- 1Department of Cardiology, Chengdu Integrated TCM and Western Medicine Hospital, Chengdu, Sichuan, China
- 2Clinical Medical School, Chengdu University of Traditional Chinese Medicine, Chengdu, Sichuan, China
Background: The Non-Exercise Estimated Cardiorespiratory Fitness (NEE-CRF) method has gained attention in recent years due to its simplicity and effectiveness. Hypertension and all-cause mortality are significant public health issues worldwide, highlighting the importance of exploring the association between NEE-CRF and these two conditions.
Methods: The data from the National Health and Nutrition Examination Survey (NHANES) and the China Health and Retirement Longitudinal Study (CHARLS) were utilized to validate the association between NEE-CRF and hypertension as well as all-cause mortality. NEE-CRF was calculated using a sex-specific longitudinal non-exercise equation. To investigate the relationship between hypertension and all-cause mortality, multivariable regression analysis, generalized additive models, smooth curve fittings, and threshold effect analysis were employed. Logistic regression was used for hypertension analysis, while Cox proportional hazards regression was applied for all-cause mortality. Additionally, we conducted stratified analyses and interaction tests among different groups.
Results: In the NHANES, after fully adjusting for covariates, each unit increase in NEE-CRF was associated with a 24% reduction in the risk of hypertension (OR: 0.76, 95% CI: 0.74–0.78) and a 12% reduction in the risk of all-cause mortality (HR: 0.88, 95% CI: 0.79–0.86). Subgroup analyses showed that the relationship between NEE-CRF and both hypertension and all-cause mortality remained negatively correlated across different subgroups. The negative association was also validated in the CHARLS.
Conclusions: Higher NEE-CRF levels may reduce the risk of developing hypertension and all-cause mortality.
Introduction
Cardiorespiratory fitness (CRF) is a recognized health indicator that refers to the ability of the lungs, heart, and skeletal muscles to acquire and utilize oxygen during physical activity, typically quantified by maximal oxygen consumption (VO2 max) (1). However, in clinical practice, standardized exercise tests to assess CRF are challenging for individuals with limited mobility and require specialized equipment and personnel, leading to increased costs (2). As a result, Jackson et al. proposed a method for estimating non-exercise cardiorespiratory fitness (NEE-CRF), which has gradually become a widely accepted approach for evaluating CRF in recent years (3). The predictive equation for NEE-CRF incorporates gender, age, easily obtainable physical measurements, and self-reported variables (4). The American Heart Association has noted that NEE-CRF can serve as an alternative to standardized exercise tests for CRF when exercise-based predictions are not sufficiently accurate (5). Numerous studies have explored the association between NEE-CRF and various diseases. For instance, research by Robert A. and colleagues consistently found that higher NEE-CRF is negatively correlated with metabolic risk (6). Some researchers have highlighted a strong association between higher NEE-CRF and a lower incidence of type 2 diabetes (7). Furthermore, a study demonstrated that NEE-CRF can effectively predict mortality even in the absence of actual exercise testing (8). The ability of NEE-CRF to assess metabolic diseases and mortality underscores its significant research potential for further exploration of its relationship with hypertension and all-cause mortality.
Hypertension is a common chronic disease associated with the regulation of the endocrine system, microcirculation, and large arteries (9). Hypertension often contributes to the development of various cardiovascular diseases, stroke, and heart and kidney failure (10). Globally, it is the third leading cause of death, with approximately 12.5% of the global population (one in every eight individuals) affected (11). According to reports from the World Health Organization, approximately 40% of individuals aged 25 and older have hypertension (12). Despite the rising prevalence of hypertension, the rates of control and treatment remain inadequate, placing a significant economic burden on countries (13). All-cause mortality is defined as the total number of deaths from all causes in a given population over a specified period, typically expressed as deaths per 1,000 or 100,000 individuals per year (14). For example, in 2022, cardiovascular diseases caused 19.8 million deaths worldwide (15), while cancer mortality rates have also continued to rise (16). Therefore, using accurate and easily obtainable health parameters like NEE-CRF to monitor the incidence of hypertension and all-cause mortality is of great value.
Currently, studies that simultaneously examine the relationship between NEE-CRF and both hypertension and all-cause mortality are limited. Systematic analyses that combine multiple databases are still scarce, particularly those focusing on different countries and populations. To address this research gap, our study utilized two authoritative public databases—the China Health and Retirement Longitudinal Study (CHARLS) and the US National Health and Nutrition Examination Survey (NHANES)—to systematically analyze the relationship between NEE-CRF, hypertension, and all-cause mortality in both American and Chinese populations.
Methods
Study population
This study includes participants from two databases, NHANES and CHARLS. NHANES is a nationwide cross-sectional survey conducted since the 1960s to assess the health and nutritional status of Americans. This survey employs a complex multistage sampling method to select nationally representative samples regularly, supported by the Centers for Disease Control and Prevention (CDC), with data released every 2 years. All study protocols must be approved by the National Center for Health Statistics Ethics Review Board, and informed consent is required from all participants (17).
We included data from six cycles between 2007 and 2018, totaling 59,842 individuals. First, we excluded participants younger than 20 years old (n = 25,072). Next, participants with missing data on CRF, hypertension, and death were excluded (n = 4,409). Subsequently, we excluded participants with missing demographic data (n = 10), missing lifestyle data (n = 1,289), missing biochemical data (n = 16,554), missing disease data (n = 420), and those with a weight of zero (n = 526). Finally, 11,562 eligible participants were included (Figure 1). The other part of the data comes from CHARLS. Detailed information on the CHARLS database and the screening process can be found in Supplementary Material 1.
