- 1Department of Medicine, Yong Loo Lin School of Medicine, National University of Singapore, Singapore, Singapore
- 2Department of Cardiology, National University Heart Centre Singapore, Singapore, Singapore
- 3Division of Neurology, University Medicine Cluster, National University Health System, Singapore, Singapore
Introduction: Heart failure (HF) is associated with ischemic stroke (IS). However, there are limited studies on the prevalence of IS, white matter hyperintensities (WMHs), and silent brain infarcts (SBIs). Furthermore, interaction with ejection fraction (EF) is unclear.
Methods: We searched three databases (viz., PubMed, Embase, and Cochrane) for studies reporting the incidence or prevalence of IS, WMHs, and SBIs in HF. A total of two authors independently selected included studies. We used random-effects models, and heterogeneity was evaluated with I2 statistic. Meta-regression was used for subgroup analysis.
Results: In total, 41 articles involving 870,002 patients were retrieved from 15,267 records. Among patients with HF, the pooled proportion of IS was 4.06% (95% CI: 2.94–5.59), and that of WMHs and SBIs was higher at 15.67% (95% CI: 4.11–44.63) and 23.45% (95% CI: 14.53–35.58), respectively. Subgroup analysis of HFpEF and HFrEF revealed a pooled prevalence of 2.97% (95% CI: 2.01–4.39) and 3.69% (95% CI: 2.34–5.77), respectively. Subgroup analysis of WMH Fazekas scores 1, 2, and 3 revealed a decreasing trend from 60.57 % (95% CI: 35.13–81.33) to 11.57% (95% CI: 10.40–12.85) to 3.07% (95% CI: 0.95–9.47). The relative risk and hazard ratio of patients with HF developing IS were 2.29 (95% CI: 1.43–3.68) and 1.63 (95% CI: 1.22–2.18), respectively. Meta-regression showed IS prevalence was positively correlated with decreasing anticoagulant usage.
Conclusion: We obtained estimates for the prevalence of IS, WMH, and SBI in HF from systematic review of the literature.
Systematic review registration: https://www.crd.york.ac.uk/prospero/display_record.php?RecordID=255126, PROSPERO [CRD42021255126].
Introduction
HF is a global health problem, and its associated socioeconomic burden is enormous (1). It is widely assumed that HF predisposes individuals to the development of thromboembolic complications (2). In Virchow's triad, reduced cardiac output contributes to venous stasis, and the ensuing compensatory neuroendocrine mechanisms culminate in a hypercoagulable state (3, 4). Last, abnormal levels of von Willebrand factor (VWF) (3, 4) and endothelium-derived nitric oxide lead to endothelial dysfunction (5). These result in complications such as ischemic stroke (IS) (6) and stroke precursors including white matter hyperintensities (WMHs) and silent brain infarcts (SBIs) (7, 8).
To date, the magnitude of the problem of HF has not been comprehensively assessed due to marked variability in population-based estimates of the prevalence and incidence of associated complications (9). In recent years, few meta-analyses have explored the link between HF and its complications of IS (10–12), SBIs, and WMHs, with most stating similar limitations. A recent study by Witt et al. cited the lack of data on left ventricular ejection fraction (LVEF) as a major limitation of their meta-analysis (12). One of the independent risk factors associated with IS is impairment of left ventricular systolic function (13). Apart from IS, HF also predisposes individuals to an increased risk of other cerebrovascular outcomes, such as WMH and SBI. Currently, WMH has been gaining increased attention due to its prognostic role as a strong risk factor for stroke (14). However, not much has been described on the relationship between HF and WMH as these were previously considered an incidental finding on cerebral magnetic resonance imaging (MRI) with little therapeutic consequence (15). Our study sought to analyze the prevalence of IS in both patients with HF and subgroups of patients with different levels of ejection fraction. We also sought to quantify the prevalence of WMH in patients with HF as they both share similar pathophysiological mechanisms, including small-vessel disease (SVD) and large-vessel atherosclerosis (16).
Similarly, SBIs, defined as infarctions in the brain parenchyma without a history of stroke and only detected by MRI, have also been associated with HF (7, 8). The wide prevalence of SBI has been estimated to far exceed symptomatic stroke, with 10 cases of SBI for every one symptomatic stroke (17) and ~25% of individuals older than 80 years have SBI (18). The resultant higher risk of strokes was mostly ischemic in nature at 89% (19). Previously, Siachos et al. demonstrated a 34% prevalence of SBI in a trial of heart failure with reduced ejection fraction (HFrEF) patients only (20). Hence, while the exact relationship between HF and SBI remains unclear, our study seeks to quantify this important relationship with the aim of intervening before SBI develops into clinically overt IS.
Many studies explored the potential prophylactic role of anticoagulants in the development of IS among patients with HF but arrived at differing conclusions. The most recent meta-analysis by Redding et al. found an association between anticoagulant usage and the prevalence of IS through analysis of pooled odds ratio of four studies and 9,000 patients. Specifically, with regard to the use of newer oral anticoagulant (NOAC), the recent COMMANDER-HF trial suggested promising reduction of stroke events with low-dose rivaroxaban in a unique population of patients who suffered a recent episode of worsening HFrEF. However, the use of anticoagulants in patients with HF is a topic that should not be taken lightly, especially with its associated bleeding risks. Meta-regression is an analytical tool to derive a regression model to quantify the correlation between factors and to account for heterogeneity between studies. Our meta-analysis seeks to shed light on the highly debated role of anticoagulant use in patients with HF through a rigorous means of analysis with the use of meta-regression of proportions, which, to the best of our knowledge, has not been used in the existing literature.
Therefore, our study aims to address the aforementioned knowledge gaps by comprehensively summarizing and analyzing existing data on the development of WMH, SBI, and IS in patients with HF. Furthermore, quantifying the prevalence of WMH and SBI as asymptomatic precursors of IS may highlight the significance of early screening and intervention.
Materials and methods
Study identification
Ethics approval and consent to participate were not required as this was a systematic review of previously published data. The data that support the findings of this study are publicly available in the published literature. The meta-analysis was registered on PROSPERO (CRD42021255126) and reported according to the 2020 Preferred Reporting Items of Systematic Reviews and Meta-Analyses (PRISMA) guidelines (21). Studies were identified by searching across three electronic databases, namely, PubMed, Embase, and Cochrane, for articles published from inception to 2 May 2021. The search terms can be found in Appendix A in Supplementary material. The inclusion and exclusion criteria of our results are listed in Table 1, and only English language articles were included. In addition, studies were identified by hand-searching the reference list of relevant studies.
A total of two reviewers determined study eligibility and independently used the planned search strategy to search for relevant literature and data extraction. Discussion and consensus with a third reviewer resolved all disagreements. Studies were selected based on the inclusion criteria during the title and abstract reviews. Duplicate studies and studies explicitly meeting the exclusion criteria such as non-original research articles were excluded. Randomized clinical trials and cohort studies involving patients with chronic HF were chosen for the full-text review stage to determine if the studies reported the cerebrovascular outcomes of patients with HF, if they distinguished between ischemic and hemorrhagic strokes, and if the study populations were not suffering from the comorbidity of stroke independent of HF.
Data extraction
Data extracted are shown in Table 2. We collected data on cerebrovascular outcomes and baseline characteristics of participants including age and gender; comorbidities such as atrial fibrillation, diabetes mellitus, hypertension, hyperlipidemia, past myocardial infarction, and past stroke; and medications, such as antiplatelets, anticoagulants, and statins. The data collection sheet also recorded the total study cohort size, HF definition used, and the duration of follow-up. For the results on the prevalence of IS, SBI, and WMH, we collected data on the number of IS, WMH, and SBI events experienced by patients with HF. The number of IS events in heart failure with preserved ejection fraction (HFpEF) and HFrEF patient groups was also collected if reported. For results on the incidence of IS, hazard ratios and risk ratios for IS in patients with HF were collected in studies which reported them.
