- 1Sri Ramachandra Institute of Higher Education and Research, Chennai, India
- 2All India Institute of Speech and Hearing (AIISH), Mysore, India
Introduction: The contribution of technology to the field of health is vast, both in diagnosis and management. More so, the use of computer-based intervention has become increasingly widespread over the past decade. Human beings experience a decline in auditory processing and cognitive skills as they age, consistent with deterioration of other bodily functions. In addition, speech perception abilities in both quiet and in the presence of noise are impacted by auditory processing abilities and cognitive skills such as working memory. This pilot study explored the use of music as an intervention for improving these skills and employed a computerized delivery of the intervention module.
Method: A battery of tests was carried out to assess the baseline auditory processing and working memory skills in eight older adults between the ages of 56 and 79 years, all of whom had normal hearing. Following the assessment, a short-term computerized music-based intervention was administered. The style of music chosen was Carnatic classical music, a genre widely practiced in Southern India. The intervention module involved note and tempo discrimination and was carried out for a maximum of 10 half-hour sessions. The multi-level intervention module was constructed and administered using Apex software. Following the intervention, the auditory processing and cognitive skills of the participants were reassessed to study any changes in their auditory processing and working memory skills.
Results and discussion: There were positive changes observed in all the auditory processing and some of the working memory abilities. This paper discusses in detail the systematic structuring of the computerized music-based intervention module and its effects on the auditory processing and cognitive skills in older adults.
1. Introduction
Technology has widely influenced health care with respect to evaluation, diagnosis, intervention as well as rehabilitation. Currently, technology augments clinical observations of physicians and aids in precision of diagnosis (Van Os et al., 2013; Dunkel et al., 2021; Tilli, 2021). The impact of the pandemic has been extensively felt in the follow up to hospitals for reviews, therapy, long duration interventions, etc. It has especially impacted the older population and people with poor health conditions due to their co-morbidities.
Hearing loss is becoming so widespread that the World Health Organization (2019) predicts that one in every ten people in the world will have disabling hearing loss by 2050. They also estimate that currently, more than 25% of people over the age of 60 years are impacted by disabling hearing loss. Auditory processing deficits are commonly observed in older adults even in the absence of any hearing loss (Füllgrabe et al., 2015; Sardone et al., 2019). Auditory processing deficits refer to difficulties in processing sounds in order to make sense of the auditory information. Normal auditory processing occurs due to the collective functioning of different mechanisms such as auditory discrimination, temporal processing (acoustic timing related information), and binaural processing (combining acoustic information from both ears) (American Speech-Language-Hearing Association, 2005). In older adults, auditory processing deficits are commonly exhibited as difficulty understanding speech in difficult listening situations such as talking over the phone, noisy environments (Rodriguez et al., 1990; Boboshko et al., 2018), difficulty maintaining attention to spoken information (Wayne et al., 2016), and asking for frequent repetitions (American Speech-Language-Hearing Association, n.d.), among others. Rehabilitation for people with auditory processing deficits involves working on the affected deficit or auditory process, environmental modifications to help improve the ease of listening, and providing assistive listening devices to enhance the acoustic quality.
Music has been repeatedly shown to have positive effects on different physical and mental aspects of the human body such as pain relief (Kumar et al., 2014), quality of sleep (Deshmukh et al., 2009), improved concentration, reduction in anxiety (Sung et al., 2010), and reduction in psychiatric symptoms (Lyu et al., 2018; Schroeder et al., 2018; Tsoi et al., 2018). Likewise, music training also has a positive impact on cognitive processes such as attention and memory, and other auditory functions such as listening in challenging situations (Besson et al., 2011; Strait and Kraus, 2011b). Patel (2011) proposed the OPERA hypothesis which also suggests that music benefits the ability of speech processing. The hypothesis states that music contributes to adaptive plasticity in the neural networks responsible for speech understanding when five conditions are met: there is Overlap in the neural networks between speech and music, music requires a higher precision of Processing from the neural networks, music elicits positive Emotions, musical activities are Repetitive, and musical activities require high levels of Attention. Patel (2014) also proposed the extended OPERA hypothesis, incorporating the effects of non-linguistic music on cognitive processes such as auditory attention and working memory. It was proposed that these cognitive processes could also benefit from musical training if the following conditions were met: there existed shared neural pathways, music placed higher demands on the cognitive processes than speech, and if music and the cognitive processes were combined with emotion, repetition, and attention. Hence, employing a music-based intervention to improve auditory processing and cognitive skills may be considered suitable.
The contribution of technology in the area of rehabilitation of individuals with hearing difficulties has been enormous. Wireless technology including Bluetooth and near frequency magnetic induction are being incorporated in amplification devices and hearing solutions (Kim and Kim, 2014). Hearing devices such as cochlear implants, hearing aids, and assistive listening devices benefit from the prime technological advances made by the industry and contribute to better quality of life of people with hearing difficulties (Hansen et al., 2019; Corey and Singer, 2021; Fabry and Bhowmik, 2021). For children with auditory processing deficits, there are computerized intervention programs such as Earobics (Concepts, 1997). Computerized interventions for auditory processing have also been studied and shown to be effective in older adults (Vaidyanath, 2015). Other commonly used computerized auditory training programs include the Computerized Learning Exercises for Aural Rehabilitation, clEAR (Tye-Murray et al., 2012), and Listening and Communication Enhancement, LACE (Sweetow and Sabes, 2007). Softwares such as FastForWord (Scientific Learning Corporation, 1998), Escuta Ativa, and others1 have also been used for the pediatric population.
The current study was an attempt to combine technology and Carnatic Classical music, a genre very common in the Southern part of India, into a music-based module to improve auditory processing and cognitive abilities. Carnatic music was chosen, as most older listeners in this region are exposed to and are interested in this music genre. The study aimed to evaluate the effectiveness of a short-term computerized intervention module which delivers music-based stimuli and its influence on auditory processing and working memory in this population. This program, if found effective, can be adopted as a clinical protocol for rehabilitation of older adults with auditory processing and working memory deficits. In the context of the ongoing pandemic, this program can also be delivered to vulnerable populations in the safety and comfort of their homes, while utilizing the advantage that technology offers.
2. Materials and methods
This pilot study examines the effect of a computerized music-based intervention module on the auditory processing and cognitive skills of older adults. Approval from the Institutional Ethics Committee was obtained prior to the initiation of the study.
2.1. Development and administration of the computerized music-based intervention module
The musical style employed in the current study was Carnatic music, a style of Indian Classical music which emerged in Southern India and is being performed globally. Scales consisting of 8 notes are called Raagaas in Indian classical music. Carnatic music has 72 such fundamental scales or raagaas. These raagaas have three notes in common—first (Sa), fifth (Pa), and eighth (Sa of the higher octave). In this study, six such scales consisting of 8 notes were chosen—Maayaamaalavagowla, Hemavathi, Vaachaspathi, Gaurimanohari, Thodi, and Kaamavardhini. The latter five scales differed from the first by five, four, three, two notes, and one note respectively (Table 1). Each of the scales were played at a base scale of D, and in tempos ranging from 120 beats per minute (BPM) to 480 BPM, with intervals of 60 BPM. This yielded a total of 7 tempos. A total of 42 scales were obtained (6 scales in 7 tempos). The music for the stimuli was played by a professional violinist trained in Carnatic music and recorded in a professional recording studio. All the stimuli were content validated by a professional musician with teaching and performing experience of more than 2 decades.
The interactive intervention module comprised of two types—note discrimination and tempo discrimination. The note discrimination task was constructed on three levels with increasing complexity, ranging from simple to a more challenging task. The first and second levels employed the twelve single notes, and the task was to discriminate between them. For generating the stimuli, scales played at a tempo of 120 BPM were chosen. The twelve octave notes were isolated using the Adobe Audition CS5.5 software. Each note was about 0.5 second long at this tempo.
The first level of note discrimination included pairs of single notes that differed from each other by 10, 9, 8, or 7 semitones, i.e., having large frequency differences, and easier to discriminate between (e.g., Ni1-Ri1 and Dha2-Ri1). Catch trials (pairs with the same note) were also included. This yielded 14 combinations and 10 catch trials. Participants were instructed to indicate if the two stimuli were ‘Same' or ‘Different'.
