- 1Biotechnology Unit, Technological Development Center, Federal University of Pelotas, Pelotas, Rio Grande do Sul, Brazil
- 2Gonçalo Moniz Institute, Oswaldo Cruz Foundation, Ministry of Health, Salvador, Bahia, Brazil
Leptospira spp. are diderm (two membranes) bacteria that infect mammals causing leptospirosis, a public health problem with global implications. Thousands of people die every year due to leptospirosis, especially in developing countries with tropical climates. Prophylaxis is difficult due to multiple factors, including the large number of asymptomatic hosts that transmit the bacteria, poor sanitation, increasing numbers of slum dwellers, and the lack of an effective vaccine. Several leptospiral recombinant antigens were evaluated as a replacement for the inactivated (bacterin) vaccine; however, success has been limited. A prospective vaccine candidate is likely to be a surface-related protein that can stimulate the host immune response to clear leptospires from blood and organs. In this study, a comprehensive bioinformatics approach based on reverse and structural vaccinology was applied toward the discovery of novel leptospiral vaccine candidates. The Leptospira interrogans serovar Copenhageni strain L1-130 genome was mined in silico for the enhanced identification of conserved β-barrel (βb) transmembrane proteins and outer membrane (OM) lipoproteins. Orthologs of the prospective vaccine candidates were screened in the genomes of 20 additional Leptospira spp. Three-dimensional structural models, with a high degree of confidence, were created for each of the surface-exposed proteins. Major histocompatibility complex II (MHC-II) epitopes were identified, and their locations were mapped on the structural models. A total of 18 βb transmembrane proteins and 8 OM lipoproteins were identified. These proteins were conserved among the pathogenic Leptospira spp. and were predicted to have epitopes for several variants of MHC-II receptors. A structural and functional analysis of the sequence of these surface proteins demonstrated that most βb transmembrane proteins seem to be TonB-dependent receptors associated with transportation. Other proteins identified included, e.g., TolC efflux pump proteins, a BamA-like OM component of the βb transmembrane protein assembly machinery, and the LptD-like LPS assembly protein. The structural mapping of the immunodominant epitopes identified the location of conserved, surface-exposed, immunogenic regions for each vaccine candidate. The proteins identified in this study are currently being evaluated for experimental evidence for their involvement in virulence, disease pathogenesis, and physiology, in addition to vaccine development.
Introduction
Leptospirosis is a zoonosis caused by spirochetes belonging to the Leptospira genus. More than 250 antigenically distinct serovars have been described for 15 infectious Leptospira spp. (10 pathogenic and 5 intermediate species) to date (1, 2). Leptospirosis is a neglected tropical disease with an estimated incidence of over one million severe cases in humans, resulting in ~60,000 fatalities (3). Furthermore, the disease has a major impact on the health of agricultural and companion animals, with serious economic consequences (4). Recombinant vaccine development is a major research focus because of the lack of effective control measures. Classical immunization strategies based on whole-cell, inactivated leptospires (bacterins), or cell wall components have been well documented (5). However, while bacterin vaccines are highly efficacious, they cause serious adverse reactions and confer short-term immunity that is restricted to the serovars used in the bacterin preparation (6).
Several research groups have used the classical approach for the identification of protein targets for use in recombinant vaccines with mixed results, reviewed in Ref. (7). The most promising targets to date are the leptospiral immunoglobulin-like (Lig) proteins. We recently showed that a LigB-based subunit vaccine protected hamsters against leptospirosis and induced sterile immunity (8). While these results will need to be confirmed by other research groups, protection conferred by LigA, with reports of up to 100% efficacy in the hamster model, has been consistently reproduced by different groups throughout the world. However, sterile immunity was not evident in LigA-vaccinated survivors (9–11). In addition, ligA is present in only three pathogenic Leptospira spp. (12), further limiting its ability to induce cross-protective immunity. Of note, LipL32, the immunodominant leptospiral lipoprotein, was extensively investigated as a vaccine candidate using different strategies (e.g., subunit, DNA vaccine, BCG, and adenovirus constructs) with inconclusive results; efficacy ranged from 12 to 87% (13–17). Another putative lipoprotein, LemA, was identified using reverse vaccinology (RV) and induced partial protection using a prime-boost strategy (18, 19). Finally, the putative outer membrane protein (OMP) OmpL37, perhaps one of the most promising antigens recently characterized, was not protective against lethal disease in the hamster model (20). The current status of leptospiral vaccine development shows that there is an unmet need for the discovery of new vaccine candidates and that further success will require reevaluation of the Leptospira genome (21).
The RV approach was first applied almost two decades ago and led to the discovery of protective vaccine candidates for several bacterial diseases (22). The best example is the recently licensed vaccine against meningococcal disease caused by Neisseria meningitidis serogroup B; the protein components of the vaccine were discovered by RV (23). However, while there are some examples of its partial application toward target discovery in the field of leptospirosis (18, 19), it has not been successfully implemented (24), reviewed in Ref. (25). RV targets are generally surface-related proteins that are recognized by the host immune system, thereby eliminating the bacteria and preventing disease. Ideally, these targets should play an important role during pathogenesis, increasing vaccine efficacy. In diderm bacteria, such as Leptospira spp. and Gram-negative bacteria, β-barrel transmembrane proteins (βb-OMPs) and some outer membrane (OM) lipid-anchored proteins (lipoproteins) are the only types of proteins that are surface exposed (26, 27). Proteins with a transmembrane α-helix (TMH) structure tend to be localized to the inner membrane and are rarely found in the OM, e.g., the Wza translocon for capsular polysaccharides in Escherichia coli (28). Even though various βb-OMPs and lipoproteins have been annotated in the Leptospira genome, many are still identified as hypothetical proteins. While some βb-OMPs and lipoproteins were characterized by means other than RV, we believe that a large number of these types of prospective vaccine antigens are yet to be discovered.
In addition to RV, recent advances in vaccine research using the structural information of antigens have led to the development of structural vaccinology (SV) (29). Based primarily on protein design for the optimization of antigen structure and consequently enhanced protection, SV is a combination of structural biology, immunology, and bioinformatics (30). Solving protein structures is time consuming, expensive, and sometimes difficult, especially for proteins such as the βb-OMPs (31, 32). Advances in structural bioinformatics has allowed reliable prediction of three-dimensional (3D) structural models of proteins based on the alignment of the query sequence to known-structure templates, which may be identified using sequence similarity searches (homology modeling) or fold recognition (threading) methods (31, 33). In contrast to homology-based methods, protein threading allows the prediction of structural models of proteins with low similarity to known proteins (no orthologs). This is attractive for leptospiral proteins, as there are no solved structures for leptospiral βb-OMPs. In the current study, we report the application of RV and SV toward the discovery of leptospiral vaccine candidates, i.e., βb-OMPs and OM lipoproteins, structural modeling, the in silico identification of major histocompatibility complex (MHC-II)-binding epitopes, and the selection of surface-related immunogenic epitopes.
Results
Identification of βb-OMPs and OM Lipoproteins in the Leptospira interrogans Genome
Reverse vaccinology was employed for the identification of surface-exposed proteins, including βb-OMPs and OM lipoproteins in the genome of L. interrogans serovar Copenhageni strain Fiocruz L1-130 (LIC). The bioinformatics workflow and total numbers of proteins identified by each bioinformatics algorithm (predictor) are shown (Figure 1). The predictors for subcellular localization (Cello, PSORTb, and Gneg-mPLoc) found 523 proteins (by 1 or more predictor) located in the OM of L. interrogans, 24 were identified by all three predictors. A total of 1,196 proteins were predicted to contain a signal peptide (SP) for translocation across the cytoplasmic membrane to, e.g., the OM. Of these, 72 proteins were identified by all three SP predictors (SignalCF, SignalP, and PrediSI). One or more of the transmembrane α-helix (TMH) predictors (Phobius, TMHMM, HHTOP, and MEMSAT) determined that 3,302 proteins did not contain a TMH, this was reduced to 2,929 proteins by all 4 predictors. The transmembrane β-barrel (βb) structure predictors (Bomp, HHomp, TMBETADISC-RBF, and MCMBB) identified 1,085 βb-OMPs, 20 of which were confirmed by all 4 predictors. Finally, 230 proteins were identified as lipoproteins by at least one of the predictors, 108 by both (LipoP and SpLip). A complete list of the proteins identified by each individual predictor is provided (Table S1 in Supplementary Material).
