- Centre for Endocrinology, William Harvey Research Institute, Barts and The London School of Medicine and Dentistry, Queen Mary University of London, London, UK
Adrenal insufficiency is a rare, but potentially fatal medical condition. In children, the cause is most commonly congenital and in recent years a growing number of causative gene mutations have been identified resulting in a myriad of syndromes that share adrenal insufficiency as one of the main characteristics. The evolution of adrenal insufficiency is dependent on the variant and the particular gene affected, meaning that rapid and accurate diagnosis is imperative for effective treatment of the patient. Common practice is for candidate genes to be sequenced individually, which is a time-consuming process and complicated by overlapping clinical phenotypes. However, with the availability, and increasing cost effectiveness of whole-exome sequencing, there is the potential for this to become a powerful diagnostic tool. Here, we report the results of whole-exome sequencing of 43 patients referred to us with a diagnosis of familial glucocorticoid deficiency (FGD) who were mutation negative for MC2R, MRAP, and STAR the most commonly mutated genes in FGD. WES provided a rapid genetic diagnosis in 17/43 sequenced patients, for the remaining 60% the gene defect may be within intronic/regulatory regions not covered by WES or may be in gene(s) representing novel etiologies. The diagnosis of isolated or familial glucocorticoid deficiency was only confirmed in 3 of the 17 patients, other genetic diagnoses were adrenal hypo- and hyperplasia, Triple A, and autoimmune polyendocrinopathy syndrome type I, emphasizing both the difficulty of phenotypically distinguishing between disorders of PAI and the utility of WES as a tool to achieve this.
Introduction
The hypothalamic–pituitary–adrenal (HPA) axis is essential in the physiological response to stress and illness. Such external stimuli trigger the production of ACTH from corticotroph cells in the anterior pituitary, under the control of hypothalamic CRH and AVP, which acts on the ACTH receptor [also known as MC2R] on the surface of adrenal cells to elicit the production of glucocorticoids (1). The adrenal gland is composed of a medulla which secretes catecholamines and a cortex which comprises three layers: the zona glomerulosa which produces mineralocorticoids, the zona fasciculata which produces glucocorticoids (cortisol in humans), and the zona reticularis which produces adrenal androgens. Adrenal insufficiency is a serious medical condition that is invariably fatal unless diagnosed and treated early. Adrenal insufficiency is classified into primary, direct impairment of the adrenal gland to secrete cortisol, or secondary, impaired ACTH secretion from the pituitary gland. Primary adrenal insufficiency (PAI) can be acquired or congenital, the acquired group including infectious, traumatic, or drug-induced causes. Congenital PAI encompasses disorders such as congenital adrenal hyperplasia (CAH) (2–4), familial glucocorticoid deficiency (FGD) (5), and autoimmune polyglandular syndrome type I (APS-1) (6) as well as many others (listed in Table 1).
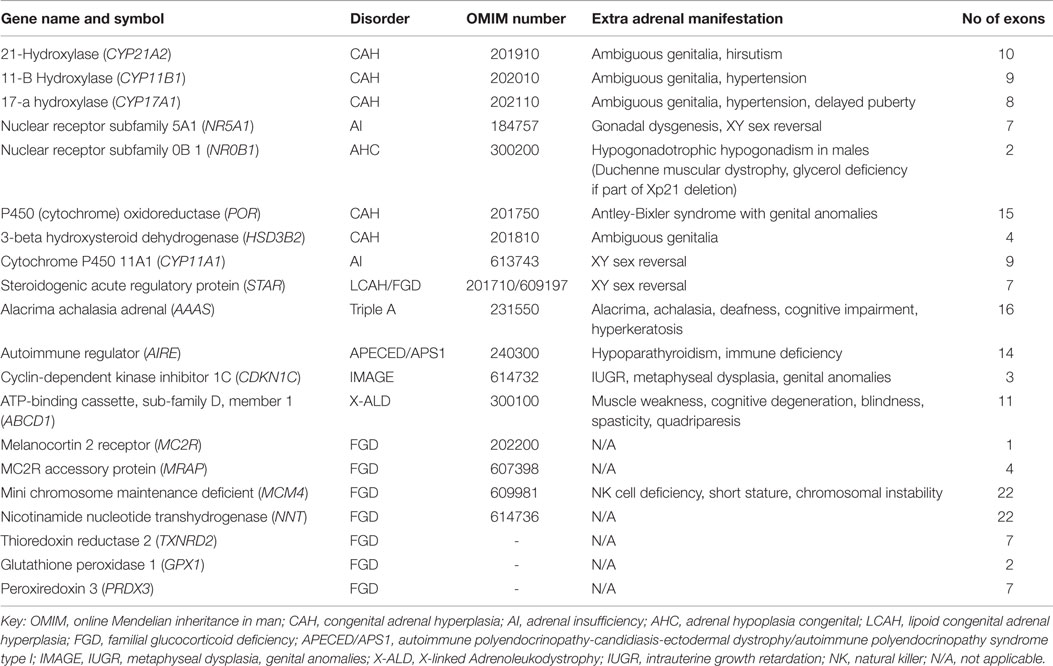
Table 1. List of disorders and gene mutations identified with primary adrenal insufficiency (PAI) as a core characteristic.
Children with PAI present with signs and symptoms resulting from low serum cortisol (such as failure to thrive, hypoglycemia, and lethargy) and from high plasma ACTH levels (hyperpigmentation – which is variable and can be dependent on ethnic origin/MC1R status). Biochemical analysis in cases of PAI will often reveal a low morning serum cortisol concentration (<80 nmol/l) paired with elevated plasma ACTH levels (>45 pmol/l), due to lack of negative feedback (7, 8). Such biochemical indicators change depending on the clinical scenario, for example, cortisol measurements maybe within normal range but inappropriately low for the given situation, for example during sepsis (9). Measurement of urea and electrolytes, plasma renin activity or concentration, and aldosterone concentration will determine the presence of mineralocorticoid deficiency. An ACTH stimulation test is often undertaken in cases of suspected adrenal insufficiency, where a synthetic form of ACTH, (ACTH [1–24], Synacthen), is administered and plasma cortisol measured 30 and 60 min later. PAI is excluded if serum cortisol concentrations are above 500 nmol/l following 250 μg of Synacthen administered IV or IM (10).
Many of the genetic disorders listed in Table 1 may present with PAI. Some of these conditions will have additional features and investigations will reveal abnormal findings, for example, adrenal cortex auto-antibodies or perturbed levels of 17-hydroxyprogesterone and very-long chain fatty acids to name a few [reviewed in Ref. (8)]. However, with recent discoveries of new genes and novel mutations in known genes causing PAI, it is becoming increasingly clear that considerable phenotypic overlap between genetic disorders occurs. This is applicable to the condition FGD, once thought to be fairly distinct from other causes of PAI (see Figure 1). FGD patients present with isolated glucocorticoid deficiency and normal mineralocorticoid production. Biochemical results in FGD point toward ACTH resistance, with serum cortisol often undetectable and extremely high plasma ACTH (11). As we learn more about the genetic causes of FGD, the boundaries between different PAI disorders or diagnostic features of FGD are being challenged (12–20).
