- 1Deutsches Herzzentrum München, Klinik für Herz- und Kreislauferkrankungen, Technische Universität München, Munich, Germany
- 2Munich Heart Alliance, German Centre for Cardiovascular Research, Munich, Germany
Coronary artery disease (CAD) and myocardial infarction (MI) remain among the leading causes of mortality worldwide, urgently demanding a better understanding of disease etiology, and more efficient therapeutic strategies. Genetic predisposition as well as the environment and lifestyle are thought to contribute to disease risk. It is likely that non-linear and complex interactions occur between these multiple factors, involving simultaneous pathological changes in diverse cell types, tissues, and organs, at multiple molecular levels. Recent technological advances have exponentially expanded the breadth of available -omics data, from genome, epigenome, transcriptome, proteome, metabolome to even the microbiome. Integration of multiple layers of information across several -omics domains, i.e., the so-called multi-omics approach, currently holds the promise as a path toward precision medicine. Indeed, a more meaningful interpretation of genotype-phenotype relationships and the development of successful therapeutics tailored to individual patients are urgently needed. In this review, we will summarize recent findings and applications of integrative multi-omics in elucidating the etiology of CAD/MI; with a special focus on established disease susceptibility loci sequentially identified in genome-wide association studies (GWAS) over the last 10 years. Moreover, in addition to the autosomal genome, we will also consider the genetic variation in our “second genome”—the mitochondrial genome. Finally, we will summarize the current challenges in the field and point to future research directions required in order to successfully and effectively apply these approaches for precision medicine.
Introduction
In the current era of high-potency statin therapy it becomes increasingly clear that even individuals with normal LDL-cholesterol levels without any conventional risk factors may develop atherosclerosis (1). The most pertinent manifestation of atherosclerosis is coronary artery disease (CAD), a highly complex disease, influenced by both multiple genetic risk variants and lifetime exposure to an atherogenic environment (2). A better understanding of the etiology of CAD and directions toward hitherto therapeutically not addressed disease mechanisms are urgently demanded (3). During the last 10 years, the genetic risk has been thoroughly explored in numerous genome-wide association studies (GWAS), leading to identification of >300 chromosomal loci which all significantly affect the risk of CAD (4–15). More than 90% of these common disease risk variants are located outside the protein-coding regions and have modest effect sizes (2, 16). Collectively they explain only ~25% of the overall disease heritability. This suggests that genetic variation may contribute to disease risk in a non-linear, interactive and complex way (17), leading to pathological changes in diverse cell types, tissues, and organs, at multiple molecular levels (18).
Recent technological advances have exponentially expanded the breadth of available -omics data (17). High-throughput monitoring of the abundance of various biological molecules and determination of their variation between different conditions on a global scale has become possible, promoting a paradigm shift in the way we approach biomedical problems (19). At the same time, it has been increasingly recognized that no single type of data can fully capture the intricacy of most complex molecular traits that manifest collectively as disease phenotypes (20–22). Rather, it is the integration of multiple layers of information across several -omics domains, i.e., the so-called multi-omics approach [also referred to as integromics or panomics (19)], that holds the promise for precision medicine (Figure 1) (19).
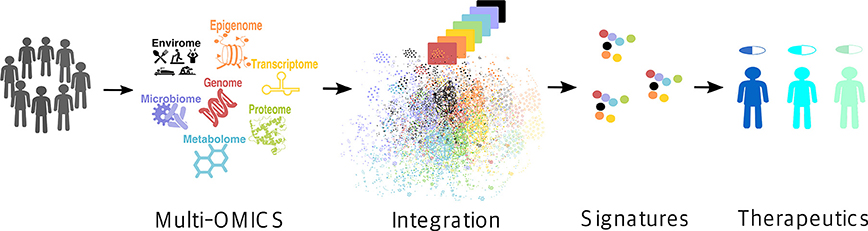
Figure 1. Multi-omics approach for precision medicine. Multi-omics (i.e., genome, epigenome, transcriptome, proteome, metabolome, microbiome, and envirome) data are collected from patients and integrated to create their individual molecular signatures (i.e., complex biomarkers), which are then used to select an appropriate drug for a particular patient, thus improving the treatment efficiency and reducing the possible side effects.
Of note, integrative analysis across multiple-omics layers can be conducted in two ways (Figure 2): pair-wise data integration and multi-dimensional i.e., network-based integration (22). Furthermore, pair-wise integrations can be divided into genetic and non-genetic correlations (22). In the first case, DNA variants (i.e., allelic distributions of single-nucleotide polymorphisms; SNPs) are tested for association with down-stream omics markers such as transcriptomic alterations, protein, metabolite or methylation levels or quantitative and qualitative measures of microbiome, via the so called quantitative trait loci (QTL) mapping. In the second scenario, one would explore correlations between down-stream omics data, e.g., correlation of CpG methylation levels to transcript expression or between metabolome and gut microbiome, however it may be difficult to infer causal relationships in such case (22). Considering the largely unexplored role of the established CAD risk loci from GWAS (23) and the central dogma that genetic variations control the transcriptome, which in turn affects e.g., the proteome (20), and metabolome (Figure 2, middle panel), our main focus will be pair-wise integrations linking genetic variation related to CAD risk to other down-stream omics layers such as epigenome, transcriptome, proteome or metabolome. Although multi-dimensional integrations have been widely used in the field of cancer research, their application in the context of CAD has so far been limited (22). Moreover, in addition to the autosomal genome, we will also consider the genetic variation in our “second genome”—the mitochondrial genome and its contribution to CAD.
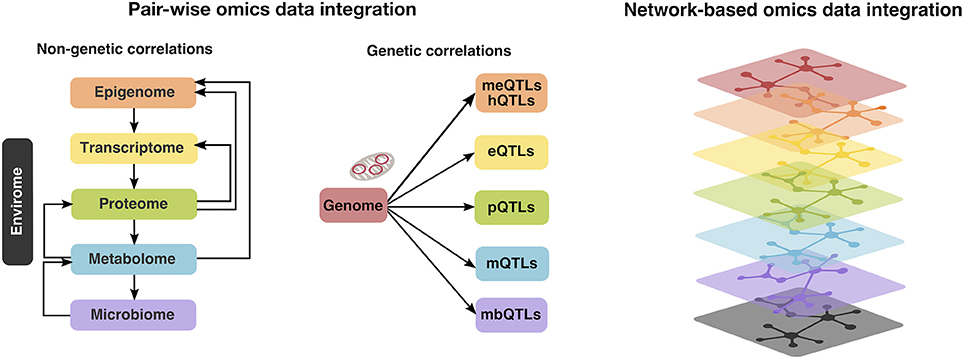
Figure 2. Multi-omics (i.e., autosomal and mitochondrial genome, epigenome, transcriptome, proteome, metabolome, microbiome, and envirome) data integration can be conducted in two ways: pair-wise integrations, which can be further divided into non-genetic (left panel) and genetic correlations (middle panel). In the first case, one would examine the correlation patterns between the down-stream omics layers (e.g., metabolome and gut microbiome), whereas the second is achieved via the so called quantitative trait loci (QTL) mapping, linking genetic variation to methylation levels (meQTLs) or histone modifications (hQTLs), transcriptome (expression QTLs; eQTLs), protein (pQTLs), metabolite (mQTLs) or measures of microbiome (mbQTLs). Alternatively, multi-dimensional i.e., network-based integration approaches (right panel) exist, however their application in the context of CAD has so far been limited (22).
Integrating Genetic Variation and Epigenome
Epigenomic signatures reflect various DNA modifications and may affect gene regulatory mechanisms that do not involve changes in the DNA sequence per se. Thereby, epigenomics may become a critical mediator of environmental influences and risk factors acting on the genome (20, 24). Three unique, but highly interrelated, epigenetic processes can be distinguished: DNA methylation, histone modifications (e.g., methylation, acetylation, phosphorylation, DP-ribosylation, and ubiquitination) and RNA-based mechanisms (e.g., microRNAs, long non-coding RNAs or lncRNAs, small interfering RNAs) (20, 24). Although, technically non-coding RNAs belong to the epigenome (20), we will discuss them in the next section, as the respective omics data are acquired via transcriptome profiling (RNA-seq).
DNA methylation and histone modifications are the best understood of the epigenetic mechanisms thus far and have been widely suggested to regulate gene expression and affect CAD risk factors including atherosclerosis, inflammation, hypertension and diabetes (25). DNA methylation consists of the covalent methylation of the C5 position of cytosine residues, when they are followed by guanine residues (CpG dinucleotides). It is partly heritable but it is also a dynamic process related to environmental stimuli and life style factors (26). Hedman et al. (27) analyzed epigenetic changes associated with lipid concentrations and identified a number of meQTLs, enriched in signals from GWAS on lipid levels and CAD. For example, genome-wide significant variants (rs563290 and its proxies), associated with LDL cholesterol and CAD at APOB, were meQTLs for a LDL cholesterol-related differentially methylated locus (Table 1 and Figure 3).
