- College of Science and Engineering, Hamad Bin Khalifa University, Qatar Foundation, Doha, Qatar
Dependable utilization of computer vision applications, such as smart surveillance, requires training deep learning networks on datasets that sufficiently represent the classes of interest. However, the bottleneck in many computer vision applications lies in the limited availability of adequate datasets. One particular application that is of great importance for the safety of cities and crowded areas is smart surveillance. Conventional surveillance methods are reactive and often ineffective in enable real-time action. However, smart surveillance is a key component of smart and proactive security in a smart city. Motivated by a smart city application which aims at the automatic identification of concerning events for alerting law-enforcement and governmental agencies, we craft a large video dataset that focuses on the distinction between small-scale violence, large-scale violence, peaceful gatherings, and natural events. This dataset classifies public events along two axes, the size of the crowd observed and the level of perceived violence in the crowd. We name this newly-built dataset the Multi-Scale Violence and Public Gathering (MSV-PG) dataset. The videos in the dataset go through several pre-processing steps to prepare them to be fed into a deep learning architecture. We conduct several experiments on the MSV-PG datasets using a ResNet3D, a Swin Transformer and an R(2 + 1)D architecture. The results achieved by these models when trained on the MSV-PG dataset, 88.37%, 89.76%, and 89.3%, respectively, indicate that the dataset is well-labeled and is rich enough to train deep learning models for automatic smart surveillance for diverse scenarios.
1 Introduction
Early identification of violent events and potential security risks is of crucial importance to governmental and law enforcement agencies. City-wide surveillance systems are setup in many countries for this purpose. For example, China, the United States of America, and the United Kingdom deploy around 15 million, 112 thousand, and 628 thousand Closed Circuit Television (CCTV) cameras, respectively (Global, 2022). These cameras are often deployed outdoors and their real-time feed is often monitored by humans to detect crime and other concerning events. Effective use of security cameras allows for the early detection and the deployment of adequate responses to such events.
Video footage coming from security cameras often require real-time continuous monitoring by humans, which poses several limitations and challenges. First, a significant amount of human capital is required whenever thousands or hundreds of thousands of cameras are deployed in a country or city. If an insufficient number of individuals are allocated for the monitoring of CCTV cameras, many concerning events caught by these cameras can go undetected. In addition, having a human inspect surveillance footage can be inefficient and prone to errors. Missing certain events, such as a protest or a large fight, or delaying their detection may have seriously negative consequences for public peace. Finally, traditional surveillance methods are reactive, requiring events to occur and be manually detected by inspectors before action is taken.
Intelligently automating the detection of concerning events, such as fights and unusually-large gatherings, captured by surveillance cameras is critical and has two main advantages. Firstly, it moves surveillance from the traditional reactive approach to a proactive approach since it alerts authorities regarding the potential for violence. Secondly, it significantly reduces the number of human operators needed for surveillance.
There is no doubt that Deep Learning (DL) has transformed many aspects of society. For instance, the literature has demonstrated that DL models have the capability to detect certain human behaviors (action recognition tasks) (Dhiman and Vishwakarma, 2019). Therefore, in order to streamline the identification of potential security risks in surveillance footage, we propose a computer vision based approach for the automatic identification of various human behaviors caught on CCTV footage. To this end, the contribution of this paper is two fold. First, we embark on a novel data collection effort to collect a video dataset that collectively represents the human behavior classes of interest. Secondly, the developed dataset is used to train a human behavior prediction model that automatically detects the human behavior classes of interest, possibly in real-time.
There were four classes of human behavior that have been identified and selected; namely Large Peaceful Gathering (LPG), Large Violent Gathering (LVG), small-scale fighting (F), and Natural (N) events. Note that these classes exist along two axes, where one axis identifies the size of the crowd and the other identifies whether or not the crowd detected is violent. In addition to conveying the behavior of the crowd to law enforcement, this multi-scale distinction, as opposed to the binary distinction often discussed in the literature (distinction between “fighting” and “no fighting” or “violence” and “no violence”), can provide information on the scale of the appropriate law enforcement response. For instance, prior works did not distinguish between a small fight between two individuals and a violent gathering of hundreds of people; both scenarios would be classified as “violent” in those works. The two scenarios, however, clearly require radically different responses from law enforcement, and thus should be seen as two different classes of events. Dedicating different classes for each of those two scenarios, “F” for the small-scale fight and “LVG” for the large violent crowd, makes for a smart surveillance system with greater utility to law enforcement. Such system detects violent action and informs law enforcement of the nature of the required response. In addition, “non-violent” crowds may sometimes require law enforcement attention, especially when the crowd is relatively large. Large crowds hold the potential for a security hazard (i.e., breaking out of violence within the peaceful crowds), thus the developed dataset also distinguishes between a small peaceful crowd and a large peaceful crowd.
A surveillance system with the capability of automatically classifying video footage into one of the aforementioned classes would provide immediate information about any concerning or potentially concerning events to governmental and law-enforcement agencies for immediate response. The benefits of such a system is that it is scalable to large-scale surveillance, whereas human supervision of a large geographical area through CCTV is unrealistic.
Motivated by the detection task outlined above, we have developed a novel video dataset, called the Multi-Scale Violence and Public Gathering (MSV-PG) dataset, that comprehensively covers the aforementioned classes of behavior. To the best of our knowledge, a similar dataset does not exist or is not available to the public. Additionally, this paper trains, tests, and assesses several DL architectures on the automatic recognition of the relevant human behaviors based on the developed and diverse dataset. The aim of employing DL on the MSV-PG dataset is to showcase the robustness of the developed dataset in training various DL algorithms for behavior recognition applications. Such an application has not been investigated in the literature. The corresponding author of this paper will make the dataset available upon request. The remainder of this paper is organized as follows: Section 2 discusses the pre-processing, labeling of the dataset and the training of selected DL models. Section 3 outlines the results achieved by the selected models. Finally, Section 4 details previous DL-based approaches for video analysis, describes previous Human Action Recognition datasets, provides commentary on possible causes for the miss-classification of samples in the proposed dataset, and outlines the main conclusions of the paper.
2 Materials and methods
Next, we outline the process of video collection and pre-processing, illustrated in Figure 1, that we used to build the MSV-PG dataset. Videos with at least one instance of one of the relevant classes are identified and obtained. Then, the starting and ending time stamps of each occurrence of an event belonging to one of the relevant classes is recorded, alongside the class of that event. In this paper, an instance is defined as an occurrence of one of the classes whose starting and ending time stamps are identified and recorded. In the training and validation phases, we feed equal-sized image sequences to a DL network. We refer to these image sequences as samples.
2.1 Dataset collection, labeling, and prepossessing
In order to identify instances of LPG, LVG, F, and N events, we define the criteria that differentiate each of the four classes as follows:
• LPG: A large number of individuals who are gathered for a singular purpose. Examples of this class are peaceful protests and gatherings of sports fans.
• LVG: A cluster of individuals of whom a “significant” number are engaged in violent action. Examples of violent action include clashes with police, property destruction, and fighting between members of the crowd.
• F: A “small” group of individuals fighting one another.
• N: Footage that shows no concerning behavior. This is a class of footage that one expects to see during regular, everyday life.
It's of crucial importance to recognize that the above definitions are not objective; they are general guidelines that were used to inform the manual video-labeling process. Specifically, during labeling, the determination of whether or not a group of people is large or small is left to the judgment of the person labeling the videos. We elected to do this because, in reality, there does not exist an objective threshold for the number of individuals that would make a group of people a “large” group as opposed to a “small group.” However, to avoid subjectivity in the labeling process, each member of our team (a total of four researchers) labeled the data and majority voting was conducting to select the final label. The first step taken to develop the MSV-PG dataset was to identify sources from which to attain relevant videos. To this end, we obtained relevant videos from YouTube and relevant video datasets which were readily available online. Relevant YouTube videos are those that include at least one instance of at least one of the relevant classes. Key words such as “demonstration,” “violence,” and “clash” were used during the crawling process of YouTube videos. In addition, relevant current and historic events (i.e., George Floyd protests, Hong Kong protests, The Capitol riot, etc.) were searched and some of the resultant videos were included in the dataset. The aim was to gather a large and diverse set of videos to enable the model to generalize to a variety of concerning scenarios.
Similarly, relevant datasets in the literature which include videos that contain instances of one or more of the four classes of interest in this paper were collected. We merged subsets of the UBI-Fights (Degardin and Proença, 2020) and the dataset introduced in Aktı et al. (2019) into the MSV-PG dataset. Although these two datasets are divided into fighting and non-fighting videos, we re-labeled these videos according to our set of classes. Figure 2 illustrates examples of samples of the four considered classes. It is important to note that data from pre-existing datasets only make up ~16% of the total dataset. The remainder of the MSV-PG dataset was collected by crawling relevant YouTube videos.
