- 1Department of Epidemiology, School of Public Health, University of Michigan, Ann Arbor, MI, United States
- 2Department of Biostatistics, School of Public Health, University of Michigan, Ann Arbor, MI, United States
- 3Urban Health Collaborative, Drexel University, Philadelphia, PA, United States
- 4Department of Epidemiology and Biostatistics, Dornsife School of Public Health, Drexel University, Philadelphia, PA, United States
- 5Division of Cardiology, Department of Medicine, Duke University School of Medicine, Durham, NC, United States
- 6Department of Medicine, Division of Geriatrics, University of Mississippi Medical Center, Jackson, MS, United States
- 7Survey Research Center, Institute for Social Research, University of Michigan, Ann Arbor, MI, United States
Low socioeconomic status (SES) and living in a disadvantaged neighborhood are associated with poor cardiovascular health. Multiple lines of evidence have linked DNA methylation to both cardiovascular risk factors and social disadvantage indicators. However, limited research has investigated the role of DNA methylation in mediating the associations of individual- and neighborhood-level disadvantage with multiple cardiovascular risk factors in large, multi-ethnic, population-based cohorts. We examined whether disadvantage at the individual level (childhood and adult SES) and neighborhood level (summary neighborhood SES as assessed by Census data and social environment as assessed by perceptions of aesthetic quality, safety, and social cohesion) were associated with 11 cardiovascular risk factors including measures of obesity, diabetes, lipids, and hypertension in 1,154 participants from the Multi-Ethnic Study of Atherosclerosis (MESA). For significant associations, we conducted epigenome-wide mediation analysis to identify methylation sites mediating the relationship between individual/neighborhood disadvantage and cardiovascular risk factors using the JT-Comp method that assesses sparse mediation effects under a composite null hypothesis. In models adjusting for age, sex, race/ethnicity, smoking, medication use, and genetic principal components of ancestry, epigenetic mediation was detected for the associations of adult SES with body mass index (BMI), insulin, and high-density lipoprotein cholesterol (HDL-C), as well as for the association between neighborhood socioeconomic disadvantage and HDL-C at FDR q < 0.05. The 410 CpG mediators identified for the SES-BMI association were enriched for CpGs associated with gene expression (expression quantitative trait methylation loci, or eQTMs), and corresponding genes were enriched in antigen processing and presentation pathways. For cardiovascular risk factors other than BMI, most of the epigenetic mediators lost significance after controlling for BMI. However, 43 methylation sites showed evidence of mediating the neighborhood socioeconomic disadvantage and HDL-C association after BMI adjustment. The identified mediators were enriched for eQTMs, and corresponding genes were enriched in inflammatory and apoptotic pathways. Our findings support the hypothesis that DNA methylation acts as a mediator between individual- and neighborhood-level disadvantage and cardiovascular risk factors, and shed light on the potential underlying epigenetic pathways. Future studies are needed to fully elucidate the biological mechanisms that link social disadvantage to poor cardiovascular health.
Introduction
Associations of individual-level socioeconomic adversity with increased risk of cardiovascular disease (CVD) are well established. The effects of socioeconomic adversity are thought to accumulate throughout a person's lifespan, as both childhood and adulthood socioeconomic status (SES) can influence CVD and its risk factors, including obesity (1–3), hypertension (4–6), diabetes (7, 8), and dyslipidemia (9–12) at a later stage (13). There is also increasing evidence suggesting that neighborhood-level social features are associated with CVD outcomes and risk factors, independent of individual-level SES (14–18). For example, in the Multi-Ethnic Study of Atherosclerosis (MESA), better neighborhood physical environment was associated with decreased body mass index (BMI) (19), higher availability of healthy food was associated with lower rates of hypertension (20), and neighborhoods with more healthy food and physical activity resources were associated with lower incidence of type 2 diabetes (21). Finally, ongoing exposure to a range of neighborhood characteristics including favorable food stores, more physical activity resources, better walking/physical activity environment, and higher neighborhood socioeconomic status was associated with higher odds of having an ideal cardiovascular health score in MESA (22).
Previous studies have proposed that epigenetic changes, which are important regulators of gene expression (23) that do not alter the underlying DNA sequence, might be a potential mechanism linking individual- and neighborhood-level social exposures to disease risk (24–27). Epigenetic mechanisms such as DNA methylation can change throughout the life course under the influence of environmental stimuli. In fact, epigenetic variation has been linked to several indicators of social disadvantage such as low childhood SES (28–32), low adult SES (29, 32), and living in disadvantaged neighborhoods (26, 33, 34). In MESA, three measures of SES (childhood SES, adult SES, and SES trajectories from childhood to adulthood) were associated with DNA methylation levels in genes related to stress reactivity and inflammation (29). Similarly, after adjusting for individual SES, neighborhood socioeconomic disadvantage and neighborhood social environment were also associated with DNA methylation in stress- and inflammation-related genes (33).
DNA methylation has also been shown to play a critical role in shaping CVD risk. Both global DNA methylation (35–37) and specific DNA methylation changes within certain candidate genes (38–40) have been associated with CVD or CVD-related biomarkers. More recently, large-scale epigenome-wide association studies have shown that methylation levels at individual CpG sites are associated with CVD (41–44) and its risk factors including measures of adiposity (45), diabetes (46–48), blood pressure (49), and lipid traits (50).
On these grounds, we hypothesized that DNA methylation acts as a mediator of at least some of the relationship between individual- and neighborhood-level social disadvantage and CVD risk factors. To date, only a few studies have formally examined whether epigenetic markers across the genome mediate the effects of social disadvantage on CVD risk factors. For instance, in adipose tissue from participants in the New England Family Study, epigenetic markers were found to mediate the association between two measures of individual-level social disadvantage (childhood SES and a broader construct of early life social disadvantage) and BMI in adulthood (51, 52). However, the sample size in these analyses was relatively small (N < 150 participants). To our knowledge, no studies have conducted mediation analysis for the association between neighborhood characteristics and CVD risk factors. Therefore, epigenome-wide studies with larger sample sizes are critically needed to comprehensively examine biological pathways linking individual- and neighborhood-level social disadvantage to CVD risk factors.
To fill this gap, we conducted formal mediation analysis to further examine the relationship between individual- and neighborhood-level social disadvantage, DNA methylation, and 11 CVD risk factors in a large, multi-ethnic, population-based sample of US adults using epigenome-wide methylation data measured from purified monocytes. We examined methylation in monocytes because they and their derived cells (i.e., macrophages and foam cells) play a crucial role in inflammation and contribute significantly to atherosclerosis, a primary cause of CVD. We then characterized the functional elements near the mediating methylation sites, examined their overlap with previously identified expression quantitative trait methylation (eQTM) sites, and tested for enrichment of biological pathways to investigate the functional role of the identified loci.
Materials and Methods
Study Population
The Multi-Ethnic Study of Atherosclerosis (MESA) is a population-based longitudinal study designed to identify risk factors for the progression of subclinical CVD (53). Between July 2000 and August 2002, a total of 6,814 non-Hispanic white, African American, Hispanic, and Chinese-American participants between the ages of 45 and 84 years were recruited from six study sites in the US, including Forsyth County, NC; Northern Manhattan and the Bronx, NY; Baltimore City and Baltimore Country, MD; St. Paul, MN; Chicago, IL; and Los Angeles County, CA. Each field center recruited participants from locally available sources, including lists of residents, lists of dwellings, and telephone exchanges. Between April 2010 and February 2012 (during MESA Exam 5), DNA methylation was measured in a random subsample of 1,264 non-Hispanic White, African American, and Hispanic MESA participants aged 55–94 years from four of the six study centers (Baltimore, MD; Forsyth County, NC; New York, NY; and St. Paul, MN). After excluding respondents with missing data on one or more variables, we focused on a subset of 1,154 individuals that had exposure, covariate and DNA methylation data for our final analyses. This study was approved by the Institutional Review Boards of all MESA field centers, the MESA Coordinating Center, and the University of Michigan.
Measures
DNA Methylation
Detailed description of DNA methylation profiling and data processing procedures can be found in Liu et al. (54). Briefly, blood was drawn in the morning after a 12 h fast. Monocytes were purified using Auto-MACs automated magnetic separation units (Miltenyi Biotec, Bergisch Gladbach, Germany). A random assignment technique was used to plate the samples in order to mitigate bias due to batch, chip, and position effects. Methylation profile was measured using the Illumina HumanMethylation450 Beadchip. Bead-level methylation data were summarized using Illumina GenomeStudio. The lumi package for R with default settings was used for quantile normalization (55). Checks for sex and race/ethnicity mismatches and outlier identification were performed using multidimensional scaling plots. Criteria for probe elimination included: “detected” methylation levels in <90% of MESA samples (detection p-value cut-off = 0.05), existence of a SNP within 10 base pairs of the target CpG site, and overlap with a non-unique region as suggested by DMRcate (56). In addition, 65 probes that measured single nucleotide polymorphisms (SNPs) rather than methylation were excluded. After these eliminations, we had a total of 403,648 autosomal probes for subsequent analyses. The raw methylation level for each site was normalized and computed as an M-value, the log ratio of methylated to unmethylated signal intensity. Even though the target cell type was monocytes, there were still some residual components from other cell types in the samples. Thus, prior to analysis, each methylation site was adjusted by regressing the methylation M-value on the estimated cell proportions of residual non-monocytes (neutrophils, B cells, T cells, and natural killer cells) and adding methylation chip and position as random effects to account for any sample contamination and batch effects. Information on the nearest gene and associated genomic features of the CpG sites were from Illumina-provided annotation files (57).
Socioeconomic Status
Maternal educational attainment was used as an indicator of childhood SES (58). At Exam 2, respondents reported the highest level of education that their mother had completed. Available response options were no school, high school degree, some college but no degree, college degree, and graduate or professional school. We created a binary variable for maternal education (less than high school = 1; high school degree or more = 0).
Adult SES was indicated by the highest level of educational attainment of the respondent, which was reported at Exam 1. Available response options were no school, grades 1–8, grades 9–11, completed high school or GED, some college but no degree, technical school certificate, associated degree, bachelor's degree, and graduate or professional school. We created a dummy variable for respondent educational attainment (less than college = 1; college degree or more = 0).
Neighborhood Characteristics
Respondents' current home addresses were collected at each MESA examination, as well as during midpoint follow-up calls between exams. Based on the information collected, an address history between Exams 1 and 5 was created for each respondent and used to construct two summary scores for neighborhood exposures (see Section Supplementary Methods in Supplementary Material for additional details).
Briefly, a neighborhood socioeconomic disadvantage score was created based on the first principal component (PC) from a principal components analysis (PCA) of 16 census-tract level variables reflecting dimensions of education, occupation, income and wealth, poverty, employment, and housing from the 2000 US Census, American Community Survey 2005–2009, and American Community Survey 2007–2011. Weighted scales were created by multiplying the PC weights by the standardized variables. The first PC was highly weighted for education, occupation, and income and wealth.
