- 1College of Health Science and Medicine, Wolaita Soddo University, Wolaita Soddo, Ethiopia
- 2College of Medicine and Health Science, Hawassa University, Hawassa, Ethiopia
- 3College of Medicine and Health Science, Dilla University, Dilla, Ethiopia
- 4Department of Anesthesia, Kotebe Metropolitan University, Addis Ababa, Ethiopia
Background: Dyslipidemia is a common public health problem in Africa. It has emerged as an important cardiovascular risk factor. It has been steadily increasing due to economic growth, urbanization, and unhealthy dietary pattern. Therefore, it is essential to identify determinants of dyslipidemia to prevent the condition and reduce its long-term sequel.
Methods: Combinations of search terms with Boolean operators were used to retrieve studies from PubMed, EMBASE, Cochrane Database, Cinahl, Scopus, Mednar, and Google Scholar. The methodological quality of each article was evaluated based on the 2017 Joanna Briggs Institute (JBI) Critical Appraisal checklist for prevalence studies. After evaluation of each study against these criteria, studies with a minimum score of 7 or above out of 9 JBI checklists were included. We included articles presented in the English language. The Cochrane Q test was used to assess the heterogeneity across studies. The visual assessment of publication bias was done by creating a funnel plot. The possible causes of heterogeneity were explored by subgroup analyses. Egger's weighted regression test was used to assess the presence of publication bias. Statistical analyses were done by using the STATA software version 14.
Result: A total of 24 articles involving 37,902 participants from 10 African countries were included. The overall pooled prevalence of dyslipidemia was 52.8 (95% CI 40.8–64.9). Individuals with a body mass index (BMI) >25.0 kg/m2 and waist circumference (WC) >94 cm were, respectively, 2.36 (95% CI (1.33–4.18), p < 0.001) and 2.33 (95% CI (0.75–0.29) p < 0.001) times more likely to develop dyslipidemia than those with lower values. Furthermore, patients with diabetes mellitus (DM) and hypertension (HTN) were 2.32 (95% CI (0.89–6.05) p < 0.001) and 2.05 (95% CI (1.31–3.21), p < 0.001) times more likely to present with dyslipidemia than non-diabetic patients and those without HTN.
Conclusion: This study revealed that the prevalence of dyslipidemia is relatively high among study participants in African countries and the independent predictors of dyslipidemia were BMI >25.0 kg/m2, WC > 94 cm, raised blood glucose level, and raised blood pressure. Therefore, there should be a pressing public health measure to prevent, identify, and treat dyslipidemia with the special emphasis on obese, diabetic, and hypertensive patients.
Background
Dyslipidemia is a state that occurs due to abnormalities in the plasma lipids. These include increased plasma total cholesterol (TC), increased low-density lipoprotein cholesterol (LDL-C), increased triglycerides (TGs), and reduced high-density lipoprotein cholesterol (HDL-C) levels. It presents either as an elevation of one of the lipids or high levels of a combination of the lipids (1, 2). The exact mechanism of dyslipidemia is not fully understood but is most likely multifactorial with genetic variations being shown to account for about 43–83% of the variability of plasma lipoprotein levels in a normal healthy population (3, 4). Dyslipidemia is an autosomal dominant disorder caused by mutations in the low-density lipoprotein receptor (LDLR) gene. As a result of defective cell-surface LDLR, clearance of LDL-C from plasma is reduced leading to the increased plasma levels of LDL-C and TC (5). Individuals with dyslipidemia have a 2-fold higher risk of developing cardiovascular diseases (CVDs) than those with normal lipid levels (6).
Both LDL-C and HDL-C regulate the amount of cholesterol in the body. An imbalance between the two can increase the risk of myocardial infarction and stroke (7). The increased levels of LDL-C aggravate the development of atherosclerosis, which is documented as the main risk factor for stroke, peripheral vascular, and ischemic heart disease (IHD) (8). However, HDL-C helps to remove cholesterol from the body, which decreases the risk of atherosclerotic CVD (6).
Though the burden of disease in Africa has been dominated by infectious diseases, countries in this region are undergoing a continuous demographic change leading to the increasing frequency of non-communicable diseases (NCDs) (9). Substantial changes in the health of the population marked by the rising burden of CVDs are set to overtake infectious diseases as the leading cause of death by 2030. This epidemiological shift is mainly driven by unhealthy lifestyles due to rapid urbanization and modernization. Recently, infectious agents are considered more frequently as causes of diseases that have been thought previously to be of non-infectious etiology, such as coronary heart disease. Additionally, lipopolysaccharide (LPS) affects the circulating macrophages and increases the production of free radicals. Free radicals are known to oxidize LDL-C and transform macrophages into foam cells, which are known to be crucial in the pathogenesis of atherosclerosis (10).
Dyslipidemia is responsible for more than half of the global IHD and more than 4 million deaths annually (11). It remains a common public health problem as abnormal serum lipid profile has been steadily increasing due to economic growth, urbanization, and unhealthy lifestyles (12). In Africa, the prevalence of dyslipidemia ranges from 5.2 (13) to 89.9% (14). The presentation of an abnormal lipid profile is common in individuals with central obesity, anti-retroviral therapy (ART), metabolic syndrome, insulin resistance, and type 2 diabetes mellitus (DM) (15). The duration of HIV treatment, advanced age, sex, low CD4 counts, smoking, alcohol consumption, depression (12, 15, 16), and a diet high in saturated fat, sedentary lifestyle, and obesogenic (17) are the major risk factors of dyslipidemia.
The prevalence of CVD is mainly result of risk factors, mostly hypertension (HTN), diabetes, and obesity (9). Dyslipidemia is also more common among patients with coexisting cardiovascular risk factors, such as HTN, diabetes, or HIV (10). A systematic review conducted in the Gambia on CVD risk factors revealed the prevalence of insufficient fruit and vegetable consumption, inadequate physical activity, and alcohol consumption was 77.8, 14.6, and 2.3%, respectively (18).
Most (80%) of the lipid disorders are associated with diet and lifestyle (17). The dietary habits of populations in low-to-middle-income countries (LMICs) have rapidly shifted to less-healthy diets (19). These consist of processed foods, away-from-home food intake, and increased use of edible oils and sugar-sweetened beverages (20).
In recent decades, the global pattern of unhealthy diets driving the occurrence of metabolic disorders and NCDs has become more important in LMICs because of the double burden of diseases in such countries that jointly constitute major causes of morbidity and mortality (21). In many African countries, surveillance and research on NCDs are still lacking (22). However, in the last decades, the number of NCDs-related deaths in the region have grown dramatically (9). This review will allow researchers to assess the disease burden of dyslipidemia in Africa where data are often scarce and populations are understudied. Assessing the prevalence of dyslipidemia can also aid researchers in predicting the future disease development of conditions contingent on dyslipidemia, such as CVD. Accurate data on dyslipidemia prevalence and risk factors can serve to inform health professionals, policy makers, and the public to manage and plan. However, different previous studies conducted on risk factors of dyslipidemia showed conflicting results. Understanding the context-specific factors associated with dyslipidemia and its potential implications are critical to designing effective interventions for the prevention and treatment of metabolic and cardiovascular effects of dyslipidemia.