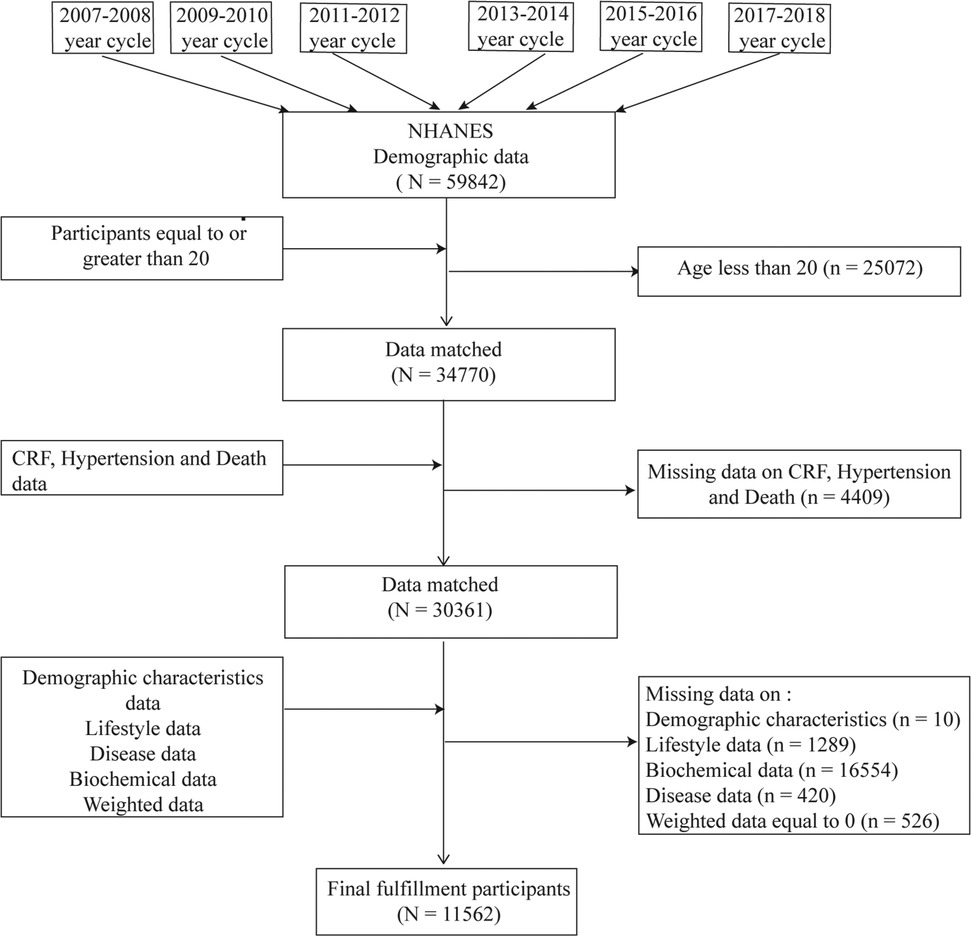
Figure 1. Flowchart of the sample selection from the 2007–2018 National Health and Nutrition Examination Survey (NHANES).
Definition of NEE-CRF
We used the gender-specific body mass index (BMI) model proposed by Jackson et al. (3) to estimate NEE-CRF in metabolic equivalents (METs). The model formulas are as follows: For males: NEE-CRF = 21.2870 + (age × 0.1654) − (age² × 0.0023) − (BMI × 0.2318) − (waist circumference × 0.0337) − (resting heart rate × 0.0390) + (active physical activity × 0.6351) − (smoker × 0.4263). For females: NEE-CRF = 14.7873 + (age × 0.1159) − (age² × 0.0017) − (BMI × 0.1534) − (waist circumference × 0.0088) − (resting heart rate × 0.0364) + (physical activity × 0.5987) − (smoker × 0.2994).
Definition of hypertension and all-cause mortality
For NHANES and CHARLS, hypertension is diagnosed based on the following criteria (18, 19): an average systolic blood pressure (SBP) of 140 mmHg or more, an average diastolic blood pressure (DBP) of 90 mmHg or more, self-reported hypertension, or the use of prescribed antihypertensive medication.
All-cause mortality in NHANES was identified using National Death Index (NDI) records up to December 31, 2019. Specific cause mortality was determined by ICD-10 codes in the same NDI records. For CHARLS's definition of all-cause mortality, refer to Supplementary Material 2.
Covariates
Based on previous studies (18), the covariates used for hypertension research utilizing the NHANES database include age (years), sex (male, female), race (mexican American, non-Hispanic Black, non-Hispanic White, other Hispanic, other Race - Including Multi-Racial), education level [Less than 9th grade/9–11th grade (Includes 12th grade with no diploma)/High school/College and Higher], marital status (married/non-married), smoking (former/never/now), physical activity (no/yes), alcohol consumption (no/yes), blood glucose (mmol/L), creatinine (µmol/L), serum uric acid (µmol/L), total cholesterol (TC, mmol/L), high-density lipoprotein cholesterol (HDL-C, mmol/L), low density lipoprotein cholesterol (LDL-C, mmol/L), triglycerides (TG, mmol/L), weight (kg), BMI (kg/m2), waist circumference (cm), diabetes (no, yes), and stroke (no, yes) (see Supplementary Material 3 for details). The covariates for all-cause mortality research with NHANES are detailed in Supplementary Material 4. For the covariates included in CHARLS, refer to Supplementary Materials 5, 6.