Statistical analysis
Pooled mean prevalence with 95% confidence intervals was calculated for IS, SBI, and WMH. Analyses were performed in RStudio (version 1.4.1106), unless stated otherwise. Meta-analysis of the proportions was performed with a one-step approach using a generalized linear mixed model (GLMM) method with a logit transformation using the function “metaprop.” In comparison to traditional two-stage methods, the one-step GLMM approach is proven to report less biased estimates, smaller errors, and greater coverage probabilities (62, 63). No continuity correction was applied, and all analyses were conducted using a random-effects model to account for methodological heterogeneity present between studies. The I2 statistic was used as an assessment of between-study variation, with an increasing I2 value representing greater levels of heterogeneity, and values of <30, 30–60, and >60% indicating low, moderate, and substantial levels of heterogeneity, respectively (64). For incidence rates, the pooled risk ratio (RR) and hazard ratio (HR) were quantitatively pooled and analyzed using Review Manager (RevMan) version 5.4, using general approaches mentioned in the Cochrane Handbook (65). To explore sources of heterogeneity, we conducted subgroup analysis. Where a minimum of two studies per subgroup was available, subgroup analyses were performed for the following studies with IS outcomes: HFrEF and HFpEF, less than and more than 1-year follow-up. For studies with WMH outcomes, subgroup analysis was performed according to the Fazekas scale (1, 2, and 3), which grades periventricular white matter and deep white matter lesions depending on the size and confluence of the lesions (22). For studies with SBI outcomes, less than and more than 1-year follow-up subgroups were performed. For subgroups that had two points of comparison, the Z-test was used to compare pooled prevalence data. For subgroups with more than two points of comparison, a Q-test was performed based on analyses of variance. A p < 0.05 was considered significant.
To account for heterogeneity between studies, meta-regression analysis was performed on variables including medication and comorbidities of patients. Antiplatelets have been widely used as prophylaxis in patients with coronary artery disease to reduce the risk of myocardial infarction and IS (66), while anticoagulation is used to lower the risk of cardioembolic stroke in patients with atrial fibrillation (67). In addition, comorbidities that have been previously known to have a strong correlation to the development of ischemic stroke was adjusted for including hypertension (68), diabetes mellitus (69), hyperlipidemia (70), and atrial fibrillation (71). Patients with prior strokes (72) or myocardial infarction (73) have also shown to be at an increased risk of ischemic stroke. The function “metareg” was used to perform a mixed-effect model meta-regression for years following HF diagnosis and anticoagulant usage. The size of the bubbles is proportional to the weights assigned to the studies. The upper and lower confidence intervals are also drawn within the figures in dotted lines. A gradient with a p < 0.05 was considered to represent significant correlation between the variables. To account for heterogeneity between studies, meta-regression analysis was performed on variables including medication and comorbidities of patients. Antiplatelets have been widely used as prophylaxis in patients with coronary artery disease to reduce the risk of myocardial infarction and IS, while anticoagulants are used to lower the risk of cardioembolic stroke in patients with atrial fibrillation. The function “metareg” was used to perform a mixed-effect model meta-regression for years following HF diagnosis and anticoagulant usage. The size of the bubbles is proportional to the weights assigned to the studies. The upper and lower confidence intervals are also drawn within the figures in dotted lines. A gradient with a p < 0.05 was considered to represent significant correlation between the variables.
Publication bias of studies was assessed using funnel plots, where the presence of asymmetry in the distribution of studies suggests bias (74). Quantitative assessment of funnel plot asymmetry was performed with linear regression analysis utilizing Egger's regression test, based on unweighted linear regression (75). An unweighted regression test was chosen over the traditional weighted test as it is no longer advocated due to the lack of theoretical justification (76). Egger's regression test was selected over a ranked correlation test as the latter is only powerful for large meta-analyses involving more than 75 studies (76).
Quality of evidence
The Newcastle–Ottawa Scale for cohort studies was used to assess the quality and risk of bias in included studies, which is summarized in Table S1 in Supplementary material. The scale assesses three main categories of selection (representativeness of the exposed cohort, selection of the non-exposed cohort, ascertainment of exposure, demonstration that the outcome of interest was not present at the start of the study), comparability (comparability of cohorts on basis of design and analysis), and exposure (assessment of outcome, if the length of the follow-up was long enough for outcomes to occur, adequacy of follow-up of cohorts). Studies could earn a maximum of nine star-points, with a maximum of 1-star point for each item within selection and outcome categories and a maximum of 2-star point given for the comparability category (77, 78). Under the adequacy of follow-up items in the outcome category, we determined a loss to follow-up of <5% as being unlikely to introduce bias. On the other hand, we suspected bias in studies with a loss of follow-up of more than 5% or an unreported loss to follow-up data.
Results
The literature search of PubMed, Embase, and Cochrane retrieved 15,267 results. After removing duplicate studies, the title and abstracts of the remaining 11,718 articles were screened; 11,505 records were excluded during the screen for reasons, namely, the study population did not include patients with HF, results did not report any cerebrovascular outcomes, or the studies were of an inappropriate type. The full-text screening excluded a further 151 articles. A total of 41 articles were included for the review and meta-analysis (20, 23–61, 79). The Preferred Reporting Items for Systemic Reviews and Meta-Analyses (PRSIMA) flowchart is presented in Figure 1.
Baseline characteristics
The baseline characteristics of the included studies comprising a total of 870,002 patients are summarized in Table 2. Across the 41 studies, comorbidities including atrial fibrillation (AF), previous stroke, and concurrent treatment including antiplatelet and anticoagulation therapy are also summarized in Table 2. Other comorbidities and treatments are available in the Table S2 in Supplementary material.
IS prevalence in patients with HF
Our meta-analysis included 35 studies, with a total of 866,482 patients, and the prevalence of IS in patients with HF is reported in Figure 2. In patients with HF, the pooled proportion of IS was 4.06% (95% CI: 2.94–5.59, I2 = 100%). In our subgroup analysis, there was a significant difference in the pooled proportion of IS at a follow-up period of 1 year and more than 1 year, which were 2.16 and 5.64%, respectively. The p-value was <0.01. The I2 statistic was 100%, which indicates that the heterogeneity of our studies was high.
HFpEF and HFrEF and time dependency of stroke risk
A total of six studies reported IS outcomes in patients with HFpEF, which had a pooled proportion of 2.97% (95% CI: 2.01–4.39). Subgroup analysis in Figure 3 showed that the pooled proportions over a follow-up period of 1 year or less and more than 1 year were 3.88% (95% CI: 2.20–6.76) and 2.51% (95% CI: 1.58–3.98), respectively. A total of nine studies reported IS outcomes in patients with HFrEF, which had a pooled proportion of 3.69% (95% CI: 2.34–5.77). Subgroup analysis showed that the pooled proportions over a follow-up period of 1 year or less and more than 1 year were 3.15% (95% CI: 1.38–7.03) and 4.09% (95% CI: 2.32–7.13), respectively. Our subgroup analysis demonstrated that there was no statistically significant difference in IS between the two groups, with a p-value of 0.48.
IS incidence in patients with HF
There was an association between patients with HF and the incidence of subsequent IS. In all, three studies reported the risk ratio (RR), and 10 studies reported the hazard ratio (HR) for HF and IS. The pooled RR was 2.29 (95% CI: 1.43–3.68), and pooled HR was 1.63 (95% CI: 1.22–2.18).
