- Phonetics Lab, Department of Linguistics, University of California, Davis, Davis, CA, United States
Text-to-speech (TTS) voices, which vary in their apparent native language and dialect, are increasingly widespread. In this paper, we test how speakers perceive and align toward TTS voices that represent American, British, and Indian dialects of English and the extent that social attitudes shape patterns of convergence and divergence. We also test whether top-down knowledge of the talker, manipulated as a “human” or “device” guise, mediates these attitudes and accommodation. Forty-six American English-speaking participants completed identical interactions with 6 talkers (2 from each dialect) and rated each talker on a variety of social factors. Accommodation was assessed with AXB perceptual similarity by a separate group of raters. Results show that speakers had the strongest positive social attitudes toward the Indian English voices and converged toward them more. Conversely, speakers rate the American English voices as less human-like and diverge from them. Finally, speakers overall show more accommodation toward TTS voices that were presented in a “human” guise. We discuss these results through the lens of the Communication Accommodation Theory (CAT).
1. Introduction
Linguistic accommodation is a phenomenon in which an interlocutor converges toward or divergences from another interlocutor's speech patterns (also known as alignment, mirroring, and imitation). According to Communication Accommodation Theory (Giles, 1973; Giles et al., 1991; CAT), accommodation is a strategic process that speakers use to serve both functional and social purposes. For example, converging toward another speaker can facilitate comprehension between two interlocutors (Audience Design, Clark and Murphy, 1982; Street and Giles, 1982; Thakerar et al., 1982; see also Interactive Account, Garrod and Pickering, 2007). This convergence happens for various linguistic features, such as vowel quality (Babel, 2010, 2012; Pardo et al., 2010; Walker and Campbell-Kibler, 2015), prosody (Bosshardt et al., 1997; D'Imperio and German, 2015; D'Imperio and Sneed, 2015), or syntax (Bock, 1986; Weatherholtz et al., 2014). Convergence can also create or maintain positive social ties and signal in-grouping with another interlocutor (see also Similarity Attraction Theory, Byrne, 1971; Giles et al., 1987). Multiple social factors have been shown to mediate phonetic alignment, including gender (Namy et al., 2002; Pardo, 2006), perceived attractiveness (Babel, 2012; Michalsky and Schoormann, 2017), and conversational roles (Pardo, 2006; Pardo et al., 2010; Zellou et al., 2021b). In many cases, both linguistic and social factors interact to create more nuanced patterns of phonetic alignment (Babel, 2010; Walker and Campbell-Kibler, 2015).
The current study focuses on inter-dialectal phonetic accommodation, or the extent that a speaker of one variety converges or diverges from the acoustic-phonetic features a speaker from another dialect produces. Dialects differ from one another in both linguistic features, such as phonetic distance, and social attitudes toward speakers, providing the opportunity to investigate the impact of phonetic and social factors on alignment patterns. This is an active area of research (Babel, 2010, 2012; Kim et al., 2011; Rao, 2013; Chakrani, 2015; Walker and Campbell-Kibler, 2015; Ross et al., 2021). Previous work in this area has found that phonetic distance between dialects can be a strong predictor of alignment patterns; however, the direction of the effect is mixed across studies. On the one hand, some studies have observed stronger convergence toward dialects that are more similar to the speakers' dialect, or have smaller phonetic distances (Kim et al., 2011; Rao, 2013). For example, Kim et al. (2011) studied convergence patterns between same- and different-dialect pairs and found that same-dialect pairs showed more convergence than different-dialect pairs, which the authors interpret as evidence that large phonetic distances discourage alignment. On the other hand, other studies have found that larger phonetic distances encourage alignment (Babel, 2010, 2012; Walters et al., 2013; Walker and Campbell-Kibler, 2015). For example, Walker and Campbell-Kibler (2015) also conducted a shadowing task with same- and different-dialectal pairs. Their results showed that shadowers converged more toward model talkers whose dialects had a larger phonetic distance from their own; additionally, shadowers converged more with lexical items whose vowels had greater variability between dialects. It is important to note that Walker and Campbell-Kibler (2015) used a difference-in-difference (DID) measure, which has been shown to be limited in its approach (e.g., Cohen Priva and Sanker, 2019); however, further analyses have found DID estimates to be useful when used alongside more holistic measures (Ross et al., 2021).
Moreover, some work has shown stronger convergence toward interlocutors who speak language varieties deemed more socially favorable (Chakrani, 2015), and in many cases, these social attitudes can mediate convergence motivated by linguistic factors (Babel, 2010; Weatherholtz et al., 2014; Clopper and Dossey, 2020; Ross et al., 2021). For instance, in a case study of speech in a natural conversational setting, Chakrani (2015) found overall convergence among Arabic speakers toward speakers of prestigious Mashreqi (Middle Eastern) dialects, and divergence away from speakers of non-prestigious Maghrebi (North African) dialects. Notably, convergence and divergence shifted over time throughout the course of the interactions, and divergence was triggered when social conversational norms were not followed.
Social perceptions additionally affect phonetic alignment patterns in situations where phonetic differences encourage alignment. For example, Babel (2010) studied convergence by New Zealand English (NZE) speakers toward Australian English (AuE) speakers and reported overall convergence; however, the extent of convergence was dependent on both phonetic distance and social factors. On the one hand, participants aligned more to vowels with larger phonetic distances from their own. On the other hand, social factors mediated both vowel-specific alignment and overall alignment. While speakers aligned more to vowels with large phonetic distances, this was only the case for AuE vowels that were not clearly identifiable as AuE by NZE speakers (cf. Hay et al., 2006). Vowels with recognizable differences were not imitated as much, suggesting that the social identities of NZE speakers affected alignment behavior at a subconscious level. NZE speakers' social attitudes toward AuE speakers, as measured by an Implicit Association Task, were also a significant predictor of overall alignment patterns, such that NZE speakers with pro-AuE scores were more likely to converge toward AuE speakers. Ross et al. (2021) further explored this phenomenon by conducting a shadowing task with talkers of the Mid-Atlantic and General American dialects. The authors used target words with phonetic variables differing between the two dialects, as well as words with no distinguishing dialect features as a baseline. Their results found that dialect-specific features facilitated convergence; however, this convergence was mediated by social beliefs, such that participants did not align toward stigmatized features in the Mid-Atlantic dialect. These findings were consistent with previous findings on alignment toward stigmatized features of dialects (e.g., Clopper and Dossey, 2020).
Recent research has begun to explore the phenomenon of linguistic accommodation in human–computer communication. The Computers Are Social Actors (CASA) theory posits that despite the top-down knowledge that they are communicating with a computer, humans still treat computers as social actors, and behave similarly toward them as they would another person (Nass et al., 1994). A large body of research supports CASA and has shown that humans show similar alignment patterns toward computers as they do in human-human communication (Bell et al., 2003; Branigan et al., 2003; Cohn et al., 2019; Zellou et al., 2021b). These alignment patterns are motivated by linguistic differences and happen at various levels, including syntactically (Branigan et al., 2003; Pearson et al., 2004), phonetically (Cohn et al., 2019; Gessinger et al., 2021; Zellou et al., 2021b), lexically (Branigan et al., 2011; Cowan et al., 2015), and prosodically (Bell et al., 2003; Suzuki and Katagiri, 2007). Current research has further investigated these phenomena by assessing vocal alignment toward voice-enabled digital assistants (voice artificial intelligence or voice-AI), such as Amazon's Alexa and Apple's Siri (Cohn et al., 2019, 2021; Zellou and Cohn, 2020; Zellou et al., 2021b; Aoki et al., 2022), and has found evidence that social factors, such as gender (Cohn et al., 2019; Snyder et al., 2019) and conversational role (Zellou et al., 2021b), additionally affect human-computer alignment.