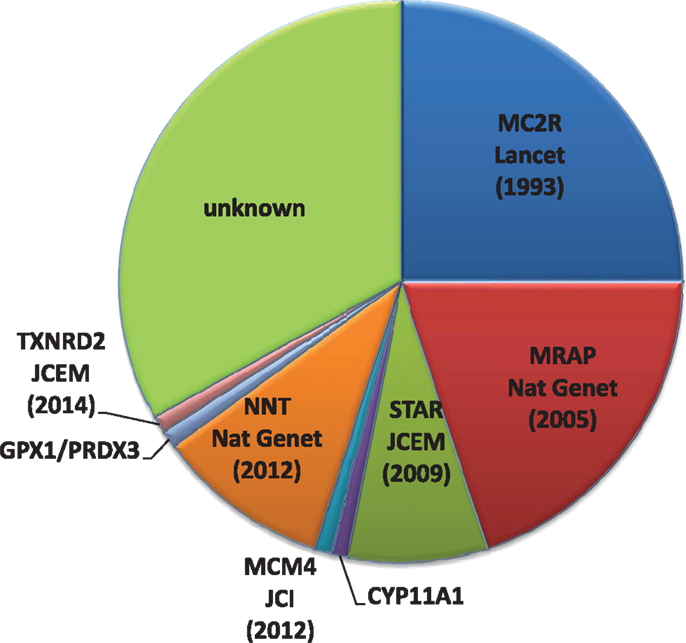
Figure 1. Pie chart indicating the percentage of FGD cases due to gene mutations in the Melanocortin 2 receptor (MC2R), MC2R accessory protein (MRAP), Mini chromosome maintenance deficient 4 (MCM4), Nicotinamide nucleotide transhydrogenase (NNT), Steroidogenic acute regulatory protein (STAR), Cytochrome p450 11A1 (CYP11A1), Thioredoxin reductase 2 (TXNRD2), Glutathione peroxidase 1 (GPX1), and Peroxiredoxin 3 (PRDX3) in our patient cohort.
Similar to many other endocrine disorders, obtaining an accurate diagnosis and understanding of the underlying pathology is essential for the management and treatment of the patient. Obtaining a genetic diagnosis will also enable prenatal screening, which in the case of CAH may inform or remove the need for antenatal treatment (21). Furthermore, a genetic diagnosis may directly alter therapeutic management of the patient removing the need for unnecessary medications lifelong (12).
In children, CAH is the commonest cause of PAI, causing 71.8% of cases in one report (22). In the cohort studied by Perry et al., this was followed by autoimmune causes (12.7%), adrenoleukodystrophy (3.9%), syndromic causes (4.9%), X-linked AHC (1%), and several were unexplained (5.8%). In this study, five international groups were involved in the mutational analysis of the various genes implicated in PAI (22).
Exome sequencing provides a robust technique by which coding variants can be identified and matched with their associated disease and complex phenotype (23). Exome sequencing was first used to identify the rare genetic mutation implicated in Miller Syndrome (23) and has been successfully used where linkage analysis has proven inadequate due to the rarity of the disease being investigated, the sporadic nature of the disease being investigated, or uninformative pedigrees. In addition, the MLL2 mutation was identified using exome sequencing as a cause of Kabuki syndrome, a rare genetic disorder. The causative genetic variant leading to Kabuki syndrome had proved difficult to determine due to the rarity of the disease, there only being approximately 400 cases worldwide (24). Further success in disease gene identification through WES includes the identification of the missense variant in SLC26A3 which results in congenital chloride diarrhea (25). In addition to using exome sequencing to identify a link between variants and disease, it has potential to be used as a diagnostic tool. In the case of congenital chloride diarrhea, exome sequencing identified the SLC26A3 variant, and future diagnosis of this condition could be achieved through exome sequencing (25). Even in cases demonstrating identification of disease causing variants using whole-genome sequencing, as in the case of hypercholesterolemia, these could often have been identified by exome sequencing (26). Many more examples, illustrating the emerging importance of exome sequencing, are summarized by Gilissen et al. (27). In PAI, similar to many other disorders, WES is often cheaper than the sequencing of all known candidate genes. Furthermore, given the lower cost associated with exome sequencing relative to whole-genome sequencing, WES sequencing may provide a robust and cost-effective screening and diagnostic tool for rare genetic conditions.
We have a cohort of >300 patients with FGD, 60% of them have a genetic diagnosis. We have previously used targeted and WES to identify novel causes of FGD in this collection (17, 18, 28). WES of a subset of our unsolved cases allowed for the screening of variants known to cause FGD as well as the possibility of discovering novel causative genes for PAI. Using PAI as an example, this paper offers a valuable commentary on the use of WES as a diagnostic tool in cases where the number of causative genes makes targeted gene sequencing more expensive than WES.
Subject and Methods
Sequencing of Candidate Genes in Familial Glucocorticoid Deficiency
Patient genomic DNA was extracted from blood leukocytes. PCR was used to amplify regions of candidate genes implicated in FGD. All patients were screened for mutations in Melanocortin-2-receptor (MC2R), Melanocortin-2-receptor accessory protein (MRAP), and steroidogenic acute regulatory protein (STAR), the most commonly mutated genes in FGD, accounting for 50% of cases (Figure 1). Most patients underwent additional screening for mutations in nicotinamide nucleotide transhydrogenase (NNT), a recently discovered FGD causal gene (primer sequences available on request). After initial denaturation of template DNA at 95oC for 5 min, a touchdown thermal cycling program was used; 10 cycles of 95oC for 30 s, 65oC for the first cycle, this was then decreased by 1oC for each subsequent cycle for 10 cycles, and then 72oC for 1 min. This was then followed by 25 cycles of 95oC for 30 s, 55oC for 30 s and 72oC for 1 min. After the last cycle, there is an extension step at 72oC for 5 min. PCR products were sequenced using ABI Prism Big Dye Sequencing kit and an ABI 377 automated DNA sequencer (Applied Biosystems) as described in the manufacturer’s instructions.
Informed consent was obtained from affected individuals and/or their parents. Ethical approval for the study was obtained from the Outer North East London Research Ethics Committee, reference number 09/H0701/12.
Exome Sequencing
Whole-exome sequencing (WES) was performed on 43 patients with a diagnosis of FGD who were negative on screening for candidate genes (described above). This included many ‘cold cases’ referred to us as isolated glucocorticoid deficiency. In addition, one sibling (of patient 9) was sequenced for the causative gene and family members underwent Sanger sequencing of candidate variants to determine segregation.
