Rumbold and Pierscionek, 2018). The potential of certain information being used to infer new information when aggregated with others is another factor leading to a perception of sensitivity. Several other issues, such as the risk of re-identification, automated profiling, behavioral tracking and trustworthiness of the person/system with whom the data is shared, are also given as potential problems to affect sensitivity evaluation of particular information types. The massive increase in sensors associated the internet-of-things (IoT) devices (e.g., sensor data, or heart-rate data from wearable devices) within the medical domain has increased the amount of health data collected from citizens. This has raised the risk of third party data access such as health professionals or even insurance companies (Levallois-Barth and Zylberberg, 2017). Sharing data with third parties may increase the risk of discrimination and also make it possible to infer the prevalence of certain pathologies. Therefore, Levallois-Barth and Zylberberg (2017) claim that even though those data items may not be potentially sensitive when considered in isolation, sensitivity evaluations may change in the future. However, surprisingly, Kim et al. (2019) revealed that within healthcare, sensitivity has no statistically significant impact on the willingness to provide privacy information even though it significantly influences the perceived privacy risk. Those conflicting findings highlight some of the challenges in sensitivity evaluations and disclosure which will be explained further in Section 2.3.
Finally, the nature of the technology also has an impact on the sensitivity evaluations and data storage decisions accordingly. For instance, due to it's immutable nature which prevents data being changed, Kolan et al. (2020) argued that personal medical data should not be stored directly on public blockchain systems. This was confirmed by Zheng et al. (2018) who also preferred not to store health information in blockchain in their proposed solution. Based on that, it can be argued that the concerns regarding the use of data in the future shapes the sensitivity evaluations of personal data.
2.2. What Types of Information Are Perceived as Sensitive?
In addition to the studies that explore the factors leading individuals to perceive certain information as sensitive, studies have also categorized data types according to the perceived sensitivity.
In one of those studies researchers identified two clusters of information that were considered more sensitive: secure identifiers (e.g., social security number) and financial information (e.g., financial accounts and credit card numbers). It is noted that basic demographics (e.g., gender, birth date) and personal “preferences” (e.g., religion, political affiliation) were seen as less sensitive by the survey respondents (Milne et al., 2017).
Another study by Markos et al. (2017), used a cross-national survey between consumers in the United States and Brazil to explore the cultural differences in the perception of sensitivity. The authors examined 42 information items concluding that US consumers generally rated information as more sensitive and were less willing to provide information to others than their Brazilian counterparts. Financial information and identifiers were observed to have the highest perceived sensitivity with security codes and passwords, financial account numbers, credit card numbers, or formal identifiers such as social security number and driving license number appeared in a cluster of highly sensitive data.
A similar study has been conducted that provided a German citizen perspective on information sensitivity (Schomakers et al., 2019). Researchers compared their results with the results from the US and Brazil (Markos et al., 2017; Milne et al., 2017) and noted that, on average, the perceptions of information sensitivity of German citizens lies between that of US and Brazilian citizens. Cluster analysis revealed that similar data items were considered highly sensitive by the three countries except that German citizens considered the credit score to appear in a medium-sensitive cluster whilst US and Brazilian citizens considered this to be in a higher-sensitivity cluster. However, in general, German citizens were reported to perceive passwords as most sensitive, followed by identifiers such as financial account numbers, passport numbers or fingerprints.
In addition to those studies that focus on general items of information, some researchers focused on specific information domains. For example, Bansal et al. (2010) focused on health information and the role of individual differences on perceived information sensitivity and disclosure in this domain. Meanwhile, Ioannou et al. (2020) focused on travel providers and their customers' privacy concerns when sharing biometric and behavioral data and the impact of these concerns on the willingness to share this data. This study highlighted the context-dependence of privacy preferences. It is reported that although travelers worry about the privacy of their data, they are still willing to share their data, and the disclosure decision is dependent upon expected benefits rather than privacy concerns. Confirming the “privacy paradox” (Norberg et al., 2007), it was found that there was no link between privacy concerns and willingness to share biometric information and that expected benefits outweigh privacy concerns in the privacy decisions made by travelers.
Research has also examined attitudes toward sharing PII and non-PII (anonymous) data (Markos et al., 2018); they differentiated the information that was already public, hypothesizing that items associated with the “private-self” are perceived as more sensitive than public-self items. Their results demonstrated that some anonymous information like diary/journal entries, hygiene habits, home information, and GPS location are considered sensitive and even more sensitive than PII, conflicting slightly with the general societal interpretation and legislative focus. More expectedly, they identified that private-self information items were perceived as more sensitive than public-self items.
2.3. When Do We Disclose More?
There are multiple debates regarding personal information disclosure in the literature, some of which consider data sensitivity and other factors such as the perception of benefit. For instance, research has found that people are more willing to disclose when their human needs such as health or security are fulfilled (Wadle et al., 2019); thus, explaining the impact of expected benefits on information disclosure.
Conversely other research proposed that the perceived privacy risks play a more significant role than the expected benefits (Keith et al., 2013). The difference in their results was explained by the high degree of realism they provided in their experiments, where participants were given a real app that dynamically showed actual data.
In another recent study, perceived privacy risks were argued to significantly reduce the intention to disclose information and the disclosure behavior, whilst privacy concerns were reported to affect disclosure intention but not the actual information disclosure behavior (Yu et al., 2020).
The impact of personal differences has also been studied; for example, less healthy individuals were more concerned about disclosing their health information arguably due to the risk of their status on employment opportunities or social standing (Bansal et al., 2016). This finding confirms previous studies by Treiblmaier and Chong (2013) who demonstrated that a higher level of perceived risk leads to a lower level of willingness to disclose personal information. The same research examined the role of trust in information disclosure and reported that the direct influence of trust in the Internet (as a communication media) is statistically insignificant. However, the trust of an online vendor (the ultimate receiver of the information) impacts the willingness to disclose.
It has also been shown that the perceived fairness of a data request also impacts personal information disclosure (Malheiros et al., 2013). The “fairness” of a data request describes the individual's belief that data being collected will be used for the purpose communicated by the data receiver and in an ethical manner. The study revealed that when participants saw a disconnect between the disclosures they were asked to make and the specified purpose of the disclosure, they consider it unfair and opted not to disclose.
The impact of anonymity has also been studied in a recent study (Schomakers et al., 2020) that reported that the critical element of online privacy and privacy in data sharing is the protection of the identity, and thus, anonymity. The most substantial effect associated with data sharing was the anonymisation level, followed by the type of data (how sensitive it is) and how much the person with whom the information is shared is trusted. It was reported that when the participants can understand why the data is useful to the receiver, they are more willing to provide data. Benefits for the self or the society are also reported as important aspects while deciding to share data. It is clear that when it comes to PII, sensitivity plays a greater role in willingness to disclose than it has for anonymous information, i.e., information that is not personally identifiable (Markos et al., 2018).
2.4. How May Non-human Agents Impact Disclosure?
A chatbot is an application created to automate tasks and imitates a real conversation with a human in their natural language (whether spoken or through a textual interface). Today, conversational agents are used in various industries, including finance and health care. In these applications, the collection of personal information is essential to provide an effective service. Consequently, research has focused on disclosing information to chatbots and the modulating factors that enable or degrade disclosure. In one of those studies, it was concluded that users disclose as much to chatbots as they would to humans (Ho et al., 2018), resulting in similar disclosure processes and outcomes. The researchers added that relatively neutral questions might not make a difference between chatbots and humans, and when asked a question that may be embarrassing and might result in negative evaluation, users were also found to respond with more disclosure intimacy to a chatbot than a human.
Another study highlighted a similar issue and noted that individuals tended to talk more freely with a chatbot, without perceiving they were being judged or making the chatbot bored of listening to them (Bjaaland and Brandtzaeg, 2018). Accessibility and anonymity are given as other characteristics of chatbots that encourage self-disclosure. “Icebreaker questions” (e.g., “how are you doing?,” “how is the weather?”) or human-like fillers (e.g., “um,” “ahh”) are also reported to lead to more effective communication and a sense of a shared experience (Bhakta et al., 2014; Bell et al., 2019).
Other research has considered the importance of context and investigated the effects of socio-emotional features on the intention to use chatbots (Ng et al., 2020). While a preference for a technical and mechanical chatbot for financially sensitive information was identified, no significant differences were observed in the disclosure of socially attributed items (such as name, date-of-birth and address) between the chatbots with and without socio-emotional traits.
