- 1Section of Cell Biology and Functional Genomics, Faculty of Medicine, Imperial College London, London, United Kingdom
- 2Metabolic Medicine, Department of Metabolism, Digestion and Reproduction, Faculty of Medicine, Imperial College London, London, United Kingdom
The inheritance of variants that lead to coding changes in, or the mis-expression of, genes critical to pancreatic beta cell function can lead to alterations in insulin secretion and increase the risk of both type 1 and type 2 diabetes. Recently developed clustered regularly interspaced short palindromic repeats (CRISPR/Cas9) gene editing tools provide a powerful means of understanding the impact of identified variants on cell function, growth, and survival and might ultimately provide a means, most likely after the transplantation of genetically “corrected” cells, of treating the disease. Here, we review some of the disease-associated genes and variants whose roles have been probed up to now. Next, we survey recent exciting developments in CRISPR/Cas9 technology and their possible exploitation for β cell functional genomics. Finally, we will provide a perspective as to how CRISPR/Cas9 technology may find clinical application in patients with diabetes.
Introduction
Type 2 diabetes (T2D) affects an estimated 425 million people worldwide, a number predicted to rise to 629 million by 2045 (1). The disease usually involves insulin resistance but is ultimately the result of pancreatic β cell failure, a sine qua non for disease development (2). In contrast, Type 1 diabetes (T1D) affects a smaller proportion of people with diabetes and is chiefly the result of pancreatic β cell destruction mediated by immune cells (3).
Both genetic susceptibility and environmental drivers, notably obesity and sedentary lifestyles, determine the overall risk of T2D (4–6). Supporting a genetic component, rare monogenic forms of the disease exist with Mendelian inheritance (7, 8). Thus, maturity onset of diabetes of the young (MODY) is a rare form of diabetes with mutations often residing in exons encoding the functional domains of transcription factors such as hepatocyte nuclear factor hepatocyte nuclear factor 1 homeobox A (HNF1A) (9) and HNF4A (10), or of proteins involved in β cell glucose metabolism such as glucokinase (GCK) (11) (Table 1).
In most cases, however, T2D is a complex polygenic trait and the search for disease-associated variants has been underway for more than three decades. Genome-wide association studies (GWAS) (6, 26–31) have now identified >500 loci in the human genome which alter T2D risk. The majority of the identified variants affect insulin secretion from pancreatic β cells, rather than insulin action (29). Similar to other complex diseases (32), identified genetic variants confer relatively small increments in risk for T2D and explain only a small proportion of heritability (6). Such “missing heritability” (32) raises many questions, including whether a person’s susceptibility to disease may depend more on the combined effect of all the variants in the “background” than on the disease variants in the “foreground”. In any case, the impact of the risk variants may depend on genetic context (including modifier genes). This situation is further complicated by disease heterogeneity, with four sub-classes of T2D recently being defined by categorical K-means clustering (33) [but see (34) for an alternative description of heterogeneity]. We note that the above interactions complicate the assessment of risk heritability at the population level, such that an overestimate cannot be ruled out.
GWAS indicates that multiple genes and pathways are likely to be involved in disease development, consistent with the very large number of variants now associated with disease risk. Indeed, interactions between tissues may mean that effects, for example on insulin secretion, may, in fact, reside not at the level of the pancreatic β cell, but rather (at least in part) in other tissues from which regulatory molecules are released, for example adipokines secreted by fat cells, which then go on to influence β cell function (35). Nevertheless, as an initial step, it is reasonable to focus on the identified variants, and the likeliest site of action (the pancreatic β cell in the case of T2D) with the goal of elucidating their impacts at the molecular and cellular level, and consequently on disease pathogenesis. More sophisticated studies, exploring inter-organ communication, for example through cell-type selective inactivation in the “non-canonical” tissue in animal models (35), or in extra-pancreatic cells types, may nonetheless be warranted to achieve an in-depth understanding of the full spectrum of actions of a given variant. Clearly, though, the long list of gene variants and of disease-relevant issues make the number of testable combinations huge and, in our view, it will be important to design experiments carefully to test targeted hypotheses.
The identified genetic variants can be divided into two categories: those in protein-coding regions (fewer than 10%) and those (> 90%) in non-coding (intergenic and intronic) regions (36, 37). Variants in protein-coding regions create changes in amino acid sequence and, as a result, may impair protein function or stability. Protein truncation and loss of binding capacity to DNA or an interaction domain with other proteins are commonly observed in transcription factors (38). These variants are therefore obvious targets for mechanistic studies, are potentially targets for drug therapy, and have been the subject of several recent studies (5, 6). We note that rare coding variants of genes at loci hosting common variants appear to contribute only ~ 25% of overall T2D risk (39).
How do intragenic or intergenic variants alter cellular function or viability, and hence impact pathogenesis? Epigenetic studies of human islets have yielded a huge amount of information on where gene regulatory elements are located on chromosomes. For example, transposase-accessible chromatin sequencing (ATAC-seq) analysis (40) identifies open chromatin regions whilst Chromatin immunoprecipitation sequencing (CHIP-seq) assays on histones and transcription factors identifies histone modifications associated with active transcription or with transcriptional repression. These approaches have identified enhancer regions and enhancer clusters characterized by the acetylation of histone H3 lysine 27 (H3K27ac) (41, 42). Enhancer clusters, also termed stretch enhancers (41) or super-enhancers (43, 44), are enriched for gene regulatory elements and can be highly islet specific. Combined with GWAS data it has been possible to show that T2D variants are enriched in islet-specific enhancer clusters (41, 42).
Importantly, studies of the 3D structure of chromatin using chromatin conformation capture (3C) and 3C-based techniques (45, 46) can reveal the physical relationship between an enhancer cluster and its target genes (47, 48). Enhancer clusters often regulate multiple genes through loop formation (48). Causal variants therefore usually reside in the active enhancer region, from where they may influence enhancer activity and thus the expression of multiple target genes.
There are several challenges in not only interpreting the information above but ultimately translating this into benefit for those living with T2D. Firstly, how can we determine which of the multiple variants that are often found at a given locus, and may be co-inherited (i.e. in strong linkage disequilibrium), is responsible for altering disease risk? Secondly, through which downstream gene(s) do these act? Thirdly, how do changes in the expression of these genes affect cellular physiology? Which cell types and systems are involved? Whilst pancreatic β cells are the most likely to be affected where insulin secretion is changed, actions of variants in other tissues, which are either sensitive to insulin or may lie “upstream” of β cells in regulatory circuits that control insulin output (e.g. entero-endocrine cells or neurones) (35), may also be involved.
Genome editing tools that target the desired genomic region and allow for variants to be altered (e.g. from risk to protective), or for more substantial changes to be made (e.g. the deletion of a longer stretch of DNA harbouring a number of variants) and can help to answer each of these questions. These technologies are evolving rapidly (Figure 1 and Table 2). The most recently developed of these, Clustered Regularly Interspaced Short Palindromic Repeat (CRISPR) technology, originally developed by Doudna, Charpentier and their colleagues (72, 73) and Zhang and his colleagues (50) has become a widely used tool for this purpose. Engineered CRISPR/Cas9 technology uses a guide RNA (gRNA) to direct CRISPR-associated endonuclease (Cas) to the target DNA and generate a double strand DNA break. Correction of a mutation or variant in the target DNA sequence can then be carried out by homology-directed DNA repair (HDR) with a donor template. Since its discovery eight years ago, CRISPR technology has evolved quickly to be a critical part of the molecular biologist’s toolbox.
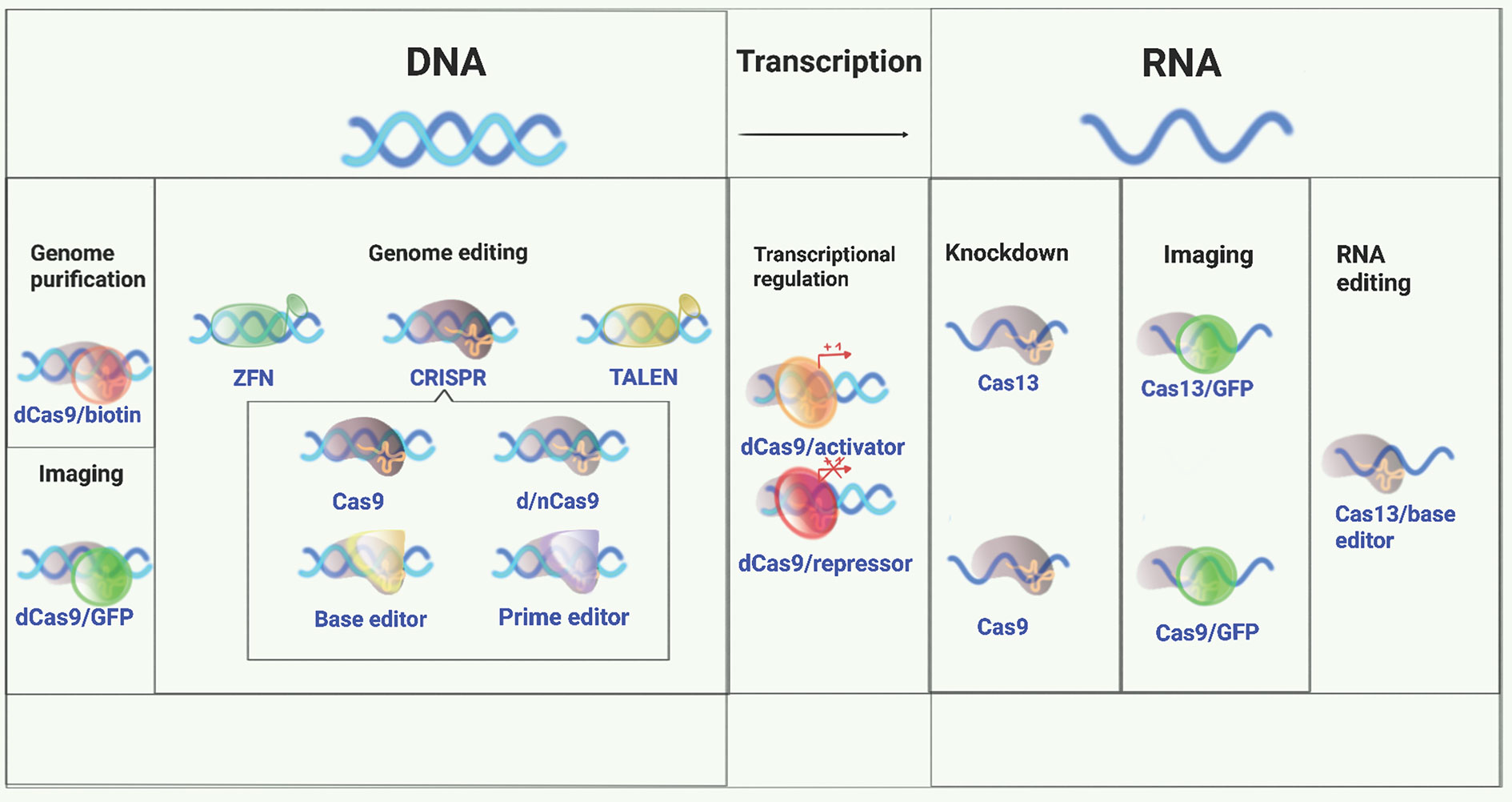
Figure 1 A versatile genome-editing toolbox. Following the original demonstration of genome editing, applications of ZFN, TALEN, and CRISPR for genome editing, regulation, monitoring, and beyond were subsequently developed (49). Conceptually, there are three major application tracks: 1. genome editing, including gene knockout, knockin, and indel formation initiated with a double strand DNA break made by a nuclease; 2. gene regulation and delivery of various functional moieties (e.g., transcription factors) to unique sites in DNA using catalytically inactivated derivatives of the same nucleases; 3. targeting single strand RNA for inactivation, editing, modification, or localization.
Below, we review recent developments in the identification of genetic variants and the elucidation of possible molecular mechanisms underlying the functional defects observed in insulin secretion. We begin by providing examples of genes and loci associated with altered T2D risk. Finally, we review the CRISPR tools that may offer the potential to correct these variants in the human β cell.
Variants Associated With Type 2 Diabetes
MODY Genes
MODY is a clinically heterogeneous group of monogenic disorders characterized by β cell dysfunction and, in several cases, effects on other disease-relevant tissues including the kidney (74). Symptoms typically appear in adolescence or young adulthood (75, 76). MODY gene mutations are characterised by autosomal dominant inheritance and high penetrance. A total of 15 genes have been described to date, all involved in β cell function (Table 1). Mutations in the glucose-phosphorylating enzyme glucokinase (GCK), and the transcription factors HNF1A and HNF4A cause up to 80% of all MODY cases (74, 76, 77).
GCK
Glucokinase converts glucose into glucose-6-phosphate the flux generating step of glycolysis in the β cell and key control point for insulin secretion (78). Heterozygous loss-of-function mutations in the GCK gene induces a decrease of glucose phosphorylation into glucose-6-phosphate (G6P), which blocks the entry of G6P into the glycolytic pathway. Insulin secretion in response to glucose is reduced and this mechanism results in non-progressive fasting hyperglycaemia in patients (79–81).
HNF1A and HNF4A
Mutations in these two genes cause a progressive insulin secretory defect and hyperglycaemia (82–84). People with these forms of MODY respond well to low-dose sulphonylurea treatment, and sometimes insulin in later life (74). HNF4A and HNF1A regulate more than a dozen genes in human islets. Mutations in HNF4A cause downregulation of GLUT-2 (SLC2A2), aldolase B, and liver-type pyruvate kinase (L-PK) in β cells which lead to defective glucose sensing in pancreatic β cells (85). Additionally, HNF1A and HNF4A regulate each other, hence defects in either factor impacts the expression of the other (86). Heterozygous mutations in HNF1A downregulate the genes involved in glucose metabolism, including both SLC2A2 and PKLR (87, 88). Ablation of Hnf1a in mice induces a reduction of β cell mass and β cell proliferation which together lead to a reduction of islet size (88). While ablation of Hnf4a in mice is embryonic lethal (89), expression of a dominant-negative form of HNF1A reduced the expression of genes involved in glycolytic and mitochondrial metabolism in these cells (90).
The other MODY gene variants associated with β cell dysfunction are rarer and the impacts more discrete (74, 91). Each type of mutation involves a specific type of MODY classified on the base of phenotypes, treatments, the extra-pancreatic features, the severity of hyperglycaemia and subsequently complications and prognosis (Table 1) (8, 91).
GWAS-Identified Variants in Protein-Coding Regions
GWAS-identified variants associated with T2D risk include single nucleotide polymorphisms (SNP), deletions, insertions and short sequence repeats (6, 92). Although the majority of the variants reside in intergenic or intragenic regions, a few (less than 5%) are in protein-coding regions. As potential drug targets, these variant-containing genes have been subjected to investigation in β cells in recent years (5) using cellular and mouse knockout systems, as described in the examples below:
SLC30A8
This gene encodes ZnT8, a zinc transporter, which is highly and selectively expressed in pancreatic islet β and, to a lesser extent, α cells. ZnT8 transports zinc ions into insulin secretory granules and is thus implicated in insulin synthesis and secretion (93, 94). SLC30A8 was first identified by GWAS in 2007 as hosting a variant affecting proinsulin processing and insulin secretion (26, 27, 39, 95). The common variant rs13266634 alters the amino acid sequence of the intracellular C-terminal domain of ZnT8 (Q325W) and has been the subject of extensive studies in the past decade. Slc30A8 null mice exhibit reduced, unaltered or increased glucose-stimulated insulin secretion (GSIS) and glycemia (96–99), as reviewed earlier (94). However, recent studies focusing on rare genetic variants such as rs200185429 in which protective allele encodes a truncated ZnT8 protein (pArg138*) demonstrated that loss of ZnT8 improves insulin secretion and β cell function both in vitro and in vivo (39, 100–102). Thus, dose-dependent changes in appear to affect overall disease risk (94) likely reflecting the multiple roles of Zn2+ ions in the β cell.
PAM
PAM (Peptidylglycine α-amidating monooxygenase) encodes an α-amidase localized to the membrane of secretory granules which is involved in insulin granule packaging and release from β cells (103). Two GWAS-identified non-synonymous SNPs in the PAM gene, rs78408340 and rs35658696, affect T2D risk (28, 104, 105). Both SNPs are associated with a reduced insulinogenic index (a measure of glucose-stimulated insulin secretion), suggesting that their effects are mediated via altered β cell function (104, 105). The two SNPs fall in the coding region of the PAM gene and lead to the amino acid changes S539W and D563G, respectively. Thomsen et al. have demonstrated that PAM deficiency results in reduced insulin content and altered dynamics of insulin secretion in a human β-cell model and in primary islets from cadaveric donors (103). The risk alleles reduce overall PAM activity via defects in expression and catalytic function (103, 106).