Statistical analyses
This study analyzed data from the NHANES database in the United States and the CHARLS database in China. For the CHARLS database, the 2011 survey data was selected as the baseline and followed up until 2020. For the NHANES database, considering sample weighting is essential. Since NHANES employs a complex, multi-stage probability sampling method to select a representative sample of participants, all our analyses incorporate sample weights to approximate national statistics. Continuous variables are presented as means and standard deviations (SD), and categorical variables are expressed as weighted percentages and counts. Firstly, we assessed group differences using Student's t-test for continuous variables and the chi-square test for categorical variables. Before performing the t-test, we verified the assumptions of normality using the Shapiro–Wilk test and assessed the homogeneity of variances using Levene's test. Secondly, we evaluated the relationship between NEE-CRF and hypertension and all-cause mortality using weighted multivariable logistic regression models and Cox proportional hazards regression models, respectively. Model 1 is the baseline model with no covariate adjustments. Model 2 adjusts for education level and marital status. Model 3 further adjusts for variables including blood glucose, creatinine, serum uric acid, TC, TG, HDL-C, LDL-C, alcohol consumption, and diabetes. Additionally, we analyzed NEE-CRF in quartiles, calculating effect sizes (OR and HR) and their 95% confidence intervals (CI). Thirdly, we identified particularly vulnerable subpopulations by examining the relationship between NEE-CRF, hypertension, and all-cause mortality in both the NHANES and CHARLS databases. For NHANES participants, subgroup analyses were conducted based on alcohol consumption (no/yes), history of stroke (no/yes), diabetes (no/yes), race (Mexican American/Other Hispanic/Other Race), and marital status (married/non-married). For CHARLS participants, subgroup analyses were conducted based on alcohol consumption (no/yes), dyslipidemia (no/yes), diabetes (no/yes), residence (rural/urban), marital status (married/non-married), and education level (elementary school or below/high school/college or above). Interaction tests were performed in both databases to explore heterogeneity across different subgroups. Fourth, we explored potential non-linear relationships between NEE-CRF and both hypertension and all-cause mortality using generalized additive models (GAM), smooth curve fittings, and threshold effect analysis. The criterion for statistical significance was set at a two-sided P-value of less than 0.05. All data analyses were performed using R software (version 4.3.2).
Results
Characteristics of the baseline population
Table 1 presents the baseline characteristics of participants stratified by hypertension status. The hypertension group includes 4,879 individuals, while the non-hypertension group comprises 6,683 individuals. The mean age of the hypertension group is 59.27 ± 14.70 years, with males accounting for 50.30% and females for 49.70%. The NEE-CRF value for this group is 7.63 ± 2.27, and the resting heart rate is 71.17 ± 12.20 bpm. In contrast, the mean age of the non-hypertension group is 42.74 ± 15.95 years, with 48.97% males and 51.03% females. The NEE-CRF value for this group is 9.38 ± 2.27, and the resting heart rate is 71.35 ± 11.06 bpm. Compared to the non-hypertension group, the hypertension group has significantly higher values for age, BMI, weight, waist circumference, TC, TG, blood glucose, serum uric acid, creatinine, as well as SBP and DBP, with these differences being statistically significant (P < 0.001). As shown in Table 2, the quartile ranges for NEE-CRF are 0.01–6.84, 6.84–8.51, 8.51–10.21, and 10.21–15.39, respectively. Additionally, we observed that participants in the fourth quartile had significantly lower age, resting heart rate, BMI, waist circumference, TC, and HDL-C compared to those with lower NEE-CRF (P < 0.001). The basic characteristics of the Chinese population are detailed in Supplementary Materials 7, 8.
Association between the NEE-CRF with hypertension and all-cause mortality
Tables 3, 4 provide a detailed overview of the relationship between NEE-CRF and hypertension as well as all-cause mortality in the American population. After adjusting for all covariates, each unit increase in NEE-CRF was associated with a 24% reduction in the risk of hypertension (OR = 0.76, 95% CI: 0.74–0.78) and a 12% reduction in mortality (HR = 0.88, 95% CI: 0.79–0.86). When divided into quartiles, the highest quartile group showed a 79% reduction in the risk of developing hypertension (OR = 0.21, 95% CI: 0.18–0.25) and a 74% reduction in mortality (HR = 0.26, 95% CI: 0.18–0.37) compared to the lowest quartile group.
In contrast, as shown in Supplementary Materials 9, 10, among the Chinese population, each unit increase in NEE-CRF is associated with a 13% reduction in the likelihood of developing hypertension (OR = 0.87, 95% CI: 0.81–0.94) and a similar 12% reduction in mortality risk (HR = 0.88, 95% CI: 0.80–0.96). Notably, when comparing the highest to the lowest quartile groups, the risk of hypertension decreased by 48% (OR = 0.52, 95% CI: 0.36–0.75), while mortality was reduced by 45% (HR = 0.55, 95% CI: 0.34–0.90).
Subgroup and interaction analyses
The results of the subgroup analyses demonstrated that NEE-CRF consistently showed a negative correlation with hypertension and all-cause mortality across different subgroups in both American and Chinese populations (Supplementary Materials 11, 12). Notably, in the American population, factors such as alcohol consumption, stroke, diabetes, and marital status significantly influenced the strength of the association between NEE-CRF and hypertension (all interaction P-values <0.05). Similarly, stroke, diabetes, and race had a significant impact on the association between NEE-CRF and all-cause mortality (all interaction P-values <0.05). However, in the Chinese population, the associations between NEE-CRF and both hypertension and all-cause mortality remained consistent across subgroups stratified by residence, alcohol consumption, diabetes, dyslipidemia, marital status, and education level (all interaction P-values >0.05).
Nonlinear relationship NEE-CRF with hypertension and all-cause mortality
The results of the GAM and smooth curve fitting shown in Figure 2 revealed a nonlinear relationship between NEE-CRF and both hypertension and all-cause mortality in the American population. The threshold analysis found that when NEE-CRF reached 6.00, the risk of hypertension hit a critical point, and when NEE-CRF reached 7.40, there was a significant change in the risk of all-cause mortality (Table 5). Specifically, when NEE-CRF was ≥7.40, each additional unit increase in NEE-CRF was associated with a 20% reduction in the risk of all-cause mortality (HR: 0.80, 95% CI 0.80–0.90). In contrast, when NEE-CRF was <7.40, changes in NEE-CRF were not significantly associated with the risk of all-cause mortality. Additionally, we observed that when NEE-CRF reached 6.00, the risk of hypertension significantly decreased (OR: 0.70, 95% CI 0.70–0.80).
