Evaluation of Cropland System Resilience to Climate Change at Municipal Scale Through Robustness, Adaptability, and Transformability: A Case Study of Hubei Province, China
- 1College of Public Administration, Central China Normal University, Wuhan, China
- 2Institute of Nature Resources Governance, Central China Normal University, Wuhan, China
- 3College of Economics and Management, Huazhong Agricultural University, Wuhan, China
- 4College of Public Administration, Huazhong University of Science and Technology, Wuhan, China
A cropland system is one of the most sensitive socio-ecological systems to climate change, such as drought and flood. Facing frequent extreme weather events worldwide, how to improve cropland system resilience to climate change (CSRCC) and thus ensure food production has been concerned. Although a small number of studies have attempted to evaluate CSRCC through single or multiple indicators, few studies have considered the perspective of the three basic capacities of resilience (i.e., robustness, adaptability, and transformability), which could ignore the dynamic characteristics of cropland system resilience against shocks within a certain period. Therefore, this study first constructs an evaluation index system from the three capacities of system resilience. Then, taking Hubei province, China, as a case and comprehensively using the methods of Delphi, AHP, and TOPSIS to assess the spatio-temporal characteristics of CSRCC at the municipal scale from 2011 to 2018. On this basis, the regional disparities of CSRCC are analyzed by using the Theil coefficient. The results show that the CSRCC of Hubei province fluctuates on a downward trend, with the lowest in 2017 and the highest in 2013. Most municipalities have witnessed a pattern of fluctuated decline, except for a few ones in the plains, such as Wuhan and Jingmen. Generally, municipalities in the plains have greater scores, while some municipalities in the southern and eastern hilly regions show higher adaptability and transformability. In addition, adaptability contributes the least to the CSRCC at the municipal scale. At last, indicator selection against different research objects, influencing mechanism of CSRCC, and policy implications are discussed. This study is expected to provide a reference for the practice in sustainable management and utilization of cropland systems.
Introduction
A cropland system is a socio-ecological system formed by the coupling interaction of cropland resources and human activities. Globally, the cropland system has been facing a series of environmental (Wang C. et al., 2021), socio-economic (Ullah and Uddin, 2021), and institutional (Niedertscheider et al., 2014) disturbances and shocks. For example, plant diseases (Gokulnath and Devi, 2021), insect pests (Gaur and Mogalapu, 2018), changes in market access (e.g., trade war and policy barriers) (He et al., 2019), land-use policies, and management measures (Mittenzwei and Øygarden, 2019) constantly affect agricultural production of the cropland system. Especially, extreme weather (such as drought, flood, and frost) caused by climate change has the most extensive and severe impacts on the cropland system (Kotzee and Reyers, 2016; Rey et al., 2017; Bombi, 2018; Yu and Wu, 2018). Between 1961 and 2010, two-thirds of the world’s cropland systems were significantly affected by at least one large-scale climate oscillation (Heino et al., 2018). The measures taken on the cropland system to address these problems may exacerbate or buffer the impact of these challenges. The ability of the cropland system to cope with risks and maintain its stability during various disturbances and shocks is considered as cropland system resilience, which reflects the complex interaction between cropland resources and their utilizers in a specific socio-geographical environment.
Resilience emphasizes the variation, uncertainty, and ability of a system to adapt to the environment (Gunderson and Holling, 2002). The concept is multifaceted and should not be determined by a single indicator or by only observing the properties of a single system (Meuwissen et al., 2019). Previous studies indicate that the concept of robustness, adaptability, and transformability are the three core capacities of system resilience, which is more composite than other similar concepts, such as vulnerability, flexibility, or adaption (Buitenhuis et al., 2020). For a specific cropland system, its resilience to climate change should involve any or all of the following (Figure 1): Robustness is the capacity to withstand the transient shock to furthest maintain the original productivity (Urruty et al., 2016); adaptability is the capacity to make full use of the existing resources to restore the original productivity after the shock, without changing the internal structure and mechanism of the cropland system (Buitenhuis et al., 2020); and transformability is the capacity of a cropland system to improve itself to cope with climate change through structural and institutional changes, or collapse due to the failure to make changes in time (Meuwissen et al., 2019). It should be emphasized that among the three capacities of CSRCC, robustness can play a role in a short period, while adaptability and transformability may only be fully manifested for a long period after a disaster occurs.
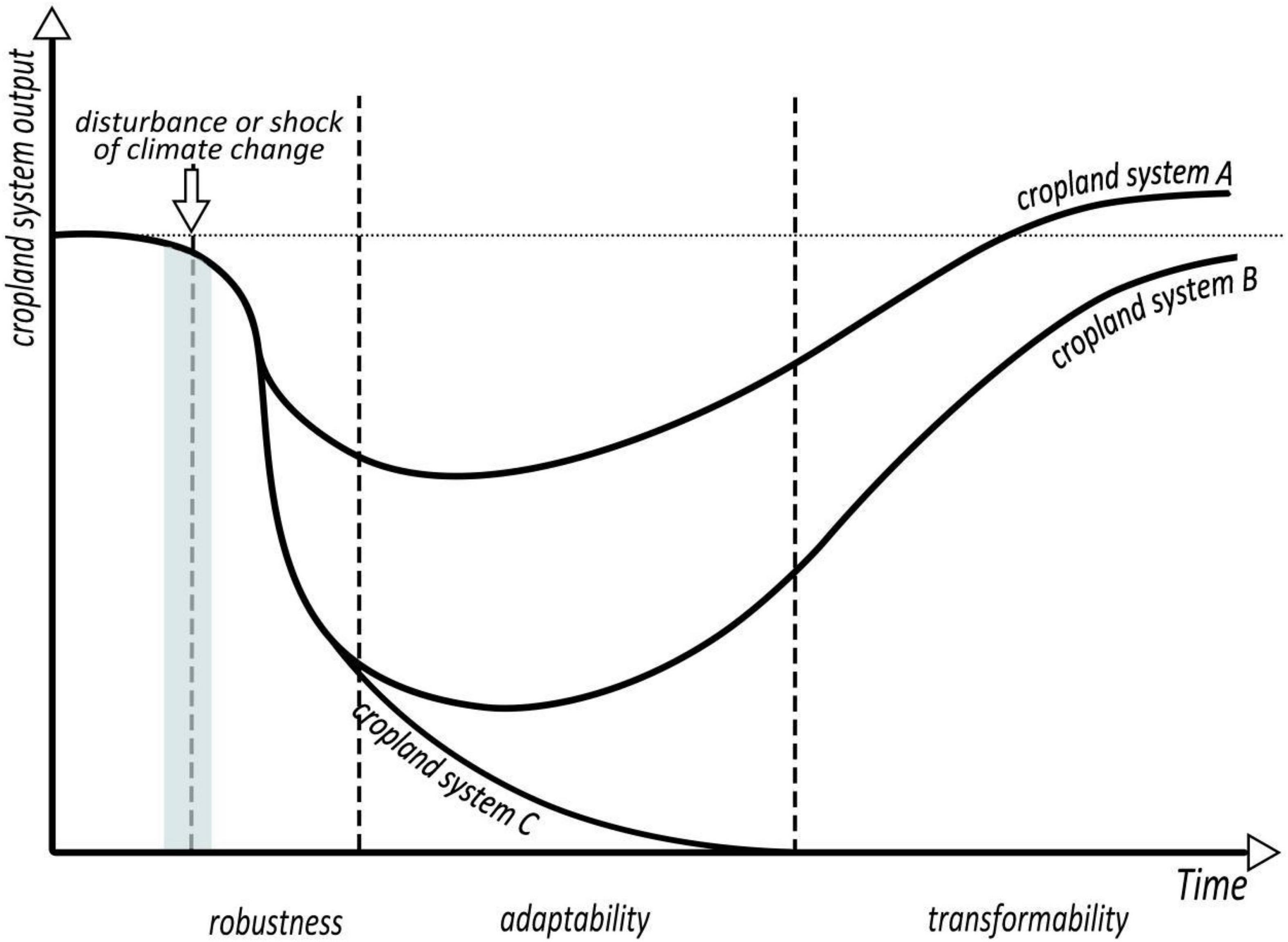
Figure 1. Schematic diagram of the three capabilities of cropland system resilience. (Cropland system A has superior resilience, due to the less impact on the phases of robustness and adaptability and even higher output in the phase of transformability than the original level. Cropland system B has medium resilience, due to more significantly fluctuated output after the shock and longer recovery time than the original level. Cropland system C has the lowest resilience due to the collapse after the shock).