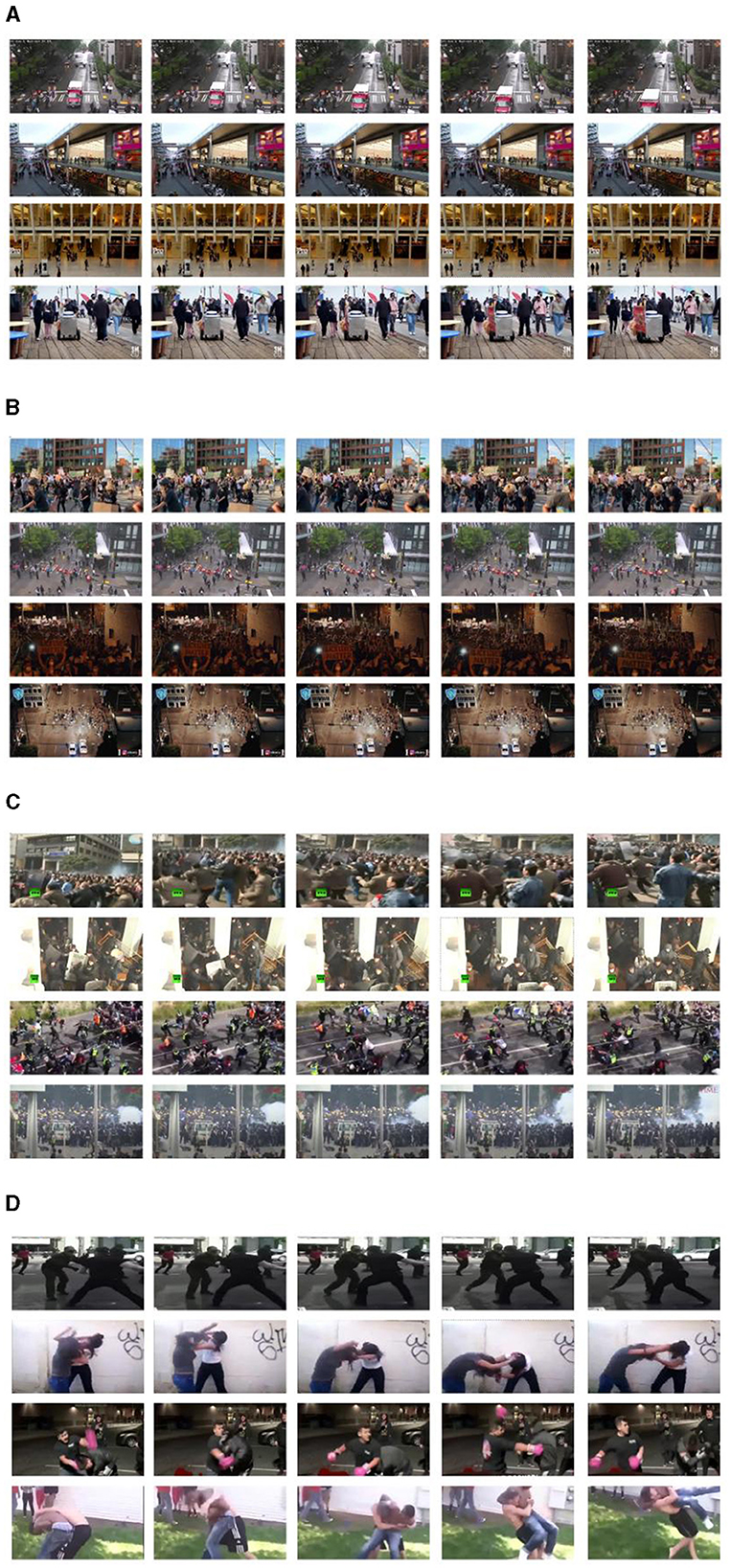
Figure 2. Examples of instances of each of the four classes in MSV-PG. (A) N. Protests continue for sixth day in Seattle, uploaded by Kiro 7 News, 4 via YouTube, licensed under YouTube Standard License. Ghjkmnfm, uploaded by Ganesh Sardar via YouTube, licensed under YouTube Standard License Inside Apple's store at World Trade Center Mall. Westfield. New York, uploaded by Another World via YouTube, licensed under YouTube Standard License. Unpermitted Vendors Defy Police, uploaded by Santa Monica via YouTube, licensed under YouTube Standard License. (B) LPG. How George Floyd's killing has inspired a diverse range of protesters uploaded by PBS NewsHour via YouTube, https://www.youtube.com/watch?v=UQFQ9Q6GT00, licensed under YouTube Standard License. China's Rebel City: The Hong Kong Protests uploaded by South China Morning Post via YouTube, licensed under YouTube Standard License. Death of George Floyd drives protests across the U.S. -and beyond, uploaded by PBS NewsHour via YouTube, licensed under YouTube Standard License. Demonstrators march through downtown Seattle streets on Election Night, uploaded by KING 5 Seattle via YouTube, licensed under YouTube Standard License. (C) LVG. Raw Video: Egypt Protesters Clash with Police, uploaded by Associated Press via YouTube, licensed under YouTube Standard License. Reproduced with permission from Hassner et al. (2012), via Violent Flows - Crowd Violence Database. (D). F. “Antifa Tries To Beat Man With Metal Baton and Gets Knocked Out In One Shot,” uploaded by American Dream via YouTube, licensed under YouTube Standard License. Reproduced with permission from Soliman et al. (2019) via “Real Life Violence Dataset”. Boxing Random Strangers At A Gas Station In The Hood! *Gone Wrong*, uploaded by Kvng Reke via YouTube, licensed under YouTube Standard License. Reproduced with permission from Soliman et al. (2019) via “Real Life Violence Dataset.”
In order to ensure uniformity among the collected videos, the frame-rate of all the videos are unified according to a frame-rate R of choice. Furthermore, a single video may contain instances of different classes at different time periods; thus, an entire video cannot be given a single label. Instead, we opted to identify portions of each video where one of the classes occurs. Namely, we identify the instances of each class in every video collected and record these instances in an annotation table. Each row entry of this table is used to define a single instance of one of the relevant classes. The row entry of an instance defines the numeric ID of the video wherein the instance was found, the starting and ending time stamps of the instance, and the class to which the instance belongs. Table 1 shows an example of an annotation table.
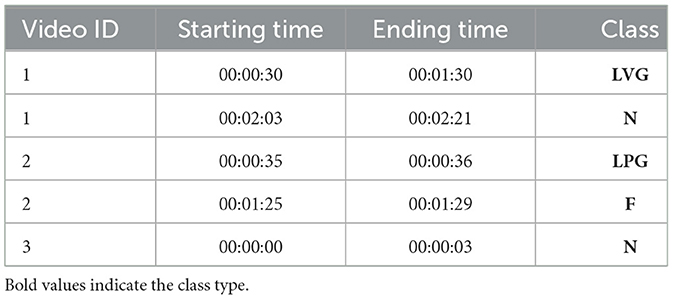
Table 1. An example annotation table describing five instances of the relevant classes occurring in three separate videos.
To facilitate model training on the MSV-PG dataset, each labeled sample should be of equal length. Thus, a length of N seconds is chosen for the length of each training/validation sample. Each sample is a sequence of frames extracted from one of the videos. Assuming that an instance of class Ci occurs in the timespan from (hi:mi:si) to (hf:mf:sf) of video Vi, the frames of that time range are extracted. Subsequently, a sliding window of length RN, the number of frames per sample, is moved through the frames of the timespan from (hi:mi:si) to (hf:mf:sf). Note that the number of frames per sample is equal to the length of the sample, in seconds, multiplied by the frame-rate of the video; Thus, RN = R×N. Since consecutive samples are almost identical, they share RN−1 frames, adding all consecutive samples inside an instance to the dataset would inflate the size of the dataset while providing minimal additional information. Instead, we define a stride parameter S that defines the number of frames that the sliding window skips after extracting each sample from a given instance. Additionally, long instances may bias the dataset in both the training and validation phases. To prevent this, we define a parameter Emax that represents the maximum number of samples to be extracted from a single instance. If the maximum number of samples that can be extracted from an instance exceeds Emax, we extract Emax samples with an equal number of frames between consecutive samples.
In the final stage, we label each sample with its class label Ci, the class of the instance from which that sample was extracted. The procedure of building the dataset is described in Algorithm 1.
