- 1Department of Exercise Science, Syracuse University, Syracuse, NY, United States
- 2Department of Kinesiology, Iowa State University, Ames, IA, United States
- 3Department of Exercise and Sport Science, University of North Carolina, Chapel Hill, NC, United States
- 4Department of Epidemiology, Gilling's School of Public Health, University of North Carolina at Chapel Hill, Chapel Hill, NC, United States
- 5Department of Emergency Medicine, School of Medicine, University of North Carolina at Chapel Hill, Chapel Hill, NC, United States
Background: The interactions between large artery function and neurovascular coupling (NVC) are emerging as important contributors to cognitive health. Women are disproportionally affected by Alzheimer's disease and related dementia later in life. Understanding large artery correlates of NVC in young women may help with preservation of cognitive health with advancing age.
Purpose: To explore the association between large artery function, NVC and cognitive performance in young women.
Methods: Vascular measurements were made in 61 women (21 ± 4 yrs) at rest and during a cognitive challenge (Stroop task). Transcranial Doppler was used to measure left middle cerebral artery (MCA) maximum velocity (Vmax), mean velocity (Vmean), and pulsatility index (PI). NVC was determined as MCA blood velocity reactivity to the Stroop task. Large artery function was determined using carotid-femoral pulse wave velocity (cfPWV) as a proxy measure of aortic stiffness and carotid ultrasound-derived measures of compliance and reactivity (diameter change to the Stroop task). Cognitive function was assessed separately using a computerized neurocognitive battery that included appraisal of response speed, executive function, information processing efficiency, memory, attention/concentration, and impulsivity.
Results: MCA Vmax reactivity was positively associated with executive function (β = 0.26, 95% CI 0.01–0.10); MCA Vmean reactivity was negatively associated with response speed (β = −0.33, 95% CI −0.19 to −0.02) and positively with memory score (β = 0.28, 95% CI 0.01–0.19). MCA PI reactivity was negatively associated with attention performance (β = −0.29, 95% CI −14.9 to −1.0). Path analyses identified significant paths (p < 0.05) between carotid compliance and carotid diameter reactivity to select domains of cognitive function through MCA reactivity.
Conclusions: NVC was associated with cognitive function in young women. Carotid artery function assessed as carotid compliance and carotid reactivity may contribute to optimal NVC in young women through increased blood flow delivery and reduced blood flow pulsatility.
Introduction
Women are disproportionally affected by Alzheimer's disease and related dementia (ADRD) with advancing age (1) and rates of later life cognitive decline may be faster in women compared to men (2). Optimal cognitive function is dependent upon the vascular response to cognitive engagement (3); that is, a reactive cerebrovasculature ensures rapid redirection of cerebral blood flow (CBF) supply to regions of increased neuronal demand (3). The matching of cerebral supply to neuronal metabolic demand is known as neurovascular coupling (NVC). Impaired NVC contributes to development of cognitive decline and may precipitate the development of ADRD (4, 5). Thus, exploration of determinants of NVC in young women may offer insight into sex-specific mechanisms governing preservation of cognitive reserve across the lifespan (6, 7).
NVC is directly dependent on large artery structure and function. The aorta and carotid arteries are inherently elastic large arteries, serving important roles as hemodynamic buffers. As such, the stiffening of these large arteries affects not only the absolute amount of blood flow delivered to the brain during cognitive engagement via the cerebral circuit, but the manner in which that flow is delivered (8). Specifically, large artery stiffness begets high pulsatile hemodynamic transmission into the microvasculature of the brain (9, 10). As the brain operates at low vascular impedance, impaired pulsatile damping to the cerebral microvasculature inflicts cerebrovascular damage, dysfunction, and corresponding cognitive decline (9, 10). There are prominent sex differences in large artery stiffness and cerebral pulsatility, with women experiencing greater increases in large artery stiffness and cerebral pulsatility, and reduced pulsatile damping, across the lifespan compared to men (11, 12). There is currently a knowledge gap regarding the influence of large artery stiffness and cerebral pulsatility on NVC and, in turn, cognitive performance in young women.
Therefore, the aims of this exploratory study were to examine: (1) the associations of large artery structure and function with NVC in young women; and (2) potential paths from large artery stiffness to cognitive function through NVC using path analysis. We hypothesized that (1) large artery stiffness would be associated with NVC in young women; and (2) significant paths would be identified between large artery stiffness, NVC, and cognitive function in young women. Findings may provide insight into the role of large artery structure and function as regulators of NVC and cognitive function in young women, offering new potential targets for preservation of cognitive healthspan and prevention of cognitive decline later in life.
Methods
Participants
Participants for this study included young women (n = 61) between 18 and 35 years of age that were recruited from the local university and surrounding community. All women were free from cardiorespiratory and cerebrovascular diseases, metabolic diseases, renal diseases, and mild cognitive impairment (determined via the Montreal Cognitive Assessment). None of the participants used antihypertensive medication. Prior to study enrollment, all participants provided written informed consent and this study was approved by the Syracuse University Institutional Review Board.
Study design and overview
Participants completed this cross-sectional study following two visits to the Human Performance Laboratory. Prior to the first visit, participants were asked to fast for 3 h. The first visit consisted of screening for mild cognitive impairment with the Montreal Cognitive Assessment (MOCA score < 26), anthropometric assessment, and familiarization with all vascular testing. Upon completing the first visit, participants were provided with an accelerometer to objectively assess physical activity levels. The second visit was scheduled ~9 days later and during the early follicular phase of the menstrual cycle. Participants arrived at the laboratory following a 12-h overnight fast. During the second visit, a finger-stick blood sample was used to obtain traditional cardiovascular and metabolic risk factors (i.e., fasting glucose and blood lipids). Participants then underwent vascular testing while in the supine position (baseline) and during two separate 3-min cognitive challenges. Vascular measures included peripheral and central blood pressure (BP), measures of aortic and carotid structure and function, and middle cerebral artery blood velocity. Following vascular-hemodynamic assessment, a 30-min computerized neurocognitive battery was administered. Participants then completed an online health history questionnaire. Both subjective factors (self-reported health history) and objective factors (body composition, body mass index [BMI], physical activity and traditional cardiovascular disease risk factors) were acquired to characterize general health status of participants.
Health screening
Participants completed a detailed online health history and sociodemographic questionnaire using REDCap (Vanderbilt University; Nashville, TN, USA).
Anthropometrics and body composition
Height was measured using a digital stadiometer (SONARIS, Detecto; Webb City, MO, USA). Weight was measured with a digital scale. Air displacement plethysmography (BodPod, Cosmed; Rome, Italy) was performed to determine body fat percentage. Healthy weight was operationally defined as a BMI between 18.5 and 24.9 kg/m2. Overweight was operationally defined as a BMI between 25 and 29.9 kg/m2 and obese class I was operationally defined as a BMI between 30 and 34.9 kg/m2.
Blood sampling
A finger stick blood sample was obtained to analyze fasting lipids and glucose using a validated point-of-care device (Cholestech LDX analyzer, Abbott Laboratories; Chicago, IL, USA).
Physical activity measurement
Participants wore an accelerometer (wGT3X, ActiGraph LLC; Pensacola, FL, USA) around the waist for 9 consecutive days to assess physical activity levels using cut-points developed by Freedson et al. (13). A complete day of accelerometer use was defined as at least 10 h of wear time while awake, which is consistent with the minimum set by the NHANES20, and a minimum of 4 days of wear data was necessary in order for participants to be included in data analysis. A cut point of 2020 activity counts/minute was used to determine the amount of time participants engaged in moderate-to-vigorous physical activity (MVPA) level.
