- 1College of First Clinical Medicine, Shandong University of Traditional Chinese Medicine, Jinan, China
- 2College of Basic Medical, Shandong University of Traditional Chinese Medicine, Jinan, China
- 3Department of Oncology, Weifang Traditional Chinese Hospital, Weifang, China
- 4Academy of Chinese Medical Sciences, Shandong University of Traditional Chinese Medicine, Qingdao, China
- 5College of Traditional Chinese Medicine, Weifang Medical University, Weifang, China
Human epidermal growth factor receptor 2 (HER2)-positive breast cancer and triple-negative breast cancer have their own genetic, epigenetic, and protein expression profiles. In the present study, based on bioinformatics techniques, we explored the prognostic targets of HER2-positive breast cancer from metabonomics perspective and developed a new risk score system to evaluate the prognosis of patients. By identifying the differences between HER2 positive and normal control tissues, and between triple negative breast cancer and normal control tissues, we found a large number of differentially expressed metabolic genes in patients with HER2-positive breast cancer and triple-negative breast cancer. Importantly, in HER2-positive breast cancer, decreased expression of metabolism-related genes ATIC, HPRT1, ASNS, SULT1A2, and HAL was associated with increased survival. Interestingly, these five metabolism-related genes can be used to construct a risk score system to predict overall survival (OS) in HER2-positive patients. The time-dependent receiver operating characteristic (ROC) curve analysis showed that the predictive sensitivity of the risk scoring system was higher than that of other clinical factors, including age, stage, and tumor node metastasis (TNM) stage. This work shows that specific transcriptional changes in metabolic genes can be used as biomarkers to predict the prognosis of patients, which is helpful in implementing personalized treatment and evaluating patient prognosis.
Introduction
Nearly 1.7 million new cases of breast cancer are reported worldwide every year (1). However, while the incidence continues to rise, breast cancer-specific mortality has fallen sharply—in part due to earlier detection, and in part due to improved adjuvant therapies (2). Increasing evidence indicates that metabolic changes may be potential biomarkers and therapeutic targets for cancer and can be of great significance in predicting outcomes and guiding treatment. Metabolic changes are one of the important characteristics of tumors. To maintain continuous proliferation, tumor cells must adjust their metabolism and nutrient acquisition methods, playing an important role in maintaining intracellular homeostasis and responding to intracellular and extracellular stimuli (3). Recent studies have shown that metabolic reprogramming, including changes in lipid metabolism, is manifested in various types of cancer, including breast cancer (4).
Metabolic pathways are tightly intertwined with cellular signals and epigenetic networks. Due to the heterogeneity of breast cancer and the diversity of molecular subtypes, the molecular mechanisms of different subtypes of breast cancer are different (5). From a molecular perspective, HER2-positive and triple-negative breast cancer have their own characteristic inheritance, epigenetics, and protein expression profiles (6). HER2-positive breast cancer has a better prognosis, which is mainly due to the effective treatment of HER2-positive breast cancer with surgery, radiotherapy and chemotherapy, endocrine therapy, molecular targeted therapy, and other treatments (7). Identification of biomarkers and cellular pathways that lead to poor prognosis can lead to the development of new effective therapies for those who do not respond to current treatments.
The reprogramming of cellular metabolism is one of the hallmarks of breast cancer. Breast cancer cells reshape the metabolic network to maintain their transformed state and survive in the harsh tumor microenvironment in ways such as promoting tumor blood vessel formation and destroying tumor immunity (8). Studies have reported that crosstalk between estrogen signaling elements and several key metabolic regulators alters the metabolism of breast cancer cells and reshapes the tumor metabolic network to accommodate poor perfusion, transient nutritional deficiencies, and added acidity (9). The relationship between cancer and metabolic pathways may reveal novel biomarkers and therapeutic targets. Targeting tumor metabolism is a potentially effective treatment for inhibiting breast cancer. Examining the metabolic phenotype between HER2-positive and triple-negative breast cancers can identify targeted metabolic changes and potential new treatment options. Identifying multiple metabolic targets for a cancer type from a large data set containing a large amount of tumor information, such as the Cancer Genome Atlas (TCGA), is an ideal step for selecting or generating useful anti-metabolic cancer therapies (10).
Methods
Data source
The TCGA database (https://cancergenome.nih.gov/) (11) provides data on mRNA expression and clinical information related to HER2-positive and triple-negative breast cancer. All the data needed for this study was downloaded from the TCGA data download window GDC (https://portal.gdc.cancer.gov/), including 161 cases of HER2-positive breast cancer samples, 115 cases of triple-negative breast cancer samples, and 34 normal samples. TCGA is used as a public open database, and the relevant information retrieved from it does not require further ethical approval.
Analysis of mRNA Expression and Extraction of Metabolism-Related Genes
The Molecular Signatures Database (MSigDB) is a collection of annotated gene sets for use with Gene Set Enrichment Analysis (GSEA) software (https://www.gsea-msigdb.org/gsea/downloads.jsp) (12). From this website, we downloaded the background pathway gene set. Using R, we extracted the metabolic pathway in the background of GSEA and compared the genes in the pathway with the related genes in HER2-positive and triple-negative breast cancers, further obtaining the metabolic genes related to HER2-positive and triple-negative breast cancers.
mRNA expression comparisons and pathway enrichment analysis
Using the edgeR software package, the metabolism-related gene expression data of HER2-positive breast cancer, triple-negative breast cancer, and normal samples were standardized, and the differences were analyzed to obtain abnormal metabolism-related mRNA. In addition, in order to understand the functional abnormalities caused by abnormal expression of metabolic genes in patients with HER2-positive breast cancer and triple-negative breast cancer, and to explore the possible biological processes and possible pathways, the functional enrichment analysis of gene expression was carried out by using the DAVID (http://david.abcc.ncifcrf.gov/) database (13). 0.05 was set as a cut-off condition for screening enrichment analysis of Kyoto encyclopedia of genes and genomes.
Survival Analysis and Construction of the Prognostic Risk Scoring System
Using the survival software package and Cox proportional hazard model, the metabolism-related mRNA of HER2-positive breast cancer was analyzed by univariate analysis, and a survival curve was drawn. The genes with P<0.01 as a cutoff condition were of great significance to HER2-positive prognosis. Then, least absolute shrinkage and selection operator (lasso) penalized Cox regression analysis (14) was used to further screen it as an independent metabolic factor for predicting the prognosis of HER2-positive breast cancer. The complexity of the minimum absolute contraction and the lasso regression of the selection operator is controlled by the coefficient lambda. Using the cross-validation program cv.glmnet to determine the lambda value with minimum error to further reduce the number of prognosis-related metabolic mRNAs selected by univariate COX analysis, the risk scoring system can be constructed.
The formula for constructing a risk scoring system is as follows:
β represents the coefficient, while Exp represents the expression value of metabolism-related mRNA. Taking the median risk score as the critical value, patients with HER2-positive breast cancer were divided into low risk and high risk groups. The survival rates of each group were compared by the Kaplan-Meier method and log-rank method.
Comparison of Predictive Ability of Risk Scoring System Combined With Clinical Factors
In this study, in order to further compare the predictive performance of our HER2-positive breast cancer risk scoring system constructed by metabolic mRNA and evaluate whether it can be independent of other clinical parameters, univariate and multivariate analysis was conducted on the clinical data of HER2-positive breast cancer patients, including age, stage, TNM stage, survival time, and survival status. P<0.05 was considered to be statistically significant.
