Integrative Bioinformatics Approaches for Identification of Drug Targets in Hypertension
- 1Department of Cardiology, University Medical Center Utrecht, University of Utrecht, Utrecht, Netherlands
- 2CAPES Foundation, Ministry of Education of Brazil, Brasília, Brazil
- 3Durrer Center for Cardiovascular Research, Netherlands Heart Institute, Utrecht, Netherlands
- 4Institute of Cardiovascular Science, Faculty of Population Health Sciences, University College London, London, United Kingdom
- 5Farr Institute of Health Informatics Research and Institute of Health Informatics, University College London, London, United Kingdom
High blood pressure or hypertension is an established risk factor for a myriad of cardiovascular diseases. Genome-wide association studies have successfully found over nine hundred loci that contribute to blood pressure. However, the mechanisms through which these loci contribute to disease are still relatively undetermined as less than 10% of hypertension-associated variants are located in coding regions. Phenotypic cell-type specificity analyses and expression quantitative trait loci show predominant vascular and cardiac tissue involvement for blood pressure-associated variants. Maps of chromosomal conformation and expression quantitative trait loci (eQTL) in critical tissues identified 2,424 genes interacting with blood pressure-associated loci, of which 517 are druggable. Integrating genome, regulome and transcriptome information in relevant cell-types could help to functionally annotate blood pressure associated loci and identify drug targets.
Introduction
Elevated blood pressure (BP) or hypertension is a heritable chronic disorder (1–3), considered the single largest contributing risk factor in disease burden and premature mortality (4). High systolic and/or diastolic BP reflects a higher risk of cardiovascular diseases (4). Genome-wide association studies (GWAS) have found association of 905 loci to BP traits (systolic - SBP, diastolic - DBP and pulse pressure -PP) to date (Table S1) (5–33). The use of larger sample sizes has helped to identify additional variants, as demonstrated by the most recent study including over 1 million people that has identified 535 novel BP loci (33). Still, this collective effort thus far has not entirely elucidated the complete genetic contribution to BP, estimated to be approximately 50–60% (34).
To add to this complexity, 90.7% of the 905 BP-associated index variants are located in intronic or intergenic regions (Table S1). Causal variants are also difficult to pinpoint because of linkage disequilibrium (LD) (35). There is now vast evidence that non-coding variants associated with disease interrupt the action of regulatory elements crucial in relevant tissues for that particular disease (36). BP loci are not only linked to cardiovascular disease but also to other diseases (Figure 1), suggesting that BP-associated variants can result in a wide range of phenotypes. Tissue specificity of genetic loci may be relevant for organ specific disease progression. For example, variants altering expression in heart may more likely affect disease progression through heart-mediated processes rather than kidney-mediated processes, and some patients may suffer of left ventricular hypertrophy while others may develop nephropathy. Thus, investigating the influence of BP variants in critical cell-types is essential in understanding disease risk and biology, and assessing the possible translation of an associated locus into a drug target. The public availability of regulatory annotations in several tissues by projects such as ENCODE (39), Roadmap (40) and GTEx (41, 42) has enabled integration of epigenetic modifications, expression quantitative trait loci (eQTLs) and –omics information with GWAS data. Integrative approaches are useful for prioritizing genes from known GWAS loci for functional follow-up, detecting novel gene-trait associations, inferring the directions of associations, and potential druggability (43–46).
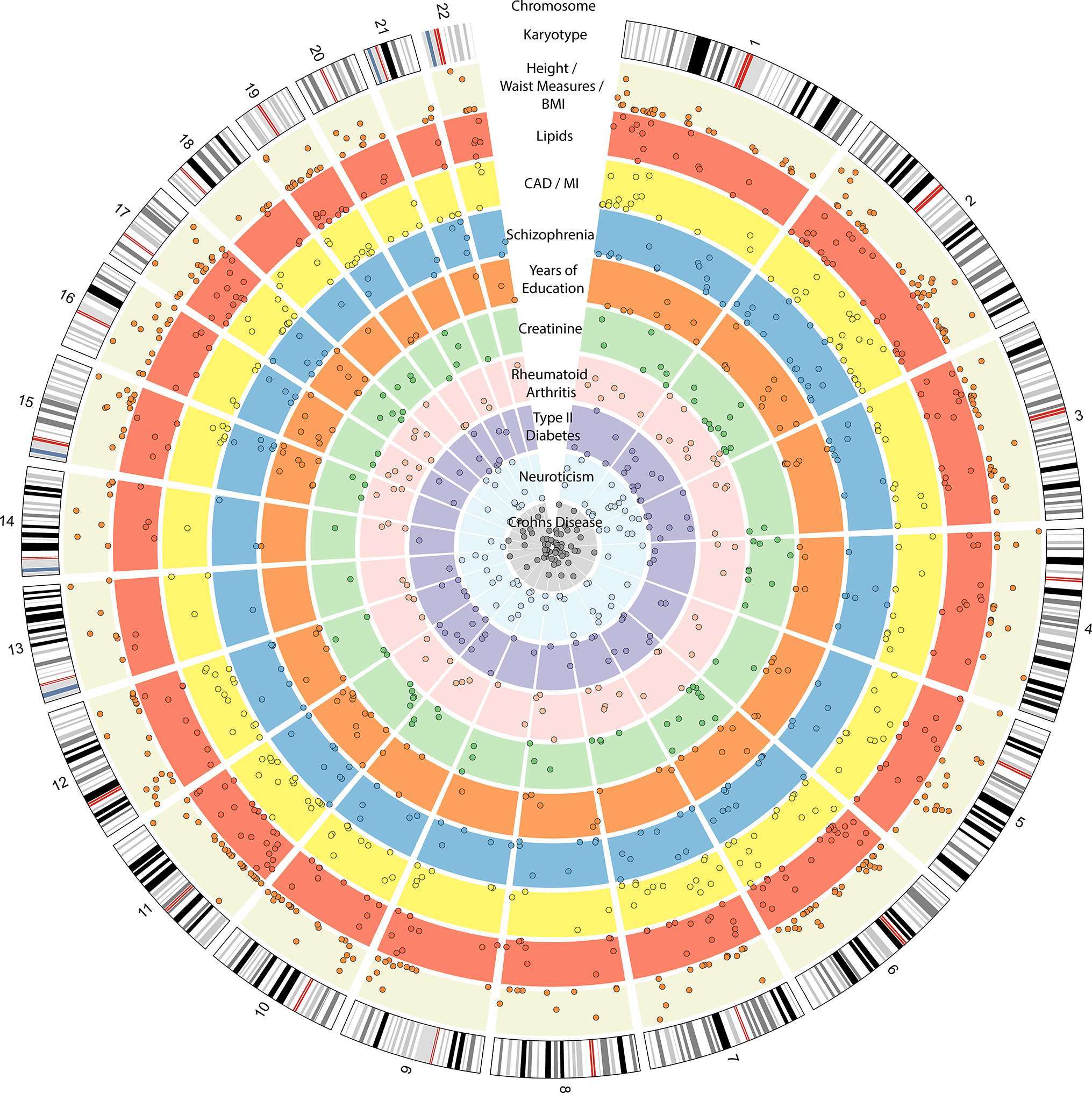
Figure 1. Circos plot showing the 10 traits from the GWAS catalog (37) with the largest number of loci also associated to BP, as identified by PhenoScanner (38) at p < 0.05 (Supplemental Methods). The outer ring represents the genomic/chromosomal location (hg19). The following inner rings show the associations to different traits. Beige: body measurements (height, body mass index (BMI), weight, waist/hip ratio, hip circumference, waist circumference. N = 358). Red: lipids (high-density lipoprotein (HDL), low-density lipoprotein (LDL), triglycerides, total cholesterol. N = 226). Yellow: coronary artery disease (CAD)/myocardial infarction (MI) (N = 206). Blue: schizophrenia (N = 135). Orange: years of education attendance (N = 101). Light green: creatinine (N = 88). Light pink: rheumatoid arthritis (N = 78). Purple: type II diabetes (N = 73). Light turquoise: neuroticism (N = 69). Light grey: Crohn’s disease (N = 67).