Brachial blood pressure
Prior to any vascular testing, participants rested for 10-min in the supine position to ensure a stable hemodynamic state (i.e., two systolic and diastolic blood pressure measures within 5 mmHg). Brachial systolic blood pressure (SBP) and diastolic blood pressure (DBP) were measured at rest according to the American Heart Association (AHA) standards (14) using a validated, automated, oscillometer device (BP786N, OMRON Healthcare, Inc., Lake Forest, IL, USA).
Carotid thickness, stiffness, and compliance
Carotid thickness, stiffness and compliance were measured to assess large artery structure and function, respectively. Carotid artery intima-media thickness (IMT), stiffness, and compliance were assessed using ultrasound (ProSound α7, Aloka; Tokyo, Japan) and a 7.5–10.0 MHz linear-array probe positioned laterally ~1 cm below the left carotid bulb. Carotid IMT was analyzed from a 10-mm longitudinal view of the far wall of the artery (obtained parallel/horizontal to the probe) as the distance from the intima lumen interface to the media adventitial border using electronic calipers and semi-automatic inbuilt software. IMT measures were obtained in triplicate during diastole determined from simultaneous ECG (R-wave) gating and averaged for subsequent analyses. Carotid distension waves were then captured across the cardiac cycle using eTracking software from the same longitudinal image as used for IMT appraisal. Carotid beta-stiffness was calculated as ln(Pmax/Pmin)/[(Dmax-Dmin)/Dmin], where P denotes carotid pressure (measured simultaneously) and D corresponds to carotid diameter. Carotid compliance was calculated as the change in area calculated from diameter across the cardiac cycle relative to the change in pressure (Areamax-Areamin/ Pmax-Pmin). Max and min refer to maximum (systolic) and minimum (diastolic) values throughout the cardiac cycle, respectively, determined from simultaneous ECG gating. ECG was also used to determine heart rate (HR). Carotid pressure waveforms were captured simultaneously via applanation tonometry (SphygmoCor, AtCor Medical; Sydney, Australia) for a 10-s epoch and a composite waveform was produced. This composite waveform was calibrated to brachial DBP, and mean blood pressure (MBP, calculated as 1/3 SBP + 2/3 DBP) based on the assumed stability of these pressures throughout the systemic circulation to determine carotid SBP (cSBP) and used for aforementioned calculations of carotid stiffness and compliance.
Aortic stiffness
Carotid-femoral pulse wave velocity was used as proxy of aortic stiffness and taken as a measure of large artery function. Aortic and carotid stiffness are not redundant measures as each is a different vascular bed with differential associations with brain structure and function. Applanation tonometry (SphygmoCor, AtCor Medical; Sydney, Australia) was used to sequentially capture right carotid and right femoral pulse waveforms. The distance between each pulse site and the suprasternal notch was obtained using a tape measure, and the aortic path length was estimated by subtracting the carotid-suprasternal notch distance from the femoral-suprasternal notch distance. Carotid-femoral pulse wave velocity (cfPWV) was then calculated as the aortic path length divided by the time delay between ventricular contraction and the generation of the foot of the pressure waveform at each pulse site (15).
Cerebral blood velocity
We measured left middle cerebral artery (MCA) mean velocity (Vmean), maximum velocity (Vmax), and pulsatility index (PI) to explore cerebral hemodynamic factors that reflect NVC. A 2 MHz transcranial Doppler probe (DWL Doppler Box-X, Compumedics; Singen, Germany) was placed at the left temporal window and signals were recorded at a depth of 45–60 mm. Vmean is conventionally used for the appraisal of NVC (16). However, PI may provide additional insight into NVC given its relation to arterial stiffness and distal/terminal cerebrovascular resistance. PI was calculated as Vmax-Vmin/Vmean. Likewise, inclusion of Vmax may complement Vmean and PI when appraising NVC. Specifically, Vmax is: (1) strongly associated with systolic velocity in the aorta (17), (2) qualitatively similar to phase contrast magnetic resonance angiography when assessing cerebrovascular reactivity (18), and (3) less influenced by Doppler artifact than measures that include diastolic velocity (Vmin) (16).
Neurovascular coupling
Vascular-hemodynamic parameters were assessed during two separate computerized 3- min Stroop color-word tasks (E-Prime, Psychology Software Tools Inc., Sharpsburg PA) following 10-min of supine rest. The Stroop test consisted of congruent (lower cognitive load) and incongruent (higher cognitive load) tasks that were performed in a randomized order. The congruent task included words appearing in the same color as the target word itself (i.e., the word blue, written in blue), whereas the incongruent task comprised words appearing in a color incongruous with the target word (i.e., the word blue, written in red). During both tasks, participants selected one of the four response items that described the color of the target word as fast and as accurately as possible using a remote clicker, thus avoiding speaking and distortion of Doppler signals. Participant accuracy was adjusted to ~50% by manipulating response time intervals between trials to standardize cognitive load between participants and prevent task disengagement for specifically high or low performing individuals. The response time interval between trials would shorten by 300 ms for every three successive trials responded to correctly and vice-versa for incorrect answers. The response time ranged from 400 to 5,000 ms. For this study, we operationally defined NVC as change in MCA hemodynamics (Vmean, Vmax, and PI) from rest to incongruent Stroop (19). The incongruent Stroop was used as our previous work has demonstrated this task to elicit a more robust hemodynamic response compared to the congruent Stroop (19, 20). Baseline MCA measures were calculated from the average of 4 consecutive 10-s epochs. Similarly, we averaged MCA measures from 4 consecutive 10-s epochs during Stroop tasks. MCA recordings were started ~30-s after initiation of each Stroop task to ensure a steady state was reached. Stroop tasks were separated by 4-min of quiet rest to ensure heart rate and blood pressure had returned to resting values (20). Additionally, we took change in carotid diameter (Dmin) from rest to incongruent Stroop as a measure of carotid artery reactivity (21).
Cognitive function battery
Following assessment of NVC, participants completed a 30-min WebNeuro computerized neuropsychological battery (Brain Resource WebNeuro, Total Brain; San Francisco, CA, USA) to test several domains of cognitive function. Participants completed tasks in the seated position with a standard mouse and keyboard. The content, convergent and divergent construct validity (22, 23) as well as test–retest reliability of WebNeuro have been established previously (24). The domains of cognitive function used in the current study included executive function, information processing efficiency, memory, attention/concentration, and impulsivity (23). These domains of cognition were tested by finger tapping, choice reaction time, memory recognition (verbal list-learning, immediate forced choice recall, and delayed forced choice recall), forward digit span, verbal interference, switching of attention, go/no-go (impulsivity), delayed memory recognition, sustained attention, and executive maze tasks.
WebNeuro data is linked to the Brain Resource International Database that takes age, self-reported biological sex, and education of participants into account when individual differences in scores are compared (23). Following each cognitive test, WebNeuro generates a summary of raw score metrics for all cognitive domains that are computed by creating the average of individual test scores (25). In order to standardize averaging across different units of measurement for each cognitive domain, raw scores were converted to norm-referenced z-scores, which were then averaged and reported as “standard ten” (STEN) scores (25). STEN scores range from 1 to 10 with a mean of 5.5 and standard deviation of 2 (26), indicating that a participant with a Mean ± SD of 5.5 ± 2 falls on the average score of the norm population. When we examined the relationship between cognitive function domains and hemodynamic responses, these STEN scores were used as representatives of cognitive outcomes in the statistical models.
Statistical analyses
Assumptions of normality were assessed quantitatively using the Shapiro-Wilk test, and qualitatively via Q-Q plots and histograms. All data are reported as mean ± standard deviation for continuous data and as n (%) for categorical data. After testing normality of distribution, we first sought to confirm the efficacy of the Stroop task in eliciting a hemodynamic response in young women. To do so, a repeated measures analysis of variance was performed to determine changes in hemodynamic parameters from baseline to the congruent and incongruent Stroop tasks. Bonferroni-corrected alpha levels were utilized to limit type I error from multiple comparisons. Effect sizes were calculated for differences across time points as partial η2 (eta squared).
