- 1Department of Obstetrics and Gynaecology, Zhongnan Hospital of Wuhan University, Wuhan, China
- 2Hubei Clinical Research Center for Prenatal Diagnosis and Birth Health, Zhongnan Hospital of Wuhan University, Wuhan, China
- 3Hubei Provincial Key Laboratory of Developmentally Originated Diseases, Wuhan University, Wuhan, China
- 4Department of Obstetrics, Maternal and Child Health Hospital of Hubei Province, Tongji Medical College, Huazhong University of Science and Technology, Wuhan, China
- 5Department of Clinical Laboratory, Center for Gene Diagnosis & Program of Clinical Laboratory Zhongnan Hospital Wuhan University, Wuhan, China
Objective: This study aims to develop and evaluate a predictive nomogram for early assessment risk factors of gestational diabetes mellitus (GDM) during early pregnancy term, so as to help early clinical management and intervention.
Methods: A total of 824 pregnant women at Zhongnan Hospital of Wuhan University and Maternal and Child Health Hospital of Hubei Province from 1 February 2020 to 30 April 2020 were enrolled in a retrospective observational study and comprised the training dataset. Routine clinical and laboratory information was collected; we applied least absolute shrinkage and selection operator (LASSO) logistic regression and multivariate ROC risk analysis to determine significant predictors and establish the nomogram, and the early pregnancy files (gestational weeks 12–16, n = 392) at the same hospital were collected as a validation dataset. We evaluated the nomogram via the receiver operating characteristic (ROC) curve, C-index, calibration curve, and decision curve analysis (DCA).
Results: We conducted LASSO analysis and multivariate regression to establish a GDM nomogram during the early pregnancy term; the five selected risk predictors are as follows: age, blood urea nitrogen (BUN), fibrinogen-to-albumin ratio (FAR), blood urea nitrogen-to-creatinine ratio (BUN/Cr), and blood urea nitrogen-to-albumin ratio (BUN/ALB). The calibration curve and DCA present optimal predictive power. DCA demonstrates that the nomogram could be applied clinically.
Conclusion: An effective nomogram that predicts GDM should be established in order to help clinical management and intervention at the early gestational stage.
Introduction
Gestational diabetes mellitus (GDM) is a universal metabolic disturbance syndrome with a complicated etiology during pregnancy; insulin resistance and pancreatic β cell failure were significant factors for the pathogenesis of the disease, which gradually leads to hyperglycemia (1–4). Hyperglycemia exposure contributes to both maternal and fetal adverse complications. The degree of dysregulation of blood glucose is highly related to the risks of obstetrical and neonatal outcomes, which include cesarean section, hypertension, preeclampsia, polyhydramnios, preterm delivery, fetal growth restriction, birth injury, and respiratory distress. In the long term, there is an increased risk of developing obesity, cardiovascular disease, and type 2 diabetes mellitus in both the mother and the offspring (5). Multiple variables have been reported in previous research, such as age, gestational week, ethnicity, obesity, lifestyle, environment, and metabolism (6, 7). Since the GDM etiology is complicated, the short-term and long-term outcomes are not optimistic and have profound influences, and the demand for early prediction and intervention is increasing.
Two acceptable diagnosis methods that are acknowledged by expert professional organizations such as the International Association of the Diabetes and Pregnancy Study Group (IADPSG) are one-step screening approach (currently preferred by the American Diabetes Association) and the two-step Carpenter–Coustan screening approach (recommended by the American College of Obstetricians and Gynecologists). The one-step screening method can diagnose more patients than the two-step screening method in a large randomized trial, and there is no statistical difference regarding maternal and neonatal adverse outcomes between these two methods (8). Both methods have their own pros and cons, and each has its own cutoff threshold (9). Due to the varying diagnostic criteria, the incidence of GDM varies from 3% to 21.2% in Asia and from 0.31% to 18% globally, and the prevalence continues to rise (10–12). The WHO recommended a 75-g anhydrous glucose load screening test for diagnosis after 8–14 h overnight fasting at 24–28 gestational weeks (13). Because pregnant women undergo the oral glucose tolerance test (OGTT) at the second stage of the trimester, early warning signs for dysglycemia may be missed.
Our study aims at establishing a nomogram to predict the risk factors of GDM during early pregnancy term and to apply early intervention. Early management and intervention of GDM improves maternal and perinatal outcomes (14, 15). Prediction models can correctly identify GDM at early gestational weeks and could mostly benefit women with targeted risk factors, which helps them focus on precision lifestyle changes. These models can be used as tools to identify risk factors and stratify diseases, which can be largely applied in clinical management and treatment (16). Using statistical modeling combined with clinical variables and laboratory information, we developed prediction tools for GDM, which can be applied in early gestational weeks.
Materials and methods
Data collection
This study is a retrospective study that recruited 1,216 pregnant women at Zhongnan Hospital of Wuhan University and Maternal and Child Health Hospital of Hubei Province from 1 February to 30 April 2020. A total of 824 pregnant women in the second and third trimesters were enrolled in the training dataset, and their clinical and laboratory data during their 12th–16th gestational weeks were retrospectively collected. We also recruited 392 pregnant women during early pregnancy as a validation dataset. We collected the following maternal clinical and laboratory information: age, gestational week, gravidity and parity history, white blood cell (WBC), red blood cell (RBC), platelet (PLT), prothrombin time (PT), activated partial thromboplastin time (APTT), thrombin time (TT), fibrinogen (FIB), total protein (TP), albumin (ALB), alkaline phosphatase (ALP), blood urea nitrogen (BUN), creatinine (Cr), uric acid (UA), fibrinogen-to-albumin ratio (FAR), blood urea nitrogen-to-creatinine ratio (BUN/Cr), and blood urea nitrogen-to-albumin (BUN/ALB). All blood samples were collected by skilled nurses, and the blood tests were taken in the laboratory of Zhongnan Hospital of Wuhan University and Maternal and Child Health Hospital of Hubei Province. The levels of these factors were measured by commercial diagnostic kits: RBC, PLT, and PLT (DXH800, UniCel automated hematology analyzer, USA); PT, APTT, and FIB (CA1500, Sysmex coagulation analyzer, USA); and TT, TP, ALP, ALB, BUN, Crea, and UA (AU5800, Beckman biochemical analyzer, USA). Inclusion criteria were as follows: (1) GDM patients with confirmed diagnosis of GDM based on the 75-g OGTT test (2010 IADPSG criteria (17); cutoff threshold values: 0 h fasting plasma glucose ≥ 5.1 mmol/L, 1 h plasma glucose ≥ 10.0 mmol/L, and 2 h plasma glucose ≥ 8.5 mmol/L) and normal pregnant women with no coexisting diseases and complications; (2) singleton pregnancy; and (3) age between 18 and 45 years. Exclusion criteria were as follows: (1) history of obstetric abnormality history, tumor, coinfection, and blood diseases; (2) presence of inflammation, cardiovascular, metabolic, immune, and endocrine diseases; and (3) type 1 diabetes mellitus or type 2 diabetes mellitus which were diagnosed before pregnancy. The details of our study process are depicted in the flowchart in Figure 1. LASSO logistic regression and multivariate ROC risk analysis were applied to establish significant factors and establish the nomogram. The early pregnancy files (gestational weeks 12–16, n = 392) were collected as a validation dataset. AUC, C-index, calibration curve, and DCA were used to evaluate the nomogram. The risk factor “age”, which acts as a continuous variable, has a poor predictive value; according to multivariate logistic regression and clinical meaning, we select the cutoff value of “30” to divide “age” as a categorical variable.