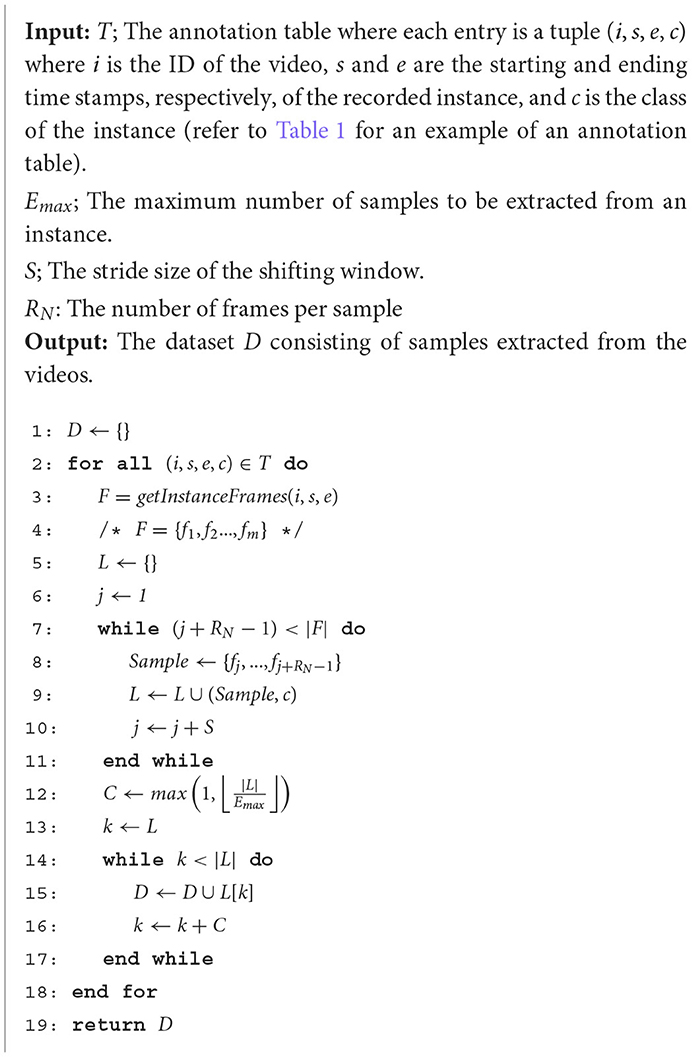
Algorithm 1. The Procedure for building the MSV-PG dataset given a set of videos and the annotation table that records when instances of the relevant classes occur in those videos.
2.2 Dataset summary
The frame-rate of the videos collected is set to 10 frames-per-second (FPS), which is a reasonable frame-rate that allows us to analyse videos in sufficient detail without requiring excess storage space. The length N of each sample is chosen to be 2 s, which is the minimum duration of any instance that can be recorded given the format of our annotation table, since the shorest instance that can be recorded is a 2-s instance that starts at timestamp h:m:s and ends at timestamp h:m:(s+1). Given the 10 FPS frame-rate and the 2-s length of each sample, RN, the number of frames per sample, is 10 × 2 = 20 frames. In our experiments, we feed a DL architecture with 10 of the 20 frames of each sample, skipping every second frame. The stride parameter S is set to 10 frames, indicating that consecutive samples from the same instance share 10 frames, or 1 s. Setting S at 10 frames would allow the trained model to thoroughly learn the actions in the videos without requiring too much storage space. Finally, the maximum number of samples to be extracted from any instance, Emax, was set to 200 samples. The 200-sample limit is determined to achieve a good balance between limiting storage space and producing a sufficiently rich dataset.
The MSV-PG dataset consists of 1,400 videos. The total duration of the instances in the dataset is ~30 h. The length distribution (in seconds) of the instances is shown in Figure 3.
2.3 Model training and testing
In this section, we discuss the models adopted to validate the MSV-PG dataset. In particular, we adopt three different DL models, (i) an 18-layer ResNet3D model (Hara et al., 2017), (ii) a Tiny Swin Transformer model (Liu et al., 2021), and (iii) an R(2 + 1)D model (Tran et al., 2018), for the validation. The selected models have produced state-of-the-art results in vision tasks and thus have been selected for the task at hand given the developed dataset. In this section, the training and testing details are outlined.
2.3.1 Deep learning models
2.3.1.1 ResNet3D model
Residual networks (ResNets) were first introduced for image classification (He et al., 2016). The architecture introduces residual connections to connect non-consecutive convolutional layers. The purpose of adding those connections between non-consecutive layers, called short-cut connections, is to overcome the problem of the degradation of training accuracy when layers are added to a DL model (He and Sun, 2015; Srivastava et al., 2015).
2.3.1.2 R(2 + 1)D model
An R(2 + 1)D model (Tran et al., 2018) utilizes (2 + 1)D convolutions to approximate conventional 3D convolutions. The (2 + 1)D convolutions split the computation into a spatial 2D convolution followed by a temporal 1D convolution. This splitting of the computation into the steps offers the advantage of increasing the complexity of functions that can be represented due to the additional ReLU between the 2D and 1D convolutions, rendering optimization easier. The (2 + 1)D convolutions are also computationally cheaper than 3D convolutions. The contrast between 3D and (2 + 1)D convolutions.
2.3.1.3 The Swin Vision Transformer
Transformer-based DL models have provided state-of-the-art performance for many computer vision problems in recent years (Chromiak, 2021). The Swin Transformer is one of the transformer architectures that is used in many computer vision works as a general backbone for both image and video based problems. In this paper, the Video Swin architecture (Liu et al., 2022), which is proposed for video recognition, is used.
The most important feature that distinguishes the Swin Transformer from other transformer-based models is that its computational complexity increases linearly with respect to image resolution. In other models, the computational complexity is quadratic with image resolution since the attention matrix is computed among all the tokens of the image. Generating pixel-level features is critical in vision problems such as image segmentation and object detection. However, the quadratic computational complexity of the attention matrix prevents the use of patches that will enable the extraction of features at the pixel level in high-resolution images. In the Swin Transformer architecture, attention matrices are computed locally in non-overlapping windows. Since the number of patches in the windows is fixed, the computational complexity grows linearly with the image resolution. In addition, the Swin Transformer generates the features in a hierarchical manner. In the first layers, small patches are used, while in the next layers, neighboring patches are gradually combined.
2.4 Training setup
For training and validation, we aimed to use 80% of the samples of each class in the dataset for training and 20% for validation. However, we also require that the samples extracted from a video be used exclusively for training or exclusively for validation. The purpose of this requirement is to make the training and validation sets totally independent to avoid biasing the DL network. In order to achieve a split that approximates this 80–20 desired split for each class while satisfying the requirement that videos used in the training and validation phases be unique, we used a simple random search method. At each iteration, a randomly-sized set of random videos from our video set is assigned for training and the rest of the videos are assigned for validation. The per-class training/validation split is then calculated. After 2 h of searching, the video split with the best per-class ratios (closest to 80:20 for each class) is used for training and validation. In our experiment, we used 1,121 videos for training the Swin Transformer model and 279 videos for validation. Furthermore, the number of instances and samples for each class used for training and validation is provided in Table 2. From Table 2, we note that the training/validation splits for each class are as follows: (1) N—79.72%/20.28%, (2) LPG—79.03%/20.97%, (3) LVG—80.01%/19.99%, and (4) F—79.80%/20.20%. Also note that there exists a significant degree of imbalance in the number of samples per class; the dataset consists of 36% N samples, 44% LPG samples, 10% LVG samples, and 10% F samples. This is due to the fact that the duration of violence is usually brief compared to the duration of peaceful events given that violence is an anomalous human behavior. Despite this, we observe that the three chosen DL models are able to recognize the general form of the four classes of interest through learning the MSV-PG dataset. The full dataset can be made available upon contacting the corresponding author of this work.
3 Results
Pre-trained models on the Kinetics-400 dataset are used in all the experiments. We train these models for five epochs and report the best result. In all the experiments performed, we set the learning rate to 0.0001 for the pre-trained layers and 0.001 for the randomly initialized classification layer. We decay the learning rates three times for each epoch by a factor of 0.9. A standard SGD is used as an optimizer with momentum and weight decay which are set to 0.9 and 0.0001, respectively. In all the experiments conducted, the input images are resized to 224 × 224 via bi-cubic interpolation and the batch size is set to 16. We applied random horizontal flipping as an augmentation technique during training.
In our experiments, two types of accuracy scores were recorded, a “sample accuracy” and an “instance accuracy.” The sample accuracy of a model is obtained by performing inference on all samples in the validation set, then dividing the number of correctly-classified validation samples by the total number of validation samples. On the other hand, the instance accuracy is recorded by first performing inference on all samples inside an instance. Then, if the class to which most samples inside the instance are classified matches the label of the instance, the number of correctly-classified instances is incremented by one. The number of correctly-classified instances is divided by the total number of instances in the validation set to obtain the instance accuracy of the model.