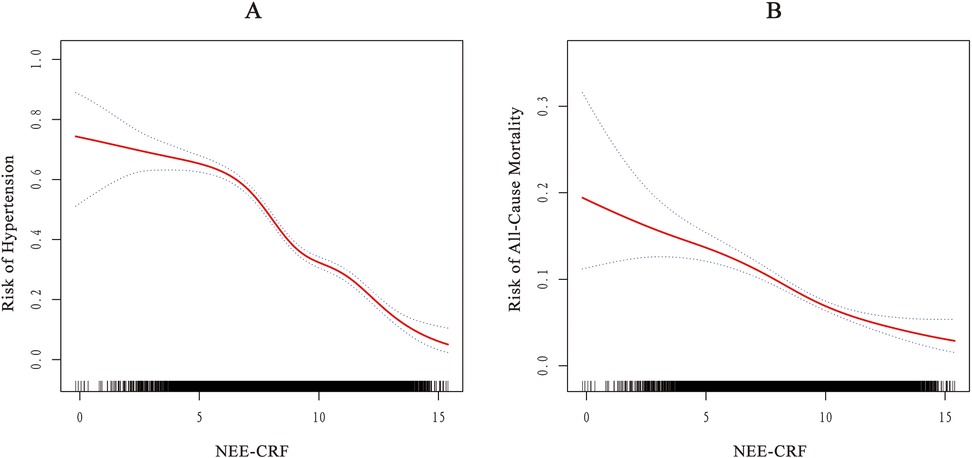
Figure 2. Smoothed curve fitting: dose-response relationship between NEE-CRF with (A) hypertension and (B) all-cause mortality.
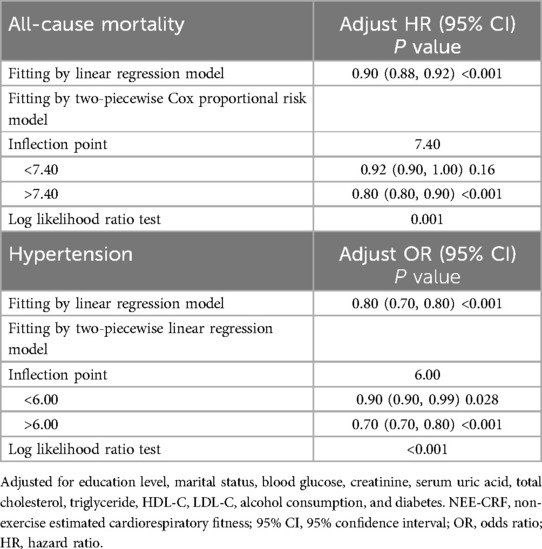
Table 5. Threshold effect analysis of NEE-CRF with hypertension and all-cause mortality using two-piecewise linear regression model in American population.
In the Chinese population, the results presented in Supplementary Material 13 also revealed a nonlinear relationship between NEE-CRF and both hypertension and all-cause mortality. Supplementary Material 14 indicated that the threshold for the association between NEE-CRF and all-cause mortality was identified as 8.74 METs. Below this threshold, NEE-CRF was significantly negatively correlated with all-cause mortality (HR: 0.66, 95% CI 0.56–0.79), whereas above the threshold, the relationship was not significant (HR: 0.96, 95% CI 0.85–1.09). Furthermore, the log-likelihood ratio test indicated that there was no statistically significant threshold in the association between NEE-CRF and hypertension (P > 0.05).
Discussion
This study, using data from the Chinese CHARLS and American NHANES databases, found that higher levels of NEE-CRF are associated with a lower risk of hypertension and all-cause mortality. These findings carry significant clinical implications. First, in resource-limited clinical settings, healthcare professionals can easily and non-invasively estimate CRF through non-exercise assessment methods, enabling earlier implementation of disease prevention and rehabilitation measures (20). This approach not only streamlines clinical procedures but also facilitates the creation of personalized health management plans for patients (21). Second, for individuals at high risk of hypertension, NEE-CRF-based data can guide healthcare professionals in promptly adjusting lifestyle recommendations, thereby effectively reducing the risk of developing the condition (21). Furthermore, improving NEE-CRF levels can help lower mortality rates from various diseases and enhance patient prognosis (22). Through continuous health management and lifestyle interventions, patients can reduce the likelihood of disease recurrence, extend their life expectancy, and improve their quality of life (22).
Previous studies have explored the relationship between NEE-CRF and both hypertension and all-cause mortality. For instance, in a rural Chinese cohort study, Zhao et al. found that patients with more than a 2% increase in NEE-CRF had a 24% lower incidence of hypertension, while those with more than a 2% decrease in NEE-CRF had a 52% higher risk (23). A follow-up study of American Caucasians indicated that both a high NEE-CRF trajectory and a high average NEE-CRF were associated with a reduced risk of hypertension (21). Similarly, Liu et al.'s study involving 4,286 elderly Chinese individuals showed that those with higher NEE-CRF exhibited better arterial pressure performance, characterized by lower systolic and diastolic blood pressure over time (24). Another study involving 330,769 American retirees demonstrated that higher NEE-CRF was independently associated with a lower risk of mortality (22). Research from England found a consistent association between NEE-CRF and both all-cause and cardiovascular mortality, with a 12%–15% reduction in all-cause mortality for every 1.6–1.7 unit increase in NEE-CRF (20). Additionally, a study on an American population reported that for each 1-MET increase in NEE-CRF, cancer mortality risk decreased by 30% in men and 28% in women (25). Since the parameters used to assess NEE-CRF are often closely related to an individual's lifestyle (e.g., diet, weight), which also significantly influence hypertension, this further underscores the connection between the two (4, 26). Lee et al.'s research pointed out that higher NEE-CRF in middle-aged Europeans was associated with lower subclinical atherosclerosis and vascular stiffness (27), both of which are high-risk factors for the development of hypertension (28). Moreover, studies have shown that good cardiorespiratory fitness implies a stronger ability to adapt to daily activities and environmental changes, thereby reducing mortality risk due to various factors (29, 30). In summary, existing literature confirms that an increase in NEE-CRF can reduce the risk of hypertension and all-cause mortality, which aligns with our study findings.