For neighborhood social environment, we assessed three neighborhood dimensions collected in 2003–2005 and 2010–2012: aesthetic quality, safety, and social cohesion. Information was obtained from questionnaires administered to MESA participants and to a random auxiliary sample of other neighborhood residents in the New York, Baltimore, and Forsyth county study sites in 2003–2005 (N = 12,034) and all study sites in 2010–2012 (N = 8,553) (59). Responses of residents within the same Census tract were aggregated to create neighborhood-level measures for each of the neighborhood dimensions investigated. Conditional empirical Bayes (CEB) estimates for the three scales were constructed as a summary index, controlling for study site, respondent age and sex, and survey type (MESA or auxiliary sample). A summary social environment scale was calculated by summing the standardized CEB estimates for the three scales.
In order to assess the effects of long-term exposure to neighborhood characteristics, we used the weighted cumulative average of each of the neighborhood measures across all available MESA examinations (maximum of 5 exams, spanning 10 years from baseline to Exam 5), with the weights proportional to the number of months the respondents resided in each neighborhood. Neighborhood variables were coded so that higher values indicate greater disadvantage or less desirable social environment.
CVD Risk Factors and Medications
We examined a total of 11 CVD risk factors measured at Exam 5 including BMI, waist circumference (WC), systolic blood pressure (SBP), diastolic blood pressure (DBP), triglycerides (TG), high-density lipoprotein cholesterol (HDL-C), low-density lipoprotein cholesterol (LDL-C), total cholesterol (TC), serum glucose, serum insulin, and hemoglobin A1c (HbA1c). In MESA, blood samples were drawn after a 12-h fast. Measurement methods for the CVD risk factors have been described previously (60, 61). In our study, log-transformation was applied to TG, HDL-C, glucose, insulin, and HbA1c. Extreme outliers in the CVD risk factors, defined as values >3 times the interquartile range below the first quartile or above the third quartile, were removed from the analyses.
Information on current medications was collected using the medication inventory method in MESA (62, 63). Participants were asked to bring their medications to the clinic. The interviewers then transcribed the names, strengths, and dosage of the medications. Specifically, we collected information on lipid-lowering drugs, antihypertensives, and diabetes medications.
Statistical Analysis
Associations Between Individual SES/Neighborhood Characteristics and CVD Risk Factors
Before formally testing for mediation, we first tested whether there was a significant association (total effect) between individual and neighborhood-level disadvantage (exposures) and CVD risk factors (outcomes). The associations between each exposure and outcome were examined separately. Model 1a tested associations of individual-level exposures (childhood SES and adult SES) with CVD risk factors, and included adjustment for age, gender, race/ethnicity, current smoking status, medication use (as appropriate), and the first 5 genetic principal components (PCs) of ancestry. Smoking was adjusted because previous studies show that many of the CpG sites associated with measures of SES are also highly associated with smoking (64). Here, we sought to identify effects of social disadvantage that were not confounded by or mediated by smoking. Genetic PCs were included because they were necessary to control for population stratification and admixture in subsequent analytic steps (i.e., when testing for mediation of the total effect by epigenetic markers, described below). We included lipid-lowering drug use as a covariate for associations with lipid traits (TC, TG, LDL-C, and HDL-C), antihypertensive use for associations with blood pressure measurements (SBP and DBP), and use of diabetes medications for associations with glycemic traits (serum glucose, insulin, and HbA1c). Model 1b tested associations of neighborhood-level exposures (neighborhood socioeconomic disadvantage and neighborhood social environment) with CVD outcomes and included all covariates in Model 1a as well as childhood SES and adult SES. P-values were controlled for multiple testing using Bonferroni correction (Bonferroni-corrected p < 0.0011, accounting for 44 tests from 11 CVD risk factors and 4 exposures).
For the significant associations in Models 1a/1b where BMI was not the dependent variable, we further adjusted for BMI (Models 2a/2b). BMI is often included in adjustment models or sensitivity analyses when studying the effect of methylation on CVD risk factors (46–50), because it may causally influence methylation at multiple loci (65). BMI may also be on the mediating pathway between social disadvantage and CVD risk factors. Thus, we wanted to explore associations between social disadvantage and CVD risk factors both before and after controlling for this potential source of confounding. Significant associations with BMI in Models 1a/1b (Bonferroni-corrected p < 0.0011), and significant associations with other CVD risk factors in Models 1a/1b (p < 0.0011) that remained associated at p < 0.05 after adjusting for BMI (Models 2a/2b) were selected for mediation analysis.
Mediation Analysis
If a significant association (total effect) was identified between an individual SES/neighborhood measure (exposure) and a CVD risk factor (outcome), we conducted epigenome-wide mediation analysis to identify specific CpG sites whose methylation may mediate at least some of the effect of the exposure on the corresponding outcome, using a cross product-based mediation approach where the mediation effect can be defined as the product of the exposure-mediator effect and the mediator-outcome effect. To obtain these two parameters for a given outcome and exposure, we used linear regression models to separately estimate the association of individual- and neighborhood-level social disadvantage with DNA methylation (the mediator) while adjusting for covariates (Equation 1), and the association of DNA methylation with the CVD risk factor while controlling for the corresponding exposure (i.e., individual or neighborhood social measure) and the same set of covariates (Equation 2). The covariates included in the regression models were the same as the covariates used in Models 1a/1b or 2a/2b to test the total effect of the exposure on the outcome. Model specification for a given outcome and exposure is provided below:
Mi: DNA methylation (M-value) for individual i.
Ai: exposure (individual SES or neighborhood characteristic) for individual i.
Ci: adjustment covariates for individual i.
Yi: CVD risk factor for individual i.
Using the models described above, the epigenetic mediation effect can be quantified by testing:
The null hypothesis is therefore comprised of three sub-null hypotheses: (1) αa = 0, βm≠0; (2) αa≠0, βm = 0; (3) αa = 0, βm = 0. To test the mediation effect, we used the JT-Comp method (66) implemented in R, which takes into account the composite nature of the null hypothesis and provides well-calibrated p-values under the null. False discovery rate (FDR) was calculated from the mediation p-values to control for multiple testing. We considered a CpG site to be a significant mediator of the association between the exposure and outcome pair if it had FDR q < 0.05. The proportion of the total effect that was mediated by each CpG site (indirect effect) was then computed by dividing the mediation effect by the total effect.
In addition, since methylation levels may be correlated at CpG sites that are spatially close or within in the same biological pathway, we conducted PCA analysis on the identified CpG mediators to reduce their dimensionality. We extracted the first 10 PCs, capturing major axes of variability, so that we could investigate the overall effects of mediation across the identified CpG mediators. Next, the first 10 PCs for each set of CpG mediators were examined in mediation models. Specifically, for each association where significant epigenetic mediation was identified by the epigenome-wide mediation analysis, we further tested the meditation effect of each methylation PC separately using the mediate() function of the mediation R package (67). The average mediation effect and the proportion of the total effect explained by each methylation PC, as well as their corresponding confidence intervals (CIs), were obtained using non-parametric bootstrapping with 10,000 iterations.
Furthermore, to identify CpG sites that remain significant after adjusting for the other mediating CpG sites, we conducted a penalization-based multivariate high-dimensional mediation analysis on the identified CpG mediators using the HIMA package in R (68, 69). Specifically, for each association where significant epigenetic mediation was identified by the epigenome-wide mediation analysis, we fit a multivariate mediator-outcome model with all of the potential CpG mediators identified by the epigenome-wide mediation analysis and used a LASSO-based regression to estimate the effect of each CpG site on the outcome while controlling for the other CpG sites. We then obtained FDR q-values from the exposure-mediator model and the multivariate mediator-outcome model separately and applied the joint significance test (i.e., the maximum value among the two FDR q-values) to determine the significance of the mediation effect. We considered a CpG site to be a significant mediator when FDR q < 0.05.
As a secondary analysis, we conducted sex-stratified analysis for the association between adult SES and BMI using Model 1a and the corresponding mediation models described above. Sex-stratified analysis was warranted since effects of social factors (1, 2, 70–73) and epigenetic markers (51, 52) on BMI have been found to differ between sexes in at least some studies.
It is important to note that this mediation analysis does not necessarily establish causal pathways between social disadvantage and CVD risk factors, but rather demonstrates statistical mediation by DNA methylation using statistical criteria. Since our study has a cross-sectional design with concurrent measurement of DNA methylation and CVD risk factors, we are unable to establish temporality between the mediator and the outcome. Any CpG sites identified in the mediation analysis are therefore correlational in nature and do not necessarily infer causal mediation. Caution should be exercised in interpreting the results from the mediation analysis.
Genomic Feature Enrichment Analysis
Genomic feature enrichment analysis was performed on the set of significant CpG mediators for each exposure/outcome pair separately. We examined whether the genomic locations of each set of CpGs were enriched for features including gene promoters, enhancers, DNAse I hypersensitivity sites (DHS), CpG islands, and CpG island flanking shores/shelves. The genomic features and the target gene(s) from the UCSC database associated with each CpG site were obtained from the annotation files provided by Illumina (57). Specifically, we considered a CpG site to be in the promoter region if it was 0–1,500 bases upstream of a transcriptional start site. A CpG site was assigned to CpG island flanking shore/shelf if it was located within 4 kb of a CpG island. To test for enrichment, we used Fisher's exact test to compare the proportion of CpG sites that reside within a certain genomic feature among CpG mediators to the proportion of non-mediating CpG sites that reside within the same feature. For any contingency table that has a zero cell count, we added 1 to each of the cells to avoid having an odds ratio of zero.
Previously in MESA, Liu et al. identified 11,203 potential cis-acting regulatory CpG sites whose methylation level was associated with the expression level of any autosomal gene within 1 Mb at q < 0.001 (eQTMs) (54). In our study, we examined the overlap between the identified CpG mediators to these previously reported eQTMs. We also investigated whether CpG mediators were more likely to be eQTMs than non-mediating CpGs. Specifically, we performed Fisher's exact test to compare the proportion of eQTMs among our identified CpG mediators to the proportion of eQTMs among non-mediating CpG sites in our sample. Furthermore, Huan et al. identified 92 putatively causal CpG sites for cardiovascular risk factors including coronary heart disease, myocardial infarction, type 2 diabetes, SBP, DBP, HDL-C, LDL-C, TC, TG, and BMI by conducting Mendelian randomization analysis using cis-mQTLs and GWASs of the CVD risk factors (74). Since the CpG sites identified in the MR analysis using mQTLs as instrumental variables are potentially causal for cardiovascular risk factors, we examined the overlap between the identified mediating CpG sites and the 92 CpG sites reported in Huan et al. (74).
Gene-Set Analysis
We employed two methods to identify GO categories and KEGG pathways that may be over-represented in the identified CpG mediators. First we used the gometh() function from the missMethyl R package, which can eliminate multiple sources of bias by taking into account the number of CpG sites within a given gene and can correct for probe density bias (75). A potential limitation of this method, however, is that it maps the CpG sites to their proximal genes using chromosomal position. Yet, it is known that methylation sites do not always act on the nearest genes and might have distant regulatory effects as well. In light of this, we also conducted enrichment analysis using the enrichGO() and enrichKEGG() functions in the clusterProfiler R package (76) so that we could define the CpGs associated with gene expression using empirical data. Genes known to be associated with the CpG mediators were extracted using the methylation-gene expression pairs (eQTMs) identified previously in MESA (54), and were used as the signal gene list. All genes associated with methylation sites included in both the previous investigation and the present study were selected as the background gene list. Additionally, to ensure that any significant enrichment was not merely due to methylation sites that tend to be active in white blood cells, each CpG site was mapped to a chromatin state based on its chromosomal location using the 15-core chromatin state predicted by ChromHMM in primary mononuclear cells from peripheral blood in the Roadmap Epigenomics Project (77). Each methylation site was then classified as being in an active or inactive region depending on the predicted chromatin state. Using this information, we further restricted both the signal and background lists to only genes associated with CpG sites in an active chromatin region as a secondary analysis. GO terms and KEGG pathways with FDR q < 0.05 were considered significant for all gene-set analyses.