Here we summarize the advances made in recent years towards unraveling the mechanisms of non-coding BP variants in disease progression with the resources mentioned above. We focus on integrative approaches that aim to prioritize BP-associated SNPs located in regulatory regions of the genome for follow-up studies (Figure 2). Genetic and molecular aspects of hypertension have been reviewed previously by others (47, 48).
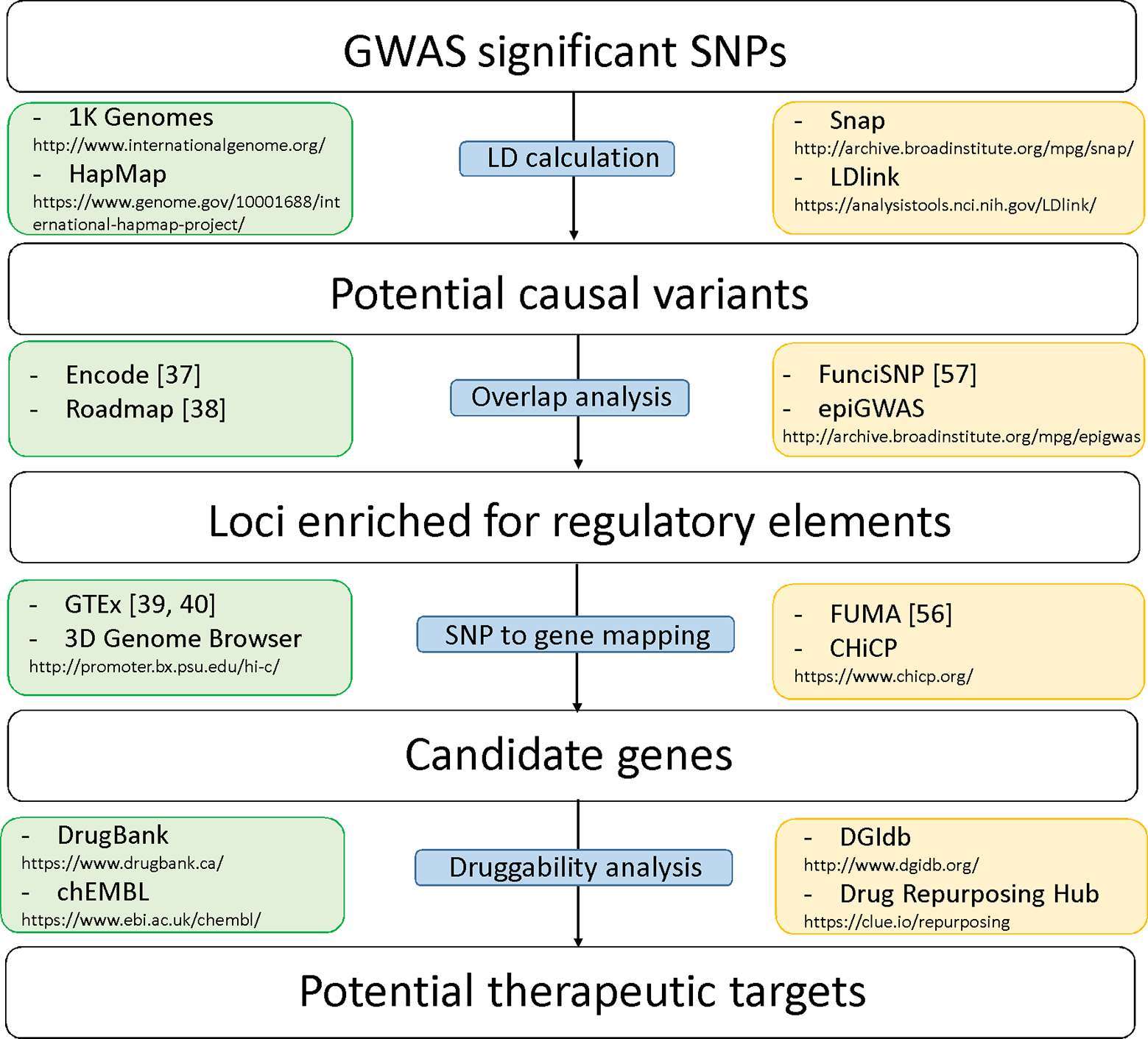
Figure 2. Diagram of analytical steps that can be followed for variant prioritization and translation of association to a potential drug target. Each step is accompanied by examples of publicly available data (green boxes on the left) and tools (yellow boxes on the right) that can be used.
Integrative Approaches Using –Omics Data
Remarkable advances have been made recently towards a better comprehension of BP genetics, the biology of disease and translation towards new therapeutics, boosted by the widespread application of high-throughput genotyping technologies. At the same time, most BP-associated variants are non-coding, making the conversion of statistical associations into target genes a great challenge. SIFT (49, 50), PROVEAN (51), PolyPhen (52), CONDEL (53) and more recently CADD (54) are scoring algorithms developed for predicting the effect of amino acid changes. Only 98 out of the 905 lead BP-associated SNPs reflect a CADD score above 12.37 (Table S2), a threshold suggested by Kicher et al. as deleterious (54). However, the causal variant inside the locus might reflect a different CADD score than the lead SNP, and pinpointing the mechanisms disturbed by the variation remains a challenge.
