- 1National Heart Centre Singapore, Singapore, Singapore
- 2Duke-NUS Medical School, Singapore, Singapore
- 3Department of Cardiology, National University Heart Centre, Singapore, Singapore
- 4Yong Loo Lin School of Medicine, National University of Singapore, Singapore, Singapore
- 5Department of Diagnostic Imaging, National University Hospital, Singapore, Singapore
- 6Institute for Infocomm Research, Agency for Science, Technology and Research, Singapore, Singapore
- 7California Medical Innovations Institute, San Diego, CA, United States
The aim of this study was to evaluate a new analytical method for calculating non-invasive fractional flow reserve (FFRAM) to diagnose ischemic coronary lesions. Patients with suspected or known coronary artery disease (CAD) who underwent computed tomography coronary angiography (CTCA) and invasive coronary angiography (ICA) with FFR measurements from two sites were prospectively recruited. Obstructive CAD was defined as diameter stenosis (DS) ≥50% on CTCA or ICA. FFRAM was derived from CTCA images and anatomical features using analytical method and was compared with computational fluid dynamics (CFD)-based FFR (FFRB) and invasive ICA-based FFR. FFRAM, FFRB, and invasive FFR ≤ 0.80 defined ischemia. A total of 108 participants (mean age 60, range: 30–83 years, 75% men) with 169 stenosed coronary arteries were analyzed. The per-vessel accuracy, sensitivity, specificity, and positive predictive and negative predictive values were, respectively, 81, 75, 86, 81, and 82% for FFRAM and 87, 88, 86, 83, and 90% for FFRB. The area under the receiver operating characteristics curve for FFRAM (0.89 and 0.87) and FFRB (0.90 and 0.86) were higher than both CTCA- and ICA-derived DS (all p < 0.0001) on per-vessel and per-patient bases for discriminating ischemic lesions. The computational time for FFRAM was much shorter than FFRB (2.2 ± 0.9 min vs. 48 ± 36 min, excluding image acquisition and segmentation). FFRAM calculated from a novel and expeditious non-CFD approach possesses a comparable diagnostic performance to CFD-derived FFRB, with a significantly shorter computational time.
Introduction
Atherosclerotic plaque deposition in the coronary arterial wall results in anatomical stenosis that may reduce perfusion and induce ischemia in the subtended myocardial territory (1). Fractional flow reserve (FFR), measured during invasive coronary angiography (ICA), is the reference standard for quantifying the functional significance of coronary artery stenoses and discriminating ischemic lesions (2, 3). However, ICA-based FFR measurement incurs additional resource utilization, increases procedural time, and is associated with greater patient discomfort (4). Recently, non-invasive FFR (FFRCT) derived from computed tomography coronary angiography (CTCA) images and computational fluid dynamics (CFD) has demonstrated feasibility for the identification of ischemic coronary lesions (5) with reasonable diagnostic accuracy (6) and prognostication (7).
Mesh generation and iterative solution of numerical equations integral to CFD demand long computational time for the calculation of time-varying instantaneous values of coronary blood flow parameters like velocity, pressure, etc. The current CFD-based FFRCT methods take 1 to 4 h per FFRCT analysis (8). Reduced-order (9–11), steady-flow (12) CFD simulations and predictive models using machine learning (13–15) may improve computational efficiency and facilitate shorter turnaround times and/or on-site analysis, which will help garner a wider adoption of non-invasive FFR.
Still an analytical method to calculate FFR non-invasively without the need for computationally demanding CFD modeling would further simplify the derivation of non-invasive FFR from CTCA images. Huo et al. (16) proposed an analytical model that embodied integral equations to be solved based on the dimensions of anatomical stenosis on CTCA and estimates of hyperemic coronary flow derived from in vitro and in vivo animal experiments. In this study, we developed an original analytical method, FFRAM, that relies on neither CFD nor other inputs other than CTCA images. Flow rate through coronary lesions (QAM) was estimated from anatomical data reconstructed from CTCA, where anatomical features known to influence the hemodynamics in stenotic arteries, including lesion length, lumen area, flow entrance, and exit angles (17), were explicitly considered. Our aim is to assess the diagnostic performance of FFRAM with reference to our previously developed CFD-based FFRB and invasive FFR in a cohort of coronary artery disease (CAD) patients.
Materials and Methods
Study Design and Study Population
The current study consecutively enrolled patients from two tertiary centers, with age ≥ 21 years, who had undergone CTCA, and were scheduled to undergo clinically indicated ICA and FFR measurement. The time difference between CTCA and ICA was 32 (19–51) days (median, interquartile range). The exclusion criteria included prior coronary revascularization, acute coronary syndrome occurring between 30 days before CTCA and ICA, angina at rest, left ventricular ejection fraction <30%, hypertrophic cardiomyopathy, significant valve disease including prosthetic heart valve, implanted pacemaker or defibrillator, complex congenital heart disease, estimated glomerular filtration rate <30 ml/min/1.73 m2, tachycardia or significant arrhythmia, iodinated contrast allergy, contraindication to beta-blocker, nitroglycerin, or adenosine, serious comorbidity with life expectancy <2 years, and pregnancy. The study was approved by the local institutional review boards, and all participants gave written informed consent.
From September 20, 2016 to March 25, 2020, 117 participants were recruited. Nine subjects were excluded: two patients with unsuccessful invasive FFR measurement and seven patients with inadequate CTCA image quality. Among the seven patients, one patient had blooming artifacts due to extreme coronary calcification (Agatston score 3441), and six patients had motion artifacts in the CTCA images. By excluding 10 vessels with missing video recordings of the FFR measurement locations, 108 participants with 169 vessels were included in the analysis (Figure 1).
ICA and FFR Measurement
For the recruited patients, invasive FFR measurement was performed according to the institutional protocol. Every participant underwent ICA via either the femoral or radial approach using 5F, 6F, or 7F diagnostic or guiding catheters (18). Angiography was performed in standard projections. Diameter stenosis at ICA (DSICA) was visually assessed (19), and lesions were deemed obstructive if DSICA ≥50%. The pressure wires/catheters used for the invasive FFR can be found in the Supplementary Material. Intra-coronary pressure was measured at the ascending aorta and distal to the coronary lesion in at least one vessel. Hyperemia was induced by either intravenous infusion (140–180 μg/kg/min) or an intracoronary bolus (60–200 μg) of adenosine. A coronary lesion was categorized as ischemic if FFR ≤ 0.80. Two consultant interventional cardiologists with extensive clinical experience reviewed the ICA images, and the lesions were evaluated based on overall consensus. In case of disagreement, a third independent cardiologist reviewed the films and provided a final diagnosis.
CTCA Acquisition
Every participant underwent CTCA on one of the following scanners with ≥256 detector rows: Toshiba Aquilion One 320 Slice, Canon Aquilion ONE Genesis 640 Slice, Philips Brilliance iCT 256-detector, Siemens Somatom Force dual source 384-detector, GE Revolution single source, and Siemens Somatom Drive dual source 256-detector. Oral beta-blocker (metoprolol) was administered to the participants with a heart rate >65 beats per min (20). Sublingual glyceryl trinitrate was administered just prior to scanning for optimal coronary vasodilation during image acquisition. Prospective electrocardiogram-triggered protocol was used to acquire image data at pre-specified phases of the heart cycle, and CTCA scan was performed at inspiratory breath-hold. Then, 50 to 75 ml of non-ionic contrast Omnipaque 350 was administered for each scan.