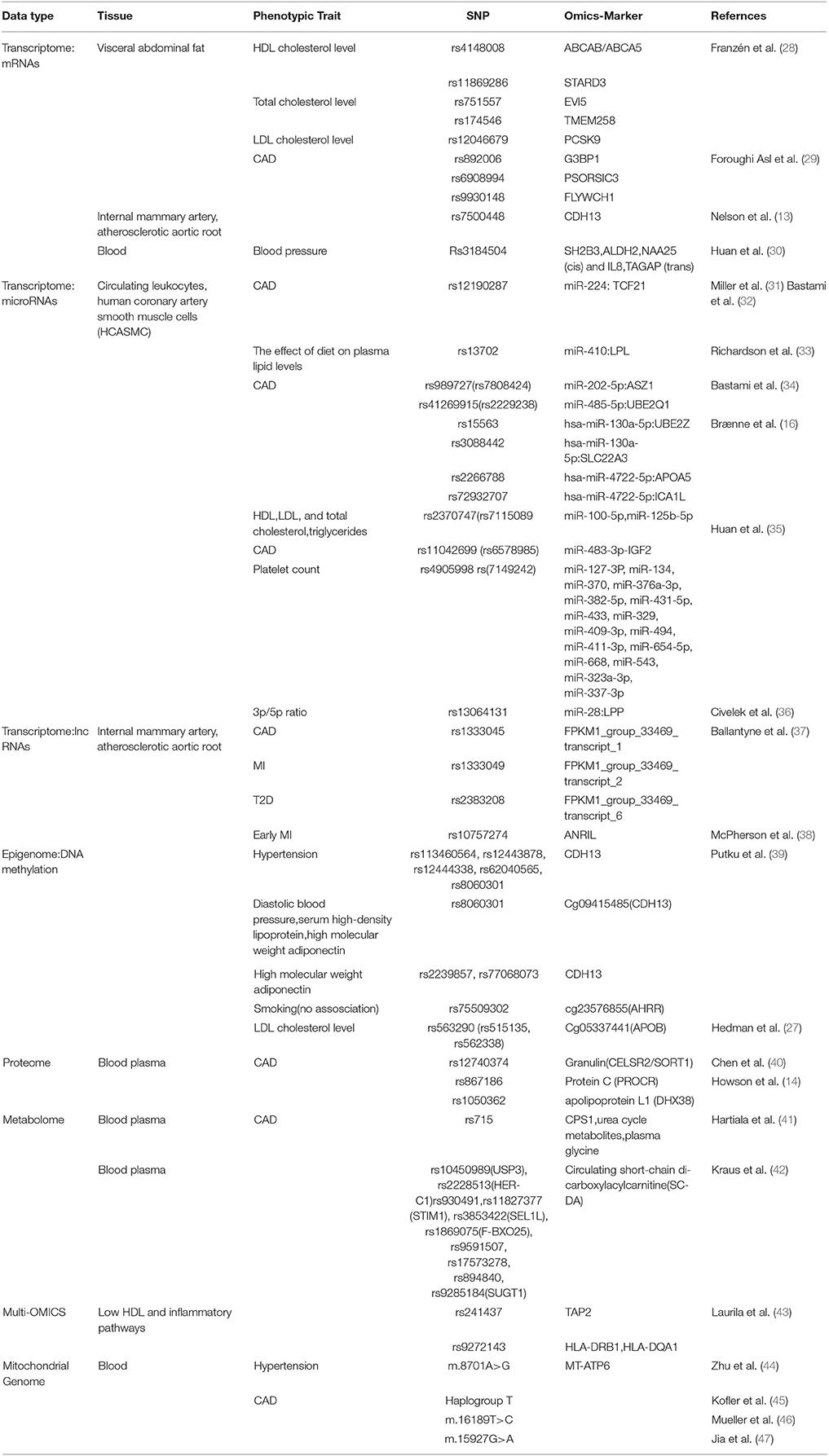
Table 1. Genetic variation related to CAD/MI risk that has been associated with other down-stream omics layers such as transcriptome (mRNA, microRNAs and lncRNAs), epigenome, proteome or metabolome.
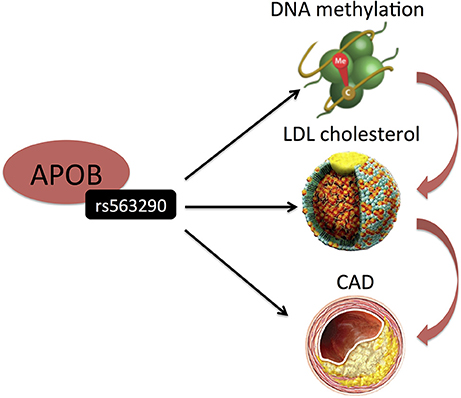
Figure 3. Hedman et al. (27) identified SNP (rs515135) in an intron of APOB to be associated with LDL-C. Its proxy was also associated with CAD. Interestingly, this SNP represents a cis-meQTL. Black arrows indicate association findings. Red arrows indicate the presumed functional cascade leading to CAD.
Furthermore, the CDH13 (T-cadherin) locus may present an interesting example in the context of epigenetics and CAD. Putku et al. (39) reported several genetic variants in the promoter of CDH13 as meQTLs in hypertension patients (Table 1), several of them being also associated with high molecular weight adiponectin, a known ligand for CDH13, the binding of which results in increased proliferation and migration of endothelial cells (39). Moreover, recently Nelson et al. (13) identified a genetic variant in the intron of CDH13, which affects expression of this gene in vascular tissues, and is genome-wide significantly associated with CAD (28) (Table 1). Interestingly, the expression levels of CDH13 and lncRNAs from the same locus showed positive correlations, suggesting a functional link, as lncRNAs are known to display correlations with the expression of their neighboring protein-coding target genes (48).
An exciting field of future research will be studies conducting parallel profiling of genetic variation with histone modifications and Hi-C and ChIA-PET-based chromatin contact maps to uncover local and distal histone quantitative trait loci (hQTLs) (49) in CAD patients.
Overall, considering the critical role of epigenetic modifications as a critical mediator of environmental influences on the genome (20, 24), we urgently need more investigations studying DNA methylation and other epigenetic modifications genome-wide and in large enough cohorts, ideally also elucidating the differences between tissues and cells in healthy vs. CAD patients. Moreover, this should be supplemented with careful documentation of multiple environmental and lifestyle factors over time, i.e., the envirome, as well as comprehensive clinical information to draw a link between the environment and CAD.
Integrating Genetic Variation and Transcriptome
Transcriptomics reflect genome-wide measures of RNA levels, both protein-coding RNA as well as the non-coding RNAs (i.e., microRNAs, lncRNAs, and small interfering RNAs) under specific conditions or in a specific cell. Moreover, the transcript levels are examined both qualitatively (i.e., which transcripts are present, identification of novel transcripts, splice sites, and RNA editing sites) and quantitatively (quantification of transcript abundance) (21).
Protein-Coding RNAs
Parallel assessments of genetic variation and transcriptome profiles across disease-relevant tissues, i.e., via mapping expression quantitative trait loci (eQTLs) to identify susceptibility genes (mainly protein-coding), has been the most commonly applied approach (28, 29, 50–52). Björkegren et al. have performed a number of integrative network analysis, linking CAD risk variants and transcriptome data in seven disease-relevant vascular and metabolic tissues, collected from up to 600 CAD patients during coronary artery bypass surgery (28, 29, 53, 54). From these investigations, visceral abdominal fat has emerged as an important gene-regulatory site for blood lipids. Several risk SNPs for HDL-, LDL-, and total cholesterol levels, as well as for CAD demonstrated significant eQTL effects in visceral abdominal fat (28, 29).
Huan et al. (30) also used integrative analysis to investigate the molecular mechanisms of blood pressure regulation and identified a blood pressure associated SNP (rs3184504) in SH2B3, also associated with the expression (eQTL) of several genes, including SH2B3, in the genetically inferred causal blood pressure gene sets (Table 1 and Figure 4). Some of these genes were also perturbed in Sh2b3−/− mice, demonstrating blood pressure-related phenotype (30). Rs3184504 has been previously also associated with CAD risk (9).
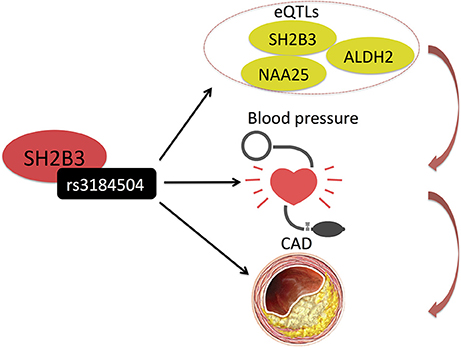
Figure 4. Huan et al. (30) uncovered a blood pressure associated SNP (rs3184504) in SH2B3, which also associates with the expression (eQTL) of several genes, including SH2B3 itself, in the genetically inferred causal blood pressure gene sets. Rs3184504 has been previously also associated with CAD risk. (9) Black arrows indicate association findings. Red arrows indicate the presumed functional cascade leading to CAD.
Much less investigated are non-coding RNA transcripts, such as micro-RNAs (miRNAs) and long non-coding RNAs (lncRNAs). Recent evidence suggests that at least some of these may play a role in CAD (55–58). Although, technically non-coding RNAs belong to the epigenome (20), we will discuss them in this section, as the respective omics data are acquired via transcriptome profiling (RNA-seq).
