- 1Department of Rehabilitation, Yamaguchi Prefectural Grand Medical Center, Hofu, Japan
- 2Division of Nursing and Laboratory Science, Yamaguchi University Graduate School of Medicine, Ube, Japan
- 3Department of Cardiology, Yamaguchi Prefectural Grand Medical Center, Hofu, Japan
Background: Strategies that accurately predict outcomes in elderly heart failure (HF) patients have not been sufficiently established. In previous reports, nutritional status, ability to perform activities of daily living (ADL), and lower limb muscle strength are known prognostic factors associated with cardiac rehabilitation (CR). In the present study, we investigated which CR factors can accurately predict one-year outcomes in elderly patients with HF among the above factors.
Methods: Hospitalized patients with HF over 65 years of age from January 2016 to January 2022 were retrospectively enrolled in the Yamaguchi Prefectural Grand Medical (YPGM) Center. They were consequently recruited to this single-center retrospective cohort study. Nutritional status, ADL, and lower limb muscle strength were assessed by geriatric nutritional risk index (GNRI), Barthel index (BI), and short physical performance battery (SPPB) at discharge, respectively. One year after discharge, the primary and secondary outcomes were evaluated by all-cause death or HF readmission and major adverse cardiac and cerebrovascular events (MACCE), respectively.
Results: Overall, 1,078 HF patients were admitted to YPGM Center. Of those, 839 (median age 84.0, 52% female) met the study criteria. During the follow-up of 228.0 days, 72 patients reached all-cause death (8%), 215 experienced HF readmission (23%), and 267 reached MACCE (30%: 25 HF death, six cardiac death, and 13 strokes). A multivariate Cox proportional hazard regression analysis revealed that the GNRI predicted the primary outcome (Hazard ratio [HR]: 0.957; 95% confidence interval [CI]: 0.934–0.980; p < 0.001) and the secondary outcome (HR: 0.963; 95%CI: 0.940–0.986; p = 0.002). Furthermore, a multiple logistic regression model using the GNRI most accurately predicted the primary and secondary outcomes compared to those with the SPPB or BI models.
Conclusion: A nutrition status model using GNRI provided a better predictive value than ADL ability or lower limb muscle strength. It should be recognized that HF patients with a low GNRI at discharge may have a poor prognosis at one year.
Introduction
Heart failure (HF) is a leading cause of morbidity and mortality, with a prevalence of 23 million worldwide (1–3). The number of patients is increasing in our super-aging society (4). Although new pharmacological (5, 6) and non-pharmacological therapies (7, 8) have been developed in the past decade, prognostic improvement in HF patients remains inadequate (9). Several studies have reported that elderly HF patients frequently present malnutrition (10), frailty (10, 11), sarcopenia (12, 13), and malnutrition resulting in poor prognosis (14, 15). In this regard, non-invasive interventions, including nutritional assessment and cardiac rehabilitation (CR), have recently attracted attention.
The Geriatric Nutritional Risk Index (GNRI), consisting of body mass index (BMI) and albumin, is a simple and versatile nutritional assessment tool in HF patients associated with all-cause or cardiac death (16–19). Previous reports have shown that a lower GNRI of less than 92 was associated with poor mortality (20, 21). On the other hand, a short physical performance battery (SPPB) provides a reasonable lower limb muscle-strength assessment value (22) and is also associated with physical balance ability. The SPPB consists of a 12-point scale, with even a one-point reduction representing a significant decline in lower limb physical function (23). The lower value of SPPB below seven is diagnosed as frailty and is associated with frequent rehospitalization and poor mortality (24–26). Moreover, the estimated activity of daily living (ADL) has been shown as another prognostic factor in HF patients, i.e., the Barthel index (BI) (27), which consists of 100 point scale assessing eating, grooming, ability to walk, and climb stairs, and management of toileting. HF patients with a low BI of less than 85 have been reported to go a poor prognosis (28).
In recent years, GNRI, BI, and SPPB have been used as rehabilitation indicators in CR to represent nutritional indices, ADL assessment, and lower limb physical activity capacity, respectively. Of these three assessment measures used in CR, it has not yet been established which is the most predictive outcome in elderly patients with HF (29–32).
This study examined which multivariate models using GNRI, SPPB, and BI best predicted outcomes at one year in hospitalized HF patients.
Method
Study population
From January 1, 2016, to January 31, 2022, at the Yamaguchi Prefectural Grand Medical (YPGM) Center, patients over 65 years, who were admitted to the emergency room due to acute decompensated HF (ADHF), hospitalized for treatment and underwent CR, were enrolled in this retrospective cohort study. The diagnosis of HF was made according to the Heart Failure Guidelines of the American Heart Association (AHA)/American College of Cardiology (ACC) (32) and the European Society of Cardiology (ESC) (31). Exclusion criteria were defined as follows: (1) in-hospital death, (2) lack of physical functional evaluation, (3) lost follow-up, and (4) no echocardiographic assessment. As part of comprehensive CR, we provided exercise therapy and nutritional guidance to all enrolled HF patients during hospitalization. Outpatient rehabilitation is provided to less than 5% of the enrolled HF inpatients. The study was performed per the Declaration of Helsinki and approved by the local institutional board at the YPGM Center (ID: 2022-013J). We applied the opt-out form to obtain informed consent by posting the document on our hospital website. Patients who did not want to participate in this study were instructed to contact the director.
Assessment with GNRI, SPPB, and BI
We evaluated the GNRI calculated as (14.89 × albumin) + 41.7 × [body weight(kg)/ideal body weight(kg)] (20) just before discharge. SPPB assessing a lower-limb function consists of gait speed (4-meter walk time), 5-second chair standing time, and balance (closing the legs, semi-tandem, and tandem). Each test scored from 0 to 4, with a total score ranging from 0 to 12 points; a higher score indicates a better lower limb function (22). BI measured the capacity in ADL, the BI is a major index with ten items, and the total BI score ranged between 0 and 100 points, with higher values indicating a higher level of independent physical state (27). The cut-off value of BI was identified that 85 points, 60 points, and 40 points, respectively (28). SPPB and BI were evaluated within five days of discharge, and body weight and serum albumin for calculating GNRI were assessed just before discharge.