The CTCA studies were read by an accredited reporting radiologist or cardiologist and verified by a second accredited reader. The diameter stenoses of coronary lesions on CTCA images (DSCTCA) were graded according to anatomical severity: normal, absent plaque, and no luminal stenosis; minimal, DSCTCA <25%; mild, 25% ≤ DSCTCA ≤ 49%; moderate, 50% ≤ DSCTCA ≤ 69%; severe, 70% ≤ DSCTCA ≤ 99%; and occluded, DSCTCA = 100% (20). A coronary lesion was deemed obstructive if DSCTCA ≥50%.
CTCA Image Segmentation and 3D Model Reconstruction
Dedicated QAngio CT software (21) (version 3.0, Medis) was used for segmentation and 3D reconstruction of coronary artery. Additional details are found in the Supplementary Material. The surface meshes of the 3D coronary artery tree model were generated using 3D Workbench (version 0.8, Medis). Figure 2 illustrates the workflow for non-invasive FFR calculation in a participant. Figure 3 depicts the detailed coronary anatomy in another participant with pertinent anatomical parameter inputs for calculating the FFRAM.
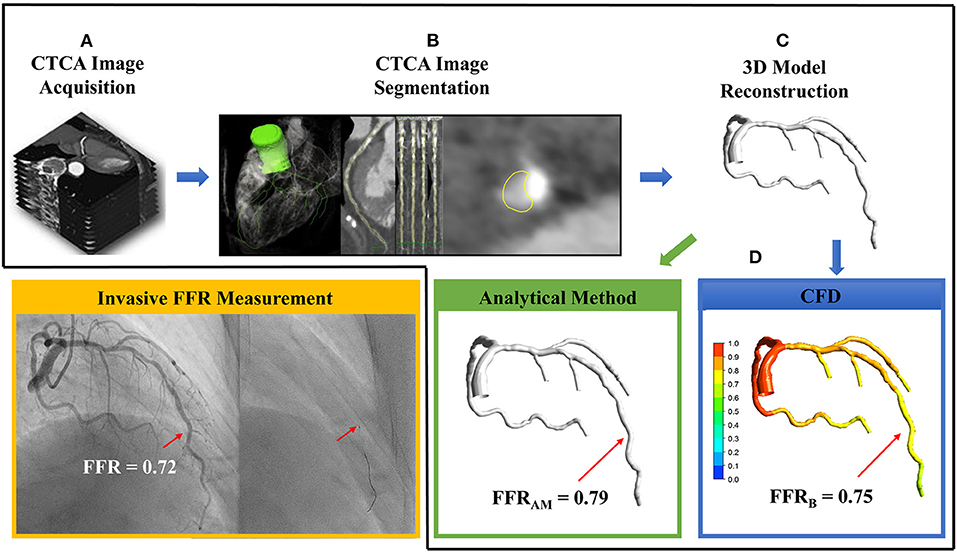
Figure 2. The sequential steps involved in the calculation of non-invasive fractional flow reserve (FFR) include (A) computed tomography coronary angiography (CTCA) image acquisition, (B) CTCA image segmentation via extracting centerlines and delineating lumen contours in the transversal and cross-sectional images, (C) 3D reconstruction of subject-specific coronary artery tree, and (D) using either analytical method to calculate FFRAM (green box) or computational fluid dynamics simulation to compute FFRB (blue box). In this case, FFRAM and FFRB were 0.79 and 0.75, respectively, at the site of invasive FFR 0.72 measured with a pressure catheter (right, inset) during invasive coronary angiography (yellow box).
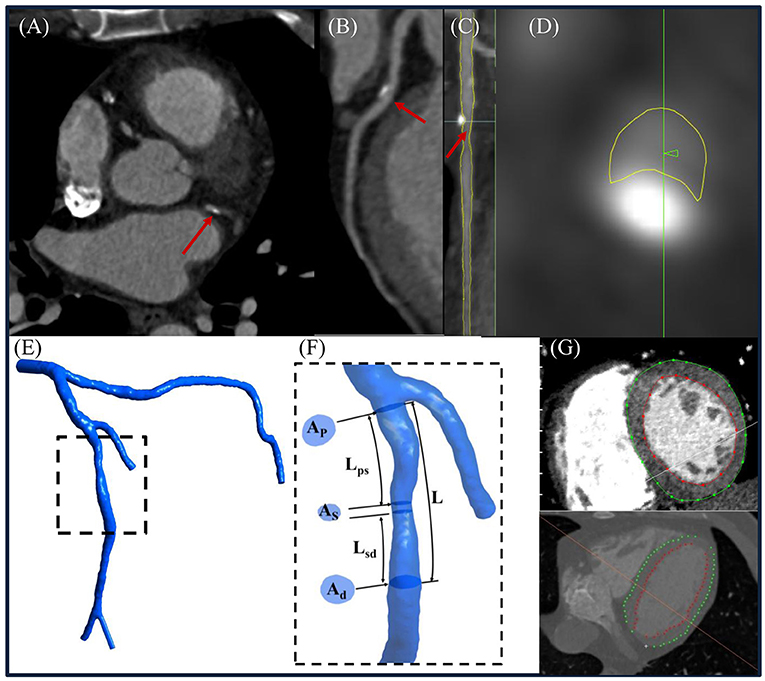
Figure 3. (A) Representative computed tomography coronary angiography (CTCA) cross-sectional slice, (B) curved multiplanar reconstructed images of a left anterior descending (LAD) coronary artery, (C) straightened LAD with segmented lumen lines (yellow color) in transversal and (D) cross-sectional views, (E) reconstructed 3D left coronary tree, and (F) enlarged view to illustrate how to measure Ap, As , Ad, Lps, Lsd, and L from the model. (G) Left ventricular (LV) endocardial and epicardial contours were delineated from CTCA images to calculate the LV mass. (Note: Ap, As, and Ad represented the lumen area at proximal, maximally stenosed, and distal segments, respectively; Lps, Lsd, and L were the length measured from the proximal end of the coronary lesion to the proximal end of the maximally stenosed segment, the distal end of the maximally stenosed segment to the distal end of the coronary lesion, and the proximal to the distal ends of the whole coronary lesion, respectively. Flow entrance and exit angles (α and β) were then calculated using Eqs. (A-1) and (A-6) in the Supplementary Material, respectively.
Total coronary flow under resting conditions, a required input parameter for non-invasive FFR estimation, is linearly related to left ventricular mass (LVM) (22). The latter was measured using validated Segment CT software (version 2.2, Medviso) (23) that semi-automatically delineated left ventricular (LV) endocardial and epicardial contours on contiguous 2D LV short-axis slices reformatted from the CTCA-reconstructed 3D whole-heart model (Figure 3).