Exome sequencing was performed using the Agilent SureSelect all exon V4 capture and paired-end (2 × 100) sequencing on an Illumina HiSeq 2000 at Otogenetics (Norcross, GA). First analysis pipeline: initially sequencing read alignment, variant calling, and annotation were performed by DNAnexus (DNAnexus Inc.1, Mountain View, CA, USA), their Nucleotide-Level Variation analysis outputs were then screened with our list of genes via the DNAnexus Classic platform which permitted variants table viewing and filtering functionality. This platform no longer exists and more recently these data were reanalyzed uploading the vcf files to Ingenuity variant analysis2. Second analysis pipeline: the raw data were also reanalyzed, aligning to the H. Sapiens GRCh37–b37 (1000genomes Phase 1) reference genome with BWA-MEM FastQ Readmapper VCF files, generated by Vendor Human Exome GATK-Lite Variant Caller (Unified Genotyper) and uploaded to Ingenuity variant analysis. Single Nucleotide Polymorphisms, with threshold coverage of at least 10 reads on the respective nucleotide, were included in the analysis. The variant files from both analyses were screened for causal variants using Ingenuity variant analysis with the filtering strategy outlined in Figure 2. Sequence changes in PAI causal genes were confirmed by PCR designed to cover the affected region followed by Sanger sequencing (primer sequences available on request).
Results
Mean target coverage across the exomes varied from 52.34 – 62.52X and > 90% of target bases were covered 10X. For both analysis pipelines, similar numbers of variants were called from 43 exomes combined, after confidence filtration the first analysis pipeline produced a total of 393,015 variants in 20,327 genes with many variants being common between samples whereas the second had 393,262 variants in 20,249 genes. 400 and 358 variants within our 20 PAI genes (Table 1) were called by first and second pipelines, respectively, and after further filtration (Figure 2) the following results were obtained. To be as inclusive as possible, data from the first analysis pipeline are detailed below except where stated (Tables 2 and 3).
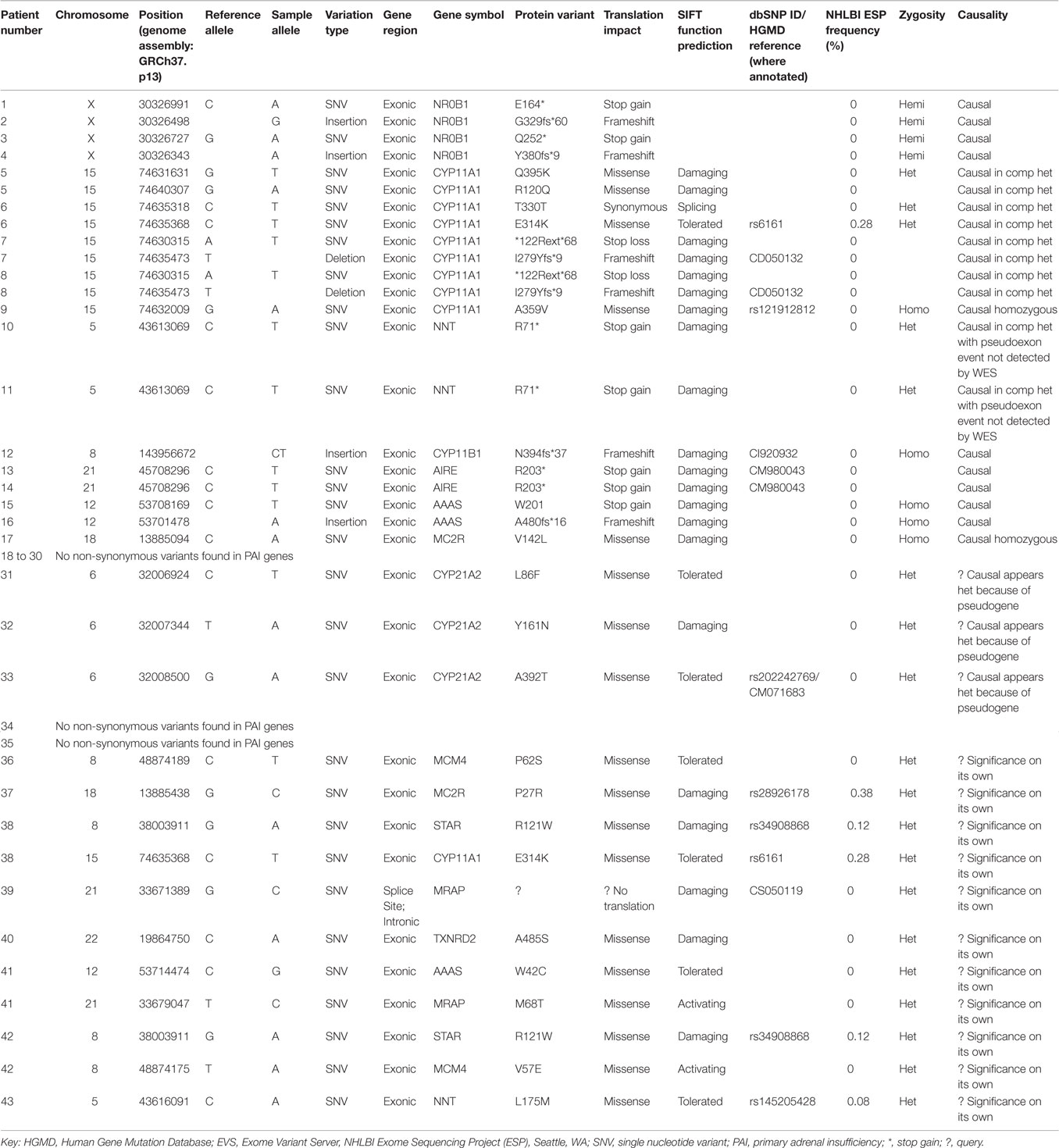
Table 2. Non-synonymous variants detected after Ingenuity filtration screening of the 20 PAI causal genes listed in Table 1.