The relationship between NEE-CRF and reduced all-cause mortality is complex and multifaceted, involving a variety of mechanisms. Some key mechanisms include: Firstly, improving NEE-CRF means the heart can pump blood more efficiently, providing adequate oxygen and nutrients to various parts of the body, thereby reducing the risk of heart disease (31). It also helps lower resting blood pressure, which reduces the incidence of cardiovascular events such as heart attacks and strokes (21). Additionally, enhanced NEE-CRF helps regulate autonomic nervous function, which normalizes the excessive activation of the sympathetic nervous system, thereby lowering the risk of fatal arrhythmias (32). Improved NEE-CRF also combats atherosclerosis and positively influences cardiovascular risk factors such as lipids, blood pressure, glucose, and obesity (33). This not only enhances metabolic health but also promotes lipid metabolism, reducing the risk of diabetes, obesity, and metabolic syndrome (34). Collectively, these benefits contribute to reduced all-cause mortality. Secondly, enhancing NEE-CRF can also improve mental and cognitive health (35). Research shows that higher levels of NEE-CRF are associated with greater gray matter volume in regions of interest related to Alzheimer's disease, reduced white matter hyperintensities, and better cognitive function and emotional states (36). These improvements not only lower the risk of developing Alzheimer's disease and depression but also protect brain structure, thereby promoting overall mental health (37). Improved mental health significantly benefits physical health, ultimately helping to reduce all-cause mortality (38). Regarding hypertension, a common chronic disease, its impact on all-cause mortality involves multiple complex physiological mechanisms and pathological processes, including: Firstly, hypertension can damage vascular endothelium and lead to arteriosclerosis. Over time, arterial walls thicken and lose elasticity, restricting blood flow and increasing the workload on the heart (39). Persistent hypertension may result in left ventricular hypertrophy, potentially progressing to heart failure, which significantly increases the risk of death (40). Additionally, hypertension is a major risk factor for coronary artery disease, leading to severe cardiac events such as angina and myocardial infarction, further raising all-cause mortality (41). Secondly, hypertension increases the risk of stroke (42). Vascular damage caused by hypertension can trigger systemic inflammatory responses, impairing endothelial function and increasing the risk of thrombosis (43). These clots can travel to the brain, causing infarction (44). Long-term hypertension also impacts small cerebral vessels, leading to narrowing, blockage, or bleeding, which increases the risk of stroke (42). Finally, long-term hypertension can cause hardening and narrowing of small renal arteries, affecting the kidneys' blood supply (45). Persistent ischemia and damage to renal tubules can lead to interstitial inflammation and fibrosis, ultimately damaging renal structure and function (46). This can result in serious consequences such as uremia, further increasing all-cause mortality (46).
Our subgroup analysis and interaction results indicate that, within the American population, increases in NEE-CRF are significantly more effective in reducing the risks of hypertension and all-cause mortality in non-diabetic and non-stroke populations compared to diabetic and stroke populations. Diabetic individuals face a higher risk of hypertension due to factors such as insulin resistance, inflammatory responses, and metabolic syndrome (47). Additionally, diabetes, being a metabolic disease, is often accompanied by high insulin levels and hyperglycemia, leading to endothelial dysfunction, which increases the likelihood of hypertension and all-cause mortality (48). For stroke patients, cardiovascular health is often severely compromised post-stroke (49). Many stroke patients may have had undiagnosed hypertension or other cardiovascular diseases prior to the event (50). For instance, chronic hypertension can result in lacunar ischemic strokes due to repeated brain injuries associated with perivascular edema, leading to hyaline deposits, fibrinoid necrosis, thickening of small artery walls, luminal narrowing, and eventually thrombosis and stroke (51). Consequently, the risk of hypertension is generally higher in stroke populations.
Our study has several strengths. Firstly, the two databases we used come from two of the world's largest countries—China and the United States—which exhibit significant differences in factors such as ethnicity and lifestyle. The consistency of findings between these two countries further strengthens our conclusions. Secondly, we not only simultaneously investigated the associations of NEE-CRF with hypertension and all-cause mortality but also employed a complex, multi-stage probability sampling method in analyzing the NHANES database, ensuring that the results are broadly representative of the entire U.S. population.
On the other hand, this study has some limitations. Firstly, the follow-up period of the CHARLS study is relatively short, limiting our ability to assess the long-term impact of NEE-CRF on hypertension and all-cause mortality. Secondly, key variables in this study were based on self-reported data, which may introduce recall bias and social desirability bias. Additionally, differences between the CHARLS and NHANES study samples, including racial, cultural, and lifestyle variations, may affect the applicability of the NEE-CRF equation across diverse populations. Additionally, while our statistical methods were robust, a more detailed standardization process for variables could further enhance the comparability between datasets. Lastly, while our statistical methods were robust, the standardization of variables across datasets could have been more explicitly detailed, which might impact cross-population comparisons.
Despite these limitations, our research demonstrates that NEE-CRF is associated with a lower risk of hypertension and reduced all-cause mortality in two different populations, highlighting its potential clinical significance.
Conclusion
These findings suggest potential clinical implications, showing a negative correlation between NEE-CRF and both hypertension and all-cause mortality. Based on these results, we recommend considering the regular assessment of NEE-CRF levels in future health monitoring and research. This approach could be valuable for hypertension prevention and may also contribute to improving overall health outcomes.
Data availability statement
Publicly available datasets were analyzed in this study. This data can be found here: availability of data and materials the datasets generated and analyzed during the current study are available in the CHARLS and NHANES website, available in http://charls.pku.edu.cn/en and https://www.cdc.gov/nchs/nhanes/index.htm, respectively.