The second level included single notes that differed from each other by 6, 5, and 4 semitones. The notes were arranged in sets of three, with one of the notes differing from the other two presented (e.g., Ri1-Pa-Pa and Ni2-Ma2-Ni2). The second level had 21 combinations. Participants indicated which one of the three stimuli were different. The first and second levels of note training involved only single notes.
The third level of note discrimination included discrimination of entire 8-note scales (Sa-Ri-Ga-Ma-Pa-Dha-Ni-Sa) in the 240 BPM tempo. This tempo was chosen out of the seven tempos recorded, as it was neither too slow nor too fast to perceive. One scale was kept constant (Maayaamaalavagowla) and repeated twice, and the third stimuli was any one of the other five scales. For example, the first and second stimuli may be Maayaamaalavagowla, and the third Thodi, in which case the correct response would be ‘3'. The easiest to discriminate between were Maayaamaalavagowla and Hemavathi, as they differed by five notes, and the most difficult to discriminate between were Maayaamaalavagowla and Kaamavardhini, as they differed by only one note. The position of Maayaamaalavagowla among the three stimuli was randomly assigned by the software for each trial.
Tempo discrimination employed 8-note scales as stimuli, similar to the stimuli used in level 3 of note discrimination. This was constructed on two levels. The first level (Figure 1) involved two 8-note scales in which the tempo difference between each scale was large: 240 BPM and 300 BPM. For example, 8-note scales with tempos of 120 BPM and 360 BPM were paired, or tempos of 240 BPM and 480 BPM were paired, for discrimination. This level included all six raagaas and yielded 30 combinations and 33 catch trials. Participants were instructed to indicate whether the two were ‘Same' or ‘Different'. The second level (Figure 2) comprised of 8-note scales differing from each other by tempos of 120 BPM and 180 BPM. Three such scales were presented in which two were of the same tempo and one had a different tempo (e.g., 120 BPM-300 BPM-300 BPM and 360 BPM-240 BPM-360 BPM). The tempo differences between the scales were smaller than the first level and had 54 combinations. Participants had to indicate which one of the three scales presented was different.
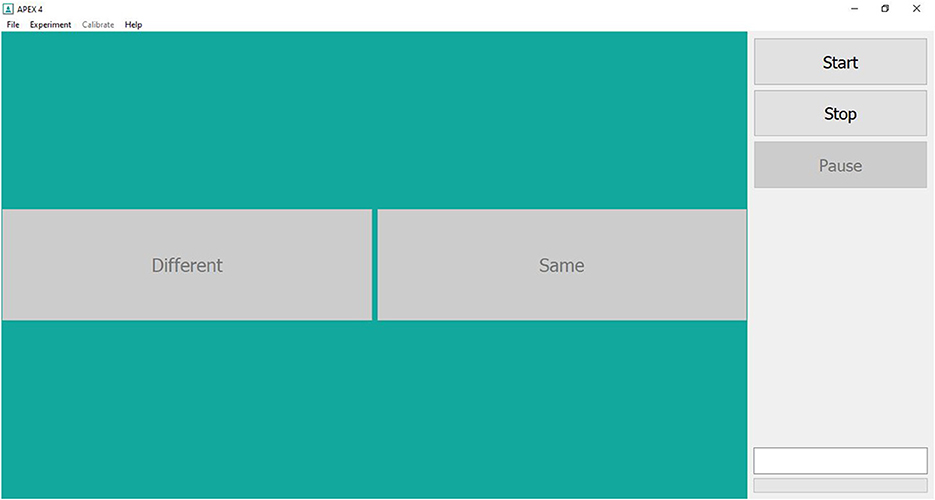
Figure 1. Screenshot of Level 1 of tempo and note discrimination module of the Computerized music-based training program on the Apex 4.1.2 software.
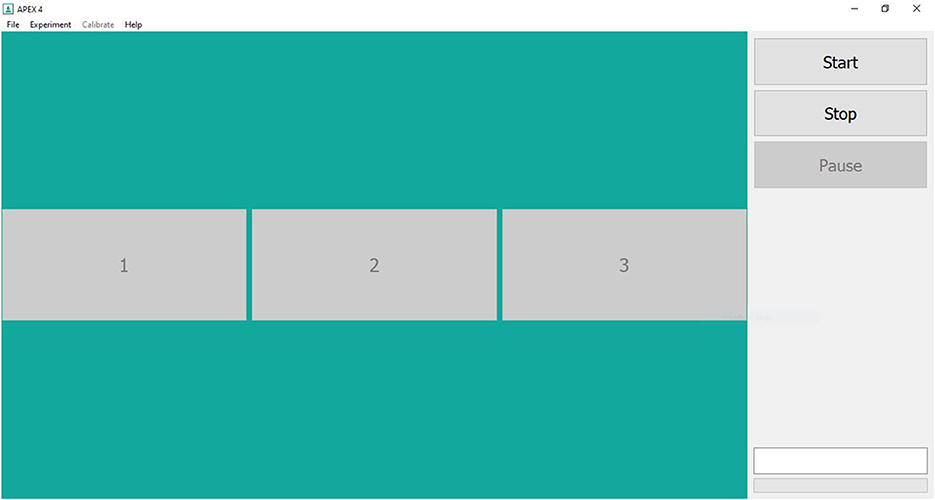
Figure 2. Screenshot of Levels 2 and 3 of note discrimination and level 2 of tempo discrimination modules of the Computerized music-based training program on the Apex 4.1.2 software.
The intervention module was structured using Apex (4.1.2), a software commonly employed in psychoacoustical experiments, developed by Francart et al. (2008). The inter-stimulus-interval was maintained at 500 ms. The subsequent set of stimuli was presented only after a response was obtained from the participants, allowing them adequate time to reflect on the differences between the stimuli presented. The participants were instructed to provide a response even if they were unsure whether their response was correct. Visual feedback was provided immediately after each response from the participant. The software flashed a green colored ‘thumbs up' picture for a correct response and a red colored ‘thumbs down' picture for a wrong response. The experiments in this software were defined using the XML format.
2.2. Participants
Eight right-handed participants (four females & four males) between the ages of 56 and 79 years (mean 63.87 years) were recruited for the study. Written informed consent was obtained from each participant prior to the commencement of the study. All the participants were native Tamil (South-Indian language) speakers and had an education of 10th grade or higher. Their hearing thresholds were screened using pure-tone audiometry to ensure audibility of 25 dB HL or better in both ears between 250 Hz and 2,000 Hz. Montreal Cognitive Assessment-Tamil (MoCA-Tamil) was administered to confirm normal cognitive functioning (score of ≥26). None of the participants had any neurological, psychiatric, or otologic conditions at the time of study or prior to the study. None of the participants were formally trained in any style of music.
2.3. Procedure
All assessments and training were carried out in a quiet room with ambient noise levels < 40 dBA, as measured using the NIOSH SLM app (version 1.2.4.60) on an Iphone SE 2020 device. The assessment of auditory processing and cognitive skills were carried out twice before initiation of the intervention and once after completion. The two pre-intervention assessments were carried out with a gap of 2 to 4 weeks between them to ensure consistency of the baseline measurement.
The auditory processing tests assessed binaural integration (Dichotic Digit Test in Tamil), speech perception in quiet and noise (Tamil Matrix Sentence Test), temporal resolution (Gap-In-Noise), temporal patterning (Duration and Pitch Pattern tests), and temporal fine structure perception of the participants. Prior to administration of these tests, practice trials were provided to familiarize the participants with the task and the response expected.
The Dichotic Digit Test in Tamil, DDT-T (Sudarsonam and Vaidyanath, 2019) consisted of 30 trials in which two digits were presented to the right ear and two to the left ear simultaneously. Participants were instructed to repeat all four digits, irrespective of the order or ear of presentation. A total score out of 30 was obtained each for single correct scores in the left and right ears, and double correct scores (correct responses from both ears). Norms developed by Sudarsonam and Vaidyanath (2019) in normal hearing young adults were used in this study.