Silent brain infarct prevalence in patients with HF
The prevalence of SBI in patients with HF is presented in Figure 4. In patients with HF, the pooled proportion of SBI was 23.45% (95% CI: 14.53–35.58). Within studies that had a follow-up period of more than a year, the pooled proportion of SBI was 21.13% (95% CI: 11.97–34.55). Only one study by Siachos et al. had a follow-up period of less than a year, with a proportion of SBI reported at 34.19% (20).
White matter hyperintensity prevalence in patients with HF
The prevalence of WMH in patients with HF is presented in Figure 5. Among studies with a Fazekas score of 1, the pooled proportion of WMH was 60.57% (95% CI: 35.13–81.33). Among studies with Fazekas scores of 2 and 3, the pooled proportion of WMH was 11.57% (95% CI: 10.40–12.85) and 3.07% (95% CI: 0.95–9.47), respectively. Our subgroup analysis demonstrated significant and distinct differences in the IS prevalence between the three scores, with a p-value < 0.01.
Meta-regression for comorbidities and medication use
To account for heterogeneity between studies, meta-regression analysis was used to integrate the prevalence rate of studies that reported the percentage of patients with different comorbidities and medication use. The figures can be found in the Supplementary material. No significant correlation was found for the variables of comorbidities including atrial fibrillation, diabetes mellitus, hypertension, hyperlipidemia, prior myocardial infarction, prior stroke, and medication use of antiplatelets and anticoagulants.
Meta-regression analysis was used to integrate the prevalence rate of 34 studies that reported the percentage of patients in their study population that were taking anticoagulants, as shown in Figure 6. We observed a significant negative correlation between the variables (p = 0.0002). The regression line demonstrated an 82% decrease in IS prevalence from studies with 0–100% anticoagulant usage. We also performed meta-regression to analyze including atrial fibrillation (AF), diabetes mellitus (DM), hyperlipidemia (HLD), hypertension (HTN), previous myocardial infarction (MI), stroke, and antiplatelet usage.
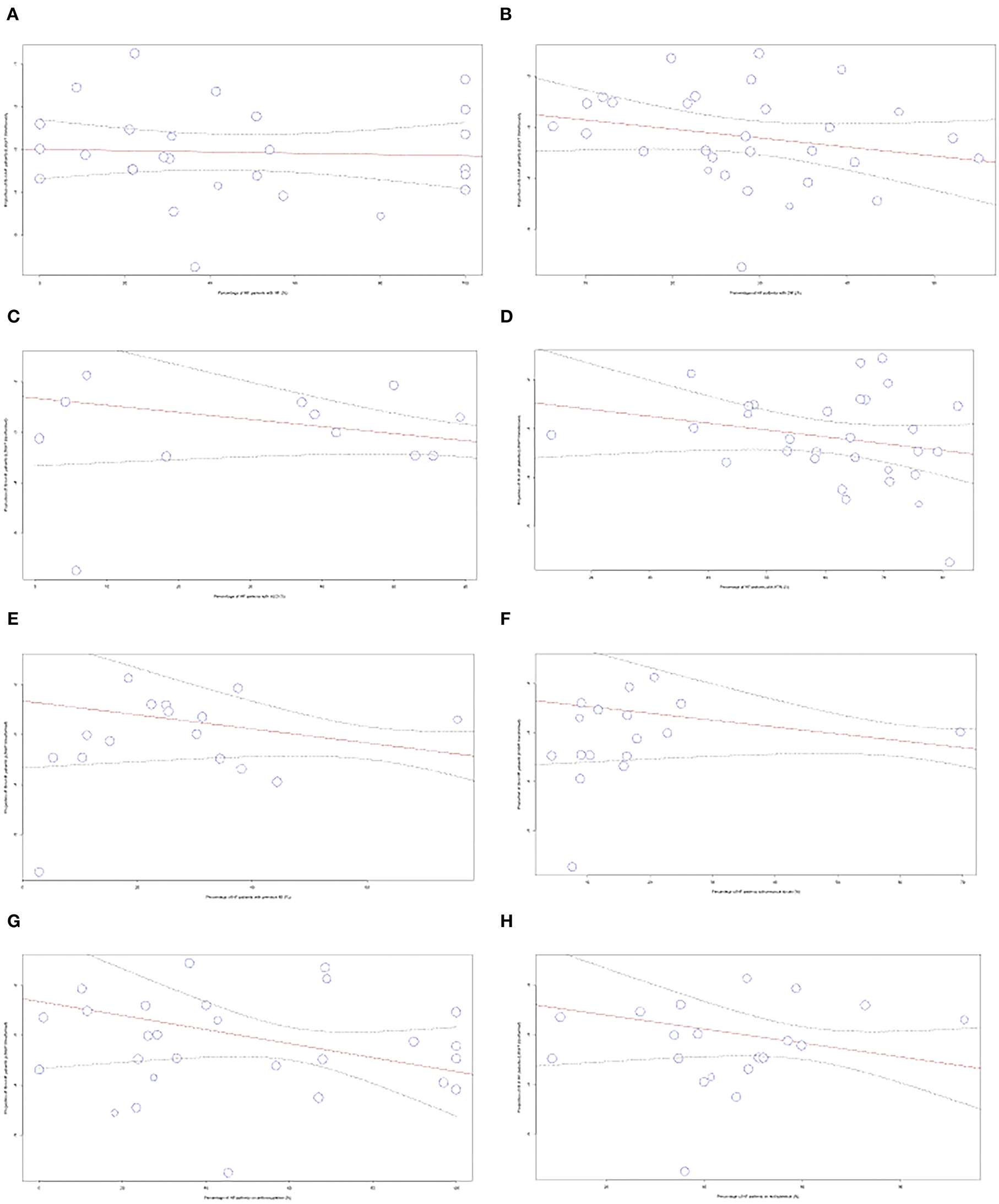
Figure 6. Meta-regression performed for comorbidities and medications used (A) AF, (B) DM, (C) HLD, (D) HTN, (E) MI, (F) Stroke, (G) Anticoagulants, (H) Antiplatelets.
Funnel plots and Egger's regression test were performed to assess for publication bias for studies reporting the prevalence of IS and SBI in patients with HF in Figures 7, 8. There was no evidence of publication bias for the prevalence of IS (p = 0.0568) and prevalence of SBI (p = 0.3543).
Discussion
Our meta-analysis for the pooled proportion of IS included 35 studies with a combined population of 866,482 patients with HF. The pooled proportion of IS was determined to be 4.06%. There is a statistically significant difference between the pooled proportion of IS in patients with HF with less than and more than 1 year of follow-up (p < 0.01). In our subgroup analysis, the pooled proportion of IS in patients with HFpEF and HFrEF was 2.97 and 3.69%, respectively, but no statistically significant difference was observed in both subgroups with different ejection fractions (p = 0.48). The prevalence of WMH and SBI in HF is 15.67% and 23.45%, respectively. Subgroup analysis found a statistically significant difference in patients with different Fazekas scores (p < 0.01) in which there was a decreasing trend in the prevalence of patients with WMH of a Fazekas score of 1 at 60.57% to Fazekas scores 2 and 3 at 11.57 and 3.07%.
Studies such as Katsanos et al. and Witt et al. have published extensively on HF and the risk of IS recurrence (11, 12). Novelty of our study lies in going beyond IS as the sole endpoint by also analyzing brain lesions leading to the development of IS. WMH and SBI are well-established risk factors of IS (15). Among the various subclinical pathological alterations of the brain parenchyma, WMH is among the most encountered anomalies on MRI (80). Despite mounting evidence of WMH as a risk of IS, few studies have established the relationship between WMH and HF. Despite different pathologies and clinical manifestations, the heart and brain display similarities in vascular anatomy and share common pathophysiological features related to vascular function (81). Small-vessel disease (SVD) is a common pathological mechanism in both WMH and HF. SVD causes coronary microvascular dysfunction, which can lead to both HF and WMH (16). Furthermore, studies have shown that large-vessel atherosclerotic disease and microembolism from unstable plaques also lead to WMH. Our results quantify a pooled proportion of 14.76% patients with HF developing WMH among the included studies. The association between WMH and HF suggests that therapeutic methods to prevent progression to the development of IS should be explored.