Results
Expression of Metabolism-Related Genes Was Altered Between HER2-Positive Breast Cancer, Triple-Negative Breast Cancer, and Normal Control Samples From the TCGA Database
In order to determine the difference in the expression of metabolic genes, we first compared the expression of mRNA in HER2-positive and triple-negative breast cancer samples with the genes of metabolism-related pathways in GSEA, so as to obtain metabolism-related genes. Then, the Edger software package was used to extract and analyze the differentially expressed data. Using | LogFC | >1 and P<0.01 as filter cutoff criteria, a total of 275 metabolic genes were obtained in HER2-positive breast cancer samples, including 154 up-regulated and 121 down-regulated (Figure 1A), while 320 metabolic genes were obtained in triple-negative breast cancer samples, including 181 up-regulated and 139 down-regulated (Figure 1B). In the cohort of difference analysis, we downloaded a total of 1096 breast cancer samples, including 161 HER2-positive breast cancer samples and 115 triple-negative breast cancer samples, which HER2-positive breast cancer accounted for 14.7% of the total and triple-negative breast cancer accounted for 10.5% of the total, and 34 cases of normal control samples. After removing the samples with incomplete prognostic information, the remaining 156 HER2-positive samples were used to construct the metabolism-related gene risk score model.
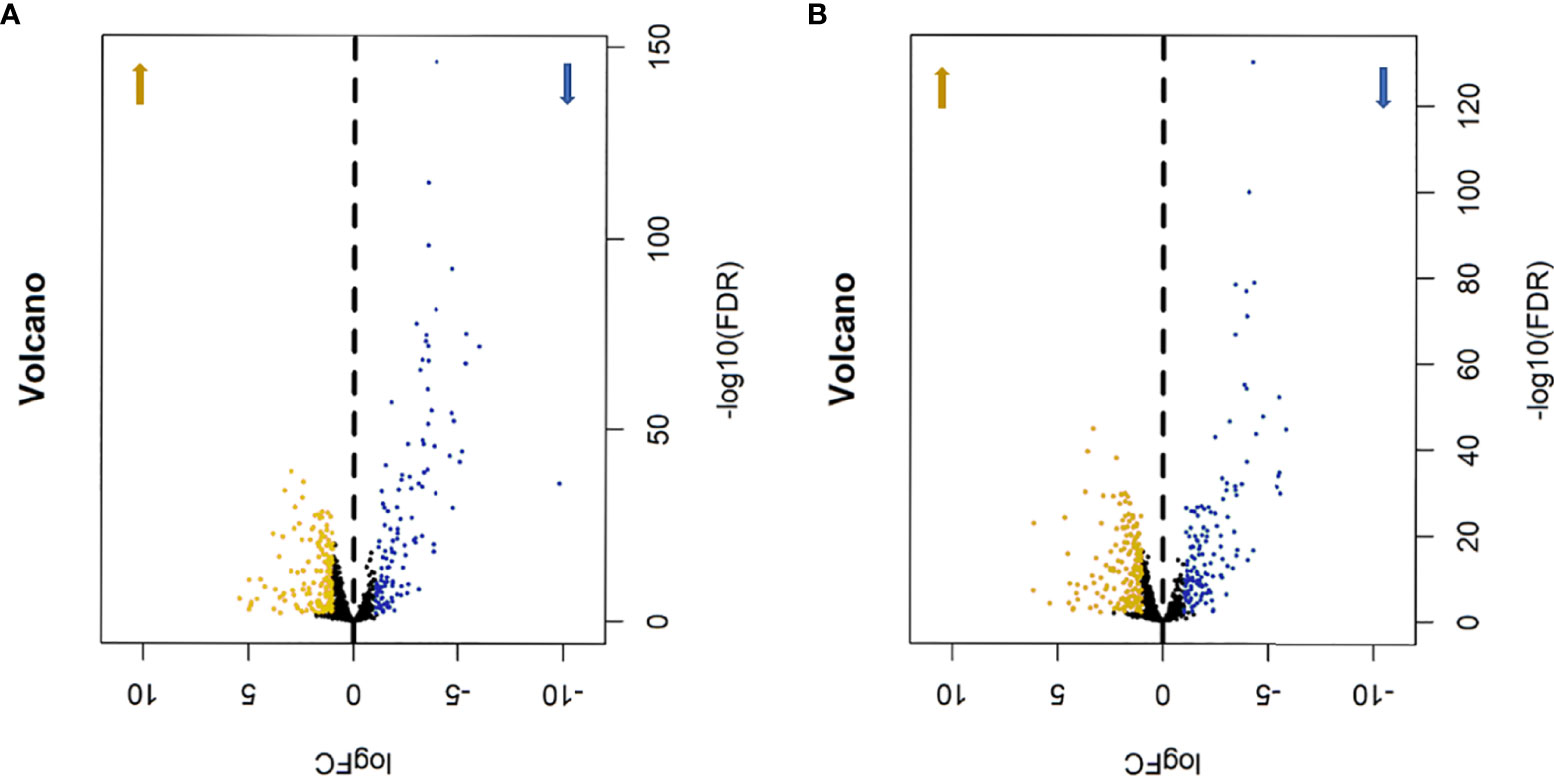
Figure 1 The volcano map of differentially expressed metabolism-related genes, Khaki represents up-regulated metabolic genes, blue represents down-regulated metabolic genes. (A) HER2-positive breast cancer, 154 up-regulated genes and 121 down-regulated genes. (B) triple-negative breast cancer, 181 up-regulated genes and 139 down-regulated genes.
Analysis of Characteristic Metabolic Pathways in HER2 Positive Breast Cancer and Triple Negative Breast Cancer
In order to further understand the characteristic metabolic pathways of HER2 positive breast cancer and triple negative breast cancer, the differential metabolism-related genes of the two types of breast cancer were enriched and separated by the DAVID database (Table 1). The results showed that there were some differences in the enrichment pathways of differential metabolic genes between the two types of breast cancer. We screened the first five pathways of the two types of breast cancer according to P value, in which purine metabolism, tyrosine metabolism, metabolism of xenobiotics by cytochrome P450, and drug metabolism were the main enrichment pathways of differential metabolic genes in HER2-positive breast cancer. The enrichment pathways of differential metabolic genes in triple-negative breast cancer mainly include purine metabolism, biosynthesis of antibiotics, pyrimidine metabolism, and metabolism of xenobiotics by cytochrome P450.
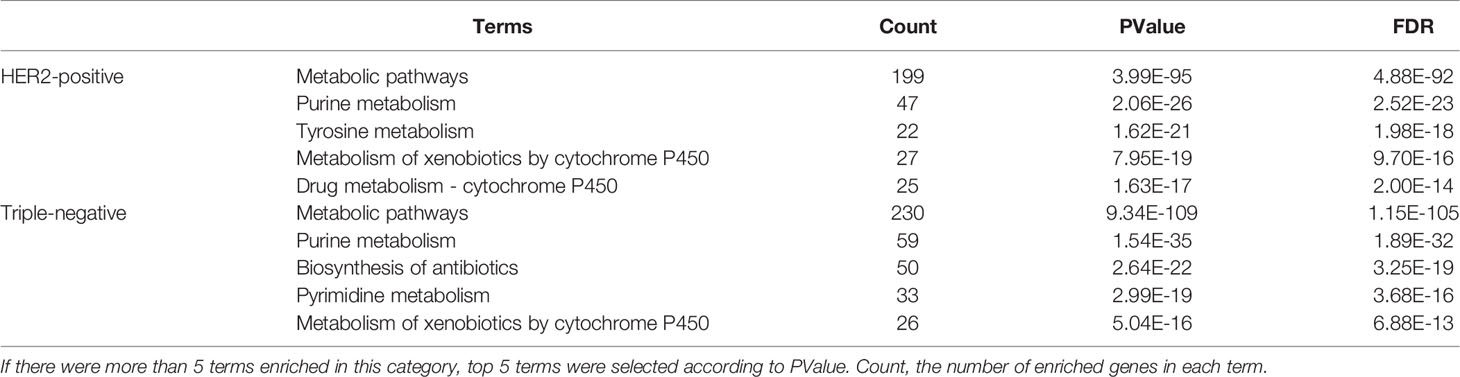
Table 1 KEGG pathway analysis about the differential metabolism-related genes in HER2-positive and triple-negative breast cancer.