The lack of coherence in the scope of the studies that investigate the impact of employing chatbots on information disclosure has encouraged us to design this study. We systematically investigate the comfort in disclosing sensitive information to a chatbot, varying the context of the domain and the sensitivity levels of data items. We aim to present a rigorous and systematic understanding of the impact on information disclosures from conversational agents.
3. Research Methodology
In order to answer our research question and achieve the individual research objectives, a rigorous methodology was defined, this was oriented around an online questionnaire and robust qualitative and quantitative data analysis. The questionnaire engaged a sample of 500 British participants and critically explored the topic of information sensitivity. We opted for a questionnaire (e.g., instead of interviews or focus groups) to reach a census representative sample of UK citizens. The questionnaire design (i.e., questions asked, sequence of questions) and subsequent data analysis techniques were composed specifically to allow us to address each research objective, and address the research question. In what follows, we explain the questionnaire design, present the participant recruitment strategy, and detail the techniques used to analyse the data gathered.
3.1. Questionnaire Design
The questionnaire was implemented on the Survey Monkey platform, and participants were asked to respond to questions posed across five sections. First, we posed questions to collect informed consent from participants. In the second section, demographic characteristics of the participants (age group, gender, and educational level) were gathered. Having gathered this biographic information, the next sections were closely associated with the research objectives. The third section targeted RO1 specifically and therefore asked participants for the reasons or factors that might lead them to consider certain personal information more sensitive than other personal information. This was presented as an open-ended question to allow participants to present any factors they viewed appropriate.
The fourth section asked participants questions about the sensitivity of a range of personal data items. These questions provide the basis for achieving RO2 (i.e., exploring the levels of sensitivity of the different personal data items), RO3 (i.e., exploring the impact of user factors on sensitivity of the different personal data items) and RO4 [i.e., enabling a comparison of British citizens sensitivity perceptions with perceptions from citizens from the US, Brazil and Germany (Markos et al., 2017; Schomakers et al., 2019)].
To determine the data items for our study, we decided to use data items covered in existing studies as a basis and enrich those lists in accordance with our research objectives. Some of the original data items by Markos et al. (2017) and Schomakers et al. (2019) were not appropriate for our scenarios and therefore were eliminated, for example: DNA profile, fingerprint, digital signature or browsing history are not easily shared with chatbots due to their nature. We paid particular attention to the differences in the sensitivity classification of Schomakers et al. (2019) to that of Markos et al. (2017). We included the data items that were assigned different sensitivity levels between those two studies. We also expanded our list with data items considered sensitive by the GDPR or any data protection acts of EU countries, the US, China and the UK. These regulations were reviewed, and any data items that were identified as requiring extra controls or given as “special categories” were added to our list.
The complete list of data items is in Table 1. In order to better understand these data items within the context of the domains we considered (health and finance), these data items were manually categorized as either General data items, Health-related information, or Financial information.
To examine participants' opinions on the sensitivity of these 40 data items, participants were asked to rank each data item on a 6-point symmetric Likert scale which ranged from “not sensitive at all” (1) to “very sensitive” (6). Throughout the study, we used a 6-point scale as done by Schomakers et al. (2019) to enable a direct comparison between nationalities. A 6-point scale has also been shown to avoid overloading the participants' discrimination abilities (Lozano et al., 2008). For the fifth and final section of the questionnaire, a set of questions was posed to assess the effects of three variables, i.e., identification (anonymous or identified), context (finance or health) and interaction means (a human or chatbot), on the comfort in disclosing personal information (RO5-7); thus, was a 2 x 2 x 2 factorial design. Participants were asked to rate their comfort level while disclosing particular data items in each of the scenarios summarized below in Table 2. For example, in scenario 1 (S1) the question was given as follows: “Assume that you are speaking to a person on an online health service website where you do not need to identify yourself (i.e., you can be anonymous). How comfortable would you feel disclosing (i.e., sharing) the personal information listed below?.” Comfort levels were assessed again on a 6-point Likert scale ranging from 1 “Not comfortable at all” to 6 “Very comfortable.”
In order to reduce the possible overload of participants, two scenarios have been eliminated from the study. These would be S7 and S8 to complete the 2 x 2 x 2 design where participants would be asked to disclose personal information to a chatbot where they needed to identify themselves. When piloting the study, it became apparent that the quality of the responses was significantly reduced beyond six scenarios. This pragmatic decision allowed us to focus on the six scenarios which would supply the most value to practitioners.
To determine the data items to use for this final part of the questionnaire, we abridged the original list of data items and selected 20 items; ten were general data items, five were health related, and five were finance related. This abridging was another pragmatic choice to reduce the load on our participants whilst still delivering a solid evidence base for practitioners. While shortening the list, we retained data items that are frequently subject to debates in the literature. Personal identifiers, data items in the special category of the GDPR or personal information related to health and finance were maintained in this list for this reason (see Table 3).
We included six attention checking questions to ensure the quality of our data. The scenarios in the second step were randomized in the questionnaire software to avoid any sequence bias. The data items (i.e., the lists of 40 and 20 items) in the questions were also randomized for the same purposes. The study has been reviewed and ethically approved by the Research Ethics and Governance Department of University of Kent and Cranfield University Research Ethics Committee.
3.2. Participants
Participants were recruited using Prolific in order to reach a census representative sample of UK citizens. Since this study's ultimate goal is to understand UK citizens' perspective, it was essential to gather responses from a representative set of the public. This platform was also selected since it has good quality and reproducibility compared to other crowdsourcing platforms (Peer et al., 2017).
Before running our questionnaire, we conducted a pilot study with 50 participants to ensure that the questionnaire design and time limits were appropriate and usable for the intended/target audience. We then released the complete questionnaire on a sample of 500 participants (i.e., representative of the UK population based on age, sex and ethnicity), paying £8.72 per hour, which is at least the UK minimum wage. In total, the questionnaire took 15 min to complete.
From the 500 responses gathered, nine participants failed more than one attention question and thus were excluded from the data analysis. We present the demographics of the final 491 participants in Table 4.
3.3. Data Analysis
To analyse the data gathered, we used techniques most appropriate for the respective question set (see Figure 1). After collecting consent and demographic characteristics of the participants at the beginning of the questionnaire, in the first step, to achieve RO1 we asked reasons or factors that lead participants to consider certain personal information as more sensitive than other personal information. We used thematic analysis to analyse this qualitative data (Braun and Clarke, 2006). Firstly, brief labels (codes) were produced for each response, and when all data had been initially coded, themes were identified, grouping responses with similar codes into the same category. Finally, the themes were reviewed to check whether the candidate themes appeared to form a coherent pattern.
The analysis conducted to achieve RO2 was descriptive and we ordered the data items by computing their average sensitivity ratings. For RO3, we built proportional-odds logistic regression models for each data type to model the effects of age, gender and education. This modeling approach allows us to build a model that predicts a particular participant's probability of giving a data item a particular sensitivity rating based on their age, gender, and education level. By exploring these model coefficients, we can gain insight into the effects of these variables on how comfortable people are disclosing sensitive information.
To achieve RO4, we used hierarchical cluster analysis (Bridges, 1966) to group data types based on their perceived sensitivity. Initially, each data item is assigned to an individual cluster before iterating through the data items and at each stage merging the two most similar clusters, continuing until there is one remaining cluster. At each iteration, the distance between clusters is recalculated using the Lance-Williams dissimilarity (Murtagh and Contreras, 2012). This clustering allowed us to build a tree diagram where the data items viewed as being of similar sensitivity are placed on close together branches.
Finally, for Research Objectives 5 to 7 we used proportional-odds logistic regression modeling to analyse the effects of anonymity, context and interaction means, using these three variables to predict the comfort level while disclosing personal information.
4. Results
This section describes the results from both the open-ended qualitative question and the quantitative results from the Likert scale questions. Further discussion of the results is explored in Section 5.