GWAS-Identified Genetic Variants at Non-Coding Genomic Locations
Whilst there are exceptions, such as those described above, most T2D-associated genomic variants lie in intergenic or intragenic regions. The underlying mechanisms through which these variants affect β cell function remain largely unknown though can involve altered transcription factor binding and thus modified expression of downstream target gene(s) (5). Examples of these and others are described below:
ADCY5
ADCY5 encodes adenylate cyclase 5, a Ca2+-inhibited type III adenylate cyclase, which catalyses the generation of cyclic AMP (cAMP) from ATP. The risk variant at rs11708067 in intron 3 of the ADCY5 gene is associated with elevated fasting glucose and implicated in defective proinsulin conversion to insulin (107–109). ADCY5 mRNA expression in islets is lowered by the possession of risk alleles. Our own data showed that ADCY5 silencing impairs glucose-induced cAMP increases and blocks β-cell glucose metabolism and intracellular signalling (110).
TCF7L2
This gene encodes the Wnt signalling-associated transcription factor, T-cell factor 7-like 2 (also termed transcription factor 7-like 2). Possession of risk alleles is associated with reduced glucose and glucagon-like peptide 1 (GLP-1) -stimulated insulin secretion (111). Functional analysis in β cell lines demonstrated that lower TCF7L2 expression reduces insulin gene expression and glucose-stimulated insulin secretion (GSIS) (112) but not KCl-induced insulin secretion (113) and lowered the expression of β cell genes regulating secretory granule fusion at the plasma membrane. In animal models, selective deletion of Tcf7l2 in the β cell (113, 114) replicates key aspects of the altered glucose homeostasis in human carriers of TCF7L2 risk alleles.
STARD10
STARD10 is a phospholipid transfer protein possessing a steroidogenic acute regulatory protein (StAR)-related lipid transfer (“StART”) domain that facilitates the transport of phosphatidylcholine and phosphatidylethanolamine between intracellular membranes (115). Functional GWAS (fGWAS) identified a set of credible variants in the intron 2 of STARD10 gene on chromosome 11 associated with impaired GSIS and, paradoxically but characteristically, with decreased proinsulin:insulin ratios (indicating improved proinsulin conversion) (116). In animal models, β cell-selective deletion of StarD10 gene in mice led to impaired glucose-stimulated Ca2+ dynamics and insulin secretion. Conversely, overexpression of StarD10 in the adult β cell improved glucose tolerance in high fat-fed animals (116). These data recapitulate the pattern of improved proinsulin processing observed at the human GWAS signal. STARD10 inactivation reduces GSIS both in mice (116) and in human EndoC-βH1 cells (117) and leads to profound changes in secretory granule structure in mouse beta cells (118). Solution of the 3D structure of STARD10 and direct binding assays revealed that STARD10 binds to and may transport inositol phospholipids, contributing to the failure of normal granule biogenesis in carriers of risk alleles (where STARD10 expression is lowered) (116).
C2CD4A and C2CD4B
Another genetic locus, present on chromosome 15q, and identified by GWAS (119) is associated with proinsulin levels and T2D risk. The risk variant of the single nucleotide polymorphism rs7172432 impaired GSIS in a non-diabetic Danish population (120). This SNP, together with others, lies in a stretch of the intergenic region between the C2CD4A and C2CD4B genes, which are located close to VPS13C, encoding a lipid transport protein (121) and which may also contribute to disease risk (122). In a recent study by Acilli and colleagues (123), β cell-selective deletion of C2cd4a in mice phenocopied the metabolic abnormalities of human carriers of polymorphisms at this locus, resulting in impaired insulin secretion during glucose tolerance tests as well as hyperglycemic clamps (123). Global deletion of C2cd4b leads to highly sexually dimorphic effects on glucose metabolism in mice (124) with evidence in females for actions in both the anterior pituitary -to modulate the secretion of follicle-stimulating hormone - and in β cells. On the other hand, and in contrast to the findings of others (123), who used the Ins2-depedent rat insulin promoter (RIP) promoter which can lead to deletion of β cells and in a subset of hypothalamic neurones in the ventromedial hypothalamus (125), systemic C2cd4a ablation had no effects on glucose homeostasis in the later study (124).
Enhancer Clusters: Key Regulatory Regions Mapped by Epigenetics and Chromatin Structure
Transcriptional misregulation is involved in the development of many diseases including cancer, ageing and diabetes (126–128). Distal regulatory elements, such as enhancers, play a major role in specifying cell-specific transcription patterns in both normal and diseased tissues. In the diabetes field, enhancer or enhancer clusters have been a focal point in recent genetic and epigenetic studies of β cells (41, 42, 129). T2D variants are significantly and specifically enriched in islet-specific enhancer regions (41, 42) consistent with the role of variants in the regulation of target gene expression. Mohlke’s group focused on a functional SNP, rs11708067, overlapping with an enhancer in the ADCY5 gene. These authors found that rs11708067 exhibits allelic differences in transcriptional activity and that deletion of this SNP-containing enhancer from rat INS1 (832/13) cells reduced the expression of ADCY5 gene as well as insulin secretion (130). This work established the possible role of a non-protein coding SNP in the regulation of insulin secretion. Stitzel’s group carried out a detailed analysis of rs7163757 at the C2CD4A/B locus (131). Located within an enhancer cluster region, the risk variant of rs7163757 displayed higher transcriptional activity suggesting an increased expression level of C2CD4A and C2CD4B in diabetic β cells. Furthermore, the transcription factor nuclear factor of activated T-cells (NF-AT) was identified as a key factor to alter the transcriptional activity brought about by the risk variant and the increase in enhancer activity (131).
Coregulation of Downstream Genes by Enhancer Clusters
Identified variants associated with diabetes risk, and their associated enhancer cluster, are often located far away in linear distance in the genome but physically interact with their downstream gene(s) through chromatin looping. To understand the spatial chromatin organization of human islets, chromosome conformation capture (3C) and related techniques such as (Hi-C) and promoter-Hi-C have been used to map 3D chromatin structure and to understand the interactions between enhancers and promoters (48, 129, 132–134). Through these approaches, it has become clear that enhancers, more specifically enhancer clusters, interact with multiple gene promoters through chromatin looping and simultaneously regulate multiple genes (47, 48). Causal variants are likely to influence enhancer activity and in turn entrain changes in signalling pathways in which these genes lie.
The study of non-coding variants involved in diabetes risk is still in its infancy. Nevertheless, as proof of concept, Ferrer’s group have demonstrated that alteration of variants containing enhancer activity by CRISPR interference affects the expression of multiple genes in EndoC-βH1 cells (48). Our own work, focusing on an enhancer cluster at the STARD10 locus, also showed that an enhancer cluster regulates not only STARD10 but also FCHSD2 through chromatin looping (117). Detailed analyses of how these and other variants affect chromatin structure, enhancer activity and gene expression are now warranted to elucidate the molecular mechanisms of disease pathways.
Genome Editing: Tools to Explore and Correct Genetic Defects
A challenge in modern medicine is to identify and correct mutations that lead to disease. In the context of studies in T2D these changes are usually (though not exclusively) most relevant in the pancreatic β cell [and as such are unlikely to impact the risk of other diseases, though this may be the case where the site of action, and causal gene(s), have roles outside of the pancreas]. As described above, different variants may play different roles and may require different strategies to correct at the genome level (135, 136). Towards this goal, early research indicated that double-strand DNA breaks (DSB) generated by an endonuclease can dramatically stimulate homology-directed recombination (HDR) in eukaryotic cells (137, 138). These observations led to the hunt for programmable and efficient endonucleases, leading to the development of meganucleases (139, 140). As part of the first generation of genome editing tools, meganuclease has shown its precision and effectiveness in genome editing (141, 142). However, given the length of its recognition sites—usually 12–40 bp—its practical application to genome editing is limited.
Pre-CRISPR Era
Targeted DNA regions need to be rendered accessible using molecular “scissors”, subsequently allowing the DNA repair machinery to insert a sequence of interest. Providing two such tools are zinc finger nuclease (ZFN) (143) and transcription activator-like effector nuclease (TALEN) (144, 145) (Table 2). Both are engineered DNA restriction enzymes made by fusing the DNA binding domain of each to a DNA cleavage domain. ZFN uses zinc finger protein repeats while TALEN uses the humanized bacterial transcription activator-like (TAL) effector to bind to target DNA. The DNA cleavage domain in both cases is a catalytically active FokI restriction endonuclease which effectively cuts both strands to induce DNA double-strand break (DSB). The cell then uses two DNA repair systems to repair DSB: the imprecise non-homologous end-joining (NHEJ) mechanism, which often generates deletion or mutations (termed “indels”) at the DNA cutting site, or the inefficient but precise homology-directed repair (HDR) mechanism using either single or double strand donor DNA as a template. Both tools have been used for genome editing in research and clinical settings. For examples, ZFN has been used to insert an OCT4-eGFP fusion gene into the OCT4 gene locus (146) and to correct a mutation in the human PIG-A gene in embryonic stem cells (ES) and induced pluripotent stem cell (iPSC) by HDR (147). ZFN has also been designed against an X-linked severe combined immune deficiency (SCID) mutation in the IL2Rγ gene (148).
Due to its more advanced DNA binding design, TALE can be engineered to bind to practically any desired DNA target, and thus has been used more widely than ZFN (145). In the diabetes field, TALEN has been used to inactivate the gagra and gcgrb genes in zebrafish (149), the Sulfonylurea Receptor 1 (Sur1) gene in the rat (150), and several transcription factors in human iPSC cells (151).
The disadvantages of both ZFN and TALEN-based strategies, however, lie in their complicated design. The FokI endonuclease must dimerize at the DNA binding domain in order to cleave DNA (152) and thus requires a pair of ZFNs or TALENs to target non-palindromic DNA sites. It is therefore difficult, especially when using ZFN, to design the DNA binding domain. Constructing the required DNAs consequently requires a high degree of skill in terms of both computational design and in molecular cloning.
CRISPR-Cas9: A Simple and Efficient Editing Tool to Generate Mutations or Corrections in Genomic DNA
Research into mechanisms of bacterial immunity identified an effective DNA editing system termed CRISPR (see above) based on an RNA-guided endonuclease directed against the foreign pathogen (72, 73). Engineered CRISPR systems contain two components: a single strand guide RNA (gRNA or sgRNA) and a CRISPR-associated endonuclease (Cas). The gRNA is a short synthetic RNA composed of a scaffold sequence necessary for Cas-binding and a user-defined 20 nucleotide spacer that defines the genomic target to be modified (Figure 1 and Table 2). The target is present immediately adjacent to a Protospacer Adjacent Motif (PAM) (153). Thus, the genomic target of the Cas protein is determined by the gRNA and only restricted by the PAM sequence. Cas is an endonuclease which induces a double strand DNA break. Various humanized Cas proteins, including the commonly used SpCas9 from Streptococcus pyogenes, have been generated and diversified to suit different purposes (153, 154). In comparison with both ZFN and TALEN, CRISPR provides far simpler design and DNA construction strategies, with compatible DNA cutting efficiency (Table 2) (155).
Cas9
Due to its simplicity and adaptability, CRISPR has rapidly become the most popular genome editing tool available for the mammalian genome (50, 63). Because NHEJ DNA repair often introduces unwanted indels at the Cas9 cutting site, CRISPR has been used to knock-out genes by introducing frameshift mutations, resulting in protein depletion (156, 157). In the diabetes field, CRISPR has also been adopted to study several genes in β cell lines and in human ES-derived β cells (21, 151, 158, 159) as well as in animals (160, 161).
The insertion of precise genetic modifications by genome editing tools is, however, limited by the relatively low efficiency of HDR compared with the higher efficiency of the NHEJ pathway. For this reason, correction of genetic mutations such as those associated with MODY has met with limited success up to now. NEHJ-mediated DNA repair after Cas9 cutting has been shown to be non-random but with a pattern of indel formation dependent on PAM sequence (162, 163). Hence, it is possible, though the chance of success is low, to achieve precise DNA modification through the NEHJ pathway. One successful example is the restoration of FANCA gene expression in haematopoietic stem cells (164). HDR efficiency is generally low (less than 2%) but, with CRISPR technology, it can be improved to 10-40%, depending on the target region (165, 166). Several attempts have been made to improve HDR efficiency by incorporating silent CRISPR-Cas-blocking mutations (167), suppressing NHEJ key molecules such as KU70, KU80, or DNA ligase IV (168, 169), modification of RAD18 (165), providing asymmetric donor DNA (170) and applying chemicals such as scr7 (169).
In addition to gene knock-out and HDR repair, genome-wide pooled CRISPR-Cas9 libraries have been used to systematically delete genes responsible for diverse phenotypes. Recent studies have shown that such loss-of-function screens using libraries comprising tens of thousands of sgRNAs can be used to identify genes involved in tumour growth and metastasis (171). In the diabetes field, similar approaches have also been used recently to identify key insulin gene regulators (172) and the genes involving in auto-immune killing of β cell transplants (173). Screens based on transcriptional interference (CRISPRi) and activation (CRISPRa) have also harnessed Cas9-based technologies for use in genome-wide studies (59, 174). In addition, recent improvements in lentiviral library generation and propagation, as well as large-scale DNA and RNA synthesis, have allowed CRISPR-Cas9 technology to be exploited across multiple model platforms (59, 175–178).
nCas9
The CRISPR-Cas9 system can tolerate certain mismatches to the DNA target since the required gRNAs are short. A disadvantage, however, is that this can promote undesired off target mutagenesis (53). To overcome this problem, the Cas9 enzyme has been modified in its catalytic domain (D10A) (nCas9) which allows the enzyme to nick single strand DNA rather than double strand breaks (DSB) (Figure 1 and Table 2). Because individual nicking is repaired with high fidelity, simultaneous nicking via appropriately offset guide RNAs is required for DNA double-strand breaks and extends the number of sites specifically recognized for target cleavage. This approach reduces off-target mutagenesis 50–1000-fold (53, 179). Furthermore, to improve HDR efficiency, nCas9 has been fused with RAD51 to insert disease-associated point mutations (180).
dCas9
Taking advantage of specific gRNA binding to target DNA sequences, CRISPR technology has been modified further to expand its applications in multiple ways (Figure 1 and Table 2) (154, 181). Thus, in addition to its ability to cut double strand DNA or nick single strand DNA (nCas9), Cas9 has been modified with lowered endonuclease activity (D10A and 840) which allows the enzyme to bind to the target DNA without cleavage. The catalytically-dead Cas9 (dCas9) can be further engineered to fuse with many tail proteins for a range of applications: 1. Visualization of a genomic locus: dCas9 is fused with a fluorophore (i.e. eGFP) to enable sequence-specific visualization of DNA and dynamic imaging of chromatin (182, 183). To further improve this technique, several tag proteins such as Suntag (54), CASFISH (184), CRISPRainbow (55), and CRISPR-sirius (56) can be fused to dCas9; 2. Transcriptional regulation. dCas9 is fused with either a transcriptional activator (e.g. VP64) (185–187) or repressor (e.g. CREB) (182, 188). Once recruited by gRNA to the DNA target site, the activator or repressor brings in a transcriptional complex to enhance or repress gene expression. 3. Epigenetic regulation. dCas9 is fused with acetyltransferases (64) or demethylases (65) to engineer epigenetic changes in the genome. 4. Purification of genome regions. dCas9 is fused with epitope tag(s) such as FLAG or Biotin to facilitate the purification of the molecules associated with a genomic region of interest in vivo (57, 58, 189).
Improved Cas Proteins
Although widely used, CRISPR-cas9 is far from perfect as a genome editing tool. The widely-used SpCas9 requires an NGG PAM sequence for target recognition, thereby restricting the targetable genomic loci. The Cas9 protein is large and therefore difficult to propagate in a suitable viral vector. To overcome these limitations, several laboratories have further improved the usage of SpCa9 or identified alternative Cas proteins in the Cas family (Figure 1). For example, a version of Cas9 with high fidelity (Cas9-HF1) has been developed to reduce off-target effects (190). An engineered SpCas9 variant (i.e. SpCas9-NG) has been generated to recognize an alternative PAM sequence (191, 192). Some of the Cas9 homologs in the Cas family recognize different PAM binding sites relative to SpCas9, thereby offering alternative DNA targeting capabilities (193). For example, Cas12b has been rationally modified to facilitate robust genome editing and to exhibit greater specificity compared to Cas9 (194). A particularly exciting discovery has been the discovery of Cpf1/Cas12a, a DNA endonuclease of smaller size relative to SpCas9 (51, 195). Cpf1 can process its own CRISPR RNA (crRNA) and can be used to simplify multiplexed genome editing. Using a single customized CRISPR array, it has been reported that up to four genes can be edited simultaneously by CRISPR-Cpf1 (195). To improve the efficacy of CRISPR editing, an alternative strategy targeting CRISPR RNA (crRNA) has also been developed. Structure-guided chemical modification of crRNA by, e.g. a 2’ O-methyl (2’ OMe) sugar modification, has be used to protect nuclease digestion and stabilize the crRNA/Cas protein complex in cells while maintaining or enhancing genome editing activity (196–199).
RNA Editing
Further expansion of CRISPR-Cas9 tools led to the discovery of CRISPR on RNA targets. Cas13a, previously known as C2c2, is programmed to cleave a single-stranded RNA target carrying complementary protospacer in bacteria (67, 200) or in mammalian cells (67) (Figure 1 and Table 2). Efficiency of silencing is comparable to RNA interference (RNAi) but offers improved specificity (67). Like dCas9, catalytically-dead Cas13a (dCas13a) has also been leveraged for programmable tracking of RNA transcripts in live cells (67, 201). In addition, Cas9 has also been modified for transcript tracking by RNA imaging (69). The latest addition of RNA editing comes from Type VI of CRISPR families termed CasRx (202).