Speech perception in quiet was assessed using Tamil Matrix Sentence Test, TMST (Krishnamoorthy and Vaidyanath, 2018) consisting of 24 lists with 10 sentences and with 5 words in each sentence. These sentences were derived from a base matrix of 50 words with 10 alternates for each word. The sentences were constructed to follow the grammatical structure of Tamil language. For example, the English translation of a Tamil Matrix sentence is Vasu bought ten wrong bags. This prevented the participants from deducing entire sentences from context. For assessing speech perception in noise, these sentences were combined with multi-talker speech babble, developed by Gnanasekar and Vaidyanath (2019), at three Signal-noise ratios (SNRs): −5 dB SNR (speech 5 dB lower than noise), 0 dB SNR (speech and noise at equal levels), and +5 dB SNR (speech 5 dB higher than noise). Participants were instructed to repeat as many words of the sentence as they could. One list was presented per ear, for each condition of sentence recognition, and a total score out of 50 was obtained.
The Gap-In-Noise test, GIN (Musiek et al., 2005) consisted of 6s noise tokens in which small intervals of silences were embedded. GIN was administered separately in each ear. The participants were instructed to listen carefully and indicate when they heard a gap in the noise. This test yielded an approximate threshold of gap detected in ms (A.th) and a percentage correct score. Norms for the GIN test in Indian population, reported by Aravindkumar et al. (2012), were considered in the current study to judge whether a person passed or failed on the test.
The Duration Pattern Test, DPT (Musiek et al., 1990) and the Pitch Pattern Test, PPT (Musiek and Pinheiro, 1987) assessed temporal patterning skills separately in each ear. DPT consisted of two pure-tones of different lengths. These were arranged in sets of three (e.g., long-long-short, or short-long-short) and the participants were instructed to report the pattern heard during each trial. PPT involved two pure tones of high and low pitches. They were arranged in a similar manner as DPT and the participants were to report the pattern heard (e.g., high-low-low, or low-high-low). Scores out of 30 were recorded for both DPT and PPT. Cut off scores obtained by Gauri (2003) were used for DPT and the norms given by Tiwari (2003) were considered for PPT.
TFS1 (Moore and Sek, 2009) was a software developed to assess monaural temporal fine structure perception. Two fundamental frequencies (F0s) were chosen − 222 Hz and 94 Hz, similar to Moore et al. (2012), and were used to test Temporal Fine Structure perception abilities in the two ears separately. The software presented two stimuli and the participants were instructed to indicate which of the stimuli they found to be fluctuating. The software employed an adaptive procedure, which, if the participant was able to complete, yielded a result in Hz. This was the minimum frequency fluctuation that was perceived by the participant. If they were unable to complete the adaptive procedure, the software provided a score out of 40. For the purpose of statistical analysis, this was converted to Hz as delineated by Moore et al. (2012) and using the detectability index d' standard tables provided by Hacker and Ratcliff (1979). The norms obtained by Moore et al. (2012) were used in the current study.
The cognitive tests were carried out using the Smriti Shravan software (version 3.0) developed at the All India Institute of Speech and Hearing, Mysuru, India. This software is a customized platform to assess auditory and visual memory. The cognitive tests included forward digit span, backward digit span, running span, N-back, and operation span tests which assessed auditory attention, sequencing, and working memory. These tests were administered binaurally. The stimuli for the cognitive tests were the Tamil digits used in the TMST, which were fed into the software. In the forward digit span test, a series of digits were presented, and the participants were instructed to repeat the digits in the same order as was presented. In the backward span, the participants were instructed to repeat the digits presented in the reverse order of presentation. Initially, two numbers were presented and if repeated correctly, the software presented three numbers and so on. A one-up one-down adaptive procedure was followed and eight reversals were employed. The final span obtained was an average of the final three reversals.
In the N-back test, a 2-back task was carried out. This entailed the participants to repeat the penultimate number heard of the set of numbers presented. In the running span, 2-span was employed, and the participants were asked to repeat the final two numbers presented. Scores out of 20 were obtained for both N-back and running span tests. Operation span alternated presentations of a simple arithmetic equation (timed to complete within 3 seconds) and a number displayed on the screen. The participant had to indicate ‘true' or ‘false' to the solution for the equation and then memorize and repeat the numbers displayed, in the correct order. The software yielded a percentage score for the arithmetic task (secondary task), an All-or-None Score Weighted (ANSW) indicating the order and correctness of the response, and a Partial Credit Score Weighted (PCSW) indicating whether the correct numbers were repeated at all.
In addition to the tests of auditory processing and working memory, the Screening checklist for Auditory Processing in Adults, SCAP-A (Vaidyanath and Yathiraj, 2014) and Noise Exposure Questionnaire (Johnson et al., 2017) were also administered. A score of ≥4 out of 12 indicated a risk of auditory processing deficit on the SCAP-A. None of the participants were found to have any history of noise exposure in the past 12 months as noted on the Noise Exposure Questionnaire.
Following the second baseline assessment, the participants began the intervention module. The intervention was provided for 10 sessions (half an hour duration) over a period of 3 weeks. Each session consisted of 15 min each of note and tempo discrimination. The software provided the participants with feedback about the correctness of each response. Each level was repeated until the participants obtained at least 80% score, after which they progressed to the next level. If there was no improvement in performance in any of the levels during three consecutive sessions, training was discontinued. The number of sessions of training administered ranged from five to ten for the eight participants reported in this study (Table 2). Immediately after the intervention concluded, a post-intervention assessment of auditory processing and cognitive skills was carried out.
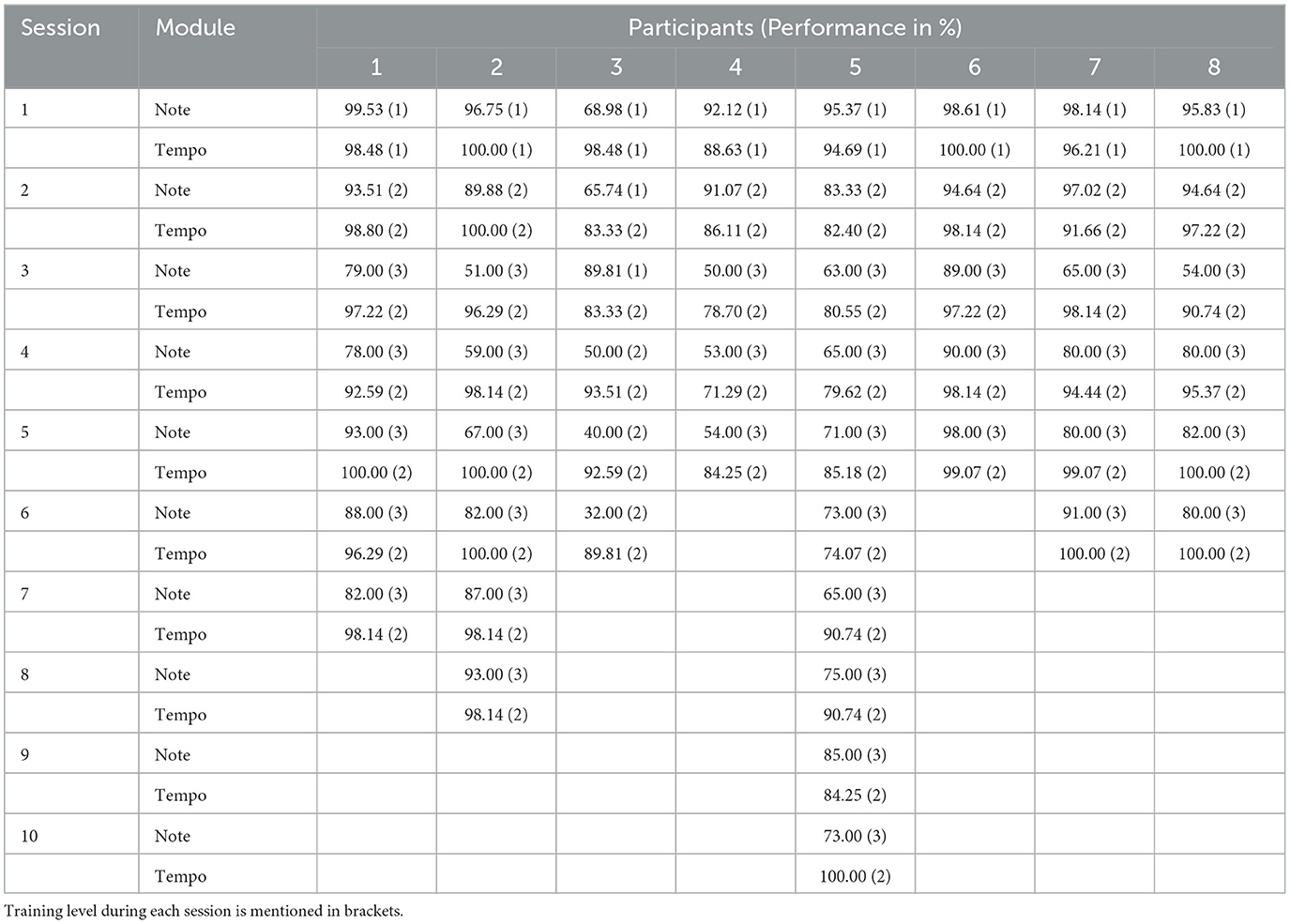
Table 2. Session wise performance of the participants on note and tempo discrimination modules of the music-based intervention.