Results
Sample Characteristics
At Exam 5, the mean age was 69.6 years and 50.8% of the participants were female (Table 1). The sample consisted of 49.1% Non-Hispanic White, 19.4% African American, and 31.5% Hispanic participants. A total of 8.4% were current smokers, 53.4% had low childhood SES, and 66.0% had low adult SES. Neighborhood socioeconomic disadvantage ranged between −4.53 and 1.87 [mean = −0.31, standard deviation (SD) = 1.10], with a higher score indicating greater disadvantage. Neighborhood social environment ranged between −6.32 and 7.94 (mean = 0.40, SD = 2.67), with a higher score indicating a worse environment. The Pearson correlation between neighborhood socioeconomic disadvantage and neighborhood social environment was 0.26.
Association Between Individual SES/Neighborhood Conditions and CVD Risk Factors
In Model 1a, low childhood SES was associated with higher SBP after Bonferroni correction (Table 2). Low adult SES was associated with higher BMI, WC, and insulin, and with lower HDL-C. In Model 1b, neighborhood socioeconomic disadvantage was associated with higher TG and with lower HDL-C. Less desirable neighborhood social environment was not associated with any CVD risk factors after correcting for multiple testing. All of the significant results from Models 1a/1b remained significant at p < 0.05 in Models 2a/2b (after BMI adjustment), except for the association between adult SES and WC (Table 2).
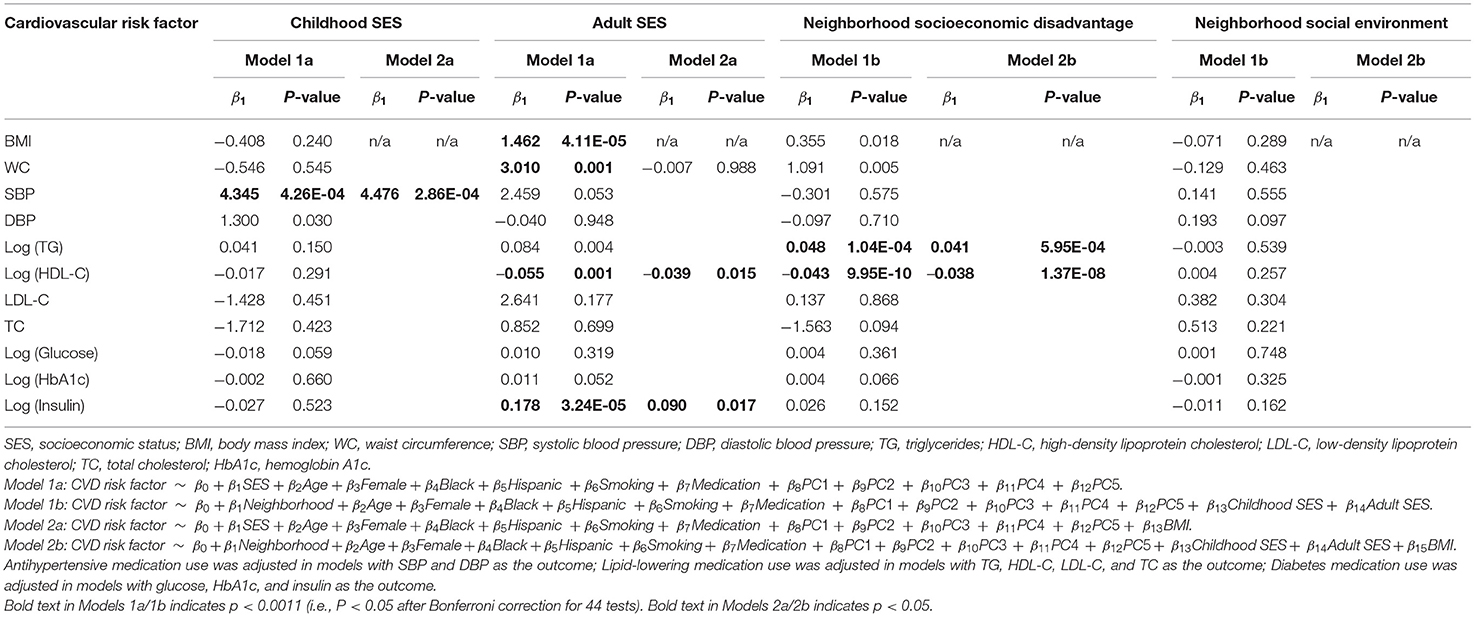
Table 2. Associations between individual SES/neighborhood characteristics and cardiovascular risk factors (N = 1,154).
Mediation Analysis
Based on the above results, we investigated epigenetic mediation of the association of childhood SES with SBP, of adult SES with BMI, HDL-C, and insulin, and of neighborhood socioeconomic disadvantage with TG and HDL-C. In Model 1a, a total of 410, 5, and 7 mediating CpG sites were identified for the associations of adult SES with BMI, HDL-C, and insulin, respectively. For the significant associations where BMI was not the dependent variable, all mediating methylation sites lost significance in Model 2a after further adjustment for BMI.
Beyond the covariates in Model 1a (age, sex, race/ethnicity, current smoking status, and the first 5 genetic PCs of ancestry), adult SES explained 1.4% of the total variability in BMI. Of this relatively small total effect of adult SES on BMI, between 7.0 and 22.0% was mediated by the 410 identified CpG loci. The complete list of summary statistics and annotations of the 410 CpG sites mediating the association between adult SES and BMI in Model 1a is provided in Supplementary Table 1, and a Manhattan plot of the mediating loci is shown in Supplementary Figure 1. PCA analysis of the 410 mediating CpGs shows that the first 10 PCs cumulatively accounted for 49.5% of the variability in the methylation levels across the 410 sites (Supplementary Figure 2). After adjusting for age, sex, race/ethnicity, smoking, and the first 5 genetic PCs of ancestry, 4 of the 10 methylation PCs (PC1, PC5, PC6, and PC8) showed significant evidence of mediation at p < 0.05, accounting for 26.6, 4.5, 11.7, and 9.8% of the total effect of adult SES on BMI, respectively (Supplementary Table 2). The cumulative proportion of the SES-BMI association mediated by these 4 independent methylation PCs was 52.6%, suggesting that the overall mediation effect across the 410 identified CpG sites explained at least 52.6% of the association between adult SES and BMI.
When modeled jointly with a LASSO-based penalty, 5 of the 410 identified CpG sites showed significant mediation effects at FDR q < 0.05, explaining 0.3, 3.4, 1.2, 1.9, and 1.2% of the association between adult SES and BMI, respectively (Supplementary Table 3). In other words, 5 CpG sites showed evidence of mediating the association between adult SES and BMI even after adjusting for the other potential mediators identified in the epigenome-wide mediation analysis. Two of the identified CpG sites (cg23983783 and cg25392060) have been previously reported as eQTMs (i.e., they are associated with the expression of nearby genes). In particular, the methylation level of cg23983783 was associated with the gene expression of N4BP2L1, which has been associated with BMI and is involved in adipocyte homeostasis (78). Another identified CpG mediator, cg06192883 in the body of myosin 5C (MYO5C), encodes a protein involved in actin-based membrane trafficking. This CpG was also identified in a recent EWAS of BMI (45) and has been associated with longitudinal changes in BMI (79).
After adjusting for age, race/ethnicity, current smoking status and the first 5 genetic PCs of ancestry, adult SES explained 0.49 and 3.5% of the variability in BMI among females and males, respectively. In sex-stratified mediation analyses for BMI, we identified 12 CpG sites mediating the association between adult SES and BMI in females and males, respectively, with FDR q < 0.05 (Supplementary Tables 4, 5). Of the CpG mediators identified in females, all sites were also identified in the pooled analysis. In contrast, two CpG mediators (cg08448711 and cg16580391) identified in males were not statistically significant in the pooled analysis. Only one CpG site, cg15633603, was common to both sexes, which was also the site with the strongest evidence for mediation in the pooled analysis. Interestingly, CpG mediators found in females explained between 29.4 and 40.0% of the effect of adult SES on BMI, whereas those identified in males explained only 11.5–15.5%, suggesting that the epigenetic mediating pathway might be more pronounced for women.
We identified 66 mediating CpG sites for the association between neighborhood socioeconomic disadvantage and HDL-C in Model 1b. Of these, 43 methylation sites showed significant evidence of mediation even after adjusting for BMI. In Model 2b (i.e., after adjusting for age, sex, race/ethnicity, smoking, lipid-lowering drug use, the first 5 genetic PCs of ancestry, childhood SES, adult SES, and BMI), the total variability in HDL-C explained by neighborhood socioeconomic disadvantage was 2.1%, of which between 5.9 and 8.7% could be attributed to each of the 43 CpG mediators. A complete list of summary statistics and annotations of the 43 CpG sites mediating the effects of neighborhood socioeconomic disadvantage on HDL-C is shown in Supplementary Table 6, and a Manhattan plot of the mediating loci is shown in Supplementary Figure 3. As previously described, we conducted PCA on these CpG mediators to characterize the overall mediation effect across the loci. Supplementary Table 7 presents the independent mediation effects of the first 10 methylation PCs, which cumulatively accounted for 66.0% of the variability in the 43 CpG sites mediating the association between neighborhood socioeconomic disadvantage and HDL-C (Supplementary Figure 2). After controlling for the same Model 2b covariates as above, methylation PC1 and PC9 showed significant evidence of mediation (p < 0.05), accounting for 17.8 and 5.5% of the relationship between neighborhood socioeconomic disadvantage and HDL-C, respectively. The cumulative proportion mediated by these two uncorrelated methylation PCs was 23.3%, suggesting that the overall mediation effect across the 43 identified CpG sites explained at least 23.3% of the association between neighborhood socioeconomic disadvantage and HDL-C.
When modeled jointly with a LASSO-based penalty, 3 of the 43 identified CpG sites showed significant mediation effects at FDR q < 0.05 (Supplementary Table 8). The 3 identified CpG mediators each explained 1.3, 0.7, and 1.1% of the association between neighborhood socioeconomic disadvantage and HDL-C, respectively (Supplementary Table 8). In particular, cg18680181 is located in the body of KIAA0391, which forms an evolutionarily conserved cluster with PSMA6 and has been reported to predispose individuals to coronary artery disease (80). In addition, haplotypes in the chromosomal region encompassing KIAA0391 and PSMA6 have been linked to coronary artery disease and myocardial infarction (81). Another CpG mediator, cg2475272, resides near SETD7, a lysine methyltransferase that methylates a wide range of targets and may play an important role in several biological processes including metabolism and immunity (82). For example, SETD7 might be involved in lipid, cholesterol, and glucose metabolism through its methylation of Farnesoid X receptor (FXR) (82).