Previous research studies on the evaluation of socio-ecological resilience mainly focus on the urban system (Bush and Doyon, 2019; Cariolet et al., 2019; Datola et al., 2022; Potapova et al., 2022; Wang et al., 2022), agricultural system (Gil et al., 2017), or food system (Tendall et al., 2015; Jacobi et al., 2018). Few studied CSRCC, which mainly centralized on the qualitative analysis of the definitions (Meyer, 2020) or connotations (Tendall et al., 2015). These studies discussed potential influential factors of CSRCC and how policies or measures can be developed to improve CSRCC (Egli et al., 2021; Javadinejad et al., 2021). Although less attention has been paid to the evaluation of CSRCC, we can refer to research on similar socio-ecological system resilience, such as farming system resilience and agricultural system resilience. Some evaluated socio-ecological resilience through a single indicator (Zampieri et al., 2021), fuzzy assessment based on an index system (Mutabazi et al., 2015; Zampieri et al., 2020), and decision behavior modeling (Badillo-Márquez et al., 2021; Herrera et al., 2022). Other studies attempted to innovate the theoretical framework for the evaluation (Altieri et al., 2015). For example, a recent study by Lyu et al. (2021) proposed an assessment framework based on cropland resource resilience, ecological resilience, production resilience, and scale structural resilience. Generally, previous research studies have evaluated cropland system resilience from various socio-ecological dimensions. However, few studies have considered the three capacities of system resilience, namely, robustness, adaptability, and transformability.
Climate change has substantially increased the frequency of extreme weather events, and it is considered one of the most serious threats to the cropland system around the world. In this context, improving CSRCC is crucial to realize the sustainable utilization of cropland, high-quality agricultural development, and regional food security. This study takes the Hubei province of China as an example and constructs the evaluation index system based on robustness, adaptability, and transformability. Methods of Delphi, AHP, and TOPSIS are used to measure the spatio-temporal characteristics of CSRCC in each municipality of Hubei province from 2011 to 2018. The regional disparities are analyzed by using the Theil coefficient.
Methods and Data
Study Area
Hubei is located in central China and the middle reaches of the Yangtze River, between north latitude 29° 01′ 53 ″–33° 6′ 47″ and east longitude 108° 21′ 42 ″–116° 07′ 50″, which is roughly surrounded by mountains on three sides (east, west, and north) and is low and flat in the middle (Figure 2). Currently, Hubei has jurisdictions over 12 prefecture-level municipalities and three provincial governing county-level municipalities, with a total area of 1.86 × 105 km2. It is rich in natural endowments and is an important agricultural commodity base of China. By the end of 2019, the cropland area of Hubei reached 4.77 × 104 km2, which was mainly distributed in the central plain. Cropland with a slope above 25° accounted for 2.31% of the total cropland area. Among them, the sloping cultivated land in mountainous areas accounted for more than 85%. Meanwhile, drastic climate change has caused constant disasters of drought and flood in Hubei.
Methods
Evaluation Index System of Cropland System Resilience to Climate Change
Climate change, such as drought and flood, has been affecting Chinese cropland use and food production dramatically for a long time. To evaluate CSRCC, this study fully considers the impacts of droughts and floods caused by climate change on the cropland system, as well as the human–land coupling relation; takes the three capacities of socio-economic system resilience, namely, the robustness, adaptability, and transformability, as the criterion layer; and selects indicators that are directly or indirectly related to cropland system resilience against drought and flood (Figure 3).
Robustness is measured by four indicators: the proportion of irrigated land area, cropland area loss rate, proportion of the valid irrigation area, and proportion of sloping cropland. Specifically, (1) irrigated land refers to the cropland with water sources and irrigation facilities to protect against drought. Thus, when dealing with droughts, irrigated land can alleviate the disaster more quickly than other croplands (Meza et al., 2020). Crops can be irrigated in time, thereby ensuring the survival rate and growing environment (Ray et al., 2018). For a certain cropland system of an administration unit, the higher the proportion of irrigated land, the higher the robustness. (2) Cropland area loss will directly lead to a decline in the agricultural output regionally and a decrease in the production function of the cropland system (Chen et al., 2019). Cropland area loss caused by construction land occupation, abandonment, or non-plantation use results in local cropland system collapse (Deng et al., 2020; Vallés-Planells et al., 2020), thereby reducing the whole CSRCC. (3) The valid irrigation area is a relatively flat cropland area with certain water sources and supporting irrigation facilities (Liu et al., 2020), which provide cropland with the possibility of resisting drought. (4) Sloping cropland indicates the croplands with a slope above 25 degrees. Those lands are not conducive to soil growth, and the soil layer is weak. Moreover, soil erosion caused by disasters, such as flood, can easily damage the sloping cropland (Tuo et al., 2018; Peng et al., 2019). Thus, the higher the proportion of sloping cropland, the lower the robustness.
Adaptability is measured by considering water pumps per unit cropland area, the proportion of electromechanical irrigation area, the growth of per capita disposable income of rural residents, and the average cropland patch size. Specifically, (1) a water pump is an agricultural machine that increases the efficiency of drainage or irrigation. When floods or droughts occur, a cropland system with more pumps per unit area of cropland can restore production more quickly and the system can recover agricultural production rapidly, so the adaptability will be stronger (Amaranto et al., 2018). (2) The proportion of electromechanical irrigation area indicates the cropland irrigated by using diesel engines, gasoline engines, electric motors, or other power machinery in the effective irrigation area. Electromechanical irrigation, rather than traditional irrigation, can improve irrigation efficiency and relieve water stress in case of drought (Liu et al., 2021). (3) The growth of per capita disposable income of rural residents has a direct relation to fast production recovery after disasters. Increased income indicates more capital that can be reinvested in the restoration of agricultural production (Borychowski et al., 2020; Dixon et al.,2021), thereby increasing adaptability. (4) Average cropland patch size is related to the patch area and the number of patches. In large patches, cropland is more concentrated and generally flat, which is conducive to mechanical operation, labor force saving, and the speed of cropland recovery (Wang T. et al., 2021). A large number of plaques in a certain area will be unfavorable for large-scale mechanized farming.
Transformability is measured by the growth of road area, number of agricultural machinery service institutions per unit of cropland area, rural labor loss, and dispersion of cropland patch. Specifically, (1) the road area includes both urban and rural roads. The role of roads in enhancing the cropland system resilience is promoting the exchange of urban and rural means of production, including labor, agricultural facilities, and technology (Vishnu et al., 2021). From this point of view, the increase in the road area is conducive to the introduction of elements that can renew the cropland system after a disaster (Kasmalkar et al., 2020). (2) Mechanized service organizations can provide technical guidance to farmers. The improvement of agricultural production technology will be beneficial to the adjustment of cropland system structure, the improvement of production efficiency, and the guidance on disaster management, thereby maintaining or even improving the production capacity of the cropland system (Van Loon et al., 2020; Batung et al., 2022). (3) Rural labor loss could directly lead to the cropland abandonment and the reduction of the input of the cropland system. The shortage of rural labor will seriously affect the allocation of labor and reduce the efficiency of disaster relief (Zhou et al., 2021). Therefore, the greater the rural labor loss, the weaker the transformability of the cropland system. (4) Dispersion of cropland patch measures the discreteness of cropland by calculating the weighted distance between the central points of each patch. The smaller the patch dispersion, the more the cropland conducive to the mechanical and human communication between the cropland patches and the higher the disaster relief efficiency and the transformability of the cropland system (Frei et al., 2020).
Index Weight Determination
The Delphi method is essentially a feedback anonymous inquiry method. Through repeated consultation with experts, a consensus is finally reached. The analytic hierarchy process (AHP) is a decision analysis method that combines qualitative and quantitative attributes. In this study, two methods are used comprehensively for the determination of index weights. Experts with relevant disciplinary backgrounds are invited to judge the importance of the criterion layer and the index layer and finally weighted. Thus, the thinking process is simple and clear, and the decision-making process is organized and more scientific.
In order to determine the weights of 12 indexes corresponding to robustness, adaptability, and transformability in the evaluation system of CSRCC, 16 experts in land resource management, environmental geography, public administration, and landscape ecology were invited for this study. In the case of no communication with each other, experts compared and scored the importance of each index relative to the higher level index to which it belongs. According to the pairwise judgment matrix constructed by expert scoring, the geometric mean is calculated and normalized. Through repeated scoring by experts, the matrix passes the consistency test, and finally, the weights of the primary and secondary indicators are obtained. The formula is as follows:
where A is the judgment matrix that passes the consistency test and n is the index number. Finally, the weights of each item in the criterion layer and the index layer are calculated (Table 1).
Calculation of Cropland System Resilience to Climate Change Based on TOPSIS
The Technique for Order Preference by Similarity to an Ideal Solution (TOPSIS) is a method that sorts evaluation objects by detecting the distance between the ideal solution and the anti-ideal solution. It can achieve the goal of replacing the optimal solution with a satisfactory solution by eliminating the poor solution and selecting a satisfactory solution (Wa̧tróbski et al., 2022). For example, the evaluation object that is closest to the ideal solution is considered the ideal solution.
(1) Standardize panel data:
The standardized calculation formulas for the positive index and the negative index are as given as follows:
where xmax(j) and xmin(j) are the maximum and minimum values of the jth evaluation index, respectively, and i is the ith evaluation unit.