Micro RNAs
MiRNAs are involved in the transcriptional control of all main cell types participating in atherosclerosis progression, including endothelial cells, vascular smooth muscle cells, and macrophages (32, 59). Several studies have investigated the differential expression patterns of miRNAs in plasma/serum, microparticles, whole blood, platelets, blood mononuclear intimal, and endothelial progenitor cells in CAD vs. non-CAD patients, as summarized by Malik et al. (60). In majority of cases, up-regulation of different miRNA in CAD patients was observed (60). Moreover, growing body of evidence suggests that genetic variations in the miRNA targetome may lead to major deleterious outcomes (61, 62). For example, Miller et al. (31) have shown that an established CAD risk variant (rs12190287) resides in the 3′ untranslated region of a transcription factor TCF21 and alters the seed binding sequence for miR-224. Moreover, allelic imbalance studies in circulating leukocytes and human coronary artery smooth muscle cells have demonstrated a significant imbalance of the TCF21 transcript levels, which correlated with genotype at rs12190287, consistent with this variant contributing to allele-specific expression differences (31). Richardson et al. (33) have reported that a variant (rs13702) in the 3'-UTR of lipoprotein lipase (LPL) disrupts the binding of miR-410 and modulates the effect of diet on plasma lipid levels (33). Recently, Bastami et al. (34) performed a more systematic computational screening, by mapping the established CAD risk variants to the miRNA targetome, identifying several links between SNPs and miRNAs (Table 1; https://www.ebi.ac.uk/gwas/). In a recent study from our group (16), we also mapped CAD risk variants from the CARDIoGRAMplusC4D GWAS meta-analyses (9), to 3′ UTR regions of genes to assess their overlaps with predicted target miRNA binding sites. Interestingly, the 3′ UTR region of MRAS was predicted to be targeted by 29 miRNAs and 23 miRNAs were predicted to bind more than one candidate CAD gene (Table 1). Thus far, there have been relatively few studies investigating genome-wide miRNA eQTLs (miR-eQTLs). Huan et al. (35) identified a genetic variant (rs2370747) associated with miR-100-5p and miR-125b-5p expression, a proxy SNP of which was also associated with lipid traits (HDL-, LDL-, and total cholesterol as well as triglycerides). Moreover, it was found that both miRNAs were also differentially expressed in relation to HDL cholesterol (35).
Civelek et al. (36) examined the genetic regulation of human adipose miRNA expression and its consequences for metabolic traits. Interestingly, this study showed, how genetic variation might influence the processing of miRNAs, i.e., the ratio of miRNA expression from the 3p and 5p arms. It is known that a miRNA precursor can give rise to two mature miRNAs from the 3p and 5p arm, one of which usually having higher expression than the other. The 3p/5p ratios of several miRNAs have been shown to be significantly different among various healthy tissues (63) and altered in pathological conditions compared with healthy controls (64). Civelek et al. demonstrated a significant association of the SNP rs13064131 with the 3p/5p ratio of miR-28, encoded from the LPP gene (Figure 5) (36). However, the SNP was not associated with the expression levels of the LPP transcript itself or with the abundance of miR-28-3p or miR-28-5p, suggesting that its effect on the 3p/5p ratio may be independent of transcription, possibly via degradation or stabilization mechanisms.
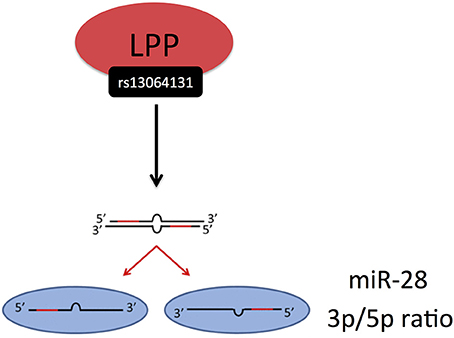
Figure 5. Civelek et al. (36) demonstrated a significant association of the SNP rs13064131 with the 3p/5p ratio of miR-28, encoded from the LPP gene. The miRNA processing and strand selection was adapted from (65).
Long Non-Coding RNAs
The recent discovery of an extensive catalog of lncRNAs—i.e., long RNA transcripts that do not code for proteins—has opened a new perspective on the importance of the RNA-based mechanisms in gene regulation (24). LncRNAs are emerging as important regulators of various cellular processes, with many possible implications in cardiovascular disease pathophysiology (57, 58). In fact, the most prominent CAD risk locus at Chr9p21 (66, 67) harbors the lncRNA—ANRIL (Antisense Non-coding RNA in the INK4 Locus, CDKN2B antisense RNA). From these, rs10757274 is the strongest genetic predictor of early MI and is not associated with established CAD risk factors such as lipoproteins or hypertension, making ANRIL a key candidate (38). Interestingly, ANRIL is found both as a linear lncRNA (linANRIL), the transcript levels of which are known to positively correlate with disease severity (68), and is also capable of forming RNA circles (circANRIL) (69). Recently, Holdt et al. (69) demonstrated that circANRIL regulates the maturation of precursor ribosomal RNA (pre-rRNA), by this impairing ribosome biogenesis and inducing nucleolar stress and apoptosis in vascular smooth muscle cells and macrophages (Figure 6). Carriers of the CAD-protective haplotype at 9p21 showed significantly increased expression of circANRIL (69).
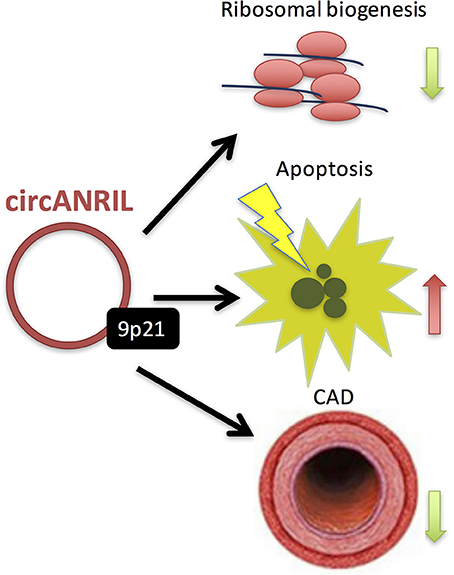
Figure 6. Recently, Holdt et al. (69) demonstrated that circANRIL regulates the maturation of precursor ribosomal RNA (pre-rRNA), by this impairing ribosome biogenesis and inducing nucleolar stress and apoptosis in vascular smooth muscle cells and macrophages. Moreover, carriers of the CAD-protective haplotype at 9p21 showed significantly increased expression of circANRIL.
Currently, there have not been many large-scale studies on lncRNAs in the context of CAD, though. Ballantyne et al. (37) recently conducted a genome-wide interrogation of long intergenic non-coding RNAs (lincRNAs) that associate with cardiometabolic traits in GWAS, including CAD and also identified a number of CAD/MI and type 2 diabetes associated SNPs at Chr9p21 that overlapped lincRNA transcripts (Table 1) (37). In STARNET (28), 5.4% of the identified cis-expression quantitative trait loci (eQTLs) were related to the expression of lncRNAs, however these have not been further explored, so far. Overall, more studies focusing on non-coding RNAs in different CAD relevant tissues in large enough cohorts will be required to yield insights into the possible functional roles of this portion of transcriptome and its genetic determinants, in healthy and disease states. Moreover, considering that lncRNAs are generally found to be more lowly-expressed, sufficient depth of coverage for RNA-seq experiments will need to be guaranteed (21).
Integrating Genetic Variation and Proteome
Proteomics uses high-throughput approaches (mainly MS-based) to quantify protein abundance, post-translational modifications and interactions (e.g., using phage display and yeast two-hybrid assays) in a tissue, cell or fluid compartment, such as plasma or urine (21). Considering that the transcriptome is not linearly proportional to proteome, that proteins are the biomolecules that execute cellular functions, and that many human diseases ultimately result from alterations in the proteome (70), such studies are urgently needed to facilitate the explorations of CAD etiology. However, proteome studies are still rare in relation to CAD, mostly due to the complex methodology involved. There have been some investigations in the past few years, aiming at characterizing the proteomes of several CAD-related tissues and cell types, including human arterial smooth muscle cells (71), platelets (72), as well as body fluids such as urine (73).
Only few studies (14, 40) have analyzed genetic variants that modify protein levels, i.e., the so-called protein quantitative trait loci (pQTLs) (Table 1). Chen et al. (40) assayed a pre-selected set of plasma proteins, identifying several pQTLs that overlapped with CAD risk SNPs and also explained a substantial proportion of inter-individual variation in protein abundance. For example, rs12740374 at the CELSR2/SORT1 locus, a variant associated with lipids and CAD, explained 15% of inter-individual variation in plasma granulin levels (Figure 7). Interestingly, progranulin binds to SORT1 and Sort1 knockout mice show markedly elevated levels of progranulin (40). Recently, it was also demonstrated that progranulin is involved in lysosomal homeostasis and lipid metabolism (74).