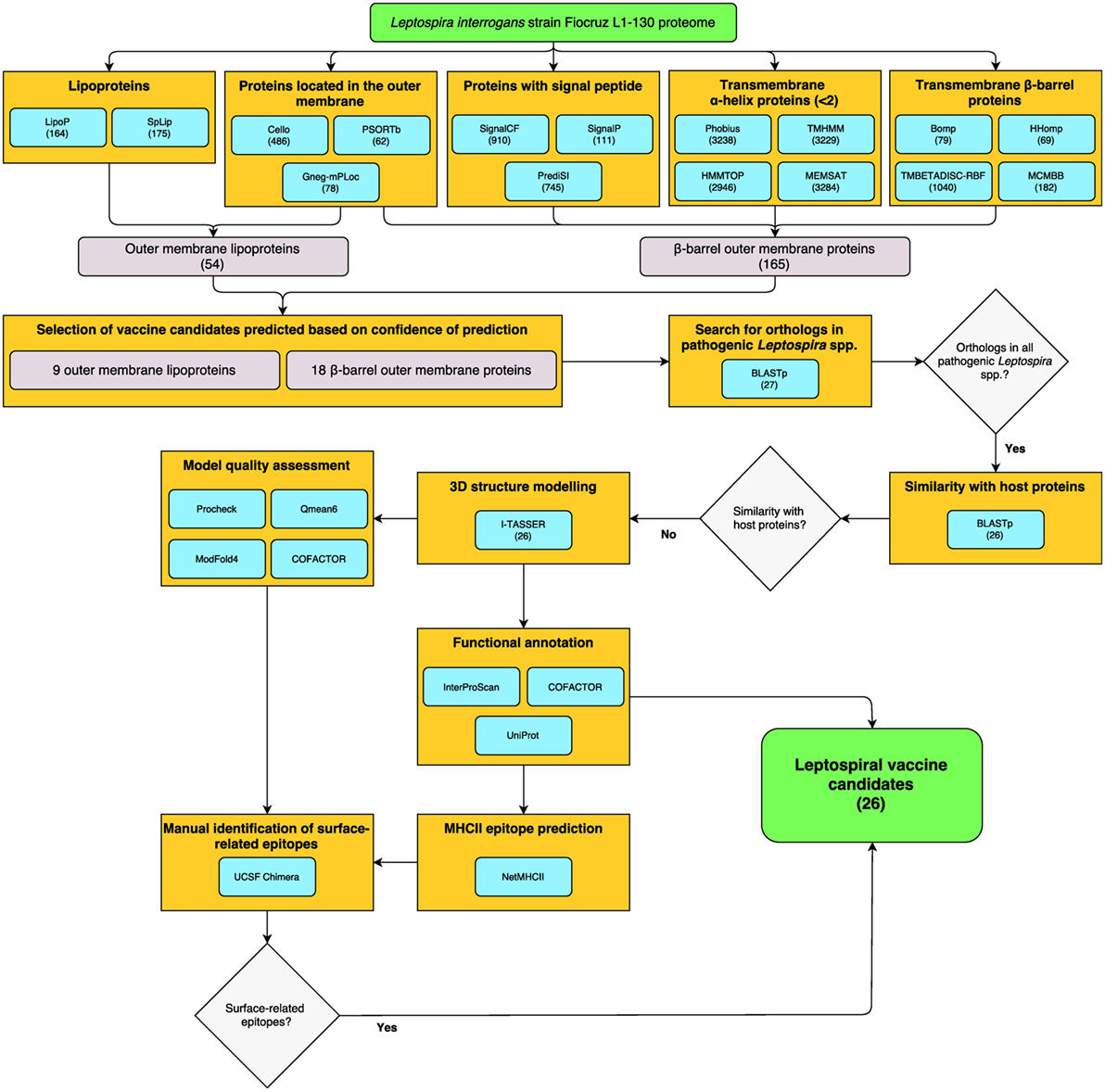
Figure 1. Reverse vaccinology (RV)- and structural vaccinology (SV)-based bioinformatics workflow for the identification of leptospiral vaccine candidates. The complete LIC genome-derived proteome provided the input sequences for 16 bioinformatics programs for the prediction of βb-outer membrane proteins (OMPs) and outer membrane (OM) lipoproteins. The number of proteins selected by each program is shown in parentheses. High confidence predicted proteins were screened using a Python algorithm. Proteins conserved among pathogenic Leptospira spp. and with no similarity to host proteins were selected for the three-dimensional (3D) structure prediction by fold recognition modeling. Functional annotation was based on the primary structure and on the 3D models. Strong binder major histocompatibility complex-II immunogenic epitopes were predicted for the selected proteins and a structural approach was employed to map the surface-related immunogenic regions in the βb-OMPs. The final list of conserved βb-OMPs and OM lipoproteins and the surface-related epitopes are novel prospective leptospiral vaccine candidates.
A total of 165 βb-OMPs were identified by at least 1 predictor, while only 1 protein (LIC10714) was identified by all predictors. In this study, a βb-OMP was defined as a protein predicted to contain a βb structure, an SP, and <2 TMHs. As an SP can be identified as a TMH, a protein containing a single, N-terminal, TMH was classified as a non-TMH protein. An OM lipoprotein was defined as a protein predicted to be located in the OM and that contained a lipobox. A total of 54 OM lipoproteins were identified by at least one predictor of each feature; however, no proteins were identified at the intersect between all the predictors for lipoproteins and cell localization. A list of the 165 βb-OMPs and 54 OM lipoproteins identified, the gene products as annotated in the LIC genome and the result for each predictor is provided (Table S2 in Supplementary Material).
Filtering Predicted Protein Features with Increased Confidence
Due to the particularities of each predictor, low agreement between the different bioinformatics tools was expected when identifying the same feature. To reduce the impact of a prediction when non-weighted (naïve) voting resulted in ambiguities (e.g., two negative and one positive result by three different predictors), we used an iterative weighted voting system. An in-house Python script was written to integrate the results and to identify those proteins with a high level of confidence in the consensus prediction among those selected by at least one predictor. The lists of 165 βb-OMPs and 54 OM lipoproteins were used as the script input and, after thousand iterations, each predictor received a final prediction weight (Table S3 in Supplementary Material). A final voting score >0.5 (0–1 scale) for each feature of interest (e.g., >0.5 for OM localization and >0.5 for lipoprotein prediction) was indicative of a high level of confidence in the prediction, resulting in the selection of 18 βb-OMPs and 9 OM lipoproteins (Table 1).
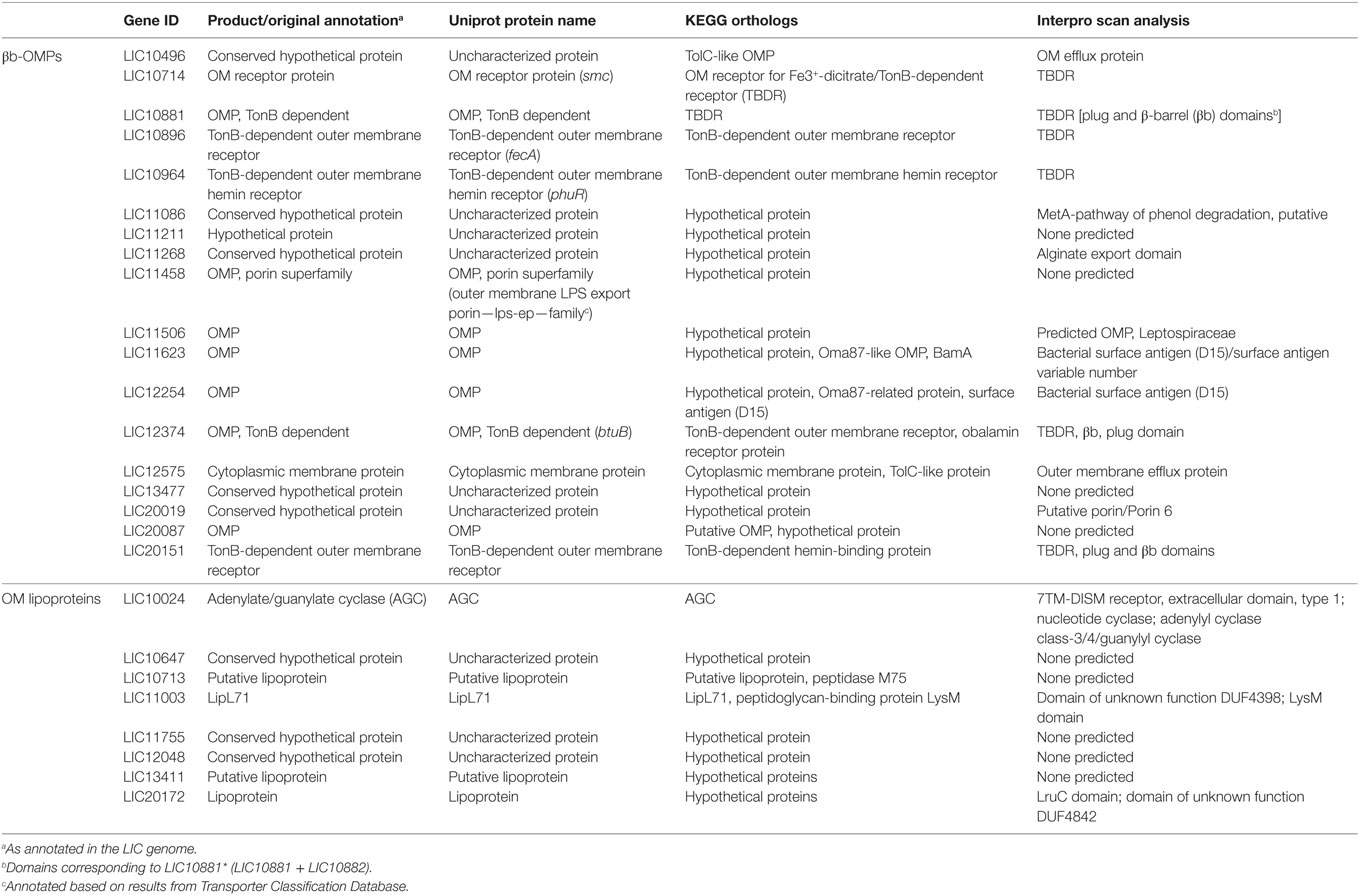
Table 1. Functional annotation of βb-outer membrane proteins (OMPs) and outer membrane (OM) lipoproteins.