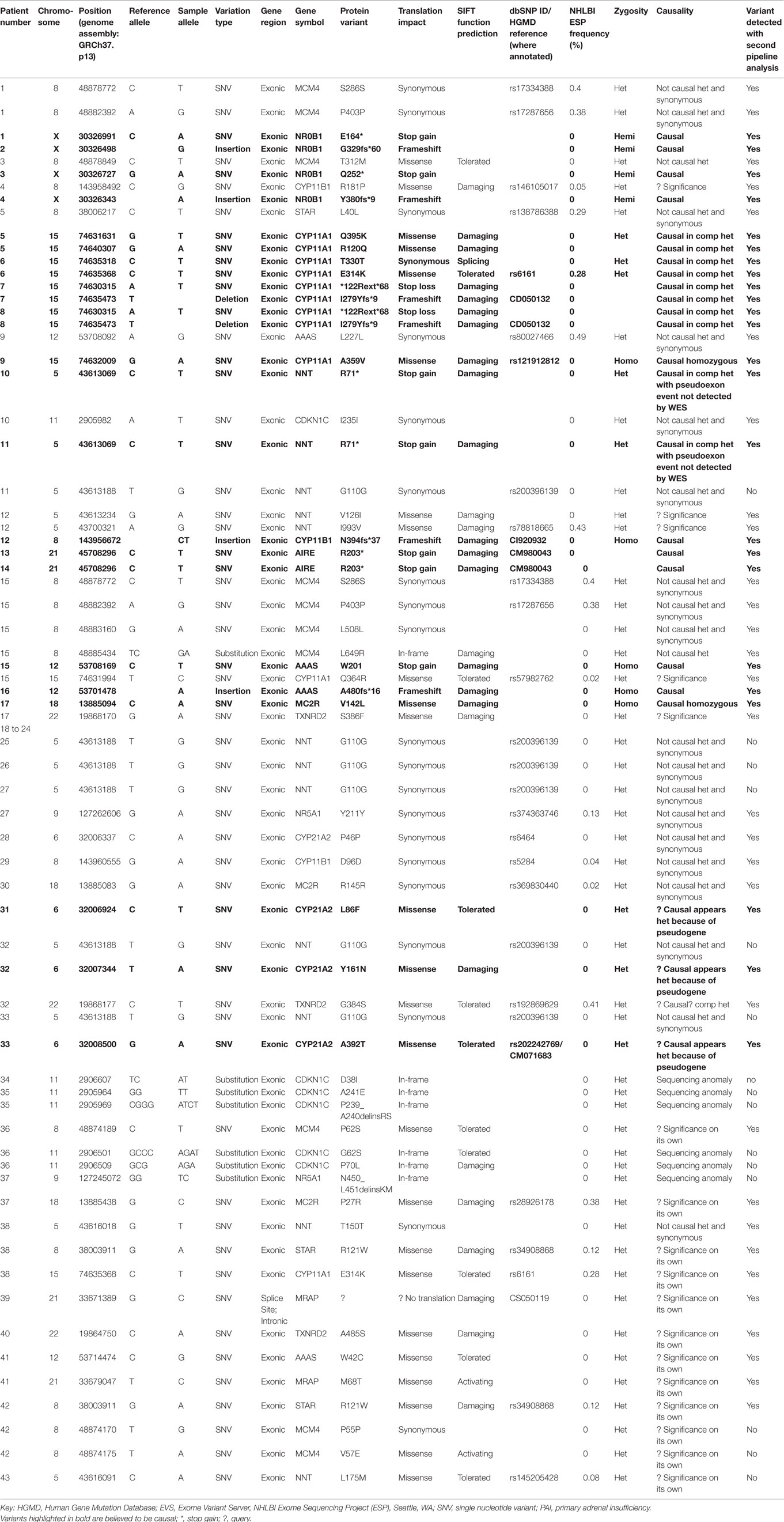
Table 3. Variants, including synonymous changes, detected after Ingenuity filtration screening of the 20 PAI causal genes listed in Table 1.
Positive Genetic Diagnosis
After Ingenuity filtration screening (Figure 2) of the 20 PAI causal genes listed in Table 1, 51 variants in 12 genes remained (Table 2), with no variants in ABCD1, CDKN1C, CYP17A1, GPX1, HSD3B2, NR5A1, POR, or PRDX3 being detected. When rare synonymous variants were included, to encompass silent changes that altered splicing (as in patient 6 below), 69 variants were discovered (Table 3) with no variants in ABCD1, CYP17A1, GPX1, HSD3B2, POR, or PRDX3 being detected.
We made a genetic diagnosis in 17 patients plus one affected sibling (of patient 9) who did not undergo WES, identifying mutations in the following genes: NR0B1 (four patients), CYP11A1 (five patients + one sib), AAAS (two patients), MC2R (one patient), CYP11B1 (one patient), AIRE (two patients), and NNT (two siblings) (patients 1–17 in Table 2). Where possible, segregation of the variant(s) with the disease was confirmed by direct Sanger sequencing of the index case and family members.
Patients 1–4, all males, were hemizygous for novel mutations in NR0B1, these were stop gain or frameshift mutations and therefore likely to be causal. Patients 5–8 had compound heterozygous variants in CYP11A1 that segregated with the disease; patient 5 was compound heterozygous for two novel missense mutations; and patient 6 was compound heterozygous for E314K (MAF 0.001%) and a silent T330T variant at the end of exon 5 of CYP11A1 which we showed, by cDNA analysis, resulted in aberrant splicing. Siblings 7 and 8 were compound heterozygous for I279Yfs*9, previously reported in lipoid congenital adrenal hyperplasia [LCAH; Hiort et al. (29); (29)] and a novel, stop loss mutation *122Rext*68. Patient 9 and a sibling had a homozygous mutation, A359V in CYP11A1 which has previously been seen in LCAH with XY sex reversal (30).
In siblings 10 and 11, we detected a heterozygous stop gain mutation, R71* in NNT, no other variants were detected by either WES or Sanger sequencing of the other NNT exons; however, the presence of a stop mutation on one allele in both siblings prompted further investigations including cDNA analysis which uncovered a pseudoexon inclusion event on the other allele. A pseudoexon is a potential exon within intronic regions of pre-mRNA that is not normally spliced into mature mRNA, it contains sequences similar to 5′ and 3′ splice-site consensus sequences, but is not normally recognized by the cellular splicing machinery. In this case the pseudoexon inclusion results in a frameshift and the introduction of a premature stop codon (31). Patient 12 had a homozygous frameshift mutation in CYP11B1, previously described in CAH (32), but also bore novel variants in NNT (see below). Siblings 13 and 14 were homozygous for a novel, stop gain mutation in AIRE, the gene responsible for APECED/APS1. Patients 15 and 16 had homozygous stop gain and frameshift mutations, respectively, in AAAS, causal for Triple A syndrome. Finally, patient 17 was homozygous for a previously described MC2R mutation (33), which had been missed on conventional sequencing. In summary, for patients 1–17 variants in PAI causal genes were detected which were likely to be responsible for their disease.
Incidental Mutations in Solved Cases
Several of the above patients, with a presumed genetic diagnosis, bore heterozygous changes in other PAI genes (Table 2); patient 3 carried p.T312M in MCM4, patient 4 carried p.R181P in CYP11B1, patient 12 had three variants, p.L361D in CYP17A1, and p.V126I and p.I993V in NNT, patient 15 had two, p.Q364R in CYP11A1 and p.L649R in MCM4, and patient 17 had two, p.H24_Y25delinsQI in NR5A1 and p.S386F in TXNRD2. The contribution of these variants to the burden of their disease is uncertain.