New strategies that make use of regulatory annotations in disease-relevant tissues have greatly expanded our ability to investigate the processes involved in BP. In particular, annotation of histone modifications and regions of open chromatin allow the identification of active transcription in specific-cell types. Similarly, maps of DNA variants affecting expression in a cell-type specific manner will be integral in BP loci interpretation. A list of cardiovascular-related cell-types researched by the ENCODE Project is presented by Munroe et al. (55). Such data can be integrated with GWAS results using bioinformatics tools (56–58). For instance, FUMA provides extensive functional annotation for all SNPs in associated loci and annotates the identified genes in biological context (57). FunciSNP investigates functional SNPs in regulatory regions of interest (58). Ensemble's Variant Effect Predictor (VEP) determines the effect of variants on genes, transcripts, and protein sequence, as well as regulatory regions, also outputting SIFT, Polyphen and CADD scores for each variant, among other information (59). Although such integrative tools are useful for variant prioritization and interpretation, not all take into consideration tissue specificity aspects. RegulomeDB, for example, is a database that annotates SNPs with known and predicted regulatory elements in the intergenic regions of the human genome, calculating a score that reflects its evidence for regulatory potential (60). However, the scoring procedure can only be performed across all available tissue types. In addition, several databases containing a broad range of tissues were made publicly available since the last update of RegulomeDB, that could be included in the tool. Together, these resources have been useful in prioritizing genes and variants in associated loci for functional follow-up experiments in many post-GWAS analyses, and can be implemented in interpretation of BP-associated loci.
Transcription Regulation: Histone Modifications and Open Chromatin
As genomic coordinates of active regulatory elements may be mapped using unique functions of chromatin, the characterization of chromatin changes in the genome in specific cell-types can be used to identify DNA variants disturbing active regulatory elements. The four core chromatin histones, H2A, H2B, H3 and H4, can suffer posttranslational modifications, such as acetylation or methylation (61). These histone modifications indicate active (euchromatin) or repressed (heterochromatin) chromatin structure, defining regulation and gene transcription (62, 63). Acetylation of histones H3 and H4, and H3 methylation at Lys4 (H3K4me3), for instance, correlate with gene transcription, whereas methylation at Lys9 correlates with gene silencing (62, 64). These modifications provide a robust readout of active regulatory positions in the genome, and have been employed for annotation in several studies (23). Histone modifications influencing arterial pressure have been observed in many tissues, including vascular smooth muscle (65). An updated phenotypic cell-type specificity analysis of the 905 BP loci using H3K4me3 mark in 125 tissues is shown in Figure 3. The most significant cell-types are cardiovascular-related (Supplemental Methods, Table S3). Other tissues with high rank in specificity are smooth muscle, fetal adrenal gland, embryonic kidney cells, CD34 and stem-cell derived CD56 +mesoderm cultured cells. These results are consistent with analyses using DNase I hypersensitivity sites (DHSs), which indicate likely binding sites of transcription factors. These results add more evidence that BP loci are enriched on regions of open chromatin (19, 20, 23, 33) (Figure S1), regulating transcription in a broad range of tissues.
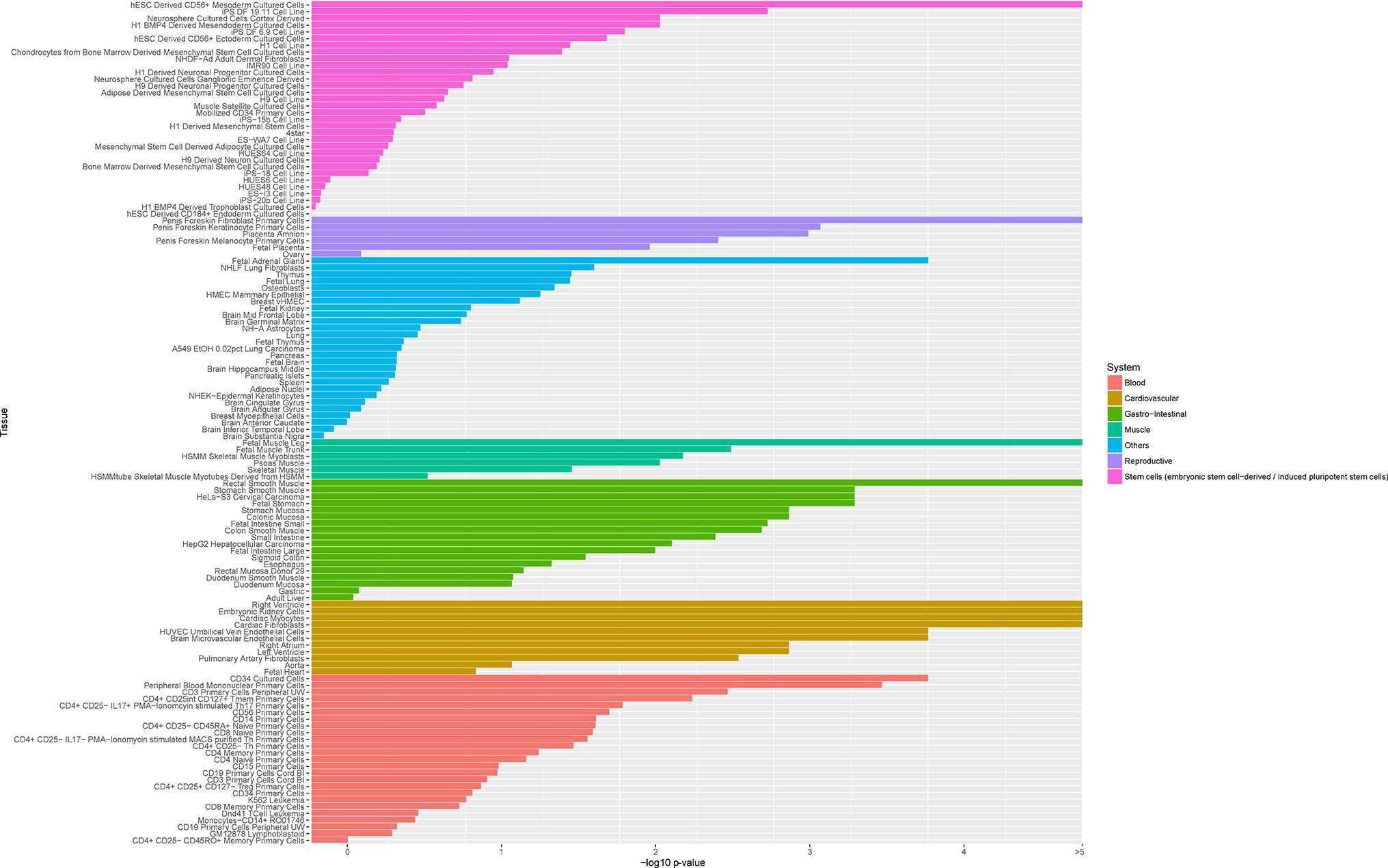
Figure 3. Ranked tissues after phenotypic cell-type specificity analysis of 905 BP SNPs using 125 H3K4me3 datasets on human tissue (Supplemental Methods, Table S3).