All the assessment and training stimuli were administered using calibrated Sennheiser HD280 Pro supra-aural headphones from a laptop with Intel(R) Core (TM) i5-6200U CPU processor @ 2.30 GHz and 8 GB RAM at an intensity level of 70 dB SPL. The pre-intervention and post-intervention assessment values were tabulated on a Microsoft Excel 365 worksheet. Statistical analysis was carried out using IBM SPSS Statistics 21 software. A Shapiro-wilk test was carried out to assess normality among the obtained data. As the data were found to be not normally distributed, and the sample size was also small, non-parametric tests (Wilcoxon Signed Ranks Test) were carried out to compare the performances of the participants before and after the intervention.
3. Results
The study examined the effects of a computerized music-based intervention module on the auditory processing and working memory abilities of older adults. This was a pilot study to an ongoing research work and hence the results of a limited sample have been reported. The Wilcoxon Signed Ranks Test was carried out between the pre-intervention baseline 1 and baseline 2, in order to verify that there was no change in the performances of the participants before the intervention was provided. No significant differences were found between the two baseline assessments in any of the tests of auditory processing and working memory (Table 3). Hence, for further analyses, the pre-intervention baseline 1 was considered for comparison with the post-intervention assessment.
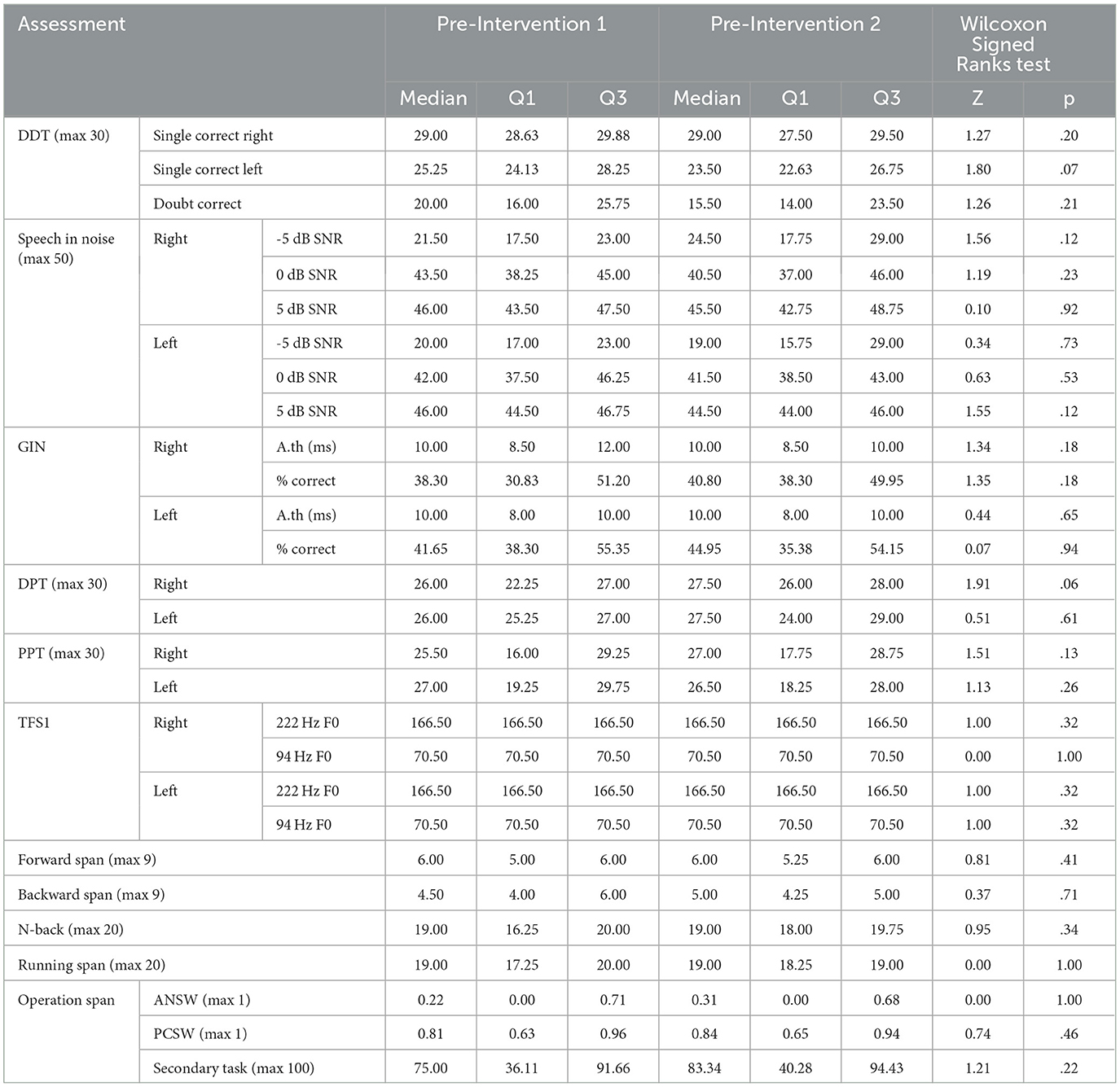
Table 3. Median and quartiles (Q1 & Q3) for scores obtained on the tests of auditory processing and working memory for the two pre-intervention assessments and Wilcoxon Signed Ranks test results comparing the two pre-intervention assessments.
The Wilcoxon Signed Ranks test comparing the difference in performance between the two ears showed a significantly better performance in the right ear compared to the left in DDT-T (Z = −2.38, p = .017). This is called the Right Ear Advantage and is commonly observed in right-handed individuals. Following the computer-based intervention, statistically significant differences were found between the pre and post intervention performances of DDT-T (right single correct and double correct scores). The right ear scores of all the participants had reached the norms while the left ear and double correct scores of two participants achieved norms.
With respect to auditory closure which was assessed using TMST in the presence of noise, performance in the right ear (−5 dB SNR and +5 dB SNR conditions) significantly improved post-intervention. There was no significant difference between the performance of the right and left ears.
Temporal processing, as assessed using GIN, also showed a significant difference between the right and left ear percentage correct scores (Z = −2.21, p = .027), but this was not observed in the A.th. There was a significant improvement observed post-intervention in both ears' A.Th (Figure 3), with five participants performing normally. Percentage scores did not exhibit a significant difference pre and post intervention.