CRISPR technology has also been developed as a diagnostic tool to detect DNA or RNA from biological samples. Examples include SHERLOCK (based on Cas13a) (69) and DETECTR (based on Cas12a) (203).
Limitations of CRISPR-Cas9
CRISPR provides a simple and easy tool not only for in vitro use but potentially also for in vivo genome editing. However, there are limitations and downsides to this approach. First, and despite considerable improvements in the technology, the risk of the off-target effect remains and must be considered carefully. Second, DSB may lead to wide-ranging deletions or recombination events involving the on-target site (204). Third, in cycling cells, DNA double strand breaks caused by Cas9 cleavage may trigger a P53 response leading to apoptosis and enrichment for potentially oncogenic P53-deficient cells (205, 206). Fourth, subjects may generate antibodies to Cas9, potentially limiting gene therapies (207, 208).
Base Editing: Conversion of a Single Nucleotide
Most variants of interest in the diabetes field are single base pair changes (see above). These present a significant challenge for CRISPR-Cas9 genome editing since, until recently, low efficiency HDR was the only way these could be introduced. To overcome this problem, Liu’s group have used various deaminases to convert a single nucleic acid into another (60). In this way, a single nuclear variant (SNV) can be converted into another nucleotide (209) (Figure 1 and Table 1).
Cytidine Deaminase
Cytidine deaminase catalyzes the conversion of cytosine into uracil (210, 211). The first cytidine base editor to be described was composed of dCas9 and the human apolipoprotein B mRNA editing enzyme (APOBEC) (60, 209, 212, 213). In the context of base editing, APOBEC deaminase is guided by dCas9 protein to the target DNA to convert the targeted C into U. The conversion results in a mismatch, U-G, which can be repaired by cellular mechanisms into U-A base-pair and eventually T-A base-pair (214) A second generation of cytidine deaminase-based DNA base editors was developed (BE2) using a chimeric protein of dCas9, APOBEC deaminase in addition to an uracil glycosylase (UGI) (60, 215). UGI achieves an error-free repair, which increases significantly the efficiency of base editing (216).
Apart from the human APOBEC protein, other types of cytidine deaminase have been coupled with either dCas9 or nCas9 (nickase) to introduce single point mutations. Activation-induced cytidine deaminase (AID) recruits the cytidine deaminase pmCDA1 which induce switch recombination or hypermutation in immunoglobulin produced by the human plasma cells in order to help the immune system neutralizing a larger number of pathogens through mutations in the fragment antigen-binding variable region (Fab). BE3 is based on the fusion of three proteins: dCas9, pmCDA1, and UGI (214, 217). This system, in common with earlier versions, is limited by the creation of indels. BE4, using two UGIs instead of one, appears to be more efficient than BE3.
Other CRISPR endonucleases such as Cpf1 can also be fused with deaminase. The chimeric deaminase coupling Cpf1, APOBEC and UGI (dLbCpf1-BE0) further extends the base-editing capacity to target sequences which cannot be reached by the Cas9 machinery (214, 218).
Adenosine Deaminase
Adenine can be deaminated to become inosine (219). Eukaryote polymerases subsequently base-pair inosine to guanosine, converting A into G (209, 214).
The first-generation adenine base editors were developed in bacteria. E. coli resistant to chloramphenicol acquire an adenine editing domain of edTAd-cas9 after antibiotic selective pressure (214). Adenosine deaminase based base editors (ABE) are able to deaminate adenine on single-stranded DNA and convert adenine into inosine (61, 209, 214).
Cytidine to the Three Other Nucleic Acids
The deamination process can also be used to convert cytosine and guanine to a diverse library of point mutations localized to a targeted region of the genome. Two new technologies, Targeted AID-mediated Mutagenesis (TAM) (220) and CRISPR-X (221), mimic the somatic hypermutation process. This process is generally useful during antibody affinity maturation, to generate localized sequence diversification. The TAM system is composed of dCas9 and activation-induced cytidine deaminase (AID) (214) and the CRISPR-X system, is composed of a dCas9 which recruits a hyperactive variant of the AID enzyme AID* (214, 221).
RNA Base Editing
ADAR proteins are adenosine deaminases that act on RNAs by converting adenosine to inosine. Inosine is read as guanine by the translational machinery, thereby base pairing with cytosine (214, 222). ADAR2 is an RNA-guided editor system which uses a catalytically-dead Cas13b (dCas13b) to localize an ADAR protein and convert A-I in a target double-stranded RNA causing an A-C mismatch (68, 222).
Limitation
Cytosine DNA base editors (CBE) have specificity limitations because of the uracil N-glycosylase activity (UNG). UNG is involved in unanticipated C-to-non-T edits (60, 209, 223). Therefore, cytosine base editors fused with one or more UNG inhibitors (UGI) show a significant increase in their editing specificity. In addition, some point mutations can allow overexpression of UGI in trans which can further improve UGI activity and thereby the editing product purity (60). The cytosine base editor also exhibits indel formation which can be reduced by fusing the bacteriophage Mu-derived Gam (Mu-GAM) protein to CBE (224).
In the case of a target sequence exhibiting several C or A bases, conversion of bases in addition to the target base can occur (“bystander editing”). Base editing systems with wide editing windows are more likely to suffer from this problem (225). Some specific mutations in the APOBEC1 domain have been shown to reduce deamination activity and lower bystander editing.
Search and Replace: A New Tool With a Simpler and Versatile Way of Genome Editing
Both CRISPR-Cas9 and base editing provide easy and rapid gene editing approaches, but they lack precision and often cause unwanted on- and off-target effects. In some cases, double strand DNA breaks can produce large deletions in nearby genome elements (204). Addressing some of these issues a new editing tool, termed prime editing or search and replace, has been developed recently by David Liu’s group (62) (Figure 1 and Table 2). Here, a catalytically-impaired Cas9 (nCas9) fused to an engineered reverse transcriptase (nCas9-RT) is programmed with a prime editing guide RNA (pegRNA) that both specify the target site and encode the desired edit. Because this approach uses nickase (nCas9), it offers much lower off-target editing than Cas9 nuclease, and thus generates fewer by-products. This strategy also offers efficiency and product purity advantages over HDR replacement, and complementary strengths and weaknesses compared to base editing.
In Vitro and In Vivo β Cell Models for Studying Genetic Variants
In order to understand the pathogenic role of diabetes-associated genetic variants, tractable β cell models are essential. Mouse models, either transgenic or knock-out, are valuable for examining the roles of single genes, but their use is more limited in studies of intergenic regions given more substantial inter-species (mouse versus human) differences in these regions. As sources of human β cells, there are currently three possibilities. Firstly, primary islets isolated from organ donors: This source is, however, limited in terms of the availability and quality of islets (226). Secondly, clonal human β cells. Immortalized human EndoC-βH1 cells were developed in recent years after infection of foetal islets with large T antigen and further inoculation of islets in immunocompromised mice (227). Later generation EndoC-βH2 (228) and EndoC-βH3 (229) cell lines were subsequently established with more advanced features including regulated deletion of the immortalizing gene. The limitation of these cell lines, however, is their extremely slow growth rate which hampers their use. Given this slow growth rate—and the fact that these lines poorly tolerate expansion from a single cell—it is virtually impossible to modify them by HDR via CRISPR editing. A third possibility are therefore islet-like cells differentiated from human embryonic stem cells (hESC) or patient-derived induced pluripotent stem cells (iPSC). In light of the limitations of the above cellular models, laboratories are now focusing on hESC or iPSC in studies of gene function throughout β cell development by differentiating hESC/iPSC cells into mature β cells (230, 231). Such directed differentiation protocols have recently been improved (21, 159).
Application of Genome Editing to Disease-Relevant Genetic Loci In β Cells
MODY. Animal models have been generated using CRISPR-Cas9 technology to study features of HNF4A (232), as well as a GCK mutant rabbit model exhibiting many features of HNF4A (233) and INS mutant piglets which are insulin-deficient (161). Similarly, a CRISPR-Cas9 nuclease was used to create the MODY gene reporter through homologous recombination, such as PDX1-eGFP reporter (234). In the latter study, a CRISPR-on system was also fused as a transcriptional activator (dCas9-VP160) to activate the transcription of endogenous human INS gene in human stem cells (235).
Patient-derived human induced pluripotent stem cells iPSCs from people with diabetes can be used to model disease in vitro, and many labs have made huge efforts to generate iPSC lines from people with MODY (236). Although most of the resulting lines have not been fully characterized (given cost limitations and the challenges associated with β cell differentiation), interesting data have been obtained. For example, an iPSC line carrying a heterozygous mutation in HNF1BS148L/+ exhibited compensatory up-regulation of several key endocrine pancreas-enriched transcription factors including PDX1 during β-cell differentiation (237). Other HNF1B mutant lines were also generated from people carrying HNF1B mutations (236–238). HNF1A mutant lines were established from patients with different variants (239) including HNF1AS142F (240) and HNF1AP291fsinsC (236). Similarly, a GCK mutant line carrying GCKV62A has been established (236). HNF1A and CEL lines have also been generated from patient samples (236).
CRISPR technology has been used recently to correct point mutations in patient-derived iPSCs to target diabetes-related gene defects. To date, the most efficient method used in iPSC is CRISPR/Cas9-based homology-directed repair (HDR). Here, a Cas9-mediated cut is generated adjacent to the site of interest. A homologous donor template with the intended nucleotide change containing silent mutations in the gRNA sequence (167) can then be recombined by HDR. This approach has been used successfully to investigate STAT3 and GATA6 mutant iPSC lines generated by reprogramming patient cells expressing a heterozygous mutation (241, 242). Mutations in both genes were corrected with CRISPR/Cas9 and completely reversed the disease phenotype.
GWAS-Identified Genes
Following the successful identification of genetic loci by GWAS, several candidate genes within or surrounding genetic loci which are thought to play roles in β cell function, in particular, in proinsulin processing and secretion, have been examined in mechanistic studies. Gene editing tools have quickly replaced techniques such as shRNA-based silencing and HDR-mediated deletion to become a mainstream technique in studies of gene function. For example, the critical β cell-enriched NEUROD1 and SLC30A8 genes were deleted in EndoC-βH1 cells using these approaches in recent studies (243). Similarly, pancreatic duodenum homeobox-1 (PDX1), an important regulator of the INS gene, was also mutated by CRISPR-Cas9 resulting in a line with defective glucose-induced Ca2+ influx and insulin secretion (244). Our laboratory has inactivated the type 2 diabetes-related STARD10 and FCHSD2 genes in EndoC-βH1 cells using a lentiviral approach and demonstrated effects on insulin secretion (and see above) (117). Furthermore, Fang et al. used CRISPR screening technology and identified several genes involved in insulin regulation in mouse MIN6 cells (172).
Gene editing in hESC/iPSC cells has also been documented. The NEUROG3 gene, a transcription factor essential for the development of pancreatic endocrine cells in mice, was knocked-out by CRISPR-Cas9 in hESC cells and confirmed there was no endocrine cells formed from pancreatic progenitors (245). Chen’s lab used CRISPR-Cas9 to knock out three β cell-specific transcription factor genes in hESC cells and proved the usefulness of this hESC cell platform (158). Similar studies were reported by Huangfu and colleagues who used either TALEN or CRISPR-Cas9 to systematically delete several β cell transcription factors, demonstrating roles in human β cell development and function (21, 151).
Enhancers and Genetic Variants
Genetic enhancer elements, critical determinants of cellular identity, are usually identified based on chromatin marks and gain-of-function potential, though only loss-of-function studies can demonstrate their requirement in the native genomic context. Various CRISPR technologies have been applied to identify potential enhancer regions (177), the critical transcriptional factor binding site for gene expression (246), long-range gene regulation in normal and malignant cells (247–249).
The application of CRISPR to studies of enhancers in β cells is still at an early stage. Malkon’s laboratory (130) have deleted a variant-containing enhancer region within the ADCY5 gene in INS1 cells and demonstrated reduced ADCY5 gene expression. Also using CRISPR-Cas9, we deleted an active enhancer within an enhancer cluster in the STARD10 locus in EndoC-βH1 cells which reduced the expression of both the STARD10 and FCHSD2 genes (117). Moreover, CRISPR interference techniques (CRISPRi and CRISPRa) have been used to modulate the transcriptional activities of several enhancer regions in GWAS-identified genetic loci to demonstrate that altered enhancer activity impacts the expression of multiple genes within enhancer hub (48). A similar attempt was also carried out on enhancer activity by CRISPRa to increase endogenous human INS gene expression (250).
Attempts to correct genetic variants via HDR have also been reported. Mutations in the INS gene affecting insulin (M1I) or proinsulin (C96R, C109Y) were corrected by HDR via CRISPR-Cas9 editing to restore insulin production in differentiated iPSC cells that mimicked neonatal diabetes (251, 252). Likewise, Shi et al. converted a patient-specific mutation in GATA6 gene and showed that the mutation involved (GATA6R456C) has a similar effect to GATA6 knockout (21). Most recently, correction of a variant in the Wolfram syndrome 1 (WFS1) gene by CRISPR-mediated HDR improved insulin secretion in iPSC-differentiated β-like cells (253). Studies on GWAS identified genetic variants especially those in the intragenic and intergenic regions have not been reported. Given their functional importance on chromatin structure, enhancer activity and gene regulation, it is predicted that variant conversion in human β cells will attract huge interests in near future studies.
Genome Editing in Animal Models
Over the past three decades, gene knockout in animals, especially in mice, has provided invaluable information of gene function (254). The traditional way of gene deletion carried out in embryonic stem cells (ES) is through homology-directed recombination to delete a piece of genomic DNA, such as an exon, to achieve systemic (whole body) knockout. Tissue or cell-selective gene knockout can be achieved by using the “Cre-loxP” system (255, 256). CRISPR-Cas9 mediated gene knockout, as a relatively new approach, provides a much simpler and effective way to achieve gene knockout in vivo, though the generation of LoxP sites flanking a gene or exon of interest is more challenging. In brief, the gRNA is synthesized, or in vitro transcribed, and then complexed with Cas9 protein to form a ribonucleoprotein (RNP) complex. This complex can be directly injected into fertilized mouse zygote (156) or electroporated in situ into the oviduct of a pregnant female mouse (257, 258). The complex exerts its effect at target DNA by generating indel (with one gRNA) or deletion (with two gRNAs) mutations or DNA replacement through HDR with a donor template (156, 156, 259, 260).
Conditional knockout (cKO) mice are also an extremely valuable tool in biomedical research because they enable detailed analyses of gene function in a tissue- and/or temporal-specific fashion. However, the conventional method for generating cKO mice is time-consuming and labour intensive, and involves making a large gene-targeting construct, transfecting and screening many embryonic stem (ES) cell clones, injecting positive ES clones into blastocysts to produce chimeric mice, and breeding the chimaeras to transmit the targeted gene through the germline. This procedure can be substantially simplified by providing a CRISPR ribonucleoprotein RNP and single strand edDNA (ssDNA) donor which carries desired changes such as insertion of loxP site (255, 259–265). Using CRISPR-Cas9, leptin and leptin receptor knockout mice have been established as tools in diabetes and obesity research (160, 255, 256). Knock-in mouse models have also been established via HDR to achieve cell-specific deletion of the gene (266).
Genome Editing: Clinical Application in Diabetes
An important goal in genetic research is to identify the genetic defects underlying disease pathogenesis and introduce corrections to restore normal gene function. In this respect, CRISPR-based technologies hold enormous potential in a therapeutic setting, offering an approach to permanently correct disease-causing mutations. The delivery of genome editing tools to the target cells can be broadly categorized into in vivo and ex vivo approaches. Both approaches have been extensively practised in the broader gene and cell therapy field and have achieved some clinical success (267). In recent years, such delivery strategies have also been extended to CRISPR-based therapeutics (267, 268). In vivo delivery aims to introduce genome editing reagents into patients systemically or locally to directly manipulate cells in the body (269). In ex vivo delivery, genome editing reagents are introduced into isolated human cells to achieve the desired genetic modification. After expansion, the genetically-modified cells are infused into patients to confer a therapeutic effect (270) (Figure 2).