Methods
The Study Protocol and Reporting
The Preferred Reporting Items for Systematic Reviews and Meta-Analyses (PRISMA) guidelines for literature search strategy, selection of studies, data extraction, and result reporting were followed while conducting this systematic review and meta-analysis. The eligibility criteria based on the Condition, Context, and Population (COCOPO) principle was adapted from the Joanna Briggs Institute (JBI) 2017 review guideline (23). Endnote (version X8) reference management software was used to download, organize, review, and cite related articles. The protocol of this review was not registered in the Prospero database.
Context
All eligible cross-sectional study, cohort study, comparative cross-sectional study, national survey, and a longitudinal study conducted on the prevalence and risk factors of dyslipidemia among patients with HIV, diabetes, HTN, and Lichen Planus, women on hormonal contraceptives, and general population in Africa were considered.
Condition
This review considered the studies that measured the outcome of interest-based prevalence and risk factors of dyslipidemia.
Variables and Measures
The primary outcome of this study was dyslipidemia. The case definitions of dyslipidemia were increased TC ≥5.17 mmol/L (≥200 mg/dl); high LDL-C ≥3.36 mmol/L (≥130 mg/dl), increased TG ≥1.7 mmol/L (≥150 mg/dl); and low HDL-C <1.03 mmol/L (<40 mg/dl) for men, <1.3 mmol/L (<50 mg/dl) for women. Study subjects who met one or more of the above criteria were categorized as having dyslipidemia. Participants who were on a lipid-lowering agent were also classified as having dyslipidemia (24, 25). Identified determinants of dyslipidemia were defined as follows: abdominal obesity was defined as WC >94 cm for men and >88 cm for women (26). BMI was classified as underweight (<18.5 kg/m2), normal (18.5–24.9 kg/m2), overweight (25.0–29.9 kg/m2), or obese (≥30 kg/m2) (27). Participants were considered as hypertensive if blood pressure (BP) is ≥140/90 mmHg. In this study, high fasting blood glucose (FBG) was defined as ≥5.6 mmol/L (≥100 mg/dl) or if the patient is on treatment for diabetes (28).
Inclusion Criteria
We included all studies that fulfilled the above case definition of dyslipidemia. However, we considered studies that were conducted on determinants of metabolic syndrome and hypercholesterolemia if risk factors of dyslipidemia were identified. In such a case, we carefully checked its compliance with the case definition before considering it for the final analysis. Furthermore, full text and/or open access articles, a study conducted in Africa, published and unpublished studies are written in the English language before August 8, 2021, were included.
Study Design and Search Strategy
The search was limited to studies published in the English language from January 2000 to July 20, 2021. For published studies, the initial restricted search of PubMed, EMBASE, Cochrane Database, Cinahl, Scopus, Mednar, and Google Scholar were undertaken. Thereafter, unpublished studies were searched from Addis Ababa University and Jimma University institutional repositories. Then, a second search term using all identified keywords and index terms was undertaken. Third, the reference list of all identified reports and articles was searched for extra studies. We searched the database with terms including:
“Dyslipid*,” “Dyslipidemia,” ”Dyslipidaemia,” “metabolic syndrome,” “hypercholesterol*,” “hypercholesterolemia,” hypercholesterolemia “prevalence,” “associated factors,” “general population,” “Sub-Saharan Africa,” and “Africa” for the articles published in English before August 8, 2021. The search detail of this systematic review with meta-analysis was uploaded as dataset 1.
Data Quality Control Measures
The methodological quality of each article was evaluated based on the 2017 JBI Critical Appraisal checklist for prevalence studies (23). After the evaluation of each study against these criteria, studies scoring 7 or above were included. On the other hand, studies scoring below 7 out of 9 criteria of Critical Appraisal instruments for prevalence studies were excluded. The quality of each study was evaluated independently by two authors (MS. O. and G. A.). The discrepancy was solved by the discussion with the third independent reviewer (BZ. W.).
Data Extraction
The data were extracted by two independent authors (MS. O. and Y. H.) using a standard and piloted form. The extracted data were the last name of the author, year of publication, country, region, study participant, frequency or prevalence of the disease, LDL-C data, and risk factors dataset 2. Discrepancies were discussed and solved by consensus or by a third reviewer (G. A.).
Statistical Analysis
All statistical data analyses were done by using Stata version 14.0 (Statacorp. LP, College Station, TX, USA). A random effects meta-analysis based on the DerSimonian and Laird approach was used to pool the prevalence of dyslipidemia in Africa. The presence of statistical heterogeneity was checked using Higgins I-squared (I2) statistics and Cochran's-Q test. Accordingly, heterogeneity was classified as low, moderate, or high when the values of I2 were 25, 50, and 75%, respectively (13). The potential sources of heterogeneity were explored by meta regression and subgroup analysis. Publication bias was viewed graphically by the funnel plots and tested through Egger© and Begg© tests (29). Sensitivity analysis was done to identify the effect of a single study on the overall estimate.
Result
Search Results
Initially, a total of 1,137 studies were retrieved from the electronic databases and 1,480 articles were retrieved through manual searching. The total number of articles retrieved through an electronic database and manual searching was 2,617. From this, 1,125 were duplicates and removed from this systemic review. The remaining 1,492 articles were screened by their title and abstract and 1,438 irrelevant studies were removed. Then, 54 full-text articles were assessed for eligibility and 14 of them were excluded on the account of not reporting the outcome of interest, 7 studies were excluded due to exclusion criteria, and 8 studies were excluded due to language. Thereafter, 5 articles were excluded upon evaluation against the critical appraisal checklist of prevalence studies (23). Finally, 24 studies were included in this systematic review with meta-analysis (Figure 1).
Study Characteristics
A total of 24 articles from 10 African countries were included in this systematic review and meta-analysis. Out of 37,902 study subjects, 12,035 patients had dyslipidemia. The sample size across the studies ranges from 90 (30) to 10,690 (31). Out of 24 articles, nine were conducted in Ethiopia, four in South Africa, three in Nigeria, and two others in Kenya. The remainders of the studies of interest were conducted in Mozambique, Zambia, Senegal, Uganda, Togo, and Malawi. The highest prevalence of dyslipidemia was reported in a study from South Africa (89.9%) (14), and from Kenya (85.6%) (32). On the other hand, the lowest prevalence of dyslipidemia of 5.2% was reported in a study from Ethiopia (13). The highest percentage of LDL-C data was reported in a study from Ethiopia (79.7%) (33) (Table 1).