(2) Build a standardized decision matrix:
The X matrix is normalized to obtain the normalized matrix Y. The weight matrix W is obtained from the weight calculation result of the evaluation index. The normalization matrix Y and the weight matrix W calculate the weight normalization matrix V, namely, the comprehensive value of the resilience level of the cropland system. There are N municipalities, and each city has M evaluation indicators. The xij represents the jth index value of ith municipality. The initial decision matrix X, normalized matrix Y, weight matrix W, and decision value matrix V are expressed, respectively, as follows:
(3) Calculate the ideal solutions and the anti-ideal solutions:
The ideal solution A+ and the anti-ideal solution A are determined by the weight normalization value of vij:
(4) Calculate the distance scale:
where S+ is the distance from the target to the ideal solution A+; S− is the distance to the anti-ideal solution A−; V+ and V− represent the distance between the jth target and the ideal solution and the anti-ideal solution, respectively; vij represents the normalized value of the ith target and the jth target; and S+ indicates the proximity between the evaluation target and the optimal target; the smaller the S+value, the better the results.
(5) Determine the ideal closeness:
In this way, the CSRCC and three capacities in Hubei province are obtained, respectively. By averaging the scores of each municipality for the same year, the overall resilience of Hubei province from 2011 to 2018 can be obtained.
Regional Disparity Analysis Based on Thiel Coefficient
The Thiel coefficient is mainly used to measure the level of regional disparities, including inter-group disparities and intra-group disparities, and it is able to decompose regional differences to clarify the source situation of the disparities. The calculation formula is as follows:
where T is the Theil coefficient; Tω and Tβ are Theil coefficients of inter-group and intra-group disparities in mountain, hilly, and plain areas, respectively; k is the terrain area (k = 1, 2, 3 indicate mountain, hilly, and plain areas, respectively); yi is the resilience value of the ith municipality; is the average of all the municipality resilience values; yk and express the sum and average of the resilience values of the municipalities in the terrain area; and Tk is the Theil coefficient of the terrain area. The larger the Tvalue, the greater the resilience disparities between the three terrain areas.
Data Source
The study data are the panel data of 16 prefecture-level municipalities of Hubei province (except for Shennongjia) from 2011 to 2018. The data of cropland, irrigated, and road areas (including urban roads and rural roads) are obtained from the Second National Land Resource Investigation of China. The data from land use and land cover change (LUCC) 30 m annual land cover datasets were obtained from Yang and Huang (2021), and then the data were processed using Fragstats 4.2 to obtain the number of patches, patch areas, patch dispersion degrees. We use DEM data from the Shuttle Radar Topography Mission (SRTM) to obtain the area of sloping cropland by ArcGIS. The data of other indexes are derived from the Hubei Rural Statistical Yearbook.
Results
Evaluation of Three Capacities of Cropland System Resilience to Climate Change in Hubei Province
Robustness
From 2011 to 2018, the robustness of the cropland system in Hubei province has changed from 0.513 to 0.502, showing a fluctuating downward trend. The robustness value of each city mainly fluctuated around 0.5, and the fluctuation range did not exceed 0.2. The maximum robustness was 0.684 in Wuhan in 2011, which is much larger than that of other cities. The main reason is that the proportion of sloping cropland in Wuhan was extremely low, only 0.007% of the cropland area, and the comprehensive scores of other indicators were high. The robustness of most municipal scales declined in 2013 and returned to their original level in 2014, with Ezhou and Jingzhou having the largest fluctuations, and Ezhou reaching the lowest value of 0.392. The robustness of municipal scales, such as Wuhan, has undergone similar changes in the following year. Municipal scales, such as Ezhou, Jingzhou, and Shiyan, increased their robustness in 2016 and returned to their original levels in 2017. Wuhan is still producing similar changes a year later. Figures 4A–C reflect the spatial distribution of cropland system robustness in Hubei province in 2011, 2015, and 2018. It can be found that high robustness is mainly located in central and southern Hubei province, such as Wuhan, while northern Hubei province has low robustness, such as Xiangyang. By observing the spatial distribution of the cropland system robustness change in Hubei from 2011 to 2018 (Figure 4D), we found that from 2011 to 2018, the robustness of only five municipal scales decreased slightly, such as Wuhan (−0.185) and Huanggang (−0.042), while most municipalities showed an upward trend and the largest increase is Shiyan (0.243). In general, the robustness of the entire cropland system in Hubei province is not very different, and the inter-annual variation is relatively small.
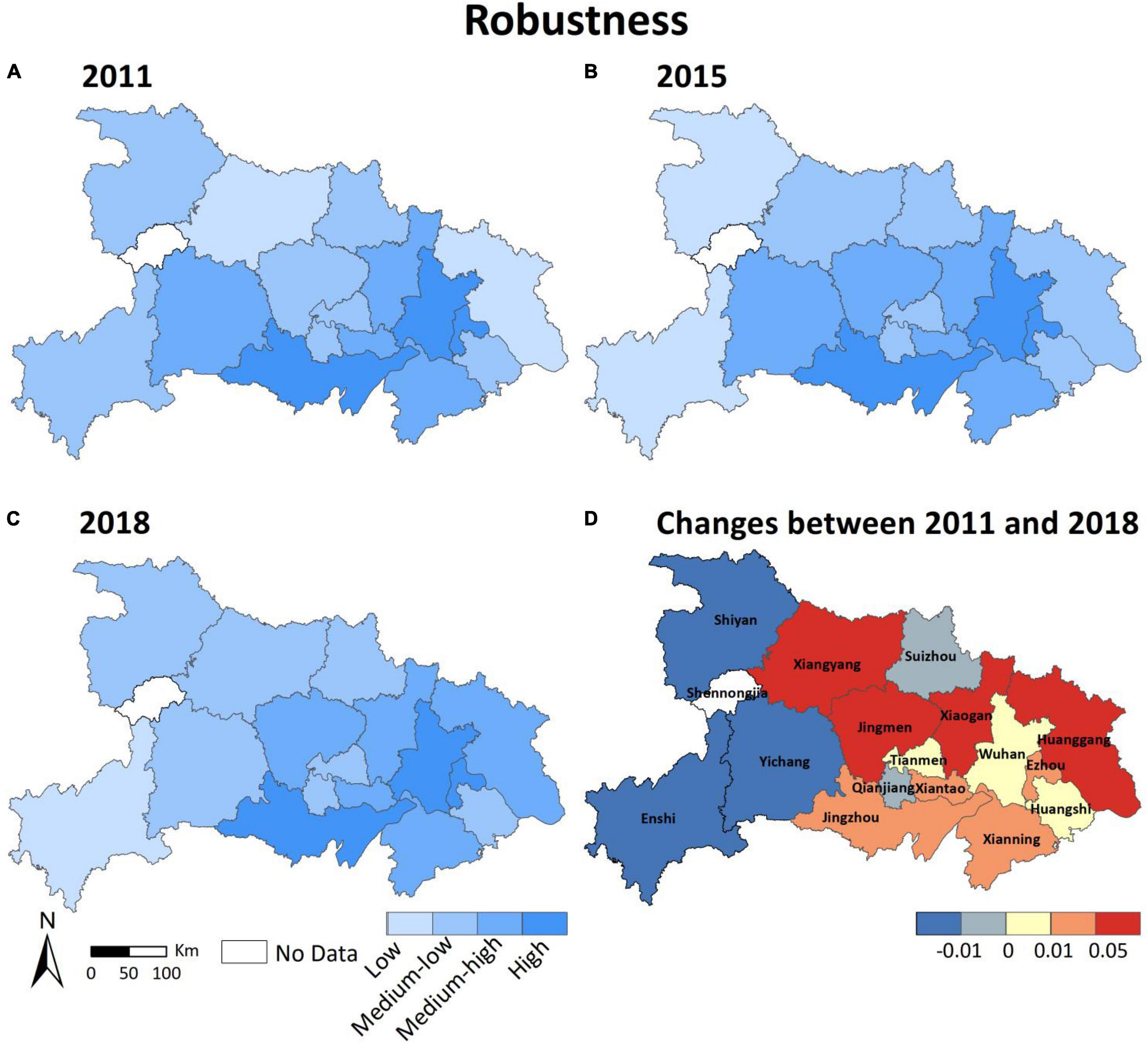
Figure 4. Robustness level of CSRCC in Hubei Province (A) Robustness of CSRCC in 2011, (B) Robustness of CSRCC in 2015, (C) Robustness of CSRCC in 2018, (D) Changes of Robustness between 2011 and 2018.
Adaptability
From 2011 to 2018, the cropland system adaptability of Hubei province has changed from 0.241 to 0.173, showing a downward trend of fluctuation. During the study period, the regional disparities of the adaptability level of Hubei province were between 0.1 and 0.5. The cropland system adaptability of most municipal scales increased in 2011–2013 and then declined. Before 2014, the value of each municipal scale fluctuated considerably, and the change in adaptability was relatively stable from 2014 to 2018. Figures 5A–C show the spatial distribution of the cropland system adaptability of Hubei province in 2011, 2015, and 2018. It shows that high adaptability is mainly found in southern and eastern Hubei, such as Jingzhou, Wuhan, Ezhou, and Tianmen, and western and northern Hubei showed generally low adaptability, such as Enshi, Shiyan, and Suizhou. Moreover, most municipalities in Hubei province showed a downward trend (Figure 5D), among which Xiaogan, Yichang, Xiantao, and Huanggang decreased by 0.232, 0.157, 0.133, and 0.117, respectively. Only Huangshi in the southeast of Hubei province showed a fluctuating upward trend, whose adaptability improved by 0.003.