No methylation sites showed evidence of mediation at FDR q < 0.05 for the associations between childhood SES and SBP, and between neighborhood socioeconomic disadvantage and TG.
Genomic Feature Enrichment Analysis
We performed bioinformatic analysis for the 410 CpG mediators of the association between adult SES and BMI and the 43 CpG mediators of the association between neighborhood socioeconomic disadvantage and HDL-C identified using JT-Comp. The genomic feature enrichment results are shown in Table 3. Bonferroni correction accounted for testing 5 genomic features and eQTMs, described below (Bonferroni-corrected p < 0.0083). Compared to non-mediating methylation sites, CpG loci mediating the association between adult SES and BMI were more likely to reside within enhancer elements and CpG island flanking shores/shelves and less likely to be in CpG islands and promoter regions. Similarly, CpG loci mediating the association between neighborhood socioeconomic disadvantage and HDL-C were more likely to reside within enhancer elements and DHS, and less likely to be found in CpG islands.
Overlap With Expression Quantitative Trait Methylation and Putatively Causal CpG Sites Associated With Methylation Quantitative Trait Loci
Among the 410 CpG mediators of the association between adult SES and BMI, 93 were also previously reported eQTMs whose methylation level was associated with gene expression in a previous MESA study (54). These 93 CpG mediators were associated with the expression of 152 cis-genes (Supplementary Table 1). Similarly, 10 of the 43 CpG mediators of the association between neighborhood socioeconomic disadvantage and HDL-C were previously reported eQTMs associated with the expression of 14 cis-genes (Supplementary Table 6). Furthermore, as shown in Table 3, CpG mediators of both the associations between adult SES and BMI and between neighborhood socioeconomic disadvantage and HDL-C were highly enriched for eQTMs (Bonferroni-corrected p = 1.72E-59 and 8.17E-08, respectively). In other words, the identified CpG mediators were enriched for association with cis-gene expression, indicating that they were more likely to influence proximal gene expression compared to non-mediating CpG sites. None of the identified CpG mediators overlapped with the 92 putatively causal CpG sites for cardiovascular risk factors previously identified from MR analysis using cis-mQTLs as instrumental variables (74).
Gene-Set Analysis
Mediating methylation sites were distributed across the genome (Supplementary Figures 1, 3). The 410 CpG mediators of the association between adult SES and BMI mapped to 286 unique genes. The 43 CpG mediators of the association between neighborhood socioeconomic disadvantage and HDL-C mapped to 31 unique genes. Using the Illumina annotation gene mapping, neither of the two sets of CpG mediators was enriched for any GO terms or KEGG pathways when controlling for the differing numbers of probes per gene after FDR correction. However, using the eQTM annotation from the previous MESA study (54) to map gene expression to CpG sites, the mediating CpG sites of adult SES and BMI were associated with expression of genes enriched in four GO biological process terms related to antigen processing and presentation at FDR q < 0.05 (Supplementary Figure 4). Similarly, mediating CpG sites of neighborhood socioeconomic disadvantage and HDL-C were associated with expression of genes enriched in nine GO terms related to biological processes such as the apoptotic process, cell death, and establishment or maintenance of cell polarity (Supplementary Figure 5). Significant GO terms for both sets of CpG mediators remained largely consistent even after excluding CpG sites located in regions of inactive chromatin in primary mononuclear cells (Supplementary Figures 6, 7). No significant over-representation in KEGG pathways was observed.
Discussion
In this study, we assessed whether differences in DNA methylation mediate the effects of individual- and neighborhood-level social disadvantage on CVD risk factors. Epigenetic mediation was identified for associations between adult SES and/or neighborhood socioeconomic disadvantage and several CVD risk factors. The majority of these mediators lost significance after further adjustment for BMI, which indicates that BMI might also be part of the epigenetic mediation pathway for the majority of CVD risk factors. Alternatively, BMI may confound the association between social disadvantage and CVD risk factors. Our results suggest that a small fraction (1.4%) of the total variability in BMI was explained by adult SES, and that this association was partially mediated by 410 CpG sites. After reducing the CpG mediators to 10 uncorrelated PCs, we found that the set of identified CpG mediators accounted for at least 52.6% of the relationship between adult SES and BMI. Similarly, our findings show that 2.1% of the total variability in HDL-C was explained by neighborhood socioeconomic disadvantage, and this association was mediated by 43 CpG sites, independent of BMI. After reducing these 43 CpG mediators to 10 uncorrelated PCs, the overall mediation effect across the loci explained at least 23.3% of the total effect of neighborhood socioeconomic disadvantage on HDL-C. Although there was no overlap with the putatively causal CpG sites previously identified from MR analysis using cis-mQTLs as instrumental variables, the CpG mediators identified in this study could still be true statistical mediators since CpG sites influenced by social factors may be different than those influenced by genetic variants.
Many of the CpG sites reported in our study have been previously linked to CVD outcomes and risk factors. Among the 410 CpG mediators of adult SES and BMI, the top-ranking CpG sites included cg15633603 and cg10508317 in the body and 5′UTR of cytokine signaling-3 (SOCS3), which encodes a protein that suppresses signaling by inflammatory cytokines such as leptin and interleukin-6 signaling that are upregulated in obesity (83). Of note, one of the SOCS3 CpGs identified in this study, cg18181703, was also identified in a recent large-scale EWAS of BMI (45), and methylation at this CpG was shown to interact with cumulative adverse life stress to influence BMI and obesity (84). Other highly ranked CpGs in our study are in the gene bodies of solute carrier 25A10 (SLC25A10), encoding a protein that increases lipid accumulation in adipose tissues (85), and phosphofurin acidic cluster sorting protein 2 (PACS2), which encodes a mitochondrial-associated membrane protein important for cellular homeostasis and implicated in obesity and other metabolic disorders including diabetes and metabolic syndrome (86).
In total, among the 410 CpG mediators of the association between adult SES and BMI, 16 sites were identified in the recent large-scale EWAS of BMI (45) (see Supplementary Table 1). Of these, two were identified as eQTMs in MESA, with cg13274938 corresponding to the C-C chemokine receptor 7 (CCR7), and cg10922280 corresponding to dipeptidase 2 (DPEP2) among other genes. The protein encoded by CCR7 has been shown in mice to play a causal role in maintaining innate and adaptive immunity contributing to adipose tissue inflammation in obesity (87). The protein encoded by DPEP2 has been shown to modulate macrophage inflammation (88), and is upregulated in subcutaneous white adipose tissue in obese women with type 2 diabetes (89). The most highly ranked MESA eQTM was cg19103704, which is associated with expression of the proteasome 26S subunit, ATPase 4 (PSMC4), a subunit of perilipin-2 which regulates intracellular lipid metabolism in macrophages, and the fc fragment of IgG binding protein (FCGBP), both of which have been associated with obesity (90, 91).
As a whole, we found that mediating CpG eQTMs were associated with expression of genes enriched in antigen processing and presentation, which is consistent with the well-established finding that chronic inflammation contributes to obesity and other related metabolic conditions. These inflammatory pathways remained significantly enriched even after removing CpG sites known to be in an inactive chromatin state in primary mononuclear cells from peripheral blood. The innate immune system has long been known to be implicated in the development of obesity and its associated diseases, and recent studies have also demonstrated the critical roles for cells of the adaptive immune system (92, 93). Specifically, antigen-driven responses have been linked to obesity. In prior studies, increased expression of genes involved in MHC class II antigen processing and presentation was found in adipocytes of obese women (94, 95). In mice, MHC class I antigen presentation was altered after lipid overload with dietary saturated fatty acid (96). Our results support and add to these previous studies by indicating that methylation of inflammation genes, particularly those involved in antigen processing and presentation, may mediate the effect of adult SES on BMI.
A growing body of evidence suggests that sex may influence the strength of the association between SES and obesity in developed countries (1, 2, 70–73). According to the National Health and Nutrition Examination Survey, 2005–2008, there was no significant association between education, a marker of adult SES, and prevalent obesity among men in the United States, but there was a negative relationship among women (70). In the present study, all of the CpG mediators that reached significance in sex-specific mediation analysis were exclusive to either males or females, except one. This is also consistent with findings from the New England Family Study that examined sex-specific methylation mediators between early life social disadvantage and adult BMI, where distinct CpG sites were identified for males and females (51, 52). Only two mediating CpGs identified in our combined sex analysis overlapped with those identified in the New England Family Study (cg05832823 and cg22679740), which were both identified in females only using adipose tissue. In this sample, a larger proportion of the association between SES and BMI was mediated by the CpG sites in females than males. However, in our study, females had a smaller total effect of adult SES on BMI (0.49%) than males (3.5%), which is in contrast to other studies that found stronger associations between SES and BMI in females (70). Our findings provide additional insight into the sex-specific nature of the epigenetic mechanisms linking SES to obesity and suggest that the epigenetic mediation pathways may operate more strongly for women.
A top-ranked mediator of neighborhood socioeconomic disadvantage and HDL-C, cg03128029, is located in the gene body of NOP58, a component of a small nucleolar ribonuclear protein (snoRNP). This CpG was previously associated with HDL in the REGICOR study (97). Interestingly, this CpG site and another top-ranking mediator of neighborhood socioeconomic disadvantage and HDL-C, cg20995564 in the zinc finger e-box binding homeobox-2 gene (ZEB2), were associated with serum CRP, an inflammatory biomarker of CVD, in both Europeans and African Americans (98). The two most significant eQTM mediators of neighborhood socioeconomic disadvantage on HDL-C were cg03254336, corresponding to expression of transcription factor 7-like 2 (TCF7L2), a major genetic risk factor for type 2 diabetes, and cg10699171, corresponding to expression of RHO family interacting cell polarization regulator 2 (FAM65B). Recently, it was found that dietary fat intake was more strongly associated with HDL-C in participants with a certain TCF7L2 genetic variant (99). Genetic variants in the FAM65B gene region were also associated with early onset dyslipidemia, specifically hyper-LDL-cholesterolemia (100). None of the CpGs identified in our study were among the significant CpGs in the most recent EWAS of HDL-C (50).
Mediating CpG eQTMs for the association between neighborhood socioeconomic disadvantage and HDL-C were enriched for genes involved in the apoptotic process, cell death, the establishment/maintenance of cell polarity, and regulation of leukocyte chemotaxis. Lipid homeostasis is known to play a key role in regulated cell death processes including apoptosis, necroptosis, and ferroptosis (101). There is also evidence that genes playing a key role in metabolic induction of apoptosis may function through regulation of lipid metabolism, including HDL-C levels (102). Macrophage polarity, the cellular differentiation into pro-inflammatory or anti-inflammatory cells, appears to be influenced by HDL in mice but not humans (103). HDL-C is known to inhibit leukocyte chemotaxis, leading to lower levels of inflammation (104). However, more work needs to be done to fully characterize the potential pathways between genes that play a role in these cellular processes and HDL-C levels.