Computation of Non-invasive FFRAM With Analytical Model
In our analytical model, , where Pa is patient-specific mean aortic pressure estimated as mean cuff pressure minus 6.8 mmHg to account for pressure drop during hyperemia (24), and ΔP1 and ΔP2 are pressure drops across the coronary lesion and from the coronary orifice to the proximal end of the coronary lesion, respectively. The latter is calculated from the Hagen–Poiseuille equation according to the viscosity of the blood, lumen area, length, and flow rate of each coronary branch (from the coronary orifice to the proximal end of the coronary lesion), respectively.
By law of energy conservation, ΔP1 entails convective and diffusive energy losses as well as energy loss attributable to sudden constriction and expansion (16). Flow separation and swirling that exacerbate energy losses and pressure drops are related to features such as lesion length, lumen area, flow entrance, exit angles, etc. (25). We applied these considerations in series to a coronary lesion model of total length L decomposed schematically into three components: a proximal contracting segment of length Lps and distal expanding segment of length Lsd, which bookend a middle maximally stenosed segment of finite length L–Lps-Lsd (Supplementary Figure 1). The respective pressure drops across the three segments ΔPps, ΔPsd, and ΔPss sum up to ΔP1 and are, from a mechanical engineering perspective, analogous to pressure drops across contracting, expanding, and straight pipes, respectively (Supplementary Material). Figure 3F illustrates how we measured the anatomical parameters L, Lps, and Lsd as well as AP, Ad, and As, the lumen areas at the proximal and distal ends of the coronary lesion, and the maximally stenosed segment, respectively. From these parameters, flow entrance (α) and exit (β) angles were derived to facilitate the calculation of ΔPps and ΔPsd (Supplementary Material).
To calculate the hyperemic flow rate of each coronary branch, we first calculated the total coronary flow rate at resting from CTCA-assessed LVM (22) and then estimated the resting flow rate through the i-th coronary branch using the scaling law (26). Finally, hyperemic flow rate through a coronary lesion located at the i-th branch of the coronary artery tree was computed as k times of its value at resting state. (24). The coefficient k reflects the magnitude of flow increase at hyperemia and is dependent on the diameter stenosis of the lesion (DS). Inputting QAM to the analytical model, ΔP1 and then FFRAM could be calculated without a need for CFD simulation (Supplementary Material).
Computation of Non-invasive FFRB Based on Reduced-Order CFD Simulation
Reduced-order CFD simulation was performed on the reconstructed 3D coronary artery tree model in deriving non-invasive FFRB measurement. Additional details can be found in our prior studies (9, 11, 27) and in the Supplementary Material. The FFRB value was extracted at the location on the 3D coronary tree model that best corresponded to the site of the FFR measurement at ICA as judged by cardiologists (JMF and CYC).
Statistical Analysis
Continuous variables were summarized as mean ± standard deviation (SD) or median (interquartile range), and the categorical variables were summarized as frequencies and percentages. Two-sample t-test, Wilcoxon rank-sum test, and Fisher's exact test were used to compare the ischemic and non-ischemic groups on continuous normally distributed variables, continuous parameters with non-normal distribution, and binary variables, respectively. For vessels with multiple lesions, the pressure drops over individual lesions were compared, and the anatomical parameters associated with the lesion contributing to the largest pressure drop were selected for statistical analysis. The DeLong test (28) was used to compare receiver operating characteristic (ROC) areas under the curve (AUCs). Accuracy, sensitivity, specificity, positive prediction value, negative predictive value (NPV), and likelihood ratios corresponding to the diagnostic threshold were calculated to enable a comparison of the discrimination capability among DSCTCA, DSICA, and non-invasive FFR indexes. SPSS (version 22, IBM, New York, USA) was used to perform the statistical analyses. Statistical significance was set at p <0.05.
Results
Patient Characteristics
Detailed demographics of the 108 participants (mean age 60 ± 9 years; 81 males) is presented in Table 1. Ethnicities included Chinese (80%), Indian/Malay (15%), and other Asians (5%) which closely reflect the ethnic percentages of the Singapore population. The majority of the participants had hypertension (64%) and hyperlipidemia (70%).
Characteristics of Flow Rate and Morphological Parameters
Among 169 vessels, 73 (43%) were ischemic (Table 2). As and Ad were significantly smaller, and α and β were significantly greater among ischemic vs. non-ischemic lesions, which contribute to the significantly greater ΔPps and ΔPsd along the contracting and expanding segments, respectively, in the ischemic lesions. There was excellent correlation between flow rates through lesions derived using empirical equations and CFD simulation (mean QAM 3.38 ± 1.93 ml/s vs. mean QCFD 3.30 ± 1.97 ml/s; r = 0.95, p < 0.0001) (Figure 4).
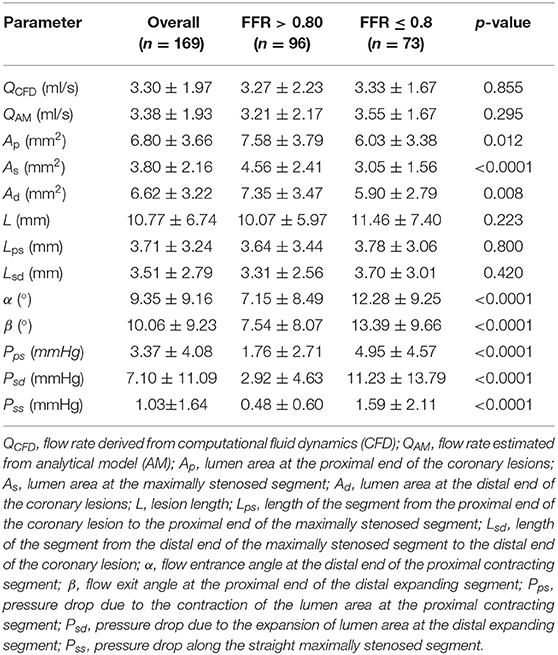
Table 2. Characteristics of flow rate, anatomical parameters, and pressure drop over various coronary lesion segments to calculate the non-invasive FFRAM overall and by study group (ischemic group: FFR ≤ 0.8; non-ischemic group: FFR > 0.8).