In our subgroup analysis of WMH, the Fazekas visual rating scale demonstrated a significant difference in the pooled proportion (p < 0.0001). This suggests a distinct heterogeneity among the prevalence of different Fazekas scores among patients with HF. A decreasing trend was observed with the proportion of patients with HF who developed WMH of Fazekas score 1 as 60.57%, Fazekas Score 2 as 11.57%, and Fazekas Score 3 as 3.07%. The scale by Fazekas et al. is an established rating tool to the quantify the severity of white matter involvement (22). Our findings suggest that a large proportion of patients with HF already have white matter involvement, with approximately more than one in every two patients with HF having WMH of a Fazekas score of 1. Furthermore, WMHs have not only been recognized as precursor lesions to stroke but a growing body of evidence strongly suggest these lesions are associated with poorer cognitive performance in patients with HF (82). A previous cross-sectional study by Sauvé et al. (83) suggested the prevalence of cognitive impairment in patients with HF was four times more frequent than in the controls. This combined with our results and the fact that impaired cognition interferes with disease control as HF treatment and monitoring demand a high degree comprehension and self-control (60) shows that WMH in patients with HF is severely under-detected. Hence, there is growing clinical importance for the early screening and recognition of these neuropathological changes.
Furthermore, in our meta-analysis of four studies involving 882 patients, we demonstrated that there is a significantly high prevalence of SBI in patients with HF in approximately one of every four patients. In light of this significant relationship between SBI and HF, there is potential relevance in the detection of SBI among patients with HF to intervene before the development of a clinically overt stroke (84). Currently, there is paucity of information in the literature on the prevention of symptomatic stroke in patients with SBI. To date, the AHA/ASA guideline is the most relevant in recommending primary stroke prevention and vascular risk reduction to prevent symptomatic stroke in individuals with SBI (85). Nevertheless, the high risk of stroke still remains in patients with SBI even after known risk factors are controlled for, and stroke prevention strategies in SBI may be a useful focus in future clinical trials. The high number of HF patients with SBI shown in our study reinforces the notion that patients with SBI should be considered as a high-risk group, and its importance should neither be underestimated nor be under-diagnosed or under-treated.
In our subgroup analysis of HF with varying levels of LVEF, there is a lower prevalence of IS in patients with HFpEF at 2.97% than in patients with HFrEF at 3.69%. This may be consistent with the theory that a lower ejection fraction leads to stasis and the increased formation of LV thrombus (86), which may embolize to the brain. Nevertheless, the relationship between LVEF and IS is not well documented (87). Currently, existing studies including this meta-analysis did not find a significant difference in the prevalence of IS between HFpEF and HFrEF phenotypes at p = 0.48 (p > 0.05). A meta-analysis by Kotecha et al. found that the incidence of IS between patients with HFpEF and HFrEF was similar (88). By contrast, Greenberg et al. found a significant 1.6-fold higher risk of IS in patients with HFrEF than in patients with HFpEF (31). A possible lack of significant difference may be attributed to the high heterogeneity in studies among patients with HFrEF and HFpEF (I2 = 98%).
We postulate that the high heterogeneity found between studies is related to the multiplicity of factors that predispose patients toward IS. Furthermore, limitations of existing studies such as Witt et al. include the inability to draw conclusions regarding patient-level characteristics such as their comorbidities and medication use, citing these as important factors that should be addressed in future research (12). Hence, meta-regression was used in our subgroup analysis to quantify the correlation between factors and account for the heterogeneity in the studies. Our study is, to the best of our knowledge, the first meta-analysis that adopted the use of meta-regression of proportions to not only establish an association but also quantify the correlation between factors that may affect the relationship between HF and IS. Our regression models found no significant correlation was found in comorbidities including atrial fibrillation (AF), diabetes mellitus (DM), hyperlipidemia (HLD), hypertension (HTN), previous myocardial infarction (MI), stroke, and antiplatelet usage.
On the other hand, meta-regression also found a significant 82% decrease in the prevalence of IS from studies with 0% to 100% of anticoagulant treatment in the study population. Our results and the pharmacological mechanism of anticoagulants theorize it as a potentially logical prophylaxis for HF as it prevents venous thrombosis in low-velocity blood flow (5). Nevertheless, there exists a controversy in the current medical scenario with regard to the use of anticoagulation in HF as existing literature is conflicted as to whether the benefits of anticoagulant usage outweigh its risks of life-threatening complications such as hemorrhagic stroke (89). Amid this dilemma, well-considered guidelines provided by the ACC/AHA and ESC still do not strongly recommend the usage of anticoagulants in the treatment of HF (90), and it is only indicated in patients with concomitant AF (91). However, this analysis may be confounded by the fact that studies also included patients with AF who are taking anticoagulants. As such, the incidence of IS in this population will be reduced. Our meta-analysis points to the growing need for future research to adopt multi-variate meta-regression for the proportion of patients with AF and anticoagulant use.
Furthermore, another possible explanation for our heterogeneity would be that a sizeable proportion of our included studies did not specify the type of HFrEF and HFpEF subgroups and were not included in our subgroup analysis, resulting in limited data. It may be helpful for future studies to focus on the specific role of LVEF on the development of IS. Future studies may also attempt to stratify results based on prevalence and severity of carotid plaques and previous vascular surgery, as well as specific cardiovascular risk factors such as valvular heart disease (92), to more accurately target the mechanisms underlying the development of these brain lesions.
Future meta-analysis may also seek to analyze the prevalence and incidence of the different subtypes of ischemic stroke and its relationship with the underlying etiology of HF. While cerebrovascular thromboembolism has been reported as the most common etiology of ischemic stroke, studies have reported varying degrees of prevalence of different subtypes of IS dependent on the etiology of HF. Vemmos et al. had previously reported that non-ischemic etiologies of HF such as valvular heart disease and dilated cardiomyopathy were related to cardioembolism, while HF arising from coronary artery disease was associated with large-vessel atherosclerosis and lacunar strokes.
Strengths and limitations
Our meta-analysis comprehensively reviewed a significantly large study population of 870,002 patients from 41 studies. While the broad spectrum of included studies is a strength of our meta-analysis, this likely contributed to heterogeneity of study demographics. For instance, most included studies examined Caucasian populations, which limits generalizability to other ethnic groups. Many studies did not publish data on anticoagulant dosages, drug type, or duration administered. The definition of HF and range of LVEF in HFrEF and HFpEF were not standardized across the included studies. There have been varying diagnostic criteria proposed for HFpEF, which could have contributed to heterogeneity in the patients included in studies, trials, and registries (90). Furthermore, some included studies chose to classify patients with LVEF within the range (40% ≤ LVEF ≤ 50%) under HFrEF, even though the most widely used classification of HFrEF would be LVEF <40% (26, 33, 46, 55, 93, 94). Future studies should use the latest available guidelines to standardize the definition of the type of heart failure (95).