Low Expression of Five Genes Is Associated With Increased Survival in Patients With HER2-Positive Breast Cancer
Changes in the expression of tumor-related metabolic genes affect disease progression, response to treatment, and functional consequences of patient survival. We analyzed the prognosis of abnormal expression of metabolism-related genes in HER2-positive breast cancer by univariate Cox method, and finally identified five genes significantly related to the survival of HER2-positive breast cancer patients, but it is worth noting that these five genes are not related to the survival of triple-negative breast cancer patients. These genes are ATIC, HPRT1, ASNS, SULT1A2, HAL. ATIC, HAL, ASNS, and HPRT1 are enriched in purine metabolism and drug metabolism as well as other enzymatic pathways and SULT1A2 is mainly enriched in the sulfur metabolism pathway. The following is a review of the relative expression of these genes in HER2-positive breast cancer and normal control tissues and their relationship with overall survival.
Compared with normal control tissues, the expression of HPRT1, ASNS, ATIC, SULT1A2, and HAL was significantly increased in HER2-positive breast cancer samples (Table 2). According to the median expression of these five genes, the overall survival time of patients with HER2-positive breast cancer was divided into two parts. We found that the low expression of the five genes was associated with good survival in HER2-positive breast cancer patients (Figure 2).
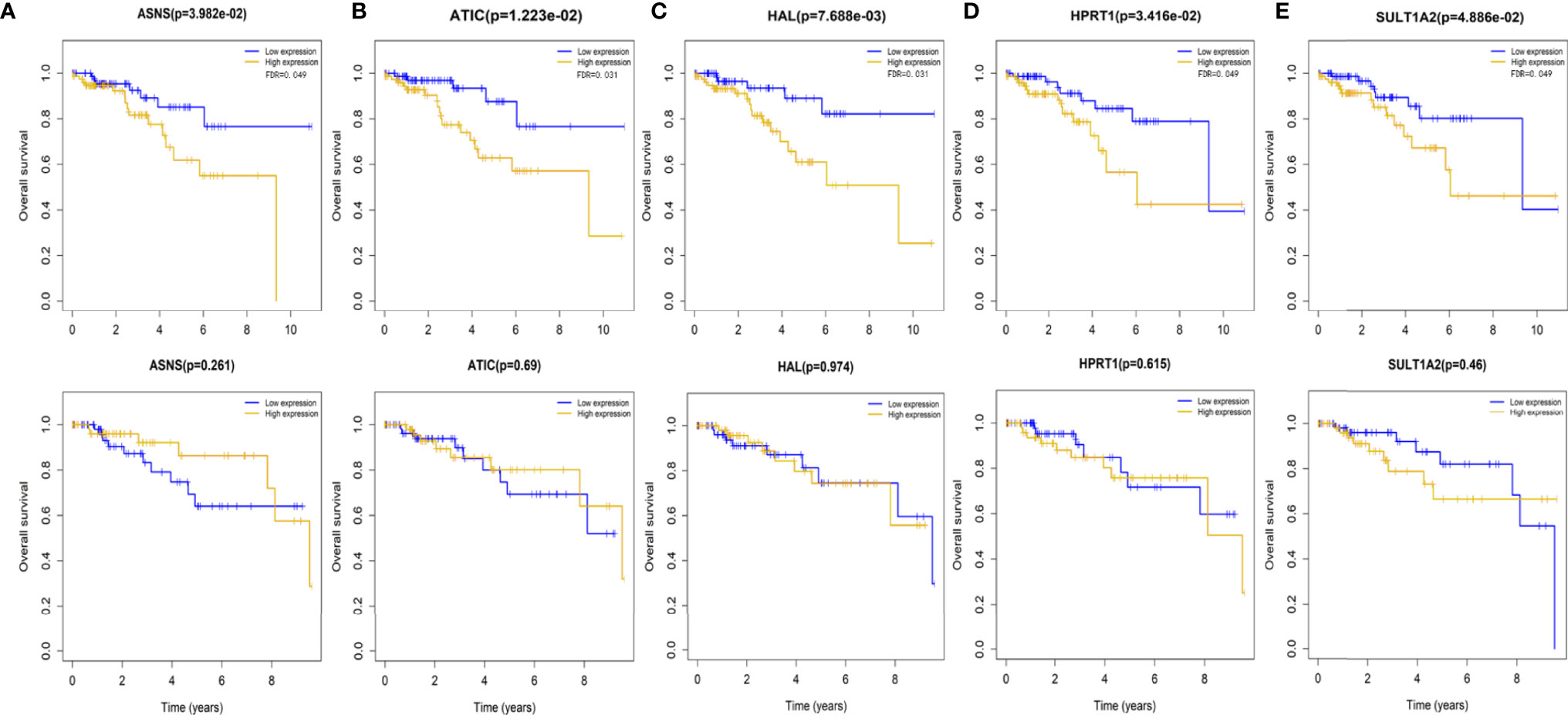
Figure 2 low expression of the five metabolism-related genes was associated with good survival in HER2-positive breast cancer patients, but not in triple-negative breast cancer patients. There were 156 positive cases of HER2-positive samples,114 cases of triple-negative samples. (A) ASNS (B) ATIC (C) HAL (D) HPRT1 (E) SULT1A2.
Derivation of the HER2-Positive Breast Cancer Risk Scoring System
According to the results of the previous analysis, five abnormally expressed metabolic genes in survival-related HER2-positive breast cancer were identified for further construction of the risk scoring system. The lambda value with minimum error was determined by the cross-validation program to screen the genes that finally construct the risk scoring system. Through analysis, we found that the five abnormally expressed metabolic genes can be used as independent prognostic factors to construct the final metabolism-related risk scoring system for HER2-positive breast cancer (Figure 3). Based on the lasso Cox regression model, the risk score was determined for each sample based on the status of the 5 genes: Risk score = (0.000198599142304591 × ATIC expression) + (0.000454057389823286 × HPRT1 expression) + (0.000161981657260441 × ASNS expression) + (0.000893755637962911 × SULT1A2 expression) + (0.0016333682644294 × HAL expression).