Conservation of Surface-Exposed Proteins among Leptospira spp. and Similarity to Mammalian Host Proteins
The genome sequences from 20 additional Leptospira spp. (Table S4 in Supplementary Material) were screened for orthologs of the 18 βb-OMPs and the 9 OM lipoproteins. Interestingly, all the pathogenic Leptospira spp. contained orthologs of the 18 βb-OMPs (Table 2), except for L. kmetyi that did not contain an ortholog of LIC10881. This protein was also absent from the intermediate and saprophytic Leptospira spp. Of the 9 OM lipoproteins, LIC12048 was absent in L. borgpetersenii, and LIC20172 was not found in Leptospira kirschneri, these proteins were, however, retained for further analysis as they were present in the majority of the pathogenic Leptospira spp. The OM lipoprotein LIC12690 was excluded from further analysis as it was only found in L. interrogans, L. kirschneri, and Leptospira noguchii. In addition, the βb-OMPs and OM lipoproteins were screened against selected mammalian proteomes for similarity to any of these potential vaccine candidates. No similarities were found among any of the leptospiral proteins and human, dogs, cattle, pig, horse, or sheep proteins (data not shown).
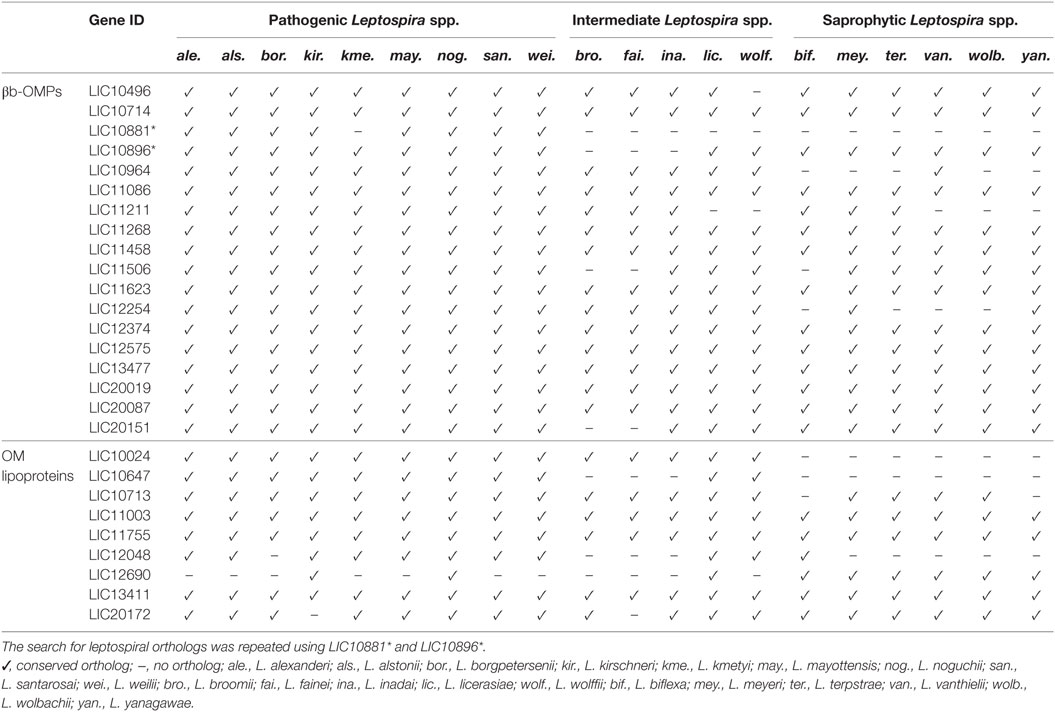
Table 2. Conservation of the 18 βb-outer membrane proteins (OMPs) and 9 outer membrane (OM) lipoproteins in 20 Leptospira spp.
Multiple Sequence Alignments of Leptospira Surface-Exposed Proteins
A multiple sequence alignment was performed with each βb-OMP and OM lipoprotein and their respective orthologs. The alignments suggested that LIC10881 and LIC10964 were truncated sequences compared to their respective orthologs (Data Sheet S1 in Supplementary Material). An analysis of the genome region that contained the LIC10881 coding sequence (CDS) revealed the presence of a possible point mutation in the last nucleotide of LIC10881, that created a stop codon. When the point mutation was altered to a tryptophan codon (TGA → TGG), the LIC10881 and LIC10882 CDS were reassembled as a single CDS, LIC10881*, and the multiple alignment of LIC10881* was no longer truncated (Data Sheet S1 in Supplementary Material). Similarly, we identified a potential frameshift mutation in the LIC10896 CDS that was altered by the insertion of a cytosine at position 2,597. Reassembly of LIC10896 and LIC10895 identified a single CDS, LIC10896*. When the LIC10896* protein sequence was included in the multiple alignment, the sequence was no longer truncated (Data Sheet S1 in Supplementary Material). The modified LIC10881* and LIC10896* proteins were used for further analysis.
Functional Annotation Based on Protein Sequence
Eleven of the 18 βb-OMPs identified in this study were originally annotated as OMPs in the LIC genome, one was annotated as a cytoplasmic membrane protein, and six were annotated as hypothetical proteins (Table 1). Seven of the eight OM lipoproteins were annotated as hypothetical proteins or putative lipoproteins in the original genome annotation. The exception was LIC10024 that was annotated as an adenylate/guanylate cyclase (AGC). The KEGG database was used to identify any corresponding orthologs and their annotations in the genomes of the other pathogenic Leptospira spp. Of note, the genome annotations of LIC10496, LIC11458, LIC11506, and LIC12575 differed substantially to those of their orthologs (Table 1).
A functional annotation was performed using InterProScan for the 18 βb-OMPs and 8 OM lipoproteins (Table 1). InterProScan identified OMP-related domains in 14 of the βb-OMPs. However, it failed to identify functional domains in four of the predicted βb-OMPs (LIC11211, LIC11458, LIC13477, and LIC20087) and five out of eight OM lipoproteins (LIC10647, LIC10713, LIC11755, LIC12048, and LIC13411). Of the remaining OM lipoproteins, two (LIC11003 and LIC20172) contained domains with an unknown function and LIC10024 was confirmed as an AGC protein.