The methylation changes that we identified as potential mediators of the associations between social factors and CVD risk factors were in monocytes. During the development of atherosclerosis, monocytes are recruited to the endothelial cells from the bloodstream and become activated by LDL or other stimulating factors. Activated monocytes then release additional inflammatory factors such as IL-6, MCP-1, and TNF-α, and differentiate into macrophages. Finally, macrophages engulf excess lipoproteins including LDL to form foam cells, which are a key characteristic of early-stage atherosclerosis (105). Therefore, monocyte and macrophage differentiation and activation are critical processes in the development of atherosclerosis. Prior research suggests that DNA methylation plays an important role in regulating these processes. A comparison of epigenome-wide methylation among human monocytes, naive macrophages, activated macrophages with pro-inflammatory or anti-inflammatory phenotypes, and foam cells activated with oxidized or acetylated LDL found that 5,870 CpG sites were differentially methylated across the 6 cell types (106). Of these, 5,780 (98%) were attributed to monocyte-to-macrophage differentiation. In our study, 96 of the 410 (23.4%) mediating CpG sites of the association between adult SES and BMI and 23 of the 43 (53.5%) mediating CpG sites of the association between neighborhood socioeconomic disadvantage and HDL-C were also involved in monocyte-to-macrophage differentiation (Supplementary Tables 1, 6) (106). In addition, several of the mediating CpG sites were located near genes known to be implicated in the process of monocyte-to-macrophage differentiation. For example, a top-ranking mediating CpG site of the association between adult SES and BMI, cg09100014, is located in the gene body of IRF8, a transcription factor well-established in monocyte differentiation (107). Furthermore, as shown in Supplementary Figures 6, 7, pathway analysis using genes whose expression levels were associated with the identified CpG mediators in an active chromatin state in primary mononuclear cells showed strong enrichment for biological processes involved in monocyte-to-macrophage differentiation such as regulation of immune response, regulation of leukocyte migration, regulation of leukocyte chemotaxis.
To our knowledge, this was the first study to examine the role of DNA methylation in mediating the relationships between individual SES and neighborhood characteristics and several CVD risk factors in a large, multi-ethnic, population-based cohort. In this study, the use of composite measures for neighborhood conditions that encompass census-based and survey-based metrics allowed us to examine multiple dimensions of neighborhood. Moreover, we adopted a high-dimensional mediation method, JT-Comp, to formally test the mediation effects of hundreds of thousands CpG sites. This method takes into account the composite nature of the null hypothesis of mediation analysis, which is often ignored by other traditionally used methods such as Sobel's normality test and thus provides better calibrated p-values under the null (108). Other high-dimensional mediation methods including DACT (109) have been proposed more recently, but in our experience JT-Comp remains the most stable method when the mediation effects are small and sparse. Nevertheless, additional exploration with other high-dimensional mediation methods may be warranted.
Our study is not without limitations. First, DNA methylation and gene expression were measured in peripheral purified monocytes. Although blood may not be the most relevant tissue type for many of the traits we studied, it has been widely and successfully used as a surrogate for targeted tissues that are not readily accessible. For example, in a large EWAS of BMI (45), methylation at 187 CpG sites associated with BMI in blood showed a moderate to strong correlation with other metabolically relevant tissues including subcutaneous and omental fat, liver, muscle, spleen, and pancreas. Furthermore, among the 187 CpG sites associated with BMI in blood, 120 also showed directional consistency and 91 were significantly associated with BMI in adipose tissue. While these findings are consistent with the idea that methylation in blood correlates with methylation in other tissues, in another study comparing DNA methylation profile in matched samples of blood and adipose tissue, only 5.2% of the CpG sites had a >0.5 correlation between blood and adipose tissue (110). Therefore, although there is some rationale for using DNA methylation measured in blood as a proxy, association analysis in more directly relevant tissues (e.g., adipose, heart, and liver) would be the next important step to validate our results and identify additional non-blood related CpG mediators.
Second, we used education as the only indicator of individual SES. There are many other aspects of SES, such as income, occupation, and wealth, which were not examined in this study. However, education is a robust indicator of SES that correlates well with many other SES measures (111, 112). In this study, we used maternal education as a measure of childhood SES because it has been suggested as a more critical determinant of child health than paternal education (58, 113, 114). In addition to better employment and higher household income, higher maternal education might improve child health through a wide range of health-promoting behaviors and health knowledge (58). We also dichotomized both adult and parental education which may have limited our ability to detect associations with the CVD risk factors (and mediation effects). The analytical strategy we used required us to simplify analyses of individual and neighborhood social determinants to isolate their independent effects. Future work should examine joint and interaction effects and further consider the many interrelated pathways through which social determinants may impact methylation and CVD risk factors. Moreover, because analyses were adjusted for race/ethnicity, we did not investigate important ways in which structural racism may impact CVD risks and the role of methylation in these relationships. Finally, although we controlled for current smoking status as a potential confounder, residual confounding might be present because we did not take other dimensions of smoking such as intensity, duration, and pack-years into account.
In this study, we used summary neighborhood scores that were constructed as weighted averages across multiple neighborhoods in order to examine the cumulative effects of neighborhood over time. By using weights proportional to the length of time the respondents resided in each neighborhood, we assumed that neighborhoods where the respondents lived for a longer period of time would have a greater impact on the proposed mediator and outcome. However, it is possible that neighborhoods lived in more recently may have a larger influence on methylation and cardiovascular risk factors than previous neighborhoods, which was not accounted for in our analysis. Future studies should consider examining how the timing of neighborhood exposures might influence the effects of neighborhood-level social disadvantage on DNA methylation and cardiovascular risk factors. In addition, we did not have the complete address history for every respondent. Between Exams 1 and 5, 1.2 and 11.6% of respondents had at least one missing value for neighborhood SES and neighborhood social environment, respectively. This may have led to misclassification of the cumulative average neighborhood exposures for the small number of respondents affected.
Another limitation is that although we performed statistical mediation analysis, our data were cross-sectional and thus we were unable to assess the temporal relationships between changes in methylation and CVD risk factors. Thus, we cannot be fully confident of the causal direction of effect between methylation and CVD risk factors. There is some support for a causal effect of changes in methylation on BMI (79), for example. However, other studies have shown that BMI tends to be more of a driver of changes in methylation than a downstream consequence (45, 65). Furthermore, in this study, we assumed that the effect of social disadvantage on cardiovascular risk factors remains the same as the level of DNA methylation changes. Thus, we did not include exposure-mediator interaction terms in the mediation analysis. However, it is worth noting that violation of this assumption may result in invalid causal conclusions (115). Further longitudinal studies that explore exposure-mediator interaction are needed to more fully delineate the causal pathways proposed here.
Moreover, we employed an analytical strategy to first test the total effect of the exposure on the outcome, and only explored potential mediation effects when the total effect was significant. In the literature on mediation analysis, whether or not total effect should be required before testing indirect effect has been subject to considerable debate. Researchers in favor of suspending the total-effect test argue that requiring a significant total effect of the exposure on the outcome may limit the power of mediation analysis, since there are several scenarios in which the total effect may not be significant even in the presence of mediation. For example, the test of the total effect may be underpowered. Alternatively, indirect effects mediated through multiple mediators could cancel each other out, resulting in a non-significant total effect. Thus, we acknowledge that by requiring a significant total effect, our findings are conservative and may miss some true indirect effects. However, this does not invalidate our mediation analysis as we intended to assess the mediation effect, rather than the indirect effect, of DNA methylation in the relationship between social disadvantage and cardiovascular risk factors. Although the terms “mediator variables” and “indirect effects” are often used interchangeably, there is an important distinction between them (116). In particular, mediation is a special, more restrictive type of intervening relationship that implies the total effect of the exposure on the outcome was present initially and sheds light on the nature of the relationship between the exposure and the outcome [see (116) for a detailed discussion]. Therefore, given that the goal of our study was to better characterize the role of methylation in linking the effects of social disadvantage on cardiovascular diseases, we limited our search space to mediation settings with a detectable total effect.
Finally, methylation levels of CpG sites that are close to each other in the same biological pathways may be correlated. In light of this, a limitation of JT-Comp and other high-dimensional univariate mediation methods is that the correlation among potential mediators is not explicitly modeled. Recently, high-dimensional mediation methods that can jointly model potential mediators, such as the Bayesian high-dimensional mediation method (BAMA) (117) and its extensions (118, 119), have been proposed. Although these joint analysis methods can reduce the multiple testing burden, increase power, and better estimate independent effects, they are computationally heavy and can only simultaneously evaluate a few thousand mediators simultaneously. Since the goal of the current study was to conduct epigenome-wide meditation analysis with nearly half a million potential mediators, such methods were impractical, and we instead carried out ad hoc PCA analyses to better characterize the overall mediation effect across the identified loci and penalization-based high-dimensional mediation analysis on the potential CpG mediators identified in the epigenome-wide mediation analysis to further fine map the identified loci.
In summary, our findings support the hypothesis that DNA methylation acts as a mediator between individual- and neighborhood-level social disadvantage and CVD risk factors, and provide insight into the underlying epigenetic mechanisms that link social disadvantage to poor cardiovascular health. Since many of the identified CpG mediators are involved in the process of monocyte-to-macrophage differentiation and macrophage polarity, our findings suggest that epigenetic regulation of monocyte differentiation and subsequent activation might be a promising avenue for further investigation. Future research is needed to replicate our findings in other independent cohorts and confirm the role of DNA methylation in mediating the association between individual- and neighborhood-level social disadvantage and cardiovascular risk factors.
Data Availability Statement
Data used in this analysis can be obtained through the MESA Data Coordinating Center (https://www.mesa-nhlbi.org/) and the database of Genotypes and Phenotypes [dbGaP accession numbers: phs000209 (MESA Cohort) and phs00420 (MESA SHARe)].
Ethics Statement
The studies involving human participants were reviewed and approved by Institutional Review Boards (IRBs) at Johns Hopkins Medical Institutions, University of Minnesota, Columbia University Medical Center, Wake Forest University Health Sciences, University of Washington, and University of Michigan. The patients/participants provided their written informed consent to participate in this study.
Author Contributions
YW and JS conceptualized and designed the study. BN, AD, YL, WZ, and XZ contributed to study design. AD, KM, and YL contributed to data collection, cleaning, and curation. YW conducted the data analysis, with assistance from WZ, SR, LS, JD, and YS. Result interpretation was performed by YW, JS, AD, SK, KB, XZ, BM, and YL. YW drafted the manuscript with assistance from JS, FA, and KK. JS provided financial support. All authors reviewed the results and approved the final version of the manuscript.
Funding
MESA and the MESA SHARe project are conducted and supported by the National Heart, Lung, and Blood Institute (NHLBI) in collaboration with MESA investigators. Support for MESA is provided by Contracts 75N92020D00001, HHSN268201500003I, N01-HC-95159, 75N92020D00005, N01-HC-95160, 75N92020D00002, N01-HC-95161, 75N92020D00003, N01-HC-95162, 75N92020D00006, N01-HC-95163, 75N92020D00004, N01-HC-95164, 75N92020D00007, N01-HC-95165, N01-HC-95166, N01-HC-95167, N01-HC-95168, N01-HC-95169, UL1-TR-000040, UL1-TR-001079, UL1-TR-001420, UL1-TR-001881, and DK063491. Funding support for the neighborhood scales dataset was provided by R01HL071759. The MESA Epigenomics and Transcriptomics Studies were funded by NIH Grants 1R01HL101250, 1RF1AG054474, R01HL126477, R01DK101921, and R01HL135009. Analysis for this study was provided by R01HL141292.