3.1 Performance analysis
In this section, we present the performance of the three adopted deep learning networks on the developed MSV-PG dataset. Then, in Section 4, will examine some validation samples whose assigned label does not match the output of our trained Swin Transformer model and show that there are some samples whose appropriate labels are indeed ambiguous.
3.1.1 Performance evaluation results
The sample accuracy and instance accuracy scores for the validation set of each class, using the three different architectures adopted, are shown in Table 3. The results demonstrate that the three architectures were able to adequately learn the MSV-PG dataset. The performance indicates that the dataset is well-labeled and can be effectively used in real-world applications. In addition, the sample and instance confusion matrices for the Swin Transformer model, the best-performing model out of the three used, are shown in Tables 4, 5, respectively.
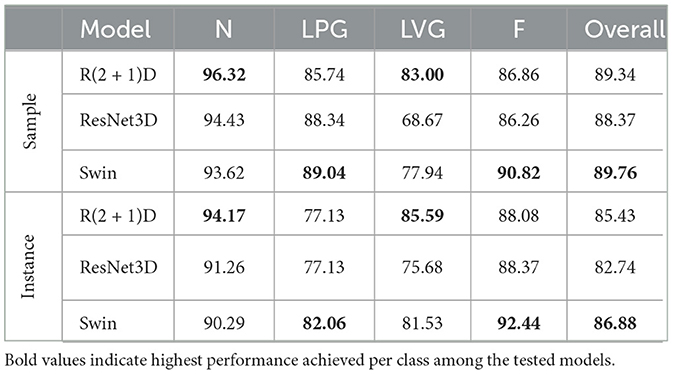
Table 3. Performance (accuracy) on the MSV-PG dataset using the R(2 + 1)D, ResNet3D, and Swin Transformer.
4 Discussion
Over the last several years, significant advances have been made in the domain of video analysis using DL (Sharma et al., 2021). DL-based video processing techniques are usually focused on human action recognition (Huang et al., 2015; Sudhakaran and Lanz, 2017; Arif et al., 2019; Dhiman and Vishwakarma, 2019; Mazzia et al., 2022), anomaly detection (Sabokrou et al., 2018; Nayak et al., 2021), and behavior analysis (Gómez A et al., 2015; Marsden et al., 2017; Sánchez et al., 2020). These techniques often utilize convolutional neural networks (CNNs) (Ji et al., 2012; Karpathy et al., 2014; Simonyan and Zisserman, 2014; Xu et al., 2015; Feichtenhofer et al., 2016; Sahoo et al., 2019; Elboushaki et al., 2020). Tran et al. (2015) first proposed inflating 2D CNNs into 3D CNNs to allow for the extraction of spatio-temporal features for human action recognition tasks. Carreira and Zisserman (2017) then introduced a Two-Stream Inflated 3D (I3D) ConvNet, which inflates the usual 2D Convnets into 3D ConvNets for the purpose of performing video analysis. Through this I3D ConvNet, they inflate the 2D ConvNets of CNN-based image classification models into 3D ConvNets and test these architectures on the Kinetics (Kay et al., 2017) video dataset. However, 3D CNNs suffer from short-term memory and are often only capable of learning human actions that occur in 1–16 frames (Varol et al., 2017). To counter this limitation, Shi et al. (2015) propose convolutional LSTMs, which replace the fully-connected input-to-state and state-to-state transitions of conventional LSTMs, a variant of RNNs, with convolutional transitions that allow for the encoding of spatial features. Furthermore, the literature includes other works where RNNs were used for a wide variety of applications including group activity recognition (Ibrahim et al., 2016), facial expression recognition (Guo et al., 2019), video segmentation (Siam et al., 2017), anomoly detection (Murugesan and Thilagamani, 2020), target tracking (Gao et al., 2019), face recognition (Gong et al., 2019), and background estimation (Savakis and Shringarpure, 2018). Many hybrids of two types of DL architectures were also proposed in the literature. For instance, Arif et al. (2019) combine 3D CNNs and LSTMs for different action recognition tasks while Yadav et al. (2019) use 2D CNN in combination with LSTMs to recognize different yoga postures. Finally, Wang et al. (2019) combine the I3D network with LSTMs by extracting low level features of video frames from the I3D network and feeding it onto LSTMs to achieve human action recognition.
Recently, transformer-based architectures have attracted significant attention. Transformers use self-attention to learn relationships between elements in sequences, which allows for attending to long-term dependencies relative to Recurrent Neural Networks (RNNs), which process elements iterativly. Furthermore, transformers are also more scalable to very large capacity models (Lepikhin et al., 2020). Finally, transformers assume less prior knowledge about the structure of the problem as compared to CNNs and RNNs (Hochreiter and Schmidhuber, 1997; LeCun et al., 2015; Goodfellow et al., 2016). These advantages have led to their success in many Computer Vision tasks such as image recognition (Dosovitskiy et al., 2021; Touvron et al., 2021) and object detection (Carion et al., 2020; Zhu et al., 2020).
Dosovitskiy et al. (2020) proposed ViT, which achieved promising results in image classification tasks by modeling the relationship (attention) between the spatial patches of an image using the standard transformer encoder (Vaswani et al., 2017). After ViT, many transformer-based video recognition methods (Arnab et al., 2021; Bertasius et al., 2021; Liu et al., 2021; Neimark et al., 2021) have been proposed. In these works, different techniques have been developed for temporal attention as well as spatial attention.
Early video datasets for action recognition include the Hollywood (Laptev et al., 2008), UCF50 (Reddy and Shah, 2013), UCF101 (Soomro et al., 2012), and the HMDB-51 (Kuehne et al., 2011) datasets. The Hollywood dataset provides annotated movie clips. Each clip in the dataset belongs to one of 51 classes, including “push,” “sit,” “clap,” “eat,” and “walk,” while the UCF50 and UCF101 datasets consist of YouTube clips grouped into one of 50 and 101 action categories, respectively. Examples of action classes in the UCF50 dataset include “Basketball Shooting” and “Pull Ups” while the action classes in UCF101 includes a wider spectrum of classes subdivided into five different categories, namely, body motion, human-human interactions, human-object interactions, and playing musical instruments and sports. The Kinetics datasets (Kay et al., 2017; Carreira et al., 2018, 2019), more recent benchmarks, significantly increase the number of classes from prior action classification datasets to 400, 600, and 700 action classes, respectively. The aforementioned pre-existing datasets are useful for testing different DL architectures but are not necessarily useful for specific practical tasks, such as surveillance, which likely require the distinction between a limited number of specific action classes.
In terms of public datasets that encompass violent scenery, a dataset focused on violence detection in movies is proposed by Demarty et al. (2014). Movie clips in this dataset are annotated as violent or non-violent scenes. Bermejo Nievas et al. (2011) introduce a database of 1,000 videos divided into two groups, namely, fights and non-fights. Hassner et al. (2012) propose the Violent Flows dataset, which focuses on crowd violence and contains two classes; violence and non-violence. Sultani et al. (2018) collected the UCF-Crime dataset, which includes clips of fighting among other crime classes (e.g, road accident, burglary, robbery, etc.).
Perez et al. (2019) proposed CCTV-fights, a dataset of 1,000 videos, whose accumulative length exceeds 8 h of real fights caught by CCTV cameras. Aktı et al. (2019) put forward a dataset of 300 videos divided equally into two classes; fight and non-fight. UBI-fights (Degardin and Proença, 2020) is another dataset which distinguishes between fighting and non-fighting videos.
We note that none of the aforementioned datasets are usable for our application independently. The Hollywood (Laptev et al., 2008) dataset does not include classes relevant to our desired application. On the other hand, the UCF50 (Reddy and Shah, 2013), UCF101 (Soomro et al., 2012), HMDBI51 (Kuehne et al., 2011), and Kinetics (Kay et al., 2017) datasets are not sufficiently focused on the task of violence detection as they also include a vast range of actions that are not interesting for violence-detection applications. Training a DL model on a dataset that cover a vast number of actions, while generally useful, is potentially detrimental when the desired application is only interested in a small subset of the actions included in that dataset. Instead, it's preferable to limit the number of classes in a dataset to ensure that the trained DL model is highly specialized in recognizing certain behaviors with high accuracy. Examples of datasets that are exclusively focused on violence detection are the Hockey (Bermejo Nievas et al., 2011), Violent Flows (Hassner et al., 2012), CCTV-fights (Perez et al., 2019), SC Fight (Aktı et al., 2019), and the UBI-fights (Degardin and Proença, 2020) datasets. These datasets are specifically constructed for violence-detection tasks and are useful for an application such as ours. However, these datasets classify human behavior along a single dimension (whether or not the behavior is violent), as opposed to our application which seeks to recognize the size and violent nature of a crowd. Due to these limitations, we conclude that the field of smart surveillance requires a new dataset which classifies human behavior according to its extent as well as its violent nature.