Echocardiographic study
A comprehensive echocardiographic examination was performed with the patient in stable condition within two weeks of admission. Two-dimensional measurements, including left ventricular end-diastolic dimension (LVDd), left ventricular end-systolic dimension (LVDs), and left atrial dimension (LAD), were obtained according to the recommendations of the American Society of Echocardiography (33). Apical four- and two-chamber views were used for calculating LVEF using biplane disk methods. The index, e′, was measured using tissue Doppler imaging, and the ratio of E-wave to e′ (E/e′) was calculated using the mean of the septal and lateral velocities (33). Trans-tricuspid pressure gradient (TR-PG) was measured using continuous-wave Doppler echocardiography. Right atrial pressure was estimated from the inferior vena cava (IVC) diameter and collapsibility. The estimated pulmonary arterial pressure (ePAP) was calculated as the sum of TR-PG and right atrial pressure.
Baseline clinical characteristics, medication, and physical functional assessment
The baseline clinical characteristics were obtained from the electrical medical records. These characteristics include the following parameters; age, sex, living alone, returning home, nursing care insurance, history of HF, etiology of HF, and New York Heart Association (NYHA) functional classification on admission and at the time of discharge. The etiology of HF patients was classified from the medical record into seven categories: ischemic heart disease (IHD), valvular heart disease (VHD), cardiomyopathy (CM), hypertensive heart disease (HHD), arrhythmia (atrial fibrillation [Afib] and complete atrioventricular block [CAVB]), other [chronic kidney disease (CKD) and anemia], and uncertain. HF co-morbidities were evaluated for the presence of hypertension, diabetes mellitus, AFib, CKD (defined as eGFR <60 ml/min/1.73 m2), chronic obstructive pulmonary disease (COPD), stroke, and orthopedic disease. Usage of the following standard medications for HF was reviewed at discharge: angiotensin-converting enzyme inhibitors (ACE-I)/angiotensin II receptor blockers (ARB)/angiotensin receptor neprilysin inhibitors (ARNI), β-blockers, tolvaptan, loop diuretics, mineralocorticoid receptor antagonists (MRAs), and sodium-glucose cotransporter 2 (SGLT2) inhibitors. The laboratory data were also obtained from medical records, including B-type natriuretic peptide (BNP) at admission, albumin, hemoglobin, creatinine, and estimated glomerular filtration rate (eGFR). Physical functions were evaluated five days before discharge. These include the SPPB test, BI, a handgrip test by a grip strength meter (T.K.K.5401 GRIP-D; Takei, Tokyo, Japan), and the quadriceps isometric strength (QIS) test by a handheld dynamometer (MT-100 mobile; Sakai Med, Tokyo, Japan) (34). Exercise tolerance was evaluated by the 6 min walking test (6MWT) (35).
Clinical outcomes and follow-up period
The primary outcome was evaluated as a composite of all-cause death or HF readmission one year after discharge. The secondary outcome was assessed as major adverse cardiac and cerebrovascular events (MACCE), i.e., cardiac death, HF death, HF readmission, acute myocardial infarction, unstable angina, aortic dissection, and stroke at one year. Medical information regarding one-year outcomes was collected from electric charts and by letter when available.
Statistical analysis
Statistical analysis was performed using the EZR on R commander (version 1.37) (36). Categorical variables were expressed as numbers, and percentage (%) or continuous variables were expressed as means ± standard deviation or median [interquartile range (IQR): 25th to 75th percentiles]. We calculated the correlation coefficient between GNRI, SPPB, and BI using Pearson's or Spearman's rank correlation coefficient.
The univariate and multivariate Cox proportional hazard regression analyses identified predictors of primary and secondary outcomes. Independent variables for multiple modeling were selected from predictive factors with p < 0.10 using the univariate analysis and previously reported predictive factors, i.e., age, sex, LVEF, SPPB, BI, GNRI, and the natural logarithm of BNP. Since the variance of BNP in HF patients is extremely large and does not represent a normal distribution, its natural logarithm (Log BNP) was used for multivariate analysis. A stepwise variable reduction method was then used for the multivariate modeling. Results were provided as hazard ratio (HR), 95% confidence interval (CI), and p-value. When the multivariate analysis identified the predictors of continuous variables, a receiver operating characteristic (ROC) analysis was employed to determine the optimal cut-off value acting as independent predictive factors, followed by the sensitivity, the specificity, and the area under the curve (AUC). Event-free ratios were estimated by the Kaplan– Meier method and compared by the log-rank test.
Moreover, we considered three multivariate prognostic risk score models. The AUC was described to compare the prognosis index of GNRI, SPPB, and BI for predicting all-cause death or HF readmission and MACCE. Three prognosis risk score models adjusted for age, sex, and LVEF were developed to compare GNRI, SPPB, and BI based on the regression coefficient (37).
A p-value of less than 0.05 indicated a statistically significant.
Results
Patient clinical characteristics
Overall, 1,078 patients with ADHF were admitted to the YPGM Center, as shown in Figure 1. Of those, 181 met the exclusion criteria. Briefly, 77 died in hospital treatment, 104 were not evaluated for physical functional assessment, and 58 patients were lost to follow-up. Ultimately, 839 patients were analyzed. The median age of included patients was 84.0 (IQR: 78.0–89.0), the prevalence of females was 436 (52%), and the length of hospital stay was 20.0 (IQR: 14.0–28.0) days in Table 1. We diagnosed 233 (28%) patients with HF with reduced LVEF (LVEF < 40%: HFrEF), 153 (18%) patients with HF with mildly reduced LVEF (LVEF 40%–49%: HFmrEF), and 453 (54%) patients with HF with preserved LVEF (LVEF ≥ 50%: HFpEF) (31, 32).
One-year outcomes of the analyzed ADHF patients
The median follow-up was 228.0 days. During the follow-up period, 72 patients reached all-cause death (8%), 215 experienced HF readmission (24%), and 267 reached MACCE (30%: 25 HF death, six cardiac death, and 13 strokes).
Univariate analysis of the primary outcome, i.e., all-cause death or HF readmission, selected 22 variables with a p-value of less than 0.10, including age, sex, LVEF, Log BNP, GNRI, SPPB, and BI. Multivariate Cox proportional hazard regression analysis revealed that the independent predictors were the history of HF, nursing care insurance, arrhythmia (etiology), LAD, Log BNP, GNRI, and BI. SPPB was not, as shown in Table 2.