An open question is whether alignment in human–voice-AI communication directly mirrors alignment patterns in human-human communication, or whether different strategies are applied with a device interlocutor. For example, Cohn et al. (2019) conducted a shadowing task with human and text-to-speech (TTS) model talkers (using Apple's Siri voices) and investigated gender-mediated phonetic alignment for both types of interlocutors. They found that male voices were imitated more than female voices for both human and device model talkers, indicating that similar gender-mediated social patterns of alignment are at play during shadowing with device talkers as with human talkers. However, gender had a larger effect on alignment toward human voices than alignment toward device voices, leading the authors to conclude that computers are not treated identically to other humans. This conclusion raised further questions about these differing alignment patterns: were participants showing different alignment patterns toward TTS voices due to the synthetic acoustic features of their voices, or due to the top-down knowledge that they are a device? Zellou and Cohn (2020) explored this question by presenting human and TTS voices in guises (e.g., TTS voice presented as a device or human) in a shadowing task. Their results demonstrated that speakers were more likely to align in vowel duration toward TTS voices when they were presented in a device guise. They also found, however, that voice type overall (human or device) was not a significant predictor of alignment patterns, suggesting that the acoustic differences between human and TTS voices were not the main driver of differences in alignment patterns. Other work has shown that people have distinct expectations about the communicative competence of technology; for example, participants explicitly rate a TTS voice as less competent and less human-like than a human voice (Cohn et al., 2022) and more robotic TTS voices as less competent, relative to more human-like TTS voices (Cowan et al., 2015; Zellou et al., 2021a). Additionally, given the identical guise for a talker-cued by an image of a human or device silhouette - listeners show worse performance on a speech-in-noise task (Aoki et al., 2022). Together, these findings suggest that top-down information about the speaker could shape communicative pressures in an interaction, such as leading to greater alignment toward apparent device addressees.
In many ways, interacting with spoken technology might parallel cross-dialectal communication; it is not uncommon for participants to select TTS voices from other dialects (e.g., American users choosing a British English Siri voice, Bilal and Barfield, 2021). Some prior work has examined cross-dialectal perceptions of these voices. For example, Tamagawa et al. (2011) tested how NZ English speakers' attitudes toward TTS voices in different dialects of English affected their overall rating of the quality of care in a healthcare setting. The authors found that US voices were rated as more robotic than NZ or British voices, and received lower performance ratings compared to NZ and British voices. The authors take this to be evidence that speakers will have lower-quality experiences with robots that are rated as having more robotic voices. They additionally hypothesized that the NZ voice was preferred due to its attractiveness as a local accent, and predicted that humans prefer TTS voices that are similar to their own accent, consistent with CAT. In another study, Cowan et al. (2015) tested lexical alignment between Irish English speakers and US and Irish English human and TTS voices in the form of a picture-naming task. Irish English-speaking participants were assigned either a human or computer partner who spoke either US or Irish English, and authors found that participants were more likely to select US lexical items when interacting with a US English-speaking partner, rather than selecting their standard Irish English lexical items. Interestingly, interlocutor type was not a significant predictor in the outcomes of the task, meaning this effect was consistent whether the partner was a human or a computer. The findings from these studies highlight gaps in understanding how dialectal differences mediate patterns of alignment in both human–human communication and human–technology communication.
1.1. Current study
The current study investigates patterns of phonetic alignment among US English speakers toward TTS voices in different dialects of English. We aim to examine how social factors – specifically, dialectal biases – contribute to alignment patterns toward apparent device and apparent human speakers. We conducted an interactive task in which participants produce target words presented in a list to an addressee. The target words were produced by the participants after hearing an interlocutor's production of that word. Addressees spoke three dialects of English: General American English, British English, and Indian English. We assessed dialectal biases by collecting social ratings for each voice from the participants after they completed their interaction with each voice. To further explore how the top-down knowledge of a talker's guise affects alignment, we presented two versions of our task: one in which all voices were presented in a device guise, and one in which all voices were presented in a human guise. We assessed convergence through an AXB perceptual rating task (Pardo, 2013; Pardo et al., 2017), in which an independent group of participants rate whether a participant's pre- or post-exposure production is more acoustically similar to the model talker's production.
Our study focused on three English dialects: General American English (US), British English (specifically, Received Pronunciation, a formal register of British English; RP), and Indian English (IN). Both RP and IN English differ from US English in vowel quality and vowel length, and IN English additionally differs from US English in voice-onset time (VOT) of word-initial stops (Awan and Stine, 2011). The target words selected for this study were chosen to emphasize the phonetic distance between US to another US speaker (no change), US to RP (small change), and US to IN English (larger change) (Bent et al., 2021). Relative to US English, the stimuli differ in either vowel length or quality in RP and/or IN English, and in VOT in IN English (Wells, 1982; Schmitt, 2007; Awan and Stine, 2011). The target words selected for this study are provided in Table 1.
To test the effect of social perceptions on alignment, we use perceived prestigiousness as a measure of social bias. Previous research has shown that RP English is typically perceived as highly prestigious, while IN English is seen as less prestigious (Giles, 1970, 1973; Coupland and Bishop, 2007). Thus, these three dialects were selected to create a comparison of a prestigious dialect and a non-prestigious dialect against a baseline comparator. Though historically RP has been presented as “prestigious” and IN as “non-prestigious,” we collected speaker-specific prestigiousness attitude ratings to determine our population-specific attitudes toward each dialect. If social biases prove to be strong predictors of alignment, we expect participants to align toward the dialect with the most positive ratings, and potentially diverge from the dialect with the lowest ratings.
For replicability and generalizability, all voices used in the current study were TTS voices generated from widely available systems (Amazon, Google, and Apple). We therefore vary whether the guise of the talker is congruent (shown an image of a device) or incongruent (shown an image of a human). If alignment is driven by functional reasons, we expect participants to align the most toward device-guise voices in an effort to communicate more effectively (Cowan et al., 2015; Cohn et al., 2022). Conversely, if alignment is driven by similarity attraction (Byrne, 1971), we might expect participants to align more toward human-guise voices (Gessinger et al., 2021).
In the following sections, we detail a norming study (Experiment 1, Section 2) in which we select the voices, the interactive speech production study (Experiment 2, Section 3), and the perceptual similarities study (Experiment 3, Section 4). Data for all three experiments, as well as supplementary data, are provided in an Open Science Framework repository for the project1.
2. Experiment 1: voice norming study
In order to select the voices to use in our experiment, we conducted a voice norming study online via Qualtrics. Our goal was to identify 6 voices (2 per dialect) with the most salient stereotypical accent (rated as being the “strongest” accent) and similar human-likeness ratings across voices.
2.1. Materials
We tested 9 US voices (4 Amazon, 2 Google, 3 Apple), 6 RP voices (2 Amazon, 2 Google, 2 Apple), and 5 IN voices (2 Amazon, 2 Google, 1 Apple). We used all female voices for the experiment to control for gender effects in alignment (Namy et al., 2002). We created an audio file for each voice, where the voice utilized a target stimulus in the form of a question (“The word, peak, is what number on your list?”), mirroring the presentation style of the stimuli in the subsequent interactive task. Recordings were produced using the Amazon Polly console through Amazon Web Services (AWS), the Google Actions console, and the command line on an Apple computer. All recordings were amplitude normalized to 65 dB.
2.2. Participants
Fifty five participants (48 female, 7 male; mean age: 20.3; SD: ± 2.0) completed the study. All participants were recruited from the University of California, Davis, psychology subjects pool, and received course credit for their participation. All participants reported English as their first language and no hearing impairments. The study was approved by the UC Davis institutional review board (IRB) and subjects completed informed consent before participating.
2.3. Procedure
For each voice, participants listened to the recorded audio file, then were asked to rate the voice on three dimensions - accent strength, perceived age, and human-likeness - on a sliding scale from 0 to 100 where every whole integer was a possible option. Each voice was presented one at a time, and participants rated each voice immediately after exposure. Voices were blocked by dialect and randomly presented within block, and participants rated all voices. Each participant additionally heard several listening comprehension questions, consisting of semantically unpredictable sentences produced by a human at a relatively lower intensity (45 dB), as an attention check.