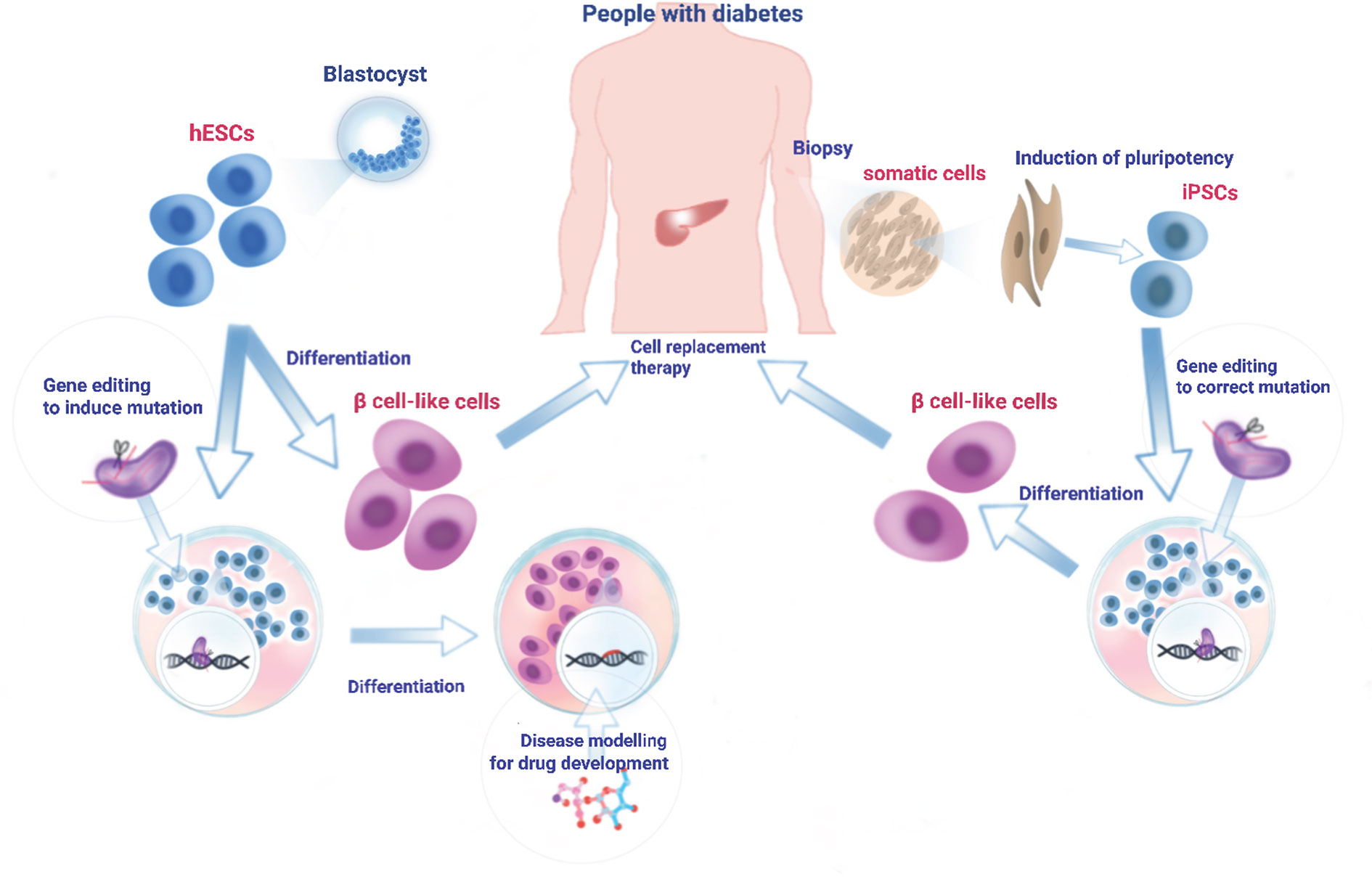
Figure 2 Generation, differentiation, and transplantation of iPSC cells into diabetic patients. A biopsy (skin fibroblasts, cord blood, or peripheral blood cells) is obtained from diabetes patient and cultured in the laboratory. Cultured cells are reprogrammed into iPSC cells using Yamanaka factors (271). To correct genetic mutation(s) or convert risk variant(s) into protective variant(s), iPSC can be edited at the genome level using one of the genome-editing tools. Engineered iPSC cells are then differentiated into β cell-like cells through a stepwise differentiation protocol by exposing the cells to specific growth factors/cytokines and signalling proteins. Alternatively, hESCs derived from healthy individuals can be cultured in the laboratory and driven to differentiate towards mature β cells. Those in vitro generated β cell-like cells which are free of mutation(s) can then be transplanted into patients to reverse diabetes. In addition, hESCs can be genome-edited in reverse to create disease-related mutation(s) and then subjected to directed β cell differentiation. Such mutation-bearing β cell-like cells can be used for the molecular mechanistic studies of disease as well as drug screening to identify therapeutic reagents.
In vivo delivery of CRISPR editing tools into pancreatic β cells in people with diabetes is likely to face enormous challenges for two main reasons: 1. β cells are postmitotic, thus disfavouring HDR-mediated CRISPR editing. 2. Selective targeting to these cells will be required, likely involving cell type-tropic viruses (272), raising evident concerns over off-target effects and toxicity. Hence, the most likely and feasible way of CRISPR editing has to be an ex vivo system where β cells can first be engineered by CRISPR editing and then transplanted into patients (Figure 2).
Ex Vivo
A major problem for cell-based treatment for diabetes patients is the lack of a suitable β cell source. hESC and iPSC cells provide potential means to produce sufficient amounts of high quality β cells for transplantation. Protocols for hESC/iPSC differentiation towards mature β cells were readily established in several laboratories (158, 230, 231, 273, 274). Importantly, these in vitro differentiated β like cells have the same physiological functions as mature β cells, i.e. producing and secreting insulin in response to various stimuli including glucose after transplantation in an immunocompromised mouse model (230, 231). However, the use of iPSC is controversial and there are some concerns over genetic and epigenetic variations in iPSCs which might affect cell function after differentiation (275).
Manipulation of hESC/iPSC cells via CRISPR-Cas9 technology provides a platform for the correction of genomic mutations not only in diabetes but in other disease fields as well (276–279). Through CRISPR-mediated HDR and base editing, it is possible to correct the vast majority of genetic variants, if not all. Conversion of GWAS-identified non-coding variants has not been conducted/documented in the diabetes field, but it seems inevitable that such work will be carried out in the near future given its importance in basic research and potential clinical application. Variants identified by GWAS are often clustered in the genome (134). Although an individual variant may change transcription factor binding on its own, neighbouring risk variants might cooperate to change the transcriptional landscape of local chromatin and thus the activity of the enhancer cluster leading to changes in the expression of multiple genes whose aggregate effect is to impair β cell function. Hence, multiplex genome-editing needs to be carried out to convert multiple risk variants into protective (non-risk) variants in hESC or iPSC cells. In this case, the off-target effects brought by multiplex gRNAs may have a large impact on the rest of the genome and raise major concerns.
In view of the above, genome editing tools need to be carefully selected. The newly developed nCas9-RT holds great potential: 1. The nCas9 nicks the DNA rather than induces DSB and therefore avoids indel formation at the cutting site; 2. The use of pegRNA, which is a combination of gRNA, reverse transcription template and primer-binding sites, increases the specificity of target DNA binding hence reduces off-targets (62); 3. While multiplex pegRNAs could target various variants including SNPs, deletions or insertions without separating DNA donors as templates, it is possible the nCas9-RT will be able to convert all variants at once. This new technique, however, is still in early development, and its editing efficiency and side-effects remain to be seen.
Future Prospectives
Recent technological developments around CRISPR-Cas9 and its derivative technologies, combined with advances in human cellular models, should accelerate our understanding of the interplay between diabetes risk-associated genetic variants and their functional roles in disease pathogenesis. These approaches may also find use in clinical applications and in drug screens (Figure 2), enhancing the development of precision medicines for personalized treatment.
Author Contributions
MH and IC wrote the first draft of the manuscript. SM provided comments and suggestions especially on MODY genes. GR devised the structure of the manuscript and revised the draft. All authors contributed to the article and approved the submitted version.
Funding
GR was supported by Wellcome Trust Senior Investigator (WT098424AIA) and Investigator (212625/Z/18/Z) Awards, MRC Programme grants (MR/R022259/1, MR/J0003042/1, MR/L020149/1) and Experimental Challenge Grant (DIVA, MR/L02036X/1), MRC (MR/N00275X/1), Diabetes UK (BDA/11/0004210, BDA/15/0005275, BDA 16/0005485) and Imperial Confidence in Concept (ICiC) grants, and a Royal Society Wolfson Research Merit Award. This project has received funding from the European Union’s Horizon 2020 research and innovation programme via the Innovative Medicines Initiative 2 Joint Undertaking under grant agreement No 115881 (RHAPSODY). This Joint Undertaking receives support from the European Union’s Horizon 2020 research and innovation programme and EFPIA.
Conflict of Interest
GR has received grant funding from Les Laboratoires Servier and is a consultant for Sun Pharmaceuticals.
The remaining authors declare that the research was conducted in the absence of any commercial or financial relationships that could be construed as a potential conflict of interest.
Abbreviations
DSB, double-strand breaks; HDR, homology-directed DNA repair; T1D, T2D, type 1, type 2 diabetes.
References
1. Cho NH, Shaw JE, Karuranga S, Huang Y, da Rocha Fernandes JD, Ohlrogge AW, et al. IDF Diabetes Atlas: Global estimates of diabetes prevalence for 2017 and projections for 2045. Diabetes Res Clin Pract (2018) 138:271–81. doi: 10.1016/j.diabres.2018.02.023
2. Cersosimo E, Triplitt C, Solis-Herrera C, Mandarino LJ, DeFronzo RA. Pathogenesis of Type 2 Diabetes Mellitus, in: Endotext . South Dartmouth (MA: MDText.com, Inc. Available at: http://www.ncbi.nlm.nih.gov/books/NBK279115/ (Accessed May 12, 2020).
3. Harlan DM, Kenyon NS, Korsgren O, Roep BO. Current Advances and Travails in Islet Transplantation. Diabetes (2009) 58:2175–84. doi: 10.2337/db09-0476
4. McCarthy MI. Progress in defining the molecular basis of type 2 diabetes mellitus through susceptibility-gene identification. Hum Mol Genet (2004) 13 Spec No 1:R33–41. doi: 10.1093/hmg/ddh057
5. Rutter GA. Dorothy Hodgkin Lecture 2014. Understanding genes identified by genome-wide association studies for type 2 diabetes. Diabetes Med J Br Diabetes Assoc (2014) 31:1480–7. doi: 10.1111/dme.12579
6. Mahajan A, Taliun D, Thurner M, Robertson NR, Torres JM, Rayner NW, et al. Fine-mapping type 2 diabetes loci to single-variant resolution using high-density imputation and islet-specific epigenome maps. Nat Genet (2018) 50:1505–13. doi: 10.1038/s41588-018-0241-6
8. Hattersley AT. Maturity-onset diabetes of the young: clinical heterogeneity explained by genetic heterogeneity. Diabetes Med J Br Diabetes Assoc (1998) 15:15–24. doi: 10.1002/(SICI)1096-9136(199801)15:1<15::AID-DIA562>3.0.CO;2-M
9. Valkovicova T, Skopkova M, Stanik J, Gasperikova D. Novel insights into genetics and clinics of the HNF1A-MODY. Endocr Regul (2019) 53:110–34. doi: 10.2478/enr-2019-0013
10. Warncke K, Kummer S, Raile K, Grulich-Henn J, Woelfle J, Steichen E, et al. Frequency and Characteristics of MODY 1 (HNF4A Mutation) and MODY 5 (HNF1B Mutation): Analysis From the DPV Database. J Clin Endocrinol Metab (2019) 104:845–55. doi: 10.1210/jc.2018-01696
11. Murphy R, Ellard S, Hattersley AT. Clinical implications of a molecular genetic classification of monogenic beta-cell diabetes. Nat Clin Pract Endocrinol Metab (2008) 4:200–13. doi: 10.1038/ncpendmet0778
12. McKinnon CM, Docherty K. Pancreatic duodenal homeobox-1, PDX-1, a major regulator of beta cell identity and function. Diabetologia (2001) 44:1203–14. doi: 10.1007/s001250100628
13. Sagen JV, Baumann ME, Salvesen HB, Molven A, Søvik O, Njølstad PR. Diagnostic screening of NEUROD1 (MODY6) in subjects with MODY or gestational diabetes mellitus. Diabetes Med J Br Diabetes Assoc (2005) 22:1012–5. doi: 10.1111/j.1464-5491.2005.01565.x
14. Horikawa Y, Enya M. Genetic Dissection and Clinical Features of MODY6 (NEUROD1-MODY). Curr Diabetes Rep (2019) 19:12. doi: 10.1007/s11892-019-1130-9
15. Johansson BB, Fjeld K, El Jellas K, Gravdal A, Dalva M, Tjora E, et al. The role of the carboxyl ester lipase (CEL) gene in pancreatic disease. Pancreatol Off J Int Assoc Pancreatol IAP Al (2018) 18:12–9. doi: 10.1016/j.pan.2017.12.001
16. Rangel-Coronado R, Chávez-García T, Mendoza-Zubieta V, Ferreira-Hermosillo A. Maturity Onset Diabetes of the Young: case report. Rev Med Inst Mex Seguro Soc (2019) 57:252–8.