No Genetic Diagnosis
By contrast, a genetic diagnosis was not confirmed in patients 18–43. For patients 18–24, no variants were detected in the genes screened; patients 25–30 only possessed heterozygous, synonymous variants in the 16 genes screened. Patients 31–33 had heterozygous changes in CYP21A2 (or its pseudogene/CYP21A1P) consistent with gene conversion events but needing further investigations to confirm this. Patients 34–43 had heterozygous, missense or frameshift variants in genes known to cause PAI, but in each case only a single allele was affected. The frameshift variants found in CDKN1C and NR5A1 in patients 34–37 proved to be sequencing anomalies and were excluded by the second analysis pipeline, whereas the missense variants in MCM4, MC2R, NNT, STAR, CYP11A1, MRAP, TXNRD2, and AAAS seen in patients 37–43 were confirmed by Sanger sequencing. The G110G and L175M NNT variants, the P55P and V57E MCM4 variants, the N450_L451delinsKM in NR5A1, and all CDKN1C variants were missed by the second analysis (Table 3 last column). A low allele fraction for the variant nucleotide accounted for the error in calling variants found in NNT and MCM4, but for the changes in NR5A1 and CDKN1C the sequence changes were not confirmed by Sanger sequencing and on close inspection in Integrative Genomics Viewer (IGV, Broad Institute3) were due to misalignment of sequencing reads within repetitive sequences. Sanger sequencing of all exons of the affected gene was carried out in each case for patients 37–43 to determine whether a causal variant could be discovered on the other allele but without success. The inheritance pattern for these genes is autosomal recessive and the causative mutations are loss of function, this would therefore suggest that either these are incidental mutations not contributing to disease, or there are regulatory region or intronic variants on the other allele in each of these cases, similar to the scenario in NNT for patients 10 and 11. This requires mRNA/cDNA analysis for which samples are unavailable at this time.
Discussion
Exome sequencing has been used to successfully identify novel variants in diseases causing adrenal insufficiency. In the case of FGD, 50% of cases are attributable to mutations in one of three genes: MC2R, MRAP, and STAR, which were linked to the disorder by traditional linkage and sequencing methods, but with the advent of next generation sequencing technologies, three further genes have been discovered and more causative genes may exist (Figure 1) (14, 17, 18, 28). Targeted exome sequencing was used to identify the first variants in NNT and subsequently 21 variants were identified as causal in FGD (18). In addition, exome sequencing was also used to identify the c.71-1insG variant in the MCM4 gene which results in the syndrome of adrenal failure, short stature, and natural killer (NK) cell deficiency in Irish Travelers (17) and the p.Y447* variant in TXNRD2 responsible for FGD in a large Kashmiri kindred (28). WES provided a rapid genetic diagnosis in 17/43 sequenced ‘FGD’ patients, in most cases identifying an alternative genetic cause of their PAI (14/17). For the remaining 60%, the gene defect may be within intronic/regulatory regions not covered by WES or may be in gene(s) representing novel etiologies.
In the case of suspected PAI, there are many candidate genes implicated (Table 1). Exome sequencing offers a rapid, cheap, and convenient sequencing technique as the exons of a large repertoire of candidate genes can be screened in one sequencing run. The findings in this cohort highlight the complex nature of adrenal disease and the range of differential diagnoses that may arise. It is critical to determine the underlying genetic cause in adrenal insufficiency since this may change on-going treatment and management. Patients with partial loss-of-function mutations in STAR or CYP11A1 presenting an adrenal-only phenotype may have associated fertility problems (14), such patients require close monitoring as they may have a window of opportunity for fertility that is lost over time. Early genetic counseling should be offered to families and discussion about options for preservation of fertility is required. Furthermore, the identification of NROB1 mutations in some of our ‘FGD’ cohort would allow clinicians to recognize and manage future issues saving their patients the anguish of later unexplained infertility.
Furthermore, adopting exome sequencing as a standard screening tool would allow for the identification of variants which may have later onset as is the case with the MCM4 variant leading to mild cortisol deficiency in childhood which declines with age after a period of normal adrenal function (17) or TXNRD2 variants which may have an extremely variable age of onset (28). Such an approach also increases the probability of new gene causality being discovered with the accrual of patients without mutations in known PAI genes.
There are, however, limitations to exome sequencing as a screening tool for known disease gene variants. The exome is thought to represent approximately 1% of the human genome, thus leaving a large section of the DNA unchecked (34). Although the exome is thought to account for 85% of disease causing mutations (25) the true incidence is unknown because of the difficulty of sequencing and characterizing intronic variants. Our results, both the discovery of a pseudoexon inclusion event and patients with single heterozygous changes, suggest the possibility that this may occur more frequently than previously recognized (31). For patients 10 and 11, the finding of a stop gain mutation in NNT in both siblings led us to invest the time to investigate the other allele, this detected a pseudoexon inclusion event deep within intron 20 (1129 bp from exon 20), a region not covered by WES. This might have been missed if it had not been coupled with a known deleterious mutation on the other allele or if it had been homozygous. Similarly, compound heterozygous intronic mutations would be missed by WES. Whole-genome sequencing would disclose such cases but the consequence of such changes can be hard to predict. Most significantly, disease causing variants which lie in the non-coding promoter and intronic sequence such as is the case with apoE4 implicated in Alzheimer’s disease are also not detected by exome sequencing (35, 36). In addition, micro RNA genes which often silence expression of protein encoding genes are often found within introns. The percentage of disease causing mutations in exonic sequences may decrease with the application of whole-genome sequencing in patients and the improvement of tools for consequence prediction.
Even when dealing with an exonic variant the prediction of its pathogenicity is not trivial and this is exacerbated when the variant is outside coding sequences. Many computational methods have been developed to address this but concordance between the results generated by such programs is poor (37). Therefore, for optimal assessment of a variants effect more than one prediction program should be utilized and, where possible, functional studies should be undertaken. Similarly, multiple software tools for alignment and variant calling are available, and each can generate different results, here again it is recommended that more than one algorithm be employed when analyzing exome data (38, 39). Particularly low concordance rates have been reported for INDELs detected by different pipelines and sequencing platforms (40, 41) stressing the need to carry out multiple analyses on data sets. Depth of coverage is also a consideration, at a mean on-target read depth of 20X, commonly achieved in rare disease exome sequencing, it is predicted that 5–15% of heterozygous and 1–4% of homozygous single nucleotide variants (SNVs) in the targeted regions will be missed (42). Sulonen et al. showed that a minimum of 11X coverage was required to make a heterozygote genotype call with 99% accuracy, whereas homozygous calls can be accurate at much lower coverage (43).
Our exome data, ordered at a depth of 30X for economy, has many exons which are not covered 30X. For new gene discovery, this depth of coverage may be sufficient but it is clearly not ideal for diagnosis where a more appropriate depth might be 100X. One way to increase depth of coverage is to use a custom enrichment panel to go forward for next-generation sequencing. Many companies are now introducing clinical exome panels designed to capture the exons of disease causing genes, e.g., OneSeq and SureSelectXT Clinical Research Exome (Agilent) which may concomitantly detect genome-wide CNVs, copy-neutral LOH (cnLOH), SNPs, and indels in one target enrichment capture.