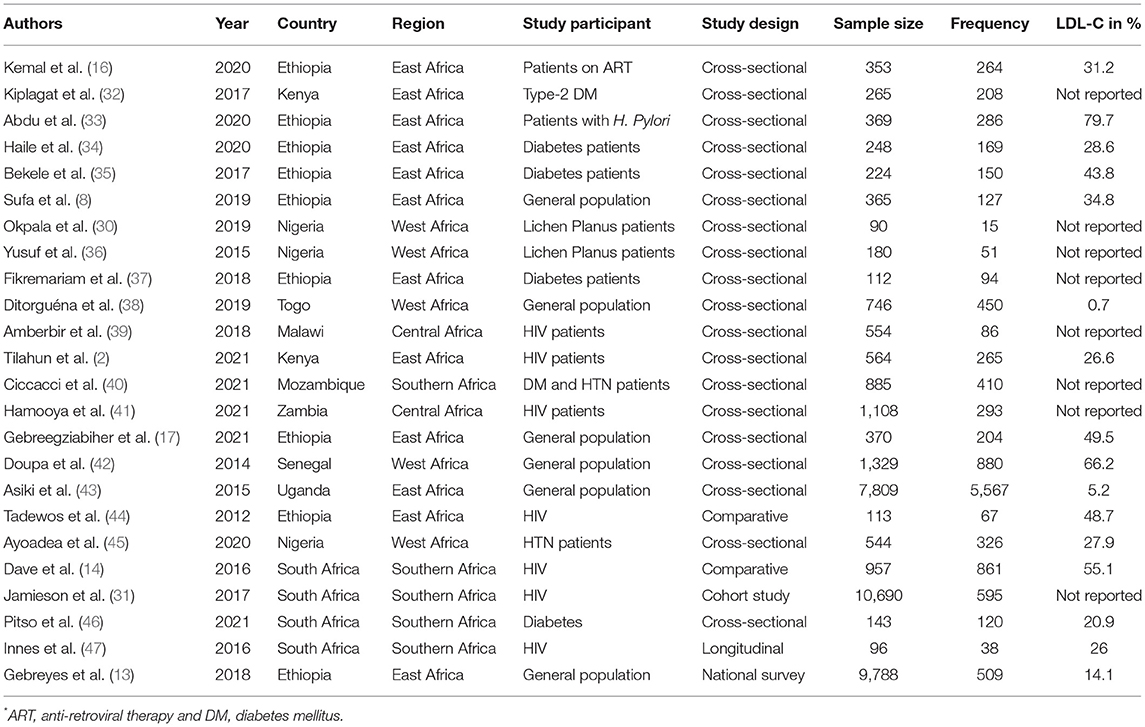
Table 1. Prevalence of dyslipidemia based on health status and for the general population in Africa, 2021.
Prevalence of Dyslipidemia
The overall pooled prevalence of dyslipidemia in Africa using the fixed effect model was 16.5 (95% CI (16.0–16.5). When using the fixed effect model, the pooled effect size of dyslipidemia showed a significant heterogeneity (I2) of 99.9% (p < 0.001). As a result, we determined the final pooled prevalence with a random-effect model to control the observed unevenness. The final pooled prevalence of dyslipidemia was 52.8 (95% CI (40.8–64.9) (Figure 2).
Heterogeneity
The subgroup analysis by the category of population and regions was done to handle the heterogeneity. In addition, we performed meta regression with sample size, prevalence, and year of publication. The details of subgroup analysis and meta regression were mentioned below.
Subgroup Analysis by the Category of Population or Study Participant
Subgroup analyses based on the underlying diseases and the countries revealed a marked variation in the prevalence of dyslipidemia. When we look at variation based on the category of study participant or population, the prevalence of dyslipidemia was the highest among the category of studies conducted on a group of participants with NCDs (patients with DM, HTN, and DM and HTN) [70.6 (95% CI (58.0–83.2)] and the general population [48.8 (95% CI (13.7–84.0)]. On the other hand, the lowest prevalence of dyslipidemia {43.7 [95% CI (18.9–68.5)]} was reported among studies conducted on a group of participants with infectious diseases (patients with HIV, H. Pylori, and Lichen Planus) (Table 2).
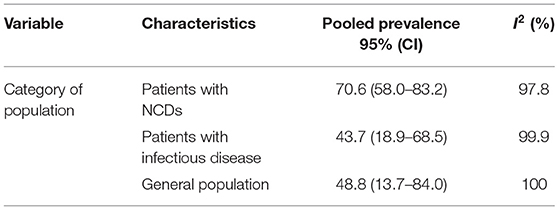
Table 2. The pooled prevalence of dyslipidemia, 95% CI, and heterogeneity estimate with a p-value for subgroup analysis.
A Subgroup of Analysis by the Region
A subgroup analysis by the region showed that there was marked variation across the regions of the African continent. The highest prevalence of dyslipidemia found in East Africa was 60.8 [(95% CI: 35.5–86.0), I2 = 99.9, p < 0.001] and Southern Africa was 53.1 (95% CI: 6.1–99.4), I2 = 100%, p < 0.001). The pooled prevalence of dyslipidemia in western Africa and Central Africa was 46.7 (95% CI: 32.4–60.9), I2 = 98.3, p < 0.001) and 21.0 (95% CI: 10.3–31.7), I2 = 96.5, p < 0.001), respectively. Out of 24 included studies, 12 studies were from East Africa, 5 studies were from western Africa, 5 studies were from southern Africa, and two studies were from central Africa. But, not a single study was included from the northern African region (Figure 3).
Meta Regression
We applied meta regression to identify the source of heterogeneity. Accordingly, random-effects meta regression was conducted by considering the year of publication, prevalence, and sample size as covariates. The analysis indicated that heterogeneity was explained by prevalence (p = 0.02) (Table 3).
Sensitivity Analysis
A sensitivity analysis was executed by removing studies step-by-step to evaluate the effect of a single study on the whole estimate. As shown in Table 4, no study exhibited an important effect on the final pooled prevalence of dyslipidemia.
Publication Bias
As indicated in Figure 4, the visual inspection of the funnel plot showed an asymmetrical distribution. When objectively evaluated against the Egger© regression test and an adjusted Begg© rank correlation test at a 5% significance level, we found p < 0.001 and 0.009, respectively. This confirmed that there was evidence of publication bias in this systematic review with meta-analysis. Therefore, Duval and Tweedie's trim and fill analysis (metatrim) using the random-effects model to see the impact of publication bias through the assumption that the funnel plot asymmetry was solely caused by publication bias might not hold for this dataset. The trim and fill analysis showed the presence of 13 unpublished studies. Considering these studies in calculating the pooled prevalence yields, an estimated pooled prevalence of dyslipidemia, which is adjusted for publication bias was found to be 13.38% [95% CI (0.52–26 24); p = 0.04] (Figure 5).
As shown in the regression graph (Figure 6), the estimated bias coefficient (intercept) is 1.14 with an SE of 0.26, giving a p < 0.001 and CI of 0.58–1.70. Thus, the test provides strong evidence for small-study effects.
The funnel plot showed a small study effect; Hhowever, the counter-enhanced funnel plot showed that small studies were found both in the area of statistical significance (shaded area) and non-statistical significance (white area). So, the asymmetry may have been caused by a number of other factors rather than the publication bias (Figure 7). Similar findings also occurred when we performed the metric counter-enhanced funnel plot (Figure 8).