2.4. Analysis and results
Mean ratings for each voice tested are reported in Supplementary Table 1 in Supplement A, and raw data are available in the OSF repository. Ratings provided by participants who failed the attention check were excluded, and the remaining ratings were combined to calculate an average for each voice based on accent strength, perceived age, and human-likeness. To ensure a strong indication of the voice's dialect, we selected the two TTS voices per dialect with the highest average ratings for accent strength. These voices also had roughly similar human-likeness scores, except for US voices which scored substantially lower overall on human-likeness (34.1 mean rating). Given these parameters, we selected the AWS Polly neural Salli (accent strength: 65.8; human-likeness: 62.5) and AWS Polly neural Joanna (accent strength: 66.9; human-likeness: 38.5) for the US dialect, AWS Polly Amy (accent strength: 61.0; human-likeness: 68.7) and Google's Google-GB2 (accent strength: 69.5; human-likeness: 52.0) for the RP dialect, and AWS Polly Aditi (accent strength: 58.2; human-likeness: 57.3) and Google's Google-IN1 (accent strength: 71.8; human-likeness: 64.1) for the IN dialect.
3. Experiment 2: interactive task
Our interactive task was designed to approximate a turn-taking conversation in which speakers repeated a word after hearing a model talker say it, but in a controlled communicative context (e.g., “The word, peak, is what number on your list?' “The word peak is number five.”).
3.1. Materials
Twenty single-word target words were selected using the dialectal criteria discussed in the introduction; namely, we selected target words that differed in either vowel length, vowel quality, or VOT between two or more dialects. The items with their lexical sets and targeted differing features by dialect are listed in Table 1. We specifically selected monosyllabic words with CV(c)c structure, and that were low-frequency (mean zipf value: 2.98); high-frequency items are less susceptible to imitation (Brysbaert and New, 2009). Each stimulus was presented in a sentence (e.g., “the word, beak, is what number on your list?”) to avoid over-emphasis in pronunciation, and to simulate a conversational format for the interactive experiment.
Similar to the stimuli creation for the voice norming study, stimuli were created using AWS and the Google Actions console. For each voice, we generated individual recordings for each stimulus within a sentence and other conversational snippets to help promote the feeling of an interactive conversation, such as introductory remarks (e.g., “Hello, my name is Rebecca. Let's get started”), using a prototypical, culturally-appropriate woman's name for each voice (see Supplementary Table 2 in Supplement B for a full list of utterances). Each voice had 6 possible pseudorandomized lists for stimuli presentation. The final product for each version of each voice was a single audio file that started with an introduction, looped through all 20 stimuli, providing the participant 3s to respond to each query, and ended with a closing remark to signal the end of the interaction. All stimuli were amplitude normalized to 65 dB.
3.2. Participants
Sixty participants (all female; mean age: 19.3, SD: ± 2.1) completed the study; 30 were assigned to the condition with the human guise, and 30 were assigned to the condition with the device guise in a between-subjects design. All participants were recruited from the University of California, Davis, psychology subjects pool, and received course credit for their participation. Students who participated in the voice norming study (experiment 1) were excluded from participating in the interactive task. All participants reported English as their first language and no hearing impairments. The study was approved by the UC Davis institutional review board (IRB) and subjects completed informed consent before participating.
3.3. Procedure
The experiment was conducted online via Qualtrics, and participants' recordings were captured with Pipe2. Participants were told that they would be taking part in an interactive experiment and would be communicating with a series of speakers. Before the start of the experiment, we asked participants to read a list of 20 sentences, each of which included the target words (e.g., “The word beak is a rhyme with seek”). These recordings served as pre-exposure baseline productions for analysis.
To investigate the question of whether apparent humanity affects alignment patterns in human–voice-AI communication, our experiment contained a between-subjects guise manipulation shown either as a smart speaker (device guise) or a human talker (human guise) (see Figure 1). To elicit this top-down knowledge of interlocutor guise, the silhouette of either a smart speaker or a silhouette of a woman was shown throughout the experimental trials.
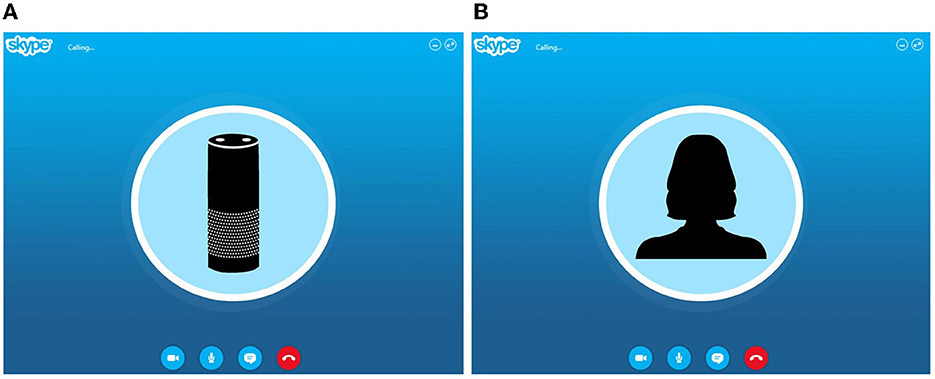
Figure 1. The depiction of a device-guise voice (A) and a human-guise voice (B). Participants were told they would be connecting with an interlocutor over Skype to elicit more natural-sounding, conversational interactions.
Each interaction started with a Skype sound to simulate “connecting” with the model talker. After the “connection” was established, the model talker introduced themself and initiated a series of questions (schematized in Figure 2). The talker would first ask a question (e.g., “The word, cog, is what number on your list?”), to which the participant would respond using a templated response shown on the screen (e.g., “The word cog is number one.”), given 3s to respond. Participants were explicitly instructed to read the templated response provided on the screen to ensure that they used the stimulus in their response. The talker would verbally acknowledge the response (e.g., “Awesome”), then move on to the next question. This question was repeated once for each stimulus (20 total). Participants interacted with all 6 voices for a total of 120 post-exposure productions (20 stimuli × 6 voices). Participants interacted with each voice for approximately 4 min. Voices were blocked by dialect and randomly presented within block, and dialect blocks were randomly presented.
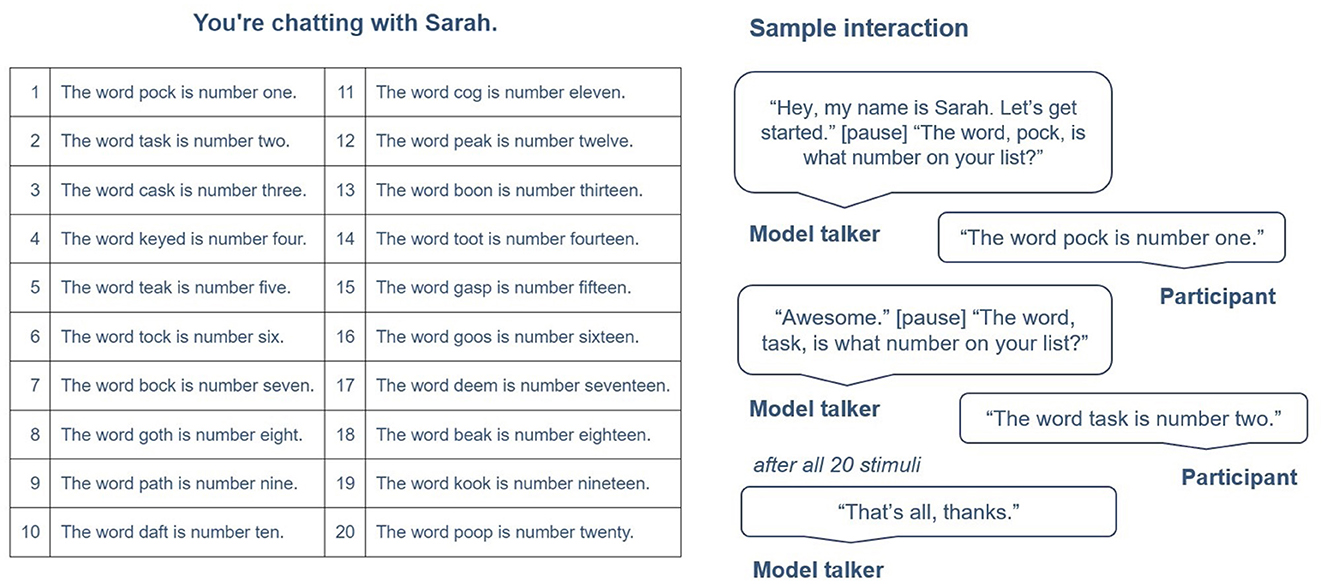
Figure 2. An example of the templated responses presented to each participant during the interactive task (in addition to the depiction of the voice shown in Figure 1), along with a sample interaction.