Ethics statement
The CHARLS is approved by the Biomedical Ethics Review Committee of Peking University, and all participants provide informed consent. The NHANES is approved by the National Center for Health Statistics Research Ethics Review Board, and all participants provide informed consent. The study methodology was carried out following relevant guidelines and regulations. The studies were conducted in accordance with the local legislation and institutional requirements. The participants provided their written informed consent to participate in this study. Written informed consent was obtained from the individual(s) for the publication of any potentially identifiable images or data included in this article.
Author contributions
M-YT: Conceptualization, Data curation, Formal analysis, Funding acquisition, Investigation, Methodology, Project administration, Resources, Software, Supervision, Validation, Visualization, Writing – original draft, Writing – review & editing. PZ: Conceptualization, Data curation, Formal analysis, Funding acquisition, Investigation, Methodology, Project administration, Resources, Software, Supervision, Validation, Visualization, Writing – original draft, Writing – review & editing. S-XZ: Conceptualization, Data curation, Formal analysis, Funding acquisition, Investigation, Methodology, Project administration, Resources, Software, Supervision, Validation, Visualization, Writing – original draft, Writing – review & editing. SW: Conceptualization, Data curation, Formal analysis, Funding acquisition, Investigation, Methodology, Project administration, Resources, Software, Supervision, Validation, Visualization, Writing – original draft, Writing – review & editing. MG: Conceptualization, Data curation, Formal analysis, Funding acquisition, Investigation, Methodology, Project administration, Resources, Software, Supervision, Validation, Visualization, Writing – original draft, Writing – review & editing.
Funding
The author(s) declare that no financial support was received for the research and/or publication of this article.
Conflict of interest
The authors declare that the research was conducted in the absence of any commercial or financial relationships that could be construed as a potential conflict of interest.
Publisher's note
All claims expressed in this article are solely those of the authors and do not necessarily represent those of their affiliated organizations, or those of the publisher, the editors and the reviewers. Any product that may be evaluated in this article, or claim that may be made by its manufacturer, is not guaranteed or endorsed by the publisher.
Supplementary material
The Supplementary Material for this article can be found online at: https://www.frontiersin.org/articles/10.3389/fcvm.2025.1497292/full#supplementary-material
Abbreviations
CRF, cardiorespiratory fitness; NEE-CRF, non-exercise cardiorespiratory fitness; CHARLS, China Health and Retirement Longitudinal Study; NHANES, National Health and Nutrition Examination Survey; CDC, Centers for Disease Control and Prevention; BMI, body mass index; SBP, systolic blood pressure; DBP, diastolic blood pressure; NDI, National Death Index; TC, total cholesterol; HDL-C, high-density lipoprotein cholesterol; LDL-C, low density lipoprotein cholesterol; TG, triglycerides; SD, standard deviation; CI, confidence interval; GAM, generalized additive model.
References
1. Raghuveer G, Hartz J, Lubans DR, Takken T, Wiltz JL, Mietus-Snyder M, et al. Cardiorespiratory fitness in youth: an important marker of health: a scientific statement from the American heart association. Circulation. (2020) 142(7):e101–18. doi: 10.1161/CIR.0000000000000866
2. Lamonte MJ. Cardiorespiratory fitness in the prevention and management of cardiovascular disease. Rev Cardiovasc Med. (2022) 23(11):382. doi: 10.31083/j.rcm2311382
3. Jackson AS, Sui X, O'Connor DP, Church TS, Lee DC, Artero EG, et al. Longitudinal cardiorespiratory fitness algorithms for clinical settings. Am J Prev Med. (2012) 43(5):512–9. doi: 10.1016/j.amepre.2012.06.032
4. Mailey EL, White SM, Wójcicki TR, Szabo AN, Kramer AF, Mcauley E. Construct validation of a non-exercise measure of cardiorespiratory fitness in older adults. BMC Public Health. (2010) 10(1):59. doi: 10.1186/1471-2458-10-59
5. Ross R, Blair SN, Arena R, Church TS, Després J-P, Franklin BA, et al. Importance of assessing cardiorespiratory fitness in clinical practice: a case for fitness as a clinical vital sign: a scientific statement from the American heart association. Circulation. (2016) 134(24):e653–99. doi: 10.1161/CIR.0000000000000461
6. Sloan RA. Estimated cardiorespiratory fitness and metabolic risks. Int J Environ. (2024) 21(5):635. doi: 10.3390/ijerph21050635
7. Sparks JR, Wang X, Lavie CJ, Jakicic JM, Sui X. Non-exercise estimated cardiorespiratory fitness and incident type 2 diabetes in adults. Diabetes Res Clin Pract. (2024) 214:111791. doi: 10.1016/j.diabres.2024.111791
8. Bonikowske AR, Taylor JL, Larson KF, Hardwick J, Ozemek C, Harber MP, et al. Evaluating current assessment techniques of cardiorespiratory fitness. Expert Rev Cardiovasc Ther. (2024) 22(6):231–41. doi: 10.1080/14779072.2024.2363393
9. Yannoutsos A, Levy BI, Safar ME, Slama G, Blacher J. Pathophysiology of hypertension. J Hypertens. (2014) 32(2):216–24. doi: 10.1097/HJH.0000000000000021
10. Cushman WC. The burden of uncontrolled hypertension: morbidity and mortality associated with disease progression. J Clinical Hypertens. (2003) 5(3):14–22. doi: 10.1111/j.1524-6175.2003.02464.x
11. Frieden TR, Jaffe MG. Saving 100 million lives by improving global treatment of hypertension and reducing cardiovascular disease risk factors. J Clinical Hypertens. (2018) 20(2):208–11. doi: 10.1111/jch.13195
12. Luepker RV, Steffen LM, Jacobs DR, Zhou X, Blackburn H. Trends in blood pressure and hypertension detection, treatment, and control 1980 to 2009. Circulation. (2012) 126(15):1852–7. doi: 10.1161/CIRCULATIONAHA.112.098517
13. Mills KT, Bundy JD, Kelly TN, Reed JE, Kearney PM, Reynolds K, et al. Global disparities of hypertension prevalence and control. Circulation. (2016) 134(6):441–50. doi: 10.1161/CIRCULATIONAHA.115.018912
14. Foreman KJ, Marquez N, Dolgert A, Fukutaki K, Fullman N, Mcgaughey M, et al. Forecasting life expectancy, years of life lost, and all-cause and cause-specific mortality for 250 causes of death: reference and alternative scenarios for 2016–40 for 195 countries and territories. Lancet. (2018) 392(10159):2052–90. doi: 10.1016/S0140-6736(18)31694-5
15. Mensah GA, Fuster V, Roth GA. A heart-healthy and stroke-free world. J Am Coll Cardiol. (2023) 82(25):2343–9. doi: 10.1016/j.jacc.2023.11.003
16. Torre LA, Siegel RL, Ward EM, Jemal A. Global cancer incidence and mortality rates and trends—an update. Cancer Epidem Biomar. (2016) 25(1):16–27. doi: 10.1158/1055-9965.EPI-15-0578
17. National Center for Health Statistics. NCHS ethics review board (ERB) approval. Available at: https://www.cdc.gov/nchs/nhanes/irba98.htm (Accessed August 18, 2024).