Additional disadvantages of WES include the fact that it cannot detect epigenetic changes, or mutations that do not alter the sequence, for instance, translocations, inversions, and repeats such as in Huntington’s disease. Consequently, exome sequencing cannot easily be used to assess gene copy number dependent disease susceptibility, as non-sequence alteration changes are not recognized. However, algorithms to address this have been and are being developed (44–46) and this is important as variations in copy number can affect the severity or susceptibility to a disease. Furthermore, silent changes, which may not affect amino acid sequence but alter splicing either directly or by alteration of splice enhancer/repressor sites, may be ignored as they are excluded from analysis by common filtration strategies. Difficulties also arise in WES in distinguishing between genes with extremely similar nucleotide sequences, where a gene possesses a paralog or pseudogene(s) with very similar sequence. For PAI, there are at least two such complications; to differentiate between CYP21A2 and its pseudogene CYP21A1P and distinguishing between the paralogs CYP11B1 and CYP11B2. This is usually achieved by PCR amplification strategies using primers spanning the distinguishing nucleotides, which are further apart than is convenient for standard PCR. Long-range PCR using allele-specific primers has been adopted by several groups to address this followed by sequencing of smaller sub-fragments (47). Currently such analyses will need to be applied in addition to WES. This report highlights the potential for exome sequencing to be used as a first pass diagnostic tool for conditions of adrenal insufficiency. However, there are ethical considerations and data protection issues which must be considered before using exome sequencing as a standard diagnostic tool (48). Exome sequencing for adrenal disease may lead to the identification of a variant implicated in a disease unrelated to that which the initial screening aimed to address. This leads to the question of whether the patient should be informed of variants which may have disease associations outside of the initial frame of consideration. It may seem appropriate that a patient should be notified if there is a strong monogenic link, but often diseases are polygenic, loosely associated, or linked only with susceptibility such as the case with the BRCA1 genes (49). Furthermore, not all diseases that have a characterized genetic cause have a treatment. It is important to consider whether it is appropriate or desirable for the patient to know about a disease for which there is no treatment. Any decision to notify of a genetic condition, whether treatment is available or not, would have to involve genetic counseling, and this would have to be an essential component of exome sequencing as a diagnostic tool. The American College of Medical Genetics (ACMG) guidelines suggest that laboratories performing diagnostic exome sequencing should report alterations in genes from among a provided minimum list of genes. This list includes 57 genes associated with 25 disorders which were chosen based on availability of preventative measures and treatment options (50, 51).
This highlights one of the abiding difficulties for WES diagnosis, if a novel variant is found in a disease causing gene how do we predict its consequence? In order to say it is causal for disease, we need to show the variant is loss- or gain-of-function which requires time-consuming and labor-intensive experiments, to check the (dys)function of the putative mutation. Such additional functional analyses will slow down the diagnostic process until such time as variant/protein prediction tools improve.
In summary, diagnostic WES provides rapid, cheap, discriminatory sequencing of all exonic sequences of known causal genes in one hit, thereby removing the necessity for a single, candidate gene, Sanger sequencing approach. However, WES does not cover intronic sequences which may harbor deleterious mutations, it does not allow easy detection of CNV, transversions, translocations, gene conversions (such as that frequently seen in CYP21A2 in CAH), or gene fusions (seen between CYP11B1/B2) and it cannot predict the functional consequence of novel variant(s). In our hands WES provided a rapid, putative genetic diagnosis in 40% of our patients, in most cases the defect was not in a known FGD gene thereby highlighting both the difficulty of phenotypically distinguishing between disorders of PAI and the utility of WES as a tool to achieve this.
Take home message: Despite the limitations, exome sequencing still provides a powerful tool in the diagnosis of single gene and increasingly multigene disorders (25, 27). This report highlights the potential for WES to be a diagnostic aid in the clinical setting with the understanding that it cannot currently replace other genetic testing. In the case of PAI, and probably many other conditions, it would prove a useful first pass screen for rapid genetic diagnosis. Combined with copy number analysis, autoimmunity screening and specific screening where pseudogenes/paralogs exist, WES provides a useful tool in the diagnostic arsenal for endocrine clinicians. It is likely that WES will be adopted for the diagnosis of other endocrine disorders, such as growth hormone insensitivity and Kallmann’s syndrome, whereby the number of genes and/or the oligogenic nature of the inheritance pattern make WES a cheaper alternative than single gene sequencing.
Conflict of Interest Statement
The authors declare that the research was conducted in the absence of any commercial or financial relationships that could be construed as a potential conflict of interest.
Acknowledgments
We would like to acknowledge the referring clinicians, the patients, and their families without whom this study could not have been performed. This work has been supported by the Medical Research Council UK Project Grant MR/K020455/1, LM and MRC/Academy of Medical Sciences Clinician Scientist Fellowship Grant G0802796, LC.
Web Resources
dbSNP, www.ncbi.nlm.nih.gov/SNP/
DNAnexus, https://dnanexus.com/
Exome Variant Server, NHLBI Exome Sequencing Project (ESP), Seattle, WA, http://evs.gs.washington.edu/EVS/[accessed November 2014].
Online Mendelian Inheritance in Man (OMIM), http://www.omim.org/
The Human Gene Mutation Database: http://www.hgmd.cf.ac.uk/ac/index.php
Ingenuity variant analysis: http://www.ingenuity.com/
Otogenetics: http://www.otogenetics.com/
GATK-Lite Variant Caller (Unified Genotyper): https://www.broadinstitute.org/gatk/gatkdocs/org_broadinstitute_gatk_tools_walkers_genotyper_UnifiedGenotyper.php
IGV: https://www.broadinstitute.org/igv/
Abbreviations
AHC, adrenal hypoplasia congenital; AI, adrenal insufficiency; APECED/APS1, autoimmune polyendocrinopathy-candidiasis-ectodermal dystrophy/autoimmune polyendocrinopathy syndrome type I; CAH, congenital adrenal hypoplasia; FGD, familial glucocorticoid deficiency; HGMD, The Human Gene Mutation Database; IMAGE, IUGR, metaphyseal dysplasia, genital anomalies; IUGR, intrauterine growth retardation; IVA, Ingenuity variant analysis; LCAH, lipoid congenital adrenal hypoplasia; MAF, minor allele frequency; N/A, not applicable; NK, natural killer; OMIM, online Mendelian inheritance in man; PAI, primary adrenal insufficiency; X-ALD, X-linked adrenoleukodystrophy.