Structural Modeling Improved the Prediction of OMP Function
For each protein sequence, the I-TASSER software predicted five 3D models and each model was ranked in order of quality by a C-score. The top-ranking models for 16 of the βb-OMPs contained a typical transmembrane βb structure (Figure 2). While the LIC11268 model that contained a transmembrane βb structure was ranked third, it was included for further analysis. The structural models of the eight OM lipoproteins are shown (Data Sheet 3 in Supplementary Material). The refined βb-OMP and OM lipoprotein models were analyzed by COFACTOR, a structure-based method for assigning biological function to protein molecules. A COFACTOR output includes the top 10 closest structures in PDB ranked by TM-score, Gene Ontology (GO) terms associated with the protein model, and the root-mean-square deviation of atomic position (RMSD) related to the best templates used for modeling. GO terms, including molecular function, biological process, and cellular location, associated with the protein 3D models were predicted based on the GOs assigned to the template structures and provided a functional insight into the selected leptospiral proteins. When more than one GO term per category was predicted for each protein, some were related to more distant templates (the last of the 10 closest structures), they were collapsed to the closest parent term on the AmiGO2 database and are shown (Figure 3) according to their frequency in the βb-OMPs and OM lipoproteins. The complete list of GO terms predicted for each protein structure is provided (Table S5 in Supplementary Material). As expected, most of the βb-OMP molecular function and biological process GO terms were related to transportation. This was supported by the cell location GO term, most of the βb-OMPs were classified as membrane or OM. The GO terms predicted for the OM lipoproteins were distributed among several different categories. Most of the GO terms for molecular function were for catalytic/binding activity and the predicted biological processes were related to metabolism. The predicted cell location GO terms were diverse (Figure 3; Table S5 in Supplementary Material).
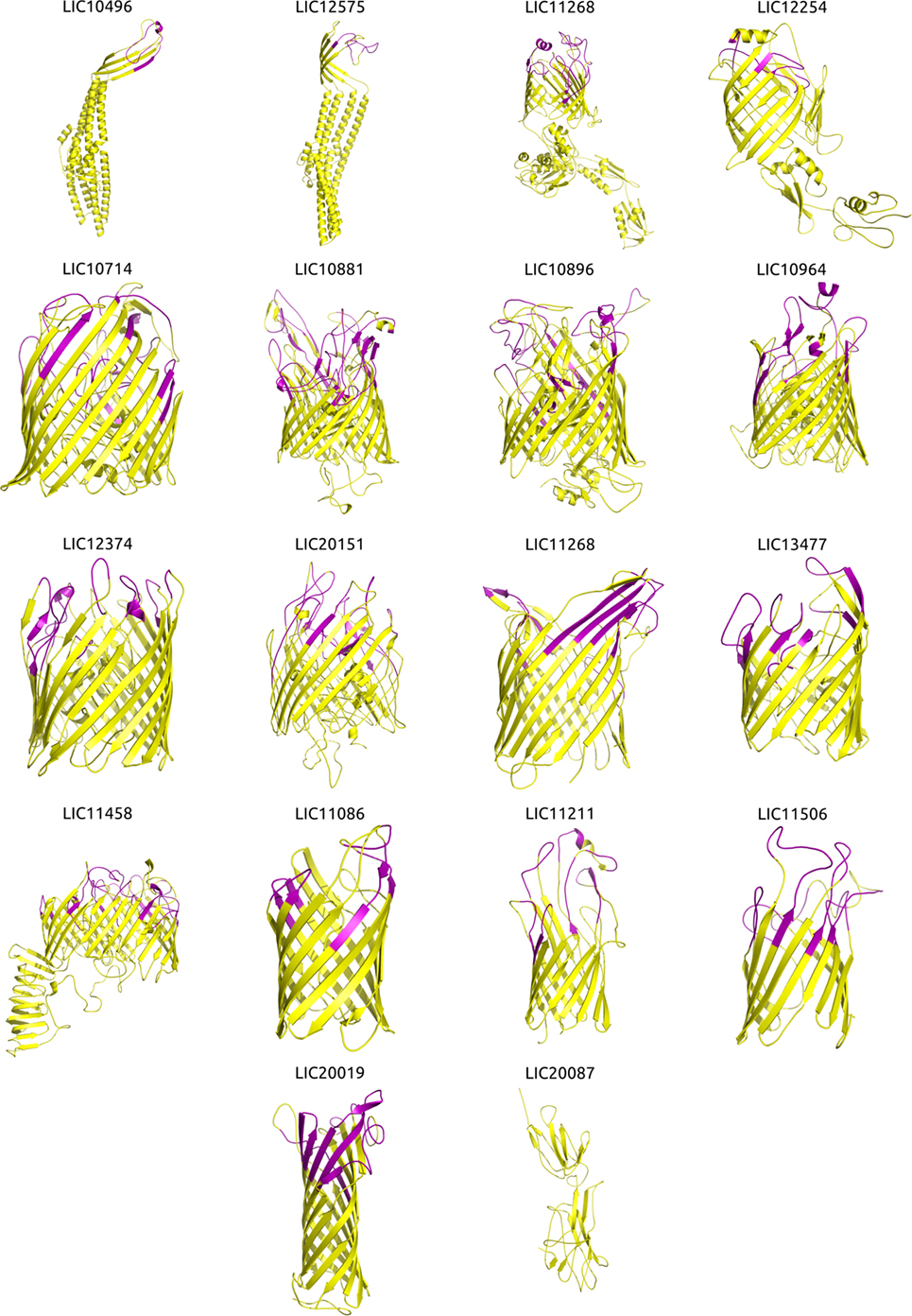
Figure 2. Three-dimensional (3D) structures of the βb-outer membrane proteins (OMPs) and mapping of the immunogenic surface-related epitopes (SRE). Structural modeling was performed using I-TASSER, and the structures were visualized and the images generated using PyMOL. Proteins are orientated as following: the upper portion is surface-exposed on the OM and the lower portion is located in the periplasmic space. The orientation was derived from an interpretation of the orientation and structure of the matching PDB structure. Immunogenic major histocompatibility complex-II epitopes (strong binders) for 14 HLAs were predicted using NetMHCII and mapped onto the βb-OMPs structural models. Immunogenic SRE are indicated in purple in each βb-OMP structure.
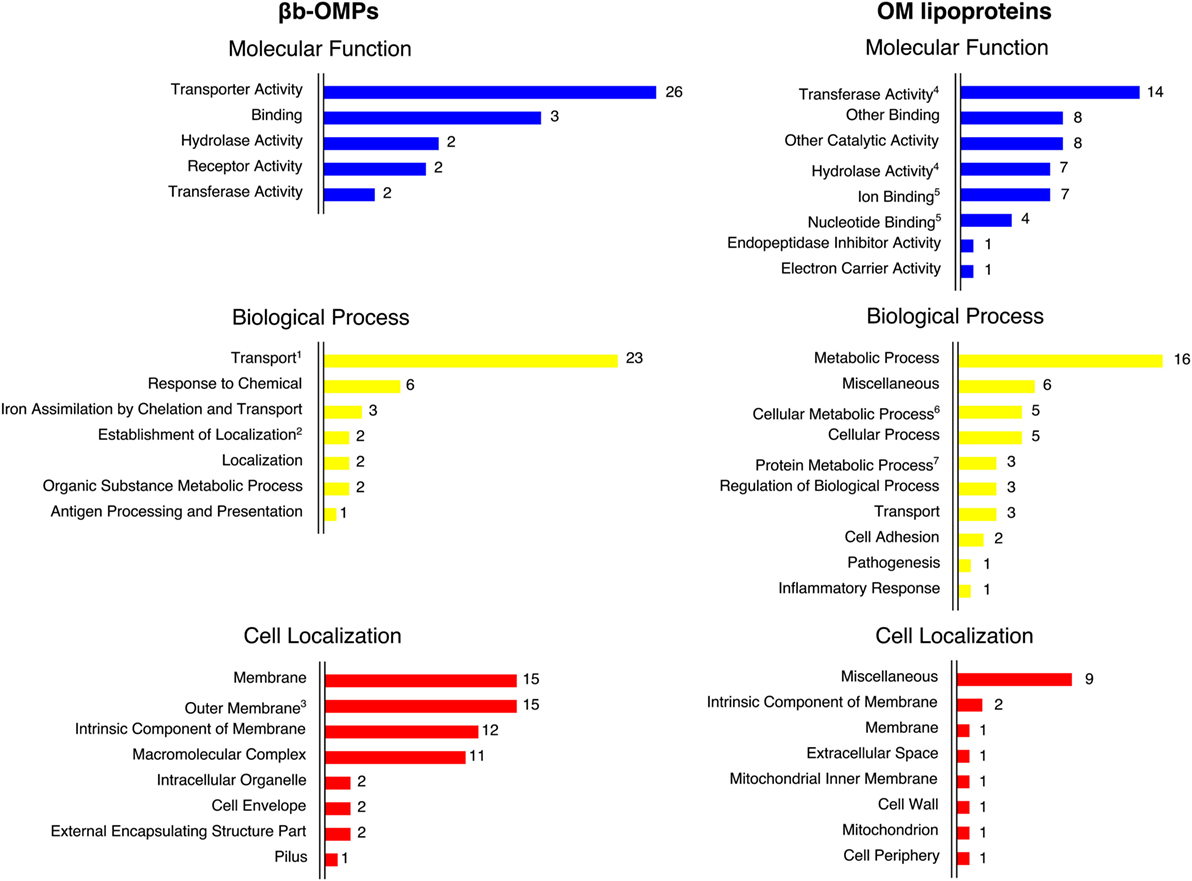
Figure 3. The Gene Ontology (GO) terms predicted by COFACTOR were based on the structural models of the βb-outer membrane proteins (OMPs) and outer membrane (OM) lipoproteins. The GO terms were assigned for three domains: molecular function, biological process, and cell localization. COFACTOR usually predicted more than one term for each GO domain for the target protein structure. The GO terms were collapsed to the closest parent term (based on the AmiGO2 database) to facilitate the overall view of the GO terms. In some cases, related terms were maintained for convenience, as indicated: 1—transport is also a child term of establishment of localization; 2—establishment of localization is also a child term of localization; 3—outer membrane is also a child term of membrane; 4—transferase activity and hydrolase activity are also children terms of catalytic activity; 5—ion binding and nucleotide binding are also children terms of binding; 6—cellular metabolic process is also a child term of metabolic process and cellular process; and 7—protein metabolic process is also a child term of metabolic process.