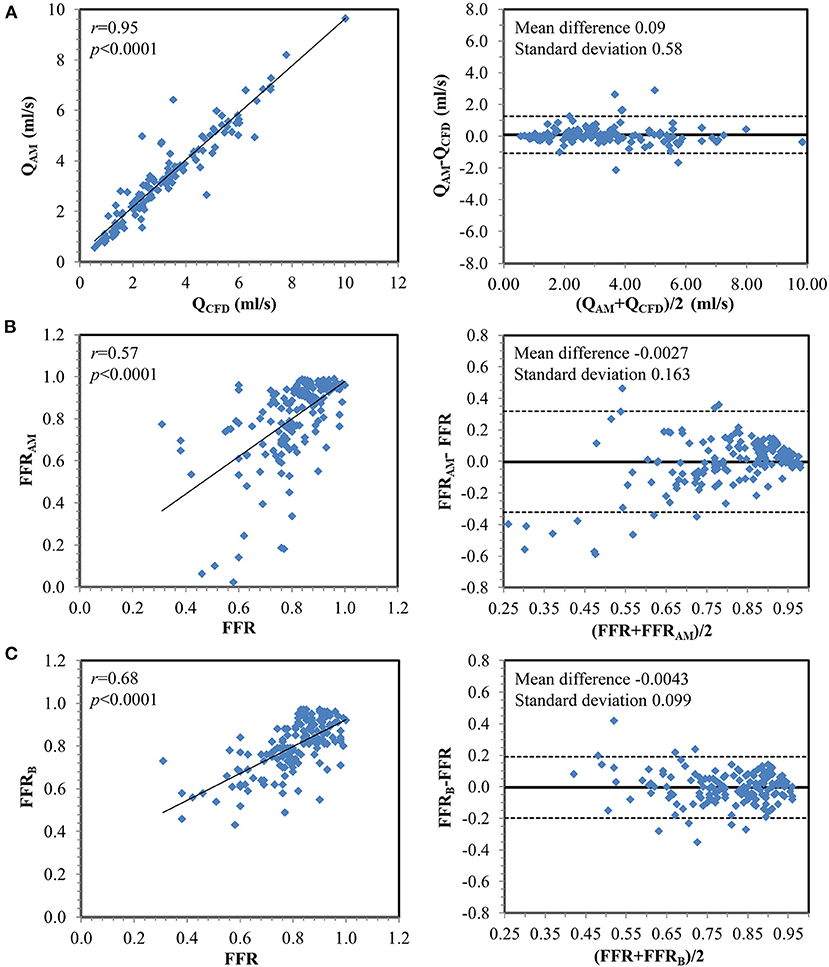
Figure 4. Correlation and Bland–Altman plots (A) between flow rates calculated from computational fluid dynamics simulation (QCFD) and estimated from the analytical model (QAM) and between invasively measured fractional flow reserve (FFR) with (B) FFRAM and (C) FFRB on a per-vessel basis.
Diagnostic Performance of FFRAM for Discriminating Ischemic Lesions
Compared with invasive FFR (mean 0.81 ± 0.13), FFRAM (mean 0.80 ± 0.20) exhibited fair correlation (r = 0.57, p < 0.0001) and agreement with small systematic biases (-0.0027 ± 0.163) (Figure 4). Performance metrics using DSCTCA ≥50%, DSICA ≥50%, FFRAM ≤ 0.8, and FFRB ≤ 0.8 to discriminate ischemic lesions are compared in Table 3 and Figure 5. On a per-vessel level, the ROC AUCs (95% CI) for FFRAM [0.89 (0.84, 0.94)] and FFRB [0.90 (0.85, 0.94)] were significantly higher than those for DSCTCA [0.61 (0.54, 0.69)] and DSICA [0.73 (0.65, 0.79)]. On a per-patient level, the ROC AUCs (95% CI) for FFRAM [0.87 (0.79, 0.93)] and FFRB [0.86 (0.78, 0.92)] were significantly higher than those for DSCTCA [0.52 (0.42, 0.62)] and DSICA [0.73 (0.64, 0.81)]. DSICA had a higher AUC than DSCTCA (both p <0.05 on per-vessel and per-patient analyses). There was no significant difference between FFRAM and FFRB in AUCs on both per-vessel and per-patient analyses (Figure 5).
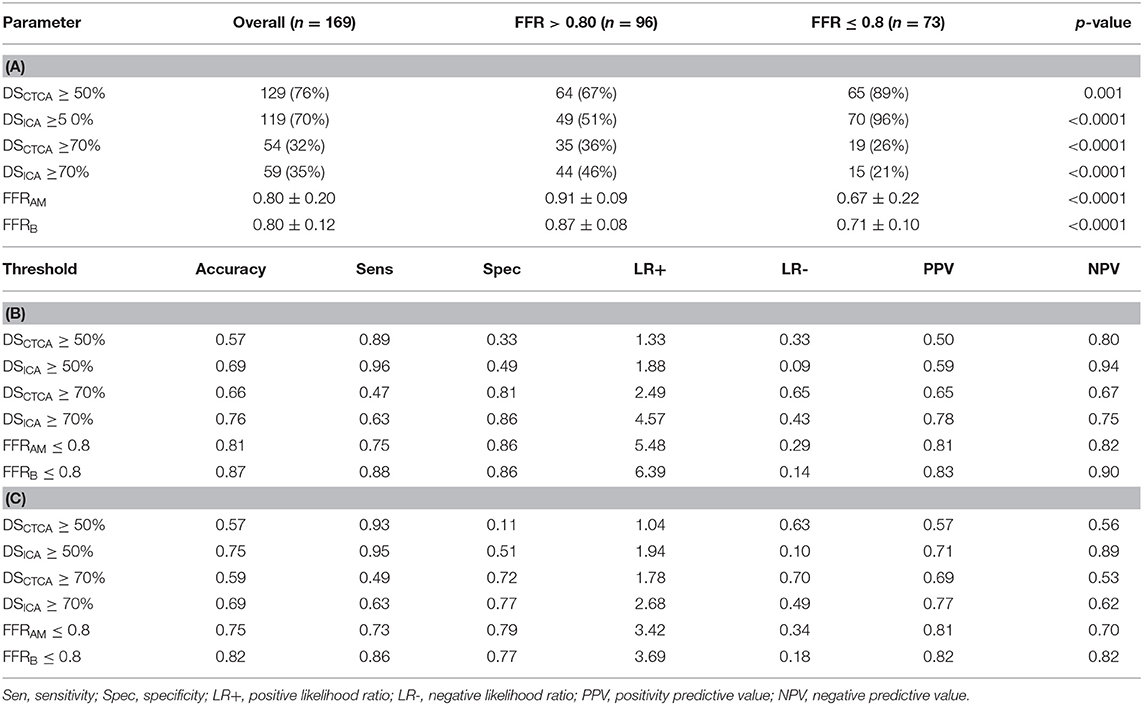
Table 3. (A) Diameter stenoses (DSCTCA and DSICA) and non-invasive FFR (FFRAM and FFRB) in study groups (FFR > 0.8 and FFR ≤ 0.8; (B) Comparison of diagnostic performance of different parameters for predicting myocardial ischemia at per-vessel level; (C) Comparison of diagnostic performance of different parameters for predicting myocardial ischemia at per-patient level.