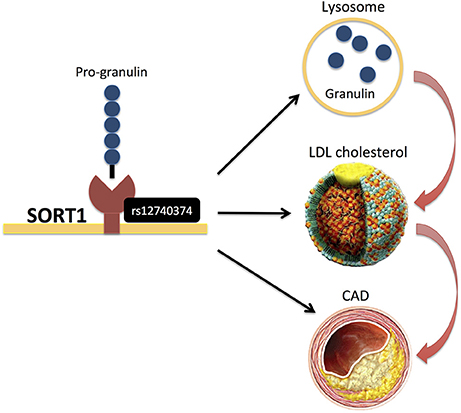
Figure 7. rs12740374 at the CELSR2/SORT1 locus, (40) a variant associated with lipids and CAD, was recently found to display pQTL effects on plasma granulin levels, and pro-granulin is known to bind to SORT1. More recently, it was also demonstrated that progranulin is involved in lysosomal homeostasis and lipid metabolism (74).
As the proteomics technologies improve over time (21), more genome-wide investigations of CAD-related alterations in proteome and also phosphorpoteome in increasing numbers of disease relevant tissues are expected to be conducted in the near future. However, as proteins are more sensitive to their environment (21), caution will have to be taken during sample preparation steps to obtain accurate and reproducible results.
Integrating Genetic Variation and Metabolome
An important additional functional layer in mutli-omics data integration is the metabolome, as it represents an integrated state of all genetic, epigenetic and environmental factors, thus providing a link between genotype and phenotype (75). Metabolomics is an omics field that systematically identifies and quantifies multiple small molecule (typically < 1,500 Daltons) types, such as amino acids, fatty acids, carbohydrates and biochemical intermediates, i.e., metabolites (21). A plethora of metabolites in blood and urine have been associated with CAD and subsequent cardiovascular events (76–79) and have been demonstrated as promising biomarkers discriminating CAD vs. non-CAD subjects (78), as well as between thrombotic MI and stable CAD cases (80). Kraus et al. (42) recently identified several genetic loci demonstrating associations with blood plasma metabolites (i.e., metabolomic quantitative trait loci; mQTLs), the strongest findings being for the circulating short-chain dicarboxylacylcarnitine (SCDA) metabolite levels with variants in genes that regulate components of endoplasmic reticulum (ER) stress (Table 1 and Figure 8) (42).
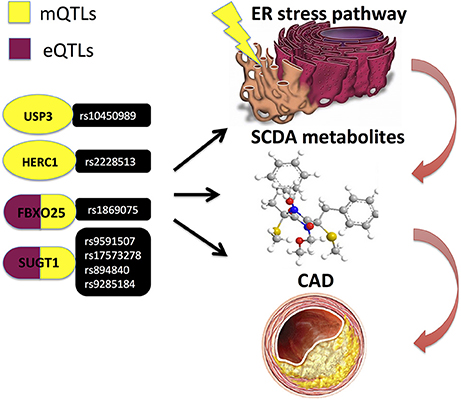
Figure 8. Kraus et al. (42) performed a pathway-level integrative analyses and observed associations of circulating short-chain dicarboxylacylcarnitine (SCDA) with variants in ER stress genes, whereof several genetic variants in FBXO25 and SUGT1 genes also demonstrated evidence of cis-regulation in expression quantitative trait loci (eQTL) analyses and independently predicted CAD events.
Besides blood and urine, metabolomic profiles of vascular and metabolomic tissues such as subcutaneous fat will need to be generated, ideally in conjunction with other omics layer data. Especially, gut microbiome would be of utmost interest, considering the close link between the two (81).
However, of note, metabolic profiles are even more prone to variability affected by sample preparation and storage conditions, as well as by several other factors including patient heterogeneity (21). Hence, the required sample size has to be carefully considered, to inspire confidence in the generated results.
Integrating Genetic Variation and Microbiome
Microbiomics investigates all the microorganisms of a given community, including bacteria, viruses, and fungi, collectively known as the microbiota (and their genes constituting the microbiome) (21). The human microbiome is enormously complex and there are substantial variations in microbiota composition between individuals resulting from seed during birth and development, diet and other environmental factors, drugs and age (21). Thousands of different bacterial species make up the human microbiomes, from which there is a small number of abundant species and a large number of rare or low abundance species, the differential functions of which remain poorly understood (82). Currently, several large scale initiatives are emerging including the American Gut Project http://americangut.org/ and the British Gut Project http://britishgut.org/, which are expected to produce a rich collection of anonymised human gut samples and lifestyle information for medical researchers.
Gut microbiome has emerged as another rich source of information and as a possible new player contributing to the CAD/MI pathogenesis (82–84). It has long been known that bacteria activate inflammatory pathways, and recent data demonstrate that the gut microbiome may also affect lipid metabolism and influences the development of obesity and atherosclerosis (84), suggesting that gut microbiota could be used as a diagnostic marker for CAD (85). The most investigated is the association between gut microbiota and fasting plasma trimethylamine N-oxide (TMAO) levels, a gut microbiota-dependent metabolite, previously also associated with CAD and stroke (81, 86). Org et al. (81) demonstrated that certain blood plasma metabolites strongly correlated with gut microbial community structure and that some of these correlations may be specific for the pre-diabetic state. LeChatelier et al. (84) used qunatitative gut microbiome information to distinguish between individuals with “high bacterial richness” and “low bacterial richness,” were the latter were characterized by increased adiposity, insulin resistance and dyslipidemia in addition to a more pronounced inflammatory phenotype. Le Chatelier Fu et al. (84) and Fu et al. (87) reported that gut microbiota richness and diversity were negatively correlated with triglycerides and positively correlated with HDL levels, however this effect was independent of age, sex and host genetics. So far, genome-wide mapping of the so-called microbiome quantitative trait loci (mbQTLs) (88) in the context of CAD has not been performed and is definitely next in line, ideally in conjunction with comprehensive profiling of metabolome in several tissues and body fluids in large enough cohorts.
Integrating Genetic Variation and Multiple Omics Datasets
An integrative analysis of genetic variation and transcriptome with additional high-throughput measurements may greatly improve the predictive power of disease networks. Zhu et al. (89) However, the number of studies conducting multi-omics integrations in the context of CAD is limited so far. Miller et al. (90) integrated genetic variation with investigations of chromatin state, enhancer activity and TF binding in human coronary artery smooth muscle cells and demonstrated, for example, that one of the lead candidate variants, rs17293632, located within an intergenic region of the SMAD3 gene, overlaps an open chromatin region. Moreover, it was observed that the major risk C allele was more associated with open chromatin and resided in a canonical AP-1 motif, which was effectively destroyed by the minor protective T allele. Preferential AP-1 binding to the risk C allele was experimentally validated using allele-specific ChIP analyses. Miller et al. (90) and Kraus et al. (42) performed a pathway-level integrative analyses, linking genetics, epigenetics, transcriptomics, and metabolomics profiles and implicating the ubiquitin proteasome system in cardiovascular disease pathogenesis. This study observed associations of circulating short-chain dicarboxylacylcarnitine (SCDA) with variants in ER stress genes, whereof several genetic variants (Table 1 and Figure 8) in FBXO25 and SUGT1 genes also demonstrated evidence of cis-regulation in expression quantitative trait loci (eQTL) analyses and independently predicted CAD events (42). Moreover, two other genes from the same ER stress pathway—BRSK2 and HOOK2—were identified as differentially methylated, when comparing individuals with high and low SCDA levels. Subsequently, experimental validation using culture of human kidney cells in the presence of levels of fatty acids found in individuals with cardiometabolic disease, demonstrated induced accumulation of SCDA metabolites in parallel with increases in the ER stress marker BiP (42).
Shu et al. (20) investigated shared genetic regulatory networks for CAD and type 2 diabetes (T2D) and their key intervening drivers in multiple populations of diverse ethnicities by performing an integrative analysis of five multi-ethnic GWAS for CAD and T2D, eQTLs, ENCODE, as well as tissue-specific gene network models (both co-expression and graphical models) from disease-relevant tissues. This study identified pathways regulating the metabolism of lipids, glucose and branched-chain amino acids, as well as pathways governing oxidation, extracellular matrix and immune response as shared pathogenic processes for both diseases and identified 15 key drivers including HMGCR, CAV1, IGF1, and PCOLCE, whose network neighbors collectively accounted for ~35% of known GWAS hits for CAD and 22% for T2D (20). Laurila et al. (43) applied a combined approach using both QTLs and canonical pathway analysis to link genomics and transcriptome analysis from the subcutaneous adipose tissue and plasma HDL lipidomics profiling, highlighting change in HDL particle quality toward putatively more inflammatory and less atheroprotective phenotype in subjects with low HDL, due to their reduced antioxidative capacity. Within the HLA region, this study found two significant, dose-dependent cis-eQTL associations with low HDL and inflammatory pathways: rs241437 in the intron of TAP2 and rs9272143 between HLA-DRB1 and HLA-DQA1, the latter also being associated with down-regulation of antioxidative pathways in HDL particles (43).
The application of multi-omics integrations in the field of CAD has so far been limited (22). Obviously, one of the main reasons for this is the current lack of appropriate data in large enough cohorts. However, considering the great promise such studies hold for precision medicine, it is expected that parallel measurements on multiple omics layers will be rapidly collected during the next couple of years, allowing also a comprehensive comparison, validation and improvement of the existing computational integration methods.