Quality of Predicted Models
The closest PDB structure (PDB code, protein name, and organism of origin) for the predicted structural models and an estimation of the quality are shown (Table 3). The quality of each predicted model was evaluated using Procheck, QMEAN6, and ModFOLD4. Procheck was used to evaluate the stereochemical quality of each protein structure and the proportion of disallowed residues in the Ramachandran plot ranged from 0.7% for LIC10496 to 11.6% for LIC20151. When assessed by QMEAN and ModFOLD, most of the βb-OMPs models had good quality values for the overall structure.
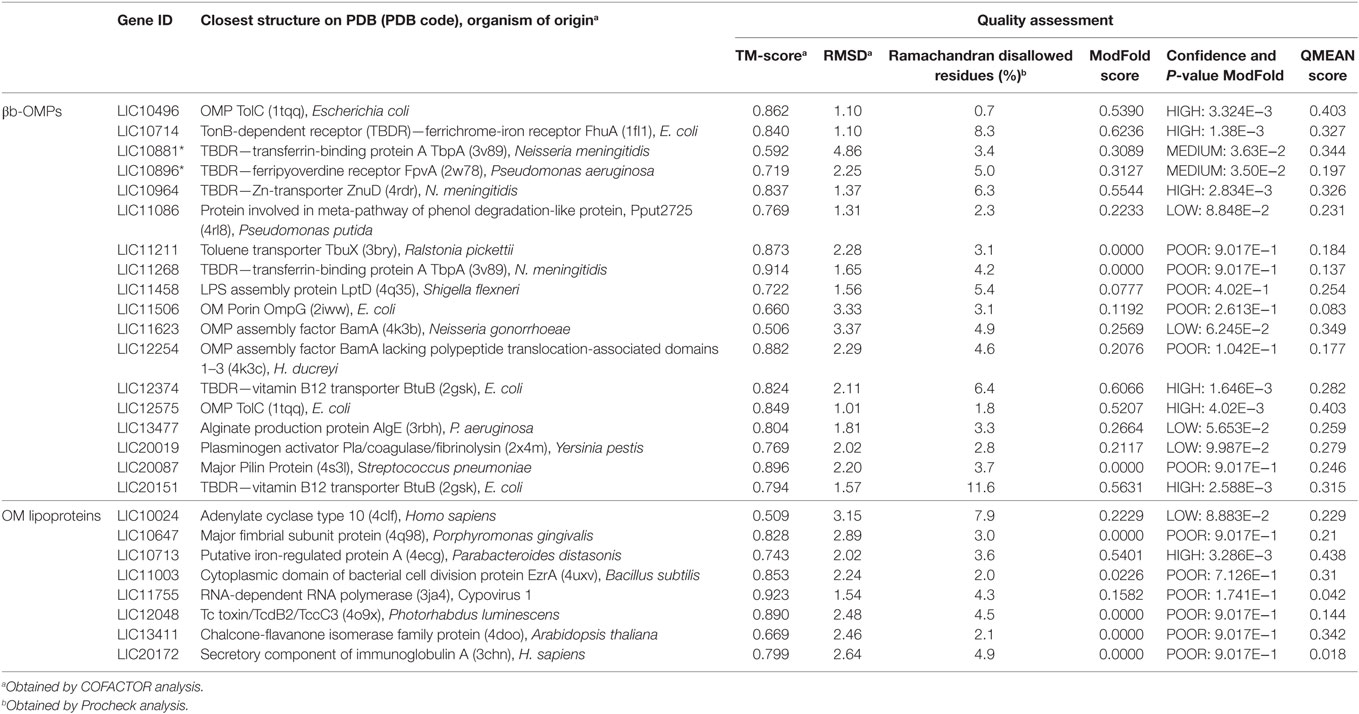
Table 3. Closest PDB structure and quality assessment for the βb-outer membrane proteins (OMPs) and outer membrane (OM) lipoproteins models.
Surface-Related Immunogenic Epitope Prediction
Due to the importance of phagocytosis in the clearance of leptospires during an infection, the presence of MHC class II-binding epitopes in the 18 βb-OMPs and 8 OM lipoproteins was evaluated. NetMHCII was used to predict 15 amino acid long peptides that can bind, at different affinity levels, to MHC class II molecules encoded by several HLAs. Predictions were made for 14 HLA-DRB alleles and only strong binder (SB) epitopes (IC50 <50 nM) were considered for analysis. Each predicted immunogenic epitope had a 9mer core that was aligned, and a consensus sequence was determined. The location of each SB epitope in their respective structural models was identified (Figure 2). The correct orientation of the βb structural models in the OM was deduced using the corresponding PDB structure as a template. The total number of 9mers and 15mers predicted for each βb-OMP and OM lipoprotein, as well as the number of surface-related 9mers and 15mers of the βb-OMPs is provided (Table 4). LIC20087 and the OM lipoproteins were not analyzed for surface-related epitopes (SREs) as they did not contain βb structures; therefore, it was not possible to determine their localization in the OM. Each immunogenic epitope (9mer core or consensus) was evaluated for conservation among the orthologous proteins. A representative set of the most conserved immunogenic SREs for each βb-OMP is shown (Figure 4). The SREs were relatively well conserved in all the βb-OMPs. The alignments of the βb-OMP immunogenic epitopes to the corresponding regions in the orthologs from pathogenic Leptospira spp. are highlighted in the alignment files (Data Sheet S1 in Supplementary Material). A list of the βb-OMP and OM lipoprotein SB epitopes for all MHC-II alleles is provided (Tables S6 and S7 in Supplementary Material, respectively).