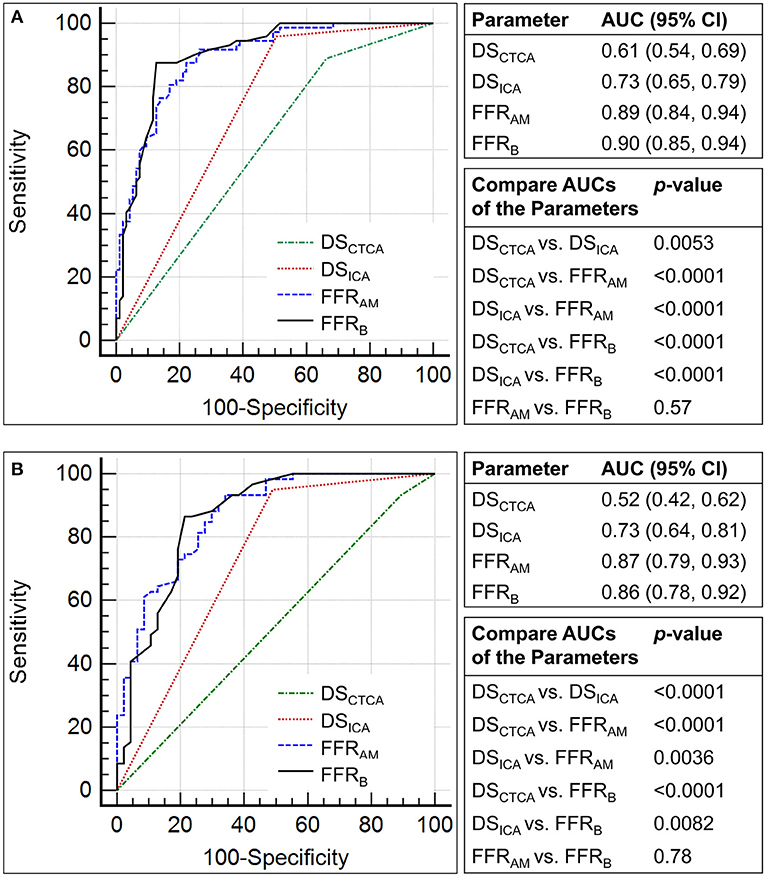
Figure 5. Comparison of the receiver operating characteristic curves for the discrimination of myocardial ischemia (fractional flow reserve, FFR ≤0.8) using diameter stenosis (DS; DSCTCA and DSICA, with a threshold of 50%) and non-invasive FFR (FFRAM and FFRB) on (A) per-vessel and (B) per-patient levels.
The performance metrics using DSCTCA ≥70%, DSICA ≥70%, FFRAM ≤ 0.8, and FFRB ≤ 0.8 to discriminate ischemic lesions are compared in Table 3 and Figure 6. On a per-vessel level, the ROC AUCs for FFRAM and FFRB were significantly higher than those for DSCTCA [0.64 (0.56, 0.71)] and DSICA [0.74 (0.67, 0.81)]. On a per-patient level, the ROC AUCs for FFRAM and FFRB were significantly higher than those for DSCTCA [0.61 (0.51, 0.70)] and DSICA [0.70 (0.60, 0.78)].
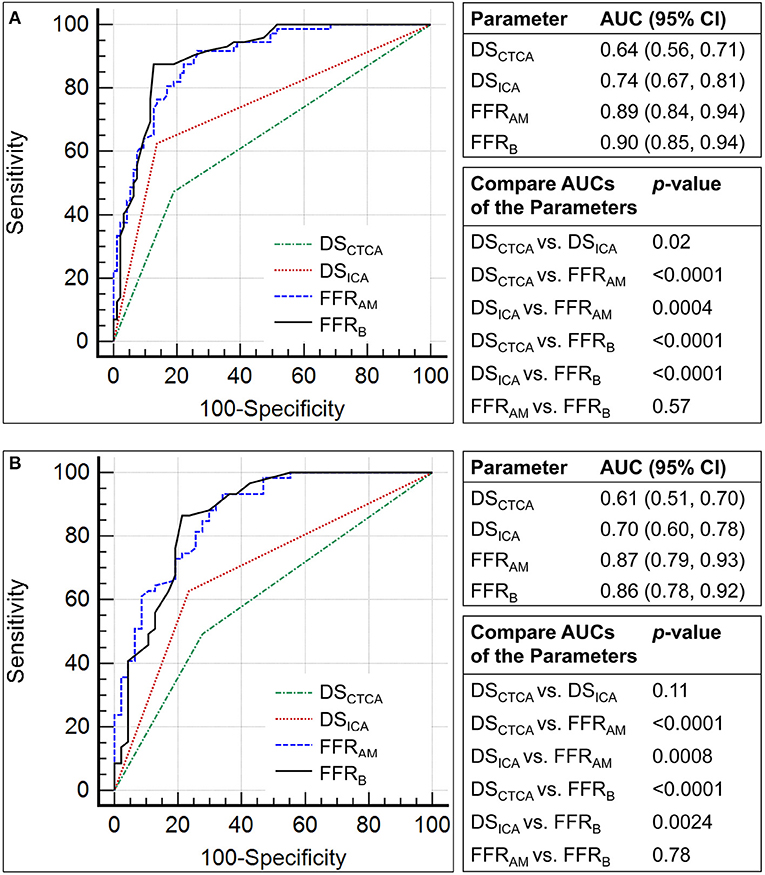
Figure 6. Comparison of the receiver operating characteristic curves for the discrimination of myocardial ischemia (fractional flow reserve, FFR ≤0.8) using diameter stenosis (DS; DSCTCA and DSICA, with a threshold of 70%) and non-invasive FFR (FFRAM and FFRB) on (A) per-vessel and (B) per-patient levels.
With invasive FFR as a reference standard, 32 lesions in 27 patients were wrongly classified with FFRAM and 22 lesions in 19 patients were wrongly classified with FFRB. At both per-vessel and per-patient levels, FFRAM and FFRB achieved a significantly improved accuracy compared with DSCTCA ≥50%, DSICA ≥50%, DSCTCA ≥70%, and DSICA ≥70% (Table 3).
Computational Time FFRAM vs. FFRB
Excluding image acquisition and segmentation, the computational time for FFRB was 48 ± 36 min (range 0.12 to 3.67 h) using parallel computation on a Dell T7800 workstation. The corresponding computational time for FFRAM was 2.2 ± 0.9 min, using a single CPU of the same workstation.
Discussion
In this study, we have developed a novel analytical method to determine FFRAM non-invasively from patient-specific 3D models reconstructed from CTCA images. The FFRAM exhibited a good correlation with invasive FFR and had a diagnostic performance close to CFD-based FFRB. We have also demonstrated the diagnostic performance of FFRB in a prospective study design. The computational time for FFRAM was much shorter than that for FFRB.
Our analytical model compartmentalized the stenosed coronary vessel into segments with distinct geometry to simplify the calculation of the corresponding pressure drops. We used anatomical information and LVM to calculate the flow rate through lesions and then input them into empirical equations with anatomical parameters measured on 3D coronary models to calculate energy loss due to the expansion and constriction of the lumen cross-section, which facilitates non-invasive FFRAM calculation. A major advantage of estimating FFRAM non-invasively using the analytical model is computational speed since the computational cost of CFD is eliminated. The analysis took slightly more than 2 min on a single CPU. This speed was achieved with little compromise in diagnostic accuracy. The flow rates through the lesions calculated in our analytical method using only anatomical information had a good correlation with that obtained by CFD simulation (r = 0.95), and the derived FFRAM demonstrated a fair correlation and good agreement with invasive FFR and was close to FFRB. For the diagnosis of ischemia, FFRAM had similar AUC (0.89 vs. 0.90, p = 0.57 and 0.87 vs. 0.86, p = 0.78 on per-vessel and per-patient bases, respectively) and specificity (86 vs. 86% and 79 vs. 77% on per-vessel and per-patient bases, respectively) but with slightly lower sensitivity (75 vs. 88% and 73 vs. 86% on per-vessel and per-patient bases, respectively) and NPV (82 vs. 90% and 70 vs. 82% on per-vessel and per-patient bases, respectively) compared with FFRB. Notably, both methods had superior diagnostic performance to routine methods, including DSCTCA and DSICA.