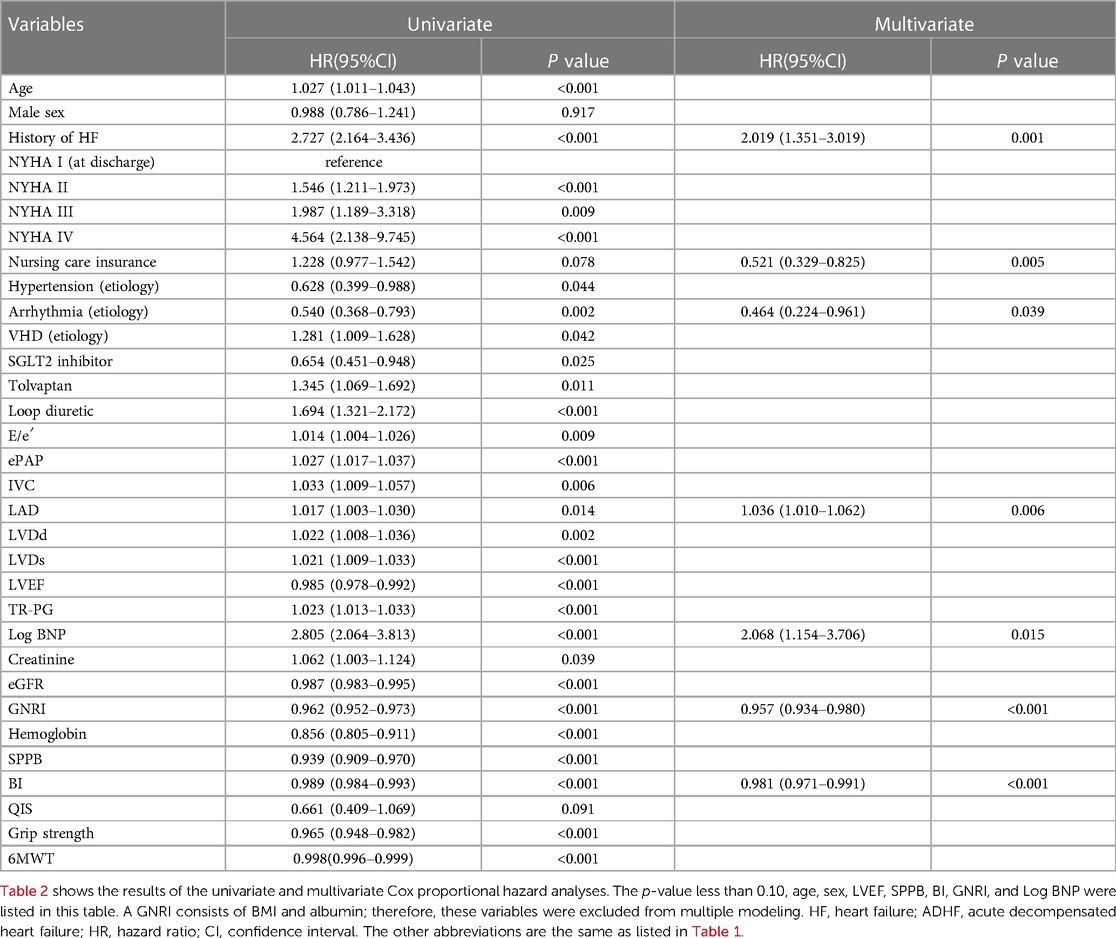
Table 2. Univariate and multivariate cox proportional hazard analyses to predict All-cause death or HF readmission after discharge of 839 patients with ADHF.
Second, for analysis of secondary outcome, i.e., MACCE, univariate analysis revealed 19 variables with a p-value of less than 0.10, including age, sex, LVEF, Log BNP, GNRI, SPPB, and BI. Multivariate Cox proportional hazard regression analysis revealed the NYHA (at discharge), VHD (etiology), LAD, LVEF, Log BNP, eGFR, and GNRI as independent predictors, while SPPB and BI were not, as shown in Table 3.
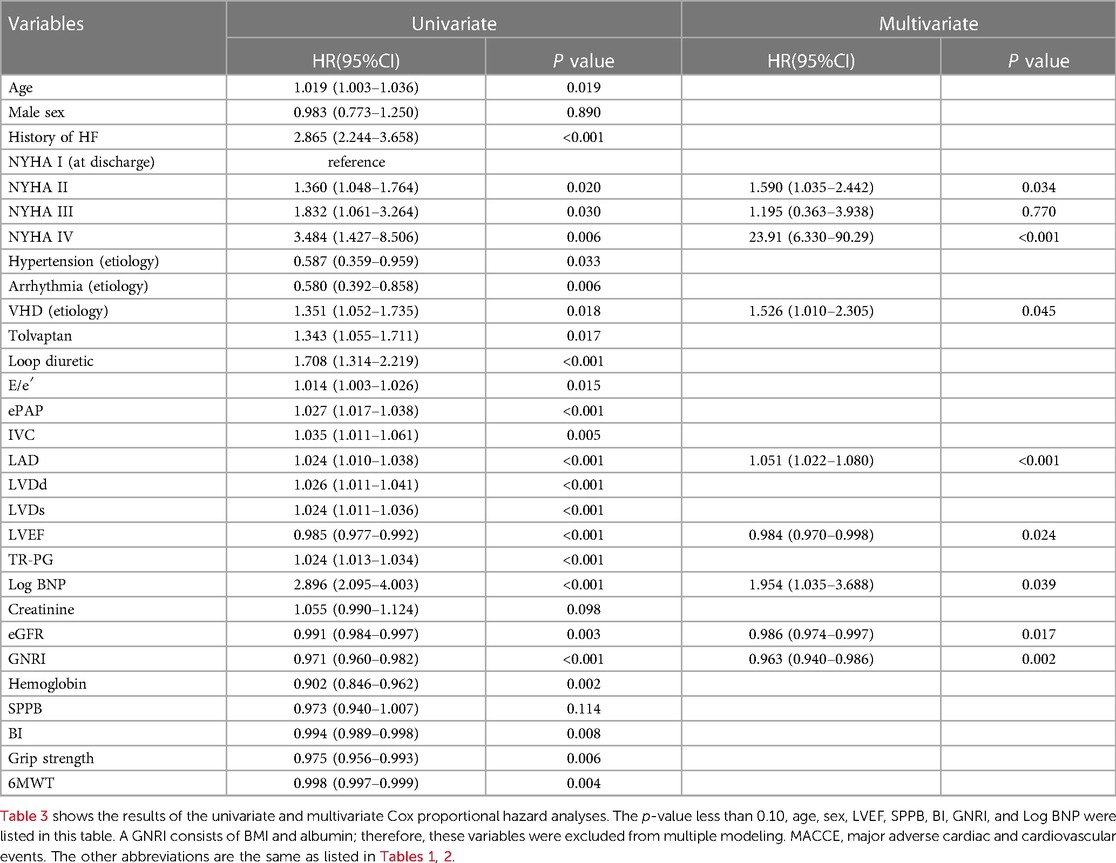
Table 3. Univariate and multivariate cox proportional hazard analyzes to predict MACCE after discharge of 839 patients with ADHF.