Conclusion
In conclusion, we estimated that four in 100 patients with HF on average suffer from an ischemic stroke, with a higher prevalence reported in studies after more than 1 year of follow-up. However, we were unable to determine any significant difference in the prevalence of IS between patients with HFpEF and HFrEF, which may have been attributed to high between-study heterogeneity and therefore may warrant future studies and reviews in this area. Our most significant finding would be the high prevalence of WMHs and SBIs in patients with HF, with nearly one in every two HF patients with WMH of a Fazekas score 1, and one in every four HF patients with SBI. This highlights the potential under-detection and under-treatment of these conditions, given that these cerebrovascular lesions predispose patients to significant cognitive impairment and risk of future strokes in the long run.
Data availability statement
The original contributions presented in the study are included in the article/Supplementary material, further inquiries can be directed to the corresponding author/s.
Author contributions
ST and CH contributed to conception and design of the study. YHT and YNT performed the statistical analysis. ST and CH wrote the first draft of the manuscript. All authors contributed to manuscript revision, read, and approved the submitted version.
Funding
This research was sponsored by the National Medical Research Council (NMRC), Singapore (NMRC/MOH-TA19Nov-0003). BT is supported by the Ministry of Health, Singapore (MOH) – Healthcare Research Scholarship – Master of Clinical Investigation (MCI). LY is supported by National Medical Research Council – NMRC/MOH-TA19Nov-003.
Acknowledgments
We would like to express our gratitude to our supervisor C-HS for his guidance and doctors from the National University Hospital of Singapore for their invaluable contribution and advice.
Conflict of interest
The authors declare that the research was conducted in the absence of any commercial or financial relationships that could be construed as a potential conflict of interest.
Publisher's note
All claims expressed in this article are solely those of the authors and do not necessarily represent those of their affiliated organizations, or those of the publisher, the editors and the reviewers. Any product that may be evaluated in this article, or claim that may be made by its manufacturer, is not guaranteed or endorsed by the publisher.
Supplementary material
The Supplementary Material for this article can be found online at: https://www.frontiersin.org/articles/10.3389/fcvm.2022.967197/full#supplementary-material
References
1. Agbor VN, Ntusi NAB, Noubiap JJ. An overview of heart failure in low- and middle-income countries. Cardiovasc Diagn Ther. (2020) 10:244–51. doi: 10.21037/cdt.2019.08.03
2. Haeusler KG, Laufs U, Endres M. Chronic heart failure and ischemic stroke. Stroke. (2011) 42:2977–82. doi: 10.1161/STROKEAHA.111.628479
3. Cugno M, Mari D, Meroni PL, Gronda E, Vicari F, Frigerio M, et al. Haemostatic and inflammatory biomarkers in advanced chronic heart failure: role of oral anticoagulants and successful heart transplantation. Br J Haematol. (2004) 126:85–92. doi: 10.1111/j.1365-2141.2004.04977.x
4. Sbarouni E, Bradshaw A, Andreotti F, Tuddenham E, Oakley CM, Cleland JG. Relationship between hemostatic abnormalities and neuroendocrine activity in heart failure. Am Heart J. (1994) 127:607–12. doi: 10.1016/0002-8703(94)90670-X
5. Lip GY, Gibbs CR. Does heart failure confer a hypercoagulable state? Virchow's triad revisited. J Am Coll Cardiol. (1999) 33:1424–6.
6. Ois A, Gomis M, Cuadrado-Godia E, Jiménez-Conde J, Rodríguez-Campello A, Bruguera J, et al. Heart failure in acute ischemic stroke. J Neurol. (2008) 255:385–9. doi: 10.1007/s00415-008-0677-1
7. Gupta A, Giambrone AE, Gialdini G, Finn C, Delgado D, Gutierrez J, et al. Silent Brain Infarction and Risk of Future Stroke: A Systematic Review and Meta-Analysis. Stroke. (2016) 47:719–25. doi: 10.1161/STROKEAHA.115.011889
8. Park JH, Heo SH, Lee MH, Kwon HS, Kwon SU, Lee JS, et al. White matter hyperintensities and recurrent stroke risk in patients with stroke with small-vessel disease. Eur J Neurol. (2019) 26:911–8. doi: 10.1111/ene.13908
9. Cowie MR, Mosterd A, Wood DA, Deckers JW, Poole-Wilson PA, Sutton GC, et al. The epidemiology of heart failure. Eur Heart J. (1997) 18:208–25. doi: 10.1093/oxfordjournals.eurheartj.a015223
10. Tang L, Wu YY, Lip GY, Yin P, Hu Y. Heart failure and risk of venous thromboembolism: a systematic review and meta-analysis. Lancet Haematol. (2016) 3:e30–44. doi: 10.1016/S2352-3026(15)00228-8
11. Katsanos AH, Parissis J, Frogoudaki A, Vrettou AR, Ikonomidis I, Paraskevaidis I, et al. Heart failure and the risk of ischemic stroke recurrence: A systematic review and meta-analysis. J Neurol Sci. (2016) 362:182–7. doi: 10.1016/j.jns.2016.01.053
12. Witt BJ, Gami AS, Ballman KV, Brown RD, Meverden RA, Jacobsen SJ, et al. The incidence of ischemic stroke in chronic heart failure: a meta-analysis. J Card Fail. (2007) 13:489–96. doi: 10.1016/j.cardfail.2007.01.009
13. Kozdag G, Ciftci E, Vural A, Selekler M, Sahin T, Ural D, et al. Silent cerebral infarction in patients with dilated cardiomyopathy: echocardiographic correlates. Int J Cardiol. (2006) 107:376–81. doi: 10.1016/j.ijcard.2005.03.055
14. Moroni F, Ammirati E, Rocca MA, Filippi M, Magnoni M, Camici PG. Cardiovascular disease and brain health: Focus on white matter hyperintensities. Int J Cardiol Heart Vasc. (2018) 19:63–9. doi: 10.1016/j.ijcha.2018.04.006
15. Debette S, Markus HS. The clinical importance of white matter hyperintensities on brain magnetic resonance imaging: systematic review and meta-analysis. BMJ. (2010) 341:c3666. doi: 10.1136/bmj.c3666
16. Camici PG, Tschöpe C, Di Carli MF, Rimoldi O, Van Linthout S. Coronary microvascular dysfunction in hypertrophy and heart failure. Cardiovasc Res. (2020) 116:806–16. doi: 10.1093/cvr/cvaa023
17. Leary MC, Saver JL. Annual incidence of first silent stroke in the United States: a preliminary estimate. Cerebrovasc Dis. (2003) 16:280–5. doi: 10.1159/000071128
18. Vermeer SE, Longstreth Jr WT, Koudstaal PJ. Silent brain infarcts: a systematic review. Lancet Neurol. (2007) 6:611–9. doi: 10.1016/S1474-4422(07)70170-9
19. Windham BG, Deere B, Griswold ME, Wang W, Bezerra DC, Shibata D, et al. Small brain lesions and incident stroke and mortality: a cohort study. Ann Intern Med. (2015) 163:22–31. doi: 10.7326/M14-2057