Conflict of Interest
The authors declare that the research was conducted in the absence of any commercial or financial relationships that could be construed as a potential conflict of interest.
Publisher's Note
All claims expressed in this article are solely those of the authors and do not necessarily represent those of their affiliated organizations, or those of the publisher, the editors and the reviewers. Any product that may be evaluated in this article, or claim that may be made by its manufacturer, is not guaranteed or endorsed by the publisher.
Acknowledgments
The authors wish to thank the MESA staff and participants.
Supplementary Material
The Supplementary Material for this article can be found online at: https://www.frontiersin.org/articles/10.3389/fcvm.2022.848768/full#supplementary-material
References
1. McLaren L. Socioeconomic status and obesity. Epidemiol Rev. (2007) 29:29–48. doi: 10.1093/epirev/mxm001
2. Sobal J, Stunkard AJ. Socioeconomic status and obesity: a review of the literature. Psychol Bull. (1989) 105:260–75. doi: 10.1037/0033-2909.105.2.260
3. Lee C, Park S. Examining cumulative inequality in the association between childhood SES and BMI from midlife to old age. J Gerontol B Psychol Sci Soc Sci. (2020) 75:1264–74. doi: 10.1093/geroni/igz038.1274
4. Kivimäki M, Smith GD, Elovainio M, Pulkki L, Keltikangas-Järvinen L, Talttonen L, et al. Socioeconomic circumstances in childhood and blood pressure in adulthood: the cardiovascular risk in young Finns study. Ann Epidemiol. (2006) 16:737–42. doi: 10.1016/j.annepidem.2006.01.004
5. Leng B, Jin Y, Li G, Chen L, Jin N. Socioeconomic status and hypertension: a meta-analysis. J Hypertens. (2015) 33:221–9. doi: 10.1097/HJH.0000000000000428
6. Matthews KA, Kiefe CI, Lewis CE, Liu K, Sidney S, Yunis C, et al. Socioeconomic trajectories and incident hypertension in a biracial cohort of young adults. Hypertension. (2002) 39:772–6. doi: 10.1161/hy0302.105682
7. Maty SC, Everson-Rose SA, Haan MN, Raghunathan TE, Kaplan GA. Education, income, occupation, and the 34-year incidence (1965-99) of Type 2 diabetes in the Alameda County Study. Int J Epidemiol. (2005) 34:1274–81. doi: 10.1093/ije/dyi167
8. Tamayo T, Christian H, Rathmann W. Impact of early psychosocial factors (childhood socioeconomic factors and adversities) on future risk of type 2 diabetes, metabolic disturbances and obesity: a systematic review. BMC Public Health. (2010) 10:525. doi: 10.1186/1471-2458-10-525
9. Gustafsson PE, Janlert U, Theorell T, Westerlund H, Hammarström A. Fetal and life course origins of serum lipids in mid-adulthood: results from a prospective cohort study. BMC Public Health. (2010) 10:484. doi: 10.1186/1471-2458-10-484
10. Brunner E, Shipley MJ, Blane D, Smith GD, Marmot MG. When does cardiovascular risk start? Past and present socioeconomic circumstances and risk factors in adulthood. J Epidemiol Community Health. (1999) 53:757–64. doi: 10.1136/jech.53.12.757
11. Brown H, Becker F, Antwi K. Association between lipid biomarkers, physical activity, and socioeconomic status in a population-based cross-sectional study in the UK. Sports Med Open. (2015) 2:25. doi: 10.1186/s40798-016-0049-9
12. Kavanagh A, Bentley RJ, Turrell G, Shaw J, Dunstan D, Subramanian SV. Socioeconomic position, gender, health behaviours and biomarkers of cardiovascular disease and diabetes. Soc Sci Med. (2010) 71:1150–60. doi: 10.1016/j.socscimed.2010.05.038
13. Pollitt RA, Rose KM, Kaufman JS. Evaluating the evidence for models of life course socioeconomic factors and cardiovascular outcomes: a systematic review. BMC Public Health. (2005) 5:7. doi: 10.1186/1471-2458-5-7
14. Diez Roux AV, Merkin SS, Arnett D, Chambless L, Massing M, Nieto FJ, et al. Neighborhood of residence and incidence of coronary heart disease. N Engl J Med. (2001) 345:99–106. doi: 10.1056/NEJM200107123450205
15. Sundquist K, Winkleby M, Ahlén H, Johansson SE. Neighborhood socioeconomic environment and incidence of coronary heart disease: a follow-up study of 25,319 women and men in Sweden. Am J Epidemiol. (2004) 159:655–62. doi: 10.1093/aje/kwh096
16. Janssen I, Boyce WF, Simpson K, Pickett W. Influence of individual- and area-level measures of socioeconomic status on obesity, unhealthy eating, and physical inactivity in Canadian adolescents. Am J Clin Nutr. (2006) 83:139–45. doi: 10.1093/ajcn/83.1.139
17. Kivimäki M, Vahtera J, Tabák AG, Halonen JI, Vineis P, Pentti J, et al. Neighbourhood socioeconomic disadvantage, risk factors, and diabetes from childhood to middle age in the Young Finns Study: a cohort study. Lancet Public Health. (2018) 3:e365–e73. doi: 10.1016/S2468-2667(18)30111-7
18. Diez Roux AV, Mair C. Neighborhoods and health. Ann N Y Acad Sci. (2010) 1186:125–45. doi: 10.1111/j.1749-6632.2009.05333.x
19. Mujahid MS, Diez Roux AV, Shen M, Gowda D, Sánchez B, Shea S, et al. Relation between neighborhood environments and obesity in the Multi-Ethnic Study of Atherosclerosis. Am J Epidemiol. (2008) 167:1349–57. doi: 10.1093/aje/kwn047
20. Kaiser P, Diez Roux AV, Mujahid M, Carnethon M, Bertoni A, Adar SD, et al. Neighborhood environments and incident hypertension in the multi-ethnic study of atherosclerosis. Am J Epidemiol. (2016) 183:988–97. doi: 10.1093/aje/kwv296
21. Christine PJ, Auchincloss AH, Bertoni AG, Carnethon MR, Sánchez BN, Moore K, et al. Longitudinal associations between neighborhood physical and social environments and incident type 2 diabetes mellitus: the multi-ethnic study of atherosclerosis (MESA). JAMA Intern Med. (2015) 175:1311–20. doi: 10.1001/jamainternmed.2015.2691
22. Unger E, Diez-Roux AV, Lloyd-Jones DM, Mujahid MS, Nettleton JA, Bertoni A, et al. Association of neighborhood characteristics with cardiovascular health in the multi-ethnic study of atherosclerosis. Circ Cardiovasc Qual Outcomes. (2014) 7:524–31. doi: 10.1161/CIRCOUTCOMES.113.000698
23. Jaenisch R, Bird A. Epigenetic regulation of gene expression: how the genome integrates intrinsic and environmental signals. Nat Genet. (2003) 33(Suppl):245–54. doi: 10.1038/ng1089
24. McGowan PO, Szyf M. The epigenetics of social adversity in early life: implications for mental health outcomes. Neurobiol Dis. (2010) 39:66–72. doi: 10.1016/j.nbd.2009.12.026
25. Olden K, Olden HA, Lin YS. The role of the epigenome in translating neighborhood disadvantage into health disparities. Curr Environ Health Rep. (2015) 2:163–70. doi: 10.1007/s40572-015-0048-x
26. Giurgescu C, Nowak AL, Gillespie S, Nolan TS, Anderson CM, Ford JL, et al. Neighborhood environment and DNA methylation: implications for cardiovascular disease risk. J Urban Health. (2019) 96:23–34. doi: 10.1007/s11524-018-00341-1
27. Notterman DA, Mitchell C. Epigenetics and understanding the impact of social determinants of health. Pediatr Clin North Am. (2015) 62:1227–40. doi: 10.1016/j.pcl.2015.05.012
28. Borghol N, Suderman M, McArdle W, Racine A, Hallett M, Pembrey M, et al. Associations with early-life socio-economic position in adult DNA methylation. Int J Epidemiol. (2012) 41:62–74. doi: 10.1093/ije/dyr147
29. Needham BL, Smith JA, Zhao W, Wang X, Mukherjee B, Kardia SL, et al. Life course socioeconomic status and DNA methylation in genes related to stress reactivity and inflammation: the multi-ethnic study of atherosclerosis. Epigenetics. (2015) 10:958–69. doi: 10.1080/15592294.2015.1085139
30. Tehranifar P, Wu HC, Fan X, Flom JD, Ferris JS, Cho YH, et al. Early life socioeconomic factors and genomic DNA methylation in mid-life. Epigenetics. (2013) 8:23–7. doi: 10.4161/epi.22989
31. Bush NR, Edgar RD, Park M, MacIsaac JL, McEwen LM, Adler NE, et al. The biological embedding of early-life socioeconomic status and family adversity in children's genome-wide DNA methylation. Epigenomics. (2018) 10:1445–61. doi: 10.2217/epi-2018-0042
32. Stringhini S, Polidoro S, Sacerdote C, Kelly RS, van Veldhoven K, Agnoli C, et al. Life-course socioeconomic status and DNA methylation of genes regulating inflammation. Int J Epidemiol. (2015) 44:1320–30. doi: 10.1093/ije/dyv060
33. Smith JA, Zhao W, Wang X, Ratliff SM, Mukherjee B, Kardia SLR, et al. Neighborhood characteristics influence DNA methylation of genes involved in stress response and inflammation: the multi-ethnic study of atherosclerosis. Epigenetics. (2017) 12:662–73. doi: 10.1080/15592294.2017.1341026
34. Lei MK, Beach SR, Simons RL, Philibert RA. Neighborhood crime and depressive symptoms among African American women: genetic moderation and epigenetic mediation of effects. Soc Sci Med. (2015) 146:120–8. doi: 10.1016/j.socscimed.2015.10.035
35. Baccarelli A, Wright R, Bollati V, Litonjua A, Zanobetti A, Tarantini L, et al. Ischemic heart disease and stroke in relation to blood DNA methylation. Epidemiology. (2010) 21:819–28. doi: 10.1097/EDE.0b013e3181f20457
36. Turunen MP, Aavik E, Ylä-Herttuala S. Epigenetics and atherosclerosis. Biochim Biophys Acta. (2009) 1790:886–91. doi: 10.1016/j.bbagen.2009.02.008
37. Smolarek I, Wyszko E, Barciszewska AM, Nowak S, Gawronska I, Jablecka A, et al. Global DNA methylation changes in blood of patients with essential hypertension. Med Sci Monit. (2010) 16:CR149–55.