Next, we determined cerebral hemodynamic contributors (both resting and reactivity separately) to the different domains of cognitive function via block-wise linear regression. Separate models examined the contribution of baseline (resting) cerebral hemodynamics and cerebral reactivity on all domains of cognitive function, with cerebral reactivity calculated as described above as MCA Vmean, Vmax, and PI during baseline (resting) subtracted from values measured during the incongruent Stroop task. Given the importance of perfusion pressure for cerebral blood flow, we entered MBP as a covariate in the first block for each model. When exploring resting cerebral factors as predictors of cognitive function, resting MBP was entered in the first block. When exploring cerebral reactivity factors as predictors of cognitive function, MBP reactivity was entered in the first block. MCA Vmean, Vmax, and PI reactivity were then entered into a second block using the stepwise function.
Path analysis was used to explore cerebral hemodynamic paths through which large artery structure and/or function might affect cognitive function (see Figure 1, Theoretical Model). To this end, we first examined strength of univariate associations of cfPWV, carotid beta-stiffness, carotid compliance, carotid reactivity and carotid IMT with cerebral reactivity measures using Pearson product-moment correlation coefficients to identify target measures of large artery structure and function for our subsequent paths associated with cognitive domains. We then constructed several paths and tested several model fit parameters, including: goodness of fit index (GFI), adjusted (for degrees of freedom) goodness of fit index (AGFI), normal fit index (NFI), relative fit index (RFI), comparative fit index (CFI), root mean square error of approximation (RMSEA), and Chi-square test. Fit was considered good if GFR, AGFI, NFI, RFI, and CFI were >0.95, RMSEA <0.05 and the p-value for the Chi-square test was > 0.05. For all other statistical tests, significance was established as p < 0.05. Data were analyzed using IBM SPSS Statistics and AMOS packages (v.27.0 Armonk, NY, USA).
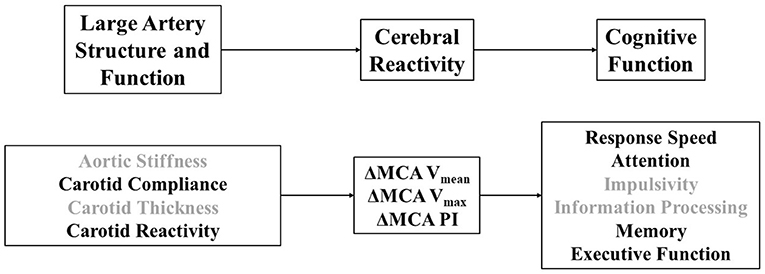
Figure 1. (Top) Theoretical model linking large artery structure (i.e., carotid intima-media thickness) and function (i.e., aortic stiffness, carotid stiffness, carotid compliance, carotid reactivity) to cerebral reactivity (MCA, middle cerebral artery; Vmean, mean blood velocity; Vmax, maximum blood velocity; PI, pulsatility index) and cognitive function. (Bottom) Operational definitions of specific physiological and cognitive constructs explored in this study that relate to our theoretical model. Darker font signifies variables that emerged as correlates across paths.
Results
Participants comprised a group a young women with intact cognitive function as evidenced by all MOCA scores being equal to or exceeding 26 (Table 1). Sixty-five percent of women had a healthy weight, 28% were considered overweight, and 7% were considered obese class I. According to accelerometry, 67% of participants were meeting or exceeding physical activity guideline recommendations. Women, on average, were completing 8,699 ± 2,297 steps per day. None self-reported a past diagnosis of CVD, diabetes, pulmonary disease, renal disease, hypertension, or dyslipidemia and none smoked, confirming our exclusion criteria. Five women reported a family history of CVD (1st degree relative/parent). None self-reported being amenorrheic and 29% were using oral contraceptives (mean duration 3.5 ± 3.0 years). All had fasting lipids and glucose within clinically acceptable ranges (Table 1).
There were stepwise increases in HR, SBP, DBP, and MBP from baseline to the congruent and incongruent Stroop (p < 0.001) confirming that an increase in cognitive load subsequently elicited an increase in hemodynamic load (Table 2). All changes in HR and BP had large effect sizes (partial η2 ≥ 0.24). Similarly, a stepwise increase in MCA blood velocities was observed from baseline to congruent and from congruent to incongruent Stroop tasks (Figure 2, p < 0.001). Large effect sizes were observed for increases in MCA Vmax and Vmean across the study timepoints. MCA PI was reduced from baseline to congruent Stroop, and further reduced from congruent to incongruent Stroop (Figure 2, p < 0.001) also producing a large effect size. Finally, there were stepwise increases in cfPWV from baseline to congruent Stroop and again to incongruent Stroop (p = 0.003) as well as stepwise increases carotid artery diameter from baseline to congruent Stroop and again to incongruent Stroop (p = 0.001), with small to modest effect sizes noted. There was no change in carotid artery compliance or carotid beta-stiffness across conditions (p > 0.05).
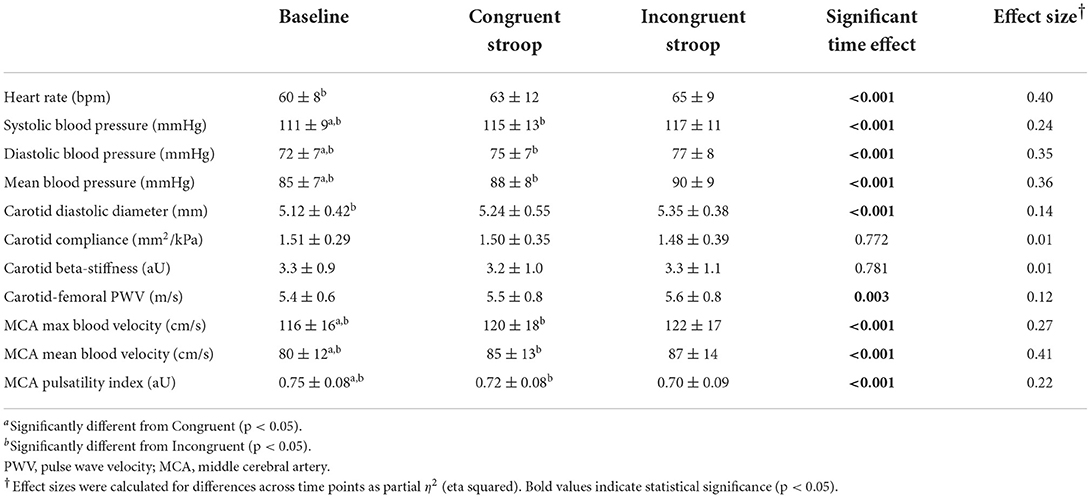
Table 2. Changes in hemodynamics and vascular function during cognitive challenge (n = 61, Mean ± SD).