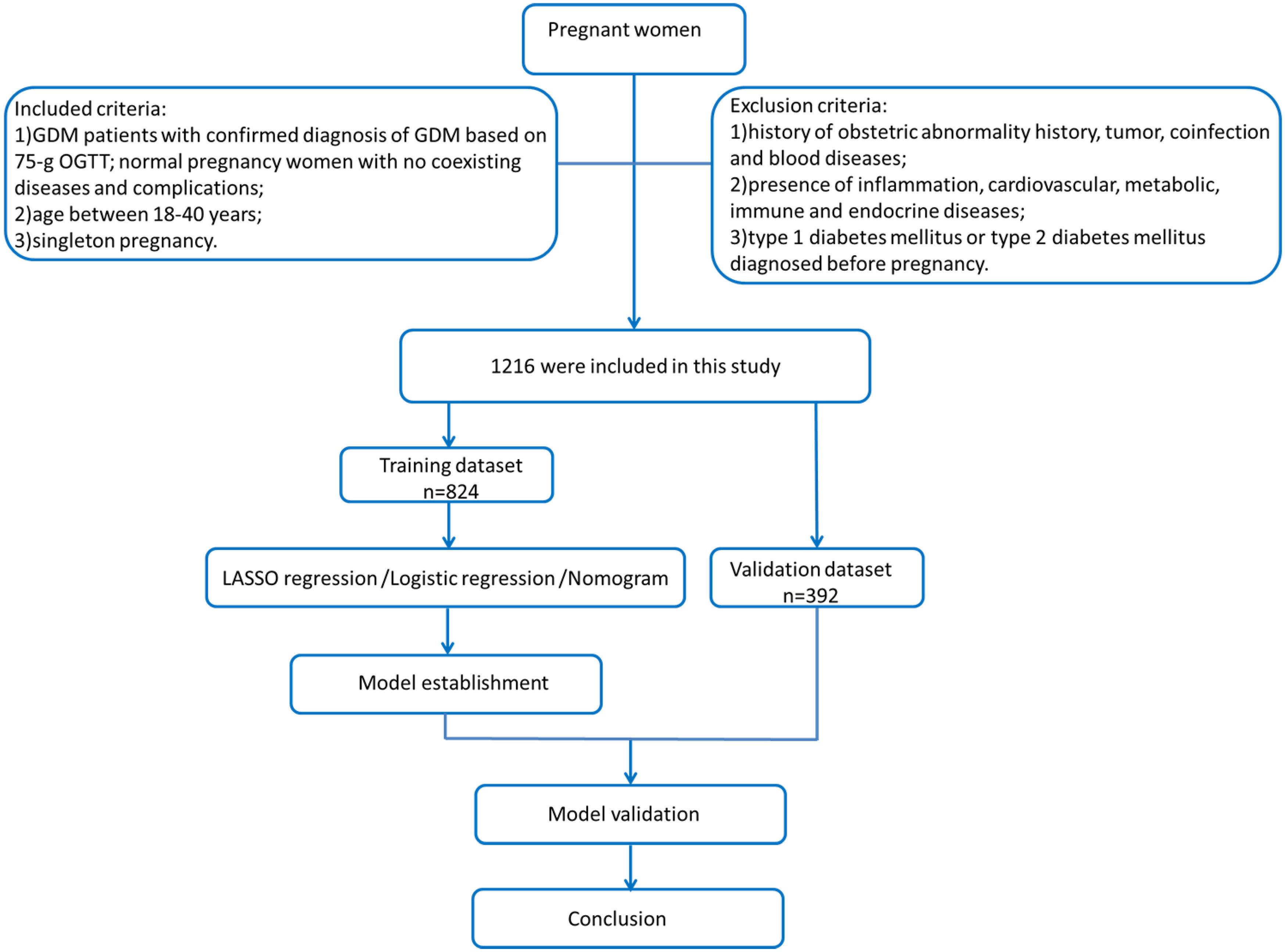
Figure 1 Flowchart of this study. A total of 1,216 pregnant women enrolled in our study were selected by inclusion criteria. The training dataset (n = 824) was used to estimate a GDM predictive model, and our study applied LASSO logistic regression and multivariate ROC risk analysis to determine significant predictors and establish the nomogram. A total of 392 early pregnant women were used as a validation dataset. We evaluate the nomogram by AUC, C-index, calibration curve, and decision curve analysis (DCA).
Statistical methods
Statistical analysis was performed with SPSS 24.0 and R 4.0.0 software (R Statistical Computing Foundation, Vienna, Austria). Continuous data were expressed as mean ± standard deviation. Clinical characteristics were compared using t-test (continuous variables) and χ2 test (categorical variables). LASSO regression was used to select the best predictive factors (18). The nomogram was established as a result of the binary logistic regression model with fivefold cross-validation. Selected factors applied in the nomogram fit the following: selected by multivariable analysis and clinically relevant. The calibration curve was applied to assess the accuracy of the predictive model (the Hosmer–Lemeshow test was used to access goodness of fit). The ROC curve evaluates discriminative ability by the area under the ROC curve (AUC). The DCA curve was conducted to determine the clinical utility and benefit of the nomogram. All cutoff values were determined by the total risk scores in the training cohort. Differences with p-value < 0.05 were considered statistically significant.