The ROC analysis of the primary outcome revealed the maximum AUC as 0.631 (95% CI; 0.592–0.670) when the cut-off value of GNRI was set to 87.6 (Figure 2A). The Kaplan–Meier curve showed a significantly higher incidence of the primary endpoint in patients with GNRI < 87.6 than in those with GNRI ≥ 87.6 (Figure 2B).
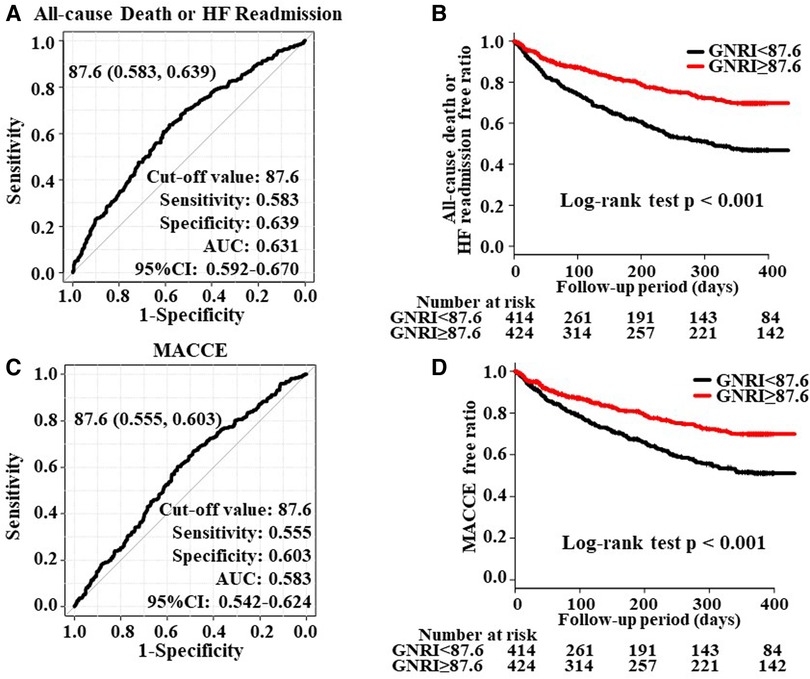
Figure 2. Panels (A, C) show the ROC curve to predict the cut-off value of all-cause death, HF readmission, and MACCE overall HF patients (n = 839). Panels (B, D) show the Kaplan-Meier curve to evaluate the cumulative incidences of all-cause death or HF readmission and MACCE.
The ROC analysis of the secondary endpoint revealed the maximum AUC as 0.583 (95% CI; 0.542–0.624; Figure 2C) and a similar Kaplan-Meier curve to the primary endpoint (Figure 2D).
Comparison with multivariate predicting risk score models using GNRI, SPPB, and BI
We then created multiple logistic models using GNRI, SPPB, and BI with age, sex, and LVEF and examined which model could provide the largest AUC. As shown in Figure 3, the GNRI model (Model 3) presented the largest AUC compared to those of the SPPB (Model 1) and the BI (Model 2) for predicting the primary endpoint. On the other hand, analysis for predicting the secondary endpoint did not reach statistical significance among the three groups (Model 4:SPPB, Model 5:BI, Model 6:GNRI), as presented in Supplementary Figure S1.
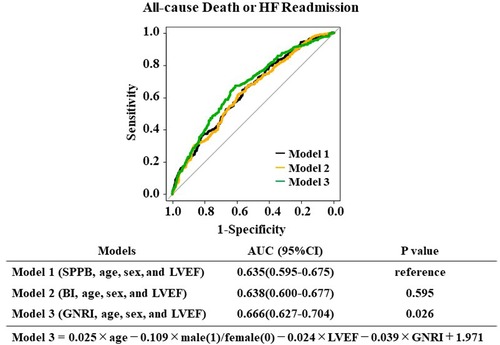
Figure 3. The risk score models for predicting all-cause death or HF readmission and MACCE were compared using the area under the receiver operating characteristic curves.
Relationships between GNRI, SPPB, BI, and one-year outcomes
Malnutrition, frailty, and low ADL may coexist in patients with poor prognoses of HF. Although SPPB, BI, and GNRI, which were analyzed in this study, each represent these indices, we thought it necessary to investigate their relationship. Therefore, we examined the correlation among GNRI, SPPB, and BI as continuous variables (Figure 4). SPPB and BI showed a high correlation coefficient (r = 0.779) compared to that between GNRI and SPPB (r = 0.369) or GNRI and BI (r = 0.412). These data suggest that GNRI is highly independent of other CR-associated factors.
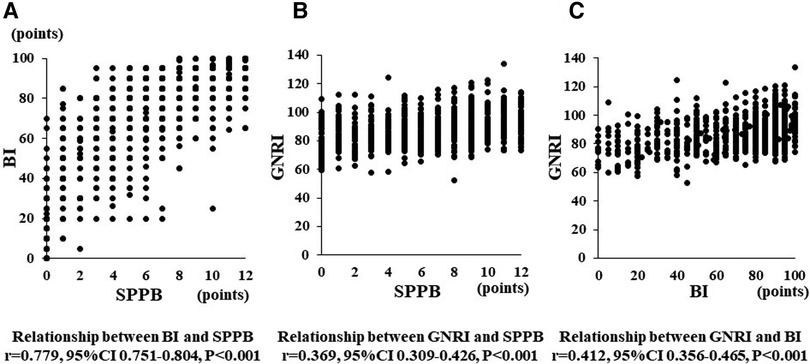
Figure 4. Panel (A–C) show a relationship between BI and SPPB, GNRI and SPPB, and GNRI and BI, respectively.