At the end of each interaction, a new block of questions appeared in which participants were asked questions about their experience with the talker. They were instructed to “please answer the following questions about your experience with [name]”, where the blank indicated the assigned name of the voice. First, they were asked to identify the talker's nationality (Where do you think [name] was from?) from a set of options3; this question was designed to test whether participants could identify the regional origin of the speaker's dialect. Next, participants provided ratings using sliding scales (0–100 where every whole integer was a possible option) to assess other socio-indexical features of the talker's voice. Based on the literature examining prestige in human-human interaction (e.g., Cargile and Bradac, 2001; Fuertes et al., 2012; McCullough et al., 2019), we asked participants to rate the talker's perceived intelligence [How intelligent did (name) seem? (0 = unintelligent to 100 = intelligent)] and perceived socioeconomic status [What do you think is (name)'s socioeconomic status? (0 = poor to 100 = wealthy)]. For example, socioeconomic status was included in order to gauge potential social biases toward speakers of different dialects of English (Dragojevic, 2017), such that low socioeconomic status scores would suggest more negative biases toward a speaker. In order to investigate social closeness, a factor shown to influence alignment (e.g., Giles et al., 1991), we also asked them to rate the talker's friendliness [How friendly was (name)? (0 = unfriendly to 100 = very friendly)]. Finally, as all voices were TTS voices, we asked them to rate the naturalness/human-likeness of the talker's voice [How natural does (name) sound? (0 = robotic to 100 = natural)]. In particular, naturalness was included to investigate whether voices presented in an inauthentic guise as a human were rated as less natural than those presented in an authentic guise (cf. Zellou and Cohn, 2020). Furthermore, previous literature has shown that speakers have more positive attitudes toward TTS voices that are rated as less robotic (Tamagawa et al., 2011).
3.4. Social ratings analysis and results
Participants successfully identified the dialect of US and IN voices (86 and 90% accuracy respectively), but showed lower accuracy in identifying the RP voices (74% accuracy). Of the incorrect answers, 17% of respondents labeled the RP voices as either Australian or New Zealander, both closely related dialects to RP English. Despite this discrepancy in accuracy for RP voices, participants reported that they were, on average, roughly equally familiar with British and Indian English accents (RP familiarity: 67.6, se: ± 3.2; IN familiarity: 65.6, se: ± 3.7). Participants additionally reported high familiarity with US accents (89.3; se: ± 2.4).
We modeled participants' ratings of the voices' naturalness, friendliness, intelligence, and socioeconomic status in separate linear regression models; mean ratings are shown in Figure 3. We additionally modeled a composite attitude rating for each voice by participant, which was calculated by averaging friendliness, intelligence, and socioeconomic status ratings. For each model, fixed effects included Dialect, which was sum coded with three contrasts (IN: 1, 0; RP: −1, −1; US: 0, 1), and Guise, which was sum coded with two contrasts (human: 1, device: −1), as well as their interaction. Random effects included by-Participant random intercepts. Model estimates for fixed effects are reported in Tables 2–6. No estimates for two-way interactions reached significance and are therefore reported in Supplement C in Supplementary Tables 3–7.
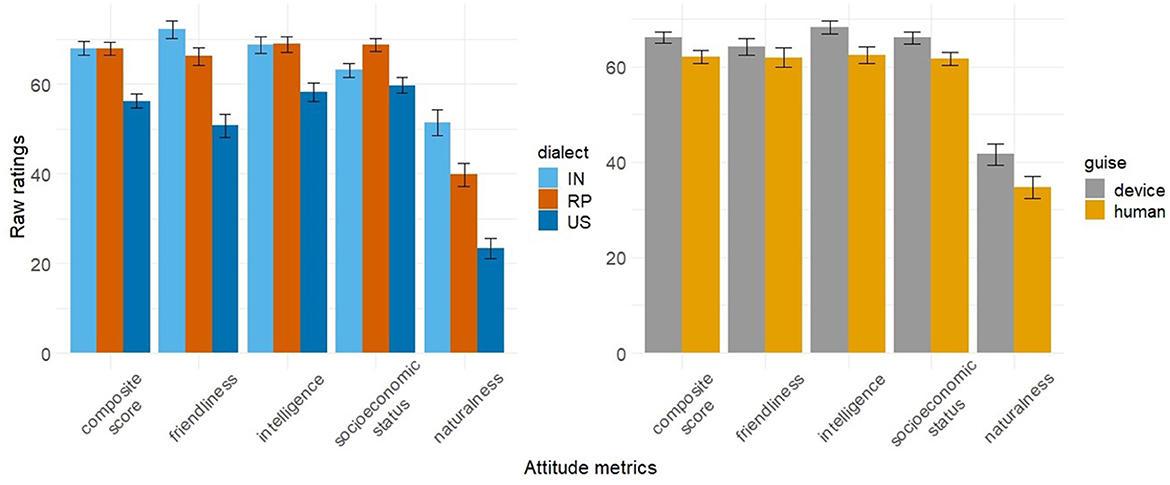
Figure 3. Average attitude ratings by dialect and guise with standard error bars. The composite score is an average of friendliness, intelligence, and perceived socioeconomic status rating by participant per voice.
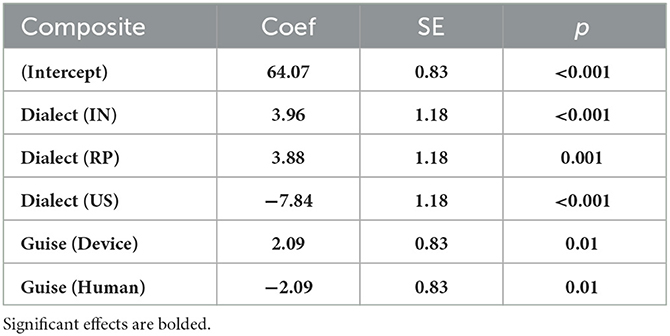
Table 2. Model estimates for composite attitude scores linear regression, including re-leveled fixed effects.
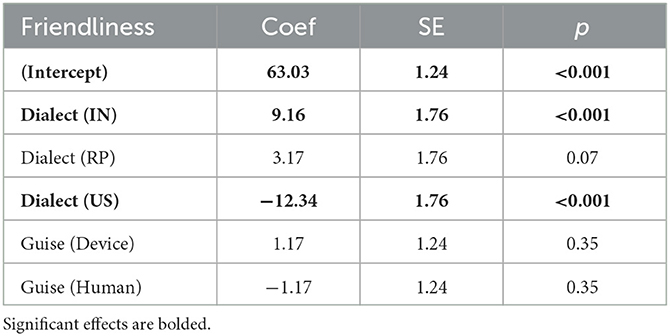
Table 3. Model estimates for friendliness scores linear regression, including re-leveled fixed effects.
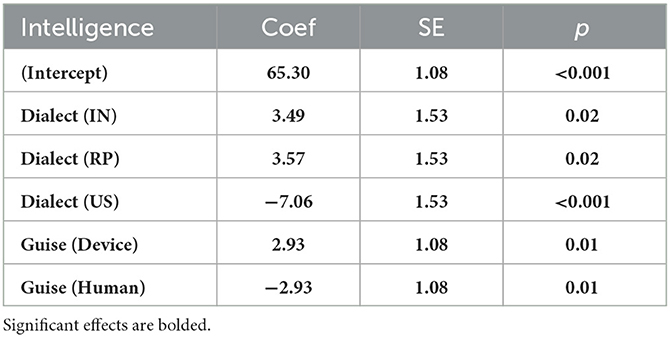
Table 4. Model estimates for intelligence scores linear regression, including re-leveled fixed effects.