Based on the 10EPP rule (19), the sample size of our predictor model should be at least 170; our study sample consists of 824 women in the training dataset and 392 women in the validation dataset, and based on the sample size of our study, the power (1 − β) calculation is equal to 1.0.
Results
Patients’ clinical characteristics
We included 824 pregnant women in the training cohort and 392 pregnant women in the validation dataset. All the p-values of these factors are greater than 0.001, which indicates that there were no statistically significant differences between the training dataset and the validation dataset as shown in Table 1. The baseline characteristics of each dataset are presented in Table 2, in which data on non-GDM and GDM pregnant women from both datasets are shown separately. We selected the following predictive factors by logistic regression analysis: age, WBC, PLT, APTT, BUN, UA, FAR, BUN/Cr, and BUN/ALB. Then, we selected the statistically significant factors in multivariate logistic regression and clinical correlated factors to establish a predictive model, including the following factors: age, FAR, BUN, BUN/Cr, and BUN/ALB (shown in Table 3).
Development and validation of the nomogram
Based on the factors selected from the training cohort, LASSO regression analysis was conducted to select the predictive factors from Table 1 and establish the model with factors shown in Table 2: Five of the eighteen variables were enrolled to build the predictive model (Figure 2). These selected factors showed significant statistical differences, and they were independent of each other. The “Rms” package was used to build a nomogram to establish a GDM diagnosis model; the nomogram was constructed to predict the risk of GDM during early pregnancy (Figure 3). These five variables are given in Table 3. The AUC aimed to evaluate the discrimination of the nomogram in Figure 4; the AUC value of the training dataset is 0.808, 95% CI: 0.770–0.842 (p < 0.05, Figure 4A), and the AUC value of the validation dataset is 0.769, 95% CI: 0.722–0.815 (p < 0.05, Figure 4B). The calibration curve was used to evaluate the predictive power shown in Figure 5. The predictive model and the validation set showed the optimal predictive degree of the fitting. The DCA demonstrated the threshold probability of the prediction model nomogram in the training and validation datasets, respectively, and it was used to evaluate the clinical effects of the nomogram more visually, which indicated that the nomogram has optimal predictive power. DCA demonstrated that the nomogram could be applied clinically (Figure 6).
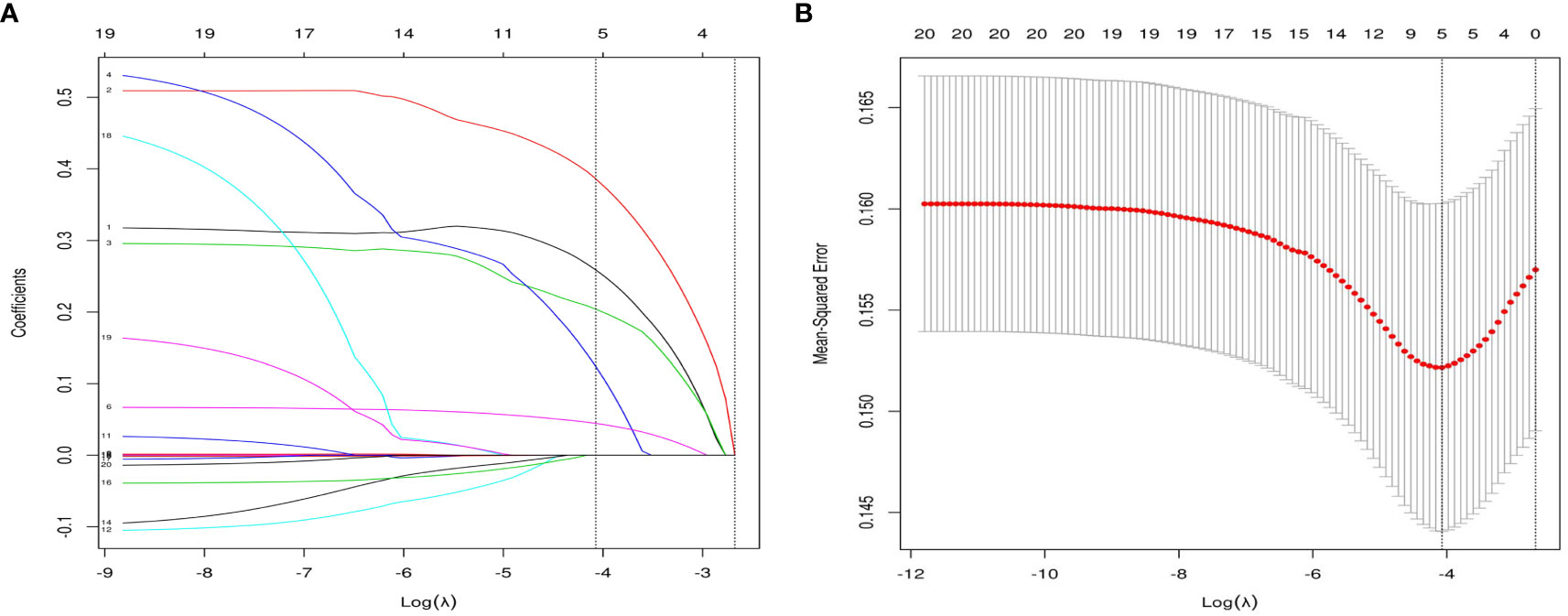
Figure 2 Variable selection by the LASSO binary logistic regression model. (A) eighteen variables with nonzero coefficients were selected by deriving the optimal lambda. (B) Following verification of the optimal parameter (λ) in the LASSO model, the mean squared error changes with respect to the Log (λ) value, and the vertical dotted line near Log (λ) = −4 is drawn based on 1 standard error criteria.