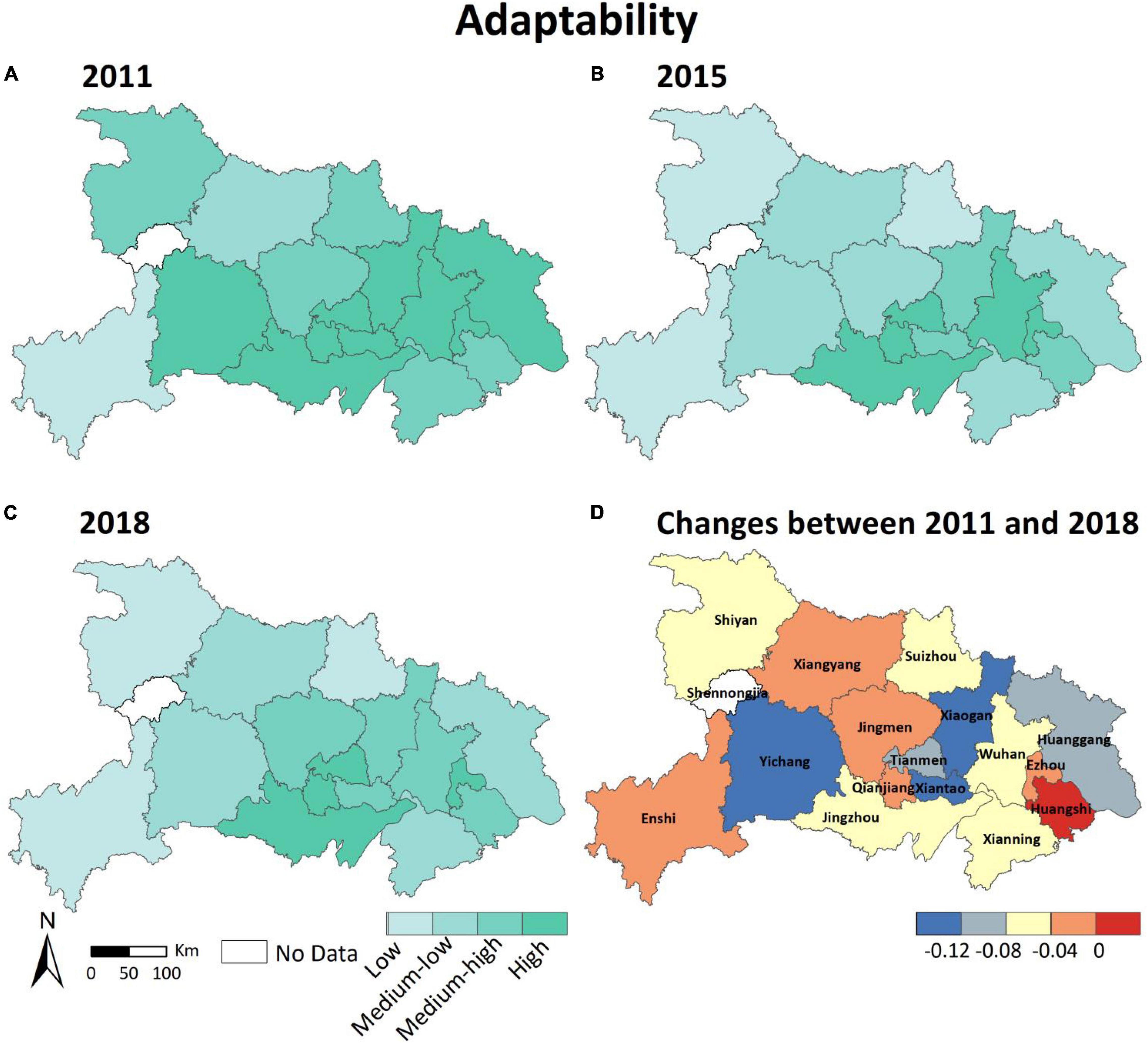
Figure 5. Adaptability level of CSRCC in Hubei Province (A) Adaptability of CSRCC in 2011, (B) Adaptability of CSRCC in 2015, (C) Adaptability of CSRCC in 2018, (D) Changes of Adaptability between 2011 and 2018.
Transformability
From 2011 to 2018, the cropland system transformability in Hubei province fluctuated between 0.494 and 0.506 but remained at 0.500 in 2011 and 2018. The values of all municipal scales were also around 0.500, and the fluctuation range did not exceed 0.095. The maximum value was 0.542 in Wuhan in 2017, and the minimum value was 0.448 in Huanggang in 2016. Figures 6A–C shows the spatial distribution of the cropland system transformability of Hubei province in 2011, 2015, and 2018, and it can be found that high transformability is mainly found in the southern and eastern Hubei province, such as Wuhan and Jingzhou, while Xiaogan in central Hubei, Xiangyang in northern Hubei, and Huanggang in eastern Hubei have generally low cropland system transformability. In addition, most municipalities in Hubei province showed a downward trend (Figure 6D), which mainly concentrated in the southeast, north, and southwest Hubei province. The value of Xiaogan has been reduced the most (−0.023). On the contrary, other municipalities showed an increase, but not by much. Except for Jingzhou, which increased by 0.37, all other municipalities showed an increase by no more than 0.001.
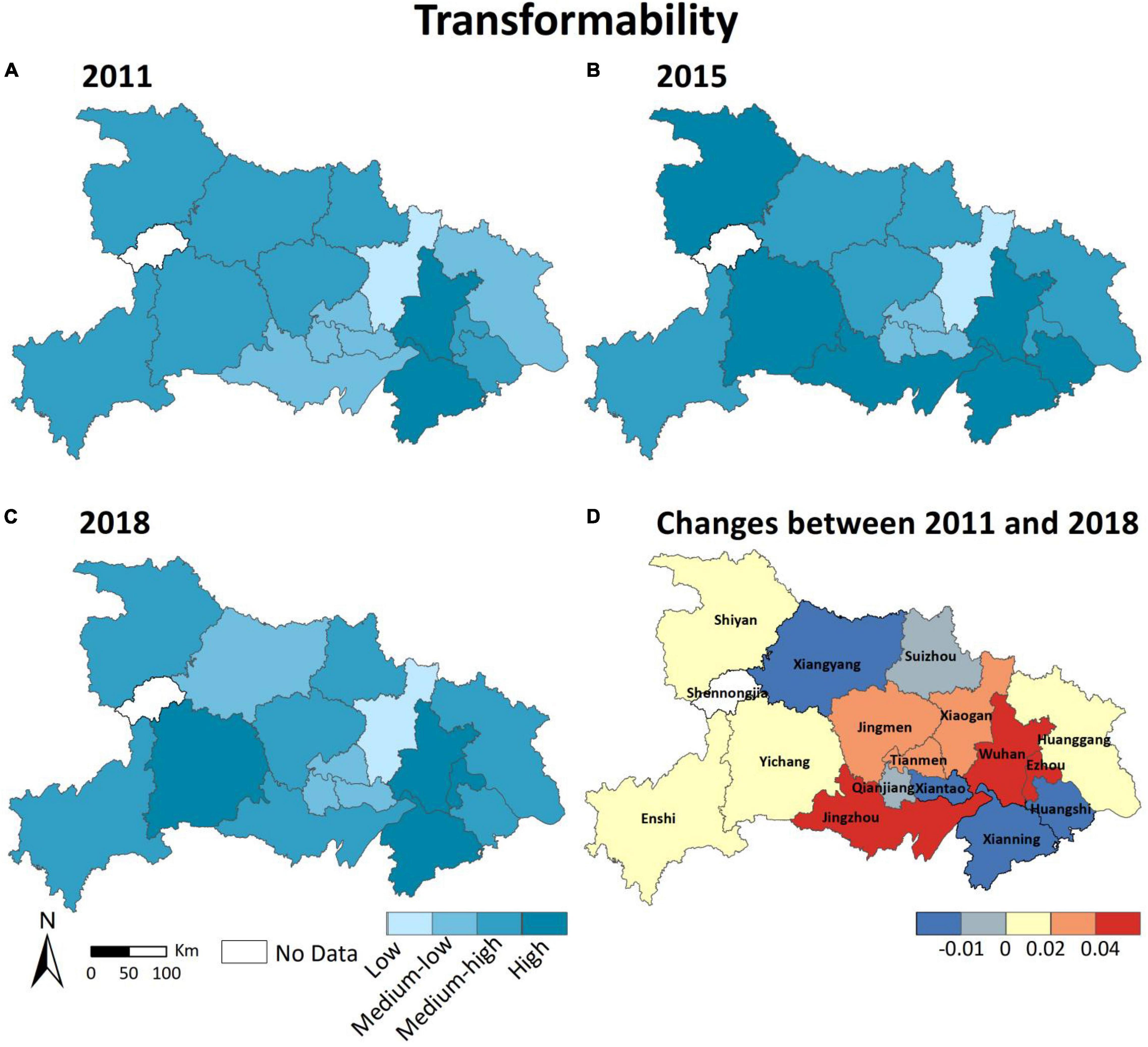
Figure 6. Transformability level of CSRCC in Hubei Province (A) Transformability of CSRCC in 2011, (B) Transformability of CSRCC in 2015, (C) Transformability of CSRCC in 2018, (D) Changes of Transformability between 2011 and 2018.