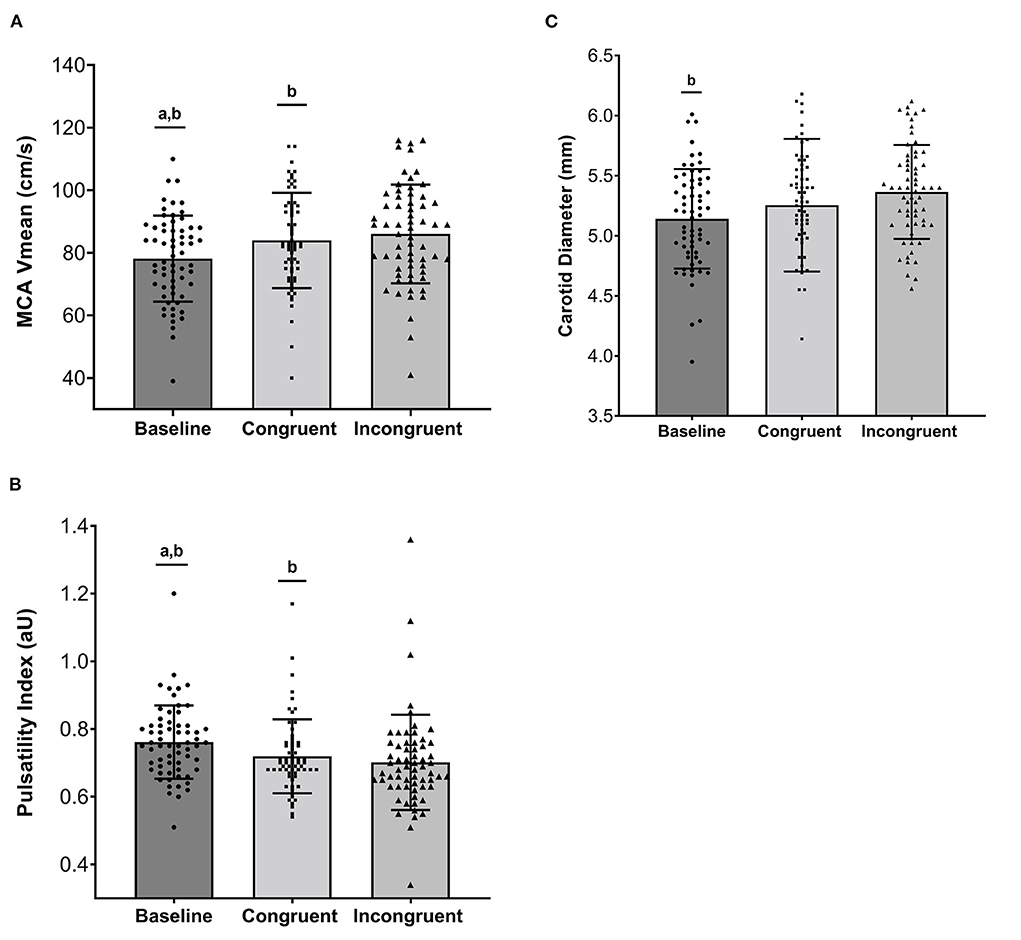
Figure 2. (A) Individual and mean ± standard deviation values for Middle Cerebral Artery Mean Blood Velocity (MCA Vmean, cm/s), (B) Middle Cerebral Artery Pulsatility Index (aU), and (C) Carotid Diameter (mm) across Baseline, Congruent Stroop, and Incongruent Stroop. aSignificantly different from Congruent (p < 0.05); bSignificantly different from Incongruent (p < 0.05).
MCA reactivity to the incongruent Stroop task revealed significant associations with select domains of cognitive function, specifically response speed, memory, and executive function. Parsimonious models from stepwise regression are presented in Table 3 (variables that fell out of each model are not presented). Variance inflation factors for all models presented in Table 3 fell between 1.05 and 1.77 suggesting minimal concern for collinearity. Baseline (resting) cerebral measures (MCA Vmax, Vmean, and PI), were not associated with any domain of cognitive function (p > 0.05 for all, Supplementary Table 1).
Results for path analyses are displayed in Figure 3. Parameters were included in the model based on initial univariate correlations and a theoretical model that broadly conceptualizes large artery structure/function affecting cerebral hemodynamics, in turn affecting cognitive function (Figure 1). Univariate correlations between measures of vascular function and NVC are presented in Supplementary Table 1. First, we explored paths from carotid compliance and MCA Vmean reactivity to response speed and memory. The path from carotid compliance to MCA Vmean reactivity approached significance (β = 0.24, p = 0.052) while the path from MCA Vmean reactivity to response speed achieved significance (β = −0.34, p = 0.003). The model fit the data moderately well (GFI = 0.96, AGFI = 0.75, NFI = 0.75; RFI = 0.24, CFI = 0.765; RMSEA = 0.22; Chi-square = 3.975, p = 0.046). The path from MCA Vmean reactivity to memory also achieved significance (β = 0.28, p = 0.022). Goodness of fit was high for the path from carotid compliance to MCA Vmean reactivity to memory (GFI = 0.99, AGFI = 0.95, NFI = 0.92; RFI = 0.74, CFI = 1.00; RMSEA = 0.00; Chi-square = 0.813, p = 0.367). Next, we explored a path from carotid compliance to MCA Vmax reactivity and executive function. Significant paths from carotid compliance to MCA Vmax reactivity (β = 0.32, p = 0.008) and MCA Vmax reactivity to executive function (β = 0.26, p = 0.038) were identified. The model fit the data well (GFI = 0.99, AGFI = 0.94, NFI = 0.93; RFI = 0.78, CFI = 1.00; RMSEA = 0.00; Chi-square = 0.857, p = 0.355). Finally, we explored a path from carotid reactivity to MCA PI reactivity and attention. Significant paths from carotid reactivity to MCA PI reactivity (β = −0.31, p = 0.013) and MCA PI reactivity to attention/concentration (β = −0.29, p = 0.021) were identified. The model fit the data well (GFI = 0.99, AGFI = 0.98, NFI = 0.98; RFI = 0.93, CFI = 1.00; RMSEA = 0.00; Chi-square = 0.265, p = 0.607).
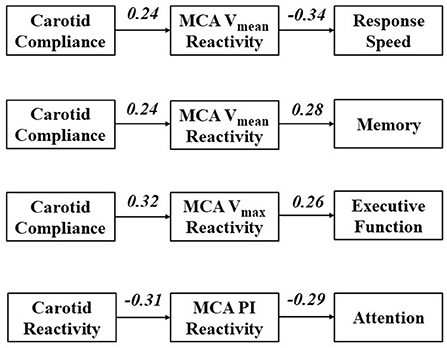
Figure 3. Path analyses exploring cross-sectional paths through which carotid artery function affects cerebral reactivity, in turn affecting domains of cognitive function. Values represent standardized regression weights.
Discussion
We identified different cerebral pathways through which large artery function may affect select domains of cognitive function in young women. Our main findings can be broadly summarized as follows: (1) measures of carotid artery function appraised as carotid artery compliance and carotid reactivity are significant correlates of NVC in young women and (2) NVC is associated with response speed, memory, attention and executive function in young women. Taken together, our findings support an association between NVC and cognitive function in young women and suggest optimal NVC may depend on a compliant carotid artery effectively dilating during times of cognitive engagement to accommodate increased flow delivery, ensuring cerebrovascular perfusion with minimal pulsatility.
CBF reactivity offers insight into cognitive function not captured by resting measures
Resting CBF, particularly hypoperfusion, has been shown to predict cognitive function in older adults (27, 28) with poor cerebrovascular function serving as an early marker of cognitive decline in older women (29). Interestingly, resting measures of CBF were not associated with cognitive function in young women in our study. We found that cerebral reactivity assessed during a cognitive challenge (our measure of NVC) had positive associations with the cognitive domains of response speed, memory, attention and executive function. Our results are consistent with previous work from Csipo et al. finding that increased cognitive workload is needed to evoke greater NVC responses in healthy young adults (30). Thus, in healthy women, increasing cognitive demand with a cognitive challenge like Stroop may stress cognitive reserves, revealing associations between cerebral perfusion and cognitive function not detected in a quiescent state. More research will be needed to unravel the clinical merit of studying NVC as studied herein as a potential biomarker of cerebrovascular health and cognitive performance.