17. Bowman P, Flanagan SE, Edghill EL, Damhuis A, Shepherd MH, Paisey R, et al. Heterozygous ABCC8 mutations are a cause of MODY. Diabetologia (2012) 55:123–7. doi: 10.1007/s00125-011-2319-x
18. Bonnefond A, Philippe J, Durand E, Dechaume A, Huyvaert M, Montagne L, et al. Whole-exome sequencing and high throughput genotyping identified KCNJ11 as the thirteenth MODY gene. PLoS One (2012) 7:e37423. doi: 10.1371/journal.pone.0037423
19. Prudente S, Jungtrakoon P, Marucci A, Ludovico O, Buranasupkajorn P, Mazza T, et al. Loss-of-Function Mutations in APPL1 in Familial Diabetes Mellitus. Am J Hum Genet (2015) 97:177–85. doi: 10.1016/j.ajhg.2015.05.011
20. Smith SB, Qu H-Q, Taleb N, Kishimoto N, Scheel DW, Lu Y, et al. Rfx6 Directs Islet Formation and Insulin Production in Mice and Humans. Nature (2010) 463:775–80. doi: 10.1038/nature08748
21. Shi Z-D, Lee K, Yang D, Amin S, Verma N, Li QV, et al. Genome Editing in hPSCs Reveals GATA6 Haploinsufficiency and a Genetic Interaction with GATA4 in Human Pancreatic Development. Cell Stem Cell (2017) 20:675–688.e6. doi: 10.1016/j.stem.2017.01.001
22. Allen HL, Flanagan SE, Shaw-Smith C, De Franco E, Akerman I, Caswell R, et al. GATA6 haploinsufficiency causes pancreatic agenesis in humans. Nat Genet (2011) 44:20–2. doi: 10.1038/ng.1035
23. Sellick GS, Barker KT, Stolte-Dijkstra I, Fleischmann C, Coleman RJ, Garrett C, et al. Mutations in PTF1A cause pancreatic and cerebellar agenesis. Nat Genet (2004) 36:1301–5. doi: 10.1038/ng1475
24. Delépine M, Nicolino M, Barrett T, Golamaully M, Mark Lathrop G, Julier C. EIF2AK3 , encoding translation initiation factor 2-α kinase 3, is mutated in patients with Wolcott-Rallison syndrome. Nat Genet (2000) 25:406–9. doi: 10.1038/78085
25. Gupta S, McGrath B, Cavener DR. PERK (EIF2AK3) Regulates Proinsulin Trafficking and Quality Control in the Secretory Pathway. Diabetes (2010) 59:1937–47. doi: 10.2337/db09-1064
26. Sladek R, Rocheleau G, Rung J, Dina C, Shen L, Serre D, et al. A genome-wide association study identifies novel risk loci for type 2 diabetes. Nature (2007) 445:881–5. doi: 10.1038/nature05616
27. Scott LJ, Mohlke KL, Bonnycastle LL, Willer CJ, Li Y, Duren WL, et al. A genome-wide association study of type 2 diabetes in Finns detects multiple susceptibility variants. Science (2007) 316:1341–5. doi: 10.1126/science.1142382
28. Fuchsberger C, Flannick J, Teslovich TM, Mahajan A, Agarwala V, Gaulton KJ, et al. The genetic architecture of type 2 diabetes. Nature (2016) 536:41–7. doi: 10.1038/nature18642
29. Voight BF, Scott LJ, Steinthorsdottir V, Morris AP, Dina C, Welch RP, et al. Twelve type 2 diabetes susceptibility loci identified through large-scale association analysis. Nat Genet (2010) 42:579–89. doi: 10.1038/ng.609
30. Zeggini E, Weedon MN, Lindgren CM, Frayling TM, Elliott KS, Lango H, et al. Replication of genome-wide association signals in UK samples reveals risk loci for type 2 diabetes. Science (2007) 316:1336–41. doi: 10.1126/science.1142364
31. Vujkovic M, Keaton JM, Lynch JA, Miller DR, Zhou J, Tcheandjieu C, et al. Discovery of 318 new risk loci for type 2 diabetes and related vascular outcomes among 1.4 million participants in a multi-ancestry meta-analysis. Nat Genet (2020) 52:680–91. doi: 10.1038/s41588-020-0637-y
32. Manolio TA, Collins FS, Cox NJ, Goldstein DB, Hindorff LA, Hunter DJ, et al. Finding the missing heritability of complex diseases. Nature (2009) 461:747–53. doi: 10.1038/nature08494
33. McCarthy MI. Painting a new picture of personalised medicine for diabetes. Diabetologia (2017) 60:793–9. doi: 10.1007/s00125-017-4210-x
34. Ahlqvist E, Storm P, Käräjämäki A, Martinell M, Dorkhan M, Carlsson A, et al. Novel subgroups of adult-onset diabetes and their association with outcomes: a data-driven cluster analysis of six variables. Lancet Diabetes Endocrinol (2018) 6:361–9. doi: 10.1016/S2213-8587(18)30051-2
35. Nguyen-Tu M-S, Martinez-Sanchez A, Leclerc I, Rutter GA, Xavier G da S. Reduced expression of TCF7L2 in adipocyte impairs glucose tolerance associated with decreased insulin secretion, incretins levels and lipid metabolism dysregulation in male mice. bioRxiv (2020). doi: 10.1101/2020.05.18.102384
36. Hrdlickova B, de Almeida RC, Borek Z, Withoff S. Genetic variation in the non-coding genome: Involvement of micro-RNAs and long non-coding RNAs in disease. Biochim Biophys Acta BBA - Mol Basis Dis (2014) 1842:1910–22. doi: 10.1016/j.bbadis.2014.03.011
37. Edwards SL, Beesley J, French JD, Dunning AM. Beyond GWASs: Illuminating the Dark Road from Association to Function. Am J Hum Genet (2013) 93:779–97. doi: 10.1016/j.ajhg.2013.10.012
38. Yahaya TO, Salisu TF. A Review of Type 2 Diabetes Mellitus Predisposing Genes. Curr Diabetes Rev (2019) 16:52–61. doi: 10.2174/1573399815666181204145806
39. Flannick J, Mercader JM, Fuchsberger C, Udler MS, Mahajan A, Wessel J, et al. Exome sequencing of 20,791 cases of type 2 diabetes and 24,440 controls. Nature (2019) 570:71–6. doi: 10.1038/s41586-019-1231-2
40. Bysani M, Agren R, Davegårdh C, Volkov P, Rönn T, Unneberg P, et al. ATAC-seq reveals alterations in open chromatin in pancreatic islets from subjects with type 2 diabetes. Sci Rep (2019) 9:7785. doi: 10.1038/s41598-019-44076-8
41. Parker SCJ, Stitzel ML, Taylor DL, Orozco JM, Erdos MR, Akiyama JA, et al. Chromatin stretch enhancer states drive cell-specific gene regulation and harbor human disease risk variants. Proc Natl Acad Sci U S A (2013) 110:17921–6. doi: 10.1073/pnas.1317023110
42. Pasquali L, Gaulton KJ, Rodríguez-Seguí SA, Mularoni L, Miguel-Escalada I, Akerman İ., et al. Pancreatic islet enhancer clusters enriched in type 2 diabetes risk-associated variants. Nat Genet (2014) 46:136–43. doi: 10.1038/ng.2870
43. Whyte WA, Orlando DA, Hnisz D, Abraham BJ, Lin CY, Kagey MH, et al. Master transcription factors and mediator establish super-enhancers at key cell identity genes. Cell (2013) 153:307–19. doi: 10.1016/j.cell.2013.03.035
44. Hnisz D, Abraham BJ, Lee TI, Lau A, Saint-André V, Sigova AA, et al. Super-enhancers in the control of cell identity and disease. Cell (2013) 155:934–47. doi: 10.1016/j.cell.2013.09.053
45. de Wit E, de Laat W. A decade of 3C technologies: insights into nuclear organization. Genes Dev (2012) 26:11–24. doi: 10.1101/gad.179804.111
46. Schmitt AD, Hu M, Ren B. Genome-wide mapping and analysis of chromosome architecture. Nat Rev Mol Cell Biol (2016) 17:743–55. doi: 10.1038/nrm.2016.104
47. Javierre BM, Burren OS, Wilder SP, Kreuzhuber R, Hill SM, Sewitz S, et al. Lineage-Specific Genome Architecture Links Enhancers and Non-coding Disease Variants to Target Gene Promoters. Cell (2016) 167:1369–1384.e19. doi: 10.1016/j.cell.2016.09.037
48. Miguel-Escalada I, Bonàs-Guarch S, Cebola I, Ponsa-Cobas J, Mendieta-Esteban J, Atla G, et al. Human pancreatic islet three-dimensional chromatin architecture provides insights into the genetics of type 2 diabetes. Nat Genet (2019) 51:1137–48. doi: 10.1038/s41588-019-0457-0
49. Komor AC, Badran AH, Liu DR. CRISPR-Based Technologies for the Manipulation of Eukaryotic Genomes. Cell (2017) 168:20–36. doi: 10.1016/j.cell.2016.10.044
50. Cong L, Ran FA, Cox D, Lin S, Barretto R, Habib N, et al. Multiplex genome engineering using CRISPR/Cas systems. Science (2013) 339:819–23. doi: 10.1126/science.1231143
51. Zetsche B, Gootenberg JS, Abudayyeh OO, Slaymaker IM, Makarova KS, Essletzbichler P, et al. Cpf1 is a single RNA-guided endonuclease of a class 2 CRISPR-Cas system. Cell (2015) 163:759–71. doi: 10.1016/j.cell.2015.09.038
52. Liu J-J, Orlova N, Oakes BL, Ma E, Spinner HB, Baney KLM, et al. CasX enzymes comprise a distinct family of RNA-guided genome editors. Nature (2019) 566:218–23. doi: 10.1038/s41586-019-0908-x
53. Ran FA, Hsu PD, Lin C-Y, Gootenberg JS, Konermann S, Trevino AE, et al. Double nicking by RNA-guided CRISPR Cas9 for enhanced genome editing specificity. Cell (2013) 154:1380–9. doi: 10.1016/j.cell.2013.08.021
54. Tanenbaum ME, Gilbert LA, Qi LS, Weissman JS, Vale RD. A protein-tagging system for signal amplification in gene expression and fluorescence imaging. Cell (2014) 159:635–46. doi: 10.1016/j.cell.2014.09.039
55. Ma H, Tu L-C, Naseri A, Huisman M, Zhang S, Grunwald D, et al. Multiplexed labeling of genomic loci with dCas9 and engineered sgRNAs using CRISPRainbow. Nat Biotechnol (2016) 34:528–30. doi: 10.1038/nbt.3526
56. Ma H, Tu L-C, Naseri A, Chung Y-C, Grunwald D, Zhang S, et al. CRISPR-Sirius: RNA Scaffolds for Signal Amplification in Genome Imaging. Nat Methods (2018) 15:928–31. doi: 10.1038/s41592-018-0174-0
57. Fujita T, Fujii H. Identification of proteins associated with an IFNγ-responsive promoter by a retroviral expression system for enChIP using CRISPR. PLoS One (2014) 9:e103084. doi: 10.1371/journal.pone.0103084
58. Liu X, Zhang Y, Chen Y, Li M, Zhou F, Li K, et al. In Situ Capture of Chromatin Interactions by Biotinylated dCas9. Cell (2017) 170:1028–1043.e19. doi: 10.1016/j.cell.2017.08.003
59. Shalem O, Sanjana NE, Zhang F. High-throughput functional genomics using CRISPR-Cas9. Nat Rev Genet (2015) 16:299–311. doi: 10.1038/nrg3899
60. Komor AC, Kim YB, Packer MS, Zuris JA, Liu DR. Programmable editing of a target base in genomic DNA without double-stranded DNA cleavage. Nature (2016) 533:420–4. doi: 10.1038/nature17946
61. Gaudelli NM, Komor AC, Rees HA, Packer MS, Badran AH, Bryson DI, et al. Programmable base editing of A•T to G•C in genomic DNA without DNA cleavage. Nature (2017) 551:464–71. doi: 10.1038/nature24644
62. Anzalone AV, Randolph PB, Davis JR, Sousa AA, Koblan LW, Levy JM, et al. Search-and-replace genome editing without double-strand breaks or donor DNA. Nature (2019) 576:149–57. doi: 10.1038/s41586-019-1711-4
63. Gilbert LA, Larson MH, Morsut L, Liu Z, Brar GA, Torres SE, et al. CRISPR-mediated modular RNA-guided regulation of transcription in eukaryotes. Cell (2013) 154:442–51. doi: 10.1016/j.cell.2013.06.044
64. Hilton IB, D’Ippolito AM, Vockley CM, Thakore PI, Crawford GE, Reddy TE, et al. Epigenome editing by a CRISPR-Cas9-based acetyltransferase activates genes from promoters and enhancers. Nat Biotechnol (2015) 33:510–7. doi: 10.1038/nbt.3199
65. Kearns NA, Pham H, Tabak B, Genga RM, Silverstein NJ, Garber M, et al. Functional annotation of native enhancers with a Cas9-histone demethylase fusion. Nat Methods (2015) 12:401–3. doi: 10.1038/nmeth.3325
66. Lei Y, Zhang X, Su J, Jeong M, Gundry MC, Huang Y-H, et al. Targeted DNA methylation in vivo using an engineered dCas9-MQ1 fusion protein. Nat Commun (2017) 8:16026. doi: 10.1038/ncomms16026
67. Abudayyeh OO, Gootenberg JS, Essletzbichler P, Han S, Joung J, Belanto JJ, et al. RNA targeting with CRISPR-Cas13. Nature (2017) 550:280–4. doi: 10.1038/nature24049
68. Cox DBT, Gootenberg JS, Abudayyeh OO, Franklin B, Kellner MJ, Joung J, et al. RNA editing with CRISPR-Cas13. Science (2017) 358:1019–27. doi: 10.1126/science.aaq0180
69. Nelles DA, Fang MY, O’Connell MR, Xu JL, Markmiller SJ, Doudna JA, et al. Programmable RNA Tracking in Live Cells with CRISPR/Cas9. Cell (2016) 165:488–96. doi: 10.1016/j.cell.2016.02.054
70. Gootenberg JS, Abudayyeh OO, Lee JW, Essletzbichler P, Dy AJ, Joung J, et al. Nucleic acid detection with CRISPR-Cas13a/C2c2. Science (2017) 356:438–42. doi: 10.1126/science.aam9321
71. Gootenberg JS, Abudayyeh OO, Kellner MJ, Joung J, Collins JJ, Zhang F. Multiplexed and portable nucleic acid detection platform with Cas13, Cas12a, and Csm6. Science (2018) 360:439–44. doi: 10.1126/science.aaq0179
72. Jinek M, Chylinski K, Fonfara I, Hauer M, Doudna JA, Charpentier E. A programmable dual-RNA-guided DNA endonuclease in adaptive bacterial immunity. Science (2012) 337:816–21. doi: 10.1126/science.1225829
73. Wiedenheft B, Sternberg SH, Doudna JA. RNA-guided genetic silencing systems in bacteria and archaea. Nature (2012) 482:331–8. doi: 10.1038/nature10886
74. Anık A, Çatlı G, Abacı A, Böber E. Maturity-onset diabetes of the young (MODY): an update. J Pediatr Endocrinol Metab JPEM (2015) 28:251–63. doi: 10.1515/jpem-2014-0384
75. Hattersley AT, Patel KA. Precision diabetes: learning from monogenic diabetes. Diabetologia (2017) 60:769–77. doi: 10.1007/s00125-017-4226-2
76. Ellard S, Bellanné-Chantelot C, Hattersley AT, European Molecular Genetics Quality Network (EMQN) MODY group. Best practice guidelines for the molecular genetic diagnosis of maturity-onset diabetes of the young. Diabetologia (2008) 51:546–53. doi: 10.1007/s00125-008-0942-y
77. Jang KM. Maturity-onset diabetes of the young: update and perspectives on diagnosis and treatment. Yeungnam Univ J Med (2020) 37:13–21. doi: 10.12701/yujm.2019.00409
78. Rutter GA, Pullen TJ, Hodson DJ, Martinez-Sanchez A. Pancreatic β-cell identity, glucose sensing and the control of insulin secretion. Biochem J (2015) 466:203–18. doi: 10.1042/BJ20141384
79. Galán M, Vincent O, Roncero I, Azriel S, Boix-Pallares P, Delgado-Alvarez E, et al. Effects of novel maturity-onset diabetes of the young (MODY)-associated mutations on glucokinase activity and protein stability. Biochem J (2006) 393:389–96. doi: 10.1042/BJ20051137
80. Sternisha SM, Miller BG. Molecular and cellular regulation of human glucokinase. Arch Biochem Biophys (2019) 663:199–213. doi: 10.1016/j.abb.2019.01.011
81. Ping F, Wang Z, Xiao X. Clinical and enzymatic phenotypes in congenital hyperinsulinemic hypoglycemia due to glucokinase-activating mutations: A report of two cases and a brief overview of the literature. J Diabetes Invest (2019) 10:1454–62. doi: 10.1111/jdi.13072
82. Ryffel GU. Mutations in the human genes encoding the transcription factors of the hepatocyte nuclear factor (HNF)1 and HNF4 families: functional and pathological consequences. J Mol Endocrinol (2001) 27:11–29. doi: 10.1677/jme.0.0270011
83. Mitchell SMS, Frayling TM. The role of transcription factors in maturity-onset diabetes of the young. Mol Genet Metab (2002) 77:35–43. doi: 10.1016/s1096-7192(02)00150-6
84. Yamagata K. Roles of HNF1α and HNF4α in pancreatic β-cells: lessons from a monogenic form of diabetes (MODY). Vitam Horm (2014) 95:407–23. doi: 10.1016/B978-0-12-800174-5.00016-8
85. Kyithar MP, Bonner C, Bacon S, Kilbride SM, Schmid J, Graf R, et al. Effects of hepatocyte nuclear factor-1A and -4A on pancreatic stone protein/regenerating protein and C-reactive protein gene expression: implications for maturity-onset diabetes of the young. J Transl Med (2013) 11:156. doi: 10.1186/1479-5876-11-156