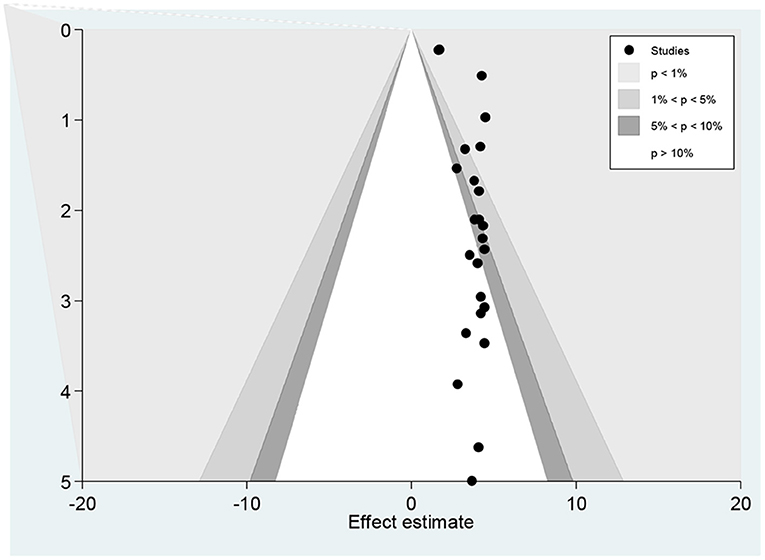
Figure 7. Counter enhanced funnel plots for publication bias for the prevalence of dyslipidemia in Africa, 2021.
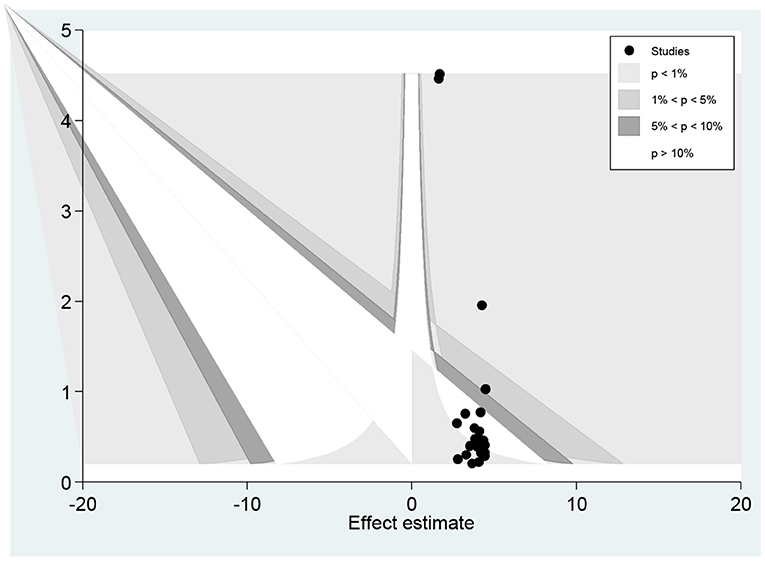
Figure 8. Meric inverse counter enhanced funnel plots of publication bias for the prevalence of dyslipidemia in Africa, 2021.
Determinants of Dyslipidemia in Africa
Furthermore, 11 variables were extracted to identify factors associated with dyslipidemia. Of these variables, 9 of them (sex, medication adherence, educational status, FBS, BP, BMI, WC, marital status, and sedentary life) were significantly associated with dyslipidemia at p < 0.05.
Patients with BMI >25 kg/m2 and patients with WC >94 cm were about 2.36 and 2.3 times more likely to develop dyslipidemia, as compared with their counterparts (odds ratio (OR): 2.36 (95% CI (1.33–4.18), p < 0.001, I2: 89.6%) and OR: 2.33 [95% CI (0.75–7.29), p < 0.001, I2: 89.6%)]. Patients with DM were about 2.3 times more likely to have dyslipidemia, as compared with non-diabetic patients {OR: 2.32 [95% CI (0.89–6.04)] p < 0.001, I2: 88.1%}. Furthermore, known smoker patients were about 132% more likely to develop dyslipidemia, as compared with non-smoker patients {OR: 1.32 [95% CI (0.74–2.35)] p = 0.05, I2: 80.7%].
This study also showed that patients with HTN were about 2 times more likely to have dyslipidemia when compared with non-hypertensive patients {OR: 2.05 [95% CI (1.31–3.21)], p < 0.001, I2: 85%}. Furthermore, patients who had no history of alcohol consumption and sedentary lifestyles were about 20% less likely to develop dyslipidemia than their counterparts {OR: 0.862 [95% CI (0.68–1.11)] and OR: 0.80 [95% CI (0.63–1.10)]}, respectively (Table 5).
Discussion
Dyslipidemia has emerged as an important cardiovascular risk factor in African countries (17). It is characterized by LDL-C increment, TG elevation, and HDL-C decrement or combined. The LDLR positioned on chromosome 19p13.2 plays a significant role in lipoprotein metabolism by mediating the uptake of cholesterol through the binding and subsequent cellular uptake of apolipoprotein-E and B- constituting lipoproteins. Mutations have been detected in different domains of the LDLR which have a distinct effect on LDLR structure and function. ATP-binding cassette A1 (ABCA1) plays a critical role in the reverse cholesterol transport system. Mutation in ABCA1, that encodes this protein, along with genes responsible for their transcription regulation, can lead to abnormality in the metabolism of lipids (48). Apolipoprotein A5 (APOA5) has been shown to be a key regulator of plasma TGs and there are several single nucleotide polymorphisms (SNPs) associated with the APOA5 gene (49). Future research should address the lack of LDL-C oxidation data generated by existing studies.
The prevalence of dyslipidemia in this review is higher than the finding of the study conducted among the hormonal contraceptive users from April to June 2014 at three health centers and one hospital in Harar town, Eastern Ethiopia (34.8%) (8). However, the present finding is lower than the findings of the study in Jimma, Southwestern Ethiopia (68.1%) (34), and South Africa (67.3%) (50). The finding of this review was also lower than the finding of a study done in Kembata, Southern Ethiopia (65.5%) (35), and Mekelle city, Northern Ethiopia (66.7%) (17). The higher prevalence of dyslipidemia might be attributed to the rapid urbanization, improved socioeconomic status, a change in the power of work, inadequate physical activity, and alteration in dietary habits.
The present study showed that the raised FBG was positively associated with dyslipidemia, which agrees with many previous studies (8, 51, 52). This might be because DM can cause a range of derangements in oxidation or reduction in lipid metabolism, which could in turn be responsible for the accumulation of lipid particles (34). We also revealed that the raised BP level was an independent predictor of dyslipidemia, which is consistent with the study conducted in Germany (52); but, the findings of this review disagree with the findings of the study reported in Iraq (53). This variation might be due to differences in the underlying disease, socio-demographic factors, genetic predisposition, dietary factors, and inadequate physical activity, which results in a higher incidence of metabolic abnormalities.
In the current review, BMI was found to have a positive association with dyslipidemia, which is consistent with many previous studies (16, 51, 54, 55). This finding is also similar to studies conducted in Ethiopia (44), Uganda (56), and Tanzania (57). However, previous works have doubted the reliability of BMI as an indicator of obesity (58, 59). Moreover, in young healthy adults, BMI and other surrogate indices of fatness, such as waist-to-height ratio and body adiposity index provide a poor prognosis of fat mass (60, 61). The observed association in other studies can be supported by the occurrence of ART associated lipodystrophy, which may be accompanied by the loss of peripheral subcutaneous fat (62). The other differences may be because the current review was conducted on participants with underlying different types of disease while other studies conducted only on patients with HIV and DM.