38. Fernández-Sanlés A, Sayols-Baixeras S, Subirana I, Degano IR, Elosua R. Association between DNA methylation and coronary heart disease or other atherosclerotic events: a systematic review. Atherosclerosis. (2017) 263:325–33. doi: 10.1016/j.atherosclerosis.2017.05.022
39. Talens RP, Jukema JW, Trompet S, Kremer D, Westendorp RG, Lumey LH, et al. Hypermethylation at loci sensitive to the prenatal environment is associated with increased incidence of myocardial infarction. Int J Epidemiol. (2012) 41:106–15. doi: 10.1093/ije/dyr153
40. Sun YV, Lazarus A, Smith JA, Chuang YH, Zhao W, Turner ST, et al. Gene-specific DNA methylation association with serum levels of C-reactive protein in African Americans. PLoS ONE. (2013) 8:e73480. doi: 10.1371/journal.pone.0073480
41. Westerman K, Sebastiani P, Jacques P, Liu S, DeMeo D, Ordovás JM, et al. methylation modules associate with incident cardiovascular disease and cumulative risk factor exposure. Clin Epigenetics. (2019) 11:142. doi: 10.1186/s13148-019-0705-2
42. Agha G, Mendelson MM, Ward-Caviness CK, Joehanes R, Huan T, Gondalia R, et al. Blood leukocyte DNA methylation predicts risk of future myocardial infarction and coronary heart disease. Circulation. (2019) 140:645–57. doi: 10.1161/CIRCULATIONAHA.118.039357
43. Shen Y, Peng C, Bai Q, Ding Y, Yi X, Du H, et al. Epigenome-wide association study indicates hypomethylation of MTRNR2L8 in large-artery atherosclerosis stroke. Stroke. (2019) 50:1330–8. doi: 10.1161/STROKEAHA.118.023436
44. Qin X, Karlsson IK, Wang Y, Li X, Pedersen N, Reynolds CA, et al. The epigenetic etiology of cardiovascular disease in a longitudinal Swedish twin study. Clin Epigenetics. (2021) 13:129. doi: 10.1186/s13148-021-01113-6
45. Wahl S, Drong A, Lehne B, Loh M, Scott WR, Kunze S, et al. Epigenome-wide association study of body mass index, and the adverse outcomes of adiposity. Nature. (2017) 541:81–6. doi: 10.1038/nature20784
46. Walaszczyk E, Luijten M, Spijkerman AMW, Bonder MJ, Lutgers HL, Snieder H, et al. DNA methylation markers associated with type 2 diabetes, fasting glucose and HbA. Diabetologia. (2018) 61:354–68. doi: 10.1007/s00125-017-4497-7
47. Meeks KAC, Henneman P, Venema A, Addo J, Bahendeka S, Burr T, et al. Epigenome-wide association study in whole blood on type 2 diabetes among sub-Saharan African individuals: findings from the RODAM study. Int J Epidemiol. (2019) 48:58–70. doi: 10.1093/ije/dyy171
48. Florath I, Butterbach K, Heiss J, Bewerunge-Hudler M, Zhang Y, Schöttker B, et al. Type 2 diabetes and leucocyte DNA methylation: an epigenome-wide association study in over 1,500 older adults. Diabetologia. (2016) 59:130–8. doi: 10.1007/s00125-015-3773-7
49. Richard MA, Huan T, Ligthart S, Gondalia R, Jhun MA, Brody JA, et al. DNA methylation analysis identifies loci for blood pressure regulation. Am J Hum Genet. (2017) 101:888–902. doi: 10.1016/j.ajhg.2017.09.028
50. Jhun MA, Mendelson M, Wilson R, Gondalia R, Joehanes R, Salfati E, et al. A multi-ethnic epigenome-wide association study of leukocyte DNA methylation and blood lipids. Nat Commun. (2021) 12:3987. doi: 10.1038/s41467-021-24600-z
51. Loucks EB, Huang YT, Agha G, Chu S, Eaton CB, Gilman SE, et al. Epigenetic mediators between childhood socioeconomic disadvantage and mid-life body mass index: the new england family study. Psychosom Med. (2016) 78:1053–65. doi: 10.1097/PSY.0000000000000411
52. Chu SH, Loucks EB, Kelsey KT, Gilman SE, Agha G, Eaton CB, et al. Sex-specific epigenetic mediators between early life social disadvantage and adulthood BMI. Epigenomics. (2018) 10:707–22. doi: 10.2217/epi-2017-0146
53. Bild DE, Bluemke DA, Burke GL, Detrano R, Diez Roux AV, Folsom AR, et al. Multi-ethnic study of atherosclerosis: objectives and design. Am J Epidemiol. (2002) 156:871–81. doi: 10.1093/aje/kwf113
54. Liu Y, Ding J, Reynolds LM, Lohman K, Register TC, De La Fuente A, et al. Methylomics of gene expression in human monocytes. Hum Mol Genet. (2013) 22:5065–74. doi: 10.1093/hmg/ddt356
55. Du P, Kibbe WA, Lin SM. Lumi: a pipeline for processing Illumina microarray. Bioinformatics. (2008) 24:1547–8. doi: 10.1093/bioinformatics/btn224
56. Peters TJ, Buckley MJ, Statham AL, Pidsley R, Samaras KV, Lord R, et al. De novo identification of differentially methylated regions in the human genome. Epigenetics Chromatin. (2015) 8:6. doi: 10.1186/1756-8935-8-6
57. Bibikova M, Barnes B, Tsan C, Ho V, Klotzle B, Le JM, et al. High density DNA methylation array with single CpG site resolution. Genomics. (2011) 98:288–95. doi: 10.1016/j.ygeno.2011.07.007
58. Desai S, Alva S. Maternal education and child health: is there a strong causal relationship? Demography. (1998) 35:71–81. doi: 10.2307/3004028
59. Mujahid MS, Diez Roux AV, Morenoff JD, Raghunathan T. Assessing the measurement properties of neighborhood scales: from psychometrics to ecometrics. Am J Epidemiol. (2007) 165:858–67. doi: 10.1093/aje/kwm040
60. Kim DS, Li YK, Bell GA, Burt AA, Vaisar T, Hutchins PM, et al. Concentration of smaller high-density lipoprotein particle (HDL-P) is inversely correlated with carotid intima media thickening after confounder adjustment: the multi ethnic study of atherosclerosis (MESA). J Am Heart Assoc. (2016) 5:977. doi: 10.1161/JAHA.115.002977
61. Paramsothy P, Knopp RH, Bertoni AG, Blumenthal RS, Wasserman BA, Tsai MY, et al. Association of combinations of lipid parameters with carotid intima-media thickness and coronary artery calcium in the MESA (Multi-Ethnic Study of Atherosclerosis). J Am Coll Cardiol. (2010) 56:1034–41. doi: 10.1016/j.jacc.2010.01.073
62. Psaty BM, Lee M, Savage PJ, Rutan GH, German PS, Lyles M. Assessing the use of medications in the elderly: methods and initial experience in the Cardiovascular Health Study. The Cardiovascular Health Study Collaborative Research Group. J Clin Epidemiol. (1992) 45:683–92. doi: 10.1016/0895-4356(92)90143-B
63. Smith NL, Psaty BM, Heckbert SR, Tracy RP, Cornell ES. The reliability of medication inventory methods compared to serum levels of cardiovascular drugs in the elderly. J Clin Epidemiol. (1999) 52:143–6. doi: 10.1016/S0895-4356(98)00141-3
64. Karlsson Linnér R, Marioni RE, Rietveld CA, Simpkin AJ, Davies NM, Watanabe K, et al. An epigenome-wide association study meta-analysis of educational attainment. Mol Psychiatry. (2017) 22:1680–90. doi: 10.1038/mp.2017.210
65. Sun D, Zhang T, Su S, Hao G, Chen T, Li QZ, et al. Body mass index drives changes in DNA methylation: a longitudinal study. Circ Res. (2019) 125:824–33. doi: 10.1161/CIRCRESAHA.119.315397
66. Huang Y-T. Genome-wide analyses of sparse mediation effects under composite null hypotheses. Ann Appl Stat. (2019) 13:60–84. doi: 10.1214/18-AOAS1181
67. Tingley D, Yamamoto T, Hirose K, Keele L, Imai K. Mediation: R package for causal mediation analysis. J Statist Software. (2014) 59:1–38. doi: 10.18637/jss.v059.i05
68. Zhang H, Zheng Y, Zhang Z, Gao T, Joyce B, Yoon G, et al. Estimating and testing high-dimensional mediation effects in epigenetic studies. Bioinformatics. (2016) 32:3150–4. doi: 10.1093/bioinformatics/btw351
69. Zhang H, Zheng Y, Hou L, Zheng C, Liu L. Mediation analysis for survival data with High-Dimensional mediators. Bioinformatics. (2021) 37:3815–21. doi: 10.1093/bioinformatics/btab564
70. Ogden CL, Lamb MM, Carroll MD, Flegal KM. Obesity and Socioeconomic Status in Adults: United States, 2005-2008. NCHS Data Brief No 50. Hyattsville, MD: National Center for Health Statistics (2010).