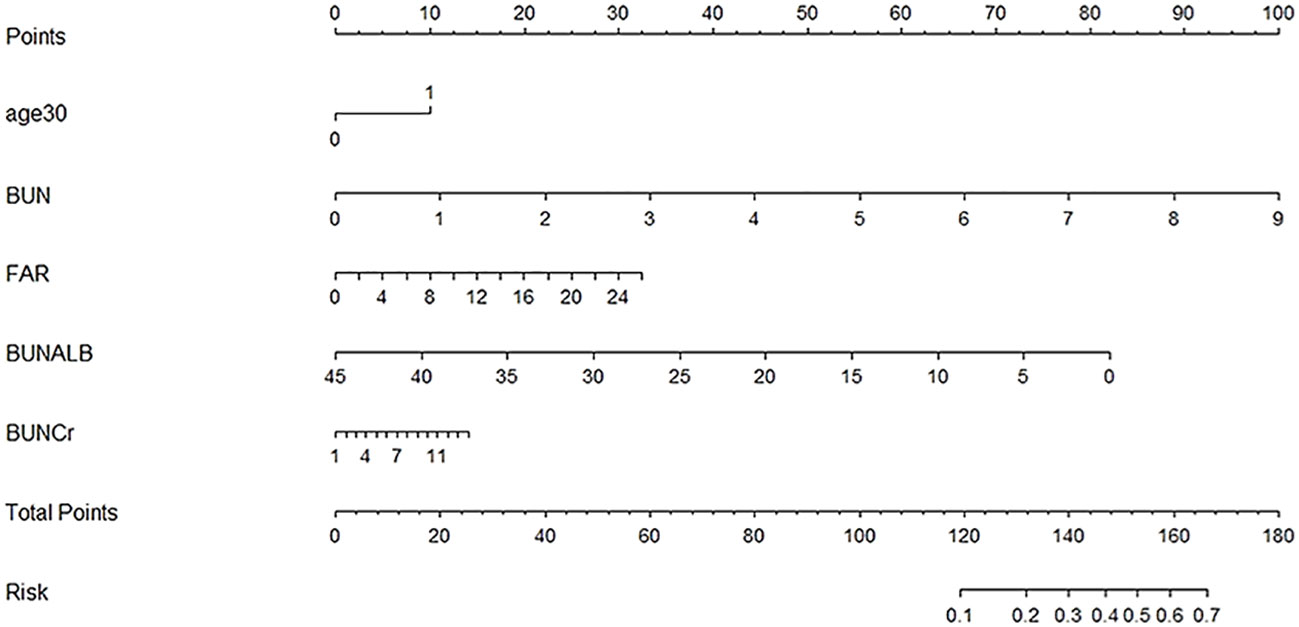
Figure 3 Nomogram to estimate the probability of GDM. A nomogram used basic pregnancy file information to predict GDM. Find the predictor points on the uppermost point scale that correspond to each variable of the pregnant woman and add them up; the total points projected to the bottom scale indicate the probability of GDM.
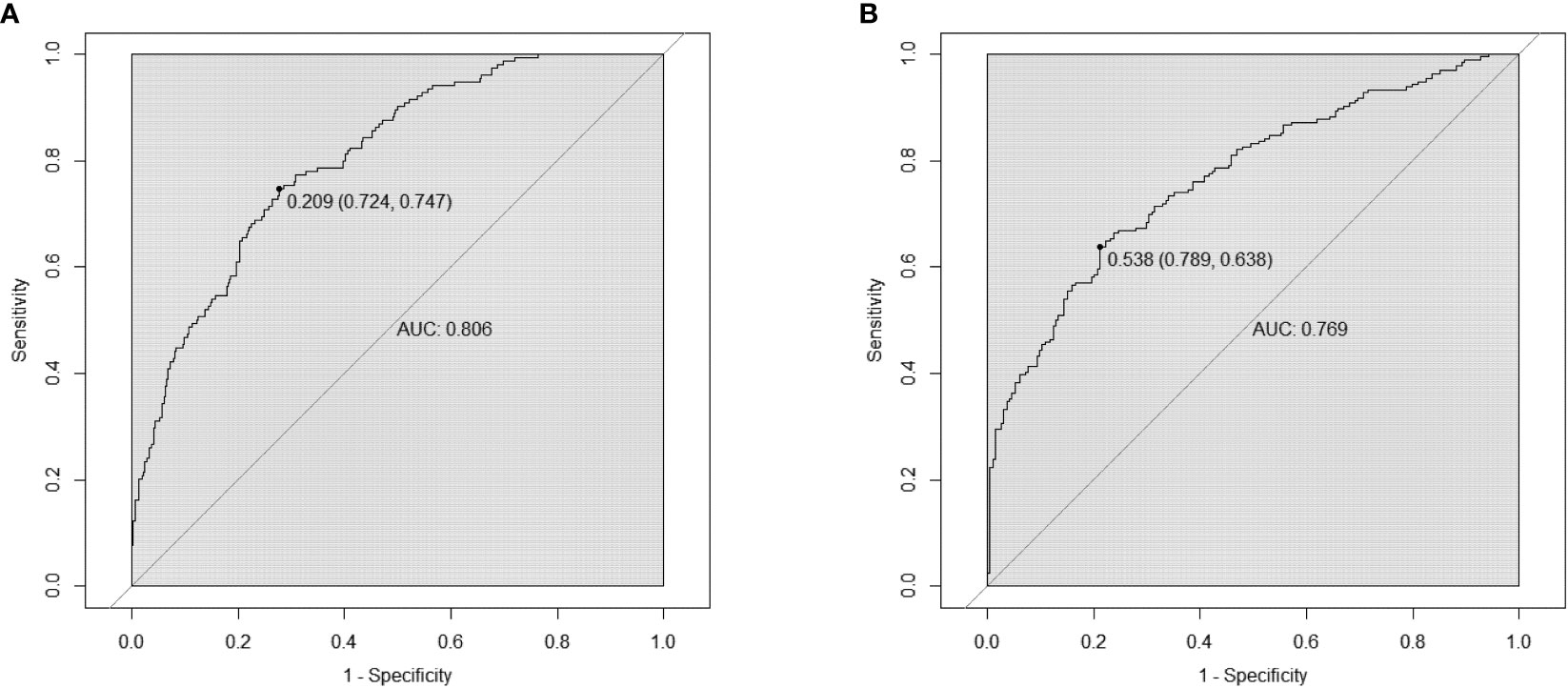
Figure 4 Receiver operating characteristic (ROC) curves of nomograms in the training dataset and validation dataset, respectively. (A) The AUC value of the training dataset is 0.808, 95% CI: 0.770–0.842 (p < 0.05). (B) The AUC value of the validation dataset is 0.769, 95% CI: 0.722–0.815 (p < 0.05).