In our review, abdominal obesity or WC ≥ 94 cm was significantly associated with dyslipidemia, which agrees with other studies (34, 63). So far, anthropometric measurements, such as BMI, have been the most widely used instrument to measure general obesity. However, BMI does not account for the variation in body fat distribution and abdominal fat (64, 65). Excess of intra-abdominal fat causes a greater risk of morbidity than the overall fat accumulation (66). Thus, WC has been by far the best indicator of both intra-abdominal and total fat (67). Generally, altered anthropometric (68) and adipose tissue size have been associated with dyslipidemia in persons with and without HIV (69, 70). We found that the prevalence of dyslipidemia was almost equally determined by both BMI and WC. This might be due to a high chance of increasing concentrations of different lipid particles with increased BMI. Therefore, the combination of different anthropometric measurements should be used to evaluate the health condition of the patient or population.
Similar to the current review, studies conducted in South Africa (71), Cameroon (72) showed that being the female gender was not associated with a higher probability of presenting with dyslipidemia. In contrast to our review, the findings of a study done in Tanzania showed that being the female gender was an independent predictor of dyslipidemia (57). The discrepancy is more likely due to gender-related exercise, habits, and biological differences between men and women (27). Moreover, this study also found that lower odds of dyslipidemia were found among participants who have attained a lower level of education when compared with those who attended college and above. This is in agreement with other studies (41, 73). In contrast, other studies found contradicting results (74, 75). This may be due to differences in lifestyle.
In this study, sex, increased BMI, increased BP, and sedentary lifestyles were significantly associated with the increased risk of dyslipidemia. As all are modifiable risk factors except sex, it is essential to deliver behavioral interventions on the identified risk factors. Dietary modification is one of the most important lifestyle changes that have been shown to significantly decrease the risk of CVD. The CVD burden is reduced by optimum diet through the replacement of unprocessed meat with low saturated fat, animal proteins, and plant proteins (76). The recommended Dietary Reference Intake (DRI) daily allowance in men aged 19–50 years is 38 g/day and women aged 19–50 years is 25 g/days, and for men aged >51 is 31 g/day and women aged >51 is 21 g/day. The recommendation for children aged 1–3 is 19 g/day and aged 4–8 is 25 g/day. For boys, aged 9–13, the DRI recommendations are 31 g/day and 38 g/days for aged 14–18. For girls aged 9–18, the DRI recommendations are 26 g/day (77). Individuals are also recommended to intake fruit and vegetables at least 5 servings per day, perform moderate activity for more than 150 min/week, do vigorous physical activity for at least 75 min/ week, reduce salt and sugar intake to prevent dyslipidemia (2).
Limitations
The limitations of this systematic review with meta-analysis include some studies did not have enough predictor variables to adequately determine the degree of its prediction, However, attempts were made to include all other potential variables that occurred across the identified database. Another limitation of this study was that we did not identify the association between diet and dyslipidemia because we could not retrieve data from original articles. To overcome such problems, important discussions were made concerning the quantity and quality of daily intake. It might also lack continental representativeness since no information was found from northern regions of the African continent. But, the demographic characteristics of the population in the North African region were almost similar to the rest of the other regions.
Conclusion
This review exposed that dyslipidemia is relatively high among study participants. The prevalence of dyslipidemia was the highest among the category of studies conducted on a group of communicable diseases and the general population. On the other hand, the lowest prevalence of dyslipidemia was reported among studies conducted on a group of infectious diseases. In addition, the highest prevalence of dyslipidemia was found among studies from East Africa and Southern Africa. The independent predictors of dyslipidemia were BMI >25.0 kg/m2, raised FBG, raised BP, and WC > 94 cm. In addition, sex, medication adherence, educational status, FBG, BP, BMI, WC, marital status, and sedentary life were strongly associated with dyslipidemia. There should also be a pressing public health measure to prevent, identify, and treat dyslipidemia with a special emphasis on obese, diabetic, and hypertensive patients. Future researchers were recommended to address the association between diet and dyslipidemia.
Data Availability Statement
The original contributions presented in the study are included in the article/Supplementary Material, further inquiries can be directed to the corresponding author/s.
Author Contributions
MO and GA were involved in the selection of study, data extraction, quality assessment, statistical analysis, results interpretation, and writing the initial and final drafts of the manuscript. GAA, BW, TT, NAw, NAy, YH, and BJ were involved in data extraction, quality assessment, statistical analysis, and writing drafts of the manuscript. All authors read and approved the final manuscript, contributed to the article, and approved the submitted version.
Conflict of Interest
The authors declare that the research was conducted in the absence of any commercial or financial relationships that could be construed as a potential conflict of interest.
Publisher's Note
All claims expressed in this article are solely those of the authors and do not necessarily represent those of their affiliated organizations, or those of the publisher, the editors and the reviewers. Any product that may be evaluated in this article, or claim that may be made by its manufacturer, is not guaranteed or endorsed by the publisher.
Acknowledgments
We would like to thank all authors of included articles.
Supplementary Material
The Supplementary Material for this article can be found online at: https://www.frontiersin.org/articles/10.3389/fcvm.2021.778891/full#supplementary-material
Abbreviations
CI, confidence interval; HIV, human immune deficiency virus; HTN, hypertension; OR, odds ratio.