The FFRAM derived from the lesion lumen area, length, flow entry and exit angles, and flow rate with fluid equations is different from diameter stenosis and other measurements of coronary morphologic information. It is related more to coronary hemodynamics and physiology. The lesion length and diameter have been employed by other investigators as indirect measures of fractional flow reserve (29). Our current study showed a greater mean value of lesion length (11.46 ± 7.40 vs. 10.07 ± 5.97 mm, p = 0.223) and a smaller lesion area (3.05 ± 1.56 vs. 4.56 ± 2.41 mm2, p < 0.0001) in the group with FFR ≤ 0.8 vs. the group with FFR >0.8. As a result, the estimated coronary morphologic index [eg., lesion length/minimal lesion diameter (29)] from our study is significant greater (12.3 vs. 4.8, p < 0.0001) in the group with FFR ≤ 0.8 vs. the group with FFR >0.8, which is in agreement with the findings from the study of Li (29). In addition to the aforementioned coronary morphologic index, other lesion geometric parameters, like flow entry and exit angles to lesions, have been associated with fluid convective and diffusive energy loss and pressure drop (3, 4). We have incorporated these additional elements in formulating the expressions for FFRAM calculation. By decomposing a coronary lesion model of finite length into a spatial series of a proximal contracting segment, middle stenotic segment, and distal expanding segment to derive the model equations, FFRAM presents an integrated assessment of coronary hemodynamics that provides a more accurate assessment of coronary physiology than morphologic stenosis index.
CTCA-Based Non-invasive FFR to Discriminate Ischemic Lesions
Recent developments in CFD and CTCA imaging have made the calculation of non-invasive FFR feasible. NXT (6) and Discover-flow trials (5) employed standard transient CFD simulation and reported accuracy, sensitivity, specificity of 86, 84, and 86% (6) and 84.3, 87.9, and 82.2% (5), respectively, on a per-vessel basis and 80, 85, and 79% (6) and 87, 93, and 82% (5) on a per-patient basis. In the current study, our previously developed reduced-order CFD-based FFRB (9) yielded commensurate accuracy, sensitivity, and specificity of 87, 88, and 86% on a per-vessel basis and 82, 86, and 77% on a per-patient basis. While there are limitations to cross-trial comparisons, the AUCs of FFRB [0.90 (0.85, 0.94) and 0.86 (0.78, 0.92) on per-vessel and per-patient bases, respectively] and FFRAM [0.89 (0.84, 0.94) and 0.87 (0.79, 0.93) on per-vessel and per-patient bases respectively] were in the similar range of and were intermediate between the AUCs reported for FFRCT in the DeFACTO [0.79 (0.72, 0.87) on a per-patient basis] (30) and NXT trials [0.93 (0.91, 0.95) and 0.90 (95% CI: 0.87 to 0.94) on per-vessel and per-patient bases, respectively) (6), suggesting that both compared favorably with standard transient CFD-based approaches.
While CFD-based non-invasive FFR can improve the diagnostic performance of DSCTCA alone, it is provided as a remote service with a long turnaround time due to the significant computational costs incurred for mesh generation and iterative solutions to solve numerical equations, which are procedures intrinsic to flow simulation (5, 6). To facilitate on-site non-invasive FFR computation, Coenen et al. (31) modeled the coronary vessel as a 1D segment for simulation and mapped the calculated cFFR onto the 3D model reconstructed from CTCA images. The computational time was reduced to 5–10 min per patient, but the accuracy was only 74.6% with invasive FFR as reference (31). Machine-learning based artificial intelligence (AI) algorithms were introduced to reduce the calculation time of non-invasive FFR in some studies that were mainly based on retrospective investigations (13–15). These required ample synthetic datasets for training before the AI algorithms could be applied. Another option to reduce computational time entails the use of analytical models. Huo et al. (16) reported an analytical method to estimate FFR from the dimensions of stenosis and hyperemic coronary flow. The method relied on in vitro or animal experiments to obtain hyperemic coronary flow, which hindered its applicability outside the laboratory. In contrast, our new analytical model uses only anatomical information and does not require in vitro or in vivo experiments. With relatively similar diagnostic performance as and lower computational demand than CFD-based approaches, the application of FFRAM for on-site non-invasive FFR analysis may become feasible.
Linkage of Parameters in the Analytical Model to Features in AI Algorithms
AI algorithms can facilitate non-invasive FFR estimation (13). The judicious selection of input parameters plays an important role in the accuracy of machine learning. Table 2 shows the list of anatomical features measured on or derived from CTCA-derived 3D coronary models and their discriminative capability for ischemic lesions. These parameters can aid in the feature selection of diagnostic AI algorithms. Flow quantitation by machine learning can also be facilitated using anatomical features since the coronary flow rates in the lesions that were derived from anatomical information showed a strong correlation with the CFD simulation results in our study (r = 0.95, p < 0.0001).
Minimal lumen area measured on intravascular ultrasound has been correlated with FFR-ascertained ischemia (32), and a minimal lumen area ≤ 3.0 mm (2) indicates a high likelihood of significant obstruction in a normal-sized coronary vessel (32). Accordingly, minimal lumen area has been adopted as one of the features for angiography-based machine learning algorithms (33). In our study, the lumen area at the site of maximum stenosis (As) was significant smaller in ischemic vs. non-ischemic lesions (3.05 ± 1.56 vs. 4.56 ± 2.41 mm2, p < 0.0001), and we believe that it is a prime candidate for feature selection in machine learning. Due to curvature changes in the stenotic region, the flow entrance and exit angles α and β were significantly different between the ischemic and non-ischemic lesions in this study. As such, their effects on FFR prediction can be explored in future machine learning, together with other anatomical parameters, such as lumen areas, lesion lengths, etc.
Despite the potential of AI to non-invasive FFR, its clinical application remains challenging. The problem in AI lies in training data paucity, clinical interpretation, commercial deployment, and safety. Our method is based on coronary morphologic parameters and fluid dynamic principles and does not need training data. Importantly, the calculation can be completed with a much shorter computational time than full computational fluid dynamics. Lastly, we have developed a visualization system for physicians to view the computational results from both anatomic modeling and calculated FFRAM and FFRB. This holds a potential application for the further personalized management of CAD patients like virtual stent simulation in our recent publication (34).
Limitations of the Study
There are limitations in this study. First, a high calcium score may preclude accurate segmentation, which is a problem common to all CTCA-based analysis. The lumen segmentations were carefully examined by two experienced radiologists in the current study to ensure the accuracy of the results. Second, hyperemia was induced by either an intravenous infusion or intracoronary bolus of adenosine; nonetheless, prior studies have reported that the intravenous infusion of adenosine yielded an identical FFR result compared with intracoronary bolus (35). Lastly, this study did not use recently developed instantaneous wave-free ratio and resting full-cycle ratio non-hyperemic indexes of coronary artery stenosis severity as a reference method.
Conclusions
In this prospective multicenter study, an analytical method that calculates non-invasive FFRAM from CTCA and anatomical features offers a novel and expeditious non-CFD approach that demonstrated good diagnostic performance for detecting ischemic coronary lesions as ascertained by invasive FFR.