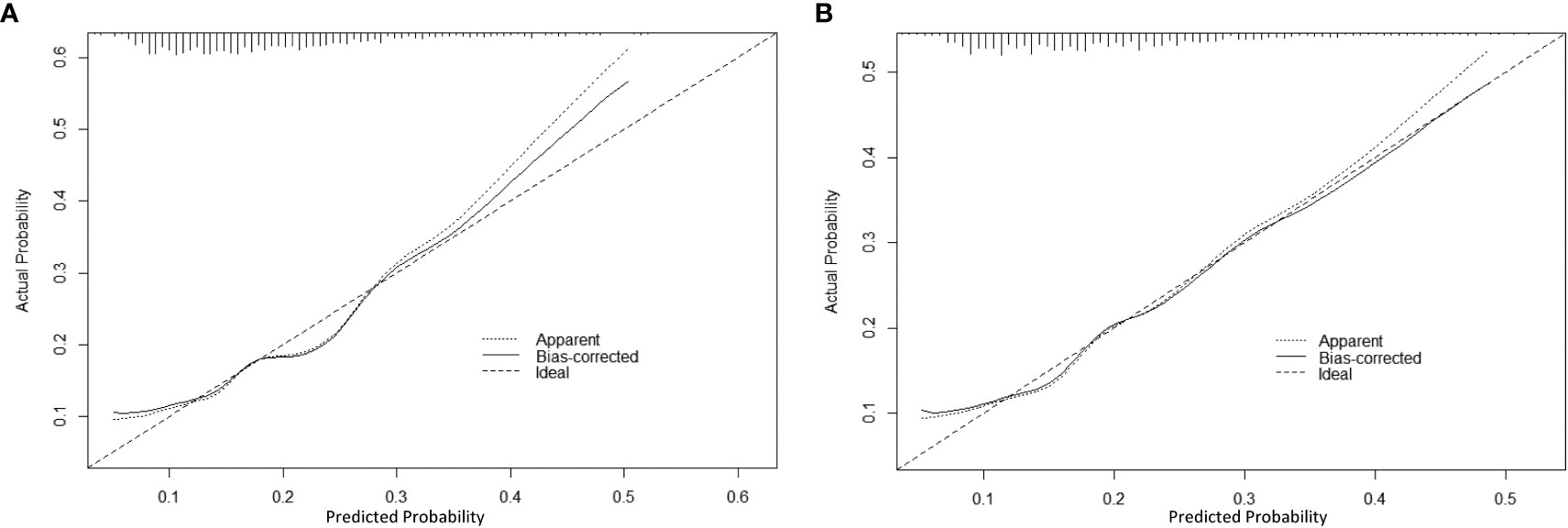
Figure 5 The calibration curve of the nomogram for predicting GDM in the training dataset and validation dataset, respectively. Calibration focused on the accuracy of the probability between the predictive model and the actually observed value. The y-axis represents the actual diagnosed cases of GDM, the x-axis represents the predicted risk of GDM, and the solid line represents the prediction of the training dataset (A) and the validation dataset (B).
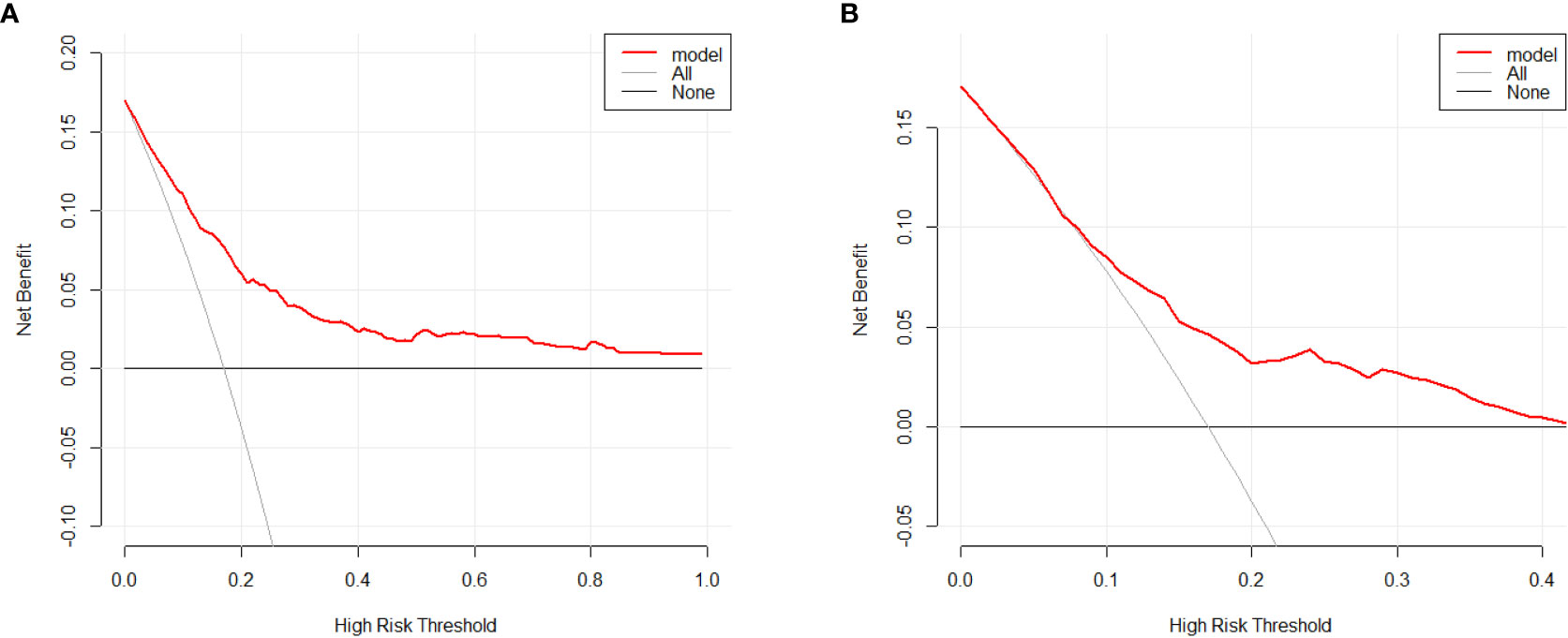
Figure 6 Decision curve analysis for the GDM risk nomogram. The y-axis estimates the net benefit, the transverse solid line represents the probability of risk that pregnant Asian women have no GDM, and the oblique solid line represents the probability of risk that pregnant Asian women have GDM. (A) Training dataset. (B) Validation dataset.