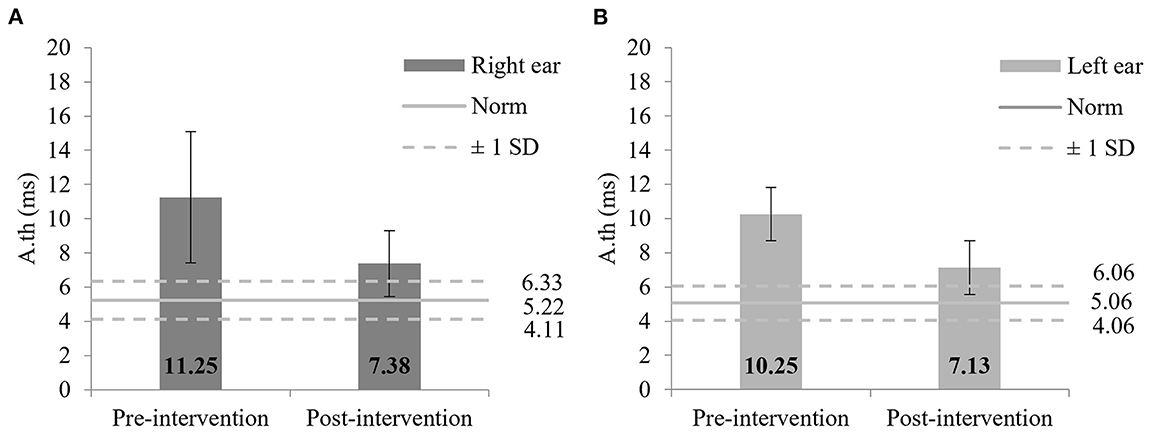
Figure 3. (A) Mean performance on GIN A.th pre and post intervention in the right ear; (B) Mean performance on GIN A.th pre and post intervention in the left ear. Error bars indicate standard deviations. Norms obtained by Aravindkumar et al. (2012) and ±1 SD in young normal hearing adults are shown.
Temporal patterning which was evaluated using DPT and PPT showed a significant improvement in both ears post-intervention (Figures 4, 5). All but one participant had normal DPT scores in the right ear and left ear scores, post intervention. Six participants showed normal PPT scores in both ears following the intervention.
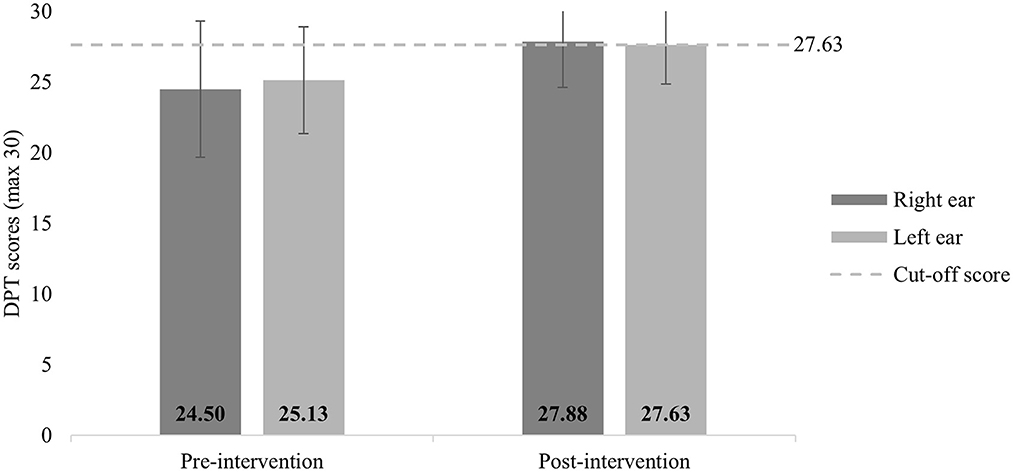
Figure 4. Mean performance on DPT pre and post intervention. Error bars indicate standard deviations. Cut-off scores obtained by Gauri (2003) for young normal hearing adults are depicted.
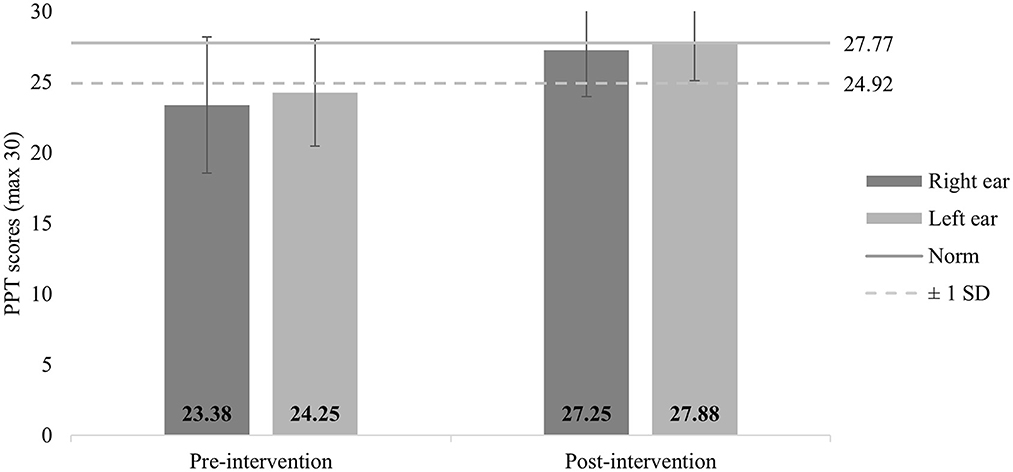
Figure 5. Mean performance on PPT pre and post intervention. Error bars indicate standard deviations. Norms obtained by Tiwari (2003) for young normal hearing adults are depicted.
Temporal Fine Structure Perception which was evaluated using the TFS1 software employed two conditions—F0 of 222 Hz and 94 Hz. A significant improvement was found in the 222 Hz condition in both ears, with three participants achieving normal levels. Despite the 94 Hz condition not showing a statistically significant difference, two participants showed betterment, reaching the normal values following the intervention.
Among the cognitive tasks, the forward digit span task showed a significant difference post-intervention. Although all the tasks showed an improvement following the intervention, the difference was not statistically significant. The self-rating questionnaire of SCAP-A showed a significant improvement with reduction in scores, with all but one falling within the range denoting no risk of auditory processing deficit (Table 4).
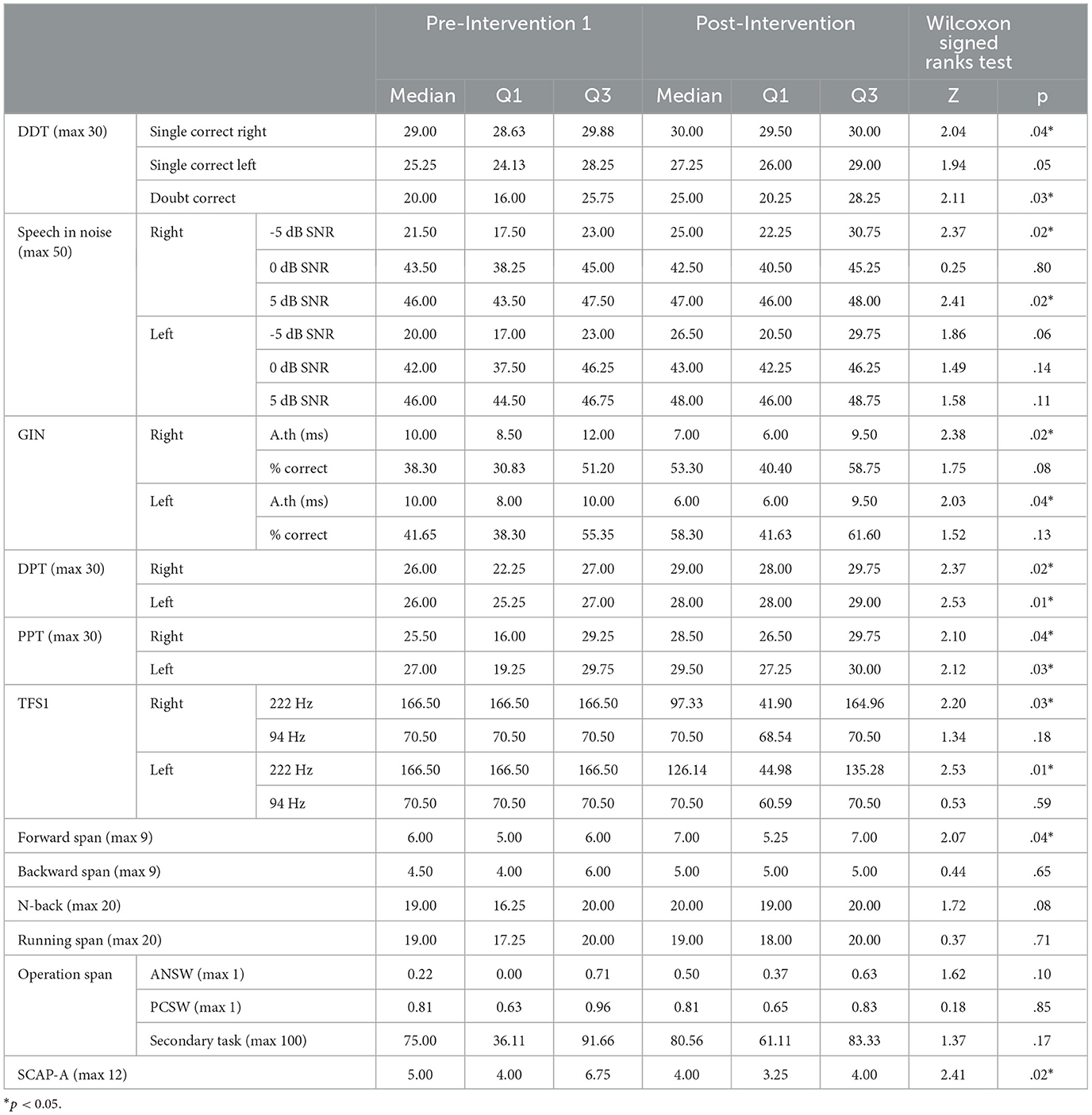
Table 4. Median and quartiles (Q1 & Q3) for scores obtained on the tests of auditory processing and working memory across pre-intervention 1 and post-intervention assessments and Wilcoxon Signed Ranks test comparing the pre and post intervention performances.