Data Availability Statement
The original contributions presented in the study are included in the article/Supplementary Material, further inquiries can be directed to the corresponding authors.
Ethics Statement
The studies involving human participants were reviewed and approved by SingHealth Centralised Institutional Review Board. The patients/participants provided their written informed consent to participate in this study.
Author Contributions
STL and LZ conceived the study design. J-MZ and HH analyzed the data. R-ST, PC, JF, LT, CC, CO, WH, LB, GK, AL, MC, KC, PL, AW, SYT, TC, STL, and LZ interpreted the results. JA performed the statistical analysis. J-MZ drafted the manuscript. R-ST, PC, JF, LT, CC, CO, RL, GC, SL, WH, JA, LB, GK, AL, MC, KC, PL, AW, SYT, TC, STL, and LZ edited and revised the manuscript. All authors read and approved the final manuscript.
Funding
This study has received funding from the National Medical Research Council Singapore (NMRC/BnB/0017/2015 and MOH-000358). The funder had no role in the design and conduct of the study; collection, management, analysis, and interpretation of the data; and preparation, review, or approval of the manuscript.
Conflict of Interest
The authors declare that the research was conducted in the absence of any commercial or financial relationships that could be construed as a potential conflict of interest.
Publisher's Note
All claims expressed in this article are solely those of the authors and do not necessarily represent those of their affiliated organizations, or those of the publisher, the editors and the reviewers. Any product that may be evaluated in this article, or claim that may be made by its manufacturer, is not guaranteed or endorsed by the publisher.
Supplementary Material
The Supplementary Material for this article can be found online at: https://www.frontiersin.org/articles/10.3389/fcvm.2021.739633/full#supplementary-material
Abbreviations
AM, analytical method; AUC, area under the receiver operating characteristic curve; CAD, coronary artery disease; CFD, computational fluid dynamics; CTCA, computed tomography coronary angiography; DS, diameter stenosis; FFR, fractional flow reserve; ICA, invasive coronary angiography; L, lesion length; LAD, left anterior descending; LR, likelihood ratio; NPV, negative predictive value; PPV, positive predictive value; Q, flow rate; ROC, receiver operating characteristic; SD, standard deviation; α, flow entrance angle; β, flow exit angle.
References
1. Min JK, Chandrashekhar Y. Atherosclerosis, stenosis, and ischemia: one primary, one secondary, and one tertiary. JACC Cardiovasc Imaging. (2018) 11:531–3. doi: 10.1016/j.jcmg.2017.12.006
2. De Bruyne B, Pijls NH, Kalesan B, Barbato E, Tonino PA, Piroth Z, et al. Fractional flow reserve-guided PCI versus medical therapy in stable coronary disease. N Engl J Med. (2012) 367:991–1001. doi: 10.1056/NEJMoa1205361
3. Xaplanteris P, Fournier S, Pijls NHJ, Fearon WF, Barbato E, Tonino PAL, et al. Five-year outcomes with PCI guided by fractional flow reserve. N Engl J Med. (2018) 379:250–9. doi: 10.1056/NEJMoa1803538
4. Benz DC, Giannopoulos AA. Fractional flow reserve as the standard of reference: all that glistens is not gold. J Nucl Cardiol. (2019) 27:1314–6. doi: 10.1007/s12350-019-01771-3
5. Koo BK, Erglis A, Doh JH, Daniels DV, Jegere S, Kim HS, et al. Diagnosis of ischemia-causing coronary stenoses by noninvasive fractional flow reserve computed from coronary computed tomographic angiograms: results from the prospective multicenter DISCOVER-FLOW (diagnosis of ischemia-causing stenoses obtained via noninvasive fractional flow reserve) study. J Am Coll Cardiol. (2011) 58:1989–97. doi: 10.1016/j.jacc.2011.06.066
6. Nørgaard BL, Leipsic J, Gaur S, Seneviratne S, Ko BS, Ito H, et al. Diagnostic performance of noninvasive fractional flow reserve derived from coronary computed tomography angiography in suspected coronary artery disease: the NXT trial (analysis of coronary blood flow using CT angiography: next steps). J Am Coll Cardiol. (2014) 63:1145–55. doi: 10.1016/j.jacc.2013.11.043
7. Ihdayhid AR, Norgaard BL, Gaur S, Leipsic J, Nerlekar N, Osawa K, et al. Prognostic value and risk continuum of noninvasive fractional flow reserve derived from coronary CT angiography. Radiology. (2019) 292:343–51. doi: 10.1148/radiol.2019182264
8. Nørgaard BL, Hjort J, Gaur S, Hansson N, Bøtker HE, Leipsic J, et al. Clinical use of coronary CTA–derived FFR for decision-making in stable CAD. JACC Cardiovasc Imaging. (2017) 10:541–50. doi: 10.1016/j.jcmg.2015.11.025
9. Zhang JM, Zhong L, Luo T, Lomarda AM, Huo Y, Yap J, et al. Simplified models of non-invasive fractional flow reserve based on CT images. PLoS ONE. (2016) 11:e0153070. doi: 10.1371/journal.pone.0153070
10. Ko BS, Cameron JD, Munnur RK, Wong DTL, Fujisawa Y, Sakaguchi T, et al. Noninvasive CT-derived FFR based on structural and fluid analysis: a comparison with invasive FFR for detection of functionally significant stenosis. JACC Cardiovasc Imaging. (2017) 10:663–73. doi: 10.1016/j.jcmg.2016.07.005
11. Zhang JM, Shuang D, Baskaran L, Wu W, Teo SK, Huang W, et al. Advanced analyses of computed tomography coronary angiography can help discriminate ischemic lesions. Int J Cardiol. (2018) 267:208–14. doi: 10.1016/j.ijcard.2018.04.020
12. Siogkas PK, Anagnostopoulos CD, Liga R, Exarchos TP, Sakellarios AI, Rigas G, et al. Noninvasive CT-based hemodynamic assessment of coronary lesions derived from fast computational analysis: a comparison against fractional flow reserve. Eur Radiol. (2019) 29:2117–26. doi: 10.1007/s00330-018-5781-8
13. Itu L, Rapaka S, Passerini T, Georgescu B, Schwemmer C, Schoebinger M, et al. A machine-learning approach for computation of fractional flow reserve from coronary computed tomography. J Appl Physiol. (2016) 121:42–52. doi: 10.1152/japplphysiol.00752.2015
14. Tang CX, Liu CY, Lu MJ, Schoepf UJ, Tesche C, Bayer RR, et al. CT FFR for ischemia-specific CAD with a new computational fluid dynamics algorithm: a Chinese multicenter study. JACC Cardiovasc Imaging. (2020) 13:980–90. doi: 10.1016/j.jcmg.2019.06.018
15. Tesche C, De Cecco CN, Baumann S, Renker M, McLaurin TW, Duguay TM, et al. Coronary CT angiography–derived fractional flow reserve: Machine learning algorithm versus computational fluid dynamics modeling. Radiology. (2018) 288:64–72. doi: 10.1148/radiol.2018171291