References
1. De Backer G, Jankowski P, Kotseva K, Mirrakhimov E, Reiner Ž, Rydén L, et al. Management of dyslipidaemia in patients with coronary heart disease: results from the ESC-EORP EUROASPIRE V survey in 27 countries. Atherosclerosis. (2019) 285:135–46. doi: 10.1016/j.atherosclerosis.2019.03.014
2. Tilahun H, Masyuko SJ, Mogaka JN, Temu T, Kinuthia J, Osoti AO, et al. Prevalence and correlates of dyslipidemia in HIV positive and negative adults in Western Kenya: a cross-sectional study. Medicine. (2021) 100:e24800. doi: 10.1097/MD.0000000000024800
3. Ríos-González BE, Luévano-Ortega KE, Saldaña-Cruz AM, González-García JR, Magaña-Torres MT. Polymorphisms of seven genes involved in lipid metabolism in an unselected Mexican population. J Genet. (2011) 90:1–6. doi: 10.1007/s12041-011-0118-2
4. Chang M-h, Yesupriya A, Ned RM, Mueller PW, Dowling NF. Genetic variants associated with fasting blood lipids in the US population: third national health and nutrition examination survey. BMC Med Genet. (2010) 11:62. doi: 10.1186/1471-2350-11-62
5. Leren TP, Berge KE. Subjects with molecularly defined familial hypercholesterolemia or familial defective apoB-100 are not being adequately treated. PLoS ONE. (2011) 6:e16721. doi: 10.1371/journal.pone.0016721
6. Thompson PD, Myerburg RJ, Levine BD, Udelson JE, Kovacs RJ. Eligibility and disqualification recommendations for competitive athletes with cardiovascular abnormalities: task force 8: coronary artery disease: a scientific statement from the American Heart Association and American College of Cardiology. Circulation. (2015) 132:e310–4. doi: 10.1161/CIR.0000000000000244
7. Janhäll S, Olofson KFG, Andersson PU, Pettersson JB, Hallquist M. Evolution of the urban aerosol during winter temperature inversion episodes. Atmosph Environ. (2006) 40:5355–66. doi: 10.1016/j.atmosenv.2006.04.051
8. Sufa B, Abebe G, Cheneke W. Dyslipidemia and associated factors among women using hormonal contraceptives in Harar town, Eastern Ethiopia. BMC Res Notes. (2019) 12:120. doi: 10.1186/s13104-019-4148-9
9. Gouda HN, Charlson F, Sorsdahl K, Ahmadzada S, Ferrari AJ, Erskine H, et al. Burden of non-communicable diseases in sub-Saharan Africa, 1990–2017: results from the Global Burden of Disease Study 2017. Lancet Glob Health. (2019) 7:e1375–87. doi: 10.1016/S2214-109X(19)30374-2
10. Ellis R. Infection and coronary heart disease. J Med Microbiol. (1997) 46:535–9. doi: 10.1099/00222615-46-7-535
11. Tripathy JP, Thakur J, Jeet G, Chawla S, Jain S, Pal A, et al. Burden and risk factors of dyslipidemia-results from a STEPS survey in Punjab India. Diabetes Metab Syndr. (2017) 11:S21–7. doi: 10.1016/j.dsx.2016.08.015
12. Tsai F-J, Cheng C-F, Lai C-H, Wu Y-C, Ho M-W, Wang J-H, et al. Effect of antiretroviral therapy use and adherence on the risk of hyperlipidemia among HIV-infected patients, in the highly active antiretroviral therapy era. Oncotarget. (2017) 8:106369. doi: 10.18632/oncotarget.22465
13. Gebreyes YF, Goshu DY, Geletew TK, Argefa TG, Zemedu TG, Lemu KA, et al. Prevalence of high bloodpressure, hyperglycemia, dyslipidemia, metabolic syndrome and their determinants in Ethiopia: evidences from the National NCDs STEPS Survey, 2015. PLoS ONE. (2018) 13:e0194819. doi: 10.1371/journal.pone.0194819
14. Dave JA, Levitt NS, Ross IL, Lacerda M, Maartens G, Blom D. Anti-retroviral therapy increases the prevalence of dyslipidemia in South African HIV-infected patients. PLoS ONE. (2016) 11:e0151911. doi: 10.1371/journal.pone.0151911
15. Bays HE, Toth PP, Kris-Etherton PM, Abate N, Aronne LJ, Brown WV, et al. Obesity, adiposity, and dyslipidemia: a consensus statement from the National Lipid Association. J Clin Lipidol. (2013) 7:304–83. doi: 10.1016/j.jacl.2013.04.001
16. Kemal A, Teshome MS, Ahmed M, Molla M, Malik T, Mohammed J, et al. Dyslipidemia and associated factors among adult patients on antiretroviral therapy in armed force comprehensive and specialized hospital, Addis Ababa, Ethiopia. HIV AIDS. (2020) 12:221. doi: 10.2147/HIV.S252391
17. Gebreegziabiher G, Belachew T, Mehari K, Tamiru D. Prevalence of dyslipidemia and associated risk factors among adult residents of Mekelle City, Northern Ethiopia. PLoS ONE. (2021) 16:e0243103. doi: 10.1371/journal.pone.0243103
18. Koller R, Agyemang C. Prevalence of cardiovascular disease risk factors in the gambia: a systematic review. Glob Heart. (2020) 15:42. doi: 10.5334/gh.827
19. Otemuyiwa IO, Adewusi SR. Food choice and meal consumption pattern among undergraduate students in two universities in Southwestern Nigeria. Nutr Health. (2012) 21:233–45. doi: 10.1177/0260106013510994
20. Popkin BM, Adair LS, Ng SW. Global nutrition transition and the pandemic of obesity in developing countries. Nutr Rev. (2012) 70:3–21. doi: 10.1111/j.1753-4887.2011.00456.x
21. Agyei-Mensah S, Aikins Ad-G. Epidemiological transition and the double burden of disease in Accra, Ghana. J Urban Health. (2010) 87:879–97. doi: 10.1007/s11524-010-9492-y
22. Haregu TN, Byrnes A, Singh K, Sathish T, Pasricha N, Wickramasinghe K, et al. A scoping review of non-communicable disease research capacity strengthening initiatives in low and middle-income countries. Glob Health Res Policy. (2019) 4:1–11. doi: 10.1186/s41256-019-0123-1
23. Buccheri RK, Sharifi C. Critical appraisal tools and reporting guidelines for evidence-based practice. Worldviews Evid Based Nurs. (2017) 14:463–472. doi: 10.1111/wvn.12258
24. Expert Panel on Detection E. Executive summary of the third report of the National Cholesterol Education Program (NCEP) expert panel on detection, evaluation, and treatment of high blood cholesterol in adults (adult treatment panel III). JAMA. (2001) 285:2486–97. doi: 10.1001/jama.285.19.2486
25. Scuteri A, Cunha PG, Rosei EA, Badariere J, Bekaert S, Cockcroft JR, et al. Arterial stiffness and influences of the metabolic syndrome: a cross-countries study. Atherosclerosis. (2014) 233:654–60. doi: 10.1016/j.atherosclerosis.2014.01.041
26. Alberti KG, Eckel RH, Grundy SM, Zimmet PZ, Cleeman JI, Donato KA, et al. Harmonizing the metabolic syndrome: a joint interim statement of the international diabetes federation task force on epidemiology and prevention; national heart, lung, and blood institute; American heart association; world heart federation; international atherosclerosis society; and international association for the study of obesity. Circulation. (2009) 120:1640–5. doi: 10.1161/CIRCULATIONAHA.109.192644
27. World Health Organization. Body Mass Index—BMI 2020. Nutrition and food security [Google Scholar] (2020).
28. Alberti KGMM, Zimmet P, Shaw J. Metabolic syndrome—a new world-wide definition. A consensus statement from the international diabetes federation. Diab Med. (2006) 23:469–80. doi: 10.1111/j.1464-5491.2006.01858.x
29. Barendregt JJ, Doi SA, Lee YY, Norman RE, Vos T. Meta-analysis of prevalence. J Epidemiol Community Health. (2013) 67:974–8. doi: 10.1136/jech-2013-203104
30. Okpala IC, Akinboro AO, Ezejoifor IO, Onunu AN, Okwara BU. Metabolic syndrome and dyslipidemia among Nigerians with lichen planus: a cross-sectional study. Indian J Dermatol. (2019) 64:303. doi: 10.4103/ijd.IJD_111_18
31. Jamieson L, Evans D, Brennan A, Moyo F, Spencer D, Mahomed K, et al. Changes in elevated cholesterol in the era of tenofovir in South Africa: risk factors, clinical management and outcomes. HIV Med. (2017) 18:595–603. doi: 10.1111/hiv.12495
32. Kiplagat SV, Lydia K, Jemimah K, Drusilla M. Prevalence of dyslipidemia and the associated factors among Type 2 diabetes patients in Turbo Sub-County, Kenya. J Endocrinol Diab. (2017) 4:1–9. doi: 10.15226/2374-6890/4/5/00190
33. Abdu A, Cheneke W, Adem M, Belete R, Getachew A. Dyslipidemia and associated factors among patients suspected to have helicobacter pylori infection at Jimma University Medical Center, Jimma, Ethiopia. Int J Gen Med. (2020) 13:311. doi: 10.2147/IJGM.S243848
34. Haile K, Timerga A. Dyslipidemia and its associated risk factors among adult type-2 diabetic patients at Jimma University Medical Center, Jimma, Southwest Ethiopia. Diab Metab Syndrome Obes Targets Ther. (2020) 13:4589. doi: 10.2147/DMSO.S283171
35. Bekele S, Yohannes T, Mohammed AE. Dyslipidemia and associated factors among diabetic patients attending Durame General Hospital in Southern Nations, Nationalities, and People's Region. Diab Metab Syndrome Obes Targets Ther. (2017) 10:265. doi: 10.2147/DMSO.S135064
36. Yusuf S, Tijjani U, Maiyaki M, Nashabaru I, Uloko A, Gezawa I. Dyslipidemia: Prevalence and associated risk factors among patients with Lichen Planus in Kano, North-West Nigeria. Nig Q J Hosp Med. (2015) 25:145–50.