Mitochondrial Genetic Variation and Downstream Omics Datasets
Dysfunction of mitochondria has been increasingly associated with obesity-related cardiometabolic diseases and CAD (91). Thus, genetic variation in the mitochondrial DNA (mtDNA), which codes for the 37 OXPHOS genes as well as further >1000 nuclear-coded genes imported into mitochondria constituting essential components for their proper functioning, needs exploration for a better understanding of CAD genetics. The mitochondrial haplogroup T (45) and mtDNA variants m.16189T>C (46) and m.15927G>A (47) have been associated with CAD in different ethnic groups. Another mitochondrial variant, m.8701A>G, has been associated with hypertension (44). This variant is located in MT-ATP6 (ATP synthase/complex V F0 subunit 6) gene, which is part of the ATP synthase enzyme, responsible for the final step of oxidative phosphorylation, and, on the functional level, using transmitochondrial hybrid cells (cybrids), it has been shown that it alters mitochondrial matrix pH and intracellular calcium dynamics (Figure 9) (92).
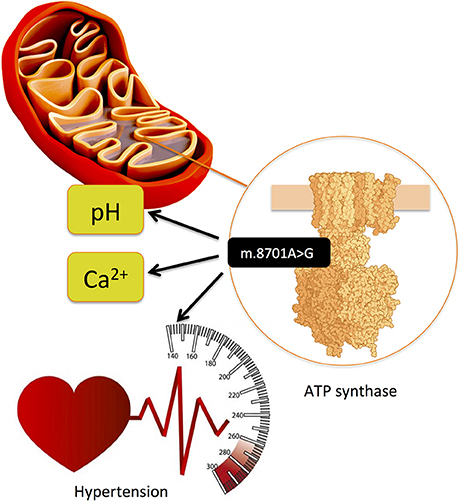
Figure 9. Mitochondrial variant m.8701A>G is located in MT-ATP6 (ATP synthase/complex V F0 subunit 6) gene, which is part of the ATP synthase enzyme, responsible for the final step of oxidative phosphorylation and has been associated with hypertension. (44) On the functional level, using transmitochondrial hybrid cells (cybrids), it has been shown that it alters mitochondrial matrix pH and intracellular calcium dynamics (92).
Similarly, other mitochondria-related omics data investigations could be of interest in the context of CAD, as Baccarelli et al. (93) reported that ATP synthesis genes including protein-encoding cytochrome c oxidase genes (MT-CO1, MT-CO2, and MT-CO3) and MT-TL1 were hypermethylated in platelets of CAD cases as compared to healthy controls (93). Using eQTLs in seven CAD relevant vascular and metabolic tissues (53) in conjunction with established CAD risk loci from GWAS (9) and time-resolved transcriptome data in the aortic arch in mice with reversible hypercholesterolemia (94, 95) we recently demonstrated a massive down-regulation of nuclear-encoded mitochondrial genes (96), specifically at the time of rapid atherosclerotic lesion expansion and foam cell formation, which was largely reversible by genetically lowering plasma cholesterol. Both mitochondrial signature genes were supported as causal for CAD in humans, as eQTLs representing their genes significantly overlapped with disease risk SNPs. In line with this, the STARNET (28) study recently examined mitochondrial (i.e., mtDNA-derived) gene expression and a markedly lower expression of mitochondrial genes in the atherosclerotic aortic arterial wall as compared to non-atherosclerotic arterial wall.
Furthermore, genetic variation of mitochondrial metabolome has remained largely unexplored. Hartiala et al. (41) searched for genetic factors associated with plasma betaine levels and determined their effect on CAD risk. This resulted in the identification of two significantly associated loci on chromosomes 2q34 and 5q14.1. The lead variant on 2q24—rs715—localized to carbamoyl-phosphate synthase 1 (CPS1), which encodes a mitochondrial enzyme that catalyzes the first committed reaction and rate-limiting step in the urea cycle. Rs715 was also significantly associated with decreased levels of urea cycle metabolites and increased plasma glycine levels. Finally, rs715 yielded a strikingly significant and protective association with decreased risk of CAD in women (41).
Finally, in recent years, it has become increasingly evident that the gut microbiome produces metabolites that influence mitochondrial function and biogenesis (97), hence the ancestral gut microbiome-mitochondrion connection and its relation to CAD might need to be explored in the near future, as well.
Resent progress in next-generation sequencing (NGS) techniques has set a scene for a second “gold rush” in mitochondrial genomics and mtDNAs are presently the most sequenced type of eukaryotic chromosome (98). At the same time, multi-omics investigations in mitochondria, mapping the genomes, transcriptomes, proteomes, and metabolomes in parallel, apart from yeast (99) have not been conducted yet. Hence, although, mitochondrial dysfunction has been associated with many human diseases, the respective proteins and pathways are not well-characterized (99), presenting an exciting future field of investigation, especially considering the fact that mitochondria play a key role in plasticity and adaptation to environmental change, including adaptation to physiological stress (100).
Conclusions and Future Directions
Given that CAD like other common complex disorders develops over time and involves both genetics and environment, full mechanistic insight will require coordinated sets of several-omics data at multiple time points, collected from many disease relevant tissues and body fluids in large enough cohorts (20, 21). Environmental risk factors can interact with the genome and perturb the epigenome to further modulate the transcriptome and proteome (20). Therefore, comprehensive monitoring and careful documentation of multiple environmental and lifestyle factors over time, i.e., the envirome, will be indispensable to yield significant insights into the complex etiology of CAD. Moreover, imaging and electronic health record data also will need to be considered. As more-omics and other data are generated, novel methods for efficient data integration, modeling, visualization and interpretation will be urgently needed to efficiently cope with this multi-dimensional data (101), and translate it into actionable precision medicine tools. Although, there has been major progresses in the development of multidimensional data integration algorithms and tools, the field is still in its infancy and the flexibility, effectiveness and robustness of data integration to extract biological insights is still restricted, especially when clinical outcomes (e.g., stable CAD vs. MI) need to be modeled (22, 101). In addition we still face a number of technical challenges related to patient sampling and profiling. For example, as already recognized by Hasin et al. and others (20, 21) human studies are often affected by various confounding factors, which are difficult or even impossible to control for (e.g., diet and medications). Clearly, also the available sample size will play an important role for the multi-omics approach to produce meaningful insights into CAD (21) and allow the generation of reliable prediction models for more efficient design of therapeutics, tailored to individual needs. According to Hasin et al. an underpowered study may not only miss true signals, but is also more likely to produce false positive results (21). Furthermore, already before and during data collection, careful attention has to be paid to data analysis requirements, e.g., sufficient depth of coverage for RNA-seq experiments (21).
Author Contributions
BV and HS drafted and edited the manuscript.
Funding
This work was supported by grants from the Fondation Leducq [CADgenomics, 12CVD02], the German Federal Ministry of Education and Research (BMBF) within the framework of ERANET on Cardiovascular Disease, Joint Transnational Call 2017 [ERA-CVD: grant JTC2017_21-040], within the framework of target validation [BlockCAD: 16GW0198K], within the framework of the e:Med research and funding concept [AbCDNet: grant 01ZX1706C and e:AtheroSysMed: grant 01ZX1313A-2014], and the European Union Seventh Framework Programme FP7/20072013, under grant agreement no. HEALTH-F2-2013-601456 (CVgenes-at-target). Further grants were received from the Deutsche Forschungsgemeinschaft (DFG) as part of the Sonderforschungsbereich CRC 1123 (B2).
Conflict of Interest Statement
The authors declare that the research was conducted in the absence of any commercial or financial relationships that could be construed as a potential conflict of interest.