Discussion
GDM is defined as dysglycemia with onset or first recognition during pregnancy (20); insulin resistance and pancreatic β cell failure have been reported to be significant factors in GDM aside from the other main causes of GDM such as maternal age, obesity, inflammation, and inadequate physical exercise (21). GDM increases maternal and neonatal adverse effects in both short-term and long-term periods. In addition, it is necessary to identify and address the risk factors of GDM early and accurately. Tools that could accurately target these GDM predictors in early pregnant women will most likely benefit these women (22).
On the other hand, early warning and intervention during early pregnancy may prevent the adverse outcomes of GDM by controlling glucose level. The first-line treatment for GDM is medical nutrition therapy, weight management, and physical activities (23–25). to "70% to 85% of women diagnosed with GDM could modify their glucose condition through targeted lifestyle changes (26). In general, an early prediction model of GDM should be established, which could positively affect prevention, treatment, and prognosis.
Prior studies indicated that BUN was dose-response related with GDM during the first trimester (27). Diabetes mellitus drives the occurrence of kidney diseases (28). Meanwhile, kidney metabolites such as urea or other uremic components may increase the risk of diabetes (29). BUN was considered as a kidney function marker; a high level of urea increases insulin resistance and suppresses insulin secretion, which is associated with an increased risk of incident diabetes mellitus (30). The underlying mechanism is as follows: urea induced the production of reactive oxygen species and restrains insulin signaling by suppressing insulin receptor substrate–serine phosphorylation (31); on the other hand, uremic metabolite accumulation impaired β-cell normal function and negatively affected glucose homeostasis (32).
Meanwhile, fibrinogen is a long-acting plasma acute-phase reactant (33), and the change in albumin level has been attributed to the changes in nutritional status; furthermore, hypoalbuminemia represents a chronic inflammatory state caused by malnutrition (33, 34). Likewise, FAR has been proven to be a more powerful inflammatory-based prognostic predictor of overall survival than other single prognostic markers (35–37); compared with healthy pregnancies, FAR was considered to be an independent risk factor for predicting spontaneous abortion, and increased FAR levels were considered to be related to the thrombotic process in recurrent abortion (38, 39). BUN/Cr is an important indicator to evaluate acute renal injury and gastrointestinal hemorrhage, and a low BUN/Cr level is associated with higher risks of total and ischemic stroke (40–42). BUN/ALB is a novel prognostic marker that has a higher predictive ability than single urea nitrogen and albumin in pneumonia and acute pulmonary embolism (43–46). Given the low cost and the abundance of laboratory offerings, and the fact that these markers provided poor clinical outcomes in previous studies, we generated a predictive nomogram of GDM through serial measures.
From an economics perspective, our study takes advantage of early pregnancy files and validates the nomogram that was set up for 824 enrolled pregnant women. In this multicenter study, we have identified five predictors, namely, age, BUN, FAR, BUN/ALB, and BUN/Cr, which were significantly associated with GDM. These five predictors are independent of each other, and research about their relationship has rarely been reported. We also developed a nomogram that could predict the incidence of GDM during early pregnancy.
Our study has strengths and limitations; this is a multicenter retrospective study with a large sample size of pregnant women, and we used an early pregnant stage dataset verified by the nomogram. The GDM predictive nomogram focused on several clinical factors which could be readily available at low cost via routine blood tests in clinical practice, and the nomogram can be performed with optimal predictive power with better combined clinical characteristics with laboratory results. This model can be widely used in less-developed and developing countries where the incidence of GDM is rapidly increasing. It provides risk assessment based on first pregnancy profiles for early detection and intervention and to control glucose level. Thus, it should be widely carried out in more basic-level hospitals. However, many factors should be considered first. We should expand the sample size via dynamic monitoring of different gestational weeks and detect more variables and risk factors during pregnancy before the model can be widely used in clinical practice.
In summary, by analyzing basic information from pregnancy files, we found five independent risk factors of GDM: age, BUN, FAR, BUN/Cr, and BUN/ALB. According to the GDM nomogram predictive model validated by the early pregnancy dataset, we could help patients’ clinical management at the early gestational stage.
Data availability statement
The datasets presented in this article are not readily available due to the nature of this research, participants of this study did not agree for their data to be shared publicly, so supporting data is not available. Requests to access the datasets should be directed toemhhbmd5dWFuemhlbkB3aHUuZWR1LmNu.
Ethics statement
The study was approved by the Institutional Ethics Committee of the Zhongnan Hospital of Wuhan University and the Maternal and Child Health Hospital of Hubei Province, the informed consent number was No. 2020072K.
Author contributions
LL wrote the first draft of the manuscript. LL, S-ML, and YZ contributed to the conception and design of the study. YZ is the first corresponding author. QZ, ZW, and FT collected the data. LL, QZ, and HL performed the data processing and analysis. YT contributed to the critical revision of the manuscript. All authors contributed to manuscript revision, and read and approved the submitted version.
Funding
The study was supported by National Natural Science Foundation of China (82172359, 81972009) and Zhongnan Hospital of Wuhan University Science, Technology and Innovation Seed Fund (No. ZNPY2019057).
Acknowledgments
We thank all the patients who have participated in this study.
Conflict of interest
The authors declare that the research was conducted in the absence of any commercial or financial relationships that could be construed as a potential conflict of interest.
Publisher’s note
All claims expressed in this article are solely those of the authors and do not necessarily represent those of their affiliated organizations, or those of the publisher, the editors and the reviewers. Any product that may be evaluated in this article, or claim that may be made by its manufacturer, is not guaranteed or endorsed by the publisher.