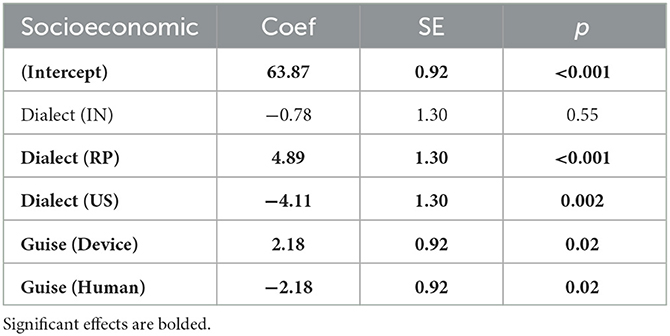
Table 5. Model estimates for perceived socioeconomic status scores linear regression, including re-leveled fixed effects.
Our first model evaluated composite attitude scores. Model estimates, reported in Table 2, showed significant effects by both Dialect and Guise. As seen in Figure 3, IN and RP voices had significantly higher composite attitude scores (68.0; se: ± 1.5 and 67.9; se: ±1.4 respectively) than US voices (56.2; se: ± 1.5). Voices presented in an authentic guise, or as a device, also had significantly higher composite attitude scores (66.2; se: ± 1.1) than those presented in an inauthentic guise, as a human (62.0; se: ± 1.4). There were no interactions between Dialect and Guise.
Our subsequent models evaluated the individual scores for friendliness, intelligence, socioeconomic status, and naturalness separately (model outputs in Tables 3–6). The models revealed that, on average, IN voices were rated significantly higher in friendliness, intelligence, and naturalness. RP voices were also rated as having higher intelligence and socioeconomic status. US voices were rated significantly lower across all categories assessed. Voices presented in an authentic guise, as a device, received significantly higher scores in intelligence, socioeconomic status, and naturalness than voices presented in an inauthentic guise, as a human.
4. Experiment 3: AXB perceptual similarity rating task
To holistically evaluate whether speakers from Experiment 2 converged toward or diverged from TTS voices, we conducted an AXB similarity rating task (Pardo, 2013; Pardo et al., 2017). The purpose of this task is to have participants rate whether the pre- or post-exposure production was more similar to the model talker voice.
4.1. Materials
Of the 60 participants from Experiment 2, 14 were excluded for producing multiple speech production errors, issues with sound quality, or incorrect completion of the task; thus, 46 total interaction participants were rated, balanced across guise. Stimuli consisted of the shadowers' baseline production of the words, the model talkers' production of the target word, and the participants' post-exposure production. To generate the AXB stimuli, we created 23 pseudorandomized lists, balancing the order of baseline and post-exposure as the 1st or 3rd sound. We concatenated the productions with 0.25 s of silence. Each word recording was amplitude normalized to 65 dB. Productions that contained artifacts or mispronunciations (e.g., “cook” [kk] for “kook” [kuk]) were excluded.
4.2. Participants
One hundred and twenty four participants (92 female, 28 male, 4 gender queer; mean age: 19.5, SD: ± 2.9) completed the AXB study. All participants were recruited from the University of California, Davis, psychology subjects pool, and received course credit for their participation. Students who participated in the norming (experiment 1) or interactive tasks (experiment 2) were excluded from participating in the AXB rating task. All participants reported English as their first language and no hearing impairments. The study was approved by the UC Davis institutional review board (IRB) and subjects completed informed consent before participating.
4.3. Procedure
The experiment was conducted online via Qualtrics. For each trial, the raters heard one AXB stimulus, where A and B were either the pre- or post-exposure interactive participant's production (balanced across stimuli) and X was the model talker production. Raters were then asked to rate whether the 1st or the 3rd production sounded more like the 2nd. We provided an additional option for “N/A; audio artifact or technical difficulty” in case raters experienced technical difficulties. 23 lists consisting of productions from 2 interactive participants each were randomly presented. Each rater evaluated all productions in one list, resulting in an average of 480 total ratings (2 participants × 240 productions). In total, each participant's productions were rated by at least 4 raters.
4.4. Analysis and results
Mean perceived similarity (assessing convergence) by dialect and guise as rated in the AXB task are shown in Figure 4. We modeled AXB responses with a mixed-effects logistic regression analysis using the brms package in R (Bürkner, 2018). The responses to the perceptual similarity task were coded as a binary variable based on whether the rater in the AXB task identified the post-exposure production as the one most like the model talker (=1) or not. Attitude was calculated as a composite score across friendliness, intelligence, and socioeconomic status ratings for each voice by speaker, and this score was standardized. The model included fixed effects for Dialect (three levels: IN, US, and RP), Guise (2 levels: human and device), Composite Attitude (continuous, standardized), Naturalness Rating (continuous, standardized), including all two-, three- and four-way interactions. The three levels for Dialect were sum coded with three contrasts (IN: 1, 0; RP: −1, −1; US: 0, 1), and the two levels for Guise were sum coded with two contrasts (human: 1, device: −1). We also included random intercepts by Participant, Model Talker, Rater, and Word. Finally, we included by-Participant random slopes for Dialect, Attitude, and Naturalness and all possible 2- and 3-way interactions between them. This model led to several divergent transitions in the sampling process. Our final model included all of the above parameters without random intercepts by model talker. The final random effects structure is provided in Equation 1, and model fixed effects posterior distributions are plotted in Figure 5. We consider the model estimates as reliable if the credible interval (CI) does not include 0, or over 95% of the sampled posterior distribution is over or under 0 in the predicted direction.
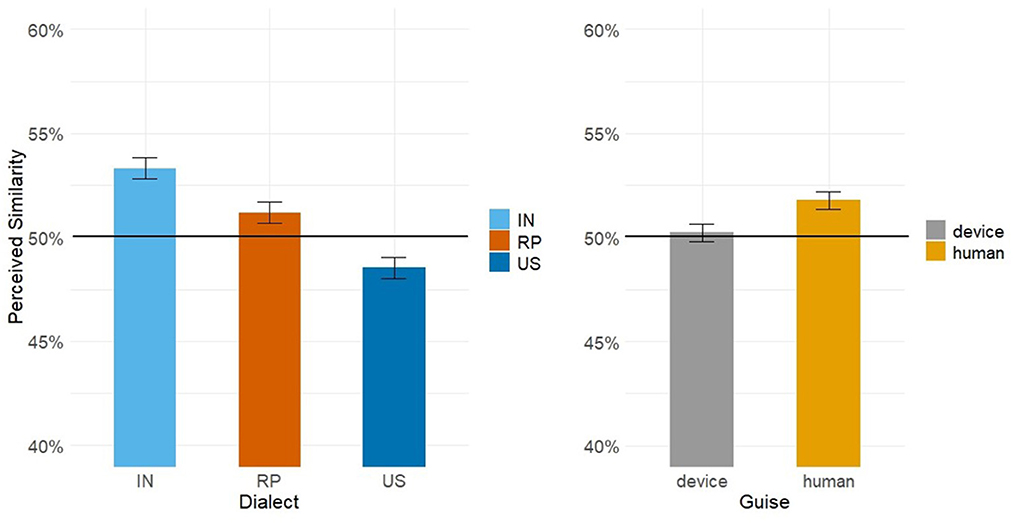
Figure 4. Mean perceived similarity by dialect and guise with standard error bars. The black line at 50% represents chance convergence.
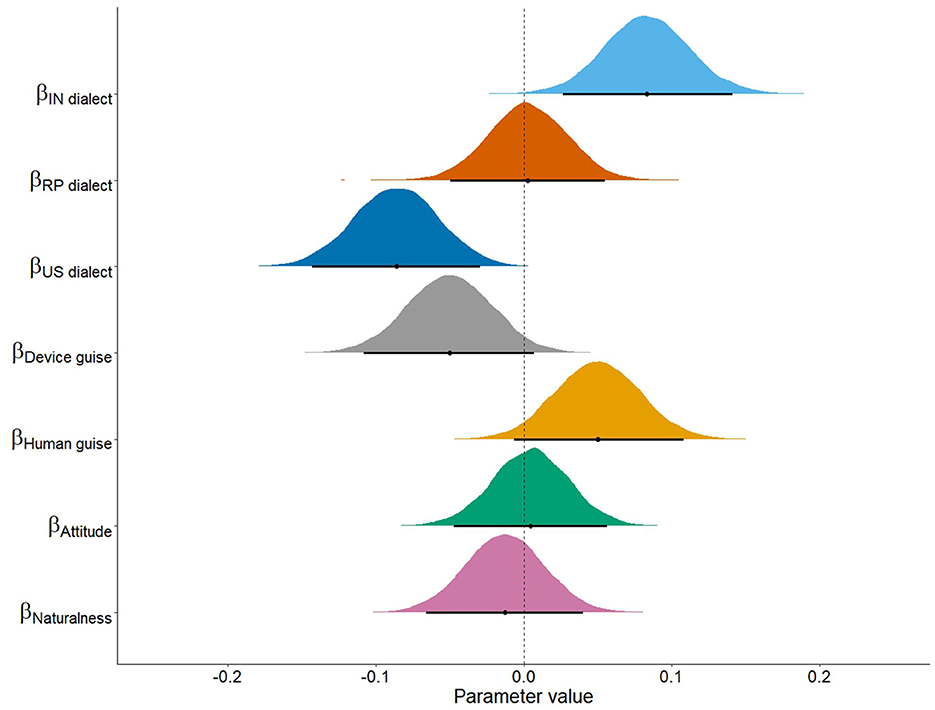
Figure 5. Posterior distributions for the fixed effects of the logistic regression. Means for each parameter are plotted as black dots along with their 95% credible intervals.