No video dataset in the literature, to the best of our knowledge, contains large gatherings, such as protests, as an action class. Protest datasets in the literature, for instance, are limited to image datasets (Clark and Regan, 2016), which documents protester demands, government responses, protest location, and protester identities. Thus, the novelty of our developed video dataset is that it is specifically aimed toward the identification of scenarios of public unrest (violent protests, fights, etc.) or scenarios which have the potential to develop into public unrest (large gatherings, peaceful protests, etc.). Large gatherings are particularly interesting and important to be carefully surviellanced as they can lead to unruly events. In specific, large gatherings that seem peaceful can evolve into a violent scenario with fighting, destruction of property, etc. In addition, the scale of violence captured can inform the scale of the response from law-enforcement. Thus, for the current task, we divide violence into small-scale violence (i.e., F) and large scale violence (i.e., LVG). To our knowledge, these aspects have been largely neglected in existing datasets, which motivates this work.
From the confusion matrices in Tables 4, 5, we note that ambiguity in labeling occurs mostly in samples whose appropriate label lies between LPG/N and F/LVG. Furthermore, we explain why a DL model could be reasonably expected to missclassify some LVG samples as LPG and some F samples as N. The confusion between these pairs of classes, in both the labeling and inference processes, accounts for the vast majority of the error illustrated in the confusion matrices in Tables 4, 5.
4.1 LPG-N misclassification
We define the class of LPGs, according to the criteria outlined in Section 2.1, as events consisting of a “large” congregation of individuals who are gathered for a singular purpose. Firstly, the threshold for the number of individuals required for a gathering to be considered a “large” one is inherently subjective.
Additionally, even if we decide that some video footage contains a “large” number of people, we must substantiate that the individuals in the footage are gathered for a singular purpose, as opposed to a large group of individuals who happen to be in one place by mere chance, before we classify said footage as LPG. This is because a large group of individuals who are gathered by chance, such as people in a public park on a holiday, is an example of a Natural (N) event. Given that our DL model classifies 2 s of footage at a time, we would expect that the classification would sometimes fail to account for the larger context of a gathering and thus missclassify some LPG samples as N, or vice-versa.
Consider the sample in Figure 4. This sample consists of a group of individuals at a traffic stop crossing the road. It's intuitive that this sample constitutes an N sample since seeing a group of people crossing a traffic light should obviously not raise concern. However, since the sample is only 2 s long and since the model receives no information about the context of the scene, a DL model might (and in this case has) missclassified this sample as an LPG, despite the fact that the appropriate label for the sample shown in Figure 4 is clearly N.
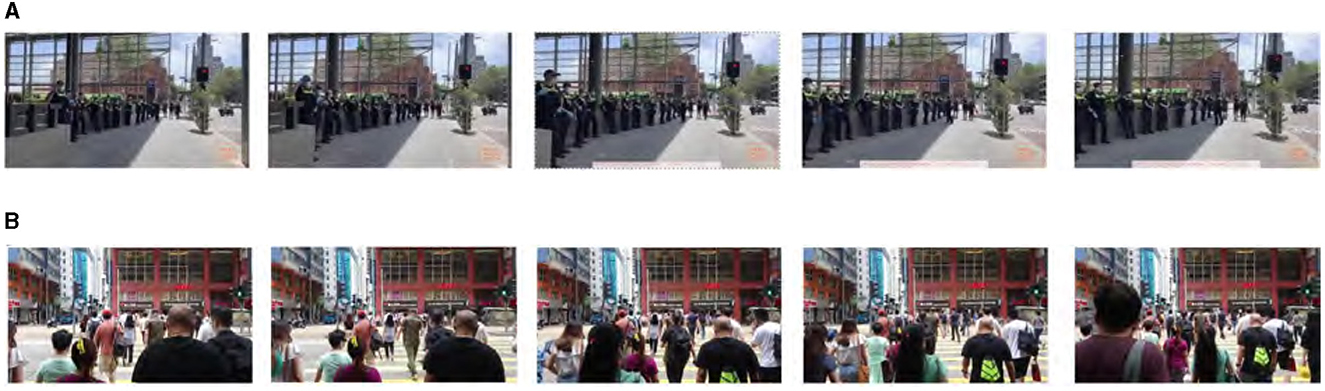
Figure 4. (A) A sample whose appropriate label lies in the blurry area between LPG and N because of the subjective nature of the term “large.” Pop-up protest against the permanent pandemic legislation on Tuesday - 09.11.21, uploaded by Real Press Media via YouTube, licensed under YouTube Standard License. (B) A sample depicting a group of individuals who, by chance, are gathered near a traffic light. Crowd walking on street, uploaded by LionReputationMarketingCoach via YouTube, licensed under YouTube Standard License.
4.2 F-LVG misclassification
We define an LVG as a gathering of a “large” number of individuals engaged in violent actions. A subset of such violent actions is fighting that might occur among a subset of the individuals in the gathering. Similarly to the N-LPG distinction, there's a subjective threshold for the number of people in a violent scene for it to be labeled as LVG instead of F. A scene where it's unclear if the number of individuals depicted satisfies this subjective threshold is shown in Figure 5A. The scene in the figure illustrates a group of individuals engaged in fighting one another. However, it's not clear if the number of people in the scene is large enough to constitute a large violent gathering (LVG) as opposed to a small-scale fight (F).
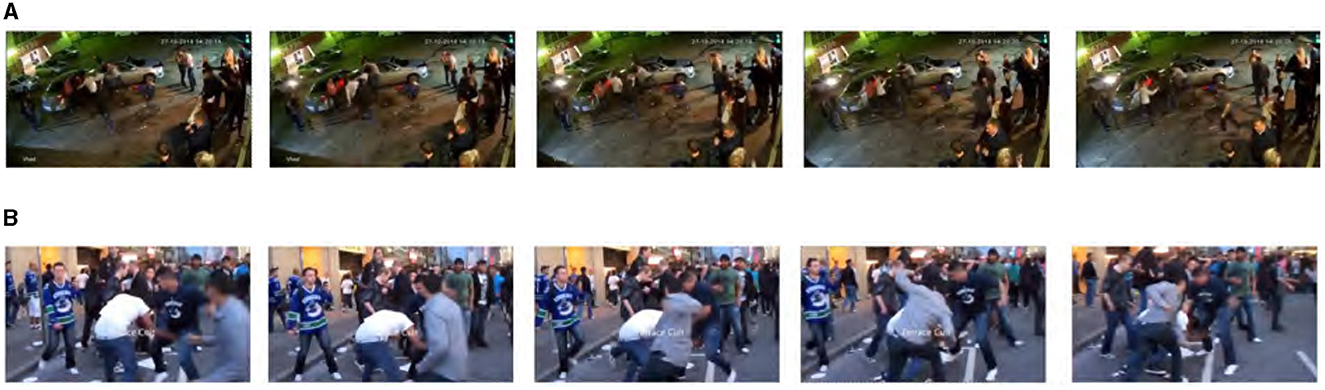
Figure 5. An Illustration of two scenes demonstrating the ambiguity between LVG and F events. (A) A scene with a violent gathering which may or may not be deemed sufficiently large to warrant an LVG label instead of an F label. Reproduced with permission from Soliman et al. (2019) via Real Life Violence Dataset. (B) A scene with large gathering with only a few people fighting, making it unclear if the label if the scene should be LVG or F. Vancouver Canucks Game 5 Stanley Cup Finals- Rogers Arena - Granville Street Party", uploaded by Marlo via YouTube, licensed under YouTube Standard License.
If a violent scene is determined to contain a “large” number of people, it's unclear how many individuals from the observed group must participate in the violent action for the appropriate label to be LVG. Intuitively, if the number of individuals who are involved in violence is small, the label given to such a scene should be F. The line between F and LVG is blurry when it comes to samples where only a subset of the individuals in a gathering are engaged in violence, such as the sample in Figure 5B. Additionally, a trained model might incorrectly label a scene of a large number of individuals, of whom only a few are engaged in fighting, as LVG instead of F since the model may see the large number of individuals in the scene, coupled with the violent action of a few individuals from the crowd, as clues that the appropriate classification of the scene is LVG.
4.3 LPG-LVG misclassification
In instances where a violent crowd is gathered, the violent action may not be central to the footage being analyzed. Namely, the violence in a scene may occur in the background or the corner of the footage such that it is not clearly evident in a frame. One such instance is shown in Figure 6A. In this figure, the large crowd is mostly peaceful, except for violence that occurs in the back of the crowd, which is difficult to notice without observing the scene carefully. As a result, it's natural for this scene to be misclassified as an LPG instead of an LVG.