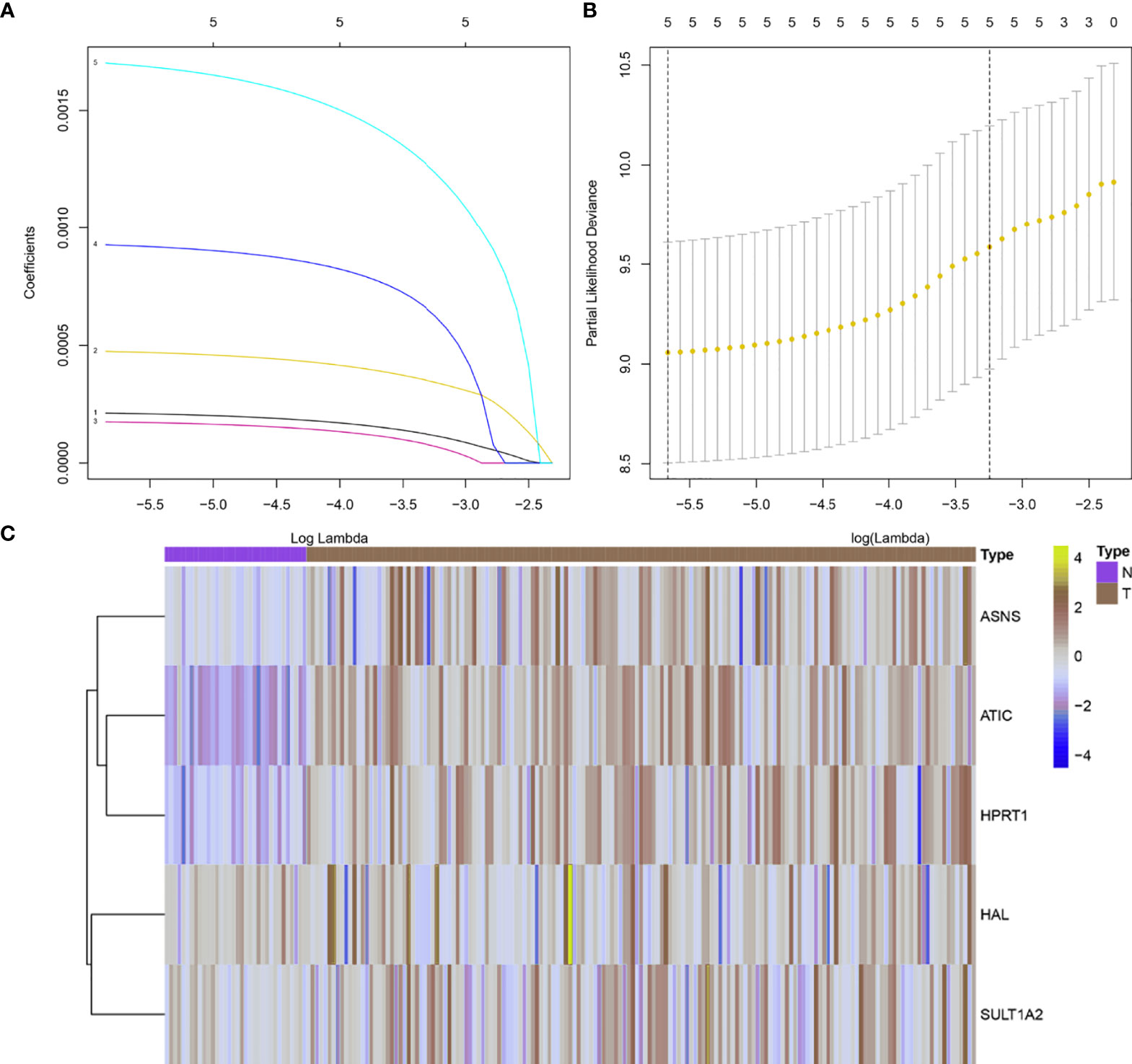
Figure 3 5 metabolism-related genes were identified for construction of the risk scoring system. (A) Validation was performed for tuning parameter selection through the LASSO regression model; (B) Elucidation for LASSO coefficient profiles of prognosis-related genes; (C) A heat map showing the expression of 5 metabolism-related genes. A total of 156 HER2-positive samples were analyzed here.
Taking the median risk score as the critical value, 156 HER2-positive breast cancer samples with complete prognostic information were divided into high risk and low risk groups, and the OS of the high risk group was significantly shorter than that of the low risk group (Figure 4). In addition, the coefficients of the five genes defined by the lasso Cox regression model are all positive, indicating that these genes are closely related to the prognostic risk of HER2-positive breast cancer patients, and high expression corresponds to shorter OS.
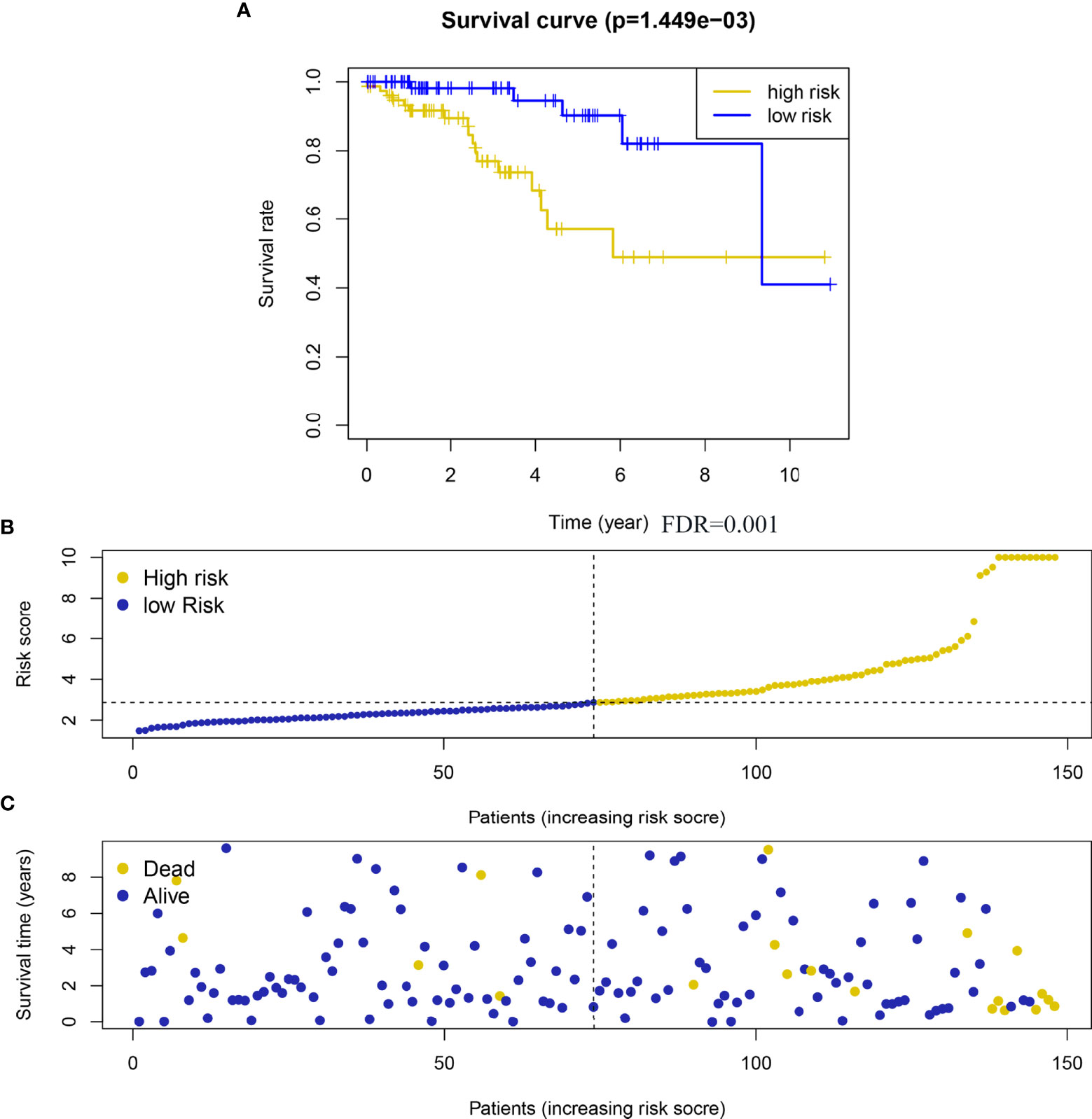
Figure 4 A risk scoring system was derived from the prognostic 5 metabolism-related genes. (A) Heatmap of the 5 metabolism-related genes expression in HER2-positive breast cancer. (B) The risk score distribution on the basis of the 5 metabolism-related genes; (C) The vital status of 156 patients with HER2-positive breast cancer in high‐ and low‐risk groups. A total of 156 HER2-positive samples were analyzed here.