CBF PI reactivity is an important component of NVC
We observed that CBF pulsatility reactivity was negatively associated with the cognitive domain of attention in young women. Previous findings demonstrated a positive correlation between pulsatility and the severity of cognitive dysfunction in patients with dementia (4), mild cognitive impairment (31), AD (32), cerebral white matter disease (33), stroke, and early vascular dementia (34). The microcirculation in areas of the brain associated with long-term memory is especially vulnerable to pulsatile stress (as assessed by MCA PI) and is altered early in aging independent of demographic and cardiovascular covariates (35, 36). Our findings did not show a connection between pulsatility and memory scores, but it is important to note that attention and memory are intricately linked as attention is of vital importance for the encoding and recovery of long-term memory (37, 38). Additionally, a recent review reports that pulsatility and cognition associations eventually spread to other cognitive domains with increasing age (39). Chronic pulsatility is implicated as a detrimental hemodynamic factor contributing to cerebral microbleeds and ischemia over time (9, 10). In an acute setting, CBF pulsatility may affect oxygen delivery to the neurovascular unit, impacting neuronal function and thus cognitive performance (19). Our findings support the contention that pulsatility may be an important hemodynamic factor to measure when assessing NVC (40, 41). Longitudinal studies will be needed to determine if the association between acute changes CBF pulsatility during cognitive challenge and lower attention scores noted in our work could be indicative of compromised long-term memory later in life.
Aortic stiffness is not associated with NVC in young women
Another noteworthy finding in the current study was that aortic stiffness was not associated with NVC in young women. Several studies in the literature indicate that vascular function as examined by aortic stiffness contributes to alterations in CBF and CBF pulsatility (42–44). Reasons for the discrepancy between our findings and others (42–44) are not clear but may be attributed to differences in age. Alterations in aortic stiffness demonstrate a gradual and incremental (non-linear) increase with advancing age in healthy individuals (45). However, women experience drastic increases in aortic stiffness at ~55–75 years of age compared to age-matched men, corresponding to the post-menopausal period (46). Parallel to substantial aortic stiffening, older women also have less pulsatile damping between the carotid and cerebral arteries compared with age-matched men, illustrating a higher transmission of pulsatile energy into the brain of older women (11, 12). This may be associated with dysfunction of the cerebral endothelial cells, a more permeable blood-brain barrier, neurovascular uncoupling, reduced CBF, and increased neuropathology (47). We suggest that the interaction of vascular aging and menopause-induced deterioration in arterial function might make the association between aortic stiffness and cognitive performance more prominent in later life. Overall, our findings suggest that aortic stiffness is not associated with cerebral reactivity and NVC in young women. Further research is needed to explore possible links between healthy vascular aging (i.e., low aortic stiffness across the lifespan) and cognitive reserve.
Carotid artery function contributes to NVC in young women
While aortic stiffness was not associated with NVC in our study, our results showed that functional properties of the carotid artery were contributors to NVC. Functional vascular properties are those operationally defined as being actively modified over a short period of time. Such dynamic changes can be mediated by a variety of hemodynamic and autonomic factors affecting vascular smooth muscle cell (VSMC) tone and acute changes in interactions between VSMCs, elastin, collagen and the extracellular matrix impacting mechanical load bearing properties of the vessel wall. We noted an association between carotid compliance and carotid reactivity with CBF reactivity in young women such that greater carotid compliance and reactivity was associated with greater CBF reactivity and lower pulsatility, respectively. The change in carotid artery diameter during cognitive challenge has been suggested to be influenced by carotid endothelial function (21). This is important to note as endothelial dysfunction has been implicated in neurovascular uncoupling in older adults (6, 48). Moreover, the strength of association with NVC was slightly greater for carotid compliance vs. carotid stiffness. Compared to compliance, carotid stiffness parameters are thought to be less sensitive to the effects of blood pressure, and thus more closely approximate intrinsic vessel wall properties, however this belief has been challenged (49). Thus, some of the effect of carotid compliance on NVC may be pressure-mediated. Overall, our findings support a growing consensus that some cerebrovascular regulation occurs at the level of the carotid artery (50). The carotid artery is not a passive conduit but a dynamic contributor to NVC. We suggest that for optimal NVC, a compliant and reactive carotid artery dilates during cognitive engagement to ensure that increases in CBF occur without excessive increases in pulsatility.
Body fat is associated with carotid artery function in young women: Implications for NVC and cognitive function
Obesity is associated with structural and functional evidence of brain aging (51), impaired NVC (52) and reduced cognitive function in midlife (53). In our study, while measures of body composition were not associated with NVC or cognitive function, higher body fat percentage was associated with lower carotid compliance and attenuated carotid reactivity. Our findings are consistent with a broader literature noting the detrimental impact of higher body fat on arterial stiffness and vascular function (54, 55). It is reasonable to speculate that the impact of body fat on carotid function in young women may have a cumulative detrimental effect on NVC and cognitive function later in life. Body fat-mediated effects of inflammation may impact NVC via direct effects on the neurovascular unit (i.e., endothelial dysfunction, perivascular astrocyte senescence, microglia dysfunction—all collectively contributing to altered cerebral dilation and perfusion) (56–58). Body fat may also have indirect effects on the neurovascular unit via the mediating role of carotid artery function on cerebrovascular pulsatility. Parenthetically, we noted an inverse association between physical activity and body fat in young women. This finding may be taken to underscore the importance of physical activity on cerebrovascular health (59, 60). In general physical activity, exercise, maintaining high cardiorespiratory fitness and select dietary interventions (e.g., time-restricted feeding, cocoa) may all favorably target the neurovascular unit and be considered therapeutic lifestyle interventions to attenuate vascular aging, preserve NVC and maintain cognitive reserve across the lifespan (58, 61–63).
Limitations and considerations
This study is subject to limitations. The cross-sectional design of our study does not allow us to establish causality or temporality of association. Hence, the results of correlations should be interpreted with caution. We performed the quantification of CBF through transcranial Doppler. Using transcranial Doppler for MCA blood velocity reactivity measurement has several advantages. Transcranial Doppler may be as accurate as computerized tomography and magnetic resonance angiography for the measurement of CBF in the basal cerebral arteries and their main branches including the MCAs (64). As the Doppler measures MCA blood velocity using the temporal window (the thinnest part of the skull in adults) (65), the potential interruption of ultrasound energy transmission from the Doppler due to bone thickness is also minimized. Nonetheless, transcranial Doppler has limitations for CBF reactivity measurement. The Doppler assumes that artery length, artery diameter, and blood viscosity are stable for velocity to be a substitute for CBF (66). Moreover, the Doppler signals can be influenced by the changes in the intracranial distal and extracranial proximal arteries (65). We examined unilateral MCA, thus, findings might not encompass the cognitive performance that could have been impacted by anterior, posterior and/or global CBF with bilateral measurement (67). Furthermore, the potential contribution of sex hormones and oral contraceptive use to vascular reactivity and cognitive function is unclear in our study as previous studies indicate estrogen- or progesterone-dependent changes in cerebrovascular hemodynamics among women (68, 69). Cognitive function, particularly executive function, may change during different phases of the menstrual cycle in women owing to the variations in the bioavailability of sex hormones (70, 71). We performed measurements during the early follicular phase of the menstrual cycle. Therefore, our findings may not capture a comprehensive picture of NVC in young women across the menstrual cycle with additional modulation by oral contraceptive use. Future studies that include men are needed to determine sex-specific effects of vascular function on NVC and cognitive function. Finally, although we included a diverse group of women in our study, we were underpowered to explore racial and ethnic variation in NVC and cognitive outcomes. Additional studies are warranted to explicitly explore the impact of race and ethnicity on vascular aging and NVC as potential mediators of racial and ethnic variation in cognitive aging.