37. Fikremariam T. Evaluation of Dyslipidemia, Nutritional Status and Other Associated Factors Among Diabetic Mellitus Patients at Felege Hiwot Referral Hospital. Bahir Dar (2020).
38. Ditorguéna WB, Guy B-eE, Apélété AY, Francis DS, Borgatia A, Souleymane P, et al. Profile and prevalence of dyslipidemia in workplace in Togo. J Health Environ Res. (2019) 5:50–3. doi: 10.11648/j.jher.20190502.13
39. Amberbir A, Singano V, Matengeni A, Ismail Z, Kawalazira G, Chan AK, et al. Dyslipidemia among rural and urban HIV patients in south-east Malawi. PLoS ONE. (2018) 13:e0197728. doi: 10.1371/journal.pone.0197728
40. Ciccacci F, Majid N, Petrolati S, Agy M, Massango C, Orlando S, et al. Hypercholesterolemia and related risk factors in a cohort of patients with diabetes and hypertension in Maputo, Mozambique. Pan Afr Med J. (2021) 38:102. doi: 10.11604/pamj.2021.38.102.27284
41. Hamooya BM, Mulenga LB, Masenga SK, Fwemba I, Chirwa L, Siwingwa M, et al. Metabolic syndrome in Zambian adults with human immunodeficiency virus on antiretroviral therapy: Prevalence and associated factors. Medicine. (2021) 100:e25236. doi: 10.1097/MD.0000000000025236
42. Doupa D, Mbengue AS, Diallo FA, Jobe M, Ndiaye A, Kane A, et al. Lipid profile frequency and the prevalence of dyslipidaemia from biochemical tests at Saint Louis University Hospital in Senegal. Pan Afr Med J. (2014) 17:75. doi: 10.11604/pamj.2014.17.75.3577
43. Asiki G, Murphy GA, Baisley K, Nsubuga RN, Karabarinde A, Newton R, et al. Prevalence of dyslipidaemia and associated risk factors in a rural population in South-Western Uganda: a community based survey. PLoS ONE. (2015) 10:e0126166. doi: 10.1371/journal.pone.0126166
44. Tadewos A, Addis Z, Ambachew H, Banerjee S. Prevalence of dyslipidemia among HIV-infected patients using first-line highly active antiretroviral therapy in Southern Ethiopia: a cross-sectional comparative group study. AIDS Res Ther. (2012) 9:31. doi: 10.1186/1742-6405-9-31
45. Ayoade OG, Umoh I, Amadi C. Dyslipidemia and associated risk factors among Nigerians with hypertension. Dubai Med J. (2020) 3:155–61. doi: 10.1159/000509570
46. Pitso L, Mofokeng TRP, Nel R. Dyslipidaemia pattern and prevalence among type 2 diabetes mellitus patients on lipid-lowering therapy at a tertiary hospital in central South Africa. BMC Endocr Disord. (2021) 21:1–10. doi: 10.1186/s12902-021-00813-7
47. Innes S, Abdullah KL, Haubrich R, Cotton MF, Browne SH. High prevalence of dyslipidemia and insulin resistance in HIV-infected pre-pubertal African children on antiretroviral therapy. Pediatr Infect Dis J. (2016) 35:e1. doi: 10.1097/INF.0000000000000927
48. Wang X, Guo H, Li Y, Wang H, He J, Mu L, et al. Interactions among genes involved in reverse cholesterol transport and in the response to environmental factors in dyslipidemia in subjects from the Xinjiang rural area. PLoS ONE. (2018) 13:e0196042. doi: 10.1371/journal.pone.0196042
49. Chen SN, Cilingiroglu M, Todd J, Lombardi R, Willerson JT, Gotto AM, et al. Candidate genetic analysis of plasma high-density lipoprotein-cholesterol and severity of coronary atherosclerosis. BMC Med Genet. (2009) 10:111. doi: 10.1186/1471-2350-10-111
50. Reiger S, Jardim TV, Abrahams-Gessel S, Crowther NJ, Wade A, Gomez-Olive FX, et al. Awareness, treatment, and control of dyslipidemia in rural South Africa: the HAALSI (Health and Aging in Africa: a Longitudinal Study of an INDEPTH Community in South Africa) study. PLoS ONE. (2017) 12:e0187347. doi: 10.1371/journal.pone.0187347
51. Wang S, Xu L, Jonas JB, You QS, Wang YX, Yang H. Prevalence and associated factors of dyslipidemia in the adult Chinese population. PLoS ONE. (2011) 6:e17326. doi: 10.1371/journal.pone.0017326
52. Du Y, Rosner BM, Knopf H, Schwarz S, Dören M, Scheidt-Nave C. Hormonal contraceptive use among adolescent girls in Germany in relation to health behavior and biological cardiovascular risk factors. J Adolesc Health. (2011) 48:331–7. doi: 10.1016/j.jadohealth.2011.01.004
53. Abdel Barry J, Flaf M, Al Namaa L, Hassan N. Lipoprotein changes in women taking low-dose combined oral contraceptive pills: a cross-sectional study in Basra, Iraq. East Mediterr Health J. 17:684–8, (2011) (2011). doi: 10.26719/2011.17.9.684
54. Lumu W, Kampiire L, Akabwai GP, Ssekitoleko R, Kiggundu DS, Kibirige D. Dyslipidaemia in a Black African diabetic population: burden, pattern and predictors. BMC Res Notes. (2017) 10:587. doi: 10.1186/s13104-017-2916-y
55. Pan J, Ren Z, Li W, Wei Z, Rao H, Ren H, et al. Prevalence of hyperlipidemia in Shanxi Province, China and application of Bayesian networks to analyse its related factors. Sci Rep. (2018) 8:1–9. doi: 10.1038/s41598-018-22167-2
56. Kazooba P, Kasamba I, Mayanja BN, Lutaakome J, Namakoola I, Salome T, et al. Cardiometabolic risk among HIV-POSITIVE Ugandan adults: prevalence, predictors and effect of long-term antiretroviral therapy. Pan Afr Med J. (2017) 27:40. doi: 10.11604/pamj.2017.27.40.9840
57. Ombeni W, Kamuhabwa AR. Lipid profile in HIV-infected patients using first-line antiretroviral drugs. J Int Assoc Providers AIDS Care. (2016) 15:164–71. doi: 10.1177/2325957415614642
58. Heo M, Faith MS, Pietrobelli A, Heymsfield SB. Percentage of body fat cutoffs by sex, age, and race-ethnicity in the US adult population from NHANES 1999–2004. Am J Clin Nutr. (2012) 95:594–602. doi: 10.3945/ajcn.111.025171
59. Shah NR, Braverman ER. Measuring adiposity in patients: the utility of body mass index (BMI), percent body fat, and leptin. PLoS ONE. (2012) 7:e33308. doi: 10.1371/journal.pone.0033308