Discussion
In the present study, we investigated whether any of the three poor prognostic factors in elderly HF patients, including low nutrition, frailty, and decline in ADL, could most accurately predict prognosis. We found that the GNRI, a simple and versatile measure of nutrition, was the most accurate and strongly correlated with prognosis one year after discharge. To the best of our knowledge, the finding has not been reported before, and we believe it is extremely important for predicting the prognosis of elderly HF patients.
GNRI, a good measure of the nutritional index in hospitalized elderly HF patients
Several indicators of malnutrition in elderly HF patients have been reported. These include albumin (16), BMI (38), MNA-SF (39), and the Global Leadership Initiative on Malnutrition (GLIM) criteria (40). Nutritional assessment of HF patients has been performed using these indices, and it has been reported that malnutrition is strongly associated with adverse outcomes (14, 15). On the other hand, which of the nutritional indices is best is controversial and was not investigated in this study. For example, the MNA-SF is a tool to evaluate nutritional status over the past three months (39). Although MNA-SF is considered a good indicator for outpatient (41), it is not suitable for accurate prediction during acute exacerbations of HF since body weight can change quickly due to systemic edema and congestion. In this regard, we expected that GNRI would be a good predictor in hospitalized patients, based on previous reports in hospitalized HFpEF patients and our previous report on its usefulness as a prognostic indicator in VHD patients (21, 42). We found that the GNRI predicted all-cause mortality or HF hospitalization one year after discharge with high predictive accuracy. Although the cut-off value of the GNRI for predicting outcomes was even lower than in previous reports, it possibly related to the high proportion of very elderly patients in this study.
Unique correlations between GNRI and other CR-associated factors in elderly HF patients
In the ROC analysis conducted in this study, a multivariate model was created by adjusting GNRI, SPPB, and BI, indicators used in CR, for age, sex, and LVEF. This method makes more systematic predictions by adding variables expected to be affected by the ROC analysis, usually performed univariately as multivariate factors (21). As a result, we found that the model using GNRI was the most accurate in predicting prognosis. In a previous study (21), GNRI was reported to be a good prognostic indicator in patients with HFpEF. The present study confirmed the benefit of the GNRI even when LVEF values are included in the prediction model, suggesting that the GNRI is an accurate predictor for patients with HFpEF and other categories of elderly HF patients. However, there is room for further investigation as to why the model using GNRI obtained the highest AUC.
The fact that GNRI, measured once before discharge, accurately predicts patient prognosis one year later is very interesting. When we examined the correlation between GNRI, SPPB, and BI in elderly HF patients, we found a strong correlation between SPPB and BI. On the other hand, the correlation coefficients between GNRI and SPPB, or GNRI and BI, were not high enough compared to that between SPPB and BI. These findings suggest that GNRI does not simply reflect ADL ability or lower muscle strength but is associated with whole-body nutritional status. Very few reports showed that CR improved GNRI value (26, 43). In contrast, SPPB and BI have been clearly shown to improve with the continuous effort of CR (44). In this sense, HF patients with high BMI are known to have a relatively preserved prognosis, i.e., an obesity paradox (14). It is suggested that GNRI is not a simple nutritional assessment tool but also a general prognostic indicator of HF patients.
In contrast, the secondary outcome, MACCE, did not differ among the three risk score models. Previous studies have suggested that non-cardiovascular death and non-cardiovascular events are associated with outcomes in elderly HF patients (45, 46). This study's high median patient age (84 years) may have contributed to the analysis results.
Recently, as a new pharmacologic therapy for chronic HF, several large clinical trials have held great promise for preventing HF hospitalization in patients with HFpEF and HFrEF (31, 47, 48). SGLT2 inhibitors act by excreting sugar ingested as food in the urine and, therefore, may induce a combined risk of weight loss in patients with HF. In this sense, they may not positively impact HF outcomes in patients with low GNRI. Nevertheless, its use is strongly recommended in HF guidelines (31, 32). In the present study, the prescription rate of SGLT2 inhibitors in patients with lower GNRI was lower than in patients with higher GNRI (Supplementary Table S1). Indeed, some cases of marked weight loss, ketoacidosis, and gastrointestinal symptoms have been experienced with administration in elderly patients. Further studies are needed to evaluate the benefit of SGLT2 inhibitors and their impact on nutritional and physical indices in hospitalized elderly HF patients.
Factors other than nutrition that affect GNRI in elderly HF patients
Albumin, used to assess GNRI, has also been reduced in HF patients due to factors other than nutrition. These factors include hemodilution due to congestion, inflammation, and reduced albumin synthesis due to a congested liver. Indeed, patients have relatively low albumin levels immediately after HF hospitalization due to the effects of intravascular volume loading, assessed low by GNRI. The intravascular capacitance decreases as HF treatment progress, and albumin is conversely thought to increase. Albumin is less likely to improve in concomitant inflammation or persistent congestive liver disease cases associated with HF (49, 50). In this regard, it should be remembered that in addition to its aspect as a nutritional indicator, the GNRI on admission also has another aspect reflecting HF severity (20, 21, 51). In contrast, GNRIs evaluated at discharge are unlikely to be influenced by the circulatory dynamics of patients with exacerbated HF. In the present study, therefore, we used the value of GNRI at discharge. Recent studies (42, 52) have shown that GNRI at discharge is a more beneficial indicator of long-term prognosis than assessment at admission, which we believe supports our assessment methodology.
We should consider that elderly HF patients who responded adequately to HF treatment are prone to reduced dietary intake due to multiple factors (53). Those factors include environmental changes due to emergency hospitalization, intestinal edema, and the influence on cognitive function (54).
Limitation
This study has several limitations. First, as our region has an advanced aging society, it is unclear whether these results can apply to patients in other hospitals or regions. Second, this study was a single-center, retrospective study with a small sample size; there were no consecutive cases and lost follow-up. Third, this cohort was exclusively Japanese, not including other races such as African American, White, Pacific, or others. Further study is required to examine the large multicenter sample size.
Conclusion
In hospitalized elderly HF patients, GNRI is associated with all-cause mortality or HF rehospitalization and MACCE; nutritional status assessed by GNRI was a significant predictor independent of age, cardiac parameters, and physical function. Although there are many challenges in intervention methods, improving the nutritional status of hospitalized elderly HF patients is highly desirable. It should also be recognized that HF patients with a low GNRI at discharge may have a poor prognosis at one year, even if other measures of HF severity, such as BNP, are better.