Methylation
In addition to histone modifications that promote transcription, BP loci have also been studied for their enrichment on DNA methylation, known to have the opposite regulatory effect. The methylation of CpG sites, presented by CpG islands in promoters, affects binding of transcription factors, resulting in gene silencing (66, 67). Abnormal CpG methylation is found in hypertension (68–70), and in many other complex diseases (71, 72). Recently, Kato et al. identified a ~2 fold enrichment associating BP variants and local DNA methylation (19). The study also demonstrates that DNA methylation in blood correlates with methylation in several other tissues. These observations add to previous indications on the function of DNA methylation in regulating BP.
Measuring the Impact of BP Risk Alleles on Gene Expression: eQTLs
Expression quantitative trait loci (eQTL) are regions harbouring nucleotides correlating with alterations in gene expression (73). Linking transcription levels to complex traits has been a follow-up step adopted by many studies (43, 74–76), driven by the increase in available data of expression patterns across tissues and populations (33, 46, 77–81). Warren et al. found that 55.1% of their identified BP-associated loci have SNPs with eQTLs in at least one tissue from GTex repository (41), with arterial tissue most frequently observed (29.9% of loci had eQTL in aorta and/or tibial artery) (21). A great enrichment of eQTLs in artery was also observed by Evangelou et al., who identified 92 novel loci with eQTL enrichment in arterial tissue and 48 in adrenal tissue (33). In summary, these studies also suggest that BP loci exert a regulatory effect mostly in vascular and cardiac tissues.
Finding the Targets: Chromosome Confirmation Capture Techniques
Mapping variation to target genes is one of the greatest challenges in the post-GWAS era, and different strategies have been developed to this end (82). One approach is the use of chromosome confirmation capture [3C (83), 4C (84, 85), Hi-C (86, 87)]. These techniques capture chromosome interactions (88), resulting in networks of interacting genetic loci (84, 85).
Warren et al. made use of this resource to investigate the target genes of non-coding SNPs, using Hi-C data from endothelial cells (HUVECs). Distal potential genes were found on 21 loci, and these genes were enriched for regulators of cardiac hypertrophy in pathway analysis (20). Kraja et al. also explored long-range chromatin interactions using endothelial precursor cell Hi-C data (89, 90), finding the link between an associated loci and a gene known to affect cell growth and death (91). More recently, Evangelou et al. used chromatin interaction Hi-C data from HUVECs (92), neural progenitor cells (NPC), mesenchymal stem cells (MSC) and tissue from the aorta and adrenal gland (93) to identify distal affected genes. They found 498 novel loci that contained a potential regulatory SNP, and in 484 loci long-range interactions were found in at least one cell-type (33).
A list of human HiC data available on BP relevant tissues is presented in Table S4. An updated version of variant to gene mapping making use of this chromatin conformation data is shown in Table S5. Promoter regions of 1,941 genes were found to interact with the 27,649 candidate SNPs (905 BP associated SNPs and vicinity) (Supplemental Methods, Figure 4). Integration with eQTL data on relevant tissues confirmed 209 of the genes mapped, and added additional 483 genes. One main goal of understanding biological mechanisms of GWAS associations and affected genes is to be able to therapeutically target them. Assessment of the druggability of a BP-associated locus depends on several factors, but overlap of these results with a recent effort on druggability suggests that 517 of these 2,424 genes are druggable (94), and 35 mapped genes are also predicted to interact with common drugs for treatment of hypertension (Table S2, Figure 4, Supplemental Methods). Interestingly, 1,774 of the genes mapped are physically located outside BP-associated loci. These results support the hypothesis that BP GWAS loci act on tissue specific regulatory gene networks. Importantly, they also show that the use of long range chromatin interaction maps can reliably identify target genes even outside the risk locus.
Discussion and Conclusions
GWAS have pinpointed over 900 loci associated with BP, and increasing sample size has shown to be crucial to identify more signals (33). However, efforts are needed to translate these results into biological inferences on causal mechanisms and understanding of disease biology. The integration of data beyond the DNA sequence is crucial to identify genes involved in BP regulated by epigenetic mechanisms.
BP variants show eQTL, histone modification and open chromatin enrichment in a broad range of tissues, mostly vascular and cardiac-related. As the interplay of regulatory elements is highly cell-type specific, the study of changes that influence chromatin structure and accessibility needs to be extended to a broad range of tissues and conditions, including disease and its stages. Rosa-Garrido et al. observed chromatin structural abnormalities when comparing healthy and diseased cardiac myocytes, concluding that heart failure involves altered enhancer-gene interactions (95). Thus, alterations in chromatin structure underlying heart disease perturbs significant interactions that contribute to gene expression. This finding suggests that high resolution chromatin conformation and epigenetic data in disease state can help in understanding how regulatory variants confer risk to disease. The availability of data in different populations will also allow fine-mapping and functional annotation across ethnic groups.
By mapping of BP-associated variants to genes using maps of chromosomal conformation in specific cell-types, we identified 1,941 genes, of which 209 show supported by eQTL mapping. Of all genes mapped (n = 2,424), 517 are predicted as druggable and 35 are predicted to interact with common antihypertensive drugs. These include successful cases such as APOB gene, predicted to be targeted by Ibersartan, an angiotensin II receptor antagonist used mainly for the treatment of hypertension (96). Interestingly, in this analysis we were also able to identify ABCC9 gene on both eQTL and HiC mapping, a gene that interacts with Minoxidil. Although originally developed as an antihypertensive vasodilator, side effects provided limitations and currently its main application occurs topically for treatment of hair loss (97, 98). This highlights the several factors involved in druggability of a target and need for extensive validation and trials. With in-silico experimental evidence supporting a plausible mechanism for association, definitive assignment of functions to putative cis-regulatory elements requires perturbation of these elements. Although the majority of associated variants add only modest effects on risk, more studies suggest combinations of SNPs are frequently necessary in order to explain these effects (99–101). CRISPR–Cas9 (Clustered Regularly Interspaced Short Palindromic Repeats) editing technology (102) permits targeted manipulation of epigenetic mechanisms linked to risk alleles (103). Finally, genes that show consequent differential expression can be further validated in vivo with the use of animal models.
In summary, the integrative approaches presented in this review help understanding the underlying biology of GWAS loci by mapping SNPs to genes and determine cell and tissue-specificity. The increase in availability of regulatory data in a broad range of tissues and disease states will expand the possibilities for integration and interpretation of association results. Studies validating the genes prioritized may identify new drug targets, enabling more effective prevention and treatment of hypertension and its consequences.
Author Contributions
DH, VT and FA contributed in study conception and design. DH was responsible for analysis and interpretation of data and drafting of manuscript. DH, VT, JS and FA provided critical revision and final approval of the manuscript.
Funding
FA is supported by a Dekker scholarship (Junior Staff Member 2014T001, Dutch Heart Foundation) and UCL Hospitals NIHR Biomedical Research Centre. Part of this work is funded through the Innovative Medicines Initiative 2 Joint Undertaking under grant agreement no 116074, BigData@Heart.
Conflict of Interest Statement
The authors declare that the research was conducted in the absence of any commercial or financial relationships that could be construed as a potential conflict of interest.