Comparative Analysis of Risk Scoring System and Clinical Factors
Through the analysis using the lasso Cox regression model, it was found that the risk score system constructed by five metabolism-related genes could be used to predict the OS of HER2-positive patients. For further analysis, we included clinical factors in this study (Table 3). Univariate Cox analysis showed that risk score, age, stage, and T stage were closely related to OS in HER2-positive patients, which was statistically significant (Figure 5A). Multivariate Cox analysis showed that age and risk score could be used as independent prognostic factors to evaluate the survival rate of patients (Figure 5B). It can be seen that, whether using univariate analysis or multivariate analysis, our risk scoring system can effectively evaluate the prognosis, which further shows the evaluation value of the model. In order to further evaluate its predictive performance, we compared the predictive sensitivity of the risk scoring system with other clinical factors through the ROC curve and found that the predictive ability of the scoring system was higher than that of other clinical factors in predicting the 1-year, 3-year, or 5-year survival rate of patients (Figure 6).
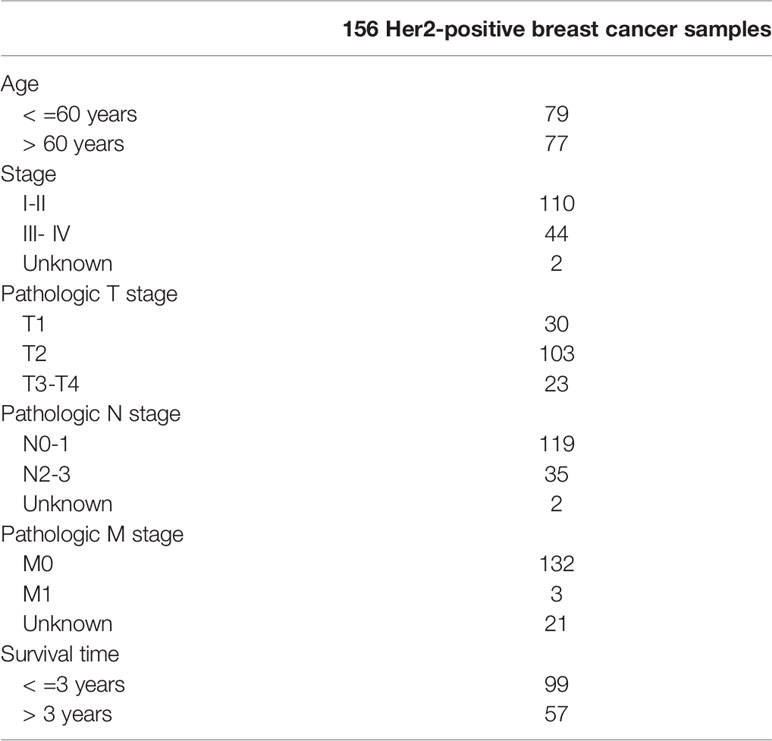
Table 3 The specific baseline clinicopathological characteristics of 156 Her2-positive breast cancer samples.
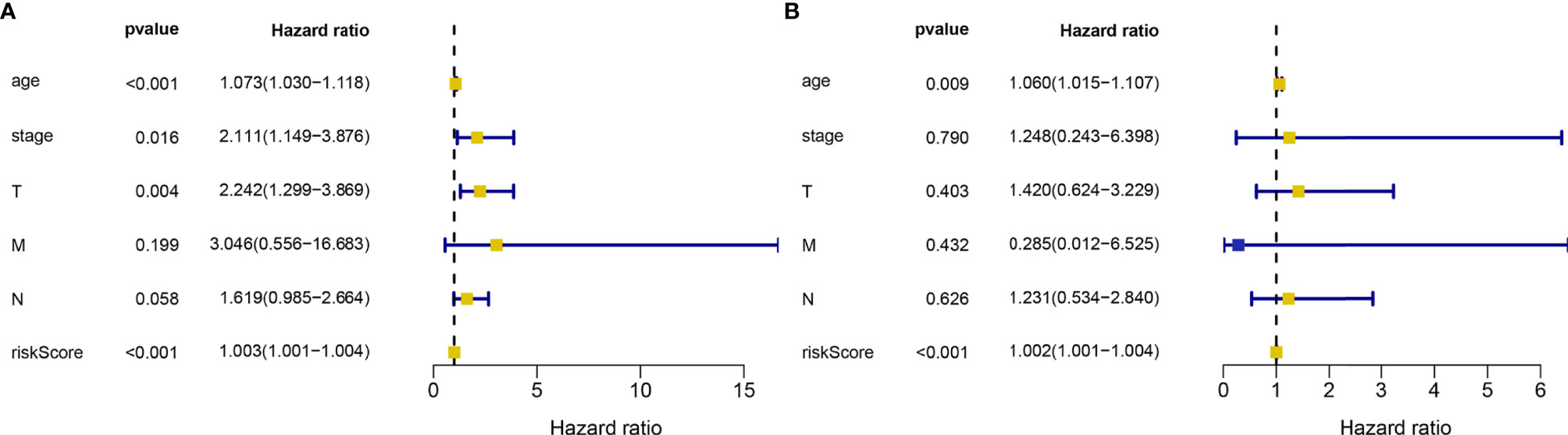
Figure 5 Univariate (A) and multivariate (B) analysis of risk score and clinical data related to HER2-positive breast cancer samples in TCGA database. The squares represent HR values, khaki means HR>1, blue means HR<1.The blue line represents the 95% confidence interval. A total of 133 HER2-positive samples were included in the analysis.