Data availability statement
The original contributions presented in the study are included in the article/Supplementary Material, further inquiries can be directed to the corresponding author/s.
Ethics statement
The studies involving human participants were reviewed and approved by the local institutional board of the Yamaguchi Prefectural Grand Medical Center (ID: 2022-J013). Written informed consent for participation was not required for this study in accordance with the national legislation and the institutional requirements.
Author contributions
MM was the primary investigator for this study, and MM and SO performed the statistical analysis and writing of the project. MM, SK and YO collated data. YI and TY were the project supervisors; they reviewed all documents and helped analyze the data, figures, and tables. KM, FN, and TU reviewed the manuscript and offered insights based on their own experiences. All authors contributed to the article and approved the submitted version.
Conflict of interest
The authors declare that the research was conducted in the absence of any commercial or financial relationships that could be construed as a potential conflict of interest.
Publisher's note
All claims expressed in this article are solely those of the authors and do not necessarily represent those of their affiliated organizations, or those of the publisher, the editors and the reviewers. Any product that may be evaluated in this article, or claim that may be made by its manufacturer, is not guaranteed or endorsed by the publisher.
Supplementary material
The Supplementary Material for this article can be found online at: https://www.frontiersin.org/articles/10.3389/fcvm.2023.1190548/full#supplementary-material.
Supplementary Figure S1
The risk score models for predicting MACCE were compared using the area under the receiver operating characteristic curves.
References
1. Heidenreich PA, Albert NM, Allen LA, Bluemke DA, Butler J, Fonarow GC, et al. Forecasting the impact of heart failure in the United States: a policy statement from the American heart association. Circ Heart Fail. (2013) 6:606–19. doi: 10.1161/HHF.0b013e318291329a
2. Teerlink JR, Alburikan K, Metra M, Rodgers JE. Acute decompensated heart failure update. Curr Cardiol Rev. (2015) 11:53–62. doi: 10.2174/1573403X09666131117174414
3. Kurmani S, Squire I. Acute heart failure: definition, classification and epidemiology. Curr Heart Fail Rep. (2017) 14:385–92. doi: 10.1007/s11897-017-0351-y
4. Hamaguchi S, Kinugawa S, Tsuchihashi-Makaya M, Goto D, Yamada S, Yokoshiki H, et al. Characteristics, management, and outcomes for patients during hospitalization due to worsening heart failure-A report from the Japanese Cardiac Registry of Heart Failure in Cardiology (JCARE-CARD). J Cardiol. (2013) 62:95–101. doi: 10.1016/j.jjcc.2013.03.009
5. McMurray JJ, Packer M, Desai AS, Gong J, Lefkowitz MP, Rizkala AR, et al. Angiotensin-neprilysin inhibition vs. enalapril in heart failure. N Engl J Med. (2014) 371:993–1004. doi: 10.1056/NEJMoa1409077
6. Zannad F, Ferreira JP, Pocock SJ, Anker SD, Butler J, Filippatos G, et al. SGLT2 Inhibitors in patients with heart failure with reduced ejection fraction: a meta-analysis of the EMPEROR-reduced and DAPA-HF trials. Lancet. (2020) 396:819–29. doi: 10.1016/s0140-6736(20)31824-9
7. Stone GW, Lindenfeld J, Abraham WT, Kar S, Lim DS, Mishell JM, et al. Transcatheter mitral-valve repair in patients with heart failure. N Engl J Med. (2018) 379:2307–18. doi: 10.1056/NEJMoa1806640
8. Mack MJ, Leon MB, Thourani VH, Makkar R, Kodali SK, Russo M, et al. Transcatheter aortic-valve replacement with a balloon-expandable valve in low-risk patients. N Engl J Med. (2019) 380:1695–705. doi: 10.1056/NEJMoa1814052
9. Chen L, Ionescu-Ittu R, Romdhani H, Guerin A, Kessler P, Borentain M, et al. Disease management and outcomes in patients hospitalized for acute heart failure in Japan. Cardiol Ther. (2021) 10:211–28. doi: 10.1007/s40119-021-00212-y
10. Tanaka S, Kamiya K, Hamazaki N, Matsuzawa R, Nozaki K, Maekawa E, et al. Incremental value of objective frailty assessment to predict mortality in elderly patients hospitalized for heart failure. J Card Fail. (2018) 24:723–32. doi: 10.1016/j.cardfail.2018.06.006
11. Volpato S, Cavalieri M, Sioulis F, Guerra G, Maraldi C, Zuliani G, et al. Predictive value of the short physical performance battery following hospitalization in older patients. J Gerontol A Biol Sci Med Sci. (2011) 66:89–96. doi: 10.1093/gerona/glq167
12. Cruz-Jentoft AJ, Baeyens JP, Bauer JM, Boirie Y, Cederholm T, Landi F, et al. Sarcopenia: European consensus on definition and diagnosis: report of the European working group on sarcopenia in older people. Age Ageing. (2010) 39:412–23. doi: 10.1093/ageing/afq034
13. Fülster S, Tacke M, Sandek A, Ebner N, Tschöpe C, Doehner W, et al. Muscle wasting in patients with chronic heart failure: results from the studies investigating co-morbidities aggravating heart failure (SICA-HF). Eur Heart J. (2013) 34:512–9. doi: 10.1093/eurheartj/ehs381
14. Anker SD, Ponikowski P, Varney S, Chua TP, Clark AL, Webb-Peploe KM, et al. Wasting as independent risk factor for mortality in chronic heart failure. Lancet. (1997) 349:1050–3. doi: 10.1016/s0140-6736(96)07015-8
15. Ponikowski P, Voors AA, Anker SD, Bueno H, Cleland JGF, Coats AJS, et al. 2016 ESC guidelines for the diagnosis and treatment of acute and chronic heart failure: the task force for the diagnosis and treatment of acute and chronic heart failure of the European Society of Cardiology (ESC)Developed with the special contribution of the Heart Failure Association (HFA) of the ESC. Eur Heart J. (2016) 37:2129–200. doi: 10.1093/eurheartj/ehw128