Supplementary Material
The Supplementary Material for this article can be found online at: https://www.frontiersin.org/articles/10.3389/fcvm.2018.00025/full#supplementary-material
References
1. Muñoz M, Pong-Wong R, Canela-Xandri O, Rawlik K, Haley CS, Tenesa A. Evaluating the contribution of genetics and familial shared environment to common disease using the UK Biobank. Nat Genet (2016) 48(9):980–3. doi: 10.1038/ng.3618
2. Feinleib M, Garrison RJ, Fabsitz R, Christian JC, Hrubec Z, Borhani NO, et al. The NHLBI twin study of cardiovascular disease risk factors: methodology and summary of results. Am J Epidemiol (1977) 106(4):284–95. doi: 10.1093/oxfordjournals.aje.a112464
3. Mongeau JG, Biron P, Sing CF. The influence of genetics and household environment upon the variability of normal blood pressure: the montreal adoption survey. Clin Exp Hypertens A (1986) 8(4-5):653–60. doi: 10.3109/10641968609046581
4. Bromfield S, Muntner P. High blood pressure: the leading global burden of disease risk factor and the need for worldwide prevention programs. Curr Hypertens Rep (2013) 15(3):134–6. doi: 10.1007/s11906-013-0340-9
5. Levy D, Ehret GB, Rice K, Verwoert GC, Launer LJ, Dehghan A, et al. Genome-wide association study of blood pressure and hypertension. Nat Genet (2009) 41(6):677–87. doi: 10.1038/ng.384
6. Newton-Cheh C, Larson MG, Vasan RS, Levy D, Bloch KD, Surti A, et al. Association of common variants in NPPA and NPPB with circulating natriuretic peptides and blood pressure. Nat Genet (2009) 41(3):348–53. doi: 10.1038/ng.328
7. Talmud PJ, Drenos F, Shah S, Shah T, Palmen J, Verzilli C, et al. Gene-centric association signals for lipids and apolipoproteins identified via the HumanCVD BeadChip. Am J Hum Genet (2009) 85(5):628–42. doi: 10.1016/j.ajhg.2009.10.014
8. Padmanabhan S, Melander O, Johnson T, Di Blasio AM, Lee WK, Gentilini D, et al. Genome-wide association study of blood pressure extremes identifies variant near UMOD associated with hypertension. PLoS Genet (2010) 6(10):e1001177. doi: 10.1371/journal.pgen.1001177
9. Ehret GB, Munroe PB, Rice KM, Bochud M, Johnson AD, Chasman DI, et al. Genetic variants in novel pathways influence blood pressure and cardiovascular disease risk. Nature (2011) 478(7367):103–9. doi: 10.1038/nature10405
10. Franceschini N, Reiner AP, Heiss G. Recent findings in the genetics of blood pressure and hypertension traits. Am J Hypertens (2011) 24(4):392–400. doi: 10.1038/ajh.2010.218
11. IBC 50K CAD Consortium. Large-scale gene-centric analysis identifies novel variants for coronary artery disease. PLoS Genet (2011) 7(9):e1002260.
12. Johnson AD, Newton-Cheh C, Chasman DI, Ehret GB, Johnson T, Rose L, et al. Association of hypertension drug target genes with blood pressure and hypertension in 86,588 individuals. Hypertension (2011) 57(5):903–10. doi: 10.1161/HYPERTENSIONAHA.110.158667
13. Johnson T, Gaunt TR, Newhouse SJ, Padmanabhan S, Tomaszewski M, Kumari M, et al. Blood pressure loci identified with a gene-centric array. Am J Hum Genet (2011) 89(6):688–700. doi: 10.1016/j.ajhg.2011.10.013
14. Padmanabhan S, Newton-Cheh C, Dominiczak AF. Genetic basis of blood pressure and hypertension. Trends Genet (2012) 28(8):397–408. doi: 10.1016/j.tig.2012.04.001
15. Franceschini N, Fox E, Zhang Z, Edwards TL, Nalls MA, Sung YJ, et al. Genome-wide association analysis of blood-pressure traits in African-ancestry individuals reveals common associated genes in African and non-African populations. Am J Hum Genet (2013) 93(3):545–54. doi: 10.1016/j.ajhg.2013.07.010
16. Ganesh SK, Tragante V, Guo W, Guo Y, Lanktree MB, Smith EN, et al. Loci influencing blood pressure identified using a cardiovascular gene-centric array. Hum Mol Genet (2013) 22(8):1663–78. doi: 10.1093/hmg/dds555
17. Tragante V, Barnes MR, Ganesh SK, Lanktree MB, Guo W, Franceschini N, et al. Gene-centric meta-analysis in 87,736 individuals of European ancestry identifies multiple blood-pressure-related loci. Am J Hum Genet (2014) 94(3):349–60. doi: 10.1016/j.ajhg.2013.12.016
18. Surendran P, Drenos F, Young R, Warren H, Cook JP, Manning AK, et al. Trans-ancestry meta-analyses identify rare and common variants associated with blood pressure and hypertension. Nat Genet (2016) 48(10):1151–61. doi: 10.1038/ng.3654
19. Kato N, Loh M, Takeuchi F, Verweij N, Wang X, Zhang W, et al. Trans-ancestry genome-wide association study identifies 12 genetic loci influencing blood pressure and implicates a role for DNA methylation. Nat Genet (2015) 47(11):1282–93. doi: 10.1038/ng.3405
20. Warren HR, Evangelou E, Cabrera CP, Gao H, Ren M, Mifsud B, et al. Genome-wide association analysis identifies novel blood pressure loci and offers biological insights into cardiovascular risk. Nat Genet (2017) 49(3):403–15. doi: 10.1038/ng.3768
21. Hoffmann TJ, Ehret GB, Nandakumar P, Ranatunga D, Schaefer C, Kwok PY, et al. Genome-wide association analyses using electronic health records identify new loci influencing blood pressure variation. Nat Genet (2017) 49(1):54–64. doi: 10.1038/ng.3715
22. Liu C, Kraja AT, Smith JA, Brody JA, Franceschini N, Bis JC, et al. Meta-analysis identifies common and rare variants influencing blood pressure and overlapping with metabolic trait loci. Nat Genet (2016) 48(10):1162–70. doi: 10.1038/ng.3660
23. Ehret GB, Ferreira T, Chasman DI, Jackson AU, Schmidt EM, Johnson T, et al. The genetics of blood pressure regulation and its target organs from association studies in 342,415 individuals. Nat Genet (2016) 48(10):1171–84. doi: 10.1038/ng.3667
24. Ganesh SK, Chasman DI, Larson MG, Guo X, Verwoert G, Bis JC, et al. Effects of long-term averaging of quantitative blood pressure traits on the detection of genetic associations. Am. J. Hum. Genet. (2014) 95(1):49–65. doi: 10.1016/j.ajhg.2014.06.002