Conclusion
Our findings suggest that cerebral reactivity during cognitive challenge offers insight into NVC and cognitive performance in young women. Increased CBF reactivity and decreased cerebral pulsatility during acute cognitive challenge are related to higher cognitive performance. Additionally, carotid artery functional properties, namely carotid compliance and carotid reactivity, are associated with CBF reactivity. Thus, optimal NVC requires a compliant carotid artery that dilates during the cognitive challenge to ensure that increased flow delivery to the cerebrovasculature occurs with minimal pulsatility. A less compliant artery with poor dilatory capacity may not adequately buffer hemodynamic pulsatility, resulting in impaired NVC and reduced cognitive performance.
Data availability statement
The raw data supporting the conclusions of this article will be made available by the authors, without undue reservation.
Ethics statement
The studies involving human participants were reviewed and approved by Syracuse University IRB. The patients/participants provided their written informed consent to participate in this study.
Author contributions
BC contributed to statistical analyses, results interpretation, and manuscript writing and reviewing. JD contributed to study design conceptualization, data collection, statistical analyses, results interpretation, and manuscript reviewing. WL and MM contributed to results interpretation and manuscript reviewing. AK contributed to data collection. PPL contributed to data collection and manuscript reviewing. LS contributed to statistical analyses, results interpretation, and manuscript reviewing. KH contributed to study design conceptualization, statistical analyses, results interpretation, manuscript writing and reviewing, and acquisition of funding. All authors contributed to the article and approved the submitted version.
Funding
Funding for this study was provided by the National Institutes of Health – National Institute of Minority Health and Health Disparities (NIMHD R03MD011306).
Acknowledgments
We thank Dr. Tiago Barreira for analyzing accelerometer data.
Conflict of interest
The authors declare that the research was conducted in the absence of any commercial or financial relationships that could be construed as a potential conflict of interest.
Publisher's note
All claims expressed in this article are solely those of the authors and do not necessarily represent those of their affiliated organizations, or those of the publisher, the editors and the reviewers. Any product that may be evaluated in this article, or claim that may be made by its manufacturer, is not guaranteed or endorsed by the publisher.
Supplementary material
The Supplementary Material for this article can be found online at: https://www.frontiersin.org/articles/10.3389/fcvm.2022.914439/full#supplementary-material
References
1. Dubal DB. Sex difference in Alzheimer's disease: an updated, balanced and emerging perspective on differing vulnerabilities. Handb Clin Neurol. (2020) 175:261–73. doi: 10.1016/B978-0-444-64123-6.00018-7
2. Levine DA, Gross AL, Briceño EM, Tilton N, Giordani BJ, Sussman JB, et al. Sex differences in cognitive decline among US adults. JAMA Network Open. (2021) 4:e210169. doi: 10.1001/jamanetworkopen.2021.0169
3. Presa JL, Saravia F, Bagi Z, Filosa JA. Vasculo-neuronal coupling and neurovascular coupling at the neurovascular unit: impact of hypertension. Front Physiol. (2020) 11:1233. doi: 10.3389/fphys.2020.584135
4. Silvestrini M, Pasqualetti P, Baruffaldi R, Bartolini M, Handouk Y, Matteis M, et al. Cerebrovascular reactivity and cognitive decline in patients with Alzheimer disease. Stroke. (2006) 37:1010–5. doi: 10.1161/01.STR.0000206439.62025.97
5. Sur S, Lin Z, Li Y, Yasar S, Rosenberg P, Moghekar A, et al. Association of cerebrovascular reactivity and Alzheimer pathologic markers with cognitive performance. Neurology. (2020) 95:e962–e72. doi: 10.1212/WNL.0000000000010133
6. Tarantini S, Tran CHT, Gordon GR, Ungvari Z, Csiszar A. Impaired neurovascular coupling in aging and Alzheimer's disease: contribution of astrocyte dysfunction and endothelial impairment to cognitive decline. Exp Gerontol. (2017) 94:52–8. doi: 10.1016/j.exger.2016.11.004
7. Duque C, Feske SK, Sorond FA. Cerebrovascular Hemodynamics in Women. Semin Neurol. (2017) 37:679–88. doi: 10.1055/s-0037-1608881
8. Heffernan KS, Augustine JA, Lefferts WK, Spartano NL, Hughes WE, Jorgensen RS, et al. Arterial stiffness and cerebral hemodynamic pulsatility during cognitive engagement in younger and older adults. Exp Gerontol. (2018) 101:54–62. doi: 10.1016/j.exger.2017.11.004
9. Chirinos JA, Segers P, Hughes T, Townsend R. Large-artery stiffness in health and disease: JACC state-of-the-art review. J Am Coll Cardiol. (2019) 74:1237–63. doi: 10.1016/j.jacc.2019.07.012
10. Mitchell GF, van Buchem MA, Sigurdsson S, Gotal JD, Jonsdottir MK, Kjartansson Ó, et al. Arterial stiffness, pressure and flow pulsatility and brain structure and function: the age, gene/environment susceptibility–Reykjavik study. Brain. (2011) 134:3398–407. doi: 10.1093/brain/awr253
11. Lefferts WK, DeBlois JP, Augustine JA, Keller AP, Heffernan KS. Age, sex, and the vascular contributors to cerebral pulsatility and pulsatile damping. J Appl Physiol. (2020) 129:1092–101. doi: 10.1152/japplphysiol.00500.2020
12. Alwatban MR, Aaron SE, Kaufman CS, Barnes JN, Brassard P, Ward JL, et al. Effects of age and sex on middle cerebral artery blood velocity and flow pulsatility index across the adult lifespan. J Appl Physiol. (2021) 130:1675–83. doi: 10.1152/japplphysiol.00926.2020
13. Freedson PS, Melanson E, Sirard J. Calibration of the computer science and applications, inc. accelerometer. Med Sci Sports Exerc. (1998) 30:777–81. doi: 10.1097/00005768-199805000-00021
14. Muntner P, Shimbo D, Carey RM, Charleston JB, Gaillard T, Misra S, et al. Measurement of blood pressure in humans: a scientific statement from the American Heart Association. Hypertension. (2019) 73:e35–66. doi: 10.1161/HYP.0000000000000087
15. Townsend RR, Wilkinson IB, Schiffrin EL, Avolio AP, Chirinos JA, Cockcroft JR, et al. Recommendations for improving and standardizing vascular research on arterial stiffness: a scientific statement from the american heart association. Hypertension. (2015) 66:698–722. doi: 10.1161/HYP.0000000000000033
16. Rosengarten B, Aldinger C, Kaufmann A, Kaps M. Comparison of visually evoked peak systolic and end diastolic blood flow velocity using a control system approach. Ultrasound Med Biol. (2001) 27:1499–503. doi: 10.1016/S0301-5629(01)00464-1
17. Aaslid R, Markwalder T-M, Nornes H. Noninvasive transcranial Doppler ultrasound recording of flow velocity in basal cerebral arteries. J Neurosurg. (1982) 57:769–74. doi: 10.3171/jns.1982.57.6.0769