References
1. Hod M, Kapur A, Sacks DA, Hadar E, Agarwal M, Di Renzo GC, et al. The international federation of gynecology and obstetrics (Figo) initiative on gestational diabetes mellitus: A pragmatic guide for diagnosis, management, and care. Int J Gynaecol Obstet (2015) 131 Suppl 3:S173–211. doi: 10.1016/s0020-7292(15)30033-3
2. Lavery JA, Friedman AM, Keyes KM, Wright JD, Ananth CV. Gestational diabetes in the united states: Temporal changes in prevalence rates between 1979 and 2010. Bjog (2017) 124(5):804–13. doi: 10.1111/1471-0528.14236
3. McIntyre HD, Catalano P, Zhang C, Desoye G, Mathiesen ER, Damm P. Gestational diabetes mellitus. Nat Rev Dis Primers (2019) 5(1):47. doi: 10.1038/s41572-019-0098-8
4. Ying W, Fu W, Lee YS, Olefsky JM. The role of macrophages in obesity-associated islet inflammation and β-cell abnormalities. Nat Rev Endocrinol (2020) 16(2):81–90. doi: 10.1038/s41574-019-0286-3
5. Kurtzhals LL, Nørgaard SK, Secher AL, Nichum VL, Ronneby H, Tabor A, et al. The impact of restricted gestational weight gain by dietary intervention on fetal growth in women with gestational diabetes mellitus. Diabetologia (2018) 61(12):2528–38. doi: 10.1007/s00125-018-4736-6
6. Wei X, Song H, Yin L, Rizzo MG, Sidhu R, Covey DF, et al. Fatty acid synthesis configures the plasma membrane for inflammation in diabetes. Nature (2016) 539(7628):294–8. doi: 10.1038/nature20117
7. Hillier TA, Pedula KL, Schmidt MM, Mullen JA, Charles MA, Pettitt DJ. Childhood obesity and metabolic imprinting: The ongoing effects of maternal hyperglycemia. Diabetes Care (2007) 30(9):2287–92. doi: 10.2337/dc06-2361
8. Hillier TA, Pedula KL, Ogasawara KK, Vesco KK, Oshiro CES, Lubarsky SL, et al. A pragmatic, randomized clinical trial of gestational diabetes screening. N Engl J Med (2021) 384(10):895–904. doi: 10.1056/NEJMoa2026028
9. 2. classification and diagnosis of diabetes: Standards of medical care in diabetes-2021. Diabetes Care (2021) 44(Suppl 1):S15–s33. doi: 10.2337/dc21-S002
10. Yuen L, Wong VW. Gestational diabetes mellitus: Challenges for different ethnic groups. World J Diabetes (2015) 6(8):1024–32. doi: 10.4239/wjd.v6.i8.1024
11. Chamberlain JJ, Doyle-Delgado K, Peterson L, Skolnik N. Diabetes technology: Review of the 2019 american diabetes association standards of medical care in diabetes. Ann Intern Med (2019) 171(6):415–20. doi: 10.7326/m19-1638
12. Lende M, Rijhsinghani A. Gestational diabetes: Overview with emphasis on medical management. Int J Environ Res Public Health (2020) 17(24):9573. doi: 10.3390/ijerph17249573
13. Koning SH, van Zanden JJ, Hoogenberg K, Lutgers HL, Klomp AW, Korteweg FJ, et al. New diagnostic criteria for gestational diabetes mellitus and their impact on the number of diagnoses and pregnancy outcomes. Diabetologia (2018) 61(4):800–9. doi: 10.1007/s00125-017-4506-x
14. Landon MB, Spong CY, Thom E, Carpenter MW, Ramin SM, Casey B, et al. A multicenter, randomized trial of treatment for mild gestational diabetes. N Engl J Med (2009) 361(14):1339–48. doi: 10.1056/NEJMoa0902430
15. Harrison J, Melov S, Kirby AC, Athayde N, Boghossian A, Cheung W, et al. Pregnancy outcomes in women with gestational diabetes mellitus by models of care: A retrospective cohort study. BMJ Open (2022) 12(9):e065063. doi: 10.1136/bmjopen-2022-065063
16. Practice bulletin no. 163 summary: Screening for fetal aneuploidy. Obstet Gynecol (2016) 127(5):979–81. doi: 10.1097/aog.0000000000001439
17. Metzger BE, Gabbe SG, Persson B, Buchanan TA, Catalano PA, Damm P, et al. International association of diabetes and pregnancy study groups recommendations on the diagnosis and classification of hyperglycemia in pregnancy. Diabetes Care (2010) 33(3):676–82. doi: 10.2337/dc09-1848
18. Friedman J, Hastie T, Tibshirani R. Regularization paths for generalized linear models Via coordinate descent. J Stat Softw (2010) 33(1):1–22.
19. Riley RD, Ensor J, Snell KIE, Harrell FE Jr., Martin GP, Reitsma JB, et al. Calculating the sample size required for developing a clinical prediction model. Bmj (2020) 368:m441. doi: 10.1136/bmj.m441
20. National Diabetes Data Group. Classification and diagnosis of diabetes mellitus and other categories of glucose intolerance. Diabetes (1979) 28(12):1039–57. doi: 10.2337/diab.28.12.1039
21. Lekva T, Norwitz ER, Aukrust P, Ueland T. Impact of systemic inflammation on the progression of gestational diabetes mellitus. Curr Diabetes Rep (2016) 16(4):26. doi: 10.1007/s11892-016-0715-9
22. White SL, Lawlor DA, Briley AL, Godfrey KM, Nelson SM, Oteng-Ntim E, et al. Early antenatal prediction of gestational diabetes in obese women: Development of prediction tools for targeted intervention. PloS One (2016) 11(12):e0167846. doi: 10.1371/journal.pone.0167846
23. Rasmussen L, Poulsen CW, Kampmann U, Smedegaard SB, Ovesen PG, Fuglsang J. Diet and healthy lifestyle in the management of gestational diabetes mellitus. Nutrients (2020) 12(10):3050. doi: 10.3390/nu12103050
24. Yamamoto JM, Kellett JE, Balsells M, García-Patterson A, Hadar E, Solà I, et al. Gestational diabetes mellitus and diet: A systematic review and meta-analysis of randomized controlled trials examining the impact of modified dietary interventions on maternal glucose control and neonatal birth weight. Diabetes Care (2018) 41(7):1346–61. doi: 10.2337/dc18-0102
25. 14. management of diabetes in pregnancy: Standards of medical care in diabetes-2020. Diabetes Care (2020) 43(Suppl 1):S183–s92. doi: 10.2337/dc20-S014