4.1. RO1: Identification of Factors Leading British Citizens to Regard Certain Information Sensitive
As mentioned previously, we asked our participants an open-ended question regarding the factors that lead them to consider a data item to be sensitive. A thematic analysis of the responses led to several factors being identified. These included some of the factors reported in the literature, such as the risk of harm, trust of interaction means, public availability of data, context, and identification. However, we identified several other areas that have been overlooked or not dealt with comprehensively. These new themes included concerns regarding the reactions from the listener, concerns regarding personal safety or mental health, consequences of disclosure on beloved ones or careers, or concerns regarding sharing information about others such as family members or friends.
The complete set of themes and codes are presented in Table 5 with the number of responses related to each theme and code. These summaries provide a useful indicator of the themes emerging from the study and the popularity of each theme.
In the remainder of this section, we provide details of the most pertinent themes emerging from our study. The names of the themes and the codes under themes are written in italics.
4.1.1. Privacy Concerns
Privacy concerns expressed by the participants while evaluating the sensitivity level of information often focused on identity theft. In our study, 35 participants expressed their concerns in a finance context where credentials or some other identifiers were given as examples to sensitive personal information due to their potential exploitation for identity fraud. Identifiers or other information used to identify individuals when used together were also considered sensitive by several participants even if identity theft was not explicitly mentioned. For some participants, it was enough to consider a piece of personal information as sensitive if it could reveal their identity.
Another concern that emerged under the privacy theme was private information. Within this code, data items were reported to be considered more sensitive if the owners of them preferred to keep them private. Medical histories and financial status are mainly considered private and, hence sensitive by those participants. These participants also mentioned unsolicited emails, phone calls or customized advertisements as an effect of sharing information about themselves. A particular category under this privacy concern pertained to personal life where preferences in life, family information or relations with partners were considered sensitive by participants.
Interestingly, respondents found some publicly available information to be sensitive due to the potential use to access more information about the individuals. Again this was most notable when that new information was related to the health or financial status of the individuals. One poignant example in this category was the name of a pet or mother's maiden name, information commonly used for security or password questions.
Other emergent concerns included the fear of being physically traced; data items that would allow individuals to be traced were considered sensitive by a group of participants: “People being able to find where I live or work or steal my identity.,” “you can use it to track somebody, find out other information related to what you have …”.
The final code related to privacy violations was the risk of third-party sharing. Some participants considered personal information sensitive when they thought it might be shared with other groups and become more widely available than expected. This concern around third-party sharing is increasingly in line with the studies that argue that third-party access leads to privacy concerns (e.g., Pang et al., 2020).
4.1.2. Two Main Contexts of Sensitive Personal Information: Health and Finance
In addition to the themes that led participants to consider certain information as more sensitive, our analysis also identified two primary contexts that heavily dominated the responses; health and finance. Hence, it is possible to report a consensus on the sensitivity of the health and finance-related information. Participants noted that these data items were expected to be given a higher standard of protection by the systems that process them. Some responses exhibited concerns regarding health information being sold or passed to insurance companies or other bodies interested in this information. Conversely, some others worried about the impact of disclosing their health status on their financial creditworthiness or career. Some participants also found health-related information inherently very private and thus sensitive, without giving any consequence as a reason.
Finance is a significantly more common response to our question when compared with health data. Several participants provided finance-related personal information as an example of sensitive information. In addition, several other data items, outside of a finance context, were considered sensitive by participants due to their impact on participants' financial status. Even though financial loss dominates the responses, some other factors such as impacts on career and financial confidentiality also led participants to find information more sensitive.
4.1.3. Financial Problems
As discussed previously, financial concerns dominated the responses. Consequences under this theme center around financial loss, financial exposure, risk of fraud and negative impacts on career. The risk of fraud appeared to be the largest concern as many participants reported information to be more sensitive if it could enable fraudulent activities. More specific responses were given by some participants where financial loss was explicitly given as a concern while evaluating the sensitivity level of information. Financial exposure, which could be considered an overlapping area between the themes Privacy and Financial problems, was another code that emerged in the responses. Finally, when evaluating the sensitivity level, several participants reflected on the impacts on their career of disclosing financial information. Political and religious affiliations, and medical histories, were popular examples given as sensitive information that participants believed could compromise their careers or aspirations.
4.1.4. Concerns Regarding the Reactions of People
Another concern of participants observed was the interpersonal reactions between the individual sharing the information and the individual to whom the information was disclosed. Under this theme, the most common reaction was embarrassment with participants reporting that information that they found embarrassing to disclose was considered sensitive.
Medical records or being a member of protected characteristics were given as examples of sensitive information since they were considered embarrassing for themselves or their families. Similarly, discrimination was another code that emerged under this category. A group of participants reported a data item to be sensitive if they believed it would invoke the prejudice or bias of others. Religious or political affiliation, sexual orientation, race, disability or genetic defects and health information were examples given as sensitive due to this concern. Disclosure of personal health information has been known to result in discrimination by employers and insurance agencies if they gain access to such information (Rindfleisch, 1997).
Participants also reported finding information sensitive if it may cause them to be judged by others. In addition to judgement, reputational harm was another factor that led participants to consider a data item sensitive. We also identified cultural conditioning, which some participants highlighted as “taboo” subjects within society and considered items related to those taboos more sensitive (e.g., sex life, political leanings) purely because of this societal/cultural conditioning.
4.1.5. Consequences of Disclosure on the Individual
A majority of responses under this theme exhibited answers where participants defined sensitive information as the information that could be misused/used against them or cause them harm. Some participants provided more specific answers and negative effects on mental health and personal safety or feelings such as harassment and fear.
Personal security was one of the most popular responses with participants linking sensitivity to a resulting security risk. It was not possible to differentiate in the majority of the responses if the given concern was about the individuals' physical security or digital security (e.g., “I have concerns about security,” “Things which might compromise my security”). However, some responses implicitly covered it where participants gave “home address” or “bank account number” as examples. Risk of crime is another code in this category. Participants were aware that some personal details could be used fraudulently and considered those sensitive. It is worthy of note here that almost all the concerns given in this category were in a financial context.
There were very few responses where participants shared their concerns regarding legal issues. Those participants reported perceiving information as sensitive if used legally against them (e.g., “official bodies can use it to deny services.”). On the other hand, one participant explicitly reported considering the costs and benefits of disclosing information into account while evaluating its sensitivity.
4.1.6. Nature of the Information
Some participants reported data as more sensitive due to its very nature. For example, characteristics can be given as intimacy of data which are generally exemplified with sexual life or other information related to personal life. Participants found these data items sensitive due to their intimate nature. Another characteristic reported was the value of the data, which determines to what extent others can use it as it is disclosed. For instance, passwords or passport numbers were seen as more sensitive than social media data since they are perceived as having a higher impact if misused. The relevance is another code that emerged which defines the relevance of the information request in the given scenario. Fairness of the request was also given as a pertinent factor: “There are certain details I would not wish to share as I do not feel they are of relevance to the data handler.”
A small group of participants considered data items that are costly to change (e.g., home address) more sensitive than items where the cost is lower (e.g., email address). Another response, albeit relatively rare, was when the data item was related to a particular group identity. For example, information about minors or vulnerable groups were considered sensitive. Existing research reported that a particular data item might only be sensitive where the individual belongs to a group that often faces discrimination (Rumbold and Pierscionek, 2018). For example, gender at birth is likely to be less sensitive for those who are cisgender compared to those who are transgender.
Some participants also considered the public availability of information while evaluating the sensitivity of it and considered that data items that were already publicly known were less sensitive.
4.1.7. Interaction Means
Disregarding the content of the information, some participants reported another essential factor; the person/system that the information is shared with. We identified several participants for whom the sensitivity of information is related to the receiver of the information. For some participants, it was explicitly a matter of trust, a data item as more sensitive if they did not trust the person or the system to whom they are disclosing it.
4.1.8. Consequences of Disclosure on Others
In addition to the previous concerns associated with the personal consequences, several responses showed a more altruistic concern. They reported considering Consequences of disclosure on others while evaluating the sensitivity of data items. They expressed their concerns regarding the security and safety of their families or beloved ones. They perceived information sensitive that could cause a risk to the security and safety of others. We have combined the generic concerns under the code Impact on others where participants provided their concerns without explicitly defining the impact. Most of these respondents stated that they would not share any information that would put people they know in trouble and consider these data items sensitive.
4.2. RO2: Sensitivity Rankings of Various Data Items
Beyond the factors that are taken into account while assessing the sensitivity of the information, we asked participants to rate 40 data items on a 6-point symmetric Likert scale from “not sensitive at all” (1) to “very sensitive” (6).