86. Li J, Ning G, Duncan SA. Mammalian hepatocyte differentiation requires the transcription factor HNF-4alpha. Genes Dev (2000) 14:464–74. doi: 10.1101/gad.14.4.464
87. Lau HH, Ng NHJ, Loo LSW, Jasmen JB, Teo AKK. The molecular functions of hepatocyte nuclear factors - In and beyond the liver. J Hepatol (2018) 68:1033–48. doi: 10.1016/j.jhep.2017.11.026
88. Servitja J-M, Pignatelli M, Maestro MA, Cardalda C, Boj SF, Lozano J, et al. Hnf1alpha (MODY3) controls tissue-specific transcriptional programs and exerts opposed effects on cell growth in pancreatic islets and liver. Mol Cell Biol (2009) 29:2945–59. doi: 10.1128/MCB.01389-08
89. Chen WS, Manova K, Weinstein DC, Duncan SA, Plump AS, Prezioso VR, et al. Disruption of the HNF-4 gene, expressed in visceral endoderm, leads to cell death in embryonic ectoderm and impaired gastrulation of mouse embryos. Genes Dev (1994) 8:2466–77. doi: 10.1101/gad.8.20.2466
90. Wang H, Antinozzi PA, Hagenfeldt KA, Maechler P, Wollheim CB. Molecular targets of a human HNF1 alpha mutation responsible for pancreatic beta-cell dysfunction. EMBO J (2000) 19:4257–64. doi: 10.1093/emboj/19.16.4257
91. McDonald TJ, Ellard S. Maturity onset diabetes of the young: identification and diagnosis. Ann Clin Biochem (2013) 50:403–15. doi: 10.1177/0004563213483458
92. Mahajan A, Wessel J, Willems SM, Zhao W, Robertson NR, Chu AY, et al. Refining the accuracy of validated target identification through coding variant fine-mapping in type 2 diabetes. Nat Genet (2018) 50:559–71. doi: 10.1038/s41588-018-0084-1
93. Chimienti F, Devergnas S, Pattou F, Schuit F, Garcia-Cuenca R, Vandewalle B, et al. In vivo expression and functional characterization of the zinc transporter ZnT8 in glucose-induced insulin secretion. J Cell Sci (2006) 119:4199–206. doi: 10.1242/jcs.03164
94. Rutter GA, Chimienti F. SLC30A8 mutations in type 2 diabetes. Diabetologia (2015) 58:31–6. doi: 10.1007/s00125-014-3405-7
95. Diabetes Genetics Initiative of Broad Institute of Harvard and MIT, Lund University, and Novartis Institutes of BioMedical Research, Saxena R, Voight BF, Lyssenko V, Burtt NP, et al. Genome-wide association analysis identifies loci for type 2 diabetes and triglyceride levels. Science (2007) 316:1331–6. doi: 10.1126/science.1142358
96. Mitchell RK, Hu M, Chabosseau PL, Cane MC, Meur G, Bellomo EA, et al. Molecular Genetic Regulation of Slc30a8/ZnT8 Reveals a Positive Association With Glucose Tolerance. Mol Endocrinol Baltim Md (2016) 30:77–91. doi: 10.1210/me.2015-1227
97. Wijesekara N, Dai FF, Hardy AB, Giglou PR, Bhattacharjee A, Koshkin V, et al. Beta cell-specific Znt8 deletion in mice causes marked defects in insulin processing, crystallisation and secretion. Diabetologia (2010) 53:1656–68. doi: 10.1007/s00125-010-1733-9
98. Lemaire K, Ravier MA, Schraenen A, Creemers JWM, Van de Plas R, Granvik M, et al. Insulin crystallization depends on zinc transporter ZnT8 expression, but is not required for normal glucose homeostasis in mice. Proc Natl Acad Sci U S A (2009) 106:14872–7. doi: 10.1073/pnas.0906587106
99. Pound LD, Sarkar SA, Benninger RKP, Wang Y, Suwanichkul A, Shadoan MK, et al. Deletion of the mouse Slc30a8 gene encoding zinc transporter-8 results in impaired insulin secretion. Biochem J (2009) 421:371–6. doi: 10.1042/BJ20090530
100. Flannick J, Thorleifsson G, Beer NL, Jacobs SBR, Grarup N, Burtt NP, et al. Loss-of-function mutations in SLC30A8 protect against type 2 diabetes. Nat Genet (2014) 46:357–63. doi: 10.1038/ng.2915
102. Dwivedi OP, Lehtovirta M, Hastoy B, Chandra V, Krentz NAJ, Kleiner S, et al. Loss of ZnT8 function protects against diabetes by enhanced insulin secretion. Nat Genet (2019) 51:1596–606. doi: 10.1038/s41588-019-0513-9
103. Thomsen SK, Raimondo A, Hastoy B, Sengupta S, Dai X-Q, Bautista A, et al. Type 2 diabetes risk alleles in PAM impact insulin release from human pancreatic β-cells. Nat Genet (2018) 50:1122–31. doi: 10.1038/s41588-018-0173-1
104. Huyghe JR, Jackson AU, Fogarty MP, Buchkovich ML, Stančáková A, Stringham HM, et al. Exome array analysis identifies new loci and low-frequency variants influencing insulin processing and secretion. Nat Genet (2013) 45:197–201. doi: 10.1038/ng.2507
105. Steinthorsdottir V, Thorleifsson G, Sulem P, Helgason H, Grarup N, Sigurdsson A, et al. Identification of low-frequency and rare sequence variants associated with elevated or reduced risk of type 2 diabetes. Nat Genet (2014) 46:294–8. doi: 10.1038/ng.2882
106. Krentz NAJ, Gloyn AL. Insights into pancreatic islet cell dysfunction from type 2 diabetes mellitus genetics. Nat Rev Endocrinol (2020) 16:202–12. doi: 10.1038/s41574-020-0325-0
107. Dupuis J, Langenberg C, Prokopenko I, Saxena R, Soranzo N, Jackson AU, et al. New genetic loci implicated in fasting glucose homeostasis and their impact on type 2 diabetes risk. Nat Genet (2010) 42:105–16. doi: 10.1038/ng.520
108. Saxena R, Hivert M-F, Langenberg C, Tanaka T, Pankow JS, Vollenweider P, et al. Genetic variation in GIPR influences the glucose and insulin responses to an oral glucose challenge. Nat Genet (2010) 42:142–8. doi: 10.1038/ng.521
109. Wagner R, Dudziak K, Herzberg-Schäfer SA, Machicao F, Stefan N, Staiger H, et al. Glucose-raising genetic variants in MADD and ADCY5 impair conversion of proinsulin to insulin. PLoS One (2011) 6:e23639. doi: 10.1371/journal.pone.0023639
110. Hodson DJ, Mitchell RK, Marselli L, Pullen TJ, Gimeno Brias S, Semplici F, et al. ADCY5 couples glucose to insulin secretion in human islets. Diabetes (2014) 63:3009–21. doi: 10.2337/db13-1607
111. Schäfer SA, Tschritter O, Machicao F, Thamer C, Stefan N, Gallwitz B, et al. Impaired glucagon-like peptide-1-induced insulin secretion in carriers of transcription factor 7-like 2 (TCF7L2) gene polymorphisms. Diabetologia (2007) 50:2443–50. doi: 10.1007/s00125-007-0753-6
112. Loder MK, da Silva Xavier G, McDonald A, Rutter GA. TCF7L2 controls insulin gene expression and insulin secretion in mature pancreatic beta-cells. Biochem Soc Trans (2008) 36:357–9. doi: 10.1042/BST0360357
113. da Silva Xavier G, Mondragon A, Sun G, Chen L, McGinty JA, French PM, et al. Abnormal glucose tolerance and insulin secretion in pancreas-specific Tcf7l2-null mice. Diabetologia (2012) 55:2667–76. doi: 10.1007/s00125-012-2600-7
114. Mitchell RK, Mondragon A, Chen L, Mcginty JA, French PM, Ferrer J, et al. Selective disruption of Tcf7l2 in the pancreatic β cell impairs secretory function and lowers β cell mass. Hum Mol Genet (2015) 24:1390–9. doi: 10.1093/hmg/ddu553
115. Olayioye MA, Vehring S, Müller P, Herrmann A, Schiller J, Thiele C, et al. StarD10, a START domain protein overexpressed in breast cancer, functions as a phospholipid transfer protein. J Biol Chem (2005) 280:27436–42. doi: 10.1074/jbc.M413330200
116. Carrat GR, Hu M, Nguyen-Tu M-S, Chabosseau P, Gaulton KJ, van de Bunt M, et al. Decreased STARD10 Expression Is Associated with Defective Insulin Secretion in Humans and Mice. Am J Hum Genet (2017) 100:238–56. doi: 10.1016/j.ajhg.2017.01.011
117. Hu M, Cebola I, Carrat G, Jiang S, Nawaz S, Khamis A, et al. Chromatin 3D interaction analysis of the STARD10 locus unveils FCHSD2 as a new regulator of insulin secretion. bioRxiv (2020). doi: 10.1101/2020.03.31.017707
118. Carrat GR, Haythorne E, Tomas A, Haataja L, Müller A, Arvan P, et al. The type 2 diabetes gene product STARD10 is a phosphoinositide-binding protein that controls insulin secretory granule biogenesis. Mol Metab (2020), 40:101015. doi: 10.1016/j.molmet.2020.101015
119. Strawbridge RJ, Dupuis J, Prokopenko I, Barker A, Ahlqvist E, Rybin D, et al. Genome-wide association identifies nine common variants associated with fasting proinsulin levels and provides new insights into the pathophysiology of type 2 diabetes. Diabetes (2011) 60:2624–34. doi: 10.2337/db11-0415
120. Grarup N, Overvad M, Sparsø T, Witte DR, Pisinger C, Jørgensen T, et al. The diabetogenic VPS13C/C2CD4A/C2CD4B rs7172432 variant impairs glucose-stimulated insulin response in 5,722 non-diabetic Danish individuals. Diabetologia (2011) 54:789–94. doi: 10.1007/s00125-010-2031-2
121. Kumar N, Leonzino M, Hancock-Cerutti W, Horenkamp FA, Li P, Lees JA, et al. VPS13A and VPS13C are lipid transport proteins differentially localized at ER contact sites. J Cell Biol (2018) 217:3625–39. doi: 10.1083/jcb.201807019
122. Mehta ZB, Fine N, Pullen TJ, Cane MC, Hu M, Chabosseau P, et al. Changes in the expression of the type 2 diabetes-associated gene VPS13C in the β-cell are associated with glucose intolerance in humans and mice. Am J Physiol Endocrinol Metab (2016) 311:E488–507. doi: 10.1152/ajpendo.00074.2016
123. Kuo T, Kraakman MJ, Damle M, Gill R, Lazar MA, Accili D. Identification of C2CD4A as a human diabetes susceptibility gene with a role in β cell insulin secretion. Proc Natl Acad Sci U S A (2019) 116:20033–42. doi: 10.1073/pnas.1904311116
124. Gharavy SNM, Owen B, Millership SJ, Chabosseau P, Pizza G, Martinez-Sanchez A, et al. Sexually dimorphic roles for the type 2 diabetes-associated C2cd4b gene in murine glucose homeostasis. bioRxiv (2020). doi: 10.1101/2020.05.18.099200
125. Gannon M, Shiota C, Postic C, Wright CV, Magnuson M. Analysis of the Cre-mediated recombination driven by rat insulin promoter in embryonic and adult mouse pancreas. Genes N Y N 2000 (2000) 26:139–42. doi: 10.1002/(sici)1526-968x(200002)26:2<139::aid-gene12>3.0.co;2-7
126. Lee TI, Young RA. Transcriptional regulation and its misregulation in disease. Cell (2013) 152:1237–51. doi: 10.1016/j.cell.2013.02.014
127. Sur I, Taipale J. The role of enhancers in cancer. Nat Rev Cancer (2016) 16:483–93. doi: 10.1038/nrc.2016.62
128. Misteli T. Higher-order genome organization in human disease. Cold Spring Harb Perspect Biol (2010) 2(8):a000794. doi: 10.1101/cshperspect.a000794
129. Lawlor N, Stitzel ML. (Epi)genomic heterogeneity of pancreatic islet function and failure in type 2 diabetes. Mol Metab (2019) 27S:S15–24. doi: 10.1016/j.molmet.2019.06.002
130. Roman TS, Cannon ME, Vadlamudi S, Buchkovich ML, Wolford BN, Welch RP, et al. A Type 2 Diabetes-Associated Functional Regulatory Variant in a Pancreatic Islet Enhancer at the ADCY5 Locus. Diabetes (2017) 66:2521–30. doi: 10.2337/db17-0464
131. Kycia I, Wolford BN, Huyghe JR, Fuchsberger C, Vadlamudi S, Kursawe R, et al. A Common Type 2 Diabetes Risk Variant Potentiates Activity of an Evolutionarily Conserved Islet Stretch Enhancer and Increases C2CD4A and C2CD4B Expression. Am J Hum Genet (2018) 102:620–35. doi: 10.1016/j.ajhg.2018.02.020
132. Varshney A, Scott LJ, Welch RP, Erdos MR, Chines PS, Narisu N, et al. Genetic regulatory signatures underlying islet gene expression and type 2 diabetes. Proc Natl Acad Sci U S A (2017) 114:2301–6. doi: 10.1073/pnas.1621192114
133. Varshney A, VanRenterghem H, Orchard P, Boyle AP, Stitzel ML, Ucar D, et al. Cell Specificity of Human Regulatory Annotations and Their Genetic Effects on Gene Expression. Genetics (2019) 211:549–62. doi: 10.1534/genetics.118.301525
134. Fernández-Tajes J, Gaulton KJ, van de Bunt M, Torres J, Thurner M, Mahajan A, et al. Developing a network view of type 2 diabetes risk pathways through integration of genetic, genomic and functional data. Genome Med (2019) 11:19. doi: 10.1186/s13073-019-0628-8
135. Tak YG, Farnham PJ. Making sense of GWAS: using epigenomics and genome engineering to understand the functional relevance of SNPs in non-coding regions of the human genome. Epigenet Chromatin (2015) 8:57. doi: 10.1186/s13072-015-0050-4
136. Mattis KK, Gloyn AL. From Genetic Association to Molecular Mechanisms for Islet-cell Dysfunction in Type 2 Diabetes. J Mol Biol (2020) 432:1551–78. doi: 10.1016/j.jmb.2019.12.045
137. Rouet P, Smih F, Jasin M. Expression of a site-specific endonuclease stimulates homologous recombination in mammalian cells. Proc Natl Acad Sci U S A (1994) 91:6064–8. doi: 10.1073/pnas.91.13.6064
138. Smih F, Rouet P, Romanienko PJ, Jasin M. Double-strand breaks at the target locus stimulate gene targeting in embryonic stem cells. Nucleic Acids Res (1995) 23:5012–9. doi: 10.1093/nar/23.24.5012
139. Choulika A, Perrin A, Dujon B, Nicolas JF. The yeast I-Sce I meganuclease induces site-directed chromosomal recombination in mammalian cells. C R Acad Sci III (1994) 317:1013–9.
140. Cohen-Tannoudji M, Robine S, Choulika A, Pinto D, El Marjou F, Babinet C, et al. I-SceI-induced gene replacement at a natural locus in embryonic stem cells. Mol Cell Biol (1998) 18:1444–8. doi: 10.1128/mcb.18.3.1444
141. Epinat J-C, Arnould S, Chames P, Rochaix P, Desfontaines D, Puzin C, et al. A novel engineered meganuclease induces homologous recombination in yeast and mammalian cells. Nucleic Acids Res (2003) 31:2952–62. doi: 10.1093/nar/gkg375
142. Pâques F, Duchateau P. Meganucleases and DNA double-strand break-induced recombination: perspectives for gene therapy. Curr Gene Ther (2007) 7:49–66. doi: 10.2174/156652307779940216
143. Kim YG, Cha J, Chandrasegaran S. Hybrid restriction enzymes: zinc finger fusions to Fok I cleavage domain. Proc Natl Acad Sci U S A (1996) 93:1156–60. doi: 10.1073/pnas.93.3.1156
144. Miller JC, Tan S, Qiao G, Barlow KA, Wang J, Xia DF, et al. A TALE nuclease architecture for efficient genome editing. Nat Biotechnol (2011) 29:143–8. doi: 10.1038/nbt.1755
145. Sanjana NE, Cong L, Zhou Y, Cunniff MM, Feng G, Zhang F. A transcription activator-like effector toolbox for genome engineering. Nat Protoc (2012) 7:171–92. doi: 10.1038/nprot.2011.431
146. Hockemeyer D, Soldner F, Beard C, Gao Q, Mitalipova M, DeKelver RC, et al. Efficient targeting of expressed and silent genes in human ESCs and iPSCs using zinc-finger nucleases. Nat Biotechnol (2009) 27:851–7. doi: 10.1038/nbt.1562
147. Zou J, Maeder ML, Mali P, Pruett-Miller SM, Thibodeau-Beganny S, Chou B-K, et al. Gene targeting of a disease-related gene in human induced pluripotent stem and embryonic stem cells. Cell Stem Cell (2009) 5:97–110. doi: 10.1016/j.stem.2009.05.023
148. Urnov FD, Miller JC, Lee Y-L, Beausejour CM, Rock JM, Augustus S, et al. Highly efficient endogenous human gene correction using designed zinc-finger nucleases. Nature (2005) 435:646–51. doi: 10.1038/nature03556
149. Li M, Dean ED, Zhao L, Nicholson WE, Powers AC, Chen W. Glucagon receptor inactivation leads to α-cell hyperplasia in zebrafish. J Endocrinol (2015) 227:93–103. doi: 10.1530/JOE-15-0284
150. Zhou X, Zhang R, Zou Z, Shen X, Xie T, Xu C, et al. Hypoglycaemic effects of glimepiride in sulfonylurea receptor 1 deficient rat. Br J Pharmacol (2019) 176:478–90. doi: 10.1111/bph.14553