Footnotes
References
1. Clark AJ, Metherell LA. Mechanisms of disease: the adrenocorticotropin receptor and disease. Nat Clin Pract Endocrinol Metab (2006) 2:282–90. doi: 10.1038/ncpendmet0165
2. Speiser PW, Dupont J, Zhu D, Serrat J, Buegeleisen M, Tusie-Luna MT, et al. Disease expression and molecular genotype in congenital adrenal hyperplasia due to 21-hydroxylase deficiency. J Clin Invest (1992) 90:584–95. doi:10.1172/JCI115897
3. Joehrer K, Geley S, Strasser-Wozak EM, Azziz R, Wollmann HA, Schmitt K, et al. CYP11B1 mutations causing non-classic adrenal hyperplasia due to 11 beta-hydroxylase deficiency. Hum Mol Genet (1997) 6:1829–34. doi:10.1093/hmg/6.11.1829
4. Ben Charfeddine I, Riepe FG, Kahloul N, Kulle AE, Adala L, Mamaï O, et al. Two novel CYP11B1 mutations in congenital adrenal hyperplasia due to steroid 11β hydroxylase deficiency in a Tunisian family. Gen Comp Endocrinol (2012) 175:514–8. doi:10.1016/j.ygcen.2011.12.017
5. Clark AJ, Mcloughlin L, Grossman A. Familial glucocorticoid deficiency associated with point mutation in the adrenocorticotropin receptor. Lancet (1993) 341:461–2. doi:10.1016/0140-6736(93)90208-X
6. Wang CY, Shi JD, Davoodi-Semiromi A, She JX. Cloning of Aire, the mouse homologue of the autoimmune regulator (AIRE) gene responsible for autoimmune polyglandular syndrome type 1 (ASP1). Genomics (1999) 55:322–6. doi:10.1006/geno.1998.5656
7. Arlt W, Allolio B. Adrenal insufficiency. Lancet (2003) 361:1881–93. doi:10.1016/S0140-6736(03)13492-7
8. Charmandari E, Nicolaides NC, Chrousos GP. Adrenal insufficiency. Lancet (2014) 383:2152–67. doi:10.1016/S0140-6736(13)61684-0
9. Cooper MS, Stewart PM. Corticosteroid insufficiency in acutely ill patients. N Engl J Med (2003) 348:727–34. doi:10.1056/NEJMra020529
10. Husebye ES, Allolio B, Arlt W, Badenhoop K, Bensing S, Betterle C, et al. Consensus statement on the diagnosis, treatment and follow-up of patients with primary adrenal insufficiency. J Intern Med (2014) 275:104–15. doi:10.1111/joim.12162
11. Meimaridou E, Hughes CR, Kowalczyk J, Chan LF, Clark AJ, Metherell LA. ACTH resistance: genes and mechanisms. Endocr Dev (2013) 24:57–66. doi:10.1159/000342504
12. Lin L, Hindmarsh PC, Metherell LA, Alzyoud M, Al-Ali M, Brain CE, et al. Severe loss-of-function mutations in the adrenocorticotropin receptor (ACTHR, MC2R) can be found in patients diagnosed with salt-losing adrenal hypoplasia. Clin Endocrinol (2007) 66:205–10. doi:10.1111/j.1365-2265.2006.02709.x
13. Chan LF, Metherell LA, Krude H, Ball C, O’riordan SM, Costigan C, et al. Homozygous nonsense and frameshift mutations of the ACTH receptor in children with familial glucocorticoid deficiency (FGD) are not associated with long-term mineralocorticoid deficiency. Clin Endocrinol (2009) 71:171–5. doi:10.1111/j.1365-2265.2008.03511.x
14. Metherell LA, Naville D, Halaby G, Begeot M, Huebner A, Nurnberg G, et al. Nonclassic lipoid congenital adrenal hyperplasia masquerading as familial glucocorticoid deficiency. J Clin Endocrinol Metab (2009) 94:3865–71. doi:10.1210/jc.2009-0467
15. Hughes CR, Chung TT, Habeb AM, Kelestimur F, Clark AJ, Metherell LA. Missense mutations in the melanocortin 2 receptor accessory protein that lead to late onset familial glucocorticoid deficiency type 2. J Clin Endocrinol Metab (2010) 95:3497–501. doi:10.1210/jc.2009-2731
16. El-Khairi R, Achermann JC. Steroidogenic factor-1 and human disease. Semin Reprod Med (2012) 30:374–81. doi:10.1055/s-0032-1324720
17. Hughes CR, Guasti L, Meimaridou E, Chuang CH, Schimenti JC, King PJ, et al. MCM4 mutation causes adrenal failure, short stature, and natural killer cell deficiency in humans. J Clin Invest (2012) 122:814–20. doi:10.1172/JCI60224
18. Meimaridou E, Kowalczyk J, Guasti L, Hughes CR, Wagner F, Frommolt P, et al. Mutations in NNT encoding nicotinamide nucleotide transhydrogenase cause familial glucocorticoid deficiency. Nat Genet (2012) 44:740–2. doi:10.1038/ng.2299
19. Miller WL. P450 oxidoreductase deficiency: a disorder of steroidogenesis with multiple clinical manifestations. Sci Signal (2012) 5:t11. doi:10.1126/scisignal.2003318
20. Tee MK, Abramsohn M, Loewenthal N, Harris M, Siwach S, Kaplinsky A, et al. Varied clinical presentations of seven patients with mutations in CYP11A1 encoding the cholesterol side-chain cleavage enzyme, P450scc. J Clin Endocrinol Metab (2013) 98:713–20. doi:10.1210/jc.2012-2828
21. Merce Fernandez-Balsells M, Muthusamy K, Smushkin G, Lampropulos JF, Elamin MB, Abu Elnour NO, et al. Prenatal dexamethasone use for the prevention of virilization in pregnancies at risk for classical congenital adrenal hyperplasia because of 21-hydroxylase (CYP21A2) deficiency: a systematic review and meta-analyses. Clin Endocrinol (2010) 73:436–44. doi:10.1111/j.1365-2265.2010.03826.x
22. Perry R, Kecha O, Paquette J, Huot C, Van Vliet G, Deal C. Primary adrenal insufficiency in children: twenty years experience at the Sainte-Justine hospital, Montreal. J Clin Endocrinol Metab (2005) 90:3243–50. doi:10.1210/jc.2004-0016
23. Ng SB, Buckingham KJ, Lee C, Bigham AW, Tabor HK, Dent KM, et al. Exome sequencing identifies the cause of a mendelian disorder. Nat Genet (2010) 42:30–5. doi:10.1038/ng.646
24. Ng SB, Bigham AW, Buckingham KJ, Hannibal MC, Mcmillin MJ, Gildersleeve HI, et al. Exome sequencing identifies MLL2 mutations as a cause of Kabuki syndrome. Nat Genet (2010) 42:790–3. doi:10.1038/ng.646
25. Choi M, Scholl UI, Ji W, Liu T, Tikhonova IR, Zumbo P, et al. Genetic diagnosis by whole exome capture and massively parallel DNA sequencing. Proc Natl Acad Sci U S A (2009) 106:19096–101. doi:10.1073/pnas.0910672106
26. Rios J, Stein E, Shendure J, Hobbs HH, Cohen JC. Identification by whole-genome resequencing of gene defect responsible for severe hypercholesterolemia. Hum Mol Genet (2010) 19:4313–8. doi:10.1093/hmg/ddq352
27. Gilissen C, Hoischen A, Brunner HG, Veltman JA. Unlocking Mendelian disease using exome sequencing. Genome Biol (2011) 12:228. doi:10.1186/gb-2011-12-9-228
28. Prasad R, Chan LF, Hughes CR, Kaski JP, Kowalczyk JC, Savage MO, et al. Thioredoxin reductase 2 (TXNRD2) mutation associated with familial glucocorticoid deficiency (FGD). J Clin Endocrinol Metab (2014) 99:E1556–63. doi:10.1210/jc.2013-3844