References
1. Fernández-Friera L, Fuster V, López-Melgar B, Oliva B, García-Ruiz JM, Mendiguren J, et al. Normal LDL-cholesterol levels are associated with subclinical atherosclerosis in the absence of risk factors. J Am Coll Cardiol. (2017) 70:2979–91. doi: 10.1016/j.jacc.2017.10.024
2. Kessler T, Vilne B, Schunkert H. The impact of genome-wide association studies on the pathophysiology and therapy of cardiovascular disease. EMBO Mol Med. (2016) 8:688–701. doi: 10.15252/emmm.201506174
3. Ference BA, Ginsberg HN, Graham I, Ray KK, Packard CJ, Bruckert E, et al. Low-density lipoproteins cause atherosclerotic cardiovascular disease. 1. Evidence from genetic, epidemiologic, and clinical studies. A consensus statement from the European Atherosclerosis Society Consensus Panel. Eur Heart J. (2017) 38:2459–72. doi: 10.1093/eurheartj/ehx144
4. Erdmann J, Grosshennig A, Braund PS, König IR, Hengstenberg C, Hall AS, et al. New susceptibility locus for coronary artery disease on chromosome 3q22.3. Nat Genet. (2009) 41:280–2. doi: 10.1038/ng.307
5. Erdmann J, Willenborg C, Nahrstaedt J, Preuss M, König IR, Baumert J, et al. Genome-wide association study identifies a new locus for coronary artery disease on chromosome 10p11.23. Eur Heart J. (2011) 32:158–68. doi: 10.1093/eurheartj/ehq405
6. Schunkert H, König IR, Kathiresan S, Reilly MP, Assimes TL, Holm H, et al. Large-scale association analysis identifies 13 new susceptibility loci for coronary artery disease. Nat Genet. (2011) 43:333–8. doi: 10.1038/ng.784
7. Charchar FJ, Bloomer LD, Barnes TA, Cowley MJ, Nelson CP, Wang Y, et al. Inheritance of coronary artery disease in men: an analysis of the role of the Y chromosome. Lancet (2012) 379:915–22. doi: 10.1016/S0140-6736(11)61453-0
8. Erdmann J, Stark K, Esslinger UB, Rumpf PM, Koesling D, de Wit C, et al. Dysfunctional nitric oxide signalling increases risk of myocardial infarction. Nature (2013) 504:432–6. doi: 10.1038/nature12722
9. CARDIoGRAMplusC4D, Consortium Deloukas P, Kanoni S, Willenborg C, Farrall M, Assimes TL, et al. Large-scale association analysis identifies new risk loci for coronary artery disease. Nat Genet. (2013) 45:25–33. doi: 10.1038/ng.2480
10. Nikpay M, Goel A, Won HH, Hall LM, Willenborg C, Kanoni S, et al. A comprehensive 1,000 Genomes-based genome-wide association meta-analysis of coronary artery disease. Nat Genet. (2015) 47:1121–30. doi: 10.1038/ng.3396
11. Myocardial Infarction Genetics and CARDIoGRAM Exome Consortia Investigators, Stitziel NO, Stirrups KE, Masca NG, Erdmann J, Ferrario PG, et al. Coding Variation in ANGPTL4, LPL, and SVEP1 and the risk of coronary disease. N Eng J Med. (2016) 374:1134–44. doi: 10.1056/NEJMoa1507652
12. Webb TR, Erdmann J, Stirrups KE, Stitziel NO, Masca NG, Jansen H, et al. Systematic evaluation of peiotropy identifies 6 further loci associated with coronary artery disease. J Am Coll Cardiol. (2017) 69:823–36. doi: 10.1016/j.jacc.2016.11.056
13. Nelson CP, Goel A, Butterworth AS, Kanoni S, Webb TR, Marouli E, et al. Association analyses based on false discovery rate implicate new loci for coronary artery disease. Nat Genet. (2017) 49:1385–91. doi: 10.1038/ng.3913
14. Howson J.MM, Zhao W, Barnes DR, Ho WK, Young R, Paul DS, et al. Fifteen new risk loci for coronary artery disease highlight arterial-wall-specific mechanisms. Nat Genet. (2017) 49:113–9. doi: 10.1038/ng.3874
15. van der Harst P, Verweij N. The identification of 64 novel genetic loci provides an expanded view on the genetic architecture of coronary artery disease. Circ Res. (2017) 122:433–43. doi: 10.1161/CIRCRESAHA.117.312086
16. Brænne I, Civelek M, Vilne B, Di Narzo A, Johnson AD, Zhao Y, et al. Prediction of causal candidate genes in coronary artery disease loci. Arterioscler Thromb Vasc Biol. (2015) 35:2207–17. doi: 10.1161/ATVBAHA.115.306108
17. Ritchie MD, Holzinger ER, Li R, Pendergrass SA, Kim D. Methods of integrating data to uncover genotype-phenotype interactions. Nat Rev Genet. (2015) 16:85–97. doi: 10.1038/nrg3868
18. Hartiala J, Schwartzman WS, Gabbay J, Ghazalpour A, Bennett BJ, Allayee H. The genetic architecture of coronary artery disease: current knowledge and future opportunities. Curr Atheroscler Rep. (2017) 19:6. doi: 10.1007/s11883-017-0641-6
19. Manzoni C, Kia DA, Vandrovcova J, Hardy J, Wood NW, Lewis PA, et al. Genome, transcriptome and proteome: the rise of omics data and their integration in biomedical sciences. Brief Bioinformat. (2016) 19:286–302. doi: 10.1093/bib/bbw114
20. Arneson D, Yang X, Shu L. Bioinformatics principles for deciphering cardiovascular diseases. Encyclop Cardiovasc Res Med. (2018) 1:273–92. doi: 10.1016/B978-0-12-801238-3.99576-X
21. Hasin Y, Seldin M, Lusis A. Multi-omics approaches to disease. Genome Biology (2017) 18:83. doi: 10.1186/s13059-017-1215-1
22. Arneson D, Shu L, Tsai B, Barrere-Cain R, Sun C, Yang X. Multidimensional integrative genomics approaches to dissecting cardiovascular disease. Front Cardiovasc Med. (2017) 4:8. doi: 10.3389/fcvm.2017.00008
23. Erdmann J, Kessler T, Munoz Venegas L, Schunkert H. A decade of genome-wide association studies for coronary artery disease: the challenges ahead. Cardiovasc Res. (2018) 114:1241–57. doi: 10.1093/cvr/cvy084
24. Turgeon PJ, Sukumar AN, Marsden PA. Epigenetics of cardiovascular disease - a new “Beat” in coronary artery disease. Med Epigenet. (2014) 2:37–52. doi: 10.1159/000360766
25. Muka T, Koromani F, Portilla E, O'Connor A, Bramer WM, Troup J, et al. The role of epigenetic modifications in cardiovascular disease: a systematic review. Int J Cardiol. (2016) 212:174–83. doi: 10.1016/j.ijcard.2016.03.062
26. Fernández-Sanlés A, Sayols-Baixeras S, Subirana I, Degano IR, Elosua R. Association between DNA methylation and coronary heart disease or other atherosclerotic events: a systematic review. Atherosclerosis (2017) 263:325–33. doi: 10.1016/j.atherosclerosis.2017.05.022
27. Hedman, ÅK Mendelson MM, Marioni RE, Gustafsson S, Joehanes R, Irvin MR. Epigenetic patterns in blood associated with lipid traits predict incident coronary heart disease events and are enriched for results from genome-wide association studies. Circulation (2017) 10:e001487. doi: 10.1161/CIRCGENETICS.116.001487
28. Franzén O, Ermel R, Cohain A, Akers NK, Di Narzo A, Talukdar HA. et al. Cardiometabolic risk loci share downstream cis- and trans-gene regulation across tissues and diseases. Science (2016) 353:827–30. doi: 10.1126/science.aad6970
29. Foroughi Asl H, Talukdar HA, Kindt AS, Jain RK, Ermel R, Ruusalepp A, et al. Expression quantitative trait loci acting across multiple tissues are enriched in inherited risk for coronary artery disease. Circ Cardiovasc Genet. (2015) 8:305–15. doi: 10.1161/CIRCGENETICS.114.000640
30. Huan T, Meng Q, Saleh MA, Norlander AE, Joehanes R, Zhu J, et al. Integrative network analysis reveals molecular mechanisms of blood pressure regulation. Mol Syst Biol. (2015) 11:799. doi: 10.15252/msb.20145399
31. Miller CL, Haas U, Diaz R, Leeper NJ, Kundu RK, Patlolla B, et al. Coronary heart disease-associated variation in TCF21 disrupts a Mir-224 binding site and miRNA-mediated regulation. PLoS Genet. (2014) 10:e1004263. doi: 10.1371/journal.pgen.1004263
32. Bastami M, Ghaderian SM, Omrani MD, Mirfakhraie R, Vakili H, Parsa SA, et al. MiRNA-related polymorphisms in MiR-146a and TCF21 are associated with increased susceptibility to coronary artery disease in an Iranian population. Genet Test Mol Biomark. (2016) 20:241–8. doi: 10.1089/gtmb.2015.0253
33. Richardson K, Nettleton JA, Rotllan N, Tanaka T, Smith CE, Lai CQ, et al. Gain-of-function lipoprotein lipase variant rs13702 modulates lipid traits through disruption of a microRNA-410 seed site. Am J Hum Genet. (2013) 92:5–14. doi: 10.1016/j.ajhg.2012.10.020
34. Bastami M, Nariman-Saleh-Fam Z, Saadatian Z, Nariman-Saleh-Fam L, Omrani MD, Ghaderian SMH, et al. The miRNA targetome of coronary artery disease is perturbed by functional polymorphisms identified and prioritized by in-depth bioinformatics analyses exploiting genome-wide association studies. Gene (2016) 594:74–81. doi: 10.1016/j.gene.2016.08.054
35. Huan T, Rong J, Liu C, Zhang X, Tanriverdi K, Joehanes R, et al. Genome-wide identification of microRNA expression quantitative trait loci. Nat Commun. (2015) 6:6601. doi: 10.1038/ncomms7601
36. Civelek M, Hagopian R, Pan C, Che N, Yang WP, Kayne PS, et al. Genetic regulation of human adipose microrna expression and its consequences for metabolic traits. Hum Mol Genet. (2013) 22:3023–37. doi: 10.1093/hmg/ddt159
37. Ballantyne RL, Zhang X, Nu-ez S, Xue C, Zhao W, Reed E, et al. Genome-wide interrogation reveals hundreds of long intergenic noncoding RNAs that associate with cardiometabolic traits. Hum Mol Genet. (2016) 25:3125–41. doi: 10.1093/hmg/ddw154
38. McPherson R, Pertsemlidis A, Kavaslar N, Stewart A, Roberts R, Cox DR, et al. A common allele on chromosome 9 associated with coronary heart disease. Science (2007) 316:1488–91. doi: 10.1126/science.1142447
39. Putku M, Kals M, Inno R, Kasela S, Org R, KoŽich V, et al. CDH13 promoter SNPs with pleiotropic effect on cardiometabolic parameters represent methylation QTLs. Hum Genet. (2015) 134:291–303. doi: 10.1007/s00439-014-1521-6
40. Chen G, Yao C, Hwang SJ, Liu C, Song C, Huan T, et al. Abstract 18806: integrated proteomic analysis of cardiovascular disease reveals novel protein quantitative trait loci. Circulation (2016).