151. Zhu Z, Li QV, Lee K, Rosen BP, González F, Soh C-L, et al. Genome Editing of Lineage Determinants in Human Pluripotent Stem Cells Reveals Mechanisms of Pancreatic Development and Diabetes. Cell Stem Cell (2016) 18:755–68. doi: 10.1016/j.stem.2016.03.015
152. Bitinaite J, Wah DA, Aggarwal AK, Schildkraut I. FokI dimerization is required for DNA cleavage. Proc Natl Acad Sci (1998) 95:10570–5. doi: 10.1073/pnas.95.18.10570
153. Doudna JA, Charpentier E. Genome editing. The new frontier of genome engineering with CRISPR-Cas9. Science (2014) 346:1258096. doi: 10.1126/science.1258096
154. Wright AV, Nuñez JK, Doudna JA. Biology and Applications of CRISPR Systems: Harnessing Nature’s Toolbox for Genome Engineering. Cell (2016) 164:29–44. doi: 10.1016/j.cell.2015.12.035
155. Gaj T, Gersbach CA, Barbas CF. ZFN, TALEN, and CRISPR/Cas-based methods for genome engineering. Trends Biotechnol (2013) 31:397–405. doi: 10.1016/j.tibtech.2013.04.004
156. Wang H, Yang H, Shivalila CS, Dawlaty MM, Cheng AW, Zhang F, et al. One-step generation of mice carrying mutations in multiple genes by CRISPR/Cas-mediated genome engineering. Cell (2013) 153:910–8. doi: 10.1016/j.cell.2013.04.025
157. Swiech L, Heidenreich M, Banerjee A, Habib N, Li Y, Trombetta J, et al. In vivo interrogation of gene function in the mammalian brain using CRISPR-Cas9. Nat Biotechnol (2015) 33:102–6. doi: 10.1038/nbt.3055
158. Zeng H, Guo M, Zhou T, Tan L, Chong CN, Zhang T, et al. An Isogenic Human ESC Platform for Functional Evaluation of Genome-wide-Association-Study-Identified Diabetes Genes and Drug Discovery. Cell Stem Cell (2016) 19:326–40. doi: 10.1016/j.stem.2016.07.002
159. Zhou X, Nair GG, Russ HA, Belair CD, Li M-L, Shveygert M, et al. LIN28B Impairs the Transition of hESC-Derived β Cells from the Juvenile to Adult State. Stem Cell Rep (2020) 14:9–20. doi: 10.1016/j.stemcr.2019.11.009
160. Roh J-I, Lee J, Park SU, Kang Y-S, Lee J, Oh A-R, et al. CRISPR-Cas9-mediated generation of obese and diabetic mouse models. Exp Anim (2018) 67:229–37. doi: 10.1538/expanim.17-0123
161. Cho B, Kim SJ, Lee E-J, Ahn SM, Lee JS, Ji D-Y, et al. Generation of insulin-deficient piglets by disrupting INS gene using CRISPR/Cas9 system. Transgenic Res (2018) 27:289–300. doi: 10.1007/s11248-018-0074-1
162. van Overbeek M, Capurso D, Carter MM, Thompson MS, Frias E, Russ C, et al. DNA Repair Profiling Reveals Nonrandom Outcomes at Cas9-Mediated Breaks. Mol Cell (2016) 63:633–46. doi: 10.1016/j.molcel.2016.06.037
163. Shen MW, Arbab M, Hsu JY, Worstell D, Culbertson SJ, Krabbe O, et al. Predictable and precise template-free CRISPR editing of pathogenic variants. Nature (2018) 563:646–51. doi: 10.1038/s41586-018-0686-x
164. Román-Rodríguez FJ, Ugalde L, Álvarez L, Díez B, Ramírez MJ, Risueño C, et al. NHEJ-Mediated Repair of CRISPR-Cas9-Induced DNA Breaks Efficiently Corrects Mutations in HSPCs from Patients with Fanconi Anemia. Cell Stem Cell (2019) 25:607–21. doi: 10.1016/j.stem.2019.08.016
165. Nambiar TS, Billon P, Diedenhofen G, Hayward SB, Taglialatela A, Cai K, et al. Stimulation of CRISPR-mediated homology-directed repair by an engineered RAD18 variant. Nat Commun (2019) 10:3395. doi: 10.1038/s41467-019-11105-z
166. Balboa D, Saarimäki-Vire J, Otonkoski T. Concise Review: Human Pluripotent Stem Cells for the Modeling of Pancreatic β-Cell Pathology. Stem Cells Dayt Ohio (2019) 37:33–41. doi: 10.1002/stem.2913
167. Paquet D, Kwart D, Chen A, Sproul A, Jacob S, Teo S, et al. Efficient introduction of specific homozygous and heterozygous mutations using CRISPR/Cas9. Nature (2016) 533:125–9. doi: 10.1038/nature17664
168. Chu VT, Weber T, Wefers B, Wurst W, Sander S, Rajewsky K, et al. Increasing the efficiency of homology-directed repair for CRISPR-Cas9-induced precise gene editing in mammalian cells. Nat Biotechnol (2015) 33:543–8. doi: 10.1038/nbt.3198
169. Maruyama T, Dougan SK, Truttmann MC, Bilate AM, Ingram JR, Ploegh HL. Increasing the efficiency of precise genome editing with CRISPR-Cas9 by inhibition of nonhomologous end joining. Nat Biotechnol (2015) 33:538–42. doi: 10.1038/nbt.3190
170. Richardson CD, Ray GJ, DeWitt MA, Curie GL, Corn JE. Enhancing homology-directed genome editing by catalytically active and inactive CRISPR-Cas9 using asymmetric donor DNA. Nat Biotechnol (2016) 34:339–44. doi: 10.1038/nbt.3481
171. Chen S, Sanjana NE, Zheng K, Shalem O, Lee K, Shi X, et al. Genome-wide CRISPR screen in a mouse model of tumor growth and metastasis. Cell (2015) 160:1246–60. doi: 10.1016/j.cell.2015.02.038
172. Fang Z, Weng C, Li H, Tao R, Mai W, Liu X, et al. Single-Cell Heterogeneity Analysis and CRISPR Screen Identify Key β-Cell-Specific Disease Genes. Cell Rep (2019) 26:3132–44.e7. doi: 10.1016/j.celrep.2019.02.043
173. Cai EP, Ishikawa Y, Zhang W, Leite NC, Li J, Hou S, et al. Genome-scale in vivo CRISPR screen identifies RNLS as a target for beta cell protection in type 1 diabetes. Nat Metab (2020) 2(9):934–45. doi: 10.1038/s42255-020-0254-1
174. Kampmann M, Horlbeck MA, Chen Y, Tsai JC, Bassik MC, Gilbert LA, et al. Next-generation libraries for robust RNA interference-based genome-wide screens. Proc Natl Acad Sci U S A (2015) 112:E3384–3391. doi: 10.1073/pnas.1508821112
175. Parnas O, Jovanovic M, Eisenhaure TM, Herbst RH, Dixit A, Ye CJ, et al. A Genome-wide CRISPR Screen in Primary Immune Cells to Dissect Regulatory Networks. Cell (2015) 162:675–86. doi: 10.1016/j.cell.2015.06.059
176. Kampmann M, Bassik MC, Weissman JS. Functional genomics platform for pooled screening and generation of mammalian genetic interaction maps. Nat Protoc (2014) 9:1825–47. doi: 10.1038/nprot.2014.103
177. Sanjana NE, Wright J, Zheng K, Shalem O, Fontanillas P, Joung J, et al. High-resolution interrogation of functional elements in the noncoding genome. Science (2016) 353:1545–9. doi: 10.1126/science.aaf7613
178. Joung J, Engreitz JM, Konermann S, Abudayyeh OO, Verdine VK, Aguet F, et al. Genome-scale activation screen identifies a lncRNA locus regulating a gene neighbourhood. Nature (2017) 548:343–6. doi: 10.1038/nature23451
179. Trevino AE, Zhang F. Genome editing using Cas9 nickases. Methods Enzymol (2014) 546:161–74. doi: 10.1016/B978-0-12-801185-0.00008-8
180. Rees HA, Yeh W-H, Liu DR. Development of hRad51-Cas9 nickase fusions that mediate HDR without double-stranded breaks. Nat Commun (2019) 10:2212. doi: 10.1038/s41467-019-09983-4
181. Sternberg SH, Doudna JA. Expanding the Biologist’s Toolkit with CRISPR-Cas9. Mol Cell (2015) 58:568–74. doi: 10.1016/j.molcel.2015.02.032
182. Chen B, Gilbert LA, Cimini BA, Schnitzbauer J, Zhang W, Li G-W, et al. Dynamic imaging of genomic loci in living human cells by an optimized CRISPR/Cas system. Cell (2013) 155:1479–91. doi: 10.1016/j.cell.2013.12.001
183. Wang S, Su J-H, Zhang F, Zhuang X. An RNA-aptamer-based two-color CRISPR labeling system. Sci Rep (2016) 6:26857. doi: 10.1038/srep26857
184. Deng W, Shi X, Tjian R, Lionnet T, Singer RH. CASFISH: CRISPR/Cas9-mediated in situ labeling of genomic loci in fixed cells. Proc Natl Acad Sci U S A (2015) 112:11870–5. doi: 10.1073/pnas.1515692112
185. Gilbert LA, Horlbeck MA, Adamson B, Villalta JE, Chen Y, Whitehead EH, et al. Genome-Scale CRISPR-Mediated Control of Gene Repression and Activation. Cell (2014) 159:647–61. doi: 10.1016/j.cell.2014.09.029
186. Konermann S, Brigham MD, Trevino AE, Joung J, Abudayyeh OO, Barcena C, et al. Genome-scale transcriptional activation by an engineered CRISPR-Cas9 complex. Nature (2015) 517:583–8. doi: 10.1038/nature14136
187. Kearns NA, Genga RMJ, Enuameh MS, Garber M, Wolfe SA, Maehr R. Cas9 effector-mediated regulation of transcription and differentiation in human pluripotent stem cells. Dev Camb Engl (2014) 141:219–23. doi: 10.1242/dev.103341
188. Parsi KM, Hennessy E, Kearns N, Maehr R. Using an Inducible CRISPR-dCas9-KRAB Effector System to Dissect Transcriptional Regulation in Human Embryonic Stem Cells. Methods Mol Biol Clifton NJ (2017) 1507:221–33. doi: 10.1007/978-1-4939-6518-2_16
189. Fujita T, Fujii H. Efficient isolation of specific genomic regions and identification of associated proteins by engineered DNA-binding molecule-mediated chromatin immunoprecipitation (enChIP) using CRISPR. Biochem Biophys Res Commun (2013) 439:132–6. doi: 10.1016/j.bbrc.2013.08.013
190. Kleinstiver BP, Pattanayak V, Prew MS, Tsai SQ, Nguyen NT, Zheng Z, et al. High-fidelity CRISPR-Cas9 nucleases with no detectable genome-wide off-target effects. Nature (2016) 529:490–5. doi: 10.1038/nature16526
191. Kleinstiver BP, Prew MS, Tsai SQ, Topkar VV, Nguyen NT, Zheng Z, et al. Engineered CRISPR-Cas9 nucleases with altered PAM specificities. Nature (2015) 523:481–5. doi: 10.1038/nature14592
192. Nishimasu H, Shi X, Ishiguro S, Gao L, Hirano S, Okazaki S, et al. Engineered CRISPR-Cas9 nuclease with expanded targeting space. Science (2018) 361:1259–62. doi: 10.1126/science.aas9129
193. Müller M, Lee CM, Gasiunas G, Davis TH, Cradick TJ, Siksnys V, et al. Streptococcus thermophilus CRISPR-Cas9 Systems Enable Specific Editing of the Human Genome. Mol Ther J Am Soc Gene Ther (2016) 24:636–44. doi: 10.1038/mt.2015.218
194. Strecker J, Jones S, Koopal B, Schmid-Burgk J, Zetsche B, Gao L, et al. Engineering of CRISPR-Cas12b for human genome editing. Nat Commun (2019) 10:212. doi: 10.1038/s41467-018-08224-4
195. Zetsche B, Heidenreich M, Mohanraju P, Fedorova I, Kneppers J, DeGennaro EM, et al. Multiplex gene editing by CRISPR-Cpf1 using a single crRNA array. Nat Biotechnol (2017) 35:31–4. doi: 10.1038/nbt.3737
196. Rahdar M, McMahon MA, Prakash TP, Swayze EE, Bennett CF, Cleveland DW. Synthetic CRISPR RNA-Cas9-guided genome editing in human cells. Proc Natl Acad Sci U S A (2015) 112:E7110–7117. doi: 10.1073/pnas.1520883112
197. Hendel A, Bak RO, Clark JT, Kennedy AB, Ryan DE, Roy S, et al. Chemically modified guide RNAs enhance CRISPR-Cas genome editing in human primary cells. Nat Biotechnol (2015) 33:985–9. doi: 10.1038/nbt.3290
198. Yin H, Song C-Q, Suresh S, Wu Q, Walsh S, Rhym LH, et al. Structure-guided chemical modification of guide RNA enables potent non-viral in vivo genome editing. Nat Biotechnol (2017) 35:1179–87. doi: 10.1038/nbt.4005
199. McMahon MA, Prakash TP, Cleveland DW, Bennett CF, Rahdar M. Chemically Modified Cpf1-CRISPR RNAs Mediate Efficient Genome Editing in Mammalian Cells. Mol Ther J Am Soc Gene Ther (2018) 26:1228–40. doi: 10.1016/j.ymthe.2018.02.031
200. Abudayyeh OO, Gootenberg JS, Konermann S, Joung J, Slaymaker IM, Cox DBT, et al. C2c2 is a single-component programmable RNA-guided RNA-targeting CRISPR effector. Science (2016) 353:aaf5573. doi: 10.1126/science.aaf5573
201. East-Seletsky A, O’Connell MR, Knight SC, Burstein D, Cate JHD, Tjian R, et al. Two distinct RNase activities of CRISPR-C2c2 enable guide-RNA processing and RNA detection. Nature (2016) 538:270–3. doi: 10.1038/nature19802
202. Konermann S, Lotfy P, Brideau NJ, Oki J, Shokhirev MN, Hsu PD. Transcriptome engineering with RNA-targeting Type VI-D CRISPR effectors. Cell (2018) 173:665–76.e14. doi: 10.1016/j.cell.2018.02.033
203. Chen JS, Ma E, Harrington LB, Da Costa M, Tian X, Palefsky JM, et al. CRISPR-Cas12a target binding unleashes indiscriminate single-stranded DNase activity. Science (2018) 360:436–9. doi: 10.1126/science.aar6245
204. Kosicki M, Tomberg K, Bradley A. Repair of double-strand breaks induced by CRISPR-Cas9 leads to large deletions and complex rearrangements. Nat Biotechnol (2018) 36:765–71. doi: 10.1038/nbt.4192
205. Ihry RJ, Worringer KA, Salick MR, Frias E, Ho D, Theriault K, et al. p53 inhibits CRISPR-Cas9 engineering in human pluripotent stem cells. Nat Med (2018) 24:939–46. doi: 10.1038/s41591-018-0050-6
206. Haapaniemi E, Botla S, Persson J, Schmierer B, Taipale J. CRISPR-Cas9 genome editing induces a p53-mediated DNA damage response. Nat Med (2018) 24:927–30. doi: 10.1038/s41591-018-0049-z
207. Wagner DL, Amini L, Wendering DJ, Burkhardt L-M, Akyüz L, Reinke P, et al. High prevalence of Streptococcus pyogenes Cas9-reactive T cells within the adult human population. Nat Med (2019) 25:242–8. doi: 10.1038/s41591-018-0204-6
208. Charlesworth CT, Deshpande PS, Dever DP, Camarena J, Lemgart VT, Cromer MK, et al. Identification of preexisting adaptive immunity to Cas9 proteins in humans. Nat Med (2019) 25:249–54. doi: 10.1038/s41591-018-0326-x
209. Rees HA, Liu DR. Base editing: precision chemistry on the genome and transcriptome of living cells. Nat Rev Genet (2018) 19:770–88. doi: 10.1038/s41576-018-0059-1
210. Bishop KN, Holmes RK, Sheehy AM, Davidson NO, Cho S-J, Malim MH. Cytidine deamination of retroviral DNA by diverse APOBEC proteins. Curr Biol CB (2004) 14:1392–6. doi: 10.1016/j.cub.2004.06.057
211. Janahi EM, McGarvey MJ. The inhibition of hepatitis B virus by APOBEC cytidine deaminases. J Viral Hepat (2013) 20:821–8. doi: 10.1111/jvh.12192
212. Shimatani Z, Kashojiya S, Takayama M, Terada R, Arazoe T, Ishii H, et al. Targeted base editing in rice and tomato using a CRISPR-Cas9 cytidine deaminase fusion. Nat Biotechnol (2017) 35:441–3. doi: 10.1038/nbt.3833
213. Yang B, Li X, Lei L, Chen J. APOBEC: From mutator to editor. J Genet Genomics Yi Chuan Xue Bao (2017) 44:423–37. doi: 10.1016/j.jgg.2017.04.009
214. Eid A, Alshareef S, Mahfouz MM. CRISPR base editors: genome editing without double-stranded breaks. Biochem J (2018) 475:1955–64. doi: 10.1042/BCJ20170793
215. Mol CD, Arvai AS, Sanderson RJ, Slupphaug G, Kavli B, Krokan HE, et al. Crystal structure of human uracil-DNA glycosylase in complex with a protein inhibitor: protein mimicry of DNA. Cell (1995) 82:701–8. doi: 10.1016/0092-8674(95)90467-0
216. Hoopes JI, Hughes AL, Hobson LA, Cortez LM, Brown AJ, Roberts SA. Avoidance of APOBEC3B-induced mutation by error-free lesion bypass. Nucleic Acids Res (2017) 45:5243–54. doi: 10.1093/nar/gkx169
217. Staszewski O, Baker RE, Ucher AJ, Martier R, Stavnezer J, Guikema JEJ. Activation-induced cytidine deaminase induces reproducible DNA breaks at many non-Ig Loci in activated B cells. Mol Cell (2011) 41:232–42. doi: 10.1016/j.molcel.2011.01.007
218. Li X, Wang Y, Liu Y, Yang B, Wang X, Wei J, et al. Base editing with a Cpf1-cytidine deaminase fusion. Nat Biotechnol (2018) 36:324–7. doi: 10.1038/nbt.4102
219. Yasui M, Suenaga E, Koyama N, Masutani C, Hanaoka F, Gruz P, et al. Miscoding properties of 2’-deoxyinosine, a nitric oxide-derived DNA Adduct, during translesion synthesis catalyzed by human DNA polymerases. J Mol Biol (2008) 377:1015–23. doi: 10.1016/j.jmb.2008.01.033