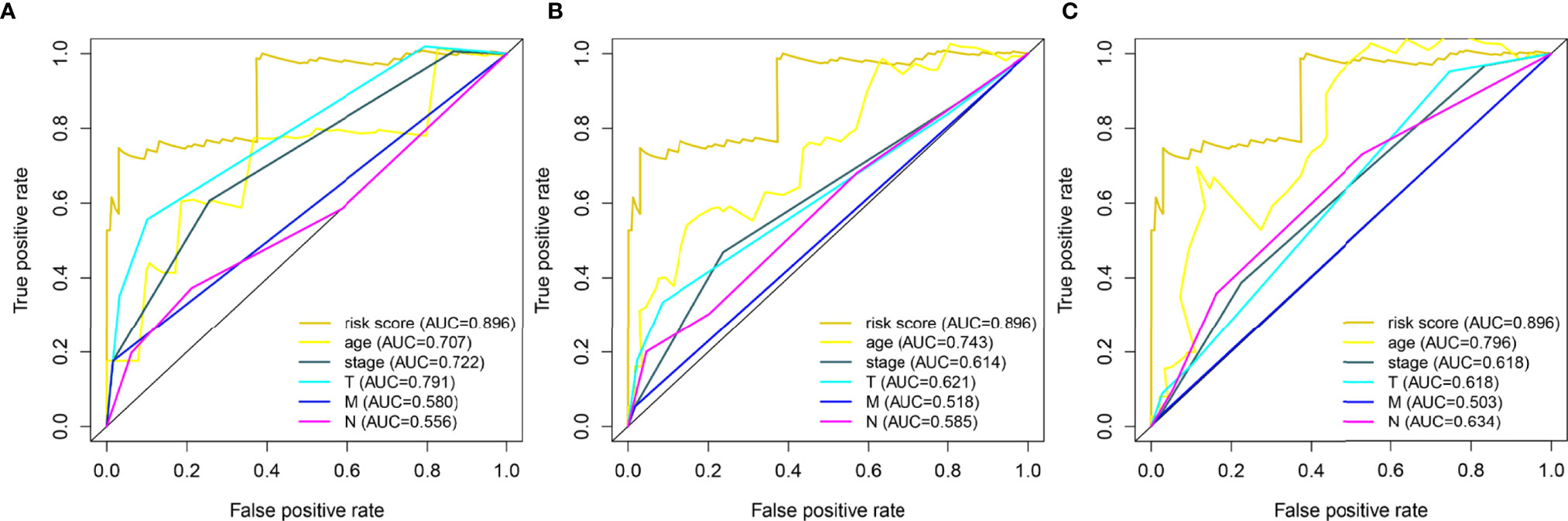
Figure 6 Time-dependent ROC curves for risk score and clinical data based on 5 metabolism-related genes. A total of 133 HER2-positive samples were included in the analysis. (A) 1 year-(B) 3 year-(C) 5year-.
Discussion
In this study, we downloaded the RNA-SEQ data of HER2-positive breast cancer and triple-negative breast cancer from the TCGA database, then identified and compared the differential metabolic genes in HER2-positive, triple-negative, and normal adjacent control tissues and analyzed the pathway enrichment. Our results show that metabolic genes in HER2-positive breast cancer were significantly enriched in purine metabolism, metabolism of xenobiotics by cytochrome pathway, tyrosine metabolism and drug metabolism, indicating that HER2-positive breast cancer is significantly related to amino acid metabolic pathways and drug metabolism. while the metabolic genes in triple-negative breast cancer were significantly enriched in purine metabolism, metabolism of xenobiotics by cytochrome pathway. This may be related to the response of HER2-positive breast cancer on a variety of therapeutic modalities. In addition, we found that the low expression of five metabolic genes (ATIC, HPRT1, ASNS, SULT1A2, and HAL) was associated with significant improvement in the survival rate of HER2-positive patients. Based on results we propose that the products of these genes may be developed as therapeutic targets for the treatment of HER2-positive breast cancer. In addition, the prognostic risk prediction model constructed by these five genes could independently predict the prognosis of HER2-positive breast cancer patients, and could be used as a biomarker for patient prognosis.Therefore, on the basis of the current clinical prognosis data, the comprehensive use of the prognostic scoring system can help clinicians to better predict the prognosis of patients, so as to adopt more suitable intervention measures for patients, so as to implement individualized treatment for patients, better treat the patient’s condition.
ATIC catalyzes two steps of bifunctional enzymes in purine biosynthesis (aminoimidazole-4-carboxamide ribonucleotide converting enzyme/IMP cyclohydrolase) and participates in catalyzing the last two steps of purine biosynthesis from scratch (15). Uncontrolled proliferation is the hallmark of cancer that leads to an increased demand for de novo synthesis of purine and pyrimidine bases required for DNA and RNA biosynthesis. The purine biosynthetic pathway is often up-regulated in cancer (16). Our results suggest that limiting ATIC may provide a unique target for limiting purine biosynthesis in HER2-positive breast cancer. Antifolate Aminoimidazole-4-carboxamide Ribonucleotide Formyltransferase (AICARFT) is a folic acid-dependent catalytic site within the ATIC gene. LSN3213128 is a potent antifolate inhibitor of AICARFT. Treatment with this inhibitor in a mouse-based triple-negative breast cancer xenograft model showed inhibition of tumor growth (17). Considering that the experiments were performed using triple-negative breast cancer, but based on the correlations we observed between the expression of ATIC and the survival outcome of HER2-positive breast cancer, the targeted ATIC products may be more toxic to HER2-positive breast cancer.
HPRT1 can transfer 5-phosphate ribosyl from 5-phosphate ribosyl pyrophosphate to purines, and plays an important role in purine nucleotide production through the purine rescue pathway. Our results show that low expression of HPRT1 was associated with improved prognosis of HER2-positive breast cancer, which may be related to HPRT1’s involvement in the purine rescue pathway. Gedatolisib is a dual PI3K/mTOR inhibitor, and levels of mRNAs encoding HPRT1 (a key component of the purine rescue pathway) differ significantly between non-small cell lung cancer cells that are sensitive or resistant to gedatolisib. The resistance mechanism of PI3K pathway inhibitors is mediated by the activation of the purine rescue pathway, which provides a purine resource for tumor nucleotide biosynthesis (18). 8-azaguanine is an anti-metabolic drug activated by HPRT1. Increasing the gene dose of HPRT1 in hyperploid breast cancer can control the sensitivity of this drug, which may be related to the function of HPRT1 in purine biosynthesis (19). The relationship between HPRT1 and drug treatment of HER2-positive breast cancer needs further exploration.
Asparagine synthetase (ASNS) is encoded by the asparagine synthetase (ASNS) gene and catalyzes the biosynthesis of L-asparagine (20). In our study, the low expression of ASNS in HER2-positive breast cancer is associated with patient survival, which may be due to ASNS-mediated asparagine synthesis in tumor cells. Asparagine synthetase is associated with apoptosis inhibition, protein biosynthesis, and mTORC1 activation in non-small cell lung cancer, which inhibits ASNS expression, and, combined with the depletion of extracellular asparagine, can reduce the risk of non-small cell lung cancer growth (21). In cells with transient nuclear topoisomerase I downregulation, ASNS expression is reduced. In contrast, nuclear topology isomerase I complementary increased ASNS, suggesting that there is a causal relationship between nuclear topoisomerase I and ASNS expression, which needs to be further explored (22). Deprivation of endogenous ASNS expression can lead to inhibition of MDA-MB-231 cell proliferation, impaired colony formation, and impeded cell cycle progression. This may be because down-regulating ASNS expression can reduce the accumulation of pyrimidine bases in breast cancer cells, which is consistent with our findings that ASNS expression is elevated in triple-negative breast cancer (23). ASNS may be an attractive therapeutic target for HER2-positive breast cancer, and warrants further studies.
Sulfotransferase 1A2 (SULT1A2) mediates the metabolic activation of DNA-binding products by cancerous N-hydroxyarylamines. As a gene in the hormone metabolism and growth factor pathway, SULT1A2 is related to breast density (24) and is involved in catechol estrogen metabolism (25). It is related to a variety of known risk factors for breast cancer carcinogenesis, and therefore may be a regulator of HER2-positive breast cancer risk. Patients with SULT1A2*2 and SULT1A2*3 alleles showed significantly higher plasma levels of 4-hydroxytamoxifen and endoxifen, and SULT1A2 appears to maintain optimal levels of 4-hydroxytamoxifen and endoxifen (26). Our results show that low expression of SULT1A2 is associated with improved survival in HER2-positive breast cancer. The risk regulation of SULT1A2 on HER2-positive breast cancer and its relevance to treatment need to be further explored.