41. Hartiala JA, Tang WH, Wang Z, Crow AL, Stewart AF, Roberts R, et al. Genome-wide association study and targeted metabolomics identifies sex-specific association of CPS1 with coronary artery disease. Nat Commun. (2016) 7:10558. doi: 10.1038/ncomms10558
42. Kraus WE, Muoio DM, Stevens R, Craig D, Bain JR, Grass E, et al. Metabolomic quantitative trait loci (mQTL) mapping implicates the ubiquitin proteasome system in cardiovascular disease pathogenesis. PLoS Genet. (2015) 11:e1005553. doi: 10.1371/journal.pgen.1005553
43. Laurila PP, Surakka I, Sarin AP, Yetukuri L, Hyötyläinen T, Söderlund S, et al. Genomic transcriptomic, and lipidomic profiling highlights the role of inflammation in individuals with low high-density lipoprotein cholesterolsignificance. Arteriosc Thromb Vasc Biol. (2013) 33:847–57. doi: 10.1161/ATVBAHA.112.300733
44. Zhu Y, Gu X, Xu C. A mitochondrial DNA A8701G mutation associated with maternally inherited hypertension and dilated cardiomyopathy in a Chinese pedigree of a consanguineous marriage. Chin Med J. (2016) 129:259–66. doi: 10.4103/0366-6999.174491
45. Kofler B, Mueller EE, Eder W, Stanger O, Maier R, Weger M, et al. Mitochondrial DNA haplogroup T is associated with coronary artery disease and diabetic retinopathy: a case control study. BMC Med Genet. (2009) 10:35. doi: 10.1186/1471-2350-10-35
46. Mueller EE, Eder W, Ebner S, Schwaiger E, Santic D, Kreindl T, et al. The mitochondrial T16189C polymorphism is associated with coronary artery disease in Middle European populations. PLoS ONE (2011) 6:e16455. doi: 10.1371/journal.pone.0016455
47. Jia Z, Wang X, Qin Y, Xue L, Jiang P, Meng Y, et al. Coronary heart disease is associated with a mutation in mitochondrial tRNA. Hum Mol Genet. (2013) 22:4064–73. doi: 10.1093/hmg/ddt256
48. Kumar V, Westra HJ, Karjalainen J, Zhernakova DV, Esko T, Hrdlickova B, et al. Human disease-associated genetic variation impacts large intergenic non-coding RNA expression. PLoS Genet. (2013) 9:e1003201. doi: 10.1371/journal.pgen.1003201
49. Grubert F, Zaugg JB, Kasowski M, Ursu O, Spacek DV, Martin AR, et al. Genetic control of chromatin states in humans involves local and distal chromosomal interactions. Cell (2015) 162:1051–65. doi: 10.1016/j.cell.2015.07.048
50. Garnier S, Truong V, Brocheton J, Zeller T, Rovital M, Wild PS, et al. Genome-wide haplotype analysis of cis expression quantitative trait loci in monocytes. PLoS Genet. (2013) 9:e1003240. doi: 10.1371/journal.pgen.1003240
51. Rotival M, Zeller T, Wild PS, Maouche S, Szymczak S, Schillert A, et al. Integrating genome-wide genetic variations and monocyte expression data reveals trans-regulated gene modules in humans. PLoS Genet. (2011) 7:e1002367. doi: 10.1371/journal.pgen.1002367
52. Lempiäinen H, Brænne I, Michoel T, Tragante V, Vilne B, Webb TR. Network analysis of coronary artery disease risk genes elucidates disease mechanisms and druggable targets. Sci Rep. (2018) 8:3434. doi: 10.1038/s41598-018-20721-6
53. Hägg S, Skogsberg J, Lundström J, Noori P, Nilsson R, Zhong H, et al. Multi-organ expression profiling uncovers a gene module in coronary artery disease involving transendothelial migration of leukocytes and lim domain binding 2: the Stockholm Atherosclerosis Gene Expression (STAGE) Study. PLoS Genet (2009) 5:e1000754. doi: 10.1371/journal.pgen.1000754
54. Talukdar HA, Foroughi Asl H, Jain RK, Ermel R, Ruusalepp A, Franzén O, et al. Cross-tissue regulatory gene networks in coronary artery disease. Cell Syst. (2016) 2:196–208. doi: 10.1016/j.cels.2016.02.002
55. Small EM, Frost RJ, Olson EN. MicroRNAs add a new dimension to cardiovascular disease. Circulation (2010) 121:1022–32. doi: 10.1161/CIRCULATIONAHA.109.889048
56. Economou EK, Oikonomou E, Siasos G, Papageorgiou N, Tsalamandris S, Mourouzis K, et al. The role of microRNAs in coronary artery disease: from pathophysiology to diagnosis and treatment. Atherosclerosis (2015) 241:624–33. doi: 10.1016/j.atherosclerosis.2015.06.037
57. Archer K, Broskova Z, Bayoumi AS, Teoh JP, Davila A, Tang Y, et al. Long non-coding RNAs as master regulators in cardiovascular diseases. Int J Mol Sci. (2015) 16:23651–67. doi: 10.3390/ijms161023651
58. El Azzouzi H, Doevendans PA, Sluijter JP. Long non-coding RNAs in heart failure: an obvious lnc. Ann Trans Med. (2016) 4:182. doi: 10.21037/atm.2016.05.06
59. Madrigal-Matute J, Rotllan N, Aranda JF, Fernández-Hernando C. MicroRNAs and atherosclerosis. Curr Atherosc Rep. (2013) 15:322. doi: 10.1007/s11883-013-0322-z
60. Malik R, Mushtaque RS, Siddiqui UA, Younus A, Aziz MA, Humayun C, et al. Association between coronary artery disease and microRNA: literature review and clinical perspective. Cureus (2017) 23:e1188. doi: 10.7759/cureus.1188
61. Bulik-Sullivan B, Selitsky S, Sethupathy P. Prioritization of genetic variants in the microRNA regulome as functional candidates in genome-wide association studies. Hum Mutat. (2013) 34:1049–56. doi: 10.1002/humu.22337
62. Ghaedi H, Bastami M, Jahani MM, Alipoor B, Tabasinezhad M, Ghaderi O, et al. A bioinformatics approach to the identification of variants associated with Type 1 and Type 2 diabetes mellitus that reside in functionally validated miRNAs binding sites. Biochem Genet. (2016) 54:211–21. doi: 10.1007/s10528-016-9713-5
63. Cloonan N, Wani S, Xu Q, Gu J, Lea K, Heater S, et al. MicroRNAs and their isomiRs function cooperatively to target common biological pathways. Genome Biol. (2011) 12:R126. doi: 10.1186/gb-2011-12-12-r126
64. Li SC, Liao YL, Ho MR, Tsai KW, Lai CH, Lin WC. Mi-RNA arm selection and isomiR distribution in gastric cancer. BMC Genomics (2012) 13:S13. doi: 10.1186/1471-2164-13-S1-S13
65. Meijer HA, Smith EM, Bushell M. Regulation of miRNA strand selection: follow the leader? Biochem Soc Trans. (2014) 42:1135–40. doi: 10.1042/BST20140142
66. Samani NJ, Erdmann J, Hall AS, Hengstenberg C, Mangino M, Mayer B, et al. Genomewide association analysis of coronary artery disease. N Eng J Med. (2007) 357:443–53. doi: 10.1056/NEJMoa072366
67. Schunkert H, Götz A, Braund P, McGinnis R, Tregouet DA, Mangino M, et al. Repeated replication and a prospective meta-analysis of the association between chromosome 9p21.3 and coronary artery disease. Circulation (2008) 117:1675–84. doi: 10.1161/CIRCULATIONAHA.107.730614
68. Holdt LM, Beutner F, Scholz M, Gielen S, Gäbel G, Bergert H, et al. ANRIL expression is associated with atherosclerosis risk at chromosome 9p21. Arteriosc Thromb Vasc Biol. (2010) 30:620–7. doi: 10.1161/ATVBAHA.109.196832
69. Holdt LM, Stahringer A, Sass K, Pichler G, Kulak NA, Wilfert W, et al. Circular non-coding RNA ANRIL modulates ribosomal RNA maturation and atherosclerosis in humans. Nat Commun. (2016) 7:12429. doi: 10.1038/ncomms12429
70. Langley SR, Dwyer J, Drozdov I, Yin X, Mayr M. Proteomics: from single molecules to biological pathways. Cardiovasc Res. (2012) 97:612–22. doi: 10.1093/cvr/cvs346
71. Dupont A, Pinet F. The proteome and secretome of human arterial smooth muscle cell. Cardiovasc Proteom. (2007) 357:225–33. doi: 10.1385/1-59745-214-9:225
72. Burkhart JM, Vaudel M, Gambaryan S, Radau S, Walter U, Martens L, et al. The first comprehensive and quantitative analysis of human platelet protein composition allows the comparative analysis of structural and functional pathways. Blood (2012) 120:73–82. doi: 10.1182/blood-2012-04-416594
73. Neisius U, Koeck T, Mischak H, Rossi SH, Olson E, Carty DM, et al. Urine proteomics in the diagnosis of stable angina. BMC Cardiovasc Disord. (2016) 16:70. doi: 10.1186/s12872-016-0246-y