4. Discussion
The current pilot study explored the use and effectiveness of a computerized music-based intervention module, in improving the auditory processing and working memory abilities in older adults. Two baseline assessments were carried out, before the short-term intervention was provided, to ensure that the auditory processing and working memory performances were comparable before the intervention. A post intervention assessment was carried out to observe if there was any improvement after the short-term intervention. The results revealed that all the auditory processing and cognitive tests, especially those assessing temporal processing abilities such as GIN, DPT, PPT, and Temporal Fine Structure perception showed improvements after the short-term music-based intervention.
It was found that in DDT-T, a difference between performances in right and left ears were present. This observation is known as the right ear advantage. The DDT-T is a test in which both ears receive different stimuli at the same time (dichotic). This ear difference can be explained by the majority of auditory neural fibers crossing over to the to the contralateral cerebral hemisphere. Therefore, the right ear is more efficient in perceiving verbal stimuli in right handed individuals who have dominant left cerebral hemispheres (Kimura, 1961). In the current study, all eight participants were right-handed, which explains the presence of right ear advantage. In addition, binaural integration performance was observed to have improved following the intervention. The sustained and focused attention for about half an hour during each session may have contributed to increased attention to the auditory stimuli presented to each ear, resulting in better DDT-T scores.
A significant improvement in speech perception in noise was observed in addition to all the tests assessing temporal processing such as GIN, DPT, and PPT. Given the contribution of temporal processing to speech perception in quiet and noise (Phillips et al., 2000; Schneider and Pichora-Fuller, 2001; Snell et al., 2002; Vaidyanath, 2015; Roque et al., 2019), it is expected that with a larger sample size, the effects on the speech perception scores will be larger. Participants also had better temporal fine structure perception following the intervention. However, greater improvement was observed in the 222 Hz F0 condition than the 94 Hz F0 condition. This was in agreement with the observations of Moore et al. (2012), as perception of temporal fine structure worsens with reduction in F0 (Moore and Sek, 2009). Pre-intervention, only two participants were able to complete the adaptive procedure in at least one ear, in either of the F0 conditions. Post-intervention, five participants were able to complete adaptive procedure in at least one F0 condition, in at least one ear. Of these, four had thresholds within normal range. These changes could be due to the manner in which the intervention module was constructed. The module incorporated temporal and frequency perception tasks extensively, beginning from simple discrimination and increasing in complexity. Level 2 and 3 of the intervention module required finer temporal and frequency discrimination between musical notes. The repeated presentations of the musical clips, which had minute differences in frequency and temporal aspects, may have resulted in the improvements in these auditory skills. A study conducted by Jain et al. (2015) that employed a computerized short term music training for normal hearing young adults showed improved speech perception in noise. A similar short term computerized music based training showed improvements in psychoacoustic skills such as frequency, intensity, and temporal perception as well, but these were not significant (Jain et al., 2014). Both training programs were short-term and involved Carnatic music. Music has long been recommended for maintaining and improving auditory skills (Strait and Kraus, 2011a,b; White-Schwoch et al., 2013), and has shown positive results even if it is a short-duration training (Lappe et al., 2011).
A significant change in forward digit span was observed, while minor individual improvements were observed in the other working memory tasks, although they did not reach significance. This could be because the N-back (2-back) and running span (2-span) tasks did not place adequate cognitive demands for the older adults. Possibly, if 3-back and 3-span were assessed, it may have necessitated a greater cognitive effort and could have judged the effect of the intervention on these processes better. Nevertheless, on the 2-back and 2-span tasks, the participants reported lesser fatigue and required lesser number of pauses between tasks, post-intervention.
Pre-intervention, five participants had SCAP-A scores >4, which indicated self-perceived auditory processing difficulties. Post-intervention, only one participant had a score >4. This was also found to be statistically significant and demonstrated that the participants experienced improved auditory processing in their daily lives after undergoing the computerized music-based intervention. The uninterrupted attention that the intervention module entailed over a period of a little more than half an hour per session may have contributed to improvement in the working memory tasks, and to the cognitive abilities required to perform the auditory processing tasks as well.
The improvements observed following the music based intervention is also supported by the OPERA hypothesis (Patel, 2011), according to which music may help improve speech perception if five conditions are fulfilled. The current protocol satisfies four of the conditions: Overlap of neural pathways between music and speech, Precision required to discriminate between the stimuli presented, Repetition of each level of the intervention until the participant reaches a score of 80%, and Attention to the minute differences between the stimuli to achieve the 80% score. As this study made use of small clips of non-linguistic instrumental music, which was unfamiliar for the participants, the Emotion criterion of the OPERA hypothesis may not be fulfilled. However, the extended OPERA hypothesis (Patel, 2014) suggests that instrumental music, due to having no linguistic component, does place a higher level of demand on auditory memory.
The advantages that this intervention module had over conventional auditory training was the usage of music and delivering it in a standardized and controlled manner using technology. For assessing the auditory processing and working memory skills of the participants, all the tests were administered in a structured manner by making use of calibrated output through headphones and computer softwares. This standard was maintained during the intervention notwithstanding the use of music, which is a diverse art form and includes multiple genres being widely practiced globally, such as classical, fusion, folk, and so on. Even in Indian Classical music, there is the Carnatic style and the Hindustani styles, both varying in theory and presentation. This control and standard could be achieved by incorporating the chosen style of music (Carnatic classical) into a software, Apex, which lent accuracy to factors such as intensity of presentation, duration of the intervention, and documentation of the participants' progress. The software recorded the responses of the participants and presented a percentage correct score at the end of each session.
A minor limitation of this study is that, although the handedness of the participants was verbally recorded, it was not assessed using a published tool. Future studies can incorporate a formal handedness assessment tool, which may aid in the interpretation of the binaural listening abilities (DDT-T). In addition, the results reported here for a limited sample size may be confirmed by the results in a larger sample of older listeners with auditory processing deficits, to be able to draw better conclusions.
In conclusion, this preliminary study found that a computerized short-term intervention protocol that makes use of Carnatic musical stimuli resulted in improvements in auditory temporal processing skills, which led to better speech perception in noise. If found effective on a larger population, the intervention protocol can be delivered to older and vulnerable individuals at their convenience, given that it is designed to be delivered using a computer software at a calibrated output. This may lead to better rehabilitation schedules and clinical resources being allocated and utilized. Prospective studies can explore the effectiveness of this computerized music-based intervention protocol on children with auditory processing disorders.