26. 13. management of diabetes in pregnancy. Diabetes Care (2017) 40(Suppl 1):S114–s9. doi: 10.2337/dc17-S016
27. Feng P, Wang G, Yu Q, Zhu W, Zhong C. First-trimester blood urea nitrogen and risk of gestational diabetes mellitus. J Cell Mol Med (2020) 24(4):2416–22. doi: 10.1111/jcmm.14924
28. Lai S. Chronic kidney disease and diabetes-a potential causal link. EBioMedicine (2016) 6:10–1. doi: 10.1016/j.ebiom.2016.03.025
29. Allison SJ. Diabetes: Urea inhibits insulin secretion in ckd. Nat Rev Nephrol (2016) 12(10):581. doi: 10.1038/nrneph.2016.131
30. Xie Y, Bowe B, Li T, Xian H, Yan Y, Al-Aly Z. Higher blood urea nitrogen is associated with increased risk of incident diabetes mellitus. Kidney Int (2018) 93(3):741–52. doi: 10.1016/j.kint.2017.08.033
31. Jha JC, Banal C, Chow BS, Cooper ME, Jandeleit-Dahm K. Diabetes and kidney disease: Role of oxidative stress. Antioxid Redox Signal (2016) 25(12):657–84. doi: 10.1089/ars.2016.6664
32. Koppe L, Nyam E, Vivot K, Manning Fox JE, Dai XQ, Nguyen BN, et al. Urea impairs β cell glycolysis and insulin secretion in chronic kidney disease. J Clin Invest (2016) 126(9):3598–612. doi: 10.1172/jci86181
33. Amaro E, Moore-Lotridge SN, Wessinger B, Benvenuti MA, An TJ, Oelsner WK, et al. Albumin and the fibrinogen-to-Albumin ratio: Biomarkers for the acute phase response following total knee arthroplasty. PloS One (2021) 16(2):e0247070. doi: 10.1371/journal.pone.0247070
34. Rothschild MA, Oratz M, Mongelli J, Schreiber SS. Effects of a short-term fast on albumin synthesis studied in vivo, in the perfused liver, and on amino acid incorporation by hepatic microsomes. J Clin Invest (1968) 47(12):2591–9. doi: 10.1172/jci105941
35. Wang Y, Xu W, Wang Y. Prognostic role of preoperative fibrinogen to albumin ratio in breast cancer. Clin Chim Acta (2020) 510:360–2. doi: 10.1016/j.cca.2020.07.055
36. Zhang J, Ding Y, Wang W, Lu Y, Wang H, Wang H, et al. Combining the Fibrinogen/Albumin ratio and systemic inflammation response index predicts survival in resectable gastric cancer. Gastroenterol Res Pract (2020) 2020:3207345. doi: 10.1155/2020/3207345
37. Xu Q, Yan Y, Gu S, Mao K, Zhang J, Huang P, et al. A novel inflammation-based prognostic score: The Fibrinogen/Albumin ratio predicts prognoses of patients after curative resection for hepatocellular carcinoma. J Immunol Res (2018) 2018:4925498. doi: 10.1155/2018/4925498
38. Usta CS, Atik TK, Ozcaglayan R, Bulbul CB, Camili FE, Adali E. Does the Fibrinogen/Albumin ratio predict the prognosis of pregnancies with abortus imminens? Saudi Med J (2021) 42(3):255–63. doi: 10.15537/smj.2021.42.3.20200695
39. Cimsir MT, Yildiz MS. Could fibrinogen to albumin ratio be a predictive marker for recurrent pregnancy loss. Int J Clin Pract (2021) 75(10):e14520. doi: 10.1111/ijcp.14520
40. Lameire N, Van Biesen W, Vanholder R. Acute renal failure. Lancet (2005) 365(9457):417–30. doi: 10.1016/s0140-6736(05)17831-3
41. Deng L, Wang C, Qiu S, Bian H, Wang L, Li Y, et al. Association between blood urea nitrogen-to-Creatinine ratio and three-month outcome in patients with acute ischemic stroke. Curr Neurovasc Res (2019) 16(2):166–72. doi: 10.2174/1567202616666190412123705
42. Peng R, Liu K, Li W, Yuan Y, Niu R, Zhou L, et al. Blood urea nitrogen, blood urea nitrogen to creatinine ratio and incident stroke: The dongfeng-tongji cohort. Atherosclerosis (2021) 333:1–8. doi: 10.1016/j.atherosclerosis.2021.08.011
43. Ryu S, Oh SK, Cho SU, You Y, Park JS, Min JH, et al. Utility of the blood urea nitrogen to serum albumin ratio as a prognostic factor of mortality in aspiration pneumonia patients. Am J Emerg Med (2021) 43:175–9. doi: 10.1016/j.ajem.2020.02.045
44. Fang J, Xu B. Blood urea nitrogen to serum albumin ratio independently predicts mortality in critically ill patients with acute pulmonary embolism. Clin Appl Thromb Hemost (2021) 27:10760296211010241. doi: 10.1177/10760296211010241
45. Küçükceran K, Ayrancı MK, Girişgin AS, Koçak S, Dündar ZD. The role of the Bun/Albumin ratio in predicting mortality in covid-19 patients in the emergency department. Am J Emerg Med (2021) 48:33–7. doi: 10.1016/j.ajem.2021.03.090
Keywords: GDM, nomogram, validation, prediction model, early pregnancy term
Citation: Li L, Zhu Q, Wang Z, Tao Y, Liu H, Tang F, Liu S-M and Zhang Y (2023) Establishment and validation of a predictive nomogram for gestational diabetes mellitus during early pregnancy term: A retrospective study. Front. Endocrinol. 14:1087994. doi: 10.3389/fendo.2023.1087994
Received: 02 November 2022; Accepted: 26 January 2023;
Published: 24 February 2023.
Edited by:
Giulio Frontino, San Raffaele Hospital (IRCCS), ItalyReviewed by:
Runyu Du, Sheng Jing Hospital Affiliated to China Medical University, ChinaAmelia Caretto, San Raffaele Hospital (IRCCS) Italy
Copyright © 2023 Li, Zhu, Wang, Tao, Liu, Tang, Liu and Zhang. This is an open-access article distributed under the terms of the Creative Commons Attribution License (CC BY). The use, distribution or reproduction in other forums is permitted, provided the original author(s) and the copyright owner(s) are credited and that the original publication in this journal is cited, in accordance with accepted academic practice. No use, distribution or reproduction is permitted which does not comply with these terms.
*Correspondence: Yuanzhen Zhang, emhhbmd5dWFuemhlbkB3aHUuZWR1LmNu; Song-Mei Liu, c21saXVAd2h1LmVkdS5jbg==