25. Kato N, Takeuchi F, Tabara Y, Kelly TN, Go MJ, Sim X, et al. Meta-analysis of genome-wide association studies identifies common variants associated with blood pressure variation in east Asians. Nat Genet (2011) 43(6):531–8. doi: 10.1038/ng.834
26. Wang Y, O'Connell JR, Mcardle PF, Wade JB, Dorff SE, Shah SJ, et al. From the Cover: Whole-genome association study identifies STK39 as a hypertension susceptibility gene. Proc Natl Acad Sci USA (2009) 106(1):226–31. doi: 10.1073/pnas.0808358106
27. Wain LV, Verwoert GC, O'Reilly PF, Shi G, Johnson T, Johnson AD. et al. Genome-wide association study identifies six new loci influencing pulse pressure and mean arterial pressure. Nat Genet (2011) 43(10):1005–11. doi: 10.1038/ng.922
28. Simino J, Shi G, Bis JC, Chasman DI, Ehret GB, Gu Xet al. Gene-age interactions in blood pressure regulation: a large-scale investigation with the CHARGE, Global BPgen, and ICBP Consortia. Am J Hum Genet (2014) 95(1):24–38. doi: 10.1016/j.ajhg.2014.05.010
29. Fox ER, Young JH, Li Y, Dreisbach AW, Keating BJ, Musani SK. et al. Association of genetic variation with systolic and diastolic blood pressure among African Americans: the candidate gene association resource study. Hum Mol Genet (2011) 20(11):2273–84. doi: 10.1093/hmg/ddr092
30. Zhu X, Feng T, Tayo BO, Liang J, Young JH, Franceschini Net al. Meta-analysis of correlated traits via summary statistics from GWASs with an application in hypertension. Am J Hum Genet (2015) 96(1):21–36. doi: 10.1016/j.ajhg.2014.11.011
31. Ho JE, Levy D, Rose L, Johnson AD, Ridker PM, Chasman DI. Discovery and replication of novel blood pressure genetic loci in the Women's Genome Health Study. J Hypertens (2011) 29(1):62–9. doi: 10.1097/HJH.0b013e3283406927
32. Parmar PG, Taal HR, Timpson NJ, Thiering E, Lehtimäki T, Marinelli M, et al. International genome-wide association study consortium identifies novel loci associated with blood pressure in children and adolescents. Circ Cardiovasc Genet (2016) 9(3):266–78. doi: 10.1161/CIRCGENETICS.115.001190
33. Evangelou E. Genetic analysis of over one million people identifies 535 novel loci for blood pressure. bioRxiv (2017).
34. Kupper N, Willemsen G, Riese H, Posthuma D, Boomsma DI, de Geus EJ. Heritability of daytime ambulatory blood pressure in an extended twin design. Hypertension (2005) 45(1):80–5. doi: 10.1161/01.HYP.0000149952.84391.54
35. Cowper-Sal Lari R, Zhang X, Wright JB, Bailey SD, Cole MD, Eeckhoute J, et al. Breast cancer risk-associated SNPs modulate the affinity of chromatin for FOXA1 and alter gene expression. Nat Genet (2012) 44(11):1191–8. doi: 10.1038/ng.2416
36. Trynka G, Sandor C, Han B, Xu H, Stranger BE, Liu XS, et al. Chromatin marks identify critical cell types for fine mapping complex trait variants. Nat Genet (2013) 45(2):124–30. doi: 10.1038/ng.2504
37. Hindorff LA, Sethupathy P, Junkins HA, Ramos EM, Mehta JP, Collins FS, et al. Potential etiologic and functional implications of genome-wide association loci for human diseases and traits. Proc Natl Acad Sci USA (2009) 106(23):9362–7. doi: 10.1073/pnas.0903103106
38. Staley JR, Blackshaw J, Kamat MA, Ellis S, Surendran P, Sun BB, et al. PhenoScanner: a database of human genotype-phenotype associations. Bioinformatics (2016) 32(20):3207–9. doi: 10.1093/bioinformatics/btw373
39. ENCODE Project Consortium. An integrated encyclopedia of DNA elements in the human genome. Nature (2012) 489(7414):57–74. doi: 10.1038/nature11247
40. Kundaje A, Meuleman W, Ernst J, Bilenky M, Yen A, Heravi-Moussavi A. et al. Integrative analysis of 111 reference human epigenomes. Nature (2015) 518(7539):317–30. doi: 10.1038/nature14248
41. GTEx Consortium. The Genotype-Tissue Expression (GTEx) project. Nat Genet (2013) 45(6):580–5. doi: 10.1038/ng.2653
42. Carithers LJ, Moore HM. The Genotype-Tissue Expression (GTEx) project. Biopreserv Biobank (2015) 13(5):307–8. doi: 10.1089/bio.2015.29031.hmm
43. Zhu Z, Zhang F, Hu H, Bakshi A, Robinson MR, Powell JE, et al. Integration of summary data from GWAS and eQTL studies predicts complex trait gene targets. Nat Genet (2016) 48(5):481–7. doi: 10.1038/ng.3538
44. Pickrell JK, Berisa T, Liu JZ, Ségurel L, Tung JY, Hinds DA. Detection and interpretation of shared genetic influences on 42 human traits. Nat Genet (2016) 48(7):709–17. doi: 10.1038/ng.3570
45. Visscher PM, Yang J. A plethora of pleiotropy across complex traits. Nat Genet (2016) 48(7):707–8. doi: 10.1038/ng.3604
46. Battle A, Brown CD, Engelhardt BE, Montgomery SB, GTEx Consortium Laboratory. Genetic effects on gene expression across human tissues. Nature (2017) 550(7675):204–13. doi: 10.1038/nature24277
47. Padmanabhan S, Caulfield M, Dominiczak AF. Genetic and molecular aspects of hypertension. Circ Res (2015) 116(6):937–59. doi: 10.1161/CIRCRESAHA.116.303647
48. Wang X, Prins BP, Sõber S, Laan M, Snieder H. Beyond genome-wide association studies: new strategies for identifying genetic determinants of hypertension. Curr Hypertens Rep (2011) 13(6):442–51. doi: 10.1007/s11906-011-0230-y
49. Kumar P, Henikoff S, Ng PC. Predicting the effects of coding non-synonymous variants on protein function using the SIFT algorithm. Nat Protoc (2009) 4(7):1073–81. doi: 10.1038/nprot.2009.86
50. Ng PC, Henikoff S. SIFT: Predicting amino acid changes that affect protein function. Nucleic Acids Res (2003) 31(13):3812–4. doi: 10.1093/nar/gkg509
51. Choi Y, Sims GE, Murphy S, Miller JR, Chan AP. Predicting the functional effect of amino acid substitutions and indels. PLoS ONE (2012) 7(10):e46688. doi: 10.1371/journal.pone.0046688
52. Adzhubei IA, Schmidt S, Peshkin L, Ramensky VE, Gerasimova A, Bork P, et al. A method and server for predicting damaging missense mutations. Nat Methods (2010) 7(4):248–9. doi: 10.1038/nmeth0410-248