Overall Evaluation of Cropland System Resilience to Climate Change in Hubei Province
The calculation results of the cropland system resilience in Hubei province from 2011 to 2018 are shown in Table 2. From 2011 to 2018, the cropland system resilience level in Hubei province generally showed a fluctuating downward trend, decreasing from 0.340 to 0.320. During the study period, the cropland system resilience levels in most municipalities of Hubei province were concentrated at 0.2–0.4. The maximum value was 0.552 in Wuhan in 2013, and the minimum value was 0.226 in Jingmen in 2016. The fluctuations in each municipality from 2011 to 2018 were also small, not exceeding 0.057, and the inter-annual variation did not exceed 0.178. We obtained the spatial distribution of the cropland system resilience level of Hubei province for 2011, 2015, and 2018 (Figures 7A–C). Among them, the high resilience level was mainly concentrated in central and southern Hubei province, such as Wuhan, Jingzhou, and Ezhou. The low resilience level was mainly concentrated in the northwest Hubei province, such as Enshi and Shiyan. Moreover, during the study period, the cropland system resilience in Jingmen, Wuhan, and Jingzhou showed an upward trend, which increased by 0.006, 0.005, and 0.003, respectively, while other municipalities all showed a downward trend (Figure 7D). The performance of cropland system resilience in Xiantao was similar to its cropland system robustness, which indicate Xiantao’s cropland system robustness has a greater impact on its cropland system resilience.
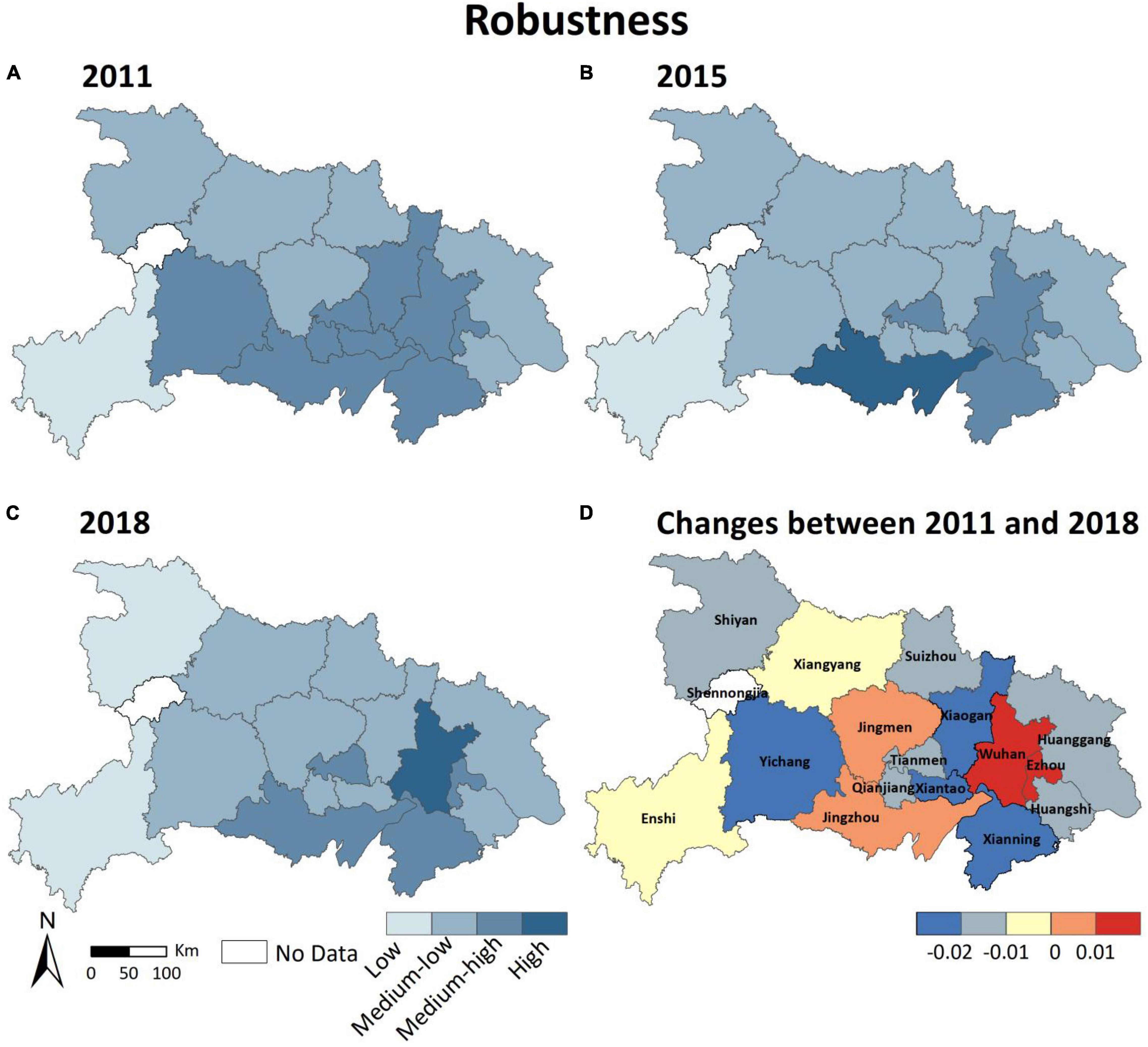
Figure 7. Resilience level of CSRCC in Hubei Province (A) Resilience of CSRCC in 2011, (B) Resilience of CSRCC in 2015, (C) Resilience of CSRCC in 2018, (D) Changes of Resilience between 2011 and 2018.
Regional Disparities of Cropland System Resilience to Climate Change
We measured the regional disparities and influence factors of the cropland system resilience in Hubei province. Figure 8 shows the changes of resilience levels in different terrain areas in Hubei province during the study period. It can be found that from 2011 to 2018, the resilience values were highest in the plain area and lowest in the mountain area. The resilience change trends of the cropland system in three terrain areas were similar. The regional disparities increased first, then decreased to a minimum in 2017, eventually returned to the original gap level.
Table 3 indicates the Thiel coefficients and their decomposition of the resilience disparities in three terrain areas of Hubei province. Judging from the changing trend of the Theil coefficients in Hubei province, there were regional differences in the cropland system resilience in Hubei province during the study period, and the differences increased in an unstable manner. The Thiel coefficients of inter-group differences increased from 2011 to 2013 and reached a maximum of 11.418 in 2013, after which the volatility decreased. Coefficients of the intra-group difference fluctuated to a greater extent, but they returned to the original level in 2018. The Theil coefficients of the inter-group differences in Hubei province were much larger than those of the intra-group differences, indicating that the elastic differences within the three topographic regions were smaller than the elastic differences among the three topographic regions. The differences in the cropland system resilience in Hubei province were mainly due to inter-group differences, indicating that the differences in the resilience of cropland systems in Hubei province were mainly due to topographic differences.
From 2011 to 2018, the cropland system resilience in three areas generally decreased slightly. The Thiel coefficients in plain areas were relatively high, especially in 2015 (0.036), and showed an upward trend. The Thiel coefficients fluctuated considerably in the mountain area but returned to the original level. The Theil coefficients in the hilly regions decreased slightly, indicating that the regional disparities of cropland system resilience in hilly areas increased and decreased in plain areas. In addition, except for 2017, the Thiel coefficients in plain areas were always greater than those of hilly and mountain areas during the study period, indicating that the regional disparities of cropland system resilience in plain areas were more significant than those in hilly and mountain areas.
Discussion
Index System for Evaluating Cropland System Resilience to Climate Change
Since the disturbances and shocks are from various aspects of social and environmental change, an undirected evaluation index system might have limited references for agricultural practice. A single indicator, such as grain output (Di Falco and Chavas, 2008; Birthal et al., 2015) or agricultural GDP (Hsiang and Jina, 2014), has been applied to indirectly reflect the resilience change. In addition, multi-dimension index systems are also constructed by previous studies (Allison and Hobbs, 2004; Tambo, 2016; Lyu et al., 2021; Nasr et al., 2021). However, few studies have focused on a specific disturbance or shock external to the system, which would be difficult to form a unified evaluation system or acknowledged indicators.
Moreover, the indicator selection may also differ considerably between the research objects. It is difficult to unify the evaluation system for both the household unit and the administrative unit. For example, research on the farmers usually considers the individual or family characteristics, the specific conditions of household cropland, and the intensity of utilization and management of cropland (Nyong et al., 2020; Thorsøe et al., 2020; Dixon et al., 2021), while research on administrative units focuses more on the regional cropland resource endowment and governance level (Meyer, 2020). Therefore, different research objects lead to differences in indicator selections. According to previous studies on the theoretical framework of social-ecological system resilience, we suggest that robustness, adaptability, and transformability could reasonably describe the dynamic characteristics of system resilience changing with time after the disturbance. Therefore, this article proposes a new evaluation index system of CSRCC based on the impacts of climate change on the cropland system, the natural endowments, and utilization characteristics of the cropland system from the three dimensions of system resilience.