Data availability statement
The raw data supporting the conclusions of this article will be made available by the authors, without undue reservation.
Ethics statement
The studies involving human participants were reviewed and approved by Institutional Ethics Committee, Sri Ramachandra Institute of Higher Education and Research. The patients/participants provided their written informed consent to participate in this study.
Author contributions
VR and RV designed the study with inputs from AU and SV. VR and RV and analyzed the data. VR carried out data collection and wrote the initial draft of the manuscript with extensive inputs from RV. All the authors have contributed to and reviewed the manuscript.
Funding
VR was funded by the Sri Ramachandra Founder-Chancellor Shri. N. P. V. Ramaswamy Udayar Research Fellowship.
Acknowledgments
The authors acknowledge the Faculty of Audiology and Speech Language Pathology at Sri Ramachandra Institute of Higher Education and Research, Chennai, India for lending their support and equipment for completing the study. All participants are also acknowledged for their participation.
Conflict of interest
The authors declare that the research was conducted in the absence of any commercial or financial relationships that could be construed as a potential conflict of interest.
Publisher's note
All claims expressed in this article are solely those of the authors and do not necessarily represent those of their affiliated organizations, or those of the publisher, the editors and the reviewers. Any product that may be evaluated in this article, or claim that may be made by its manufacturer, is not guaranteed or endorsed by the publisher.
Footnotes
1. ^CTS Informatics. Escuta Ativa [Computer Software].
References
American Speech-Language-Hearing Association (2005). (Central) Auditory Processing Disorders-The Role of the Audiologist. [Position Statement], Available online at: www.asha.org/policy (accessed January 13, 2023).
American Speech-Language-Hearing Association (n.d.). Central Auditory Processing Disorder. (Practice Portal). Available online at: http://www.asha.org/Practice-Portal/Clinical-Topics/Central-Auditory-Processing-Disorder/ (accessed November, 20, 2022).
Aravindkumar, R., Shivashankar, N., Satishchandra, P., Sinha, S., Saini, J., and Subbakrishna, D. K. (2012). Temporal resolution deficits in patients with refractory complex partial seizures and mesial temporal sclerosis (MTS). Epilepsy Behav. 24, 126–130. doi: 10.1016/j.yebeh.2012.03.004
Besson, M., Chobert, J., and Marie, C. (2011). Transfer of training between music and speech: common processing, attention, and memory. Front. Psychol. 2, 94. doi: 10.3389/fpsyg.2011.00094
Boboshko, M., Zhilinskaya, E., and Maltseva, N. (2018). “Characteristics of Hearing in Elderly People,” in Gerontology, eds. G., D'Onofrio, D., Sancarlo, and A., Greco (London: IntechOpen). doi: 10.5772/intechopen.75435
Corey, R. M., and Singer, A. C. (2021). “Adaptive Binaural Filtering for a Multiple-Talker Listening System Using Remote and On-Ear Microphones,” in 2021 IEEE Workshop on Alications of Signal Processing to Audio and Acoustics (WASPAA) 1–5. doi: 10.1109/WASPAA52581.2021.9632703
Deshmukh, A. D., Sarvaiya, A. A., Seethalakshmi, R., and Nayak, A. S. (2009). Effect of Indian classical music on quality of sleep in depressed patients: A randomized controlled trial. Nordic J. Music Ther. 18, 70–78. doi: 10.1080/08098130802697269
Dunkel, L., Fernandez-Luque, L., Loche, S., and Savage, M. O. (2021). Digital technologies to improve the precision of paediatric growth disorder diagnosis and management. Growth Hormone IGF Res. 59, 1–8. doi: 10.1016/j.ghir.2021.101408
Fabry, D. A., and Bhowmik, A. K. (2021). Improving speech understanding and monitoring health with hearing aids using artificial intelligence and embedded sensors. Semin Hear. 42, 295–308. doi: 10.1055/s-0041-1735136
Francart, T., van Wieringen, A., and Wouters, J. (2008). APEX 3: a multi-purpose test platform for auditory psychophysical experiments. J. Neurosci. Methods 172, 283–293. doi: 10.1016/j.jneumeth.2008.04.020
Füllgrabe, C., Moore, B. C. J., and Stone, M. A. (2015). Age-group differences in speech identification despite matched audiometrically normal hearing: contributions from auditory temporal processing and cognition. Front. Aging Neurosci. 6, 1–25. doi: 10.3389/fnagi.2014.00347
Gauri, D. T. (2003). Development of Norms on Duration Pattern Test. Mysore: All India Institute of Speech and Hearing. Available online at: http://203.129.241.86:8080/xmlui/handle/123456789/4479 (accessed May 8, 2023).
Gnanasekar, S., and Vaidyanath, R. (2019). Perception of tamil mono-syllabic and bi-syllabic words in multi-talker speech babble by young adults with normal hearing. J. Audiol. Otol. 23, 181–186. doi: 10.7874/jao.2018.00465
Hacker, M. J., and Ratcliff, R. (1979). A revisted table of d′ for M-alternative forced choice. Percept. Psychophy. 26, 168–170. doi: 10.3758/BF03208311
Hansen, J. H. L., Ali, H., Saba, J. N., Ram, C. M. C., Mamun, N., Ghosh, R., et al. (2019). “CCi-MOBILE: Design and Evaluation of a Cochlear Implant and Hearing Aid Research Platform for Speech Scientists and Engineers,” in IEEE EMBS International Conference on Biomedical & Health Informatics (BHI). doi: 10.1109/BHI.2019.8834652
Jain, C., Mohamed, H., and Kumar, A. U. (2014). Short-term musical training and pyschoacoustical abilities. Audiol. Res. 102, 40–45. doi: 10.4081/audiores.2014.102
Jain, C., Mohamed, H., and Kumar, A. U. (2015). The effect of short-term musical training on speech perception in noise. Audiol. Res. 111, 5–8. doi: 10.4081/audiores.2015.111
Johnson, T. A., Cooper, S., Stamper, G. C., and Chertoff, M. (2017). Noise exposure questionnaire: a tool for quantifying annual noise exposure. J. Am. Acad. Audiol. 28, 14–35. doi: 10.3766/jaaa.15070
Kim, J. S., and Kim, C. H. (2014). A review of assistive listening device and digital wireless technology for hearing instruments. Korean J. Audiol. 18, 105–111. doi: 10.7874/kja.2014.18.3.105
Kimura, D. (1961). Cerebral dominance and the perception of verbal stimuli. Canadian J. Psychol. 15, 166–171. doi: 10.1037/h0083219
Krishnamoorthy, T., and Vaidyanath, R. (2018). Development and validation of Tamil Matrix Sentence Test. Unpublished Masters dissertation, Sri Ramachandra Medical College and Research Institute.