53. González-Pérez A, López-Bigas N. Improving the assessment of the outcome of nonsynonymous SNVs with a consensus deleteriousness score, Condel. Am J Hum Genet (2011) 88(4):440–9. doi: 10.1016/j.ajhg.2011.03.004
54. Kircher M, Witten DM, Jain P, O'Roak BJ, Cooper GM, Shendure J. A general framework for estimating the relative pathogenicity of human genetic variants. Nat Genet (2014) 46(3):310–5. doi: 10.1038/ng.2892
55. Munroe PB, Barnes MR, Caulfield MJ. Advances in blood pressure genomics. Circ Res (2013) 112(10):1365–79. doi: 10.1161/CIRCRESAHA.112.300387
56. Tak YG, Farnham PJ. Making sense of GWAS: using epigenomics and genome engineering to understand the functional relevance of SNPs in non-coding regions of the human genome. Epigenetics Chromatin (2015) 8:57. doi: 10.1186/s13072-015-0050-4
57. Watanabe K, Taskesen E, van Bochoven A, Posthuma D. Functional mapping and annotation of genetic associations with FUMA. Nat Commun (2017) 8(1):p. 1826. doi: 10.1038/s41467-017-01261-5
58. Coetzee SG, Rhie SK, Berman BP, Coetzee GA, Noushmehr H. FunciSNP: an R/bioconductor tool integrating functional non-coding data sets with genetic association studies to identify candidate regulatory SNPs. Nucleic Acids Res (2012) 40(18):e139. doi: 10.1093/nar/gks542
59. Mclaren W, Gil L, Hunt SE, Riat HS, Ritchie GR, Thormann A, et al. The Ensembl Variant Effect Predictor. Genome Biol (2016) 17(1):122. doi: 10.1186/s13059-016-0974-4
60. Boyle AP, Hong EL, Hariharan M, Cheng Y, Schaub MA, Kasowski M, et al. Annotation of functional variation in personal genomes using RegulomeDB. Genome Res (2012) 22(9):1790–7. doi: 10.1101/gr.137323.112
61. Marmorstein R, Trievel RC. Histone modifying enzymes: structures, mechanisms, and specificities. Biochim Biophys Acta (2009) 1789(1):58–68. doi: 10.1016/j.bbagrm.2008.07.009
62. Jenuwein T, Allis CD. Translating the histone code. Science (2001) 293(5532):1074–80. doi: 10.1126/science.1063127
63. Sullivan BA, Karpen GH. Centromeric chromatin exhibits a histone modification pattern that is distinct from both euchromatin and heterochromatin. Nat Struct Mol Biol (2004) 11(11):1076–83. doi: 10.1038/nsmb845
64. Fischle W, Wang Y, Allis CD. Binary switches and modification cassettes in histone biology and beyond. Nature (2003) 425(6957):475–9. doi: 10.1038/nature02017
65. Wise IA, Charchar FJ. Epigenetic Modifications in Essential Hypertension. Int J Mol Sci (2016) 17(4):451. doi: 10.3390/ijms17040451
66. Holliday R, Pugh JE. DNA modification mechanisms and gene activity during development. Science (1975) 187(4173):226–32. doi: 10.1126/science.1111098
67. Riggs AD. X inactivation, differentiation, and DNA methylation. Cytogenet Cell Genet (1975) 14(1):9–25. doi: 10.1159/000130315
68. Frey FJ. Methylation of CpG islands: potential relevance for hypertension and kidney diseases. Nephrol Dial Transplant (2005) 20(5):868–9. doi: 10.1093/ndt/gfh746
69. Smolarek I, Wyszko E, Barciszewska AM, Nowak S, Gawronska I, Jablecka A, et al. Global DNA methylation changes in blood of patients with essential hypertension. Med Sci Monit (2010) 16(3):CR149–55.
70. Raftopoulos L, Katsi V, Makris T, Tousoulis D, Stefanadis C, Kallikazaros I. Epigenetics, the missing link in hypertension. Life Sci (2015) 129:22–6. doi: 10.1016/j.lfs.2014.08.003
71. Muhonen P, Holthofer H. Epigenetic and microRNA-mediated regulation in diabetes. Nephrol Dial Transplant (2009) 24(4):1088–96. doi: 10.1093/ndt/gfn728
72. Mill J, Tang T, Kaminsky Z, Khare T, Yazdanpanah S, Bouchard L, et al. Epigenomic profiling reveals DNA-methylation changes associated with major psychosis. Am J Hum Genet (2008) 82(3):696–711. doi: 10.1016/j.ajhg.2008.01.008
73. Albert FW, Kruglyak L. The role of regulatory variation in complex traits and disease. Nat Rev Genet (2015) 16(4):197–212. doi: 10.1038/nrg3891
74. Gusev A, Ko A, Shi H, Bhatia G, Chung W, Penninx BW, et al. Integrative approaches for large-scale transcriptome-wide association studies. Nat Genet (2016) 48(3):245–52. doi: 10.1038/ng.3506
75. Musunuru K, Strong A, Frank-Kamenetsky M, Lee NE, Ahfeldt T, Sachs KV, et al. From noncoding variant to phenotype via SORT1 at the 1p13 cholesterol locus. Nature (2010) 466(7307):714–9. doi: 10.1038/nature09266
76. Gamazon ER, Wheeler HE, Shah KP, Mozaffari SV, Aquino-Michaels K, Carroll RJet al. A gene-based association method for mapping traits using reference transcriptome data. Nat. Genet. (2015) 47(9):1091–8. doi: 10.1038/ng.3367
77. Xia K, Shabalin AA, Huang S, Madar V, Zhou YH, Wang W, et al. seeQTL: a searchable database for human eQTLs. Bioinformatics (2012) 28(3):451–2. doi: 10.1093/bioinformatics/btr678
78. GTEx Consortium. Human genomics. The Genotype-Tissue Expression (GTEx) pilot analysis: multitissue gene regulation in humans. Science (2015) 348(6235):648–60. doi: 10.1126/science.1262110
79. Yang TP, Beazley C, Montgomery SB, Dimas AS, Gutierrez-Arcelus M, Stranger BE, et al. Genevar: a database and Java application for the analysis and visualization of SNP-gene associations in eQTL studies. Bioinformatics (2010) 26(19):2474–6. doi: 10.1093/bioinformatics/btq452
80. Westra HJ, Peters MJ, Esko T, Yaghootkar H, Schurmann C, Kettunen J, et al. Systematic identification of trans eQTLs as putative drivers of known disease associations. Nat Genet (2013) 45(10):1238–43. doi: 10.1038/ng.2756
81. Lappalainen T, Sammeth M, Friedländer MR, 't Hoen PA, Monlong J, Rivas MAet al. Transcriptome and genome sequencing uncovers functional variation in humans. Nature (2013) 501(7468):506–11. doi: 10.1038/nature12531
82. Spain SL, Barrett JC. Strategies for fine-mapping complex traits. Hum Mol Genet (2015) 24(R1):R111–R119. doi: 10.1093/hmg/ddv260