Influencing Mechanism of Cropland System Resilience to Climate Change
Since the cropland system is a socio-ecological system, the factors influencing CSRCC can be roughly divided into two categories: natural environmental factors and socio-economic factors. Natural environmental factors include climatic conditions, water resource distribution, topographic and geomorphic characteristics, soil properties, and landscape features. For example, Di Falco and Chavas (2008) argued that greater landscape heterogeneity supports higher species diversity and thus makes the agricultural system more resilient to future climate change. Lyu et al. (2021) believed that nature reserve area is an important factor affecting CSRCC because the establishment of nature reserves would hinder the large-scale sustainable management of cropland. In addition, geomorphic characteristics also deeply impact CSRCC because of weaker robustness to natural hazards and enormous difficulty in implementing agricultural mechanization in mountain or hilly areas (Li et al., 2018). In this study, natural endowment (cropland area loss rate) and topographic (sloping cropland proportion) and landscape (average cropland patch size, dispersion of cropland patch) features are considered to have important influences on CSRCC.
From the perspective of socio-economic influences, farming profitability, labor force characteristics, regional economic development levels, agricultural market variation, cropland infrastructure, etc. could impact CSRCC distinctly. Holling (2001) noted that profitability simultaneously increases the resilience potential of the cropland system. Some argued that global market trade tends to decrease, rather than enhance, the adaptability of the cropland system (Milestad and Darnhofer, 2003; Cabell and Oelofse, 2012). In addition, regional economic development has weak adaptability of cropland (Yang et al., 2021). This study also suggests that agricultural technology and innovation could also be favorable to the improvement of CSRCC.
Implications for Chinese Cropland Protection Policies
Improving CSRCC is critical to averting large-scale future shortages and to ensuring food security, as well as good nutrition. China is a developing country with a large population but less available land. To ensure food security, the central government has proposed strict cropland protection policies to realize the “trinity” protection of quantity, quality, and ecology of cropland (Liang et al., 2015; Liu et al., 2017; Wu et al., 2017). In addition, China has also implemented “high-standard farmland construction” to improve cropland quality, agricultural infrastructure, and ecological maintenance, thus ensuring stable crop yields, despite various uncertain external disturbances. The implementation of the policies has objectively improved CSRCC but has not directly incorporated the resilience into the institutional system. Moreover, the weak agricultural infrastructure and risk resistance of the cropland system have not been fundamentally changed in China (Qin and Wu, 2021; Yu et al., 2022). This study suggests that the resilience-oriented policies should be proposed to cope with a range of future disturbances and shocks from environment and socio-economic changes. (1) Giving full play to the production advantages of different regions to optimize configuration of the cropland system; (2) effectively coordinating the resources of land, water, labor force, finance, etc., to improve the agricultural infrastructure to climate change, for increasing the robustness of the cropland system; (3) promoting agricultural diversity to improve the adaptability of the cropland system to climate change; and (4) increasing investment in agricultural technology innovation and cultivating agricultural talents to continuously strengthen the transformability of the cropland system are required.
Limitations and Prospect
This study constructed an evaluation index system of CSRCC at the municipal scale on the basis of the connotations of socio-ecological system resilience and used both of social statistic data and spatial data to explore the spatio-temporal characteristics of CSRCC. The research results could provide a reference for comprehensively regulating the CSRCC and improving regional sustainable agricultural development. However, there are still some limitations: the robustness, adaptability, and transformability of CSRCC constitute a continuous process, and the order in which they occur is fixed. The interactions and conceptual boundaries are still blurry, which makes indicator selection confusing. Some important indicators have two or three attributes of the three capacities of CSRCC at the same time (Manevska-Tasevska et al., 2021; Nicholas-Davies et al., 2021). In our evaluation index system, for example, the cropland area loss rate is negative to the robustness and adaptability. The loss of rural labor force not only is not conducive to the transformation of the cropland system but also has a negative impact on the recovery of the cropland system after the disasters. The indicator selection is not specific enough and needs to be verified and continuously explored with mechanism analysis. Meanwhile, the accessibility and accuracy of data also limited the indicator selection. Specifically, cropland biodiversity, soil property, cropland infrastructure, etc. are the potential indicators of CSRCC, which are inaccessible.
On this basis, this study puts forward the following questions worthy of further research: (1) How to construct a systematic and integrated evaluation system for multi-scale CSRCC? (2) How to choose evaluation indicators by coupling the macro-level of socio-economic factors and the micro-level of natural environmental factors? (3) What are the important influence factors driving the regional disparity of CSRCC? (4) How do existing polices affect CSRCC, and how can specific policies be developed to improve CSRCC?
Conclusion
Based on the connotations of socio-ecological system resilience, we propose a new evaluation index system from robustness, adaptability, and transformability. Delphi, AHP, and TOPSIS methods are used to measure the CSRCC from 2011 to 2018 at the municipal scale in Hubei province, China. The regional disparities of CSRCC are analyzed by using the Theil coefficient. The results show that the CSRCC in Hubei province is generally low. There are a few municipalities whose CSRCC has been improved, while most have decreased. The cropland system resilience in Hubei province shows an increasing trend in the central south and a decreasing trend in the west. Specifically, CSRCC in the central and southern Hubei plains is high, such as Wuhan, Jingzhou, and Ezhou, while that in the western and northern Hubei mountain regions is low, such as Enshi and Shiyan. The construction of an evaluation index system at multi-scale, the influence mechanism of CSRCC change, and the policy implications are discussed. As a prospect, we aim to improve the evaluation system on the basis of the verification of the interactions between the three capacities of cropland system resilience, clarify the macro- and micro-mechanisms which affect CSRCC, and propose more practical policies in further studies.
Data Availability Statement
Publicly available datasets were analyzed in this study. This data can be found here: https://zenodo.org/record/4417810#.YnksmfjP2Un.
Author Contributions
MY collected the data, analyzed the data, wrote, and revised manuscripts. SS analyzed the data, wrote, and revised the manuscripts. XF provided data processing methods. XYL and XHL provided suggestions on research design and the manuscript. BY designed the study and revised the manuscript. All authors agreed to be accountable for the content of the work.
Funding
This work was funded by the National Natural Science Foundation of China (42101280), the Humanities and Social Science Fund of the Ministry of Education (20YJC630182, 19YJC790054), and the Key Project of Philosophy and Social Sciences Research, Ministry of Education (20JZD015).
Conflict of Interest
The authors declare that the research was conducted in the absence of any commercial or financial relationships that could be construed as a potential conflict of interest.
Publisher’s Note
All claims expressed in this article are solely those of the authors and do not necessarily represent those of their affiliated organizations, or those of the publisher, the editors and the reviewers. Any product that may be evaluated in this article, or claim that may be made by its manufacturer, is not guaranteed or endorsed by the publisher.
Acknowledgments
We acknowledge the contributions of specific colleagues, institutions, or agencies that aided the efforts of this research.