16. Uthamalingam S, Kandala J, Daley M, Patvardhan E, Capodilupo R, Moore SA, et al. Serum albumin and mortality in acutely decompensated heart failure. Am Heart J. (2010) 160:1149–55. doi: 10.1016/j.ahj.2010.09.004
17. Hamaguchi S, Kinugawa S, Goto D, Tsuchihashi-Makaya M, Yokota T, Yamada S, et al. Predictors of long-term adverse outcomes in elderly patients over 80 years hospitalized with heart failure. - A report from the Japanese Cardiac Registry of Heart Failure in Cardiology (JCARE-CARD). Circ J. (2011) 75:2403–10. doi: 10.1253/circj.cj-11-0267
18. Takiguchi M, Yoshihisa A, Miura S, Shimizu T, Nakamura Y, Yamauchi H, et al. Impact of body mass index on mortality in heart failure patients. Eur J Clin Invest. (2014) 44:1197–205. doi: 10.1111/eci.12354
19. Matsushita M, Shirakabe A, Hata N, Shinada T, Kobayashi N, Tomita K, et al. Association between the body mass index and the clinical findings in patients with acute heart failure: evaluation of the obesity paradox in patients with severely decompensated acute heart failure. Heart Vessels. (2017) 32:600–8. doi: 10.1007/s00380-016-0908-9
20. Bouillanne O, Morineau G, Dupont C, Coulombel I, Vincent JP, Nicolis I, et al. Geriatric nutritional risk index: a new index for evaluating at-risk elderly medical patients. Am J Clin Nutr. (2005) 82:777–83. doi: 10.1093/ajcn/82.4.777
21. Kinugasa Y, Kato M, Sugihara S, Hirai M, Yamada K, Yanagihara K, et al. Geriatric nutritional risk index predicts functional dependency and mortality in patients with heart failure with preserved ejection fraction. Circ J. (2013) 77:705–11. doi: 10.1253/circj.cj-12-1091
22. Guralnik JM, Simonsick EM, Ferrucci L, Glynn RJ, Berkman LF, Blazer DG, et al. A short physical performance battery assessing lower extremity function: association with self-reported disability and prediction of mortality and nursing home admission. J Gerontol. (1994) 49:M85–94. doi: 10.1093/geronj/49.2.m85
23. Perera S, Mody SH, Woodman RC, Studenski SA. Meaningful change and responsiveness in common physical performance measures in older adults. J Am Geriatr Soc. (2006) 54:743–9. doi: 10.1111/j.1532-5415.2006.00701.x
24. Newman AB, Gottdiener JS, McBurnie MA, Hirsch CH, Kop WJ, Tracy R, et al. Associations of subclinical cardiovascular disease with frailty. J Gerontol A Biol Sci Med Sci. (2001) 56:M158–66. doi: 10.1093/gerona/56.3.m158
25. Sze S, Zhang J, Pellicori P, Morgan D, Hoye A, Clark AL. Prognostic value of simple frailty and malnutrition screening tools in patients with acute heart failure due to left ventricular systolic dysfunction. Clin Res Cardiol. (2017) 106:533–41. doi: 10.1007/s00392-017-1082-5
26. Perracini MR, Mello M, de Oliveira Máximo R, Bilton TL, Ferriolli E, Lustosa LP, et al. Diagnostic accuracy of the short physical performance battery for detecting frailty in older people. Phys Ther. (2020) 100:90–8. doi: 10.1093/ptj/pzz154
27. Mahoney FI, Barthel DW. Functional evaluation: the barthel index. Md State Med J. (1965) 14:61–5.14258950
28. Granger CV, Dewis LS, Peters NC, Sherwood CC, Barrett JE. Stroke rehabilitation: analysis of repeated Barthel index measures. Arch Phys Med Rehabil. (1979) 60:14–7.420565
29. Long L, Mordi IR, Bridges C, Sagar VA, Davies EJ, Coats AJ, et al. Exercise-based cardiac rehabilitation for adults with heart failure. Cochrane Database Syst Rev. (2019) 1:Cd003331. doi: 10.1002/14651858.CD003331.pub5
30. Kamiya K, Sato Y, Takahashi T, Tsuchihashi-Makaya M, Kotooka N, Ikegame T, et al. Multidisciplinary cardiac rehabilitation and long-term prognosis in patients with heart failure. Circ Heart Fail. (2020) 13:e006798. doi: 10.1161/circheartfailure.119.006798
31. McDonagh TA, Metra M, Adamo M, Gardner RS, Baumbach A, Böhm M, et al. 2021 ESC guidelines for the diagnosis and treatment of acute and chronic heart failure. Eur Heart J. (2021) 42:3599–726. doi: 10.1093/eurheartj/ehab368
32. Correction to: 2022 AHA/ACC/HFSA guideline for the management of heart failure: a report of the American college of cardiology/American heart association joint committee on clinical practice guidelines. Circulation. (2022) 145:e1033. doi: 10.1161/cir.0000000000001073
33. Nagueh SF, Smiseth OA, Appleton CP, Byrd BF 3rd, Dokainish H, Edvardsen T, et al. Recommendations for the evaluation of left ventricular diastolic function by echocardiography: an update from the American society of echocardiography and the European association of cardiovascular imaging. J Am Soc Echocardiogr. (2016) 29:277–314. doi: 10.1016/j.echo.2016.01.011
34. Kamiya K, Masuda T, Tanaka S, Hamazaki N, Matsue Y, Mezzani A, et al. Quadriceps strength as a predictor of mortality in coronary artery disease. Am J Med. (2015) 128:1212–9. doi: 10.1016/j.amjmed.2015.06.035
35. ATS Committee on Proficiency Standards for Clinical Pulmonary Function Laboratories. ATS statement: guidelines for the six-minute walk test. Am J Respir Crit Care Med. (2002) 166:111–7. doi: 10.1164/ajrccm.166.1.at1102
36. Kanda Y. Investigation of the freely available easy-to-use software ‘EZR’ for medical statistics. Bone Marrow Transplant. (2013) 48:452–8. doi: 10.1038/bmt.2012.244
37. Kinugasa Y, Kato M, Sugihara S, Hirai M, Kotani K, Ishida K, et al. A simple risk score to predict in-hospital death of elderly patients with acute decompensated heart failure–hypoalbuminemia as an additional prognostic factor. Circ J. (2009) 73:2276–81. doi: 10.1253/circj.cj-09-0498