60. Zaccagni L, Barbieri D, Gualdi-Russo E. Body composition and physical activity in Italian university students. J Transl Med. (2014) 12:1–9. doi: 10.1186/1479-5876-12-120
61. Heymsfield SB, Scherzer R, Pietrobelli A, Lewis CE, Grunfeld C. Body mass index as a phenotypic expression of adiposity: quantitative contribution of muscularity in a population-based sample. Int J Obes. (2009) 33:1363–73. doi: 10.1038/ijo.2009.184
62. Liu E, Armstrong C, Spiegelman D, Chalamilla G, Njelekela M, Hawkins C, et al. First-line antiretroviral therapy and changes in lipid levels over 3 years among HIV-infected adults in Tanzania. Clin Infect Dis. (2013) 56:1820–8. doi: 10.1093/cid/cit120
63. Katz EG, Stevens J, Truesdale KP, Cai J, Adair LS, North KE. Hip circumference and incident metabolic risk factors in Chinese men and women: the People's Republic of China study. Metab Syndrome Relat Disord. (2011) 9:55–62. doi: 10.1089/met.2010.0045
64. World Health Organization. Physical status: The use of and interpretation of anthropometry, Report of a WHO Expert Committee. World Health Organization (1995).
65. Dalton M, Cameron AJ, Zimmet PZ, Shaw JE, Jolley D, Dunstan DW, et al. Waist circumference, waist–hip ratio and body mass index and their correlation with cardiovascular disease risk factors in Australian adults. J Intern Med. (2003) 254:555–63. doi: 10.1111/j.1365-2796.2003.01229.x
66. Visscher T, Kromhout D, Seidell J. Long-term and recent time trends in the prevalence of obesity among Dutch men and women. Int J Obes. (2002) 26:1218–24. doi: 10.1038/sj.ijo.0802016
67. Lemieux S, Prud'homme D, Bouchard C, Tremblay A, Després J-P. A single threshold value of waist girth identifies normal-weight and overweight subjects with excess visceral adipose tissue. Am J Clin Nutr. (1996) 64:685–93. doi: 10.1093/ajcn/64.5.685
68. Yang YJ, Park H-J, Won K-B, Chang H-J, Park G-M, Kim Y-G, et al. Relationship between the optimal cut-off values of anthropometric indices for predicting metabolic syndrome and carotid intima-medial thickness in a Korean population. Medicine. (2019) 98:e17620. doi: 10.1097/MD.0000000000017620
69. Kahn CR, Wang G, Lee KY. Altered adipose tissue and adipocyte function in the pathogenesis of metabolic syndrome. J Clin Invest. (2019) 129:3990–4000. doi: 10.1172/JCI129187
70. Gadekar T, Dudeja P, Basu I, Vashisht S, Mukherji S. Correlation of visceral body fat with waist–hip ratio, waist circumference and body mass index in healthy adults: a cross sectional study. Med J Armed Forces India. (2020) 76:41–6. doi: 10.1016/j.mjafi.2017.12.001
71. Guira O, Tiéno H, Diendéré AE, Sagna Y, Diallo I, Yaméogo B, et al. Features of metabolic syndrome and its associated factors during highly active antiretroviral therapy in Ouagadougou (Burkina Faso). J Int Assoc Providers AIDS Care. (2016) 15:159–63. doi: 10.1177/2325957415601503
72. Worm SW, Friis-Møller N, Bruyand M, Monforte ADA, Rickenbach M, Reiss P, et al. High prevalence of the metabolic syndrome in HIV-infected patients: impact of different definitions of the metabolic syndrome. AIDS. (2010) 24:427–35. doi: 10.1097/QAD.0b013e328334344e
73. Dinsa GD, Goryakin Y, Fumagalli E, Suhrcke M. Obesity and socioeconomic status in developing countries: a systematic review. Obes Rev. (2012) 13:1067–79. doi: 10.1111/j.1467-789X.2012.01017.x
74. Bune GT, Yalew AW, Kumie A. Predictors of metabolic syndrome among people living with HIV in Gedeo-Zone, Southern-Ethiopia: a case–control study. HIV AIDS. (2020) 12:535. doi: 10.2147/HIV.S275283
75. Gebrie A, Sisay M, Gebru T. Dyslipidemia in HIV/AIDS infected patients on follow up at referral hospitals of Northwest Ethiopia: a laboratory-based cross-sectional study. Obes Med. (2020) 18:100217. doi: 10.1016/j.obmed.2020.100217
76. Zhubi-Bakija F, Bajraktari G, Bytyçi I, Mikhailidis DP, Henein MY, Latkovskis G, et al. The impact of type of dietary protein, animal versus vegetable, in modifying cardiometabolic risk factors: a position paper from the International Lipid Expert Panel (ILEP). Clin Nutr. (2021) 40:255–76. doi: 10.1016/j.clnu.2020.05.017
Keywords: dyslipidemia, risk factors, lipid profile, Africa, abnormal lipid metabolism, metabolic syndrome, non-communicable disease, hypercholesterolemia
Citation: Obsa MS, Ataro G, Awoke N, Jemal B, Tilahun T, Ayalew N, Woldegeorgis BZ, Azeze GA and Haji Y (2022) Determinants of Dyslipidemia in Africa: A Systematic Review and Meta-Analysis. Front. Cardiovasc. Med. 8:778891. doi: 10.3389/fcvm.2021.778891
Received: 17 September 2021; Accepted: 29 December 2021;
Published: 23 February 2022.
Edited by:
Changcheng Zhou, University of California, Riverside, United StatesReviewed by:
Kailash Gulshan, Cleveland State University, United StatesDemeke Belay, Debre Tabor University, Ethiopia
Copyright © 2022 Obsa, Ataro, Awoke, Jemal, Tilahun, Ayalew, Woldegeorgis, Azeze and Haji. This is an open-access article distributed under the terms of the Creative Commons Attribution License (CC BY). The use, distribution or reproduction in other forums is permitted, provided the original author(s) and the copyright owner(s) are credited and that the original publication in this journal is cited, in accordance with accepted academic practice. No use, distribution or reproduction is permitted which does not comply with these terms.
*Correspondence: Mohammed S. Obsa, bXN1bGVpbWFuNDNAZ21haWwuY29t