References
Allison, H. E., and Hobbs, R. J. (2004). Resilience, adaptive capacity, and the “lock-in trap” of the Western Australian agricultural region. Ecol. Soc. 9:3. doi: 10.5751/ES-00641-090103
Altieri, M. A., Nicholls, C. I., Henao, A., and Lana, M. A. (2015). Agroecology and the design of climate change-resilient farming systems. Agron. Sustain. Dev. 35, 869–890. doi: 10.1007/s13593-015-0285-2
Amaranto, A., Munoz-Arriola, F., Corzo, G., Solomatine, D. P., and Meyer, G. (2018). Semi-seasonal groundwater forecast using multiple data-driven models in an irrigated cropland. J. Hydroinf. 20, 1227–1246. doi: 10.2166/hydro.2018.002
Badillo-Márquez, A. E., Aguilar-Lasserre, A. A., Miranda-Ackerman, M. A., Sandoval-González, O. O., Villanueva-Vásquez, D., and Posada-Gómez, R. (2021). An agent-based model-driven decision support system for assessment of agricultural vulnerability of sugarcane facing climatic change. Mathematics 9:3061. doi: 10.3390/math9233061
Batung, E. S., Mohammed, K., Kansanga, M. M., Nyantakyi-Frimpong, H., and Luginaah, I. (2022). Credit access and perceived climate change resilience of smallholder farmers in semi-arid northern Ghana. Environ. Dev. Sustain. 1–30. doi: 10.1007/s10668-021-02056-x
Birthal, P. S., Negi, D. S., Khan, M. T., and Agarwal, S. (2015). Is Indian agriculture becoming resilient to droughts? Evidence from rice production systems. Food Policy 56, 1–12. doi: 10.1016/j.foodpol.2015.07.005
Bombi, P. (2018). Potential impacts of climate change on Welwitschia mirabilis populations in the Namib Desert, southern Africa. J. Arid Land 10, 663–672. doi: 10.1007/s40333-018-0067-1
Borychowski, M., Stȩpień, S., Polcyn, J., Tošović-Stevanović, A., Ćalović, D., Lalić, G., et al. (2020). Socio-economic determinants of small family farms’ resilience in selected central and eastern european countries. Sustainability 12:10362. doi: 10.3390/su122410362
Buitenhuis, Y., Candel, J. J., Termeer, K. J., and Feindt, P. H. (2020). Does the Common Agricultural Policy enhance farming systems’ resilience? Applying the Resilience Assessment Tool (ResAT) to a farming system case study in the Netherlands. J. Rural Stud. 80, 314–327. doi: 10.1016/j.jrurstud.2020.10.004
Bush, J., and Doyon, A. (2019). Building urban resilience with nature-based solutions: How can urban planning contribute? Cities 95:102483. doi: 10.1016/j.cities.2019.102483
Cabell, J. F., and Oelofse, M. (2012). An indicator framework for assessing agroecosystem resilience. Ecol. Soc. 17:18. doi: 10.5751/ES-04666-170118
Cariolet, J. M., Vuillet, M., and Diab, Y. (2019). Mapping urban resilience to disasters–A review. Sustain. Cities Soc. 51:101746. doi: 10.1016/j.scs.2019.101746
Chen, Y., Xie, W., and Xu, X. (2019). Changes of population, built-up land, and cropland exposure to natural hazards in China from 1995 to 2015. Int. J. Disaster Risk Sci. 10, 557–572. doi: 10.1007/s13753-019-00242-0
Datola, G., Bottero, M., De Angelis, E., and Romagnoli, F. (2022). Operationalising resilience: a methodological framework for assessing urban resilience through System Dynamics Model. Ecol. Model. 465:109851. doi: 10.1016/j.ecolmodel.2021.109851
Deng, C., Wang, H., Gong, S., Zhang, J., Yang, B., and Zhao, Z. (2020). Effects of urbanization on food-energy-water systems in mega-urban regions: a case study of the Bohai MUR, China. Environ. Res. Lett. 15:044014. doi: 10.1088/1748-9326/ab6fbb
Di Falco, S., and Chavas, J. P. (2008). Rainfall shocks, resilience, and the effects of crop biodiversity on agroecosystem productivity. Land Econ. 84, 83–96. doi: 10.3368/le.84.1.83
Dixon, J. M., Weerahewa, J., Hellin, J., Rola-Rubzen, M. F., Huang, J., Kumar, S., et al. (2021). Response and resilience of Asian agrifood systems to COVID-19: an assessment across twenty-five countries and four regional farming and food systems. Agric. Syst. 193:103168. doi: 10.1016/j.agsy.2021.103168
Egli, L., Schröter, M., Scherber, C., Tscharntke, T., and Seppelt, R. (2021). Crop diversity effects on temporal agricultural production stability across European regions. Reg. Environ. Change 21: 96. doi: 10.1007/s10113-021-01832-9
Frei, B., Queiroz, C., Chaplin-Kramer, B., Andersson, E., Renard, D., Rhemtulla, J. M., et al. (2020). A brighter future: complementary goals of diversity and multifunctionality to build resilient agricultural landscapes. Glob. Food Secur. 26:100407. doi: 10.1016/j.gfs.2020.100407
Gaur, N., and Mogalapu, S. (2018). “Pests of soybean,” in Pests and their Management, ed. Omkar Singapore: Springer, 137–162. doi: 10.1007/978-981-10-8687-8_6
Gil, J. D., Cohn, A. S., Duncan, J., Newton, P., and Vermeulen, S. (2017). The resilience of integrated agricultural systems to climate change. Wiley Interdiscip. Rev. Clim. Change 8:e461. doi: 10.1002/wcc.461
Gokulnath, B. V., and Devi, G. U. (2021). Identifying and classifying plant disease using resilient LF-CNN. Ecol. Inform. 63:101283. doi: 10.1016/j.ecoinf.2021.101283
Gunderson, L. H., and Holling, C. S. (2002). Panarchy: Understanding Transformations in Human and Natural Systems. Washington, DC: Island Press.
He, R., Zhu, D., Chen, X., Cao, Y., Chen, Y., and Wang, X. (2019). How the trade barrier changes environmental costs of agricultural production: an implication derived from China’s demand for soybean caused by the US-China trade war. J. Clean. Prod. 227, 578–588. doi: 10.1016/j.jclepro.2019.04.192
Heino, M., Puma, M. J., Ward, P. J., Gerten, D., Heck, V., Siebert, S., et al. (2018). Two-thirds of global cropland area impacted by climate oscillations. Nat. Commun. 9:1257. doi: 10.1038/s41467-017-02071-5
Herrera, H., Schütz, L., Paas, W., Reidsma, P., and Kopainsky, B. (2022). Understanding resilience of farming systems: insights from system dynamics modelling for an arable farming system in the Netherlands. Ecol. Model. 464:109848. doi: 10.1016/j.ecolmodel.2021.109848
Holling, C. S. (2001). Understanding the complexity of economic, ecological, and social systems. Ecosystems 4, 390–405. doi: 10.1007/s10021-001-0101-5
Hsiang, S. M., and Jina, A. S. (2014). “The causal effect of environmental catastrophe on long-run economic growth: Evidence from 6,700 cyclones.”NBER Working Papers 20352. Cambridge, MA: National Bureau of Economic Research. doi: 10.3386/w20352
Jacobi, J., Mukhovi, S., Llanque, A., Augstburger, H., Käser, F., Pozo, C., et al. (2018). Operationalizing food system resilience: an indicator-based assessment in agroindustrial, smallholder farming, and agroecological contexts in Bolivia and Kenya. Land Use Policy 79, 433–446. doi: 10.1016/j.landusepol.2018.08.044
Javadinejad, S., Dara, R., and Jafary, F. (2021). Analysis and prioritization the effective factors on increasing farmers resilience under climate change and drought. Agric. Res. 10, 497–513. doi: 10.1007/s40003-020-00516-w
Kasmalkar, I. G., Serafin, K. A., Miao, Y., Bick, I. A., Ortolano, L., Ouyang, D., et al. (2020). When floods hit the road: resilience to flood-related traffic disruption in the San Francisco Bay Area and beyond. Sci. Adv. 6:eaba2423. doi: 10.1126/sciadv.aba2423
Kotzee, I., and Reyers, B. (2016). Piloting a social-ecological index for measuring flood resilience: a composite index approach. Ecol. Indic. 60, 45–53. doi: 10.1016/j.ecolind.2015.06.018
Li, W., Wei, X., Zhu, R., and Guo, K. (2018). Study on factors affecting the agricultural mechanization level in China based on structural equation modeling. Sustainability 11:51. doi: 10.3390/su11010051
Liang, C., Penghui, J., Manchun, L., Liyan, W., Yuan, G., Yuzhe, P., et al. (2015). Farmland protection policies and rapid urbanization in China: a case study for Changzhou City. Land Use Policy 48, 552–566. doi: 10.1016/j.landusepol.2015.06.014
Liu, D., Li, M., Ji, Y., Fu, Q., Li, M., Faiz, M. A., et al. (2021). Spatial-temporal characteristics analysis of water resource system resilience in irrigation areas based on a support vector machine model optimized by the modified gray wolf algorithm. J. Hydrol. 597:125758. doi: 10.1016/j.jhydrol.2020.125758
Liu, X., Zhao, C., and Song, W. (2017). Review of the evolution of cultivated land protection policies in the period following China’s reform and liberalization. Land Use Policy 67, 660–669. doi: 10.1016/j.landusepol.2017.07.012
Liu, Y., Zou, L., and Wang, Y. (2020). Spatial-temporal characteristics and influencing factors of agricultural eco-efficiency in China in recent 40 years. Land Use Policy 97:104794. doi: 10.1016/j.landusepol.2020.104794
Lyu, X., Wang, Y., Niu, S., and Peng, W. (2021). Spatio-temporal pattern and influence mechanism of cultivated land system resilience: case from China. Land 11:11. doi: 10.3390/land11010011
Manevska-Tasevska, G., Petitt, A., Larsson, S., Bimbilovski, I., Meuwissen, M. P., Feindt, P. H., et al. (2021). Adaptive governance and resilience capacity of farms: the fit between farmers’ decisions and agricultural policies. Front. Environ. Sci. 9:214. doi: 10.3389/fenvs.2021.668836
Meuwissen, M. P., Feindt, P. H., Spiegel, A., Termeer, C. J., Mathijs, E., de Mey, Y., et al. (2019). A framework to assess the resilience of farming systems. Agric. Syst. 176:102656. doi: 10.1016/j.agsy.2019.102656
Meyer, M. A. (2020). The role of resilience in food system studies in low-and middle-income countries. Glob. Food Secur. 24:100356. doi: 10.1016/j.gfs.2020.100356
Meza, I., Siebert, S., Döll, P., Kusche, J., Herbert, C., Eyshi Rezaei, E., et al. (2020). Global-scale drought risk assessment for agricultural systems. Nat. Hazards Earth Syst. Sci. 20, 695–712. doi: 10.5194/nhess-20-695-2020
Milestad, R., and Darnhofer, I. (2003). Building farm resilience: the prospects and challenges of organic farming. J. Sustain. Agric. 22, 81–97. doi: 10.1300/J064v22n03_09
Mittenzwei, K., and Øygarden, L. (2019). The Increasing Impact of Environmental Policies on Agriculture: Perspectives from Norway 172nd EAAE Seminar. Brussels: European Association of Agricultural Economists.