38. Sharma A, Lavie CJ, Borer JS, Vallakati A, Goel S, Lopez-Jimenez F, et al. Meta-analysis of the relation of body mass index to all-cause and cardiovascular mortality and hospitalization in patients with chronic heart failure. Am J Cardiol. (2015) 115:1428–34. doi: 10.1016/j.amjcard.2015.02.024
39. Sargento L, Satendra M, Almeida I, Sousa C, Gomes S, Salazar F, et al. Nutritional status of geriatric outpatients with systolic heart failure and its prognostic value regarding death or hospitalization, biomarkers and quality of life. J Nutr Health Aging. (2013) 17:300–4. doi: 10.1007/s12603-013-0030-y
40. Cederholm T, Jensen GL, Correia M, Gonzalez MC, Fukushima R, Higashiguchi T, et al. GLIM Criteria for the diagnosis of malnutrition—a consensus report from the global clinical nutrition community. Clin Nutr. (2019) 38:1–9. doi: 10.1016/j.clnu.2018.08.002
41. Kinugasa Y, Sota T, Kamitani H, Nakayama N, Nakamura K, Hirai M, et al. Diagnostic performance of nutritional indicators in patients with heart failure. ESC Heart Fail. (2022) 9:2096–106. doi: 10.1002/ehf2.13886
42. Miura M, Okuda S, Murata K, Nagai H, Ueyama T, Nakao F, et al. Malnutrition and frailty are critical determinants of 6-month outcome in hospitalized elderly patients with heart failure harboring surgically untreated functional mitral regurgitation. Front Cardiovasc Med. (2021) 8:764528. doi: 10.3389/fcvm.2021.764528
43. Li H, Cen K, Sun W, Feng B. Prognostic value of geriatric nutritional risk index in elderly patients with heart failure: a meta-analysis. Aging Clin Exp Res. (2021) 33:1477–86. doi: 10.1007/s40520-020-01656-3
44. Kitzman DW, Whellan DJ, Duncan P, Pastva AM, Mentz RJ, Reeves GR, et al. Physical rehabilitation for older patients hospitalized for heart failure. N Engl J Med. (2021) 385:203–16. doi: 10.1056/NEJMoa2026141
45. Hamaguchi S, Kinugawa S, Sobirin MA, Goto D, Tsuchihashi-Makaya M, Yamada S, et al. Mode of death in patients with heart failure and reduced vs. preserved ejection fraction: report from the registry of hospitalized heart failure patients. Circ J. (2012) 76:1662–9. doi: 10.1253/circj.cj-11-1355
46. Nagai T, Yoshikawa T, Saito Y, Takeishi Y, Yamamoto K, Ogawa H, et al. Clinical characteristics, management, and outcomes of Japanese patients hospitalized for heart failure with preserved ejection fraction- A report from the Japanese heart failure syndrome with preserved ejection fraction (JASPER) registry. Circ J. (2018) 82:1534–45. doi: 10.1253/circj.CJ-18-0073
47. Packer M, Anker SD, Butler J, Filippatos G, Pocock SJ, Carson P, et al. Cardiovascular and renal outcomes with empagliflozin in heart failure. N Engl J Med. (2020) 383:1413–24. doi: 10.1056/NEJMoa2022190
48. Anker SD, Butler J, Filippatos G, Ferreira JP, Bocchi E, Böhm M, et al. Empagliflozin in heart failure with a preserved ejection fraction. N Engl J Med. (2021) 385:1451–61. doi: 10.1056/NEJMoa2107038
49. Ballmer PE. Causes and mechanisms of hypoalbuminaemia. Clin Nutr. (2001) 20:271–3. doi: 10.1054/clnu.2001.0439
50. Chojkier M. Inhibition of albumin synthesis in chronic diseases: molecular mechanisms. J Clin Gastroenterol. (2005) 39:S143–6. doi: 10.1097/01.mcg.0000155514.17715.39
51. Ouchi S, Miyazaki T, Shimada K, Sugita Y, Shimizu M, Murata A, et al. Low docosahexaenoic acid, dihomo-gamma-linolenic acid, and arachidonic acid levels associated with long-term mortality in patients with acute decompensated heart failure in different nutritional statuses. Nutrients. (2017) 9. doi: 10.3390/nu9090956
52. Ono M, Mizuno A, Kohsaka S, Shiraishi Y, Kohno T, Nagatomo Y, et al. Geriatric nutritional risk index at hospital admission or discharge in patients with acute decompensated heart failure. J Clin Med. (2023) 12. doi: 10.3390/jcm12051891
53. Giezenaar C, Chapman I, Luscombe-Marsh N, Feinle-Bisset C, Horowitz M, Soenen S. Ageing is associated with decreases in appetite and energy intake—A meta-analysis in healthy adults. Nutrients. (2016) 8. doi: 10.3390/nu8010028
Keywords: heart failure, geriatric nutritional risk index (GNRI), short performance physical battery, barthel index (BI), cardiac rehabilitation (CR)
Citation: Miura M, Okuda S, Murata K, Ohno Y, Katou S, Nakao F, Ueyama T, Yamamoto T and Ikeda Y (2023) The impact of geriatric nutritional risk index on one-year outcomes in hospitalized elderly patients with heart failure. Front. Cardiovasc. Med. 10:1190548. doi: 10.3389/fcvm.2023.1190548
Received: 21 March 2023; Accepted: 16 May 2023;
Published: 30 May 2023.
Edited by:
Kenichi Hongo, Jikei University School of Medicine, JapanReviewed by:
Keisuke Kida, St. Marianna University School of Medicine, JapanTetsuro Miyazaki, Juntendo University Urayasu Hospital, Japan
© 2023 Miura, Okuda, Murata, Ohno, Katou, Nakao, Ueyama, Yamamoto and Ikeda. This is an open-access article distributed under the terms of the Creative Commons Attribution License (CC BY). The use, distribution or reproduction in other forums is permitted, provided the original author(s) and the copyright owner(s) are credited and that the original publication in this journal is cited, in accordance with accepted academic practice. No use, distribution or reproduction is permitted which does not comply with these terms.
*Correspondence: Masakazu Miura cG1wLmt0cy5uZ3UwODIwQGdtYWlsLmNvbQ== Yasuhiro Ikeda eXNpa2VkYS15bWdAdW1pbi5hYy5qcA==
†These authors have contributed equally to this work