18. Leung J, Behpour A, Sokol N, Mohanta A, Kassner A. Assessment of intracranial blood flow velocities using a computer controlled vasoactive stimulus: a comparison between phase contrast magnetic resonance angiography and transcranial Doppler ultrasonography. J Magnet Reson Imag. (2013) 38:733–8. doi: 10.1002/jmri.23911
19. Lefferts WK, DeBlois JP, Barreira TV, Heffernan KS. Neurovascular coupling during cognitive activity in adults with controlled hypertension. J Appl Physiol. (2018) 125:1906–16. doi: 10.1152/japplphysiol.00100.2018
20. Heffernan KS, Spartano NL, Augustine JA, Lefferts WK, Hughes WE, Mitchell GF, et al. Carotid artery stiffness and hemodynamic pulsatility during cognitive engagement in healthy adults: a pilot investigation. Am J Hypertens. (2015) 28:615–22. doi: 10.1093/ajh/hpu198
21. Naqvi TZ, Hyuhn HK. Cerebrovascular mental stress reactivity is impaired in hypertension. Cardiovasc Ultrasound. (2009) 7:1–14. doi: 10.1186/1476-7120-7-32
22. Paul RH, Lawrence J, Williams LM, Richard CC, Cooper N, Gordon E. Preliminary validity of “integneuroTM”: a new computerized battery of neurocognitive tests. Int J Neurosci. (2005) 115:1549–67. doi: 10.1080/00207450590957890
23. Silverstein SM, Berten S, Olson P, Paul R, Williams LM, Cooper N, et al. Development and validation of a World-Wide-Web-based neurocognitive assessment battery: WebNeuro. Behav Res Methods. (2007) 39:940–9. doi: 10.3758/BF03192989
24. Williams LM, Simms E, Clark CR, Paul RH, Rowe D, Gordon E. The test-retest reliability of a standardized neurocognitive and neurophysiological test battery:“neuromarker”. Int J Neurosci. (2005) 115:1605–30. doi: 10.1080/00207450590958475
25. Routledge KM, Burton KLO, Williams LM, Harris A, Schofield PR, Clark CR, et al. The shared and unique genetic relationship between mental well-being, depression and anxiety symptoms and cognitive function in healthy twins. Cogn Emot. (2017) 31:1465–79. doi: 10.1080/02699931.2016.1232242
26. Canfield AA. The “sten” scale-a modified C-scale. Educ Psychol Meas. (1951) 11:295–7. doi: 10.1177/001316445101100213
27. Moonen JE, Sabayan B, Sigurdsson S, Buchem MAv, Gudnason V, Meirelles O, et al. Contributions of cerebral blood flow to associations between blood pressure levels and cognition: the age, gene/environment susceptibility-Reykjavik study. Hypertension. (2021) 77:2075–83. doi: 10.1161/HYPERTENSIONAHA.120.16894
28. Wolters FJ, Zonneveld HI, Hofman A, Lugt AVD, Koudstaal PJ, Vernooij MW, et al. Cerebral perfusion and the risk of dementia. Circulation. (2017) 136:719–28. doi: 10.1161/CIRCULATIONAHA.117.027448
29. Wong RHX, Evans HM, Howe PRC. Poor cerebrovascular function is an early marker of cognitive decline in healthy postmenopausal women. Alzheimer's Dement Transl Res Clin Intervent. (2016) 2:162–8. doi: 10.1016/j.trci.2016.07.003
30. Csipo T, Lipecz A, Mukli P, Bahadli D, Abdulhussein O, Owens CD, et al. Increased cognitive workload evokes greater neurovascular coupling responses in healthy young adults. PLoS ONE. (2021) 16:e0250043. doi: 10.1371/journal.pone.0250043
31. Chung C-P, Lee H-Y, Lin P-C, Wang P-N. Cerebral artery pulsatility is associated with cognitive impairment and predicts dementia in individuals with subjective memory decline or mild cognitive impairment. J Alzheimer's Dis. (2017) 60:625–32. doi: 10.3233/JAD-170349
32. Lee S-T, Jung K-H, Lee Y-S. Decreased vasomotor reactivity in Alzheimer's disease. J Clin Neurol. (2007) 3:18–23. doi: 10.3988/jcn.2007.3.1.18
33. Webb AJ, Simoni M, Mazzucco S, Kuker W, Schulz U, Rothwell PM. Increased cerebral arterial pulsatility in patients with leukoaraiosis: arterial stiffness enhances transmission of aortic pulsatility. Stroke. (2012) 43:2631–6. doi: 10.1161/STROKEAHA.112.655837
34. Bateman GA, Levi CR, Schofield P, Wang Y, Lovett EC. Quantitative measurement of cerebral haemodynamics in early vascular dementia and Alzheimer's disease. J Clin Neurosci. (2006) 13:563–8. doi: 10.1016/j.jocn.2005.04.017
35. Conley AC, Karayanidis F, Jolly TAD, Yang M-H, Hsieh S. Cerebral arterial pulsatility and global white matter microstructure impact spatial working memory in older adults with and without cardiovascular risk factors. Front Aging Neurosci. (2020) 12:245. doi: 10.3389/fnagi.2020.00245
36. Vikner T, Eklund A, Karalija N, Malm J, Riklund K, Lindenberger U, et al. Cerebral arterial pulsatility is linked to hippocampal microvascular function and episodic memory in healthy older adults. J Cerebral Blood Flow Metabol. (2021) 41:1778–90. doi: 10.1177/0271678X20980652
37. Aly M, Turk-Browne NB. Attention stabilizes representations in the human hippocampus. Cerebral Cortex. (2016) 26:783–96. doi: 10.1093/cercor/bhv041
38. Chun MM, Turk-Browne NB. Interactions between attention and memory. Curr Opin Neurobiol. (2007) 17:177–84. doi: 10.1016/j.conb.2007.03.005
39. Singer J, Trollor JN, Baune BT, Sachdev PS, Smith E. Arterial stiffness, the brain and cognition: a systematic review. Ageing Res Rev. (2014) 15:16–27. doi: 10.1016/j.arr.2014.02.002
40. Squair JW, Lee AH, Sarafis ZK, Chan F, Barak OF, Dujic Z, et al. Network analysis identifies consensus physiological measures of neurovascular coupling in humans. J Cerebral Blood Flow Metabol Off J Int Soc Cerebral Blood Flow Metabol. (2020) 40:656–66. doi: 10.1177/0271678X19831825
41. Phillips AA, Chan FH, Zheng MMZ, Krassioukov AV, Ainslie PN. Neurovascular coupling in humans: physiology, methodological advances and clinical implications. J Cerebral Blood Flow Metabol Off J Int Soc Cerebral Blood Flow Metabol. (2016) 36:647–64. doi: 10.1177/0271678X15617954
42. Iulita MF, Noriega de la Colina A, Girouard H. Arterial stiffness, cognitive impairment and dementia: confounding factor or real risk? J Neurochem. (2018) 144:527–48. doi: 10.1111/jnc.14235
43. Rouch L, Cestac P, Sallerin B, Andrieu S, Bailly H, Beunardeau M, et al. Pulse wave velocity is associated with greater risk of dementia in mild cognitive impairment patients. Hypertension. (2018) 72:1109–16. doi: 10.1161/HYPERTENSIONAHA.118.11443
44. Yukutake T, Yamada M, Fukutani N, Nishiguchi S, Kayama H, Tanigawa T, et al. Arterial stiffness predicts cognitive decline in Japanese community-dwelling elderly subjects: a one-year follow-up study. J Atheroscler Thromb. (2015) 22:637–44. doi: 10.5551/jat.27391
45. Mitchell GF, Parise H, Benjamin EJ, Larson MG, Keyes MJ, Vita JA, et al. Changes in arterial stiffness and wave reflection with advancing age in healthy men and women: the Framingham Heart Study. Hypertension. (2004) 43:1239–45. doi: 10.1161/01.HYP.0000128420.01881.aa
46. Moreau KL, Hildreth KL. Vascular aging across the menopause transition in healthy women. Adv Vascul Med. (2014) 2014:204390. doi: 10.1155/2014/204390
47. Kehmeier MN, Walker AE. Sex differences in large artery stiffness: implications for cerebrovascular dysfunction and Alzheimer's disease. Front Aging. (2021) 2:791208. doi: 10.3389/fragi.2021.791208