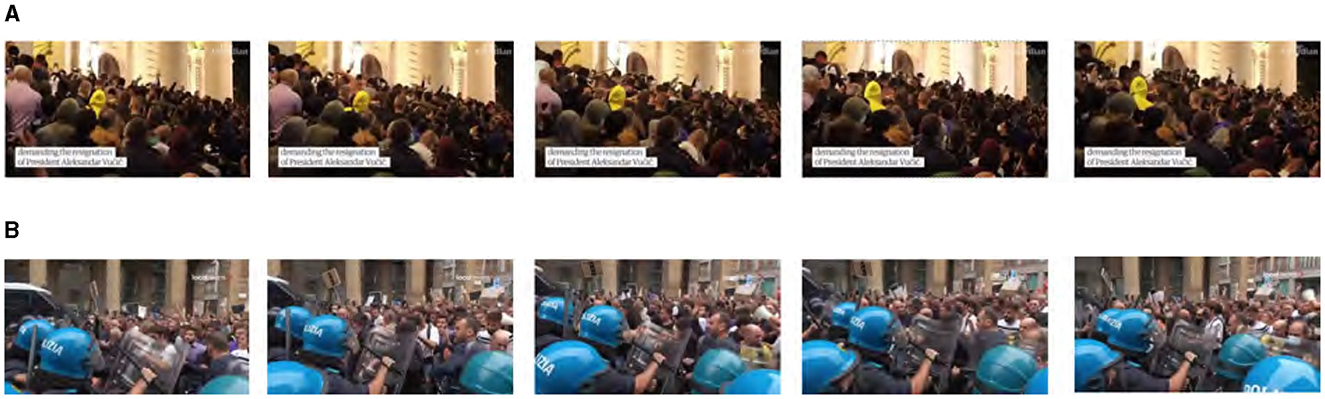
Figure 6. (A) A scene showing a large crowd where violence only occurs in the periphery of the scene, making it hard to notice. Serbian protesters clash with police over government handling of coronavirus, uploaded by Guardian News via YouTube, licensed under YouTube Standard License. (B) A scene of a large crowd where violence occurs only at the end of the scene, which may lead to a DL model failing to catch the late-occurring violence in this scene. Green Pass, tafferugli a Milano: polizia affronta i No Green Pass, uploaded by Local Team via YouTube, licensed under YouTube Standard License.
In an otherwise peaceful crowds, brief moments (e.g., 0.5 s or less) of violent action might occur. In that case, given that our model examines 2-s samples of the incoming footage, the information about the occurrence of violence might be drowned out by information about a peaceful gathering. An example of this is in Figure 6B. As we will see next, this phenomenon also occurs in fighting scenes where the fight is ignored by a DL model in favor of a largely empty background depicting uninteresting, or natural, events.
4.4 F-N misclassification
A fight scene that occurs in an open or largely-empty outdoor area, such as the one in Figure 7A, may be missclassified by a DL model as N. This can be due to the fact that the fight only occurs in a small region of the video scene while the rest of the scene appears to be “natural.” Such missclassifications are particularly prevalent with footage coming from CCTV cameras with wide fields of view.
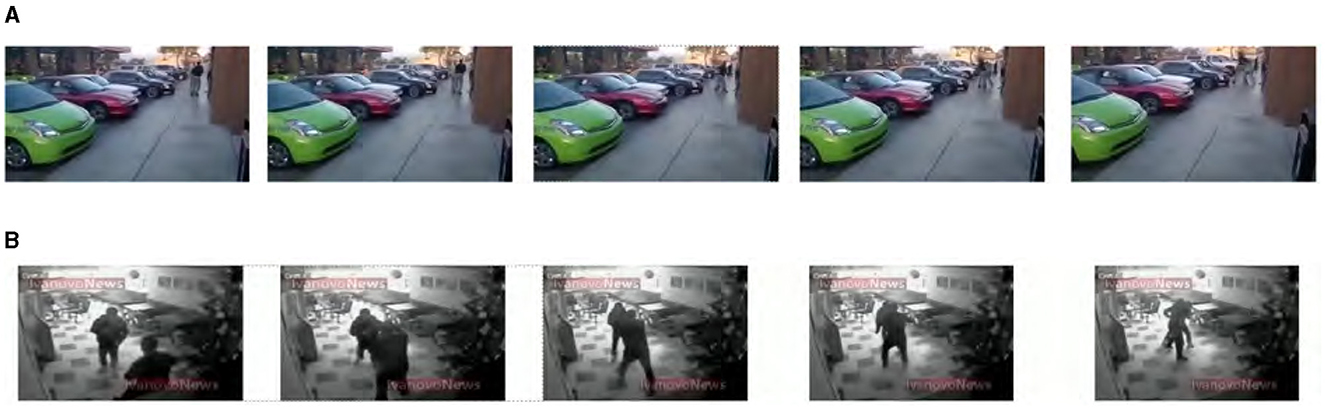
Figure 7. (A) A fight scene in a largely-empty area which may be misclassified as N. Reproduced with permission from Soliman et al. (2019) via Real Life Violence Dataset. (B) A mostly-natural scene where a fight occurs at the end, possibly confusing a DL model to classify it as N instead of F. Reproduced with permission from Degardin and Proença (2020) via UBI-fights dataset.
As is the case with LVG-labeled instances, a fight, like the one in Figure 7B, might continue for a small fraction of a 2-s sample. It's predictable that such a sample may be classified as N instead of F.
4.5 Conclusions
This work was motivated by the task of surveillance in an outdoor area and automatically identifying note-worthy events for law enforcement. This paper presented a new dataset that divides video footage into peaceful gatherings, violent gatherings, small-scale fighting, and natural events. Based on the classification of the captured video, security agencies can be notified and a respond appropriately to the nature of the class of event identified. The dataset presented in this work was validated by using it to train three different architectures with different characteristics, namely, ResNet3D, R(2 + 1)D and the Swin Transformer. The validation results show that the dataset is sufficiently generalized and can be used to train models that can be deployed for real-world surveillance. The dataset described in this paper can be obtained by contacting the corresponding author of this paper.
Data availability statement
The raw data supporting the conclusions of this article will be made available by the authors, without undue reservation.
Ethics statement
Written informed consent was not obtained from the individual(s) for the publication of any potentially identifiable images or data included in this article because videos collected from publicly available sites (YouTube) and datasets.
Author contributions
The ideas presented in this paper were conceptualized by MQ and were discussed with YY, AE, and EB. AE collected the presented dataset and EB ran the experiments on the collected dataset, which are presented in this paper. Finally, the text of this paper was written by AE and EB and was reviewed by MQ and YY. All authors contributed to the article and approved the submitted version.
Funding
This publication was made possible by AICC03-0324-200005 from the Qatar National Research Fund (a member of Qatar Foundation). The findings herein reflect the work, and are solely the responsibility of the authors.
Conflict of interest
The authors declare that the research was conducted in the absence of any commercial or financial relationships that could be construed as a potential conflict of interest.
Publisher's note
All claims expressed in this article are solely those of the authors and do not necessarily represent those of their affiliated organizations, or those of the publisher, the editors and the reviewers. Any product that may be evaluated in this article, or claim that may be made by its manufacturer, is not guaranteed or endorsed by the publisher.
References
Aktı, Ş., Tataroğlu, G. A., and Ekenel, H. K. (2019). “Vision-based fight detection from surveillance cameras,” in 2019 Ninth International Conference on Image Processing Theory, Tools and Applications (IPTA) (Istanbul: IEEE), 1–6. doi: 10.1109/IPTA.2019.8936070
Arif, S., Wang, J., Ul Hassan, T., and Fei, Z. (2019). 3d-cnn-based fused feature maps with LSTM applied to action recognition. Future Internet 11:42. doi: 10.3390/fi11020042
Arnab, A., Dehghani, M., Heigold, G., Sun, C., Lučić, M., and Schmid, C. (2021). “Vivit: a video vision transformer,” in Proceedings of the IEEE/CVF International Conference on Computer Vision (Montreal, QC: IEEE), 6836–6846. doi: 10.1109/ICCV48922.2021.00676
Bermejo Nievas, E., Deniz Suarez, O., Bueno García, G., and Sukthankar, R. (2011). “Violence detection in video using computer vision techniques,” in International Conference on Computer Analysis of Images and Patterns Berlin: (Springer), 332–339. doi: 10.1007/978-3-642-23678-5_39
Bertasius, G., Wang, H., and Torresani, L. (2021). “Is space-time attention all you need for video understanding?” in ICML, volume 2, 4.