83. Dekker J, Rippe K, Dekker M, Kleckner N. Capturing chromosome conformation. Science (2002) 295(5558):1306–11. doi: 10.1126/science.1067799
84. Simonis M, Klous P, Splinter E, Moshkin Y, Willemsen R, de Wit E, et al. Nuclear organization of active and inactive chromatin domains uncovered by chromosome conformation capture-on-chip (4C). Nat Genet (2006) 38(11):1348–54. doi: 10.1038/ng1896
85. Zhao Z, Tavoosidana G, Sjölinder M, Göndör A, Mariano P, Wang S, et al. Circular chromosome conformation capture (4C) uncovers extensive networks of epigenetically regulated intra- and interchromosomal interactions. Nat Genet (2006) 38(11):1341–7. doi: 10.1038/ng1891
86. Belton JM, Mccord RP, Gibcus JH, Naumova N, Zhan Y, Dekker J. Hi-C: a comprehensive technique to capture the conformation of genomes. Methods (2012) 58(3):268–76. doi: 10.1016/j.ymeth.2012.05.001
87. van Berkum NL, Lieberman-Aiden E, Williams L, Imakaev M, Gnirke A, Mirny LA, et al. Hi-C: a method to study the three-dimensional architecture of genomes. J Vis Exp (2010) (39):1869. doi: 10.3791/1869
88. Rivera CM, Ren B. Mapping human epigenomes. Cell (2013) 155(1):39–55. doi: 10.1016/j.cell.2013.09.011
89. Javierre BM, Burren OS, Wilder SP, Kreuzhuber R, Hill SM, Sewitz Set al. Lineage-specific genome architecture links enhancers and non-coding disease variants to target gene promoters. Cell (2016) 167(5):1369–84. doi: 10.1016/j.cell.2016.09.037
90. Schofield EC, Carver T, Achuthan P, Freire-Pritchett P, Spivakov M, Todd JA, et al. CHiCP: a web-based tool for the integrative and interactive visualization of promoter capture Hi-C datasets. Bioinformatics (2016) 32(16):2511–3. doi: 10.1093/bioinformatics/btw173
91. Kraja AT, Cook JP, Warren HR, Surendran P, Liu C, Evangelou E, et al. New Blood Pressure-Associated Loci Identified in Meta-Analyses of 475 000 Individuals. Circ Cardiovasc Genet (2017) 10(5):e001778. doi: 10.1161/CIRCGENETICS.117.001778
92. Rao SS, Huntley MH, Durand NC, Stamenova EK, Bochkov ID, Robinson JT, et al. A 3D map of the human genome at kilobase resolution reveals principles of chromatin looping. Cell (2014) 159(7):1665–80. doi: 10.1016/j.cell.2014.11.021
93. Schmitt AD, Hu M, Jung I, Xu Z, Qiu Y, Tan CL, et al. A Compendium of Chromatin Contact Maps Reveals Spatially Active Regions in the Human Genome. Cell Rep (2016) 17(8):2042–59. doi: 10.1016/j.celrep.2016.10.061
94. Finan C, Gaulton A, Kruger FA, Lumbers RT, Shah T, Engmann J, et al. The druggable genome and support for target identification and validation in drug development. Sci Transl Med (2017) 9(383):eaag1166. doi: 10.1126/scitranslmed.aag1166
95. Rosa-Garrido M, Chapski DJ, Schmitt AD, Kimball TH, Karbassi E, Monte E, et al. High-resolution mapping of chromatin conformation in cardiac myocytes reveals structural remodeling of the epigenome in heart failure. Circulation (2017) 136(17):1613–25. doi: 10.1161/CIRCULATIONAHA.117.029430
96. Liljedahl U, Lind L, Kurland L, Berglund L, Kahan T, Syvänen AC. Single nucleotide polymorphisms in the apolipoprotein B and low density lipoprotein receptor genes affect response to antihypertensive treatment. BMC Cardiovasc Disord (2004) 4(1):16. doi: 10.1186/1471-2261-4-16
97. Chandrashekar BS, Nandhini T, Vasanth V, Sriram R, Navale S. Topical minoxidil fortified with finasteride: an account of maintenance of hair density after replacing oral finasteride. Indian Dermatol Online J (2015) 6(1):17–20. doi: 10.4103/2229-5178.148925
98. Harakalova M, van Harssel JJ, Terhal PA, van Lieshout S, Duran K, Renkens I, et al. Dominant missense mutations in ABCC9 cause Cantú syndrome. Nat Genet (2012) 44(7):793–6. doi: 10.1038/ng.2324
99. Trynka G, Hunt KA, Bockett NA, Romanos J, Mistry V, Szperl A, et al. Dense genotyping identifies and localizes multiple common and rare variant association signals in celiac disease. Nat Genet (2011) 43(12):1193–201. doi: 10.1038/ng.998
100. Stahl EA, Wegmann D, Trynka G, Gutierrez-Achury J, Do R, Voight BF, et al. Bayesian inference analyses of the polygenic architecture of rheumatoid arthritis. Nat Genet (2012) 44(5):483–9. doi: 10.1038/ng.2232
101. Edwards SL, Beesley J, French JD, Dunning AM. Beyond GWASs: illuminating the dark road from association to function. Am J Hum Genet (2013) 93(5):779–97. doi: 10.1016/j.ajhg.2013.10.012
102. Cong L, Ran FA, Cox D, Lin S, Barretto R, Habib N, et al. Multiplex genome engineering using CRISPR/Cas systems. Science (2013) 339(6121):819–23. doi: 10.1126/science.1231143
Keywords: hypertension, blood pressure, epigenetic regulation, GWAS, data integration, functional annotation, drug target identification.
Citation: Hemerich D, van Setten J, Tragante V and Asselbergs FW (2018). Integrative Bioinformatics Approaches for Identification of Drug Targets in Hypertension. Front. Cardiovasc. Med. 5:25. doi: 10.3389/fcvm.2018.00025
Received: 19 January 2018; Accepted: 12 March 2018;
Published: 04 April 2018
Edited by:
Jeanette Erdmann, University of Lübeck, GermanyReviewed by:
Pallavi R. Devchand, Icahn School of Medicine at Mount Sinai, United StatesMelanie Boerries, Deutsches Krebsforschungszentrum (DKFZ), Germany
Yuqi Zhao, University of California, Los Angeles, United States
Copyright © 2018 Hemerich, van Setten, Tragante and Asselbergs. This is an open-access article distributed under the terms of the Creative Commons Attribution License (CC BY). The use, distribution or reproduction in other forums is permitted, provided the original author(s) and the copyright owner are credited and that the original publication in this journal is cited, in accordance with accepted academic practice. No use, distribution or reproduction is permitted which does not comply with these terms.
*Correspondence: Folkert W. Asselbergs, F.W.Asselbergs@umcutrecht.nl