48. Owens CD, Mukli P, Csipo T, Lipecz A, Silva-Palacios F, Dasari TW, et al. Microvascular dysfunction and neurovascular uncoupling are exacerbated in peripheral artery disease, increasing the risk of cognitive decline in older adults. Am J Physiol Heart Circul Physiol. (2022) 322:H924–h35. doi: 10.1152/ajpheart.00616.2021
49. Lim J, Pearman ME, Park W, Alkatan M, Machin DR, Tanaka H. Impact of blood pressure perturbations on arterial stiffness. Am J Physiol Regul Integ Compar Physiol. (2015) 309:R1540–R5. doi: 10.1152/ajpregu.00368.2015
50. Willie CK, Tzeng YC, Fisher JA, Ainslie PN. Integrative regulation of human brain blood flow. J Physiol. (2014) 592:841–59. doi: 10.1113/jphysiol.2013.268953
51. Angoff R, Himali JJ, Maillard P, Aparicio HJ, Vasan RS, Seshadri S, et al. Relations of metabolic health and obesity to brain aging in young to middle-aged adults. J Am Heart Assoc. (2022) 11:e022107. doi: 10.1161/JAHA.121.022107
52. Tarantini S, Csiszar A, Ungvari Z. Midlife obesity impairs neurovascular coupling responses. Obesity. (2021) 29:17. doi: 10.1002/oby.23072
53. Singh-Manoux A, Czernichow S, Elbaz A, Dugravot A, Sabia S, Hagger-Johnson G, et al. Obesity phenotypes in midlife and cognition in early old age: the Whitehall II cohort study. Neurology. (2012) 79:755–62. doi: 10.1212/WNL.0b013e3182661f63
54. Ferreira I, Twisk JW, van Mechelen W, Kemper HC, Seidell JC, Stehouwer CD. Current and adolescent body fatness and fat distribution: relationships with carotid intima-media thickness and large artery stiffness at the age of 36 years. J Hypertens. (2004) 22:145–55. doi: 10.1097/00004872-200401000-00024
55. Fernberg U, Op 't Roodt J, Fernström M, Hurtig-Wennlöf A. Body composition is a strong predictor of local carotid stiffness in Swedish, young adults: the cross sectional Lifestyle, biomarkers, and atherosclerosis study. BMC Cardiovasc Disord. (2019) 19:205. doi: 10.1186/s12872-019-1180-6
56. Toth P, Tarantini S, Csiszar A, Ungvari Z. Functional vascular contributions to cognitive impairment and dementia: mechanisms and consequences of cerebral autoregulatory dysfunction, endothelial impairment, and neurovascular uncoupling in aging. Am J Physiol Heart Circul Physiol. (2017) 312:H1–h20. doi: 10.1152/ajpheart.00581.2016
57. Csipo T, Lipecz A, Ashpole NM, Balasubramanian P, Tarantini S. Astrocyte senescence contributes to cognitive decline. GeroScience. (2020) 42:51–5. doi: 10.1007/s11357-019-00140-9
58. Balasubramanian P, Kiss T, Tarantini S, Nyúl-Tóth Á, Ahire C, Yabluchanskiy A, et al. Obesity-induced cognitive impairment in older adults: a microvascular perspective. Am J Physiol Heart Circul Physiol. (2021) 320:H740–61. doi: 10.1152/ajpheart.00736.2020
59. Spartano NL, Davis-Plourde KL, Himali JJ, Andersson C, Pase MP, Maillard P, et al. Association of accelerometer-measured light-intensity physical activity with brain volume: the framingham heart study. JAMA Network Open. (2019) 2:e192745. doi: 10.1001/jamanetworkopen.2019.2745
60. Spartano NL, Demissie S, Himali JJ, Dukes KA, Murabito JM, Vasan RS, et al. Accelerometer-determined physical activity and cognitive function in middle-aged and older adults from two generations of the Framingham Heart Study. Alzheimer's Dementia. (2019) 5:618–26. doi: 10.1016/j.trci.2019.08.007
61. Barnes JN, Pearson AG, Corkery AT, Eisenmann NA, Miller KB. Exercise, arterial stiffness, and cerebral vascular function: potential impact on brain health. J Int Neuropsychol Soc JINS. (2021) 27:761–75. doi: 10.1017/S1355617721000394
62. Balasubramanian P, DelFavero J, Ungvari A, Papp M, Tarantini A, Price N, et al. Time-restricted feeding (TRF) for prevention of age-related vascular cognitive impairment and dementia. Ageing Res Rev. (2020) 64:101189. doi: 10.1016/j.arr.2020.101189
63. Sorond FA, Hurwitz S, Salat DH, Greve DN, Fisher ND. Neurovascular coupling, cerebral white matter integrity, and response to cocoa in older people. Neurology. (2013) 81:904–9. doi: 10.1212/WNL.0b013e3182a351aa
64. Alexandrov AV, Sloan MA, Tegeler CH, Newell DN, Lumsden A, Garami Z, et al. Practice standards for transcranial Doppler (TCD) ultrasound. Part II Clinical indications and expected outcomes. J Neuroimag. (2012) 22:215–24. doi: 10.1111/j.1552-6569.2010.00523.x
65. Akif Topcuoglu M. Transcranial Doppler ultrasound in neurovascular diseases: diagnostic and therapeutic aspects. J Neurochem. (2012) 123:39–51. doi: 10.1111/j.1471-4159.2012.07942.x
66. Serrador JM, Picot PA, Rutt BK, Shoemaker JK, Bondar RL. MRI measures of middle cerebral artery diameter in conscious humans during simulated orthostasis. Stroke. (2000) 31:1672–8. doi: 10.1161/01.STR.31.7.1672
67. Silvestrini M, Cupini LM, Matteis M, Troisi E, Caltagirone C. Bilateral simultaneous assessment of cerebral flow velocity during mental activity. J Cerebral Blood Flow Metabol. (1994) 14:643–8. doi: 10.1038/jcbfm.1994.80
68. Krause DN, Duckles SP, Pelligrino DA. Influence of sex steroid hormones on cerebrovascular function. J Appl Physiol. (2006) 101:1252–61. doi: 10.1152/japplphysiol.01095.2005
69. Robison LS, Gannon OJ, Salinero AE, Zuloaga KL. Contributions of sex to cerebrovascular function and pathology. Brain Res. (2019) 1710:43–60. doi: 10.1016/j.brainres.2018.12.030
70. Phillips SM, Sherwin BB. Variations in memory function and sex steroid hormones across the menstrual cycle. Psychoneuroendocrinology. (1992) 17:497–506. doi: 10.1016/0306-4530(92)90008-U
Keywords: cerebral pulsatility, cognitive function, vascular stiffness, blood pressure, neurovascular coupling
Citation: Cilhoroz BT, DeBlois JP, Lefferts WK, Keller AP, Pagan Lassalle P, Meyer ML, Stoner L and Heffernan KS (2022) Exploration of cerebral hemodynamic pathways through which large artery function affects neurovascular coupling in young women. Front. Cardiovasc. Med. 9:914439. doi: 10.3389/fcvm.2022.914439
Received: 06 April 2022; Accepted: 25 July 2022;
Published: 12 August 2022.
Edited by:
Michiaki Nagai, Hiroshima City Asa Hospital, JapanReviewed by:
Stefano Tarantini, University of Oklahoma Health Sciences Center, United StatesAndrew Donald Robertson, University of Waterloo, Canada
Robert Matthew Brothers, University of Texas at Arlington, United States
Copyright © 2022 Cilhoroz, DeBlois, Lefferts, Keller, Pagan Lassalle, Meyer, Stoner and Heffernan. This is an open-access article distributed under the terms of the Creative Commons Attribution License (CC BY). The use, distribution or reproduction in other forums is permitted, provided the original author(s) and the copyright owner(s) are credited and that the original publication in this journal is cited, in accordance with accepted academic practice. No use, distribution or reproduction is permitted which does not comply with these terms.
*Correspondence: Kevin S. Heffernan, a3NoZWZmZXJAc3lyLmVkdQ==