Our model estimates by Dialect were reliable for the IN dialect and US dialect; we see reliable convergence to the IN voices and divergence from the US voices. Attitude and Naturalness scores, however, were not reliable predictors of convergence patterns. We also observed an effect of Guise, with greater convergence toward voices in the apparent human guise. Model estimates are summarized in Table 7. Estimates for two-, three-, and four-way interactions were not reliable and are thus listed in Supplement C in Supplementary Table 8.
5. Discussion
This research aimed to examine how speakers phonetically accommodate cross-dialectal interlocutors while employing human-computer communication as a case study. The current study tested how social biases affect alignment patterns in human communication with voice-AI, and additionally explored the effect of top-down knowledge (i.e., talker humanity) on alignment patterns. We asked participants to interact with TTS voices in US, British, and Indian dialects of English, which vary in phonetic distance from one another and perceived social prestige. We evaluated the effect of humanity by deploying two versions of our experiment: one in which the TTS voices were presented in an authentic guise, and one in which the TTS voices were presented as humans.
Our results revealed that participants aligned the most toward IN voices, and diverged from US voices. We predicted that speakers would align the most toward RP voices and the least toward IN voices, with US voices acting as a baseline. These predictions were based on previous research on attitudes toward standard British dialects and Indian dialects of English (Giles, 1970, 1973; Coupland and Bishop, 2007), which stated that standard British dialects were typically seen as prestigious, and Indian dialects were seen as less prestigious. However, attitude scores revealed that our participant pool does not hold these same beliefs: IN voices scored the highest for friendliness, tied with RP voices for intelligence, and scored the second highest for perceived socioeconomic status, resulting in the highest average composite attitude score. Thus, given these attitude scores, speakers phonetically converged toward voices with the highest attitudinal ratings (the IN voices), and phonetically diverged from the voices with the lowest attitudinal ratings (the US voices). These alignment patterns are in line with predictions from CAT, which asserts that speakers will converge toward another interlocutor that they have positive social feelings toward (Giles et al., 1987, 1991). US voices were additionally rated as being less natural (i.e., more robotic), in line with participant ratings of US TTS voices in Tamagawa et al. (2011). Despite this pattern, our statistical analysis found that attitude scores were not a reliable predictor of alignment patterns in our data above-and-beyond the dialect patterns, suggesting that speakers are relatively consistent in both ratings and alignment.
While tentative, another possible explanation for greater alignment toward Indian English is phonetic distance. Recent work has shown a greater number of phonetic “edits” from American English varieties to Hindi-English than to RP (Bent et al., 2021). In our stimuli, many items contained a contrast in VOT in addition to a contrast in vowel length or quality, meaning that there was more space for US speakers to converge toward IN voices than RP voices. Previous research has found that large phonetic distances encourage alignment (e.g., Walker and Campbell-Kibler, 2015), but others have claimed that large phonetic distances lead to less alignment between dialects of the same language (e.g., Kim et al., 2011). Future work performing an acoustic analysis to measure the difference in pre- and post-exposure productions of the stimuli can further explore this question.
Another possible explanation for these results is that the novelty of an IN-accented voice led participants to pay more attention to, and thus converge more toward, IN voices. Previous research has found that novel voices attract more attention, and increased attention toward an interlocutor's voice might lead to more convergence (e.g., Babel et al., 2014). One of the questions we asked each participant in the exit survey was, “Rate how familiar you are with (American, British, Indian) English accents.” We found that average familiarity ratings for interactive participants were similar for British and Indian accents. However, this question targeted participants' experiences with these accents in general; thus, it is possible that a participant could be familiar with a given accent, but not within a voice-AI context.
We additionally found an effect of model talker humanity on phonetic alignment patterns, such that speakers aligned more toward voices presented as a human than as a device. We predicted that one of two phenomena could happen with alignment toward voices in different guises: participants would either align more toward device-guise voices in an effort to facilitate communication (Cowan et al., 2015; Cohn et al., 2022; given prior beliefs about TTS voices being less competent) or align more toward human-guise voices, in line with Similarity Attraction Theory (Byrne, 1971). Our results demonstrate that participants reliably align toward human-guise voices, indicating that the top-down knowledge of another speaker's identity is sufficient to affect alignment patterns, despite similar bottom-up acoustic features. These findings also suggest that social factors may play a stronger role than functional factors in alignment patterns with TTS voices. Our findings are in line with similar studies by Gessinger et al. (2021) and Aoki et al. (2022), but contra Zellou and Cohn (2020). Despite aligning more toward human-guise voices, human-guise voices had lower average naturalness, friendliness, intelligence, and socioeconomic status scores than device-guise voices, suggesting that TTS voices presented in an inauthentic guise trigger feelings of disgust or discomfort, in line with a possible Uncanny Valley effect (Mori, 1970; Mitchell et al., 2011).
The findings of this research have both theoretical impacts for the fields of linguistics and communication, but also tangible implications for our understanding of dyadic and group intercultural communication. Our research builds upon previous findings in human-human communication and demonstrates that speakers have different phonetic accommodation patterns in intra- and inter-dialectal dyads in human-computer communication. As the use of virtual assistants becomes more commonplace in professional group settings, understanding how humans perceive and interact with cross-cultural voices can inform improvements in virtual assistant technology and lead to more productive uses for teams.
5.1. Limitations and future directions
This study has several limitations that can serve as avenues for future research. First, we exclusively used TTS voices. Future work using human voices—and comparing them to TTS voices—can shed further insight into both dialect-level effects, as well as the role of guise in shaping ratings and accommodation behaviors. Relatedly, we used just two ‘exemplars' of speakers from each dialect category, all of whom were female voices; future work using a wider variety of dialects, as well as speaker characteristics (e.g., varying in age, gender, race/ethnicity) can probe how social attitudes shape cross-cultural speech interactions more broadly. Another limitation of our study was that participants had difficulty accurately identifying RP voices compared to US or IN voices (74% accuracy vs. 86 and 90% respectively). Future studies could more strongly signal dialect, perhaps using country flags alongside model talker images, to avoid misidentification of each dialect.
Another possible limitation in our study design was in the question selection for gauging social and dialectal biases. It is possible that an assessment such as the Implicit Association Task (Babel, 2010) would yield better measurements for implicit biases than questions that draw the participants' attention to what is being rated. Furthermore, our ratings also asked participants to rate the talkers' socio-indexical features on a sliding scale from 0 to 100, building off of related work assessing human and TTS voices (Cohn et al., 2020; Zellou and Cohn, 2020). However, it is possible that participants vary in the way they interpret the 100-point scale; future work using Likert responses with defined categories could reduce cognitive load and better reflect differences across participants (for a discussion, see Ouwehand et al., 2021).
Finally, another consideration for future research is to investigate individual differences in alignment patterns. For example, conducting individual analyses of participants' phonetic spaces prior to measuring alignment could more thoroughly investigate differences in phonetic distance or reveal speaker-specific alignment patterns.