Kumar, T. S., Muthuraman, M., and Krishnakumar, R. (2014). Effect of the raga ananda bhairavi in post operative pain relief management. Indian J. Surg. 76, 363–370. doi: 10.1007/s12262-012-0705-3
Lappe, C., Trainor, L. J., Herholz, S. C., and Pantev, C. (2011). Cortical plasticity induced by short-term multimodal musical rhythm training. PLoS ONE 6, 1–8. doi: 10.1371/journal.pone.0021493
Lyu, J., Zhang, J., Mu, H., Li, W., Mei, C., Qian, X., et al. (2018). The effects of music therapy on cognition, psychiatric symptoms, and activities of daily living in patients with Alzheimer's disease. J. Alzheimer's Dis. 64, 1347–1358. doi: 10.3233/JAD-180183
Moore, B. C. J., and Sek, A. (2009). Development of a fast method for determining sensitivity to temporal fine structure. Int. J. Audiol. 48, 161–171. doi: 10.1080/14992020802475235
Moore, B. C. J., Vickers, D. A., and Mehta, A. (2012). The effects of age on temporal fine structure sensitivity in monaural and binaural conditions. Int. J. Audiol. 51, 715–721. doi: 10.3109/14992027.2012.690079
Musiek, F. E., Baran, J. A., and Pinheiro, M. L. (1990). Duration pattern recognition in normal subjects and patients with cerebral and cochlear lesions. Audiology 29, 304–313. doi: 10.3109/00206099009072861
Musiek, F. E., and Pinheiro, M. L. (1987). Frequency Patterns in Cochlear, Brainstem, and Cerebral Lesions: Reconnaissance mélodique dans les lésions cochléaires, bulbaires et corticales. Audiology 26, 79–88. doi: 10.3109/00206098709078409
Musiek, F. E., Shinn, J. B., Jirsa, R., Bamiou, D.-E., Baran, J. A., and Zaida, E. (2005). GIN (Gaps-In-Noise) test performance in subjects with confirmed central auditory nervous system involvement. Ear Hear. 26, 608–618. doi: 10.1097/01.aud.0000188069.80699.41
Patel, A. (2011). Why would musical training benefit the neural encoding of speech? The OPERA Hypothesis. Front. Psychol. 2, 1–14. doi: 10.3389/fpsyg.2011.00142
Patel, A. D. (2014). Can nonlinguistic musical training change the way the brain processes speech? The expanded OPERA hypothesis. Hear. Res. 308, 98–108. doi: 10.1016/j.heares.2013.08.011
Phillips, S. L., Gordon-Salant, S., Fitzgibbons, P. J., and Yeni-Komshian, G. (2000). Frequency and temporal resolution in elderly listeners with good and poor word recognition. J. Speech Lang. Hear. Res. 43, 217–228. doi: 10.1044/jslhr.4301.217
Rodriguez, G. P., DiSarno, N. J., and Hardiman, C. J. (1990). Central auditory processing in normal-hearing elderly adults. Audiology 29, 85–92. doi: 10.3109/00206099009081649
Roque, L., Karawani, H., Gordon-Salant, S., and Anderson, S. (2019). Effects of age, cognition, and neural encoding on the perception of temporal speech cues. Front. Neurosci. 13, 1–15. doi: 10.3389/fnins.2019.00749
Sardone, R., Battista, P., Panza, F., Lozupone, M., Griseta, C., Castellana, F., et al. (2019). The age-related central auditory processing disorder: silent impairment of the cognitive ear. Front. Neurosci. 13, 619. doi: 10.3389/fnins.2019.00619
Schneider, B. A., and Pichora-Fuller, M. K. (2001). Age-related changes in temporal processing: implications for speech perception. Semin. Hear. 22, 227–240. doi: 10.1055/s-2001-15628
Schroeder, R. W., Martin, P. K., Marsh, C., Carr, S., Richardson, T., Kaur, J., et al. (2018). An individualized music-based intervention for acute neuropsychiatric symptoms in hospitalized older adults with cognitive impairment: a prospective, controlled, nonrandomized trial. Gerontol. Geriatr. Med. 4, 1–9. doi: 10.1177/2333721418783121
Snell, K. B., Mapes, F. M., Hickman, E. D., and Frisina, D. R. (2002). Word recognition in competing babble and the effects of age, temporal processing, and absolute sensitivity. J. Acoust. Soc. Am. 112, 720–727. doi: 10.1121/1.1487841
Strait, D., and Kraus, N. (2011a). Can you hear me now? Musical training shapes functional brain networks for selective auditory attention and hearing speech in noise. Front. Psychol. 2, 113. doi: 10.3389/fpsyg.2011.00113
Strait, D., and Kraus, N. (2011b). Playing music for a smarter ear: cognitive, perceptual and neurobiological evidence. Music Percept. 29, 133–146. doi: 10.1525/mp.2011.29.2.133
Sudarsonam, R., and Vaidyanath, R. (2019). Development of Dichotic Digit Test in Tamil. Unpublished Masters dissertation, Sri Ramachandra Institute of Higher Education and Research.
Sung, H.-C., Chang, A. M., and Lee, W.-L. (2010). A preferred music listening intervention to reduce anxiety in older adults with dementia in nursing homes. J. Clin. Nurs. 19, 1056–1064. doi: 10.1111/j.1365-2702.2009.03016.x
Sweetow, R. W., and Sabes, J. H. (2007). Listening and communication enhancement (LACE). Semin. Hear. 28, 133–141. doi: 10.1055/s-2007-973439
Tilli, T. M. (2021). Precision medicine: technological impact into breast cancer diagnosis, treatment and decision making. J. Person. Med. 11, 1348. doi: 10.3390/jpm11121348
Tiwari, S. (2003). Maturational Effect of Pitch Pattern Sequence Test. Mysore: All India Institute of Speech and Hearing. Available online at: http://203.129.241.86:8080/xmlui/handle/123456789/4490 (accessed May 8, 2023).
Tsoi, K. K. F., Chan, J. Y. C., Ng, Y. M., Lee, M. M. Y., Kwok, T. C. Y., and Wong, S. Y. S. (2018). Receptive music therapy is more effective than interactive music therapy to relieve behavioral and psychological symptoms of dementia: a systematic review and meta-analysis. J. Am. Med. Directors Assoc. 19, 568–576.e3. doi: 10.1016/j.jamda.2017.12.009
Tye-Murray, N., Sommers, M. S., Mauzé, E., Schroy, C., Barcroft, J., and Spehar, B. (2012). Using patient perceptions of relative benefit and enjoyment to assess auditory training. J. Am. Acad. Audiol. 23, 623–634. doi: 10.3766/jaaa.23.8.7
Vaidyanath, R. (2015). Effect of temporal processing training in older adults with temporal processing deficits. Unpublished Doctoral Thesis, University of Mysore.
Vaidyanath, R., and Yathiraj, A. (2014). Screening checklist for auditory processing in adults (SCAP-A): Development and preliminary findings. J. Hear. Sci. 4, 33–43. doi: 10.17430/890788
Van Os, J., Delespaul, P., Wigman, J., Myin-Germeys, I., and Wichers, M. (2013). Beyond DSM and ICD: introducing “precision diagnosis” for psychiatry using momentary assessment technology. World Psychiat. 12, 113–117. doi: 10.1002/wps.20046
Wayne, R. V., Hamilton, C., Jones Huyck, J., and Johnsrude, I. S. (2016). Working memory training and speech in noise comprehension in older adults. Front. Aging Neurosci. 8, 1–15. doi: 10.3389/fnagi.2016.00049
White-Schwoch, T., Carr, K. W., Anderson, S., Strait, D. L., and Kraus, N. (2013). Older adults benefit from music training early in life: biological evidence for long-term training-driven plasticity. J. Neurosci. 33, 17667–17674. doi: 10.1523/JNEUROSCI.2560-13.2013
World Health Organization (2019). Deafness and hearing loss. World Health Organization. Available online at: https://www.who.int/news-room/fact-sheets/detail/deafness-and-hearing-loss (accessed July 25, 2019).
Keywords: older adults, music-based intervention, computerized, auditory processing (AP), cognition, working memory, intervention
Citation: Ramadas V, Vaidyanath R, Uppunda AK and Viswanathan S (2023) Computerized music-based intervention module for auditory processing and working memory in older adults. Front. Comput. Sci. 5:1158046. doi: 10.3389/fcomp.2023.1158046
Received: 03 February 2023; Accepted: 05 June 2023;
Published: 20 June 2023.
Edited by:
Kat Agres, National University of Singapore, SingaporeReviewed by:
Usha Shastri, Kasturba Medical College, Mangalore, IndiaC. S. Vanaja, Bharati Vidyapeeth Deemed University, India
Copyright © 2023 Ramadas, Vaidyanath, Uppunda and Viswanathan. This is an open-access article distributed under the terms of the Creative Commons Attribution License (CC BY). The use, distribution or reproduction in other forums is permitted, provided the original author(s) and the copyright owner(s) are credited and that the original publication in this journal is cited, in accordance with accepted academic practice. No use, distribution or reproduction is permitted which does not comply with these terms.
*Correspondence: Ramya Vaidyanath, cmFteWF2YWlkeWFuYXRoQHNyaXJhbWFjaGFuZHJhLmVkdS5pbg==