20. Siachos T, Vanbakel A, Feldman DS, Uber W, Simpson KN, Pereira NL. Silent strokes in patients with heart failure. J Card Fail. (2005) 11:485–9. doi: 10.1016/j.cardfail.2005.04.004
21. Page MJ, McKenzie JE, Bossuyt PM, Boutron I, Hoffmann TC, Mulrow CD, et al. The PRISMA 2020 statement: an updated guideline for reporting systematic reviews. BMJ. (2021) 372:n71. doi: 10.1136/bmj.n71
22. Fazekas F, Chawluk JB, Alavi A, Hurtig HI, Zimmerman RA, MR. signal abnormalities at 1.5 T in Alzheimer's dementia and normal aging. AJR Am J Roentgenol. (1987) 149:351–6. doi: 10.2214/ajr.149.2.351
23. Adelborg K, Szépligeti S, Sundbøll J, Horváth-Puhó E, Henderson VW, Ording A, et al. Risk of stroke in patients with heart failure: a population-based 30-year cohort study. Stroke. (2017) 48:1161–8. doi: 10.1161/STROKEAHA.116.016022
24. Alberts VP, Bos MJ, Koudstaal P, Hofman A, Witteman JC, Stricker B, et al. Heart failure and the risk of stroke: the Rotterdam study. Eur J Epidemiol. (2010) 25:807–12. doi: 10.1007/s10654-010-9520-y
25. Berger JS, Peterson E, LalibertÉ F, Germain G, Lejeune D, Schein J, et al. Risk of ischemic stroke in patients newly diagnosed with heart failure: focus on patients without atrial fibrillation. J Card Fail. (2019) 25:436–47. doi: 10.1016/j.cardfail.2018.03.012
26. Berkovitch A, Mazin I, Younis A, Shlomo N, Nof E, Goldenberg I, et al. CHA2DS2-VASc score performance to predict stroke after acute decompensated heart failure with and without reduced ejection fraction. Europace. (2019) 21:1639–45. doi: 10.1093/europace/euz192
27. Chi G, Januzzi JL, Korjian S, Daaboul Y, Goldhaber SZ, Hernandez AF, et al. N-terminal pro-B-type natriuretic peptide and the risk of stroke among patients hospitalized with acute heart failure: an APEX trial substudy. J Thromb Thrombolysis. (2017) 44:457–65. doi: 10.1007/s11239-017-1552-7
28. Chou YL, Liou JT, Cheng CC, Tsai MC, Lin WS, Cheng SM, et al. The association of ischaemic stroke in patients with heart failure without atrial flutter/fibrillation. Heart. (2020) 106:616–23. doi: 10.1136/heartjnl-2019-315646
29. de Peuter OR, Souverein PC, Klungel OH, Büller HR, de Boer A, Kamphuisen PW. Non-selective vs. selective beta-blocker treatment and the risk of thrombo-embolic events in patients with heart failure. Eur J Heart Fail. (2011) 13:220–6. doi: 10.1093/eurjhf/hfq176
30. Friberg L, Lund LH. Heart failure: a weak link in CHA(2) DS(2)-VASc. ESC Heart Fail. (2018) 5:231–9. doi: 10.1002/ehf2.12262
31. Greenberg B, Peterson ED, Berger JS, Laliberté F, Zhao Q, Germain G, et al. Ejection fraction, B-type natriuretic peptide and risk of stroke and acute myocardial infarction among patients with heart failure. Clin Cardiol. (2019) 42:277–84. doi: 10.1002/clc.23140
32. Hamatani Y, Nagai T, Nakai M, Nishimura K, Honda Y, Nakano H, et al. Elevated plasma D-dimer level is associated with short-term risk of ischemic stroke in patients with acute heart failure. Stroke. (2018) 49:1737–40. doi: 10.1161/STROKEAHA.118.021899
33. Hjalmarsson C, Fu M, Zverkova Sandström T, Schaufelberger M, Ljungman C, Andersson B, et al. Risk of stroke in patients with heart failure and sinus rhythm: data from the Swedish Heart Failure Registry. ESC Heart Failure. (2021) 8:85–94. doi: 10.1002/ehf2.13091
34. Iguchi M, Tezuka Y, Ogawa H, Hamatani Y, Takagi D, An Y, et al. Incidence and Risk Factors of Stroke or Systemic Embolism in Patients With Atrial Fibrillation and Heart Failure-The Fushimi AF Registry. Circ J. (2018) 82:1327–35. doi: 10.1253/circj.CJ-17-1155
35. Kang SH, Kim J, Park JJ, Oh IY, Yoon CH, Kim HJ, et al. Risk of stroke in congestive heart failure with and without atrial fibrillation. Int J Cardiol. (2017) 248:182–7. doi: 10.1016/j.ijcard.2017.07.056
36. Kim TH, Yang PS, Uhm JS, Kim JY, Pak HN, Lee MH, et al. CHA(2)DS(2)-VASc Score (Congestive Heart Failure, Hypertension, Age ≥75 [Doubled], Diabetes Mellitus, Prior Stroke or Transient Ischemic Attack [Doubled], Vascular Disease, Age 65-74, Female) for stroke in asian patients with atrial fibrillation: a Korean Nationwide sample cohort study. Stroke. (2017) 48:1524–30. doi: 10.1161/STROKEAHA.117.016926
37. Komori T, Eguchi K, Tomizawa H, Ishikawa J, Hoshide S, Shimada K, et al. Factors associated with incident ischemic stroke in hospitalized heart failure patients: a pilot study. Hypertens Res. (2008) 31:289–94. doi: 10.1291/hypres.31.289
38. Kondo T, Yamada T, Morita T, Furukawa Y, Tamaki S, Iwasaki Y, et al. The CHADS2 score predicts ischemic stroke in chronic heart failure patients without atrial fibrillation: comparison to other stroke risk scores. J Am Coll Cardiol. (2016) 67:1308. doi: 10.1016/S0735-1097(16)31309-2
39. Lip GY, Rasmussen LH, Skjøth F, Overvad K, Larsen TB. Stroke and mortality in patients with incident heart failure: the Diet, Cancer and Health (DCH) cohort study. BMJ Open. (2012) 2:975. doi: 10.1136/bmjopen-2012-000975
40. Loh E, Sutton MS, Wun CC, Rouleau JL, Flaker GC, Gottlieb SS, et al. Ventricular dysfunction and the risk of stroke after myocardial infarction. N Engl J Med. (1997) 336:251–7. doi: 10.1056/NEJM199701233360403
41. McMurray JJ, Ezekowitz JA, Lewis BS, Gersh BJ, van Diepen S, Amerena J, et al. Left ventricular systolic dysfunction, heart failure, and the risk of stroke and systemic embolism in patients with atrial fibrillation: insights from the ARISTOTLE trial. Circ Heart Fail. (2013) 6:451–60. doi: 10.1161/CIRCHEARTFAILURE.112.000143
42. Mehra MR, Vaduganathan M, Fu M, Ferreira JP, Anker SD, Cleland JGF, et al. A comprehensive analysis of the effects of rivaroxaban on stroke or transient ischaemic attack in patients with heart failure, coronary artery disease, and sinus rhythm: the COMMANDER HF trial. Eur Heart J. (2019) 40:3593–602. doi: 10.1093/eurheartj/ehz427
43. Melgaard L, Gorst-Rasmussen A, Lane DA, Rasmussen LH, Larsen TB, Lip GY. Assessment of the CHA2DS2-VASc score in predicting ischemic stroke, thromboembolism, and death in patients with heart failure with and without atrial fibrillation. JAMA. (2015) 314:1030–8. doi: 10.1001/jama.2015.10725
44. Merkler AE, Chen ML, Parikh NS, Murthy SB, Yaghi S, Goyal P, et al. Association between heart transplantation and subsequent risk of stroke among patients with heart failure. Stroke. (2019) 50:583–7. doi: 10.1161/STROKEAHA.118.023622
45. Nakano H, Hamatani Y, Nagai T, Nakai M, Nishimura K, Sumita Y, et al. Current practice and effects of intravenous anticoagulant therapy in hospitalized acute heart failure patients with sinus rhythm. Sci Rep. (2021) 11:1202. doi: 10.1038/s41598-020-79700-5