Histidine ammonia lyase (HAL) is regulated by protein content and hormones (such as glucocorticoids and glucagon) in the diet (27). Histidine catabolism plays a key role in the formation and/or proliferation of certain stem cells, such as intestinal stem cells (28). Tumor cells are in a state of continuous division and proliferation, and amino acid metabolism is vigorous. The high expression level of HAL leads to a poor prognosis of estrogen receptor-positive breast cancer, which may be related to the involvement of HAL in amino acid metabolism and may be a potential therapeutic target (29). Our results show that low expression of HAL is associated with improved survival in HER2-positive breast cancer, suggesting that inhibition of tumor cell metabolism may be beneficial for tumor prognosis.
Based on the lasso Cox regression model, we constructed a risk scoring system and found that ATIC, HPRT1, ASNS, SULT1A2, and HAL were independently related to overall survival, indicating that collective changes in the expression of these five genes may be powerful predictors of clinical outcomes. Our results support this hypothesis, a total of 156 HER2-positive breast cancer samples with complete prognostic information were divided into high-risk and low-risk groups. The OS of the high-risk group was significantly shorter than that of the low-risk group. Therefore, the expression of these genes may have prognostic effects. Next, univariate and multivariate Cox analysis verified the predictive independence of the risk scoring system, and the predictive ability of the scoring system was higher than that of other clinical factors in predicting the 1-year, 3-year, or 5-year survival rate of patients. In addition, ATIC, HPRT1, ASNS, SULT1A2, and HAL may represent attractive therapeutic targets for HER2-positive breast cancer, and require further validation studies, especially considering that treatment with specific inhibitors may alter the expression of these metabolic genes.
Our study had a few limitations. Our breast cancer mRNA research data is derived from the TCGA database, but mRNA expression does not necessarily reflect protein expression levels (30). In addition, the RNA-seq data from the TCGA reflects the average mRNA expression in the entire tumor, but cannot recognize the difference in RNA expression among various tumor cells (that is, the expression differences between various tumor cells obtained from single-cell RNA sequencing platforms) (31). Finally, one must be aware that TCGA contains breast cancer data from a single, high-quality cohort, and it will be useful to verify these genes in other cohorts in the future. However, despite these limitations, the five metabolic genes identified in this study provide an interesting and reliable starting point and can provide new insights and directions for further study of disease mechanisms and treatment strategies at the metabolic level.
Conclusion
Metabonomics can be used to identify novel prognostic markers or potential therapeutic targets. In the present study, a risk scoring system was constructed based on the expression data of metabolism-related genes in HER2-positive breast cancer. Patients were divided into different grades according to the expression of differential metabolic genes ATIC, HPRT1, ASNS, SULT1A2, and HAL in the system to evaluate the prognosis of patients. The products of these genes may be useful new therapeutic targets for HER2-positive breast cancer. Inhibition of the function of these metabolic genes can modulate the metabolism of tumors with low levels of metabolic gene expression, thus improving the survival rate of HER2-positive breast cancer patients.
Data Availability Statement
The raw data supporting the conclusions of this manuscript will be made available by the authors, without undue reservation, to any qualified researcher.
Ethics Statement
Ethical review and approval was not required for the study on human participants in accordance with the local legislation and institutional requirements. Written informed consent for participation was not required for this study in accordance with the national legislation and the institutional requirements.
Author Contributions
CG and CS conceived and designed the study; JZ and CL performed data analysis; CZ and LL contributed analysis tools; CG and HL wrote the paper. All authors contributed to the article and approved the submitted version.
Funding
This work is supported by the grants from National Natural Science Foundation of China (81973677) and National Natural Science Foundation of China (82174222).
Conflict of Interest
The authors declare that the research was conducted in the absence of any commercial or financial relationships that could be construed as a potential conflict of interest.
Publisher’s Note
All claims expressed in this article are solely those of the authors and do not necessarily represent those of their affiliated organizations, or those of the publisher, the editors and the reviewers. Any product that may be evaluated in this article, or claim that may be made by its manufacturer, is not guaranteed or endorsed by the publisher.
References
1. Cui H, Kong H, Peng F, Wang C, Zhang D, Tian J, et al. Inferences of Individual Drug Response-Related Long Non-Coding RNAs Based on Integrating Multi-Omics Data in Breast Cancer. Mol Ther Nucleic Acids (2020) 20:128–39. doi: 10.1016/j.omtn.2020.01.038
2. Munoz D, Near AM, van Ravesteyn NT, Lee SJ, Schechter CB, Alagoz O, et al. Effects of Screening and Systemic Adjuvant Therapy on ER-Specific US Breast Cancer Mortality. J Natl Cancer Inst (2014) 106. doi: 10.1093/jnci/dju289
3. Li X, Wenes M, Romero P, Huang SC, Fendt SM, Ho PC. Navigating Metabolic Pathways to Enhance Antitumour Immunity and Immunotherapy. Nat Rev Clin Oncol (2019) 16:425–41. doi: 10.1038/s41571-019-0203-7
4. Dinarvand N, Khanahmad H, Hakimian SM, Sheikhi A, Rashidi B, Pourfarzam M. Evaluation of Long-Chain Acyl-Coenzyme A Synthetase 4 (ACSL4) Expression in Human Breast Cancer. Res Pharm Sci (2020) 15:48–56. doi: 10.4103/1735-5362.278714
5. Li XX, Wang LJ, Hou J, Liu HY, Wang R, Wang C, et al. Identification of Long Noncoding RNAs as Predictors of Survival in Triple-Negative Breast Cancer Based on Network Analysis. BioMed Res Int (2020) 2020:8970340. doi: 10.1155/2020/8970340
6. Ronchi A, Pagliuca F, Zito Marino F, Accardo M, Cozzolino I, Franco R. Current and Potential Immunohistochemical Biomarkers for Prognosis and Therapeutic Stratification of Breast Carcinoma. Semin Cancer Biol (2020) 72:114–22. doi: 10.1016/j.semcancer.2020.03.002
7. Waks AG, Winer EP. Breast Cancer Treatment: A Review. Jama (2019) 321:288–300. doi: 10.1001/jama.2018.19323