The participants' ratings for each data item are displayed in Figure 2, the data items are ordered by the average rating. Our results showed that passwords represented the most sensitive data type for UK citizens, with 92% of participants giving it the highest rating, followed by bank account credentials and credit card number, with 87 and 83% of respondents giving it the highest rating. The following items are formally identifiable information, namely national ID number and passport number, which match the concerns given regarding identity from the first part of the questionnaire. The least sensitive items were hair color, gender and height, which are typically observable human characteristics.
4.3. RO3: Influence of User Factors
In order to examine the influence of user factors (age group, gender, education) on the perception of sensitivity, we built a proportional odds logistic regression model for each data type. We identified those data items which demonstrated a sensitivity that had a statistically significant effect (using a p-value less than 0.05) from one of these factors.
The gender of the respondents was a modulating factor on the perception of the sensitivity of an income level, with female respondents typically considering the sensitivity higher than male participants, (see Figure 3). This was also true for IP address, criminal records, weight and sexually transmitted disease. Conversely, male participants considered smoking habits and the number of children to be more sensitive than female participants.
The data items on which education has significant impact are current location, political affiliation and sex life. The level of education led to the sensitivity being perceived as higher for political affiliation. Education also modulated the perceived sensitivity of the current location with those who left education before achieving a post-16 qualification identifying a significantly lower sensitivity, also seen in the sensitivity of the sex life data item. Note, this analysis controlled for the age variable, so this is not an artifact from age measures.
The respondents' age was also observed to have significant effects on perceived sensitivity. The Credit score was considered significantly less sensitive by the majority of the participants aged between 18 and 24. This age group also tends to consider date of birth, email address and mother's maiden name less sensitive when compared to other older groups. Looking across these final three data items with factors that have a relationship with age, there tends to be an increase in sensitivity with age until the 45–54 age group before decreasing in the 55 plus age group.
4.4. RO4: Exploring Cultural Differences via Cluster Analysis
We conducted a cluster analysis on the sensitivity of the data items as done by Markos et al. (2017) and Schomakers et al. (2019). However, we used hierarchical clustering in order to gain a high-fidelity understanding of the relationship between data items; the result is shown in Figure 4. Using a silhouette analysis, we found four clusters to be the most appropriate for our data set. Each cluster was cross-referenced with the ranking in Figure 2 to label the four clusters of data categories (very highly, highly, medium and low sensitive) as shown in Table 6. Previous work heuristically categorized data items into three groups as highly, medium and less sensitive. However, our empirical clustering result differentiated a small group of data types from the other highly sensitive data. We grouped those items under the title of “Very highly sensitive data” in our categorization.
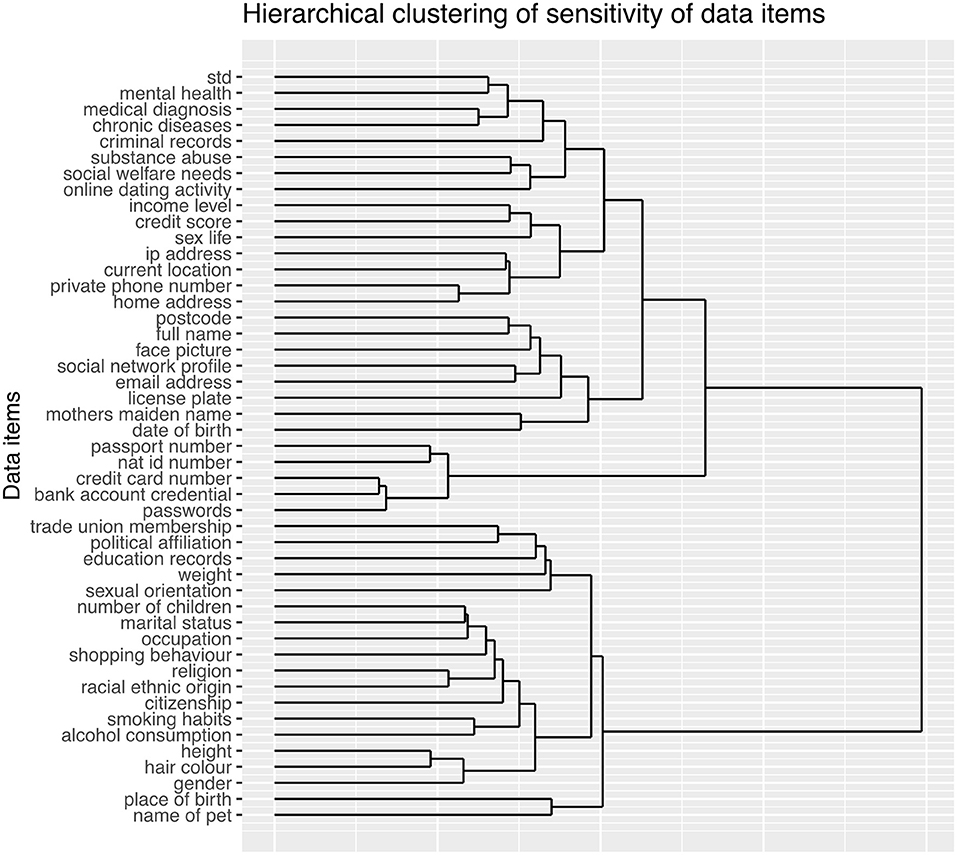
Figure 4. GCSEs are the qualifications taken in years 10 and 11 of secondary school in the UK (typically at the age of 14–16). A-levels are a subject-based qualification offered by the educational bodies in the UK to students completing secondary or pre-university education.
When previous research compared international measures of data sensitivity (Schomakers et al., 2019) it was reported that there was only one difference regarding the high sensitivity data category when they compared their results with Markos et al. (2017), which largely revealed a consensus between three countries (US, Brazilian and Germany) in this category. We see similar results with data types considered highly sensitive by those countries also appeared in the same category (or in the “Very highly sensitive data” category) in our study. In our study, several additional items appeared in this category, notably Income level, current location, private phone number, and home address were considered highly sensitive. In contrast, they belonged to medium or even low sensitive data in the German, Brazilian and US data sets. In our study, the categorization for Credit score was the same with the Brazilian and US data set, which differs from the medium sensitivity given by German citizens.
Among the items UK citizens placed in a medium sensitive data category, five items (mother's maiden name, license plate number, email address, social network profile, face picture and post code) were in the low sensitivity data types for German citizens. However, mother's maiden name, social network profile and face picture were medium sensitive not only for UK citizen but also for US and Brazilian citizens. The vehicle license plate number appeared in the medium category in our results yet was considered highly sensitive by US and Brazilian citizens and low by German citizens. The categorization of the postcode and email address was identical across all nationalities.
It is possible to report an international consensus on the low sensitive data items. Almost all data types in this category in our study were ranked into the same category as previous studies. The only difference is sexual orientation which was given a medium sensitive by German citizens.
4.5. RO5: Impact of the Context on Information Disclosure
The initial analysis focusing on the relationship between context and comfort in disclosing information is largely in agreement with the literature. The size of the effects is the largest seen in the study. The analysis of the data items across all scenarios is shown in Figure 5. In this figure, a positive model effect shows participants being more comfortable disclosing in a health context and a negative model effect showing participants being more comfortable disclosing in a finance context.
There is a clear separation between the information domain and the disclosure domain, with all finance information showing negative model effects (more comfort in disclosing within a finance domain); however, there are noteworthy data items with smaller effects. There was a statistically significant effect on ethnic origin and religion where participants were more comfortable disclosing this within a health context than in the finance context. Also of interest is the small but significant effect on disclosing a criminal record; participants were more comfortable disclosing in the finance domain. However, this could be related to regulations surrounding the requirement for accurate disclosure of information in such cases.
Following a similar analysis to the previous section, we considered the pairwise comparison between scenarios S1 and S2, S3 and S4, and S5 and S6 (from Table 2). This results in the measures of the effect of the domain in three different scenarios: disclosing anonymously to a chatbot, disclosing anonymously to a human, and disclosing non-anonymously to a human. The effect of domain across the data items is shown in Figure 6.