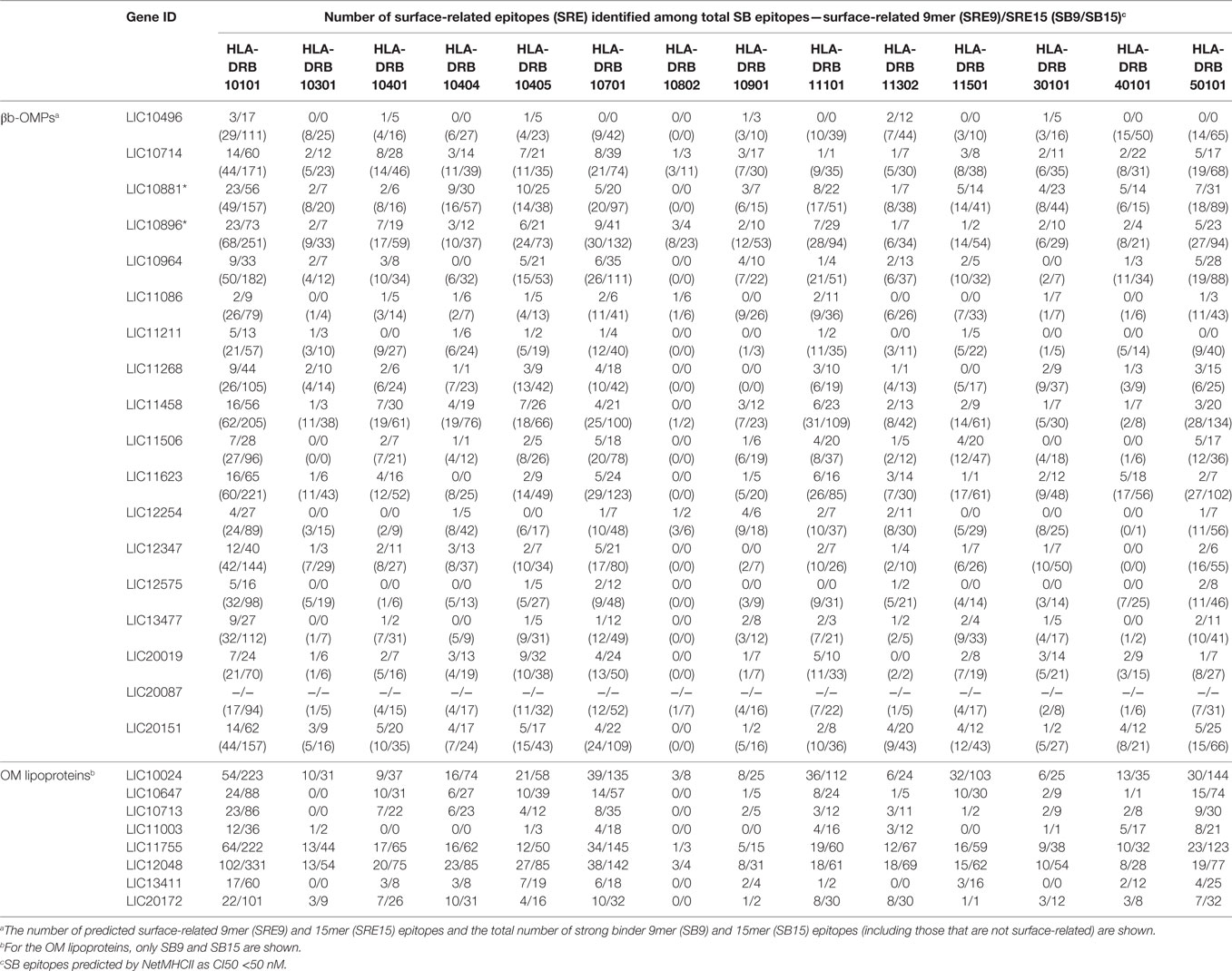
Table 4. Number of predicted strong binder (SB) major histocompatibility complex-II (MHC-II) epitopes in each βb-outer membrane protein (OMP) and outer membrane (OM) lipoproteins for 14 HLAs.
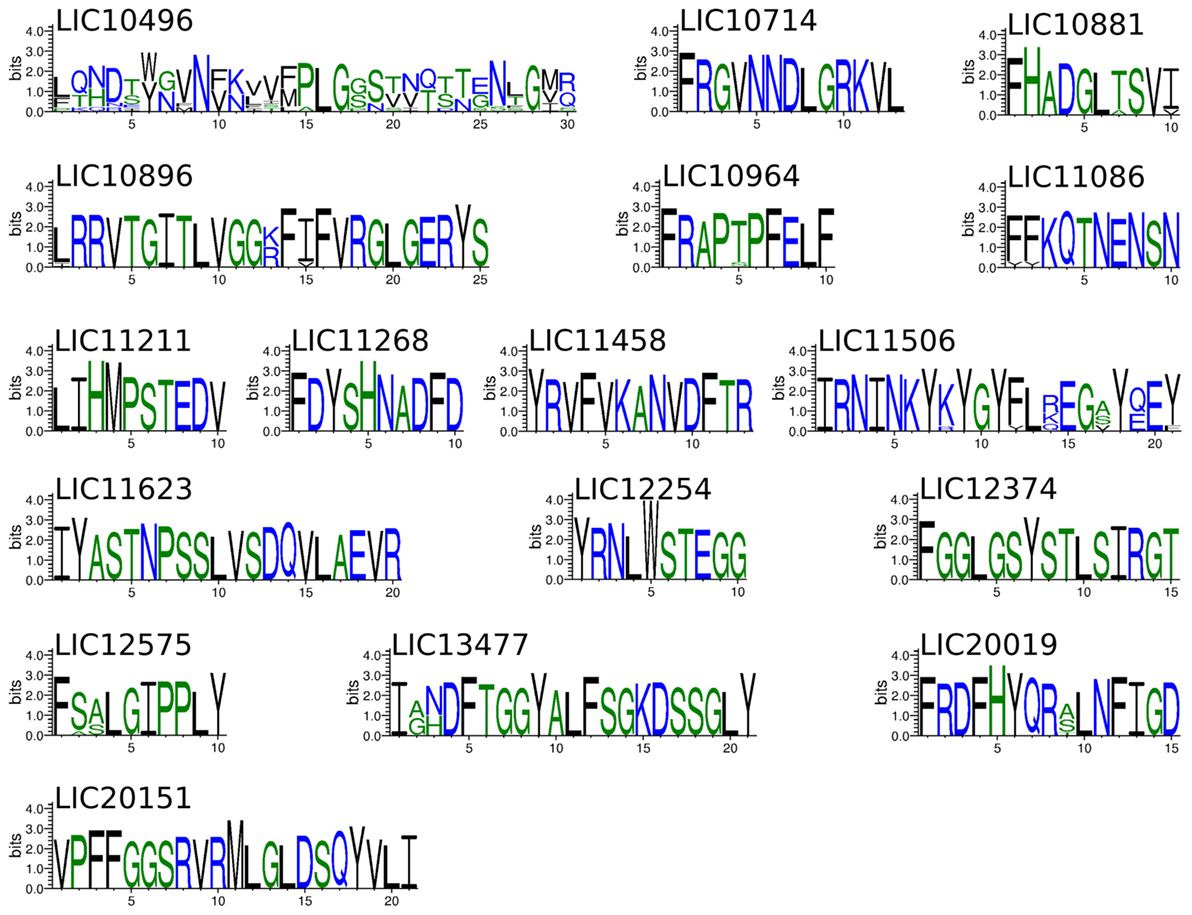
Figure 4. Sequence logos showing the frequency of amino acid residues at each position of the immunogenic surface-related epitopes (SREs) identified in the βb-outer membrane proteins (OMPs), according to multiple sequence alignment of the orthologs from pathogenic Leptospira spp. Only the most conserved SRE from each βb-OMPs is shown. The overall height of the stack indicates the level of sequence conservation at that position, while the height of symbols within the stack indicates the relative frequency of each amino at that position.
Discussion
The main principle of RV is to evaluate all the potential vaccine candidates encoded in the genome of the pathogen of interest and to reduce the number of vaccine targets to a number that can be reasonably tested in the laboratory (34). The initial screening is achieved by using bioinformatics to identify all surface-exposed proteins (potential vaccine candidates) and this typically reduces the number of targets 10-fold, from thousands to hundreds of proteins. Screening using in vitro assays further reduces the number of vaccine candidates and hence the number of laboratory animals required for efficacy testing. However, as no immune correlates for leptospirosis have been identified to date, the only way to screen the proteins identified as surface-exposed is to use a lethal animal model and look for protection (35). Therefore, the in silico identification of surface-exposed proteins must be sufficiently rigorous to screen out undesirable proteins, yet be sensitive enough to include all potential vaccine candidates.
In diderm bacteria, vaccine targets include transmembrane proteins (βb-OMPs), lipoproteins anchored to the outer leaflet of the OM, and possibly secreted proteins, particularly those that interact with the βb-OMPs. As there is no concrete evidence that Leptospira spp. secrete proteins, their role in pathogenesis is unclear, and therefore, there is no supporting data for their use as vaccine candidates. The prediction of the antiparallel transmembrane β-sheets that form βb proteins is usually achieved with a high degree of confidence (36). The identification of the SP, the βb secondary structure, the absence of TMHs, and homology to known proteins is a straightforward process. Lipoprotein prediction is based on the identification of a lipobox and localization to the OM is based on homology to known OM lipoproteins (37, 38). In the current study, 16 different bioinformatics programs were used to analyze the LIC proteome, one of the most studied Leptospira strains and one that is routinely used as the challenge strain in animal models of leptospirosis (7). A total of 165 βb-OMPs and 54 OM lipoproteins were identified in the first round of screening. However, the absence of experimental data for leptospiral proteins severely limits the use of protein sets with known subcellular localization and structures that can be used to verify the prediction based on true or false analyses. To overcome this limitation, we developed an algorithm that reduced the final target list of potential vaccine candidates to 18 βb-OMPs and 8 OM lipoproteins, each predicted with a high degree of confidence. The algorithm was intended to normalize, calculate a weight, and transform the data based on a measure of agreement among the predictors. This was achieved by giving higher weights to those predictors whose results were confirmed by other predictors and lower weights to those predictors with higher rates of disagreement; the result of the voting was expected to be more reliable, as confirmed in the current study. The bioinformatics pipeline and algorithm developed in this study, including the SV approach, can be applied to the identification of OMPs and vaccine candidates in any other diderm pathogen with a known genome sequence.