8. Wang YP, Lei QY. Perspectives of Reprogramming Breast Cancer Metabolism. Adv Exp Med Biol (2017) 1026:217–32. doi: 10.1007/978-981-10-6020-5_10
9. Kulkoyluoglu-Cotul E, Arca A, Madak-Erdogan Z. Crosstalk Between Estrogen Signaling and Breast Cancer Metabolism. Trends Endocrinol Metabol: TEM (2019) 30:25–38. doi: 10.1016/j.tem.2018.10.006
10. Cooper LA, Demicco EG, Saltz JH, Powell RT, Rao A, Lazar AJ. PanCancer Insights From The Cancer Genome Atlas: The Pathologist's Perspective. J Pathol (2018) 244:512–24. doi: 10.1002/path.5028
11. Tomczak K, Czerwinska P, Wiznerowicz M. The Cancer Genome Atlas (TCGA): An Immeasurable Source of Knowledge. Contemp Oncol (Poz Pol) (2015) 19:A68–77. doi: 10.5114/wo.2014.47136
12. Subramanian A, Tamayo P, Mootha VK, Mukherjee S, Ebert BL, Gillette MA, et al. Gene Set Enrichment Analysis: A Knowledge-Based Approach for Interpreting Genome-Wide Expression Profiles. Proc Natl Acad Sci USA (2005) 102:15545–50. doi: 10.1073/pnas.0506580102
13. Jiao X, Sherman BT, Huang da W, Stephens R, Baseler MW, Lane HC, et al. DAVID-WS: A Stateful Web Service to Facilitate Gene/Protein List Analysis. Bioinf (Oxf Engl) (2012) 28:1805–6. doi: 10.1093/bioinformatics/bts251
14. Gao J, Kwan PW, Shi D. Sparse Kernel Learning With LASSO and Bayesian Inference Algorithm. Neural Networks Off J Int Neural Net Soc (2010) 23:257–64. doi: 10.1016/j.neunet.2009.07.001
15. Cheong CG, Wolan DW, Greasley SE, Horton PA, Beardsley GP, Wilson IA. Crystal Structures of Human Bifunctional Enzyme Aminoimidazole-4-Carboxamide Ribonucleotide Transformylase/IMP Cyclohydrolase in Complex With Potent Sulfonyl-Containing Antifolates. J Biol Chem (2004) 279:18034–45. doi: 10.1074/jbc.M313691200
16. Fales KR, Njoroge FG, Brooks HB, Thibodeaux S, Torrado A, Si C, et al. Discovery of N-(6-Fluoro-1-Oxo-1,2-Dihydroisoquinolin-7-Yl)-5-[(3R)-3-Hydroxypyrrolidin-1-Yl]T Hiophene-2-Sulfonamide (LSN 3213128), a Potent and Selective Nonclassical Antifolate Aminoimidazole-4-Carboxamide Ribonucleotide Formyltransferase (AICARFT) Inhibitor Effective at Tumor Suppression in a Cancer Xenograft Model. J Med Chem (2017) 60:9599–616. doi: 10.1021/acs.jmedchem.7b01046
17. Brooks HB, Meier TI, Geeganage S, Fales KR, Thrasher KJ, Konicek SA, et al. Characterization of a Novel AICARFT Inhibitor Which Potently Elevates ZMP and has Anti-Tumor Activity in Murine Models. Sci Rep (2018) 8:15458. doi: 10.1038/s41598-018-33453-4
18. Makinoshima H, Umemura S, Suzuki A, Nakanishi H, Maruyama A, Udagawa H, et al. Metabolic Determinants of Sensitivity to Phosphatidylinositol 3-Kinase Pathway Inhibitor in Small-Cell Lung Carcinoma. Cancer Res (2018) 78:2179–90. doi: 10.1158/0008-5472.CAN-17-2109
19. Choudhary A, Zachek B, Lera RF, Zasadil LM, Lasek A, Denu RA, et al. Identification of Selective Lead Compounds for Treatment of High-Ploidy Breast Cancer. Mol Cancer Ther (2016) 15:48–59. doi: 10.1158/1535-7163.MCT-15-0527
20. Richards NG, Schuster SM. Mechanistic Issues in Asparagine Synthetase Catalysis. Adv Enzymol Relate Area Mol Biol (1998) 72:145–98. doi: 10.1002/9780470123188.ch5
21. Gwinn DM, Lee AG, Briones-Martin-Del-Campo M, Conn CS, Simpson DR, Scott AI, et al. Oncogenic KRAS Regulates Amino Acid Homeostasis and Asparagine Biosynthesis via ATF4 and Alters Sensitivity to L-Asparaginase. Cancer Cell (2018) 33:91–107.e6. doi: 10.1016/j.ccell.2017.12.003
22. Miao ZH, Player A, Shankavaram U, Wang YH, Zimonjic DB, Lorenzi PL, et al. Nonclassic Functions of Human Topoisomerase I: Genome-Wide and Pharmacologic Analyses. Cancer Res (2007) 67:8752–61. doi: 10.1158/0008-5472.CAN-06-4554
23. Yang H, He X, Zheng Y, Feng W, Xia X, Yu X, et al. Down-Regulation of Asparagine Synthetase Induces Cell Cycle Arrest and Inhibits Cell Proliferation of Breast Cancer. Chem Biol Drug Des (2014) 84:578–84. doi: 10.1111/cbdd.12348
24. Ellingjord-Dale M, Lee E, Couto E, Ozhand A, Qureshi S, Hofvind S, et al. Polymorphisms in Hormone Metabolism and Growth Factor Genes and Mammographic Density in Norwegian Postmenopausal Hormone Therapy Users and Non-Users. Breast Cancer Res BCR (2012) 14:R135. doi: 10.1186/bcr3337
25. Hui Y, Yasuda S, Liu MY, Wu YY, Liu MC. On the Sulfation and Methylation of Catecholestrogens in Human Mammary Epithelial Cells and Breast Cancer Cells. Biol Pharm Bull (2008) 31:769–73. doi: 10.1248/bpb.31.769
26. Fernandez-Santander A, Gaibar M, Novillo A, Romero-Lorca A, Rubio M, Chicharro LM, et al. Relationship Between Genotypes Sult1a2 and Cyp2d6 and Tamoxifen Metabolism in Breast Cancer Patients. PloS One (2013) 8:e70183. doi: 10.1371/journal.pone.0070183
27. Aleman G, Ortiz V, Langley E, Tovar AR, Torres N. Regulation by Glucagon of the Rat Histidase Gene Promoter in Cultured Rat Hepatocytes and Human Hepatoblastoma Cells. Am J Physiol Endocrinol Metab (2005) 289:E172-9. doi: 10.1152/ajpendo.00584.2004
28. Okada M, Miller TC, Fu L, Shi YB. Direct Activation of Amidohydrolase Domain-Containing 1 Gene by Thyroid Hormone Implicates a Role in the Formation of Adult Intestinal Stem Cells During Xenopus Metamorphosis. Endocrinology (2015) 156:3381–93. doi: 10.1210/en.2015-1190
29. Wang CY, Chiao CC, Phan NN, Li CY, Sun ZD, Jiang JZ, et al. Gene Signatures and Potential Therapeutic Targets of Amino Acid Metabolism in Estrogen Receptor-Positive Breast Cancer. Am J Cancer Res (2020) 10:95–113.
30. Fortelny N, Overall CM, Pavlidis P, Freue GVC. Can We Predict Protein From mRNA Levels? Nature (2017) 547:E19–20. doi: 10.1038/nature22293
Keywords: HER2-positive breast cancer, metabonomics, prognostic risk scoring system, lasso cox regression analysis, survival prediction
Citation: Gao C, Li H, Zhou C, Liu C, Zhuang J, Liu L and Sun C (2022) Survival-Associated Metabolic Genes and Risk Scoring System in HER2-Positive Breast Cancer. Front. Endocrinol. 13:813306. doi: 10.3389/fendo.2022.813306
Received: 25 January 2022; Accepted: 11 April 2022;
Published: 19 May 2022.
Edited by:
Ke-Da Yu, Fudan University, ChinaReviewed by:
Zahra Rattray, University of Strathclyde, United KingdomWenbin Zhou, Nanjing Medical University, China
Copyright © 2022 Gao, Li, Zhou, Liu, Zhuang, Liu and Sun. This is an open-access article distributed under the terms of the Creative Commons Attribution License (CC BY). The use, distribution or reproduction in other forums is permitted, provided the original author(s) and the copyright owner(s) are credited and that the original publication in this journal is cited, in accordance with accepted academic practice. No use, distribution or reproduction is permitted which does not comply with these terms.
*Correspondence: Changgang Sun, c2NnZG9jdG9yQDEyNi5jb20=
†These authors have contributed equally to this work and share first authorship