This scenario-centered analysis clearly shows the strength of the domain effect. The domain effect is common throughout all interaction means and degrees of anonymity. An analysis of the models shows no data items where this domain effect is modulated by interaction or anonymity, and there seems to be no mechanism to significantly override or reduce this effect.
4.6. RO6: Impact of Interaction Means on Information Disclosure
The sixth research objective focused on the interaction means that elicited the disclosure; the model coefficients from the analysis of each data item are shown in Figure 7. Nearly two-thirds of the data items show a positive model coefficient (at a 95% confidence), indicating participants were more comfortable disclosing to a human than a chatbot. There were no data items that participants preferred to disclose to machines rather than humans. There was no effect from any of the biographic measures (such as age, gender and education).
Using the same modeling approach, we compared the impacts of interaction while disclosing personal information in health and finance anonymously. To achieve this, we paired the data from scenarios S1 and S5 and scenarios S2 and S6 (shown in Table 2). We then created a multinomial logistic regression to predict the perceptions of the sensitivity of a data item as a function of the interaction means (chatbot or human). The model coefficients are shown in Figure 8, with a positive effect being related to more comfort in disclosing to a human than to a chatbot (the error bounds represent the 95% confidence limit).
From these results, we observe that participants felt more comfortable disclosing sensitive information to humans, particularly in the health context. Sexually transmitted diseases, sex life, mental health, medical diagnosis or chronic diseases are data items that were preferred to be disclosed to a human by our participants. However, we can interpret this as preferring to talk to real people rather than chatbots when they need empathy and rapport in the dyadic.
Within the finance domain, only the credit score and income level data items showed a significant effect (with a 95% confidence) with interaction means. We can argue that using a chatbot will have a more negligible effect on the disclosures we would expect to be made within the finance domain.
4.7. RO7: Impact of Anonymity on Information Disclosure
This analysis considered the effect of anonymity on the disclosure of sensitive information. The logistic regression model coefficients are shown in Figure 9. A positive model effect related to greater comfort in disclosing when non-anonymous (i.e., the individual is identified) and a negative model coefficient demonstrates greater comfort in disclosing when the participant was anonymous.
The effect of anonymity is much smaller than other factors in this analysis. However, it does provide statistically significant effects for several data items, most notably sex life and sexually transmitted disease. Interestingly, this also includes political affiliation and alcohol consumption.
Two data items that showed a positive model effect (more comfortable in disclosing when done non-anonymously) were the mother's maiden name (something intuitively related to identity) and bank account credentials.
Considering the scenario-specific evaluation, we paired scenarios S1 and S3, and S2 and S4 to identify the effect of anonymity within the two contexts when disclosing to a human. The model effect is shown in Figure 10 with a positive model coefficient being related to more comfort in disclosing when identified a negative effect coming from more comfort in disclosing when anonymous.
From these results, we can see a small effect from anonymity across the two scenarios. Within the health domain, there is a small effect associated with the sex life data item, but broadly there are very few significant effects associated with this domain. When considering the finance domain in Figure 10 there are minor effects associated with some data items noted in the previous broader analysis. There is also a small negative effect associated with the disclosures associated with sex life in the finance domain; however, this is an out of domain disclosure whilst significant, this is likely to be an unusual disclosure.
5. Discussion
In this section, we summarize and discuss our key findings for each research objective outlined previously. Furthermore, we consider the novelty of this work as compared to existing research in the field.
5.1. The Factors That Make Information Sensitive for UK Citizens (RO1)
The first research objective was to investigate the primary factors that lead British citizens to regard information as sensitive. Our findings demonstrate that there are three key general topics of note; concerns about the potential consequences of disclosure (this relates to themes privacy, financial problems, reactions, consequences of disclosure on me, consequences of disclosure on others), the fundamental nature of the information (themes context, nature of information), and concerns regarding the person/system the information is shared with (theme interaction means).
For those with privacy concerns, the main code identified was identity theft. Identity theft, the act of obtaining sensitive information about another person without their knowledge, and using this information to commit theft or fraud, is estimated to cost the UK around £190 billion every year (National Crime Agency, 2021). CIFAS, a UK-based Fraud Prevention Services, stated that in 2019, more than 364,000 cases of fraudulent conduct were recorded on their National Fraud Database with an increase of 13 per cent compared to 2018 (CIFAS, 2019). It is promising to observe the degree of awareness of this risk within the UK population; acknowledging that awareness is only the first step to prevention.
In addition, we identified several participants' decision-making was related to financial implications, with concerns regarding financial loss being one of the significant codes that emerged from the qualitative analysis. Those findings are reinforced by the items which received the highest sensitivity ratings in the quantitative phase of the study. The bank account credential, credit card number appeared in the top three most sensitive items (see Figure 2). They also confirm prior study which reported the possibility of harm as one of the main factors considered when assessing sensitivity (Ohm, 2014).
Our results also uniquely highlight another concern that is generally overlooked by the privacy studies or regulations: disclosure of information belonging to others and impacts on personal information disclosure on others. Responses revealed that some participants consider information sensitive if this information belongs to others. Personal information studies in the literature are generally self-disclosure studies where the information is assumed to belong to the participant. It is also the same for the sensitivity studies where the owner of the information is assumed to be the person whose opinion or behavior is observed. Our analysis identifies concerns regarding both data belonging to others and the effect of information disclosure on others, particularly the potential harms to others. This observation indicates a societal maturity in identifying the second-order effects of disclosure.
As seen in Figure 2, personal data items categorized in a special category by the GDPR were not identified as being sensitive by our participants. We can identify the sensitivity of political affiliation, sexual orientation and trade-union membership as similar and not regarded as very sensitive; for example, a similar ranking was exhibited by weight and a much lower ranking than, for instance, income level or credit score. More interestingly, religion and ethnic origin were considered a very low sensitivity similar levels as marital status or occupation. Here it is worthy of note that, as mentioned before, this research aims to provide a British perspective on information sensitivity. It is well-understood that the perceived sensitivity of a particular type of data varies widely, both between societies or ethnic groups and within those groups (Rumbold and Pierscionek, 2018). The agency individuals have to protect their data, and hence the vulnerability of the individuals data affect the perceived sensitivity. Some of the data items categorized as special category by the GDPR (e.g., racial or ethnic origin or religion) may well have attracted higher sensitivity rankings if this study was constrained to minority ethnic groups rather than the general public.
5.2. Influences of User Factors on Perceived Sensitivity (RO2)
Our study also allowed us to identify variability in the perceptions of the sensitivity of data items based on the data subjects biographic information. For example, when we considered the age of the data subject, we found several interesting effects. Our findings are partially consistent with the literature that generally report that younger age groups share more information and are less concerned about information privacy, e.g., Miltgen and Peyrat-Guillard (2014) and Van den Broeck et al. (2015). It is also consistent with the literature that privacy is the most common barrier for older people to use smart technologies (Harris et al., 2022).
We can enrich those findings with fine-grained data items; for example, “credit score” was ranked less sensitive by those under 25. We hypothesize that this is because this group do not normally require high credit levels (for example, purchasing a house) and hence are unlikely to be discriminated against based on that level. The same can be said of date-of-birth, which steadily becomes more sensitive during working age until retirement when it becomes less sensitive. Again there is a clear parallel with discrimination within the workplace. We believe that our detailed findings can help develop individually tailored information collection systems that recognize and respect different privacy concerns among different demographics groups.
The final two data items that show an effect with age are email address and mother's maiden name, both of which show a low sensitivity for 18–24 years with a higher level across the other age groups but with a peak in the 45–54 cohort. The reduced level of sensitivity associated with young people can be explained by the peak in the group representing Xennials or late Gen X who had an analog childhood but digital adulthood and have retained some of the understanding of the formative years of digital life. Older participants potentially have come to digital life when the internet and digital socialization norms are more formed rather than growing up alongside the transformation.
When it comes to the impact of education levels on perceived information sensitivity, we found several conflicting findings in the literature. While there are studies that claim that individuals with lower educational levels tend to be less concerned about their personal information, e.g., Rainie et al. (2013) and Blank et al. (2014), there are also those which report no differences in privacy concerns depending on education levels (Li, 2011). Our study highlights that differences in the perception of sensitivity based on education are only prevalent regarding some information types (e.g., current location, political affiliation and sex life). Within the education level, there does appear to be a breakpoint between those who achieved post-16 education, most notably in location and sex life; note this has been controlled for participant age.