18. Tan M-Y, Mo C-Y, Zhao Q. The association between magnesium depletion score and hypertension in US adults: evidence from the national health and nutrition examination survey (2007–2018). Biol Trace Elem Res. (2023) 202(10):4418–30. doi: 10.1007/s12011-023-04034-y
19. Luo J-H, Zhang T-M, Yang L-L, Cai Y-Y, Yang Y. Association between relative muscle strength and hypertension in middle-aged and older Chinese adults. BMC Public Health. (2023) 23(1):2087. doi: 10.1186/s12889-023-17007-6
20. Stamatakis E, Hamer M, O'Donovan G, Batty GD, Kivimaki M. A non-exercise testing method for estimating cardiorespiratory fitness: associations with all-cause and cardiovascular mortality in a pooled analysis of eight population-based cohorts. Eur Heart J. (2012) 34(10):750–8. doi: 10.1093/eurheartj/ehs097
21. Patel PH, Gates M, Kokkinos P, Lavie CJ, Zhang J, Sui X. Non-exercise estimated cardiorespiratory fitness and incident hypertension. Am J Med. (2022) 135(7):906–14. doi: 10.1016/j.amjmed.2022.01.048
22. Vainshelboim B, Myers J, Matthews CE. Non-exercise estimated cardiorespiratory fitness and mortality from all-causes, cardiovascular disease, and cancer in the NIH-AARP diet and health study. Eur J Prev Cardiol. (2020) 29(4):599–607. doi: 10.1093/eurjpc/zwaa131
23. Zhao Y, Fu X, Ke Y, Wu Y, Qin P, Hu F, et al. Independent and joint associations of estimated cardiorespiratory fitness and its dynamic changes and obesity with the risk of hypertension: a prospective cohort. J Hum Hypertens. (2024) 38(5):413–9. doi: 10.1038/s41371-024-00910-9
24. Liu Y, Zhu J, Yu J, Zhang X. Cardiorespiratory fitness and metabolic risk in Chinese population: evidence from a prospective cohort study. BMC Public Health. (2024) 24(1):522. doi: 10.1186/s12889-024-17742-4
25. Wang Y, Chen S, Zhang J, Zhang Y, Ernstsen L, Lavie CJ, et al. Nonexercise estimated cardiorespiratory fitness and all-cancer mortality: the NHANES III study. Mayo Clinic Proc. (2018) 93(7):848–56. doi: 10.1016/j.mayocp.2018.01.004
26. Hermansen K. Diet, blood pressure and hypertension. Br J Nutr. (2000) 83(S1):S113–9. doi: 10.1017/S0007114500001045
27. Lee J, Song RJ, Musa Yola I, Shrout TA, Mitchell GF, Vasan RS, et al. Association of estimated cardiorespiratory fitness in midlife with cardiometabolic outcomes and mortality. JAMA Netw Open. (2021) 4(10):e2131284. doi: 10.1001/jamanetworkopen.2021.31284
28. Boutouyrie P, Chowienczyk P, Humphrey JD, Mitchell GF. Arterial stiffness and cardiovascular risk in hypertension. Circ Res. (2021) 128(7):864–86. doi: 10.1161/CIRCRESAHA.121.318061
29. Myers J, Mcauley P, Lavie CJ, Despres J-P, Arena R, Kokkinos P. Physical activity and cardiorespiratory fitness as major markers of cardiovascular risk: their independent and interwoven importance to health Status. Prog Cardiovasc Dis. (2015) 57(4):306–14. doi: 10.1016/j.pcad.2014.09.011
30. Cooper R, Kuh D, Hardy R, Mortality Review Group. Objectively measured physical capability levels and mortality: systematic review and meta-analysis. Br Med J. (2010) 341:c4467. doi: 10.1136/bmj.c4467
31. Gander JC, Sui X, Hébert JR, Lavie CJ, Hazlett LJ, Cai B, et al. Addition of estimated cardiorespiratory fitness to the clinical assessment of 10-year coronary heart disease risk in asymptomatic men. Prev Med Rep. (2017) 7:30–7. doi: 10.1016/j.pmedr.2017.05.008
32. Laukkanen JA, Kurl S, Kunutsor SK. Interplay between fitness, systolic blood pressure and sudden cardiac death (from a cohort study). Am J Cardiol. (2023) 200:124–7. doi: 10.1016/j.amjcard.2023.05.009
33. Ekblom-Bak E, Ekblom B, Vikström M, De Faire U, Hellénius M-L. The importance of non-exercise physical activity for cardiovascular health and longevity. Br J Sports Med. (2013) 48(3):233–8. doi: 10.1136/bjsports-2012-092038
34. Lee I, Kim S, Kang H. Non-exercise based estimation of cardiorespiratory fitness mediates associations between comorbidities and health-related quality of life in older Korean adults with diabetes. Int J Environ. (2020) 17(4):1164. doi: 10.3390/ijerph17041164
35. Shigdel R, Stubbs B, Sui X, Ernstsen L. Cross-sectional and longitudinal association of non-exercise estimated cardiorespiratory fitness with depression and anxiety in the general population: the HUNT study. J Affect Disord. (2019) 252:122–9. doi: 10.1016/j.jad.2019.04.016