220. Ma Y, Zhang J, Yin W, Zhang Z, Song Y, Chang X. Targeted AID-mediated mutagenesis (TAM) enables efficient genomic diversification in mammalian cells. Nat Methods (2016) 13:1029–35. doi: 10.1038/nmeth.4027
221. Hess GT, Tycko J, Yao D, Bassik MC. Methods and Applications of CRISPR-Mediated Base Editing in Eukaryotic Genomes. Mol Cell (2017) 68:26–43. doi: 10.1016/j.molcel.2017.09.029
222. Källman AM, Sahlin M, Ohman M. ADAR2 A–>I editing: site selectivity and editing efficiency are separate events. Nucleic Acids Res (2003) 31:4874–81. doi: 10.1093/nar/gkg681
223. Landrum MJ, Lee JM, Benson M, Brown G, Chao C, Chitipiralla S, et al. ClinVar: public archive of interpretations of clinically relevant variants. Nucleic Acids Res (2016) 44:D862–868. doi: 10.1093/nar/gkv1222
224. Komor AC, Zhao KT, Packer MS, Gaudelli NM, Waterbury AL, Koblan LW, et al. Improved base excision repair inhibition and bacteriophage Mu Gam protein yields C:G-to-T:A base editors with higher efficiency and product purity. Sci Adv (2017) 3:eaao4774. doi: 10.1126/sciadv.aao4774
225. Kim YB, Komor AC, Levy JM, Packer MS, Zhao KT, Liu DR. Increasing the genome-targeting scope and precision of base editing with engineered Cas9-cytidine deaminase fusions. Nat Biotechnol (2017) 35:371–6. doi: 10.1038/nbt.3803
226. Hart NJ, Powers AC. Use of human islets to understand islet biology and diabetes: progress, challenges and suggestions. Diabetologia (2019) 62:212–22. doi: 10.1007/s00125-018-4772-2
227. Ravassard P, Hazhouz Y, Pechberty S, Bricout-Neveu E, Armanet M, Czernichow P, et al. A genetically engineered human pancreatic β cell line exhibiting glucose-inducible insulin secretion. J Clin Invest (2011) 121:3589–97. doi: 10.1172/JCI58447
228. Scharfmann R, Pechberty S, Hazhouz Y, von Bülow M, Bricout-Neveu E, Grenier-Godard M, et al. Development of a conditionally immortalized human pancreatic β cell line. J Clin Invest (2014) 124:2087–98. doi: 10.1172/JCI72674
229. Benazra M, Lecomte M-J, Colace C, Müller A, Machado C, Pechberty S, et al. A human beta cell line with drug inducible excision of immortalizing transgenes. Mol Metab (2015) 4:916–25. doi: 10.1016/j.molmet.2015.09.008
230. Pagliuca FW, Millman JR, Gürtler M, Segel M, Van Dervort A, Ryu JH, et al. Generation of functional human pancreatic β cells in vitro. Cell (2014) 159:428–39. doi: 10.1016/j.cell.2014.09.040
231. Rezania A, Bruin JE, Arora P, Rubin A, Batushansky I, Asadi A, et al. Reversal of diabetes with insulin-producing cells derived in vitro from human pluripotent stem cells. Nat Biotechnol (2014) 32:1121–33. doi: 10.1038/nbt.3033
232. Chen L, Vasoya RP, Toke NH, Parthasarathy A, Luo S, Chiles E, et al. HNF4 Regulates Fatty Acid Oxidation and Is Required for Renewal of Intestinal Stem Cells in Mice. Gastroenterology (2020) 158:985–999.e9. doi: 10.1053/j.gastro.2019.11.031
233. Song Y, Sui T, Zhang Y, Wang Y, Chen M, Deng J, et al. Genetic deletion of a short fragment of glucokinase in rabbit by CRISPR/Cas9 leading to hyperglycemia and other typical features seen in MODY-2. Cell Mol Life Sci CMLS (2019). doi: 10.1007/s00018-019-03354-4
234. Basiri M, Behmanesh M, Tahamtani Y, Khalooghi K, Moradmand A, Baharvand H. The Convenience of Single Homology Arm Donor DNA and CRISPR/Cas9-Nickase for Targeted Insertion of Long DNA Fragment. Cell J Yakhteh (2017) 18:532–9. doi: 10.22074%2Fcellj.2016.4719"10.22074/cellj.2016.4719
235. Lee Y, Choi HY, Kwon A, Park H, Park M-H, Kim J-W, et al. Generation of a PDX1-EGFP reporter human induced pluripotent stem cell line, KSCBi005-A-3, using the CRISPR/Cas9 system. Stem Cell Res (2019) 41:101632. doi: 10.1016/j.scr.2019.101632
236. Teo AKK, Windmueller R, Johansson BB, Dirice E, Njolstad PR, Tjora E, et al. Derivation of human induced pluripotent stem cells from patients with maturity onset diabetes of the young. J Biol Chem (2013) 288:5353–6. doi: 10.1074/jbc.C112.428979
237. Teo AKK, Lau HH, Valdez IA, Dirice E, Tjora E, Raeder H, et al. Early Developmental Perturbations in a Human Stem Cell Model of MODY5/HNF1B Pancreatic Hypoplasia. Stem Cell Rep (2016) 6:357–67. doi: 10.1016/j.stemcr.2016.01.007
238. Yabe SG, Iwasaki N, Yasuda K, Hamazaki TS, Konno M, Fukuda S, et al. Establishment of maturity-onset diabetes of the young-induced pluripotent stem cells from a Japanese patient. J Diabetes Invest (2015) 6:543–7. doi: 10.1111/jdi.12334
239. Stepniewski J, Kachamakova-Trojanowska N, Ogrocki D, Szopa M, Matlok M, Beilharz M, et al. Induced pluripotent stem cells as a model for diabetes investigation. Sci Rep (2015) 5:8597. doi: 10.1038/srep08597
240. Griscelli F, Ezanno H, Soubeyrand M, Feraud O, Oudrhiri N, Bonnefond A, et al. Generation of an induced pluripotent stem cell (iPSC) line from a patient with maturity-onset diabetes of the young type 3 (MODY3) carrying a hepatocyte nuclear factor 1-alpha (HNF1A) mutation. Stem Cell Res (2018) 29:56–9. doi: 10.1016/j.scr.2018.02.017
241. Tiyaboonchai A, Cardenas-Diaz FL, Ying L, Maguire JA, Sim X, Jobaliya C, et al. GATA6 Plays an Important Role in the Induction of Human Definitive Endoderm, Development of the Pancreas, and Functionality of Pancreatic β Cells. Stem Cell Rep (2017) 8:589–604. doi: 10.1016/j.stemcr.2016.12.026
242. Saarimäki-Vire J, Balboa D, Russell MA, Saarikettu J, Kinnunen M, Keskitalo S, et al. An Activating STAT3 Mutation Causes Neonatal Diabetes through Premature Induction of Pancreatic Differentiation. Cell Rep (2017) 19:281–94. doi: 10.1016/j.celrep.2017.03.055
243. Grotz AK, Abaitua F, Navarro-Guerrero E, Hastoy B, Ebner D, Gloyn AL. A CRISPR/Cas9 genome editing pipeline in the EndoC-βH1 cell line to study genes implicated in beta cell function. Wellcome Open Res (2019) 4:150. doi: 10.12688/wellcomeopenres.15447.1
244. Cardenas-Diaz FL, Leavens KF, Kishore S, Osorio-Quintero C, Chen Y-J, Stanger BZ, et al. A Dual Reporter EndoC-βH1 Human β-Cell Line for Efficient Quantification of Calcium Flux and Insulin Secretion. Endocrinology (2020) 161(2):bqaa005. doi: 10.1210/endocr/bqaa005
245. McGrath PS, Watson CL, Ingram C, Helmrath MA, Wells JM. The Basic Helix-Loop-Helix Transcription Factor NEUROG3 Is Required for Development of the Human Endocrine Pancreas. Diabetes (2015) 64:2497–505. doi: 10.2337/db14-1412
246. Canver MC, Smith EC, Sher F, Pinello L, Sanjana NE, Shalem O, et al. BCL11A enhancer dissection by Cas9-mediated in situ saturating mutagenesis. Nature (2015) 527:192–7. doi: 10.1038/nature15521
247. Ren G, Jin W, Cui K, Rodrigez J, Hu G, Zhang Z, et al. CTCF-Mediated Enhancer-Promoter Interaction Is a Critical Regulator of Cell-to-Cell Variation of Gene Expression. Mol Cell (2017) 67:1049–58.e6. doi: 10.1016/j.molcel.2017.08.026
248. Akıncılar SC, Khattar E, Boon PLS, Unal B, Fullwood MJ, Tergaonkar V. Long-Range Chromatin Interactions Drive Mutant TERT Promoter Activation. Cancer Discov (2016) 6:1276–91. doi: 10.1158/2159-8290.CD-16-0177
249. Lupiáñez DG, Kraft K, Heinrich V, Krawitz P, Brancati F, Klopocki E, et al. Disruptions of topological chromatin domains cause pathogenic rewiring of gene-enhancer interactions. Cell (2015) 161:1012–25. doi: 10.1016/j.cell.2015.04.004
250. Giménez CA, Ielpi M, Mutto A, Grosembacher L, Argibay P, Pereyra-Bonnet F. CRISPR-on system for the activation of the endogenous human INS gene. Gene Ther (2016) 23:543–7. doi: 10.1038/gt.2016.28
251. Ma S, Viola R, Sui L, Cherubini V, Barbetti F, Egli D. β Cell Replacement after Gene Editing of a Neonatal Diabetes-Causing Mutation at the Insulin Locus. Stem Cell Rep (2018) 11:1407–15. doi: 10.1016/j.stemcr.2018.11.006
252. Balboa D, Saarimäki-Vire J, Borshagovski D, Survila M, Lindholm P, Galli E, et al. Insulin mutations impair beta-cell development in a patient-derived iPSC model of neonatal diabetes. eLife (2018) 7:e38519. doi: 10.7554/eLife.38519
253. Maxwell KG, Augsornworawat P, Velazco-Cruz L, Kim MH, Asada R, Hogrebe NJ, et al. Gene-edited human stem cell-derived β cells from a patient with monogenic diabetes reverse preexisting diabetes in mice. Sci Transl Med (2020) 12(540):eaax9106. doi: 10.1126/scitranslmed.aax9106
254. da Silva Xavier G, Bellomo EA, McGinty JA, French PM, Rutter GA. Animal models of GWAS-identified type 2 diabetes genes. J Diabetes Res (2013) 2013:906590. doi: 10.1155/2013/906590
255. Liu Y, Du Y, Xie W, Zhang F, Forrest D, Liu C. Generation of Conditional Knockout Mice by Sequential Insertion of Two loxP Sites In Cis Using CRISPR/Cas9 and Single-Stranded DNA Oligonucleotides. Methods Mol Biol Clifton NJ (2019) 1874:191–210. doi: 10.1007/978-1-4939-8831-0_11
256. Smith LIF, Hill TG, Bowe JE. Generating Beta-Cell-Specific Transgenic Mice Using the Cre-Lox System. Methods Mol Biol Clifton NJ (2020) 2128:181–205. doi: 10.1007/978-1-0716-0385-7_13
257. Ohtsuka M, Sato M, Miura H, Takabayashi S, Matsuyama M, Koyano T, et al. i-GONAD: a robust method for in situ germline genome engineering using CRISPR nucleases. Genome Biol (2018) 19:25. doi: 10.1186/s13059-018-1400-x
258. Burgio G. Redefining mouse transgenesis with CRISPR/Cas9 genome editing technology. Genome Biol (2018) 19:27. doi: 10.1186/s13059-018-1409-1
259. Nelson CE, Hakim CH, Ousterout DG, Thakore PI, Moreb EA, Castellanos Rivera RM, et al. In vivo genome editing improves muscle function in a mouse model of Duchenne muscular dystrophy. Science (2016) 351:403–7. doi: 10.1126/science.aad5143
260. Tabebordbar M, Zhu K, Cheng JKW, Chew WL, Widrick JJ, Yan WX, et al. In vivo gene editing in dystrophic mouse muscle and muscle stem cells. Science (2016) 351:407–11. doi: 10.1126/science.aad5177
261. Yang H, Wang H, Shivalila CS, Cheng AW, Shi L, Jaenisch R. One-step generation of mice carrying reporter and conditional alleles by CRISPR/Cas-mediated genome engineering. Cell (2013) 154:1370–9. doi: 10.1016/j.cell.2013.08.022
262. Platt RJ, Chen S, Zhou Y, Yim MJ, Swiech L, Kempton HR, et al. CRISPR-Cas9 knockin mice for genome editing and cancer modeling. Cell (2014) 159:440–55. doi: 10.1016/j.cell.2014.09.014
263. Mandasari M, Sawangarun W, Katsube K, Kayamori K, Yamaguchi A, Sakamoto K. A facile one-step strategy for the generation of conditional knockout mice to explore the role of Notch1 in oroesophageal tumorigenesis. Biochem Biophys Res Commun (2016) 469:761–7. doi: 10.1016/j.bbrc.2015.12.006
264. Miura H, Quadros RM, Gurumurthy CB, Ohtsuka M. Easi-CRISPR for creating knock-in and conditional knockout mouse models using long ssDNA donors. Nat Protoc (2018) 13:195–215. doi: 10.1038/nprot.2017.153
265. Quadros RM, Miura H, Harms DW, Akatsuka H, Sato T, Aida T, et al. Easi-CRISPR: a robust method for one-step generation of mice carrying conditional and insertion alleles using long ssDNA donors and CRISPR ribonucleoproteins. Genome Biol (2017) 18:92. doi: 10.1186/s13059-017-1220-4
266. Ackermann AM, Zhang J, Heller A, Briker A, Kaestner KH. High-fidelity Glucagon-CreER mouse line generated by CRISPR-Cas9 assisted gene targeting. Mol Metab (2017) 6:236–44. doi: 10.1016/j.molmet.2017.01.003
267. Barrangou R, Doudna JA. Applications of CRISPR technologies in research and beyond. Nat Biotechnol (2016) 34:933–41. doi: 10.1038/nbt.3659
268. Huang C-H, Lee K-C, Doudna JA. Applications of CRISPR-Cas Enzymes in Cancer Therapeutics and Detection. Trends Cancer (2018) 4:499–512. doi: 10.1016/j.trecan.2018.05.006
269. Yin H, Song C-Q, Dorkin JR, Zhu LJ, Li Y, Wu Q, et al. Therapeutic genome editing by combined viral and non-viral delivery of CRISPR system components in vivo. Nat Biotechnol (2016) 34:328–33. doi: 10.1038/nbt.3471
270. Wang D, Zhang F, Gao G. CRISPR-Based Therapeutic Genome Editing: Strategies and In Vivo Delivery by AAV Vectors. Cell (2020) 181:136–50. doi: 10.1016/j.cell.2020.03.023
271. Takahashi K, Tanabe K, Ohnuki M, Narita M, Ichisaka T, Tomoda K, et al. Induction of pluripotent stem cells from adult human fibroblasts by defined factors. Cell (2007) 131:861–72. doi: 10.1016/j.cell.2007.11.019
272. Kopp JL, Grompe M, Sander M. Stem cells versus plasticity in liver and pancreas regeneration. Nat Cell Biol (2016) 18:238–45. doi: 10.1038/ncb3309
273. Russ HA, Parent AV, Ringler JJ, Hennings TG, Nair GG, Shveygert M, et al. Controlled induction of human pancreatic progenitors produces functional beta-like cells in vitro. EMBO J (2015) 34:1759–72. doi: 10.15252/embj.201591058
274. Shi Z-D, Soh C-L, Zhu Z, Huangfu D. Genome Editing and Directed Differentiation of hPSCs for Interrogating Lineage Determinants in Human Pancreatic Development. J Vis Exp JoVE (2017). doi: 10.3791/55267
275. Liang G, Zhang Y. Genetic and epigenetic variations in iPSCs: potential causes and implications for application. Cell Stem Cell (2013) 13:149–59. doi: 10.1016/j.stem.2013.07.001
276. Nandal A, Mallon B, Telugu BP. Efficient Generation and Editing of Feeder-free IPSCs from Human Pancreatic Cells Using the CRISPR-Cas9 System. J Vis Exp JoVE (2017). doi: 10.3791/56260
277. Millette K, Georgia S. Gene Editing and Human Pluripotent Stem Cells: Tools for Advancing Diabetes Disease Modeling and Beta-Cell Development. Curr Diabetes Rep (2017) 17:116. doi: 10.1007/s11892-017-0947-3
278. Beer NL, Gloyn AL. Genome-edited human stem cell-derived beta cells: a powerful tool for drilling down on type 2 diabetes GWAS biology. F1000Research (2016) 5:F1000 Faculty Rev-1711. doi: 10.12688/f1000research.8682.1
Keywords: genome editing, beta cell, genome-wide association studies, maturity onset of diabetes of the young, stem cells, mouse models
Citation: Hu M, Cherkaoui I, Misra S and Rutter GA (2020) Functional Genomics in Pancreatic β Cells: Recent Advances in Gene Deletion and Genome Editing Technologies for Diabetes Research. Front. Endocrinol. 11:576632. doi: 10.3389/fendo.2020.576632
Received: 26 June 2020; Accepted: 17 September 2020;
Published: 08 October 2020.
Edited by:
Åke Sjöholm, Gävle Hospital, SwedenReviewed by:
Jocelyn Elizabeth Manning Fox, University of Alberta, CanadaBridget K. Wagner, Broad Institute, United States
Copyright © 2020 Hu, Cherkaoui, Misra and Rutter. This is an open-access article distributed under the terms of the Creative Commons Attribution License (CC BY). The use, distribution or reproduction in other forums is permitted, provided the original author(s) and the copyright owner(s) are credited and that the original publication in this journal is cited, in accordance with accepted academic practice. No use, distribution or reproduction is permitted which does not comply with these terms.
*Correspondence: Guy A. Rutter, Zy5ydXR0ZXJAaW1wZXJpYWwuYWMudWs=