The final biographic element we explored was the effect of gender on perceptions of sensitivity. Gender provided the largest number of data items that were modulated by this factor. Our study identified an apparent social stigma that female participants felt when disclosing criminal records, sexually transmitted diseases, and weight. We can also explain the higher perceived sensitivity rating of income level in female participants by cultural factors, which can be different in a more patriarchal society. Even though the UK is one of the countries where the lowest levels of legal discrimination are measured against women (Georgetown University's Institute for Women, Peace and Security, 2020) there is still a disconnect between the genders in terms of pay, and it naturally follows that there is a difference in the perceived sensitivity.
Our results appear to support (Knijnenburg et al., 2013) who hypothesized that information disclosure behaviors consisted of multiple related dimensions and disclosure behaviors do not differ among groups overall, but rather in their disclosure tendencies per type of information. The results are also consistent with the results from RQ1.
5.3. UK Perspective on the Sensitivity of the Different Data Items and Identification of Cultural Differences (RO3 and RO4)
Our results confirmed the consensus on the high perceived sensitivity of the finance-related information and identifiers, which appeared in the same category as Markos et al. (2017) and Schomakers et al. (2019). When we reflect on the least sensitive items (hair color, gender, height), the common feature is that they are typically visible to the public. These appear consistent with the hypothesis from Markos et al. (2018) who predicted that public information is considered less sensitive compared to private-self information (inner states, personal history, and specific features of the self).
We conclude a degree of consensus on what constitutes sensitivity across German, US, Brazilian and UK citizens. However, respondents in our study and our rigorous empirical approach identified several “very” highly sensitive data items that formed a discrete cluster above those seen in the other studies. We also saw several elements promoted to the high-sensitivity cluster (e.g., income level, private phone number) compared to other nations, even compared to another western European country. This discontinuity shows that whilst international regulatory frameworks are undoubtedly essential to provide a degree of data protection, we must also have mechanisms to support the cultural differences within individual nations. Considering the internationalized nature of today's information society, we believe that such findings are important to consider while designing information systems that allow trans-border data flows, or for those systems designed and built in a different socio-economic environment to which they will be deployed.
5.4. Impact of the Context on Information Disclosure (RO5)
Our fifth Research Objective focused on the effect of context on the comfort of disclosing information. Our results broadly align with the literature; however, we highlight the magnitude of this effect; the strength of this effect is nearly ten times greater than any other identified in the study. Figure 5 clearly shows that health-related information is shared with significantly more comfort in a health context. Similarly, the finance-related information is shared more comfortably in a finance context. Also interesting were the data items related to religion and ethnic origin, which exhibited significant preferences for disclosure in the medical domain. It is conceivable that ethnic origin may result in a predisposition to certain illnesses (Cooper, 2004) and justifies a disclosure in the health domain; it is unlikely that the same is true in the financial domain. The effect of context is also not mediated by the scenario and appears to be consistent whether disclosing anonymously to a human or a chatbot or disclosing non-anonymously to a human; this is shown in Figure 6. These findings confirm the impact of relevance on the perceived sensitivity. From a regulatory perspective, this could be interpreted as a clear validation of the data minimization principle of the GDPR, which requires data collection to be adequate and limited to what is necessary.
5.5. Interaction Means and Comfort to Disclose (RO6)
Our penultimate research objective (RO6) focused on the interaction means whether the disclosure was direct to a human or through a chatbot mediated communication. In general, we found participants were more comfortable disclosing directly to a human rather than a chatbot; this was particularly the case with medical diagnosis, chronic diseases and mental health issues, shown in Figure 7. This preference for face-to-face human reporting has been seen in many sensitive domains, for example, within community reporting associated with violent extremism (Thomas et al., 2020). In these cases, it is very often difficult for the individual to make the disclosure. The natural interaction between humans and the perception of control is essential to support and enable these disclosures.
When this interaction means is considered in the scenario-specific conditions, we see a slightly more complicated picture. Within the health-based scenario, our participants still prefer disclosing to a human over a chatbot. Again the locus of control and the perception of engaged feedback may encourage participants to be more comfortable disclosing to a human. The other data item that showed a preference was occupation. Those findings contradict with the literature where users were reported to prefer chatbots or to respond with more disclosure intimacy to chatbots than a human (Bjaaland and Brandtzaeg, 2018; Ho et al., 2018). We can hypothesize at this point that within a healthcare setting, the perception of discussing and enriching the disclosure and providing more background as to the day-to-day tasks may drive this preference. When we consider the finance scenario, we generally see little difference between disclosure to a human or a chatbot. An indication that sensitive disclosures in this domain are less likely to be reduced through the use of conversational agents. The only data items that showed a significant effect were the credit score and income level; similarly to the occupation data item within the healthcare setting, we believe that this is a disclosure that the participant may view as requiring more enrichment or explanation. Hence, a factual disclosure with no interaction or feedback may be perceived as less desirable, leading to a perception of more comfort in disclosing to a human.
5.6. Anonymity and Comfort to Disclose (RO7)
The final research objective (RO7) focused on the effect of anonymity on the person making the disclosure. When considered abstractly, it was clear that several data items demonstrated a preference for anonymous disclosure, such as sex life and sexually transmitted diseases and alcohol consumption and political affiliation, which is inline with the previous findings (Schomakers et al., 2019). This observation would appear to match well to the qualitative results as well, which suggested that the reaction of others was an important element when judging whether items were sensitive or not.
As with the previous research objective, when this is contextualized within a real scenario, the results are more nuanced. We can see from Figure 10 that there is no preference for anonymity within the healthcare setting—nearly all data items showed no significant difference in the comfort with being anonymous or identified. We have already demonstrated the strength of the context in the sensitivity of disclosures. We would suggest that the healthcare context and the professional reputation of the National Health Service in the UK lead to participants seeing no value in being anonymous. The only data item that showed a preference for anonymous disclosure was associated with sex life, which was only just significant at the 95% level.
When considering the finance domain, several preferences for anonymity were observed; these were mostly tied to disclosures related to health, although these effects are minor and only just significant. Hence it is difficult to draw a meaningful conclusion from this domain; however, it may hint that when disclosures are made out of domain, individuals may be more comfortable disclosing if anonymous.
6. Conclusion
This final section draws together our research contributions from our rigorous analytical study of this challenging problem.
6.1. Theoretical Contributions
Our study presents a detailed capture of the perspective of UK citizens regarding the sensitivity of personal information. Three main factors lead British citizens to assign higher sensitivity scores to data items; consequences of disclosure, nature of the information and the concerns regarding with whom the person/system the information is shared. Identity theft and financial loss are the main concerns of the individuals, which is consistent with the risk-based definition of sensitive personal information in regulatory documents. In addition, high sensitivity scores assigned to health and financially related information indicate that there is a consensus on what constitutes sensitivity across German, the US, Brazilian and the UK. However, British citizens regard some items as highly sensitive as compared to the other three countries. These discrepancies highlight the challenge of providing trans-national regulation and should be noted by those managing information security where data flows cross regulatory borders.
We also identified individual characteristics that modulate perceptions of sensitive data. We identified age, gender and education level as influencing the sensitivity of particular data items; these modulating characteristics mapped well to the qualitative explanations of the factors that made data items sensitive.
The context or the fairness of the request has the most significant impact on the comfort level felt while disclosing personal information. Disclosure of highly sensitive personal information such as sex life, sexually transmitted disease or alcohol consumption was observed to be affected by anonymity. Participants reported disclosing those items with significantly more comfort when they do not have to reveal their identities.
This study has developed a systematic understanding of UK citizens' perceptions of sensitive information, showing a degree of consensus with previous studies and some unique insights. We particularly note the effect of the relevance of the disclosure and the effect of the interaction means, whether a human-mediated disclosure or a disclosure mediated by a conversational agent. In general, we highlighted the preference to disclose sensitive personal information to a human rather than a conversational agent. These findings should be considered in the design and management of information within systems that involve sensitive disclosures and hence sensitive data, particularly in the healthcare domain, where our findings are most significant.