6. Conclusion
This research tested how social attitudes mediated human–voice-AI alignment patterns to TTS voices in dialects of English. We found that participants converged the most toward the dialect with the highest attitude ratings – the Indian English dialect. We hypothesize that linguistic factors, such as phonetic distance, may additionally contribute to alignment patterns in our data. We additionally found that participants converged more toward TTS voices presented as a human than TTS voices presented as a device. Taken together, these findings reveal a rich interplay of social factors in cross-dialectal speech interactions, which will be even more relevant with advances in computer-mediated and technology-directed communication.
Data availability statement
The datasets presented in this study can be found in online repositories. The names of the repository/repositories and accession number(s) can be found at: https://doi.org/10.17605/OSF.IO/U3JQE.
Ethics statement
The studies involving human participants were reviewed and approved by the UC Davis Institutional Review Board. The patients/participants provided their written informed consent to participate in this study.
Author contributions
ND, MC, and GZ contributed to the conception and design of the study. ND and MC programmed the experiments. ND performed data cleaning and statistical analysis. All authors contributed to manuscript drafting, revision, read, and approved the submitted version.
Funding
This research was supported by the National Science Foundation SBE Postdoctoral Research Fellowship to MC under grant no. 1911855 and an Amazon research grant to GZ.
Conflict of interest
The authors declare that the research was conducted in the absence of any commercial or financial relationships that could be construed as a potential conflict of interest.
Publisher's note
All claims expressed in this article are solely those of the authors and do not necessarily represent those of their affiliated organizations, or those of the publisher, the editors and the reviewers. Any product that may be evaluated in this article, or claim that may be made by its manufacturer, is not guaranteed or endorsed by the publisher.
Supplementary material
The Supplementary Material for this article can be found online at: https://www.frontiersin.org/articles/10.3389/fcomp.2023.1204211/full#supplementary-material
Footnotes
1. ^https://doi.org/10.17605/OSF.IO/U3JQE
3. ^Options: Australia, Canada, India, Ireland, New Zealand, South Africa, United Kingdom, United States of America, Other/Non-identifiable.
References
Aoki, N. B., Cohn, M., and Zellou, G. (2022). The clear speech intelligibility benefit for text-to-speech voices: effects of speaking style and visual guise. JASA Exp. Lett. 2, 045204. doi: 10.1121/10.0010274
Awan, S., and Stine, C. (2011). Voice onset time in Indian English-accented speech. Clin. Ling. Phonetics 25, 998–1003. doi: 10.3109/02699206.2011.619296
Babel, M. (2010). Dialect divergence and convergence in New Zealand English. Lang. Soc. 39, 437–456. doi: 10.1017/S0047404510000400
Babel, M. (2012). Evidence for phonetic and social selectivity in spontaneous phonetic imitation. J. Phon. 40, 177–189. doi: 10.1016/j.wocn.2011.09.001
Babel, M., McGuire, G., Walters, S., and Nicholls, A. (2014). Novelty and social preference in phonetic accommodation. Lab. Phonol. 5, 123–150. doi: 10.1515/lp-2014-0006
Bell, L., Gustafson, J., and Heldner, M. (2003). Prosodic adaptation in human-computer interaction. Proc. ICPHS 3, 833–836.
Bent, T., Holt, R. F., Engen, K. J., van Jamsek, I. A., Arzbecker, L. J., Liang, L., and Brown, E. (2021). How pronunciation distance impacts word recognition in children and adults. J. Acous. Soc. Am. 150, 4103. doi: 10.1121/10.0008930
Bilal, D., and Barfield, J. K. (2021). Hey there! what do you look like? user voice switching and interface mirroring in voice-enabled digital assistants (VDAs). Proc. Assoc. Inf. Sci. Technol. 58, 1–12. doi: 10.1002/pra2.431
Bock, J. K. (1986). Syntactic persistence in language production. Cogni. Psychol. 18, 355–387. doi: 10.1016/0010-0285(86)90004-6
Bosshardt, H. G., Sappok, C., Knipschild, M., and Hölscher, C. (1997). Spontaneous imitation of fundamental frequency and speech rate by nonstutterers and stutterers. J. Psycholing. Res. 26, 425–448. doi: 10.1023/A:1025030120016
Branigan, H., Pickering, M., Pearson, J., McLean, J., and Nass, C. (2003). “Syntactic alignment between computers and people: the role of belief about mental states,” in Proceedings of the Twenty-fifth Annual Conference of the Cognitive Science Society. Hillsdale, NJ, USA: Lawrence Erlbaum Associate, 186–191.
Branigan, H. P., Pickering, M. J., Pearson, J., McLean, J. F., and Brown, A. (2011). The role of beliefs in lexical alignment: Evidence from dialogs with humans and computers. Cognition 121, 41–57. doi: 10.1016/j.cognition.2011.05.011
Brysbaert, M., and New, B. (2009). Moving beyond Kučera and Francis: a critical evaluation of current word frequency norms and the introduction of a new and improved word frequency measure for American English. Behav. Res. Methods 41, 977–990. doi: 10.3758/BRM.41.4.977
Bürkner, P. C. (2018). Advanced Bayesian multilevel modeling with the R package brms. R J. 10, 395–411. doi: 10.32614/RJ-2018-017
Cargile, A. C., and Bradac, J. J. (2001). Attitudes toward language: a review of speaker-evaluation research and a general process model. Annal. Int. Commun. Assoc. 25, 347–382. doi: 10.1080/23808985.2001.11679008
Chakrani, B. (2015). Arabic interdialectal encounters: Investigating the influence of attitudes on language accommodation. Lang. Commun. 41, 17–27. doi: 10.1016/j.langcom.2014.10.006
Clark, H. H., and Murphy, G. L. (1982). Audience design in meaning and reference. Adv. Psychol. 9, 287–299. doi: 10.1016/S0166-4115(09)60059-5
Clopper, C. G., and Dossey, E. (2020). Phonetic convergence to Southern American English: Acoustics and perception. J. Acous. Soc. Am. 147, 671. doi: 10.1121/10.0000555
Cohen Priva, U., and Sanker, C. (2019). Limitations of difference-in-difference for measuring convergence. Lab. Phonol. 10, 1–29. doi: 10.5334/labphon.200
Cohn, M., Ferenc Segedin, B., and Zellou, G. (2019). “Imitating siri: socially-mediated vocal alignment to device and human voices,” in Proceedings of the Annual Conference of the International Speech Communication Association, INTERSPEECH. University of California, Davis, 1813–1817.
Cohn, M., Jonell, P., Kim, T., Beskow, J., and Zellou, G. (2020). “Embodiment and gender interact in alignment to TTS voices,” in Proceedings of the Cognitive Science Society (Montreal, QC), 220–226.
Cohn, M., Predeck, K., Sarian, M., and Zellou, G. (2021). Prosodic alignment toward emotionally expressive speech: comparing human and Alexa model talkers. Speech Commun. 135, 66–75. doi: 10.1016/j.specom.2021.10.003
Cohn, M., Segedin, B. F., and Zellou, G. (2022). Acoustic-phonetic properties of Siri- and human-directed speech. J. Phonetics 90, 101123. doi: 10.1016/j.wocn.2021.101123
Coupland, N., and Bishop, H. (2007). Ideologised values for british accents. J. Socioling. 11, 74–93. doi: 10.1111/j.1467-9841.2007.00311.x
Cowan, B. R., Branigan, H. P., Obregón, M., Bugis, E., and Beale, R. (2015). Voice anthropomorphism, interlocutor modelling and alignment effects on syntactic choices in human–computer dialogue. Int. J. Hum. Comput. Studies 83, 27–42. doi: 10.1016/j.ijhcs.2015.05.008
D'Imperio, M., and German, J. S. (2015). “Phonetic detail and the role of exposure in dialect imitation,” in Proceedings of the 18th International Congress of Phonetic Sciences (Glasgow).
D'Imperio, M. D., and Sneed, J. (2015). Phonetic Detail and the Role of Exposure in Dialect Imitation. 18th International Congress of Phonetic Sciences.
Dragojevic, M. (2017). Language Attitudes. Oxford Research Encyclopedia of Communication. Oxford: Oxford University Press.