Carion, N., Massa, F., Synnaeve, G., Usunier, N., Kirillov, A., Zagoruyko, S., et al. (2020). “End-to-end object detection with transformers,” in Computer Vision - ECCV 2020 (Springer International Publishing), 213–229.
Carreira, J., Noland, E., Banki-Horvath, A., Hillier, C., and Zisserman, A. (2018). A short note about kinetics-600. arXiv. [Preprint]. doi: 10.48550/arXiv.1808.01340
Carreira, J., Noland, E., Hillier, C., and Zisserman, A. (2019). A short note on the kinetics-700 human action dataset. arXiv. [Preprint]. doi: 10.48550/arXiv.1907.06987
Carreira, J., and Zisserman, A. (2017). “Quo vadis, action recognition? A new model and the kinetics dataset,” in Proceedings of the IEEE Conference on Computer Vision and Pattern Recognition (Honolulu, HI: IEEE), 6299–6308. doi: 10.1109/CVPR.2017.502
Chromiak, M. P. (2021). “Exploring recent advancements of transformer based architectures in computer vision,” in Selected Topics in Applied Computer Science (Maria Curie-Skłodowska University Press), 59–75.
Degardin, B., and Proença, H. (2020). “Human activity analysis: iterative weak/self-supervised learning frameworks for detecting abnormal events,” in 2020 IEEE International Joint Conference on Biometrics (IJCB) (Houston, TX: IEEE), 1–7. doi: 10.1109/IJCB48548.2020.9304905
Demarty, C.-H., Ionescu, B., Jiang, Y.-G., Quang, V. L., Schedl, M., Penet, C., et al. (2014). “Benchmarking violent scenes detection in movies,” in 2014 12th International Workshop on Content-Based Multimedia Indexing (CBMI) (Klagenfurt: IEEE), 1–6. doi: 10.1109/CBMI.2014.6849827
Dhiman, C., and Vishwakarma, D. K. (2019). A review of state-of-the-art techniques for abnormal human activity recognition. Eng. Appl. Arti. Intell. 77, 21–45. doi: 10.1016/j.engappai.2018.08.014
Dosovitskiy, A., Beyer, L., Kolesnikov, A., Weissenborn, D., Zhai, X., Unterthiner, T., et al. (2020). An image is worth 16x16 words: transformers for image recognition at scale. arXiv [preprint]. doi: 10.48550/arXiv.2010.11929
Dosovitskiy, A., Beyer, L., Kolesnikov, A., Weissenborn, D., Zhai, X., Unterthiner, T., et al. (2021). “An image is worth 16x16 words: transformers for image recognition at scale,” in International Conference on Learning Representations.
Elboushaki, A., Hannane, R., Afdel, K., and Koutti, L. (2020). MULTID-CNN: a multi-dimensional feature learning approach based on deep convolutional networks for gesture recognition in rgb-d image sequences. Expert Syst. Appl. 139:112829. doi: 10.1016/j.eswa.2019.112829
Feichtenhofer, C., Pinz, A., and Zisserman, A. (2016). “Convolutional two-stream network fusion for video action recognition,” in Proceedings of the IEEE Conference on Computer Vision and Pattern Recognition (Las Vegas, NV: IEEE), 1933–1941. doi: 10.1109/CVPR.2016.213
Gao, C., Yan, J., Zhou, S., Varshney, P. K., and Liu, H. (2019). Long short-term memory-based deep recurrent neural networks for target tracking. Inf. Sci. 502, 279–296. doi: 10.1016/j.ins.2019.06.039
Gómez A, H. F., Tomás, R. M., Tapia, S. A., Caballero, A. F., Ratté, S., Eras, A. G., et al. (2015). “Identification of loitering human behaviour in video surveillance environments,” in International Work-Conference on the Interplay Between Natural and Artificial Computation (Berlin: Springer), 516–525. doi: 10.1007/978-3-319-18914-7_54
Gong, S., Shi, Y., and Jain, A. (2019). “Low quality video face recognition: multi-mode aggregation recurrent network (MARN),” in Proceedings of the IEEE/CVF International Conference on Computer Vision Workshops (Seoul: IEEE). doi: 10.1109/ICCVW.2019.00132
Goodfellow, I., Bengio, Y., and Courville, A. (2016). Deep Learning. MIT Press. Availabl online at: http://www.deeplearningbook.org (accessed June, 2023).
Guo, J.-M., Huang, P.-C., and Chang, L.-Y. (2019). “A hybrid facial expression recognition system based on recurrent neural network,” in 2019 16th IEEE International Conference on Advanced Video and Signal Based Surveillance (AVSS) (Taipei: IEEE), 1–8. doi: 10.1109/AVSS.2019.8909888
Hara, K., Kataoka, H., and Satoh, Y. (2017). “Learning spatio-temporal features with 3d residual networks for action recognition,” in Proceedings of the IEEE International Conference on Computer Vision Workshops (Venice: IEEE), 3154–3160. doi: 10.1109/ICCVW.2017.373
Hassner, T., Itcher, Y., and Kliper-Gross, O. (2012). “Violent flows: real-time detection of violent crowd behavior,” in 2012 IEEE Computer Society Conference on Computer Vision and Pattern Recognition Workshops (Providence, RI: IEEE), 1–6. doi: 10.1109/CVPRW.2012.6239348
He, K., and Sun, J. (2015). “Convolutional neural networks at constrained time cost,” in Proceedings of the IEEE Conference on Computer Vision and Pattern Recognition (Boston, MA: IEEE), 5353–5360. doi: 10.1109/CVPR.2015.7299173
He, K., Zhang, X., Ren, S., and Sun, J. (2016). “Deep residual learning for image recognition,” in Proceedings of the IEEE Conference on Computer Vision and Pattern Recognition (Las Vegas, NV: IEEE), 770–778. doi: 10.1109/CVPR.2016.90
Hochreiter, S., and Schmidhuber, J. (1997). Long short-term memory. Neural Comput. 9, 1735–1780. doi: 10.1162/neco.1997.9.8.1735
Huang, C.-D., Wang, C.-Y., and Wang, J.-C. (2015). “Human action recognition system for elderly and children care using three stream convnet,” in 2015 International Conference on Orange Technologies (ICOT) (Hong Kong: IEEE), 5–9. doi: 10.1109/ICOT.2015.7498476
Ibrahim, M. S., Muralidharan, S., Deng, Z., Vahdat, A., and Mori, G. (2016). A hierarchical deep temporal model for group activity recognition. In Proceedings of the IEEE Conference on Computer Vision and Pattern Recognition, pages 1971-1980. doi: 10.1109/CVPR.2016.217
Ji, S., Xu, W., Yang, M., and Yu, K. (2012). 3d convolutional neural networks for human action recognition. IEEE Trans. Pattern Anal. Mach. Intell. 35, 221–231. doi: 10.1109/TPAMI.2012.59
Karpathy, A., Toderici, G., Shetty, S., Leung, T., Sukthankar, R., Fei-Fei, L., et al. (2014). “Large-scale video classification with convolutional neural networks,” in 2014 IEEE Conference on Computer Vision and Pattern Recognition (Columbus, OH: IEEE), 1725–1732. doi: 10.1109/CVPR.2014.223
Kay, W., Carreira, J., Simonyan, K., Zhang, B., Hillier, C., Vijayanarasimhan, S., et al. (2017). The kinetics human action video dataset. arXiv. [Preprint]. doi: 10.48550/arXiv.1705.06950
Kuehne, H., Jhuang, H., Garrote, E., Poggio, T., and Serre, T. (2011). “HMDB51: a large video database for human motion recognition,” in 2011 International Conference on Computer Vision (Barcelona: IEEE), 2556–2563. doi: 10.1109/ICCV.2011.6126543
Laptev, I., Marszalek, M., Schmid, C., and Rozenfeld, B. (2008). “Learning realistic human actions from movies,” in 2008 IEEE Conference on Computer Vision and Pattern Recognition (Anchorage, AK: IEEE), 1–8. doi: 10.1109/CVPR.2008.4587756
LeCun, Y., Bengio, Y., and Hinton, G. (2015). Deep learning. Nature 521, 436–44. doi: 10.1038/nature14539
Lepikhin, D., Lee, H., Xu, Y., Chen, D., Firat, O., Huang, Y., et al. (2020). Gshard: scaling giant models with conditional computation and automatic sharding. arXiv. [Preprint]. doi: 10.48550/arXiv.2006.16668
Liu, Z., Lin, Y., Cao, Y., Hu, H., Wei, Y., Zhang, Z., et al. (2021). “Swin transformer: hierarchical vision transformer using shifted windows,” in Proceedings of the IEEE/CVF International Conference on Computer Vision (Montreal, QC: IEEE), 10012–10022. doi: 10.1109/ICCV48922.2021.00986