6.2. Managerial Contributions
We contribute to the literature by investigating the impact of emerging technologies, particularly conversational agents (or chatbots), on the disclosure of personal data. Such disclose is a key security concern for both those disclosing their data and for organizations seeking to facilitate accurate, high-integrity disclosures. Despite the existence of studies that show the facilitator role of chatbots on information disclosure, no study, to our knowledge, has evaluated the perceived sensitivity of data items at granular level when they are disclosed to a chatbot. We also consequently identify the contexts where chatbots can enable individuals to disclose sensitive information more comfortably. In addition to providing general insights into how persons in the UK perceive sensitive information, our findings can contribute to the design of chatbots; most notably, defining an evidence-base to support agent use in the most appropriate usage contexts increasing the comfort of disclosing and ultimately ensuring more accurate responses. We specifically investigate two main contexts in our research; health and finance. These contexts have a regulatory demand for high levels of security and data protection, and are traditionally where chatbots are heavily adopted and sensitive personal information is frequently collected and processed (Stiefel, 2018; Ng et al., 2020). Our findings help demonstrate the relationship between the disclosed personal information and the context in which it is disclosed, ultimately uncovering the impact of usage context on disclosure of different data items. Finally, we explore the effect of anonymity, specifically identifying what personal data the UK public prefer to disclose anonymously. These observations provide novel insights for the information collection systems used in the UK by uncovering the factors that lead to perceptions of high sensitivity and hence the comfort (or discomfort) in the disclosure process.
6.3. Limitations and Future Work
While we believe our study was robust and has made several substantial contributions to the research, some limitations must be acknowledged. Firstly, our results represent self-reported sensitivity evaluations and may not reflect the lived behaviors of our participants. However, this approach allowed us to obtain and compare several sensitivity evaluations across several contexts. It also compares well with previous works in the field (e.g., Markos et al., 2017; Schomakers et al., 2019), which followed a similar methodological approach. However, we are aware that it might be possible to collect more accurate results when the participants assess their comfort levels while practicing the given scenarios.
Consequently, to validate our findings, our next step will explore the disclosure behaviors in an experimental context involving both human and chatbot mediated disclosures. Another issue faced in this study is the vagueness regarding the benefits of the disclosure and the perceived risk/trust to the interaction means. In our experimental approach, we intend to ensure a clear and consistent perception of the benefit of disclosure.
We also removed two scenarios from our 2 x 2 x 2 study; this meant that we could not fully explore all combinations of factors. However, this pragmatic decision has significantly improved the quality of the results and allowed us to draw some robust conclusions from the remaining six scenarios. Future work could consider the value in exploring all scenarios and thereby fully understanding all factors.
Data Availability Statement
The raw data supporting the conclusions of this article will be made available by the authors, without undue reservation.
Ethics Statement
The studies involving human participants were reviewed and approved by Research Ethics Governance Department of University of Kent and Cranfield University Research Ethics Committee. The patients/participants provided their written informed consent to participate in this study.
Author Contributions
RB-S, JN, and DH contributed to conception and design of the study and wrote sections of the manuscript. RB-S and JN collected the data. DH and RB-S performed the statistical analysis. RB-S wrote the first draft of the manuscript. All authors contributed to manuscript revision, read, and approved the submitted version.
Funding
This research was conducted as a part of a UK EPSRC-funded project, A Platform for Responsive Conversational Agents to Enhance Engagement and Disclosure (PRoCEED) (Grant Nos: EP/S027297/1 and EP/S027211/1).
Conflict of Interest
The authors declare that the research was conducted in the absence of any commercial or financial relationships that could be construed as a potential conflict of interest.
Publisher's Note
All claims expressed in this article are solely those of the authors and do not necessarily represent those of their affiliated organizations, or those of the publisher, the editors and the reviewers. Any product that may be evaluated in this article, or claim that may be made by its manufacturer, is not guaranteed or endorsed by the publisher.
References
Ackerman, M. S., Cranor, L. F., and Reagle, J. (1999). “Privacy in e-commerce: examining user scenarios and privacy preferences,” in Proceedings of the 1st ACM Conference on Electronic Commerce. (Denver, CO: ACM), 1–8.
Aiello, G., Donvito, R., Acuti, D., Grazzini, L., Mazzoli, V., Vannucci, V., et al. (2020). Customers' willingness to disclose personal information throughout the customer purchase journey in retailing: the role of perceived warmth. J. Retail. 96, 490–506. doi: 10.1016/j.jretai.2020.07.001
Bansal, G., Fatemeh,Zahedi, M., and Gefen, D. (2010). The impact of personal dispositions on information sensitivity, privacy concern and trust in disclosing health information online. Decis. Support Syst. 49, 138–150. doi: 10.1016/j.dss.2010.01.010
Bansal, G., Zahedi, F. M., and Gefen, D. (2016). Do context and personality matter? trust and privacy concerns in disclosing private information online. Inf. Manag. 53, 1–21. doi: 10.1016/j.im.2015.08.001
Belen Sağlam, R., and Nurse, J. R. C. (2020). “Is your chatbot GDPR compliant? Open issues in agent design,” in Proceedings of the 2nd Conference on Conversational User Interfaces. (Bilbao), 1–3.
Belen Sağlam, R., Nurse, J. R. C., and Hodges, D. (2022). Personal information: perceptions, types and evolution. J. Inf. Security Appl. 66, 103163. doi: 10.1016/j.jisa.2022.103163
Bell, S., Wood, C., and Sarkar, A. (2019). “Perceptions of chatbots in therapy,” in Extended Abstracts of the 2019 CHI Conference on Human Factors in Computing Systems. (Glasgow), 1–6.
Bhakta, R., Savin-Baden, M., and Tombs, G. (2014). “Sharing secrets with robots?” in EdMedia + Innovate Learning. (Waynesville, NC: Association for the Advancement of Computing in Education), 2295–2301.
Bjaaland, M., and Brandtzaeg, P. (2018). Youth and News in a Digital Media Environment, Chapter Chatbots as a New User Interface for Providing Health Information to Young People. Novi, MI: Nordicom.
Blank, G., Bolsover, G., and Dubois, E. (2014). A new privacy paradox: young people and privacy on social network sites. Prepared Ann. Meet. Am. Sociol. Assoc. 17, 1–35. doi: 10.2139/ssrn.2479938
Braun, V., and Clarke, V. (2006). Using thematic analysis in psychology. Qual. Res. Psychol. 3, 77–101. doi: 10.1191/1478088706qp063oa
Bridges Jr, C. C. (1966). Hierarchical cluster analysis. Psychol. Rep. 18, 851–854. doi: 10.2466/pr0.1966.18.3.851
CIFAS (2019). Annual Report 2019. Available online at: https://www.cifas.org.uk/about-cifas/annual-reports/annual-report-2019.
Cooper, R. S. (2004). Genetic factors in ethnic disparities in health. Crit. Perspect. Racial Ethnic Disparities Late Life 267, 269–309. Available online at: https://europepmc.org/books/n/nap11086/a2000af96ddd00182/?extid=20669464&src=med&fid=a2000af96ddd00196
European Parliament (2016). Regulation (EU) (2016) 2016/679 of the European Parliament and of the Council of 27 April on the protection of natural persons with regard to the processing of personal data and on the free movement of such data, and repealing Directive 95/46/EC (General Data Protection Regulation). Official Journal of the European Union.
Georgetown University's Institute for Women, Peace and Security (2020). Women Peace and Security Index 2019/20. Available online at: https://giwps.georgetown.edu/wp-content/uploads/2019/12/WPS-Index-2019-20-Report.pdf.
Harris, M. T., Rogers, W. A., and Blocker, K. A. (2022). Older adults and smart technology: facilitators and barriers to use. Front. Comput. Sci. 41, 835927. doi: 10.3389/fcomp.2022.835927
Ho, A., Hancock, J., and Miner, A. S. (2018). Psychological, relational, and emotional effects of self-disclosure after conversations with a chatbot. J. Commun. 68, 712–733. doi: 10.1093/joc/jqy026
Ioannou, A., Tussyadiah, I., and Lu, Y. (2020). Privacy concerns and disclosure of biometric and behavioral data for travel. Int. J. Inf. Manag. 54, 102122. doi: 10.1016/j.ijinfomgt.2020.102122
Keith, M. J., Thompson, S. C., Hale, J., Lowry, P. B., and Greer, C. (2013). Information disclosure on mobile devices: re-examining privacy calculus with actual user behavior. Int. J. Hum. Comput. Stud. 71, 1163–1173. doi: 10.1016/j.ijhcs.2013.08.016
Kim, D., Park, K., Park, Y., and Ahn, J.-H. (2019). Willingness to provide personal information: perspective of privacy calculus in iot services. Comput. Hum. Behav. 92, 273–281. doi: 10.1016/j.chb.2018.11.022
Knijnenburg, B. P., Kobsa, A., and Jin, H. (2013). Dimensionality of information disclosure behavior. Int. J. Hum. Comput. Stud. 71, 1144–1162. doi: 10.1016/j.ijhcs.2013.06.003
Kolan, A., Tjoa, S., and Kieseberg, P. (2020). “Medical blockchains and privacy in Austria - technical and legal aspects,” in Proceedings of the 2020 International Conference on Software Security and Assurance (Altoona, PA: IEEE), 1–9.