29. Hiort O, Holterhus PM, Werner R, Marschke C, Hoppe U, Partsch CJ, et al. Homozygous disruption of P450 side-chain cleavage (CYP11A1) is associated with prematurity, complete 46,XY sex reversal, and severe adrenal failure. J Clin Endocrinol Metab (2005) 90:538–41. doi:10.1210/jc.2004-1059
30. al Kandari H, Katsumata N, Alexander S, Rasoul MA. Homozygous mutation of P450 side-chain cleavage enzyme gene (CYP11A1) in 46, XY patient with adrenal insufficiency, complete sex reversal, and agenesis of corpus callosum. J Clin Endocrinol Metab (2006) 91:2821–6. doi:10.1210/jc.2005-2230
31. Novoselova TV, Rath SR, Carpenter K, Pachter N, Dickinson JE, Price G, et al. NNT pseudoexon activation as a novel mechanism for disease in two siblings with familial glucocorticoid deficiency. J Clin Endocrinol Metab (2015) 100:E350–4. doi:10.1210/jc.2014-3641
32. Helmberg A, Ausserer B, Kofler R. Frame shift by insertion of 2 basepairs in codon 394 of CYP11B1 causes congenital adrenal hyperplasia due to steroid 11 beta-hydroxylase deficiency. J Clin Endocrinol Metab (1992) 75:1278–81. doi:10.1210/jcem.75.5.1430088
33. Penhoat A, Naville D, El Mourabit H, Buronfosse A, Berberoglu M, Ocal G, et al. Functional relationships between three novel homozygous mutations in the ACTH receptor gene and familial glucocorticoid deficiency. J Mol Med (2002) 80:406–11. doi:10.1007/s00109-002-0333-7
34. Ng SB, Turner EH, Robertson PD, Flygare SD, Bigham AW, Lee C, et al. Targeted capture and massively parallel sequencing of 12 human exomes. Nature (2009) 461:272–6. doi:10.1038/nature08250
35. Mui S, Briggs M, Chung H, Wallace RB, Gomez-Isla T, Rebeck GW, et al. A newly identified polymorphism in the apolipoprotein E enhancer gene region is associated with Alzheimer’s disease and strongly with the epsilon 4 allele. Neurology (1996) 47:196–201. doi:10.1212/WNL.47.1.196
36. Lambert JC, Pasquier F, Cottel D, Frigard B, Amouyel P, Chartier-Harlin MC. A new polymorphism in the APOE promoter associated with risk of developing Alzheimer’s disease. Hum Mol Genet (1998) 7:533–40. doi:10.1093/hmg/7.3.533
37. Thusberg J, Olatubosun A, Vihinen M. Performance of mutation pathogenicity prediction methods on missense variants. Hum Mutat (2011) 32:358–68. doi:10.1002/humu.21445
38. O’Rawe J, Jiang T, Sun G, Wu Y, Wang W, Hu J, et al. Low concordance of multiple variant-calling pipelines: practical implications for exome and genome sequencing. Genome Med (2013) 5(3):28. doi:10.1186/gm432
39. Altmann A, Weber P, Bader D, Preuss M, Binder EB, Müller-Myhsok B. A beginners guide to SNP calling from high-throughput DNA-sequencing data. Hum Genet (2012) 131:1541–54. doi:10.1007/s00439-012-1213-z
40. Fang H, Wu Y, Narzisi G, O’Rawe JA, Barrón LT, Rosenbaum J, et al. Reducing INDEL calling errors in whole genome and exome sequencing data. Genome Med (2014) 6:89. doi:10.1186/s13073-014-0089-z
41. Lam HY, Clark MJ, Chen R, Chen R, Natsoulis G, O’Huallachain M, et al. Performance comparison of whole-genome sequencing platforms. Nat Biotechnol (2012) 30:78–82. doi:10.1038/nbt.2065
42. Meynert AM, Bicknell LS, Hurles ME, Jackson AP, Taylor MS. Quantifying single nucleotide variant detection sensitivity in exome sequencing. BMC Bioinformatics (2013) 14:195. doi:10.1186/1471-2105-14-195
43. Sulonen AM, Ellonen P, Almusa H, Lepistö M, Eldfors S, Hannula S, et al. Comparison of solution-based exome capture methods for next generation sequencing. Genome Biol (2011) 12:R94. doi:10.1186/gb-2011-12-9-r94
44. Li J, Lupat R, Amarasinghe KC, Thompson ER, Doyle MA, Ryland GL, et al. CONTRA: copy number analysis for targeted resequencing. Bioinformatics (2012) 28:1307–13. doi:10.1093/bioinformatics/bts146
45. Fromer M, Purcell SM. Using XHMM software to detect copy number variation in whole-exome sequencing data. Curr Protoc Hum Genet (2014) 81:7.23.1–7.23.21. doi:10.1002/0471142905.hg0723s81
46. Wang W, Wang W, Sun W, Crowley JJ, Szatkiewicz JP. Allele-specific copy-number discovery from whole-genome and whole-exome sequencing. Nucleic Acids Res (2015). doi:10.1093/nar/gkv319
47. Lee HH. Mutational analysis of CYP21A2 gene and CYP21A1P pseudogene: long-range PCR on genomic DNA. Methods Mol Biol (2014) 1167:275–87. doi:10.1007/978-1-4939-0835-6_19
48. Robertson JA. The $1000 genome: ethical and legal issues in whole genome sequencing of individuals. Am J Bioeth (2003) 3:W–IF1. doi:10.1162/152651603322874762
49. Miki Y, Swensen J, Shattuck-Eidens D, Futreal PA, Harshman K, Tavtigian S, et al. A strong candidate for the breast and ovarian cancer susceptibility gene BRCA1. Science (1994) 266:66–71. doi:10.1126/science.7545954
50. Jarvik GP, Amendola LM, Berg JS, Brothers K, Clayton EW, Chung W, et al. Return of genomic results to research participants: the floor, the ceiling, and the choices in between. Am J Hum Genet (2014) 94:818–26. doi:10.1016/j.ajhg.2014.04.009
Keywords: adrenal insufficiency, whole-exome sequencing, FGD, genetics
Citation: Chan LF, Campbell DC, Novoselova TV, Clark AJL and Metherell LA (2015) Whole-exome sequencing in the differential diagnosis of primary adrenal insufficiency in children. Front. Endocrinol. 6:113. doi: 10.3389/fendo.2015.00113
Received: 25 March 2015; Accepted: 10 July 2015;
Published: 05 August 2015
Edited by:
Nils P. Krone, University of Birmingham, UKCopyright: © 2015 Chan, Campbell, Novoselova, Clark and Metherell. This is an open-access article distributed under the terms of the Creative Commons Attribution License (CC BY). The use, distribution or reproduction in other forums is permitted, provided the original author(s) or licensor are credited and that the original publication in this journal is cited, in accordance with accepted academic practice. No use, distribution or reproduction is permitted which does not comply with these terms.
*Correspondence: Louise A. Metherell, Centre for Endocrinology, William Harvey Research Institute, Barts and The London School of Medicine and Dentistry, Queen Mary University of London, Charterhouse Square, London EC1M 6BQ, UK,bC5hLm1ldGhlcmVsbEBxbXVsLmFjLnVr