74. Evers BM, Rodriguez-Navas C, Tesla RJ, Prange-Kiel J, Wasser CR, Yoo KS, et al. Lipidomic and transcriptomic basis of lysosomal dysfunction in progranulin deficiency. Cell Rep. (2017) 20:2565–74. doi: 10.1016/j.celrep.2017.08.056
75. Krumsiek J, Bartel J, Theis FJ. Computational approaches for systems metabolomics. Curr Opin Biotechnol. (2016) 39:198–206. doi: 10.1016/j.copbio.2016.04.009
76. Teupser D, Baber R, Ceglarek U, Scholz M, Illig T, Gieger C, et al. Genetic regulation of serum phytosterol levels and risk of coronary artery diseaseclinical perspective. Circulation (2010) 3:331–9. doi: 10.1161/CIRCGENETICS.109.907873
77. Shah SH, Sun JL, Stevens RD, Bain JR, Muehlbauer MJ, Pieper KS, et al. Baseline metabolomic profiles predict cardiovascular events in patients at risk for coronary artery disease. Am Heart J. (2012) 163:844–50. doi: 10.1016/j.ahj.2012.02.005
78. Krishnan S, Huang J, Lee H, Guerrero A, Berglund L, Anuurad E, et al. Combined high-density lipoprotein proteomic and glycomic profiles in patients at risk for coronary artery disease. J Proteome Res. (2015) 14:5109–18. doi: 10.1021/acs.jproteome.5b00730
79. Feng Q, Liu Z, Zhong S, Li R, Xia H, Jie Z, et al. Integrated metabolomics and metagenomics analysis of plasma and urine identified microbial metabolites associated with coronary heart disease. Sci Rep. (2016) 6:22525. doi: 10.1038/srep22525
80. Trainor PJ, Hill BG, Carlisle SM, Rouchka EC, Rai SN, Bhatnagar A, et al. Systems characterization of differential plasma metabolome perturbations following thrombotic and non-thrombotic myocardial infarction. J Proteom. (2017) 160:38–46. doi: 10.1016/j.jprot.2017.03.014
81. Org E, Blum Y, Kasela S, Mehrabian M, Kuusisto J, Kangas AJ, et al. Relationships between gut microbiota, plasma metabolites, and metabolic syndrome traits in the METSIM cohort. Genome Biol. (2017) 18:70. doi: 10.1186/s13059-017-1194-2
82. Ranjan R, Rani A, Metwally A, McGee HS, Perkins DL. Analysis of the microbiome: advantages of whole genome shotgun versus 16S amplicon sequencing. Biochem Biophys Res Commun. (2016) 469:967–77. doi: 10.1016/j.bbrc.2015.12.083
83. Caesar R, Fåk F, Bäckhed F. Effects of gut microbiota on obesity and atherosclerosis via modulation of inflammation and lipid metabolism. J Int Med. (2010) 268:320–8. doi: 10.1111/j.1365-2796.2010.02270.x
84. Le Chatelier E, Nielsen T, Qin J, Prifti E, Hildebrand F, Falony G, et al. Richness of human gut microbiome correlates with metabolic markers. Nature (2013) 500:541–6. doi: 10.1038/nature12506
85. Emoto T, Yamashita T, Kobayashi T, Sasaki N, Hirota Y, Hayashi T, et al. Characterization of gut microbiota profiles in coronary artery disease patients using data mining analysis of terminal restriction fragment length polymorphism: gut microbiota could be a diagnostic marker of coronary artery disease. Heart Vessels (2016) 32:39–46. doi: 10.1007/s00380-016-0841-y
86. Senthong V, Li XS, Hudec T, Coughlin J, Wu Y, Levison B, et al. Plasma trimethylamine N-Oxide, a gut microbe–generated phosphatidylcholine metabolite, is associated with atherosclerotic burden. J Am Coll Cardiol. (2016) 67:2620–8. doi: 10.1016/j.jacc.2016.03.546
87. Fu J, Bonder MJ, Cenit MC, Tigchelaar EF, Maatman A, Dekens JA, et al. The gut microbiome contributes to a substantial proportion of the variation in blood lipids. Circ Res. (2015) 117:817–24. doi: 10.1161/CIRCRESAHA.115.306807
88. Blekhman R, Goodrich JK, Huang K, Sun Q, Bukowski R, Bell JT, et al. Host genetic variation impacts microbiome composition across human body sites. Genome Biol. (2015) 16:191. doi: 10.1186/s13059-015-0759-1
89. Zhu J, Sova P, Xu Q, Dombek KM, Xu EY, Vu H, et al. Stitching together multiple data dimensions reveals interacting metabolomic and transcriptomic networks that modulate cell regulation. PLoS Biol. (2012) 10:e1001301. doi: 10.1371/journal.pbio.1001301
90. Miller CL, Pjanic M, Wang T, Nguyen T, Cohain A, Lee JD, et al. Integrative functional genomics identifies regulatory mechanisms at coronary artery disease loci. Nat Commun. (2016) 7:12092. doi: 10.1038/ncomms12092
91. Ballinger SW. Mitochondrial dysfunction in cardiovascular disease. Free Radic Biol Med. (2005) 38:1278–95. doi: 10.1016/j.freeradbiomed.2005.02.014
92. Kazuno AA, Munakata K, Nagai T, Shimozono S, Tanaka M, Yoneda M, et al. Identification of mitochondrial DNA polymorphisms that alter mitochondrial matrix pH and intracellular calcium dynamics. PLoS Genet. (2006) 2:e128. doi: 10.1371/journal.pgen.0020128
93. Baccarelli AA, Byun HM. Platelet mitochondrial dna methylation: a potential new marker of cardiovascular disease. Clin Epigenet. (2015) 7:44. doi: 10.1186/s13148-015-0078-0
94. Skogsberg J, Lundström J, Kovacs A, Nilsson R, Noori P, Maleki S, et al. Transcriptional profiling uncovers a network of cholesterol-responsive atherosclerosis target genes. PLoS Genet (2008) 4:e1000036. doi: 10.1371/journal.pgen.1000036
95. Björkegren JL, Hägg S, Talukdar HA, Foroughi Asl H, Jain RK, Cedergren C, et al. Plasma cholesterol-induced lesion networks activated before regression of early, mature, and advanced atherosclerosis. PLoS Genet (2014) 10:e1004201. doi: 10.1371/journal.pgen.1004201
96. Vilne B, Skogsberg J, Foroughi Asl H, Talukdar HA, Kessler T, Björkegren JLM, et al. Network analysis reveals a causal role of mitochondrial gene activity in atherosclerotic lesion formation. Atherosclerosis (2017) 267:39–48. doi: 10.1016/j.atherosclerosis.2017.10.019
97. Franco-Obregón A, Gilbert JA. The microbiome-mitochondrion connection: common ancestries, common mechanisms, common goals. mSystems (2017) 2:e00018–17. doi: 10.1128/mSystems.00018-17
98. Smith DR. The past present and future of mitochondrial genomics: have we sequenced enough mtDNAs? Brief Func Genom. (2016) 15:47–54. doi: 10.1093/bfgp/elv027
99. Stefely JA, Kwiecien NW, Freiberger EC, Richards AL, Jochem A, Rush MJP, et al. Mitochondrial protein functions elucidated by multi-omic mass spectrometry profiling. Nat Biotechnol. (2016) 34:1191–7. doi: 10.1038/nbt.3683
100. Picard M, McEwen BS, Epel ES, Sandi C. An energetic view of stress: focus on mitochondria. Front Neuroendocrinol. (2018) 49:72–85. doi: 10.1016/j.yfrne.2018.01.001
Keywords: cardiovascular disease, multiomics, genomics, transcriptomics, metabolomics, gut microbiome
Citation: Vilne B and Schunkert H (2018) Integrating Genes Affecting Coronary Artery Disease in Functional Networks by Multi-OMICs Approach. Front. Cardiovasc. Med. 5:89. doi: 10.3389/fcvm.2018.00089
Received: 25 March 2018; Accepted: 22 June 2018;
Published: 17 July 2018.
Edited by:
Jeanette Erdmann, Universität zu Lübeck, GermanyReviewed by:
Krishna Aragam, Massachusetts General Hospital, Harvard Medical School, United StatesFrancesco Danilo Tiziano, Università Cattolica del Sacro Cuore, Italy
Copyright © 2018 Vilne and Schunkert. This is an open-access article distributed under the terms of the Creative Commons Attribution License (CC BY). The use, distribution or reproduction in other forums is permitted, provided the original author(s) and the copyright owner(s) are credited and that the original publication in this journal is cited, in accordance with accepted academic practice. No use, distribution or reproduction is permitted which does not comply with these terms.
*Correspondence: Heribert Schunkert, c2NodW5rZXJ0QGRobS5taG4uZGU=