Levallois-Barth, C., and Zylberberg, H. (2017). “A purpose-based taxonomy for better governance of personal data in the internet of things era: the example of wellness data,” in Data Protection and Privacy:(In) visibilities and Infrastructures (Cham: Springer), 139–161.
Li, Y. (2011). Empirical studies on online information privacy concerns: Literature review and an integrative framework. Commun. Assoc. Inf. Syst. 28, 28. doi: 10.17705/1CAIS.02828
Lozano, L. M., Garcia-Cueto, E., and Mu niz, J. (2008). Effect of the number of response categories on the reliability and validity of rating scales. Methodology 4, 73–79. doi: 10.1027/1614-2241.4.2.73
Malheiros, M., Preibusch, S., and Sasse, M. A. (2013). “‘Fairly truthful': the impact of perceived effort, fairness, relevance, and sensitivity on personal data disclosure,” in International Conference on Trust and Trustworthy Computing (London: Springer), 250–266.
Markos, E., Labrecque, L. I., and Milne, G. R. (2018). A new information lens: The self-concept and exchange context as a means to understand information sensitivity of anonymous and personal identifying information. J. Interact. Mark. 42, 46–62. doi: 10.1016/j.intmar.2018.01.004
Markos, E., Milne, G. R., and Peltier, J. W. (2017). Information sensitivity and willingness to provide continua: a comparative privacy study of the united states and brazil. J. Public Policy Mark. 36, 79–96. doi: 10.1509/jppm.15.159
Milne, G. R., Pettinico, G., Hajjat, F. M., and Markos, E. (2017). Information sensitivity typology: mapping the degree and type of risk consumers perceive in personal data sharing. J. Consum. Affairs 51, 133–161. doi: 10.1111/joca.12111
Miltgen, C. L., and Peyrat-Guillard, D. (2014). Cultural and generational influences on privacy concerns: a qualitative study in seven european countries. Eur. J. Inf. Syst. 23, 103–125. doi: 10.1057/ejis.2013.17
Murtagh, F., and Contreras, P. (2012). Algorithms for hierarchical clustering: an overview. WIREs Data Min. Knowl. Disc. 2, 86–97. doi: 10.1002/widm.53
National Crime Agency (2021). Fraud-The Threat From Fraud. Available online at: https://www.nationalcrimeagency.gov.uk/what-we-do/crime-threats/fraud-and-economic-crime.
Ng, M., Coopamootoo, K. P., Toreini, E., Aitken, M., Elliot, K., and van Moorsel, A. (2020). “Simulating the effects of social presence on trust, privacy concerns and usage intentions in automated bots for finance,” in 2020 IEEE European Symposium on Security and Privacy Workshops (EuroS&PW) (Genoa: IEEE), 190–199.
Norberg, P. A., Horne, D. R., and Horne, D. A. (2007). The privacy paradox: personal information disclosure intentions versus behaviors. J. Consum. Affairs 41, 100–126. doi: 10.1111/j.1745-6606.2006.00070.x
Ohm, P. (2014). Sensitive information. South Calif Law Rev. 88, 1125–1196. Available online at:https://heinonline.org/HOL/LandingPage?handle=hein.journals/scal88&div=39&id=&page=
Pang, P. C.-I., McKay, D., Chang, S., Chen, Q., Zhang, X., and Cui, L. (2020). Privacy concerns of the australian my health record: Implications for other large-scale opt-out personal health records. Inf. Process. Manag. 57, 102364. doi: 10.1016/j.ipm.2020.102364
Peer, E., Brandimarte, L., Samat, S., and Acquisti, A. (2017). Beyond the turk: alternative platforms for crowdsourcing behavioral research. J. Exp. Soc. Psychol. 70, 153–163. doi: 10.1016/j.jesp.2017.01.006
Rainie, L., Kiesler, S., Kang, R., Madden, M., Duggan, M., Brown, S., et al. (2013). Anonymity, Privacy, and Security Online. Washington, DC: Pew Research Center.
Rindfleisch, T. C. (1997). Privacy, information technology, and health care. Commun. ACM 40, 92–100. doi: 10.1145/257874.257896
Rumbold, J. M., and Pierscionek, B. K. (2018). What are data? A categorization of the data sensitivity spectrum. Big Data Res. 12, 49–59. doi: 10.1016/j.bdr.2017.11.001
Schomakers, E.-M., Lidynia, C., Müllmann, D., and Ziefle, M. (2019). Internet users' perceptions of information sensitivity-insights from germany. Int. J. Inf. Manag. 46, 142–150. doi: 10.1016/j.ijinfomgt.2018.11.018
Schomakers, E.-M., Lidynia, C., and Ziefle, M. (2020). All of me? Users' preferences for privacy-preserving data markets and the importance of anonymity. Electron. Markets 30, 649–665. doi: 10.1007/s12525-020-00404-9
Stiefel, S. (2018). ‘The chatbot will see you now': mental health confidentiality concerns in software therapy. Sci. Technol. Law Rev. 20.1 doi: 10.2139/ssrn.3166640. Available online at: https://heinonline.org/HOL/LandingPage?handle=hein.journals/cstlr20&div=12&id=&page=
Thomas, P., Grossman, M., Christmann, K., and Miah, S. (2020). Community reporting on violent extremism by “intimates”: emergent findings from international evidence. Crit. Stud. Terrorism 13, 1–22. doi: 10.1080/17539153.2020.1791389
Treiblmaier, H., and Chong, S. (2013). “Trust and perceived risk of personal information as antecedents of online information disclosure: Results from three countries,” in Global Diffusion and Adoption of Technologies for Knowledge and Information Sharing (IGI Global), 41–361.
Van den Broeck, E., Poels, K., and Walrave, M. (2015). Older and wiser? Facebook use, privacy concern, and privacy protection in the life stages of emerging, young, and middle adulthood. Soc. Media Soc. 1, 1–11. doi: 10.1177/2056305115616149
Wadle, L. -M., Martin, N., and Ziegler, D. (2019). “Privacy and personalization: The trade-off between data disclosure and personalization benefit,” in Adjunct Publication of the 27th Conference on User Modeling, Adaptation and Personalization (Larnaca Cyprus), 319–324.
Yu, L., Li, H., He, W., Wang, F.-K., and Jiao, S. (2020). A meta-analysis to explore privacy cognition and information disclosure of internet users. Int. J. Inf. Manag. 51, 102015. doi: 10.1016/j.ijinfomgt.2019.09.011
Keywords: personal information disclosure, information sensitivity, privacy, chatbots, conversational agents, artificial intelligence, personal information
Citation: Belen-Saglam R, Nurse JRC and Hodges D (2022) An Investigation Into the Sensitivity of Personal Information and Implications for Disclosure: A UK Perspective. Front. Comput. Sci. 4:908245. doi: 10.3389/fcomp.2022.908245
Received: 30 March 2022; Accepted: 08 June 2022;
Published: 30 June 2022.
Edited by:
Gualtiero Volpe, University of Genoa, ItalyCopyright © 2022 Belen-Saglam, Nurse and Hodges. This is an open-access article distributed under the terms of the Creative Commons Attribution License (CC BY). The use, distribution or reproduction in other forums is permitted, provided the original author(s) and the copyright owner(s) are credited and that the original publication in this journal is cited, in accordance with accepted academic practice. No use, distribution or reproduction is permitted which does not comply with these terms.
*Correspondence: Jason R. C. Nurse, ai5yLmMubnVyc2VAa2VudC5hYy51aw==