16. Huo Y, Svendsen M, Choy JS, Zhang Z-D, Kassab GS. A validated predictive model of coronary fractional flow reserve. J R Soc Interface. (2012) 9:1325–38. doi: 10.1098/rsif.2011.0605
17. Ahmadi A, Stone GW, Leipsic J, Serruys PW, Shaw L, Hecht H, et al. Association of coronary stenosis and plaque morphology with fractional flow reserve and outcomes. JAMA Cardiol. (2016) 1:350–7. doi: 10.1001/jamacardio.2016.0263
18. Bashore TM, Balter S, Barac A, Byrne JG, Cavendish JJ, Chambers CE, et al. 2012 American college of cardiology foundation/society for cardiovascular angiography and interventions expert consensus document on cardiac catheterization laboratory standards update. J Am Coll Cardiol. (2012) 59:2221–305. doi: 10.1016/j.jacc.2012.02.010
19. Adjedj J, Xaplanteris P, Toth G, Ferrara A, Pellicano M, Ciccarelli G, et al. Visual and quantitative assessment of coronary stenoses at angiography versus fractional flow reserve: the impact of risk factors. Circ Cardiovasc Imaging. (2017) 10:e006243. doi: 10.1161/CIRCIMAGING.117.006243
20. Abbara S, Blanke P, Maroules CD, Cheezum M, Choi AD, Han BK, et al. SCCT guidelines for performance of coronary computed tomographic angiography: a report of the Society of Cardiovascular Computed Tomography Guidelines Committee. J Cardiovasc Comput Tomogr. (2009) 3:190–204. doi: 10.1016/j.jcct.2009.03.004
21. Heo R, Park H-B, Lee BK, Shin S, Arsanjani R, Min JK, et al. Optimal boundary detection method and window settings for coronary atherosclerotic plaque volume analysis in coronary computed tomography angiography: comparison with intravascular ultrasound. Eur Radiol. (2016) 26:3190–8. doi: 10.1007/s00330-015-4121-5
22. Wieneke H, von Birgelen C, Haude M, Eggebrecht H, Möhlenkamp S, Schmermund A, et al. Determinants of coronary blood flow in humans: quantification by intracoronary doppler and ultrasound. J Appl Physiol. (2005) 98:1076–82. doi: 10.1152/japplphysiol.00724.2004
23. Zhang JM, Chandola G, Tan RS, Chai P, Teo LLS, Low R, et al. Quantification of effects of mean blood pressure and left ventricular mass on noninvasive fast fractional flow reserve. Am J Physiol Heart Circ Physiol. (2020) 319:H360–9. doi: 10.1152/ajpheart.00135.2020
24. Wilson RF, Wyche K, Christensen BV, Zimmer S, Laxson DD. Effects of adenosine on human coronary arterial circulation. Circulation. (1990) 82:1595–606. doi: 10.1161/01.CIR.82.5.1595
25. Rennels DC, Hudson HM. Pipe Flow: A Practical and Comprehensive Guide. John Wiley & Sons, Inc. Hoboken, New Jersey (2012). doi: 10.1002/9781118275276
26. Huo Y, Kassab GS. Intraspecific scaling laws of vascular trees. J R Soc Interface. (2012) 9:190–200. doi: 10.1098/rsif.2011.0270
27. Zhong L, Zhang JM, Su B, Tan RS, Allen JC, Kassab GS. Application of patient-specific computational fluid dynamics in coronary and intra-cardiac flow simulations: challenges and opportunities. Front Physiol. (2018) 9:742. doi: 10.3389/fphys.2018.00742
28. DeLong ER, DeLong D, Clarke-Pearson D. Comparing the areas under two or more correlated receiver operating characteristic curves: a nonparametric approach. Biometrics. (1988) 44:837–45. doi: 10.2307/2531595
29. Li M, Zhang J, Pan J, Lu Z. Coronary stenosis: morphologic index characterized by using CT angiography correlates with fractional flow reserve and is associated with hemodynamic status. Radiology. (2013) 269:713–21. doi: 10.1148/radiol.13122550
30. Nakazato R, Park HB, Berman DS, Gransar H, Koo BK, Erglis A, et al. Noninvasive fractional flow reserve derived from computed tomography angiography for coronary lesions of intermediate stenosis severity results from the DeFACTO study. Circ Cardiovasc Imaging. (2013) 6:881–9. doi: 10.1161/CIRCIMAGING.113.000297
31. Coenen A, Lubbers MM, Kurata A, Kono A, Dedic A, Chelu RG, et al. Fractional flow reserve computed from noninvasive CT angiography data: diagnostic performance of an on-site clinician-operated computational fluid dynamics algorithm. Radiology. (2015) 274:674–83. doi: 10.1148/radiol.14140992
32. Kang J, Koo BK, Hu X, Lee JM, Hahn JY, Yang HM, et al. Comparison of fractional flow reserve and intravascular ultrasound-guided intervention strategy for clinical outcomes in patients with intermediate stenosis (FLAVOUR): rationale and design of a randomized clinical trial. Am Heart J. (2018) 199:7–12. doi: 10.1016/j.ahj.2017.11.001
33. Cho H, Lee JG, Kang SJ, Kim WJ, Choi SY, Ko J, et al. Angiography-based machine learning for predicting fractional flow reserve in intermediate coronary artery lesions. J Am Heart Assoc. (2019) 8:e011685. doi: 10.1161/JAHA.118.011685
34. Chandola G, Zhang JM, Tan RS, Chai P, Teo L, Allen JC, et al. Computed tomography coronary angiography and computational fluid dynamics based on fractional flow reserve before and after percutaneous coronary intervention. Front Bioeng Biotechnol. (2021) 9:739667. doi: 10.3389/fbioe.2021.739667
Keywords: coronary artery disease, fractional flow reserve, computed tomography coronary angiography, analytical method, non-invasive
Citation: Zhang J-M, Han H, Tan R-S, Chai P, Fam JM, Teo L, Chin CY, Ong CC, Low R, Chandola G, Leng S, Huang W, Allen JC, Baskaran L, Kassab GS, Low AFH, Chan MY-Y, Chan KH, Loh PH, Wong ASL, Tan SY, Chua T, Lim ST and Zhong L (2021) Diagnostic Performance of Fractional Flow Reserve From CT Coronary Angiography With Analytical Method. Front. Cardiovasc. Med. 8:739633. doi: 10.3389/fcvm.2021.739633
Received: 11 July 2021; Accepted: 10 September 2021;
Published: 20 October 2021.
Edited by:
Grigorios Korosoglou, GRN Klinik Weinheim, GermanyCopyright © 2021 Zhang, Han, Tan, Chai, Fam, Teo, Chin, Ong, Low, Chandola, Leng, Huang, Allen, Baskaran, Kassab, Low, Chan, Chan, Loh, Wong, Tan, Chua, Lim and Zhong. This is an open-access article distributed under the terms of the Creative Commons Attribution License (CC BY). The use, distribution or reproduction in other forums is permitted, provided the original author(s) and the copyright owner(s) are credited and that the original publication in this journal is cited, in accordance with accepted academic practice. No use, distribution or reproduction is permitted which does not comply with these terms.
*Correspondence: Liang Zhong, emhvbmcubGlhbmdAbmhjcy5jb20uc2c=