Structural vaccinology represents the cutting edge of vaccine target discovery and development. Several approaches have been investigated (29) although all are based on the common theme of protein structural data. However, most of these approaches use information from experimentally determined protein structures (e.g., X-ray diffraction), which it is time consuming and expensive, especially when compared to in silico modeling (39). In the last decade, protein structure modeling has improved enormously, as demonstrated by the critical assessment of protein structure prediction (CASP) experiments (40). I-TASSER, the modeling tool used for leptospiral βb-OMPs and OM lipoproteins structural modeling, was ranked number one for structure and function prediction in the most recent CASP experiments (40, 41). I-TASSER was shown to be as precise as structure solving by crystallography (42); it predicts structure models based on protein threading, even allowing model prediction for proteins with low sequence similarity to any other protein, as it considers sequence to structure fold recognition. Even though I-TASSER was expected to provide high-quality structural models, some of the models generated in the current study were considered poor quality, as expected for proteins with unknown functions (33). The overall quality of a protein structure prediction is indicative that it might not be similar to the template structures; however, the overall folding is representative of how a given protein folds. In the case of βb-OMPs, the most unreliable regions were the folded loops and plug domains (data not shown); however, the transmembrane β-sheets were well predicted, giving a spatial and structural insight into where the surface-exposed part of the protein is located. Therefore, poor quality models were included in the epitope prediction analysis and mapping of the SREs in the structure. Modeling was particularly important for uncharacterized proteins with no known functional domains and that had low or no similarity to other known proteins. Overall model quality can be improved by separating different domains and modeling them separately. However, this approach was not available for proteins with no functional domains and could have generated final models that did not represent the fully resolved structure (39). We generated structural models that included a βb for 17 of the 18 predicted βb-OMPs. LIC20087 was the exception and even though the structural model was highly similar to a pilin (43), it did not contain a βb structure. Although there were some low-quality scores for some of the structural models, all the predicted models had high TM-scores and low RMSDs and this allowed COFACTOR to predict function based on the structural model. Function prediction based on structure is a valuable alternative during an in silico investigation of protein function, particularly in cases where no other similarity was found.
Leptospiral βb-OMPs and OM lipoproteins represent ideal targets for vaccine development, they are surface-exposed, and therefore amenable to recognition by the host immune system. Substantial evidence supports the theory that a protective immune response is antibody-based (5); to date, only bovines have been shown to require a cellular immune response for protection (44). As leptospires are extracellular pathogens, phagocytosis plays an important role in the immune response, as pathogenic leptospires can resist complement-mediated killing (44). Following phagocytosis, proteins from the pathogen are processed by antigen-presenting cells and presented via MHC-II molecules to T-helper cells that can stimulate inflammation and activation of B-cells for antibody production (44). Therefore, we screened the novel βb-OMPs and OM lipoproteins for the presence of MHC-II SB epitopes. I-TASSER cannot predict protein structure in the context of the bacterial outer membrane. Therefore, the orientation of the OMPs models in the OM was determined by an interpretation of the orientation of the closest PDB structure from the COFACTOR analysis. We identified the location of the epitopes in the structural models of the βb-OMPs. These epitopes are likely to be exposed on the surface of the bacteria and therefore bind to MHC-II receptors, subsequently triggering an immune response. This approach is particularly relevant to βb-OMPs, these proteins usually contain long surface-exposed loops, but they also contain immunodominant regions that are not surface-exposed (Figure 2; Table 4; Table S6 in Supplementary Material). Therefore, recombinant vaccines should not use the entire βb-OMP in vaccine preparations to prevent stimulation of a non-protective immune response. The inclusion of only the surface-exposed MHC-II epitopes in the vaccine preparation would be more likely to stimulate a protective immune response. Each of the proteins included in the final target list will be discussed in detail in the following sections.
LIC10496 and LIC12575 Are TolC-Like Proteins
LIC10496 was originally annotated as a hypothetical protein and LIC12575 was annotated as a cytoplasmic membrane protein. However, their orthologs were annotated as TolC-like OMPs in the other pathogenic Leptospira spp. Furthermore, functional annotation by InterProScan predicted that they contained an OM efflux protein domain (Table 1), a property of TolC proteins, and a component of Type I secretion systems. In diderm bacteria, TolC is an OM efflux protein that forms a trimeric channel composed of a 12-stranded βb that spans the OM (4 transmembrane β-sheets per monomer) and long periplasmic α-helices that span the periplasm. The channel is connected to the cytoplasm by a TMH inner membrane protein channel. TolC transporters are involved in the transport of a variety of substrates, from small molecules to protein secretion. TolC proteins from Edwardsiella tarda (45), Listeria monocytogenes (46), and Salmonella paratyphi (47) were reported to be protective vaccine antigens. The structural models of the leptospiral TolC proteins were highly similar to an E. coli TolC protein (48). Both were characterized as high-quality models, with high ModFOLD and QMEAN scores and the lowest number of disallowed residues on the Ramachandran plot (Table 3). Interestingly, the extracellular loops connecting the β-sheets in the βb were predicted to contain MHC-II epitopes. Both leptospiral TolC-like proteins were conserved in all Leptospira spp., except for L. wolffii that has no ortholog for LIC10496. Structure-based GO predictions for these proteins suggested a possible relation to copper ions, siderophores, or protein transport (Table S5 in Supplementary Material). A previous study linked LIC12575 to the leptospiral response when exposed to in vivo like conditions (49). These data support the idea that LIC10496 and LIC12575 are promising vaccine candidates.
LIC10714, LIC10881, LIC10896, LIC10964, LIC12374, and LIC20151 Are TonB-Dependent Receptors
A TonB-dependent receptor (TBDR) domain was identified in all these proteins and this was supported by the structural models that predicted TBDRs. All the leptospiral TBDR models were reliable in terms of quality, this was expected as there is a high degree of similarity among TBDRs even in different bacteria (50). TBDRs are a βb proteins comprised of 22 amphipathic β-strands, and a globular plug domain that is folded up inside the barrel. Indeed, this structural folding was observed for LIC10881* and LIC10896* that were originally partial CDSs, supporting our finding that LIC10882 and LIC10895 are not proteins but are instead part of the LIC10881 and LIC10896 CDS, respectively, as in seen in other Leptospira spp. Interestingly, LIC10881* was absent in saprophytic and intermediate Leptospira spp., suggesting a potential role in pathogenesis. Gram-negative bacteria contain variable numbers of TBDRs, the E. coli genome contains 7 TBDRs (50); while other bacteria can contain up to 65 TBDRs (51). The LIC genome contains 13 genes that are annotated as TBDRs (52). While orthologs of LIC10714 in other Leptospira spp. genomes were annotated as TBDRs, LIC10714 was annotated as an OM receptor protein. LIC10714 contained the characteristic domains and the model included a 22-stranded βb with high structural similarity to FhuA from E. coli (53), even though it was suggested to be a leptospiral FecA (54). An ortholog of LIC10714 in L. biflexa was knocked out, resulting in a mutant with impaired ability to use iron citrate, iron chloride, iron sulfate, and aerobactin as iron sources, suggesting a role in iron metabolism (55). LIC10714 was also found to bind fibronectin (renamed as MFn2) and it was suggested that the extracellular loops could play a role in the bacterial adhesion process (56). The structural model of LIC10881* was similar to TbpA, a transferrin transporter in pathogenic Neisseria spp. (57). The LIC10896* 3D model was similar to FpvA, a pyoverdine-Fe transporter from Pseudomonas aeruginosa (58). LIC10964 was predicted to be a hemin transporter encoded by phuR, this gene is absent from all saprophytic Leptospira spp. except for L. vanthielii (Table 2). Of note, phuR was upregulated in the infection-mimicking model of leptospiral growth within dialysis membrane chambers (DMCs) implanted in the rat peritoneal cavity (59). The LIC10964 model was highly similar to the N. meningitidis zinc transporter, ZnuD (60). The LIC12374 and LIC20151 models were structurally similar to the E. coli BtuB cobalamin (vitamin B12) transporter (61). It has been suggested that pathogenic Leptospira spp. are autotrophic for vitamin B12 (12).