Fuertes, J. N., Gottdiener, W. H., Martin, H., Gilbert, T. C., and Giles, H. (2012). A meta-analysis of the effects of speakers' accents on interpersonal evaluations. Eur. J. Soc. Psychol. 42, 120–133. doi: 10.1002/ejsp.862
Garrod, S., and Pickering, M. (2007). Alignment in dialogue. Oxford Handb. Psycholing. 5, 1–16. doi: 10.1093/oxfordhb/9780198568971.013.0026
Gessinger, I., Raveh, E., Steiner, I., and Möbius, B. (2021). Phonetic accommodation to natural and synthetic voices: behavior of groups and individuals in speech shadowing. Speech Commun. 127, 43–63. doi: 10.1016/j.specom.2020.12.004
Giles, H. (1970). Evaluative reactions to accents. Educ. Rev. 41, 211–227. doi: 10.1080/0013191700220301
Giles, H., Coupland, J., and Coupland, N. (1991). Accommodation theory: communication, context, and consequences. Contexts Accommod. 14, 1–68. doi: 10.1017/CBO9780511663673.001
Giles, H., Mulac, A., Bradac, J. J., and Johnson, P. (1987). Speech accommodation theory: the first decade and beyond. Annal. Int. Commun. Assoc. 10, 13–48. doi: 10.1080/23808985.1987.11678638
Hay, J., Nolan, A., and Drager, K. (2006). From fush to feesh: exemplar priming in speech perception. Ling. Rev. 23, 351–379. doi: 10.1515/TLR.2006.014
Kim, M., Horton, W. S., and Bradlow, A. R. (2011). Phonetic convergence in spontaneous conversations as a function of interlocutor language distance. Lab. Phonol. 2, 125–156. doi: 10.1515/labphon.2011.004
McCullough, E. A., Clopper, C. G., and Wagner, L. (2019). The development of regional dialect locality judgments and language attitudes across the life span. Child Dev. 90, 1080–1096. doi: 10.1111/cdev.12984
Michalsky, J., and Schoormann, H. (2017). Pitch convergence as an effect of perceived attractiveness and likability. Proc. Interspeech 2253–2256. doi: 10.21437/Interspeech.2017-1520
Mitchell, W. J., Szerszen Sr, K. A., Lu, A. S., Schermerhorn, P. W., Scheutz, M., and MacDorman, K. F. (2011). A mismatch in the human realism of face and voice produces an uncanny valley. i-Perception 2, 10–12. doi: 10.1068/i0415
Namy, L. L., Nygaard, L. C., and Sauerteig, D. (2002). Gender differences in vocal accommodation: the role of perception. J. Lang. Soc. Psychol. 21, 422–432. doi: 10.1177/026192702237958
Nass, C., Steuer, J., and Tauber, E. (1994). Computers are social actors. proceedings for conference on human factors in computing systems. Hum. Fact. Comput. 94, 122–129. doi: 10.1145/259963.260288
Ouwehand, K., Kroef, A., van der Wong, J., and Paas, F. (2021). Measuring cognitive load: are there more valid alternatives to likert rating scales? Front. Educ. 6, 702616. doi: 10.3389/feduc.2021.702616
Pardo, J. S. (2006). On phonetic convergence during conversational interaction. J. Acous. Soc. Am. 119, 2382–2393. doi: 10.1121/1.2178720
Pardo, J. S. (2013). Measuring phonetic convergence in speech production. Front. Psychol. 4, 559. doi: 10.3389/fpsyg.2013.00559
Pardo, J. S., Jay, I. C., and Krauss, R. M. (2010). Conversational role influences speech imitation. Attention Percep. Psychophys. 72, 2254–2264. doi: 10.3758/BF03196699
Pardo, J. S., Urmanche, A., Wilman, S., and Wiener, J. (2017). Phonetic convergence across multiple measures and model talkers. Attention Percep. Psychophys. 79, 637–659. doi: 10.3758/s13414-016-1226-0
Pearson, J., Pickering, M., Branigan, H., McLean, J., Nass, C., and Hu, J. (2004). “The influence of beliefs about an interlocutor on lexical and syntactic alignment: Evidence from human-computer dialogjues,” in 10th Annual Conference Architectures and Mechanisms of Language Processing.
Rao, G. N. (2013). Measuring phonetic convergence: Segmental and suprasegmental speech adaptations during native and non-native talker interactions (Dissertation). Faculty of the Graduate School of the University of Texas at Austin, Texas, United States.
Ross, J. P., Lilley, K. D., Clopper, C. G., Pardo, J. S., and Levi, S. V. (2021). Effects of dialect-specific features and familiarity on cross-dialect phonetic convergence. J. Phonet. 86, 101041. doi: 10.1016/j.wocn.2021.101041
Schmitt, H. (2007). The case for the epsilon symbol (ε) in RP dress. J. Int. Phon. Assoc. 37, 321–328. doi: 10.1017/S0025100307003131
Snyder, C., Cohn, M., and Zellou, G. (2019). Individual variation in cognitive processing style predicts differences in phonetic imitation of device and human voices. Proc. Annual Conf. Speech Commun. Assoc. INTERSPEECH 23, 116–120. doi: 10.21437/Interspeech.2019-2669
Street, R. L., and Giles, H. (1982). Speech accommodation theory: a social cognitive approach to language and speech behavior. Soc. Cognit. Commun. 193226, 193–226.
Suzuki, N., and Katagiri, Y. (2007). Prosodic alignment in human-computer interaction. Connect. Sci. 19, 131–141. doi: 10.1080/09540090701369125
Tamagawa, R., Watson, C. I., Kuo, I. H., Macdonald, B. A., and Broadbent, E. (2011). The effects of synthesized voice accents on user perceptions of robots. Int. J. Soc. Robotics 3, 253–262. doi: 10.1007/s12369-011-0100-4
Thakerar, J. N., Giles, H., and Cheshire, J. (1982). Psychological and linguistic parameters of speech accommodation theory. Adv. Soc. Psychol. Lang. 205, 205–255.
Walker, A., and Campbell-Kibler, K. (2015). Repeat what after whom? Exploring variable selectivity in a cross-dialectal shadowing task. Front. Psychol. 6, 1–18. doi: 10.3389/fpsyg.2015.00546
Walters, S. A., Babel, M. E., and McGuire, G. (2013). The role of voice similarity in accommodation. Proc. Meetings Acoustics 19, 060047. doi: 10.1121/1.4800716
Weatherholtz, K., Campbell-Kibler, K., and Jaeger, T. F. (2014). Socially-mediated syntactic alignment. Lang. Var. Change 26, 387–420. doi: 10.1017/S0954394514000155
Zellou, G., and Cohn, M. (2020). “Top-down effect of apparent humanness on vocal alignment toward human and device interlocutors,” in Proceedings of the 42nd Annual Meeting of the Cognitive Science Society. p. 3490–3496.
Zellou, G., Cohn, M., and Block, A. (2021a). Partial compensation for coarticulatory vowel nasalization across concatenative and neural text-to-speech. J. Acous. Soc. Am. 149, 3424–3436. doi: 10.1121/10.0004989
Keywords: voice-activated artificially intelligent (voice-AI) assistant, human-computer interaction, phonetic accommodation, dialect imitation, apparent guise
Citation: Dodd N, Cohn M and Zellou G (2023) Comparing alignment toward American, British, and Indian English text-to-speech (TTS) voices: influence of social attitudes and talker guise. Front. Comput. Sci. 5:1204211. doi: 10.3389/fcomp.2023.1204211
Received: 11 April 2023; Accepted: 16 June 2023;
Published: 03 July 2023.
Edited by:
Ingo Siegert, Otto von Guericke University Magdeburg, GermanyReviewed by:
Sai Sirisha Rallabandi, Technical University of Berlin, GermanyVered Silber-Varod, Tel Aviv University, Israel
Copyright © 2023 Dodd, Cohn and Zellou. This is an open-access article distributed under the terms of the Creative Commons Attribution License (CC BY). The use, distribution or reproduction in other forums is permitted, provided the original author(s) and the copyright owner(s) are credited and that the original publication in this journal is cited, in accordance with accepted academic practice. No use, distribution or reproduction is permitted which does not comply with these terms.
*Correspondence: Nicole Dodd, bmNkb2RkQHVjZGF2aXMuZWR1