36. Mcauley E, Szabo AN, Mailey EL, Erickson KI, Voss M, White SM, et al. Non-exercise estimated cardiorespiratory fitness: associations with brain structure, cognition, and memory complaints in older adults. Ment Health Phys Act. (2011) 4(1):5–11. doi: 10.1016/j.mhpa.2011.01.001
37. Boots EA, Schultz SA, Oh JM, Larson J, Edwards D, Cook D, et al. Cardiorespiratory fitness is associated with brain structure, cognition, and mood in a middle-aged cohort at risk for Alzheimer’s disease. Brain Imaging Behav. (2014) 9(3):639–49. doi: 10.1007/s11682-014-9325-9
38. Keyes CLM, Simoes EJ. To flourish or not: positive mental health and all-cause mortality. Am J Public Health. (2012) 102(11):2164–72. doi: 10.2105/AJPH.2012.300918
39. Frąk W, Wojtasińska A, Lisińska W, Młynarska E, Franczyk B, Rysz J. Pathophysiology of cardiovascular diseases: new insights into molecular mechanisms of atherosclerosis, arterial hypertension, and coronary artery disease. Biomedicines. (2022) 10(8):1938. doi: 10.3390/biomedicines10081938
40. Yildiz M, Oktay AA, Stewart MH, Milani RV, Ventura HO, Lavie CJ. Left ventricular hypertrophy and hypertension. Prog Cardiovasc Dis. (2020) 63(1):10–21. doi: 10.1016/j.pcad.2019.11.009
41. Shao C, Wang J, Tian J, Tang Y-D. Coronary artery disease: from mechanism to clinical practice. Adv Exp Med Biol. (2020) 1177:1–36. doi: 10.1007/978-981-15-2517-9_1
42. Murphy SJX, Werring DJ. Stroke: causes and clinical features. Medicine. (2020) 48(9):561–6. doi: 10.1016/j.mpmed.2020.06.002
43. Xu S, Ilyas I, Little PJ, Li H, Kamato D, Zheng X, et al. Endothelial dysfunction in atherosclerotic cardiovascular diseases and beyond: from mechanism to pharmacotherapies. Pharmacol Rev. (2021) 73(3):924–67. doi: 10.1124/pharmrev.120.000096
44. Di Chiara T, Del Cuore A, Daidone M, Scaglione S, Norrito RL, Puleo MG, et al. Pathogenetic mechanisms of hypertension–brain-induced complications: focus on molecular mediators. Int J Mol Sci. (2022) 23(5):2445. doi: 10.3390/ijms23052445
45. De Bhailis ÁM, Kalra PA. Hypertension and the kidneys. Br J Hosp Med. (2022) 83(5):1–11. doi: 10.12968/hmed.2021.0440
46. Panizo S, Martínez-Arias L, Alonso-Montes C, Cannata P, Martín-Carro B, Fernández-Martín JL, et al. Fibrosis in chronic kidney disease: pathogenesis and consequences. Int J Mol Sci. (2021) 22(1):408. doi: 10.3390/ijms22010408
47. Silveira Rossi JL, Barbalho SM, Reverete De Araujo R, Bechara MD, Sloan KP, Sloan LA. Metabolic syndrome and cardiovascular diseases: going beyond traditional risk factors. Diabetes Metab Res Rev. (2021) 38(3):e3502. doi: 10.1002/dmrr.3502
48. Xue C, Chen K, Gao Z, Bao T, Dong L, Zhao L, et al. Common mechanisms underlying diabetic vascular complications: focus on the interaction of metabolic disorders, immuno-inflammation, and endothelial dysfunction. Cell Commun Signal. (2023) 21(1):298. doi: 10.1186/s12964-022-01016-w
49. Sposato LA, Hilz MJ, Aspberg S, Murthy SB, Bahit MC, Hsieh C-Y, et al. Post-stroke cardiovascular complications and neurogenic cardiac injury. J Am Coll Cardiol. (2020) 76(23):2768–85. doi: 10.1016/j.jacc.2020.10.009
50. Yen F-S, Wei JC-C, Chiu L-T, Hsu C-C, Hwu C-M. Diabetes, hypertension, and cardiovascular disease development. J Transl Med. (2022) 20(1):9. doi: 10.1186/s12967-021-03217-2
Keywords: non-exercise estimated cardiorespiratory fitness, hypertension, all-cause mortality, NHANES, CHARLS
Citation: Tan M-Y, Zhang P, Zhu S-X, Wu S and Gao M (2025) The association of non-exercise estimated cardiorespiratory fitness with hypertension and all-cause mortality in American and Chinese populations: evidence from NHANES and CHARLS. Front. Cardiovasc. Med. 12:1497292. doi: 10.3389/fcvm.2025.1497292
Received: 16 September 2024; Accepted: 31 March 2025;
Published: 15 April 2025.
Edited by:
Giuseppe Caminiti, Università telematica San Raffaele, ItalyReviewed by:
Maysa Alves Rodrigues Brandao Rangel, Federal University of São Paulo, BrazilEwerton Amorim, Universidade Estadual de Ciências da Saúde de Alagoas, Brazil
Copyright: © 2025 Tan, Zhang, Zhu, Wu and Gao. This is an open-access article distributed under the terms of the Creative Commons Attribution License (CC BY). The use, distribution or reproduction in other forums is permitted, provided the original author(s) and the copyright owner(s) are credited and that the original publication in this journal is cited, in accordance with accepted academic practice. No use, distribution or reproduction is permitted which does not comply with these terms.
*Correspondence: Ming Gao, MTgyMjQ0MDkzNTVAMTYzLmNvbQ==