Liu, Z., Ning, J., Cao, Y., Wei, Y., Zhang, Z., Lin, S., et al. (2022). “Video swin transformer,” in Proceedings of the IEEE/CVF Conference on Computer Vision and Pattern Recognition (New Orleans, LA: IEEE), 3202–3211. doi: 10.1109/CVPR52688.2022.00320
Marsden, M., McGuinness, K., Little, S., and O'Connor, N. E. (2017). “Resnetcrowd: a residual deep learning architecture for crowd counting, violent behaviour detection and crowd density level classification,” in 2017 14th IEEE International Conference on Advanced Video and Signal Based Surveillance (AVSS) (Lecce: IEEE), 1–7. doi: 10.1109/AVSS.2017.8078482
Mazzia, V., Angarano, S., Salvetti, F., Angelini, F., and Chiaberge, M. (2022). Action transformer: a 520 self-attention model for short-time pose-based human action recognition. Pattern Recognit. 124:108487. doi: 10.1016/j.patcog.2021.108487
Murugesan, M., and Thilagamani, S. (2020). Efficient anomaly detection in surveillance videos based on multi layer perception recurrent neural network. Microprocess. Microsyst. 79:103303. doi: 10.1016/j.micpro.2020.103303
Nayak, R., Pati, U. C., and Das, S. K. (2021). A comprehensive review on deep learning-based methods for video anomaly detection. Image Vis. Comput. 106:104078. doi: 10.1016/j.imavis.2020.104078
Neimark, D., Bar, O., Zohar, M., and Asselmann, D. (2021). “Video transformer network,” in Proceedings of the IEEE/CVF International Conference on Computer Vision (Montreal, BC: IEEE), 3163–3172. doi: 10.1109/ICCVW54120.2021.00355
Perez, M., Kot, A. C., and Rocha, A. (2019). “Detection of real-world fights in surveillance videos,” in ICASSP 2019-2019 IEEE International Conference on Acoustics, Speech and Signal Processing (ICASSP) (Brighton: IEEE), 2662–2666. doi: 10.1109/ICASSP.2019.8683676
Reddy, K., and Shah, M. (2013). Recognizing 50 human action categories of web videos. Mach. Vis. Appl. 24, 971–981. doi: 10.1007/s00138-012-0450-4
Sabokrou, M., Fayyaz, M., Fathy, M., Moayed, Z., and Klette, R. (2018). Deep-anomaly: fully convolutional neural network for fast anomaly detection in crowded scenes. Comput. Vis. Image Underst. 172, 88–97. doi: 10.1016/j.cviu.2018.02.006
Sahoo, S. R., Dash, R., Mahapatra, R. K., and Sahu, B. (2019). “Unusual event detection in surveillance video using transfer learning,” in 2019 International Conference on Information Technology (ICIT) (Bhubaneswar: IEEE), 319–324. doi: 10.1109/ICIT48102.2019.00063
Sánchez, F. L., Hupont, I., Tabik, S., and Herrera, F. (2020). Revisiting crowd behaviour analysis through deep learning: taxonomy, anomaly detection, crowd emotions, datasets, opportunities and prospects. Inf. Fusion 64, 318–335. doi: 10.1016/j.inffus.2020.07.008
Savakis, A., and Shringarpure, A. M. (2018). “Semantic background estimation in video sequences,” in 2018 5th International Conference on Signal Processing and Integrated Networks (SPIN) (Noida: IEEE), 597–601. doi: 10.1109/SPIN.2018.8474279
Sharma, V., Gupta, M., Kumar, A., and Mishra, D. (2021). Video processing using deep learning techniques: a systematic literature review. IEEE Access 9, 139489–139507. doi: 10.1109/ACCESS.2021.3118541
Shi, X., Chen, Z., Wang, H., Yeung, D.-Y., Wong, W.-K., and Woo, W.-c. (2015). Convolutional LSTM network: a machine learning approach for precipitation nowcasting. Adv. Neural Inf. Process. Syst. 28, 28–37.
Siam, M., Valipour, S., Jagersand, M., and Ray, N. (2017). “Convolutional gated recurrent networks for video segmentation,” in 2017 IEEE International Conference on Image Processing (ICIP) (Beijing: IEEE), 3090–3094. doi: 10.1109/ICIP.2017.8296851
Simonyan, K., and Zisserman, A. (2014). Two-stream convolutional networks for action recognition in videos. Adv. Neural Inf. Process. Syst. 1, 27–36.
Soliman, M., Kamal, M., Nashed, M., Mostafa, Y., Chawky, B., and Khattab, D. (2019). “Violence recognition from videos using deep learning techniques”, in Proceeding of 9th International Conference on Intelligent Computing and Information Systems (ICICIS'19) (Cairo), 79–84.
Soomro, K., Zamir, A. R., and Shah, M. (2012). Ucf101: a dataset of 101 human actions classes from videos in the wild. arXiv. [Preprint]. doi: 10.48550/arXiv.1212.0402
Srivastava, R. K., Greff, K., and Schmidhuber, J. (2015). Highway networks. arXiv. [Preprint]. doi: 10.48550/arXiv.1505.00387
Sudhakaran S. and Lanz, O.. (2017). Learning to Detect Violent Videos Using Convolutional Long Short-Term Memory.
Sultani, W., Chen, C., and Shah, M. (2018). “Real-world anomaly detection in surveillance videos,” in Proceedings of the IEEE Conference on Computer Vision and Pattern Recognition (Salt Lake City, UT: IEEE), 6479–6488. doi: 10.1109/CVPR.2018.00678
Touvron, H., Cord, M., Douze, M., Massa, F., Sablayrolles, A., and Jégou, H. (2021). “Training data-efficient image transformers and distillation through attention,” in International Conference on Machine Learning. PMLR.
Tran, D., Bourdev, L., Fergus, R., Torresani, L., and Paluri, M. (2015). “Learning spatiotemporal features with 3d convolutional networks,” in Proceedings of the IEEE International Conference on Computer Vision (Santiago: IEEE), 4489–4497. doi: 10.1109/ICCV.2015.510
Tran, D., Wang, H., Torresani, L., Ray, J., LeCun, Y., and Paluri, M. (2018). “A closer look at spatiotemporal convolutions for action recognition,” in Proceedings of the IEEE conference on Computer Vision and Pattern Recognition (Salt Lake City, UT: IEEE), 6450–6459. doi: 10.1109/CVPR.2018.00675
Varol, G., Laptev, I., and Schmid, C. (2017). Long-term temporal convolutions for action recognition. IEEE Trans. Pattern Anal. Mach. Intell. 40, 1510–1517. doi: 10.1109/TPAMI.2017.2712608
Vaswani, A., Shazeer, N., Parmar, N., Uszkoreit, J., Jones, L., Gomez, A. N., et al. (2017). Attention is all you need. Adv. Neural Inf. Process. Syst. 30, 30–41.
Wang, X., Miao, Z., Zhang, R., and Hao, S. (2019). I3d-LSTM: a new model for human action recognition. IOP Conf. Ser.: Mater. Sci. Eng. 569:032035. doi: 10.1088/1757-899X/569/3/032035
Xu, Z., Yang, Y., and Hauptmann, A. G. (2015). “A discriminative cnn video representation for event detection,” in Proceedings of the IEEE Conference on Computer Vision and Pattern Recognition(Boston, MA: IEEE), 1798–1807. doi: 10.1109/CVPR.2015.7298789
Yadav, S. K., Singh, A., Gupta, A., and Raheja, J. L. (2019). Real-time yoga recognition using deep learning. Neural Comput. Appl. 31, 9349–9361. doi: 10.1007/s00521-019-04232-7
Keywords: crowd analysis, smart surveillance, violence detection, human action recognition, computer vision
Citation: Elzein A, Basaran E, Yang YD and Qaraqe M (2024) A novel multi-scale violence and public gathering dataset for crowd behavior classification. Front. Comput. Sci. 6:1242690. doi: 10.3389/fcomp.2024.1242690
Received: 19 June 2023; Accepted: 29 February 2024;
Published: 10 May 2024.
Edited by:
Pier Luigi Mazzeo, National Research Council (CNR), ItalyReviewed by:
Yunxue Shao, Nanjing Tech University, ChinaRui-Yang Ju, National Taiwan University, Taiwan
Copyright © 2024 Elzein, Basaran, Yang and Qaraqe. This is an open-access article distributed under the terms of the Creative Commons Attribution License (CC BY). The use, distribution or reproduction in other forums is permitted, provided the original author(s) and the copyright owner(s) are credited and that the original publication in this journal is cited, in accordance with accepted academic practice. No use, distribution or reproduction is permitted which does not comply with these terms.
*Correspondence: Marwa Qaraqe, mqaraqe@hbku.edu.qa