Mutabazi, K. D., Amjath-Babu, T. S., and Sieber, S. (2015). Influence of livelihood resources on adaptive strategies to enhance climatic resilience of farm households in Morogoro, Tanzania: an indicator-based analysis. Reg. Environ. Change 15, 1259–1268. doi: 10.1007/s10113-015-0800-7
Nasr, B. J., Chaar, H., Bouchiba, F., and Zaibet, L. (2021). Assessing and building climate change resilience of farming systems in Tunisian semi-arid areas. Environ. Sci. Pollut. Res. 28, 46797–46808. doi: 10.1007/s11356-021-13089-0
Nicholas-Davies, P., Fowler, S., Midmore, P., Coopmans, I., Draganova, M., Petitt, A., et al. (2021). Evidence of resilience capacity in farmers’ narratives: accounts of robustness, adaptability and transformability across five different European farming systems. J. Rural Stud. 88, 388–399. doi: 10.1016/j.jrurstud.2021.07.027
Niedertscheider, M., Kuemmerle, T., Müller, D., and Erb, K. H. (2014). Exploring the effects of drastic institutional and socio-economic changes on land system dynamics in Germany between 1883 and 2007. Glob. Environ. Change 28, 98–108. doi: 10.1016/j.gloenvcha.2014.06.006
Nyong, A. P., Ngankam, T. M., and Felicite, T. L. (2020). Enhancement of resilience to climate variability and change through agroforestry practices in smallholder farming systems in Cameroon. Agrofor. Syst. 94, 687–705. doi: 10.1007/s10457-019-00435-y
Peng, X., Tong, X., Hao, L., and Wu, F. (2019). Applicability of biochar for limiting interrill erosion and organic carbon export of sloping cropland in a semi-arid area of China. Agric. Ecosyst. Environ. 280, 68–76. doi: 10.1016/j.agee.2019.04.021
Potapova, E., Kruchina, E., Barkhatova, O., and Makarov, A. (2022). “Disasters and Resilient City,” in Proceedings of the IOP Conference Series: Earth and Environmental Science, Bristol. doi: 10.1088/1755-1315/988/3/032007
Qin, X., and Wu, H. (2021). Rural Infrastructure and Poverty in China. Paper Presented at the 31st International Conference of Agricultural Economists (ICAE), Noida.
Ray, R. L., Fares, A., and Risch, E. (2018). Effects of drought on crop production and cropping areas in Texas. Agric. Environ. Lett. 3:170037. doi: 10.2134/ael2017.11.0037
Rey, D., Holman, I. P., and Knox, J. W. (2017). Developing drought resilience in irrigated agriculture in the face of increasing water scarcity. Reg. Environ. Change 17, 1527–1540. doi: 10.1007/s10113-017-1116-6
Tambo, J. A. (2016). Adaptation and resilience to climate change and variability in north-east Ghana. Int. J. Disaster Risk Reduct. 17, 85–94. doi: 10.1016/j.ijdrr.2016.04.005
Tendall, D. M., Joerin, J., Kopainsky, B., Edwards, P., Shreck, A., Le, Q. B., et al. (2015). Food system resilience: defining the concept. Glob. Food Secur. 6, 17–23. doi: 10.1016/j.gfs.2015.08.001
Thorsøe, M., Noe, E., Maye, D., Vigani, M., Kirwan, J., Chiswell, H., et al. (2020). Responding to change: farming system resilience in a liberalized and volatile European dairy market. Land Use Policy 99:105029. doi: 10.1016/j.landusepol.2020.105029
Tuo, D., Xu, M., and Gao, G. (2018). Relative contributions of wind and water erosion to total soil loss and its effect on soil properties in sloping croplands of the Chinese Loess Plateau. Sci. Total Environ. 633, 1032–1040. doi: 10.1016/j.scitotenv.2018.03.237
Ullah, K. M., and Uddin, K. (2021). The relationships between economic growth and cropland changes in Bangladesh: an evidence based on annual land cover data. Environ. Chall. 5:100252. doi: 10.1016/j.envc.2021.100252
Urruty, N., Tailliez-Lefebvre, D., and Huyghe, C. (2016). Stability, robustness, vulnerability and resilience of agricultural systems. A review. Agron. Sustain. Dev. 36:15. doi: 10.1007/s13593-015-0347-5
Vallés-Planells, M., Galiana, F., and Díez Torrijos, I. (2020). Agricultural abandonment and resilience in a Mediterranean periurban traditional agroecosystem: a landscape approach. Ecol. Soc. 25:4845. doi: 10.5751/ES-11346-250105
Van Loon, J., Woltering, L., Krupnik, T. J., Baudron, F., Boa, M., and Govaerts, B. (2020). Scaling agricultural mechanization services in smallholder farming systems: case studies from sub-Saharan Africa. South Asia, and Latin America. Agric. Syst. 180:102792. doi: 10.1016/j.agsy.2020.102792
Vishnu, N., Kameshwar, S., and Padgett, J. E. (2021). Road transportation network hazard sustainability and resilience: correlations and comparisons. Struct. Infrastruct. Eng. 1–21. doi: 10.1080/15732479.2021.1945114
Wang, C., Wang, Z., Gao, Y., and Zhang, X. (2021). Planular-vertical distribution and pollution characteristics of cropland soil Hg and the estimated soil–air exchange fluxes of gaseous Hg over croplands in northern China. Environ. Res. 195:110810. doi: 10.1016/j.envres.2021.110810
Wang, M., Zhang, Y., Bakhshipour, A., Liu, M., Rao, Q., and Liu, Z. (2022). Designing coupled LID–GREI urban drainage systems: resilience assessment and decision-making framework. Sci. Total Environ. 834:155267. doi: 10.1016/j.scitotenv.2022.155267
Wang, T., Li, H., and Huang, Y. (2021). The complex ecological network’s resilience of the Wuhan metropolitan area. Ecol. Indic. 130:108101. doi: 10.1016/j.ecolind.2021.108101
Wa̧tróbski, J., Ba̧czkiewicz, A., Ziemba, E., and Sałabun, W. (2022). Sustainable cities and communities assessment using the DARIA-TOPSIS method. Sustain. Cities Soc. 83:103926. doi: 10.1016/j.scs.2022.103926
Wu, Y., Shan, L., Guo, Z., and Peng, Y. (2017). Cultivated land protection policies in China facing 2030: dynamic balance system versus basic farmland zoning. Habitat Int. 69, 126–138. doi: 10.1016/j.habitatint.2017.09.002
Yang, J., and Huang, X. (2021). The 30 m annual land cover datasets and its dynamics in China from 1990 to 2020. Earth Syst. Sci. Data 13, 3907–3925. doi: 10.5194/essd-13-3907-2021
Yang, Y., Guo, H., Wang, D., Ke, X., Li, S., and Huang, S. (2021). Flood vulnerability and resilience assessment in China based on super-efficiency DEA and SBM-DEA methods. J. Hydrol. 600:126470. doi: 10.1016/j.jhydrol.2021.126470
Yu, J., and Wu, J. (2018). The sustainability of agricultural development in China: the agriculture–environment nexus. Sustainability 10:1776. doi: 10.3390/su10061776
Yu, T., Mahe, L., Li, Y., Wei, X., Deng, X., and Zhang, D. (2022). Benefits of crop rotation on climate resilience and its prospects in China. Agronomy 12:436. doi: 10.3390/agronomy12020436
Zampieri, M., Toreti, A., Ceglar, A., De Palma, P., Chatzopoulos, M., and Michetti, M. (2021). Analysing the resilience of agricultural production systems with ResiPy, the Python production resilience estimation package. SoftwareX 15:100738. doi: 10.1016/j.softx.2021.100738
Zampieri, M., Weissteiner, C. J., Grizzetti, B., Toreti, A., van den Berg, M., and Dentener, F. (2020). Estimating resilience of crop production systems: from theory to practice. Sci. Total Environ. 735:139378. doi: 10.1016/j.scitotenv.2020.139378
Keywords: cropland system, resilience to climate change, regional disparity, spatio-temporal change, food security
Citation: Yan M, Yang B, Sheng S, Fan X, Li X and Lu X (2022) Evaluation of Cropland System Resilience to Climate Change at Municipal Scale Through Robustness, Adaptability, and Transformability: A Case Study of Hubei Province, China. Front. Ecol. Evol. 10:943265. doi: 10.3389/fevo.2022.943265
Received: 13 May 2022; Accepted: 01 June 2022;
Published: 30 June 2022.
Edited by:
Jun Yang, Northeastern University, ChinaReviewed by:
Liye Wang, Wuhan University, ChinaQiushi Zhou, Zhejiang University, China
Andong Guo, Zhejiang University, China
Dongxue Zhao, The University of Queensland, Australia
Chaohui Yin, Henan Agricultural University, China
Copyright © 2022 Yan, Yang, Sheng, Fan, Li and Lu. This is an open-access article distributed under the terms of the Creative Commons Attribution License (CC BY). The use, distribution or reproduction in other forums is permitted, provided the original author(s) and the copyright owner(s) are credited and that the original publication in this journal is cited, in accordance with accepted academic practice. No use, distribution or reproduction is permitted which does not comply with these terms.
*Correspondence: Bohan Yang, bohan.yang@ccnu.edu.cn