71. Bann D, Johnson W, Li L, Kuh D, Hardy R. Socioeconomic inequalities in body mass index across adulthood: coordinated analyses of individual participant data from three British Birth Cohort Studies Initiated in 1946, 1958 and 1970. PLoS Med. (2017) 14:e1002214. doi: 10.1371/journal.pmed.1002214
72. Hajizadeh M, Campbell MK, Sarma S. Socioeconomic inequalities in adult obesity risk in Canada: trends and decomposition analyses. Eur J Health Econ. (2014) 15:203–21. doi: 10.1007/s10198-013-0469-0
73. Vernay M, Malon A, Oleko A, Salanave B, Roudier C, Szego E, et al. Association of socioeconomic status with overall overweight and central obesity in men and women: the French Nutrition and Health Survey 2006. BMC Public Health. (2009) 9:215. doi: 10.1186/1471-2458-9-215
74. Huan T, Joehanes R, Song C, Peng F, Guo Y, Mendelson M, et al. Genome-wide identification of DNA methylation QTLs in whole blood highlights pathways for cardiovascular disease. Nat Commun. (2019) 10:4267. doi: 10.1038/s41467-019-12228-z
75. Phipson B, Maksimovic J, Oshlack A. MissMethyl: an R package for analyzing data from Illumina's HumanMethylation450 platform. Bioinformatics. (2016) 32:286–8. doi: 10.1093/bioinformatics/btv560
76. Yu G, Wang LG, Han Y, He QY. ClusterProfiler: an R package for comparing biological themes among gene clusters. OMICS. (2012) 16:284–7. doi: 10.1089/omi.2011.0118
77. Kundaje A, Meuleman W, Ernst J, Bilenky M, Yen A, Heravi-Moussavi A, et al. Integrative analysis of 111 reference human epigenomes. Nature. (2015) 518:317–30. doi: 10.1038/nature14248
78. Watanabe K, Matsumoto A, Tsuda H, Iwamoto S. N4BP2L1 interacts with dynactin and contributes to GLUT4 trafficking and glucose uptake in adipocytes. J Diabetes Investig. (2021) 12:1958–66. doi: 10.1111/jdi.13623
79. Geurts YM, Dugué PA, Joo JE, Makalic E, Jung CH, Guan W, et al. Novel associations between blood DNA methylation and body mass index in middle-aged and older adults. Int J Obes. (2018) 42:887–96. doi: 10.1038/ijo.2017.269
80. Gomes AV. Genetics of proteasome diseases. Scientifica. (2013) 2013:637629. doi: 10.1155/2013/637629
81. Alsmadi O, Muiya P, Khalak H, Al-Saud H, Meyer BF, Al-Mohanna F, et al. Haplotypes encompassing the KIAA0391 and PSMA6 gene cluster confer a genetic link for myocardial infarction and coronary artery disease. Ann Hum Genet. (2009) 73:475–83. doi: 10.1111/j.1469-1809.2009.00534.x
82. Batista IAA, Helguero LA. Biological processes and signal transduction pathways regulated by the protein methyltransferase SETD7 and their significance in cancer. Signal Transduct Target Ther. (2018) 3:19. doi: 10.1038/s41392-018-0017-6
83. Wunderlich CM, Hövelmeyer N, Wunderlich FT. Mechanisms of chronic JAK-STAT3-SOCS3 signaling in obesity. JAKSTAT. (2013) 2:e23878. doi: 10.4161/jkst.23878
84. Xu K, Zhang X, Wang Z, Hu Y, Sinha R. Epigenome-wide association analysis revealed that SOCS3 methylation influences the effect of cumulative stress on obesity. Biol Psychol. (2018) 131:63–71. doi: 10.1016/j.biopsycho.2016.11.001
85. Mizuarai S, Miki S, Araki H, Takahashi K, Kotani H. Identification of dicarboxylate carrier Slc25a10 as malate transporter in de novo fatty acid synthesis. J Biol Chem. (2005) 280:32434–41. doi: 10.1074/jbc.M503152200
86. Li C, Li L, Yang M, Zeng L, Sun L. PACS-2: a key regulator of mitochondria-associated membranes (MAMs). Pharmacol Res. (2020) 160:105080. doi: 10.1016/j.phrs.2020.105080
87. Hellmann J, Sansbury BE, Holden CR, Tang Y, Wong B, Wysoczynski M, et al. CCR7 maintains nonresolving lymph node and adipose inflammation in obesity. Diabetes. (2016) 65:2268–81. doi: 10.2337/db15-1689
88. Yang X, Yue Y, Xiong S. Dpep2 emerging as a modulator of macrophage inflammation confers protection against CVB3-induced viral myocarditis. Front Cell Infect Microbiol. (2019) 9:57. doi: 10.3389/fcimb.2019.00057
89. Heemskerk MM, Giera M, Bouazzaoui FE, Lips MA, Pijl H, van Dijk KW, et al. Increased PUFA content and 5-lipoxygenase pathway expression are associated with subcutaneous adipose tissue inflammation in obese women with type 2 diabetes. Nutrients. (2015) 7:7676–90. doi: 10.3390/nu7095362
90. Pisano E, Pacifico L, Perla FM, Liuzzo G, Chiesa C, Lavorato M, et al. Upregulated monocyte expression of PLIN2 is associated with early arterial injury in children with overweight/obesity. Atherosclerosis. (2021) 327:68–75. doi: 10.1016/j.atherosclerosis.2021.04.016
91. Andrade S, Morais T, Sandovici I, Seabra AL, Constância M, Monteiro MP. Adipose tissue epigenetic profile in obesity-related dysglycemia - a systematic review. Front Endocrinol. (2021) 12:681649. doi: 10.3389/fendo.2021.681649
92. Sell H, Habich C, Eckel J. Adaptive immunity in obesity and insulin resistance. Nat Rev Endocrinol. (2012) 8:709–16. doi: 10.1038/nrendo.2012.114
93. McLaughlin T, Ackerman SE, Shen L, Engleman E. Role of innate and adaptive immunity in obesity-associated metabolic disease. J Clin Invest. (2017) 127:5–13. doi: 10.1172/JCI88876
94. Deng T, Lyon CJ, Minze LJ, Lin J, Zou J, Liu JZ, et al. Class II major histocompatibility complex plays an essential role in obesity-induced adipose inflammation. Cell Metab. (2013) 17:411–22. doi: 10.1016/j.cmet.2013.02.009
95. Klimcáková E, Roussel B, Márquez-Quiñones A, Kovácová Z, Kováciková M, Combes M, et al. Worsening of obesity and metabolic status yields similar molecular adaptations in human subcutaneous and visceral adipose tissue: decreased metabolism and increased immune response. J Clin Endocrinol Metab. (2011) 96:E73–82. doi: 10.1210/jc.2010-1575
96. Shaikh SR, Mitchell D, Carroll E, Li M, Schneck J, Edidin M. Differential effects of a saturated and a monounsaturated fatty acid on MHC class I antigen presentation. Scand J Immunol. (2008) 68:30–42. doi: 10.1111/j.1365-3083.2008.02113.x
97. Sayols-Baixeras S, Subirana I, Lluis-Ganella C, Civeira F, Roquer J, Do AN, et al. Identification and validation of seven new loci showing differential DNA methylation related to serum lipid profile: an epigenome-wide approach. The REGICOR study. Hum Mol Genet. (2016) 25:4556–65. doi: 10.1093/hmg/ddw285
98. Ligthart S, Marzi C, Aslibekyan S, Mendelson MM, Conneely KN, Tanaka T, et al. DNA methylation signatures of chronic low-grade inflammation are associated with complex diseases. Genome Biol. (2016) 17:255. doi: 10.1186/s13059-016-1119-5
99. Bodhini D, Gaal S, Shatwan I, Ramya K, Ellahi B, Surendran S, et al. Interaction between TCF7L2 polymorphism and dietary fat intake on high density lipoprotein cholesterol. PLoS ONE. (2017) 12:e0188382. doi: 10.1371/journal.pone.0188382
100. Yamada Y, Kato K, Oguri M, Horibe H, Fujimaki T, Yasukochi Y, et al. Identification of 12 novel loci that confer susceptibility to early-onset dyslipidemia. Int J Mol Med. (2019) 43:57–82. doi: 10.3892/ijmm.2018.3943
101. Agmon E, Stockwell BR. Lipid homeostasis and regulated cell death. Curr Opin Chem Biol. (2017) 39:83–9. doi: 10.1016/j.cbpa.2017.06.002
102. Chang HT, Liu CC, Chen ST, Yap YV, Chang NS, Sze CI, et al. domain-containing oxidoreductase in neuronal injury and neurological diseases. Oncotarget. (2014) 5:11792–9. doi: 10.18632/oncotarget.2961
103. Colin S, Fanchon M, Belloy L, Bochem AE, Copin C, Derudas B, et al. HDL does not influence the polarization of human monocytes toward an alternative phenotype. Int J Cardiol. (2014) 172:179–84. doi: 10.1016/j.ijcard.2013.12.168
104. Nofer JR, Kehrel B, Fobker M, Levkau B, Assmann G, von Eckardstein A, et al. and arteriosclerosis: beyond reverse cholesterol transport. Atherosclerosis. (2002) 161:1–16. doi: 10.1016/S0021-9150(01)00651-7
105. Yu XH, Fu YC, Zhang DW, Yin K, Tang CK. Foam cells in atherosclerosis. Clin Chim Acta. (2013) 424:245–52. doi: 10.1016/j.cca.2013.06.006
106. Dekkers KF, Neele AE, Jukema JW, Heijmans BT, de Winther MPJ. Human monocyte-to-macrophage differentiation involves highly localized gainand loss of DNA methylation at transcription factor binding sites. Epigenetics Chromatin. (2019) 12:34. doi: 10.1186/s13072-019-0279-4
107. Lawrence T, Natoli G. Transcriptional regulation of macrophage polarization: enabling diversity with identity. Nat Rev Immunol. (2011) 11:750–61. doi: 10.1038/nri3088
108. Zeng P, Shao Z, Zhou X. Statistical methods for mediation analysis in the era of high-throughput genomics: current successes and future challenges. Comput Struct Biotechnol J. (2021) 19:3209–24. doi: 10.1016/j.csbj.2021.05.042
109. Liu Z, Shen J, Barfield R, Schwartz J, Baccarelli AA, Lin X. Large-scale hypothesis testing for causal mediation effects with applications in genome-wide epigenetic studies. J Am Stat Assoc. (2021) 117:1–15. doi: 10.1101/2020.09.20.20198226
110. Huang YT, Chu S, Loucks EB, Lin CL, Eaton CB, Buka SL, et al. Epigenome-wide profiling of DNA methylation in paired samples of adipose tissue and blood. Epigenetics. (2016) 11:227–36. doi: 10.1080/15592294.2016.1146853
111. Winkleby MA, Jatulis DE, Frank E, Fortmann SP. Socioeconomic status and health: how education, income, and occupation contribute to risk factors for cardiovascular disease. Am J Public Health. (1992) 82:816–20. doi: 10.2105/AJPH.82.6.816
112. Metcalf P, Scragg R, Davis P. Relationship of different measures of socioeconomic status with cardiovascular disease risk factors and lifestyle in a New Zealand workforce survey. N Z Med J. (2007) 120:U2392.
113. Caldwell JC. Education as a factor in mortality decline an examination of Nigerian data. Popul Stud. (1979) 33:395–413. doi: 10.2307/2173888
114. Aslam M, Kingdon GG. Parental education and child health—understanding the pathways of impact in Pakistan. World Dev. (2012) 40:2014–32. doi: 10.1016/j.worlddev.2012.05.007
115. VanderWeele T. Explanation in Causal Inference: Methods for Mediation and Interaction. New York, NY: Oxford University Press (2015).
116. Mathieu JE, Taylor SR. Clarifying conditions and decision points for mediational type inferences in organizational behavior. J Org Behav. (2006) 27:1031–56. doi: 10.1002/job.406
117. Song Y, Zhou X, Zhang M, Zhao W, Liu Y, Kardia SLR, et al. Bayesian shrinkage estimation of high dimensional causal mediation effects in omics studies. Biometrics. (2019). doi: 10.1101/467399
118. Song Y, Zhou X, Kang J, Aung MT, Zhang M, Zhao W, et al. Bayesian hierarchical models for high-dimensional mediation analysis with coordinated selection of correlated mediators. Stat Med. (2021) 40:6038–56. doi: 10.1002/sim.9168
Keywords: social epigenomics, socioeconomic status, adversity, epigenome-wide association study, obesity, diabetes, hypertension
Citation: Wang YZ, Zhao W, Ammous F, Song Y, Du J, Shang L, Ratliff SM, Moore K, Kelly KM, Needham BL, Diez Roux AV, Liu Y, Butler KR, Kardia SLR, Mukherjee B, Zhou X and Smith JA (2022) DNA Methylation Mediates the Association Between Individual and Neighborhood Social Disadvantage and Cardiovascular Risk Factors. Front. Cardiovasc. Med. 9:848768. doi: 10.3389/fcvm.2022.848768
Received: 05 January 2022; Accepted: 29 April 2022;
Published: 19 May 2022.
Edited by:
Abhijeet R. Sonawane, Brigham and Women's Hospital and Harvard Medical School, United StatesReviewed by:
Julian Hecker, Harvard University, United StatesKarl Kelsey, Brown University, United States
Copyright © 2022 Wang, Zhao, Ammous, Song, Du, Shang, Ratliff, Moore, Kelly, Needham, Diez Roux, Liu, Butler, Kardia, Mukherjee, Zhou and Smith. This is an open-access article distributed under the terms of the Creative Commons Attribution License (CC BY). The use, distribution or reproduction in other forums is permitted, provided the original author(s) and the copyright owner(s) are credited and that the original publication in this journal is cited, in accordance with accepted academic practice. No use, distribution or reproduction is permitted which does not comply with these terms.
*Correspondence: Jennifer A. Smith, c21qZW5uQHVtaWNoLmVkdQ==; orcid.org/0000-0002-3575-5468