46. Nakayama M, Konishi M, Akiyama E, Morita Y, Fukutomi Y, Nakayama N, et al. Prognostic role of bronchial asthma in patients with heart failure. Heart Vessels. (2020) 35:808–16. doi: 10.1007/s00380-020-01555-7
47. Qualls LG, Greiner MA, Eapen ZJ, Fonarow GC, Mills RM, Klaskala W, et al. Postdischarge international normalized ratio testing and long-term clinical outcomes of patients with heart failure receiving warfarin: findings from the ADHERE registry linked to Medicare claims. Clin Cardiol. (2013) 36:757–65. doi: 10.1002/clc.22206
48. Shintani Y, Takahama H, Hamatani Y, Nishimura K, Kanzaki H, Kusano K, et al. Ischemic stroke risk during post-discharge phases of heart failure: association of left ventricular concentric geometry. Heart Vessels. (2020) 35:564–75. doi: 10.1007/s00380-019-01522-x
49. Tai YH, Chang CC, Yeh CC, Sung LC, Hu CJ, Cherng YG, et al. Long-term risk of stroke and poststroke outcomes in patients with heart failure: two nationwide studies. Clin Epidemiol. (2020) 12:1235–44. doi: 10.2147/CLEP.S261179
50. Tseng AS, William Schleifer J, Shen WK, McBane R, Mankad S, Esser H, et al. Real-world incidence of efficacy and safety outcomes in patients on direct oral anticoagulants with left ventricular systolic dysfunction at a tertiary referral center. Clin Cardiol. (2017) 40:1328–32. doi: 10.1002/clc.22833
51. Tütüncü S, Honold M, Koehler K, Deckwart O, Koehler F, Haeusler KG. Non-invasive telemedical care in heart failure patients and stroke: post hoc analysis of TIM-HF and TIM-HF2 trials. ESC Heart Fail. (2020) 7:884–91. doi: 10.1002/ehf2.12679
52. Vemmos K, Ntaios G, Savvari P, Vemmou AM, Koroboki E, Manios E, et al. Stroke aetiology and predictors of outcome in patients with heart failure and acute stroke: a 10-year follow-up study. Eur J Heart Fail. (2012) 14:211–8. doi: 10.1093/eurjhf/hfr172
53. Witt BJ, Brown RD, Jacobsen SJ, Weston SA, Ballman KV, Meverden RA, et al. Ischemic stroke after heart failure: a community-based study. Am Heart J. (2006) 152:102–9. doi: 10.1016/j.ahj.2005.10.018
54. Wolsk E, Lamberts M, Hansen ML, Blanche P, Køber L, Torp-Pedersen C, et al. Thromboembolic risk stratification of patients hospitalized with heart failure in sinus rhythm: a nationwide cohort study. Eur J Heart Fail. (2015) 17:828–36. doi: 10.1002/ejhf.309
55. Yusuf S, Pfeffer MA, Swedberg K, Granger CB, Held P, McMurray JJ, et al. Effects of candesartan in patients with chronic heart failure and preserved left-ventricular ejection fraction: the CHARM-Preserved Trial. Lancet. (2003) 362:777–81. doi: 10.1016/S0140-6736(03)14285-7
56. Zhirov I, Safronova N, Osmolovskaya Y, Alshevskaya A, Moskalev A, Tereshchenko S. Predictors of unfavorable outcomes in patients with atrial fibrillation and concomitant heart failure with different ejection fractions: RIF-CHF register one-year follow-up. Cardiol Res Pract. (2019) 2019:1692104. doi: 10.1155/2019/1692104
57. Zhou X, Shi R, Wu G, Zhu Q, Zhou C, Wang L, et al. The prevalence, predictors, and outcomes of spontaneous echocardiographic contrast or left ventricular thrombus in patients with HFrEF. ESC Heart Fail. (2021) 8:1284–94. doi: 10.1002/ehf2.13196
58. Davis PH, Clarke WR, Bendixen BH, Adams Jr HP, Woolson RF, Culebras A. Silent cerebral infarction in patients enrolled in the TOAST study. Neurology. (1996) 46:942–8. doi: 10.1212/WNL.46.4.942
59. Oliveira MMC, Sampaio EES, Kawaoka JR, Hatem MAB, Câmara EJN, Fernandes AMS, et al. Silent Cerebral Infarctions with Reduced, Mid-Range and Preserved Ejection Fraction in Patients with Heart Failure. Arq Bras Cardiol. (2018) 111:419–22. doi: 10.5935/abc.20180140
60. Frey A, Sell R, Homola GA, Malsch C, Kraft P, Gunreben I, et al. Cognitive deficits and related brain lesions in patients with chronic heart failure. JACC Heart Fail. (2018) 6:583–92. doi: 10.1016/j.jchf.2018.03.010
61. Stegmann T, Chu ML, Witte VA, Villringer A, Kumral D, Riedel-Heller SG, et al. Heart failure is independently associated with white matter lesions: insights from the population-based LIFE-Adult Study. ESC Heart Fail. (2021) 8:697–704. doi: 10.1002/ehf2.13166
62. Nyaga VN, Arbyn M, Aerts M. Metaprop: a Stata command to perform meta-analysis of binomial data. ArchPublic Health. (2014) 72:39. doi: 10.1186/2049-3258-72-39
63. Lin L, Chu H. Meta-analysis of proportions using generalized linear mixed models. Epidemiology. (2020) 31:1232. doi: 10.1097/EDE.0000000000001232
64. Higgins JPT, Thompson SG. Quantifying heterogeneity in a meta-analysis. Stat Med. (2002) 21:1539–58. doi: 10.1002/sim.1186
66. Antithrombotic Trialists' Collaboration. Collaborative meta-analysis of randomised trials of antiplatelet therapy for prevention of death, myocardial infarction, and stroke in high risk patients. BMJ. (2002) 324:71–86. doi: 10.1136/bmj.324.7329.71
67. Shahpouri MM, Mousavi S, Khorvash F, Mousavi SM, Hoseini T. Anticoagulant therapy for ischemic stroke: a review of literature. J Res Med Sci. (2012) 17:396–401. doi: 10.1161/CIRCULATIONAHA.120.050438
68. Hägg-Holmberg S, Dahlström EH, Forsblom CM, Harjutsalo V, Liebkind R, Putaala J, et al. The role of blood pressure in risk of ischemic and hemorrhagic stroke in type 1 diabetes. Cardiovasc Diabetol. (2019) 18:88. doi: 10.1186/s12933-019-0891-4
69. Chen R, Ovbiagele B, Feng W. Diabetes and stroke: epidemiology, pathophysiology, pharmaceuticals and outcomes. Am J Med Sci. (2016) 351:380–6. doi: 10.1016/j.amjms.2016.01.011
70. Lewis A, Segal A. Hyperlipidemia and primary prevention of stroke: does risk factor identification and reduction really work? Curr Atheroscler Rep. (2010) 12:225–9. doi: 10.1007/s11883-010-0117-4
71. Meschia JF, Bushnell C, Boden-Albala B, Braun LT, Bravata DM, Chaturvedi S, et al. Guidelines for the primary prevention of stroke. Stroke. (2014) 45:3754–832. doi: 10.1161/STR.0000000000000046
72. Hacke W, Bassand J-P, Virdone S, Camm AJ, Fitzmaurice DA, Fox KAA, et al. Prior stroke and transient ischemic attack as risk factors for subsequent stroke in atrial fibrillation patients: a report from the GARFIELD-AF registry. Int J Stroke. (2019) 15:308–17. doi: 10.1177/1747493019891516
73. Putaala J, Nieminen T. Stroke risk period after acute myocardial infarction revised. J Am Heart Assoc. (2018) 7:e011200. doi: 10.1161/JAHA.118.011200
74. Sterne JAC, Sutton AJ, Ioannidis JPA, Terrin N, Jones DR, Lau J, et al. Recommendations for examining and interpreting funnel plot asymmetry in meta-analyses of randomised controlled trials. BMJ. (2011) 343:d4002. doi: 10.1136/bmj.d4002
75. Egger M, Davey Smith G, Schneider M, Minder C. Bias in meta-analysis detected by a simple, graphical test. BMJ. (1997) 315:629–34. doi: 10.1136/bmj.315.7109.629
76. Rothstein HR, Sutton AJ, Borenstein M. Publication bias in meta-analysis. Prevent Assess Adjust. (2005) 2005:1–7. doi: 10.1002/0470870168.ch1
77. Kojima G, Avgerinou C, Iliffe S, Walters K. Adherence to mediterranean diet reduces incident frailty risk: systematic review and meta-analysis. J Am Geriatr Soc. (2018) 66:783–8. doi: 10.1111/jgs.15251
78. Saraiva MD, Suzuki GS, Lin SM, de Andrade DC, Jacob-Filho W, Suemoto CK. Persistent pain is a risk factor for frailty: a systematic review and meta-analysis from prospective longitudinal studies. Age Ageing. (2018) 47:785–93. doi: 10.1093/ageing/afy104
79. Kozdag G, Ciftci E, Ural D, Sahin T, Selekler M, Agacdiken A, et al. Silent cerebral infarction in chronic heart failure: ischemic and nonischemic dilated cardiomyopathy. Vasc Health Risk Manag. (2008) 4:463–9. doi: 10.2147/VHRM.S2166
80. Wardlaw JM, Valdés Hernández MC, Muñoz-Maniega S. What are white matter hyperintensities made of? Relevance to vascular cognitive impairment. J Am Heart Assoc. (2015) 4:001140. doi: 10.1161/JAHA.114.001140
81. Moroni F, Ammirati E, Hainsworth AH, Camici PG. Association of white matter hyperintensities and cardiovascular disease: the importance of microcirculatory disease. Circ Cardiovasc Imaging. (2020) 13:e010460. doi: 10.1161/CIRCIMAGING.120.010460
82. Alosco ML, Brickman AM, Spitznagel MB, Griffith EY, Narkhede A, Raz N, et al. Independent and interactive effects of blood pressure and cardiac function on brain volume and white matter hyperintensities in heart failure. J Am Soc Hypertens. (2013) 7:336–43. doi: 10.1016/j.jash.2013.04.011
83. Sauvé MJ, Lewis WR, Blankenbiller M, Rickabaugh B, Pressler SJ. Cognitive impairments in chronic heart failure: a case controlled study. J Card Fail. (2009) 15:1–10. doi: 10.1016/j.cardfail.2008.08.007
84. Smith EE, Saposnik G, Biessels GJ, Doubal FN, Fornage M, Gorelick PB, et al. Prevention of stroke in patients with silent cerebrovascular disease: a scientific statement for healthcare professionals from the American Heart Association/American Stroke Association. Stroke. (2017) 48:e44–71. doi: 10.1161/STR.0000000000000116
85. Kernan WN, Ovbiagele B, Black HR, Bravata DM, Chimowitz MI, Ezekowitz MD, et al. Guidelines for the prevention of stroke in patients with stroke and transient ischemic attack: a guideline for healthcare professionals from the American Heart Association/American Stroke Association. Stroke. (2014) 45:2160–236. doi: 10.1161/STR.0000000000000024
86. Aljaber NN, Mattash ZA, Alshoabi SA, Alhazmi FH. The prevalence of left ventricular thrombus among patients with low ejection fraction by trans-thoracic echocardiography. Pak J Med Sci. (2020) 36:673–7. doi: 10.12669/pjms.36.4.1972
87. Solomon SD, Anavekar N, Skali H, McMurray JJ, Swedberg K, Yusuf S, et al. Influence of ejection fraction on cardiovascular outcomes in a broad spectrum of heart failure patients. Circulation. (2005) 112:3738–44. doi: 10.1161/CIRCULATIONAHA.105.561423
88. Kotecha D, Chudasama R, Lane DA, Kirchhof P, Lip GY. Atrial fibrillation and heart failure due to reduced versus preserved ejection fraction: a systematic review and meta-analysis of death and adverse outcomes. Int J Cardiol. (2016) 203:660–6. doi: 10.1016/j.ijcard.2015.10.220
89. Kumar G, Goyal MK. Warfarin versus aspirin for prevention of stroke in heart failure: a meta-analysis of randomized controlled clinical trials. J Stroke Cerebrovasc Dis. (2013) 22:1279–87. doi: 10.1016/j.jstrokecerebrovasdis.2012.09.015
90. Senni M, Paulus WJ, Gavazzi A, Fraser AG, Díez J, Solomon SD, et al. New strategies for heart failure with preserved ejection fraction: the importance of targeted therapies for heart failure phenotypes. Eur Heart J. (2014) 35:2797–815. doi: 10.1093/eurheartj/ehu204
91. van der Meer P, Gaggin HK, Dec GW. ACC/AHA versus ESC guidelines on heart failure: JACC guideline comparison. J Am Coll Cardiol. (2019) 73:2756–68. doi: 10.1016/j.jacc.2019.03.478
92. Ay H, Furie KL, Singhal A, Smith WS, Sorensen AG, Koroshetz WJ. An evidence-based causative classification system for acute ischemic stroke. Ann Neurol. (2005) 58:688–97. doi: 10.1002/ana.20617
93. Alqahtani M, AlKhtaami A, AlGobain M, Aljohani N, Bin SA, AlShalati F, et al. Gender differences in the clinical characteristics of Saudi heart failure patients with subsequent stroke. Ann Saudi Med. (2013) 33:387–91. doi: 10.5144/0256-4947.2013.387
94. Massie BM, Carson PE, McMurray JJ, Komajda M, McKelvie R, Zile MR, et al. Irbesartan in patients with heart failure and preserved ejection fraction. N Engl J Med. (2008) 359:2456–67. doi: 10.1056/NEJMoa0805450
Keywords: heart failure, ischemic stroke, white matter hyperintensities, silent stroke, prevalence
Citation: Tan S, Ho CESM, Teo YN, Teo YH, Chan MY-Y, Lee C-H, Evangelista LKM, Lin W, Chong Y-F, Yeo T-C, Sharma VK, Wong RCC, Tan BYQ, Yeo LLL, Chai P and Sia C-H (2022) Prevalence and incidence of stroke, white matter hyperintensities, and silent brain infarcts in patients with chronic heart failure: A systematic review, meta-analysis, and meta-regression. Front. Cardiovasc. Med. 9:967197. doi: 10.3389/fcvm.2022.967197
Received: 12 June 2022; Accepted: 03 August 2022;
Published: 15 September 2022.
Edited by:
Andre Rodrigues Duraes, Federal University of Bahia, BrazilReviewed by:
Suvasini Lakshmanan, University of Iowa Hospitals and Clinics, United StatesHanbin Cui, Ningbo First Hospital, China
Paolo Sciarrone, Gabriele Monasterio Tuscany Foundation (CNR), Italy
Copyright © 2022 Tan, Ho, Teo, Teo, Chan, Lee, Evangelista, Lin, Chong, Yeo, Sharma, Wong, Tan, Yeo, Chai and Sia. This is an open-access article distributed under the terms of the Creative Commons Attribution License (CC BY). The use, distribution or reproduction in other forums is permitted, provided the original author(s) and the copyright owner(s) are credited and that the original publication in this journal is cited, in accordance with accepted academic practice. No use, distribution or reproduction is permitted which does not comply with these terms.
*Correspondence: Ching-Hui Sia, Y2hpbmdfaHVpX3NpYUBudWhzLmVkdS5zZw==
†These authors have contributed equally to this work
‡ORCID: Ching-Hui Sia orcid.org/0000-0002-2764-2869