The actual molecule transported by a TBDR is difficult to predict by bioinformatics and should be determined experimentally. TBDRs play an important role in pathogenicity, they are conserved among the pathogenic Leptospira spp., and a significant portion of its structure was predicted to be surface-exposed. MHC-II epitopes were predicted for the TBDRs and several were located on the surface-exposed region of the protein. While TBDRs have been explored as vaccine candidates in other bacterial diseases (62–64), they have not yet been evaluated as experimental vaccines against leptospirosis. TBDRs transport essential molecules and if this is blocked, e.g., by antibodies, it would be a potentially lethal event.
Are LIC11268 and LIC13477 Alginate Transporters?
Although both proteins were originally annotated as hypothetical proteins, InterProScan found an alginate export domain in LIC11268 (Table 1). However, the structural model of LIC11268 suggested it was a βb-OMP with high structural similarity to the neisserial TbpA transferrin transporter, a known TBDR (57). LIC13477 was identified as an alginate transporter based on structural annotation (Table 3) and was structurally similar to the OMP AlgE from P. aeruginosa (65). AlgE contains 18-stranded β-sheets in the transmembrane βb and is different to other TBDRs. The predicted models of both LIC11268 and LIC13477 contained an 18-stranded βb. Although an alternative, low quality, model of LIC11268 contained 20-stranded β-sheets (data not shown). Alginate is a polysaccharide in biofilms (66) and both L. biflexa and L. interrogans contain a complete set of genes for alginate biosynthesis (52, 67). Nevertheless, alginate exporters have not yet been identified in L. interrogans. The in silico modeling of LIC11268 and LIC13477 suggested they may play a role in the transport of alginate to the extracellular milieu, but this will need to be confirmed experimentally. Both proteins were predicted to contain a βb structure, potentially spanning the leptospiral OM. Immunogenic epitopes were identified in the surface-exposed regions and both proteins were conserved among pathogenic Leptospira spp., suggesting they are promising vaccine candidates.
LIC11623 and LIC12254 Are BamA-Like βb-OMPs
Both proteins were originally annotated as OMPs, while in the current study, they were found to contain the bacterial surface antigen D15/Oma87 domains (Table 1). Of note, the orthologs of LIC11623 and LIC12254 in other Leptospira spp. were annotated as Oma87-like proteins. These BamA-like proteins are responsible for the assembly of βb-OMPs in Gram-negative bacteria. BamA is the OM component of the βb assembly machinery (Bam) complex and consists of a transmembrane βb and five polypeptide translocation-associated (POTRA) domains that extend into the periplasm. Both the βb and the five POTRA domains were identified in the LIC11623 model, and the closest PDB structure was BamA from Neisseria gonorrhoeae (68). Interestingly, although the LIC12254 model was similar to BamA, three of the POTRA domains (P1, P2, and P3) were missing. The closest PDB structure was HdBamAΔ3 from H. ducreyi, it too lacks three POTRA domains (68). Immunization with recombinant D15/Oma87 induced protective immune responses against Haemophilus influenza (69) and Pasteurella multocida (70). In addition, LIC12254 was only found in two of the saprophytic Leptospira spp., suggesting a possible role in pathogenesis. Although LIC11623 was described as a BamA-like protein in L. interrogans (52, 54), neither of these proteins have been evaluated as a vaccine candidate.
LIC11458 Is an LptD-Like Export Porin
LIC11458 was identified as LptD/OstA, a member of the OM LPS export porin (LPS-ep) family (Tables 1 and 3). In diderm bacteria, LptD is responsible for LPS assembly in the OM (71). The structural model of LIC11458 was similar to the Shigella flexneri LptD, containing a transmembrane βb and a periplasmic β-jellyroll domain (72). The predicted transmembrane architecture of this protein forms a large 26-stranded βb with immunogenic surface-exposed epitopes. LPS is an essential virulence factor in pathogenic Leptospira spp. and, in contrast to the highly variable LPS molecule, LIC11458 was conserved among Leptospira spp. An immune response directed against LIC11458, as well as stimulating opsonizing leptospires, could potentially impair LPS assembly, ultimately killing the bacteria.
LIC11086, LIC11211, and LIC11506 Are Transport Proteins
LIC11086 was predicted to contain a MetA-pathway domain for phenol degradation. The structural model of LIC11086 was highly similar to the crystal structure of Pput2725, a protein from Pseudomonas putida F1, a microorganism that can biodegrade hydrocarbons in the environment (73). Both structures contained a 12-stranded barrel with an N-terminal segment preceding the first β-strand that blocks the barrel. Proteins with this domain are predicted to transport hydrophobic molecules through the membrane, usually trichlorophenol and some are relatively well characterized (74, 75). LIC11211 was originally annotated as a hypothetical protein. The structural model of LIC11211 resembled TodX, an aromatic hydrocarbon transporter, also from P. putida (76). TodX is a 14-stranded βb, with an N-terminal flexible hatch domain. Both Pput2725 and TodX are members of the FadL family, a transporter of hydrophobic molecules in E. coli (77). P. putida and other biodegradation bacteria, such as Ralstonia pickettii, have intracellular pathways for the degradation of toxic hydrocarbons that enter the cells via FadL, with structural changes in the hatch domain (76). The role of hydrophobic molecule transporters in Leptospira spp. is unknown. LIC11506 was predicted to contain an OMP domain that is restricted to the Leptospiraceae (Leptospira and Leptonema). The structural model of LIC11506 was similar to E. coli OmpG, a βb with 14 antiparallel β-strands involved in the transport of carbohydrates into the cell (78). All three predicted leptospiral βb-OMPs have orthologs in all pathogenic strains; however, the LIC11506 and LIC11211 orthologs were absent in two species of intermediate pathogenicity (Table 2).
LIC20019 and LIC20087
Both proteins are encoded on chromosome II, LIC20019 was annotated as a hypothetical protein while LIC20087 was annotated as an OMP. However, functional annotation showed that while LIC20019 contained a putative OMP porin 6 domain, exclusive to Leptospira spp., no such domain was identified in LIC20087. Furthermore, LIC20019 was modeled as a perfect βb, while LIC20087 was the only protein among the βb-OMPs identified in the present study that did not contain a βb. The LIC20087 model displayed structural similarity to the Type II pilus protein PitB from Streptococcus pneumoniae (79). The presence of pili or their function in Leptospira spp. is unknown; however, it was predicted to be surface-exposed and represents a potential vaccine candidate. As it is not expected for pili to be intrinsic to the OM, LIC20087 was not evaluated for the presence of surface-exposed immunogenic epitopes. Whether LIC20087 was misidentified as a βb-OMP due to the presence of several β-sheets in the pilus subunit protein or due to a poor threading template model, will need to be further investigated. This protein was shown to be immunogenic during infection and a possible candidate for early leptospirosis diagnosis (80). LIC20019 was, however, modeled as an βb-OMP, with high structural similarity to the plasminogen activator Pla from Yersinia pestis (81). Pla is an OM protease (omptin) whose inactivation drastically reduces Y. pestis virulence (82). Omptins are widely distributed among Enterobacteriaceae and have several functions (83). Some leptospiral proteins have been reported to bind plasminogen, however, just a few were investigated for cellular localization. Besides an apparent redundancy in the extracellular component binding proteins in Leptospira spp., LIC20019 was predicted to be exposed on the bacterial surface and to be highly immunogenic, a potentially strong vaccine candidate.
The OM Lipoproteins
Leptospiral lipoproteins that were previously shown to be protective in the hamster model of leptospirosis, such as LigA (LIC10465), LigB (LIC10464), and LemA (LIC11058) were not selected among the list of nine predicted OM lipoproteins in this work. While these proteins were predicted by both LipoP and SpLip as lipoproteins (Table S1 in Supplementary Material), they were not consistently predicted to be located in the OM by the localization predictors. LemA was included in the list of the 54 OM lipoproteins (Table S2 in Supplementary Material) as it was identified by Cello as an OM protein. These observations are indicative that the list of 54 OM lipoproteins and 165 βb-OMPs included potential vaccine candidates that should not necessarily be excluded from future studies.
Of the eight OM lipoproteins selected, LIC10024 was the only lipoprotein originally annotated as an AGC. LIC11003 was annotated as LipL71 and the remaining six proteins were annotated as conserved hypothetical proteins or putative lipoproteins. In the current study, LIC11003 and LIC20172 were identified as peptidoglycan-binding proteins LysM (also known as LruA) and LruC, respectively. LruA and LruC were previously described as leptospiral recurrent uveitis-associated proteins A, B, and C (84, 85). LruC was experimentally demonstrated to be an OM lipoprotein but was not exposed on the bacterial surface, it was located in the inner leaflet of the OM (84). In contrast, LruA was exposed on the leptospiral surface, with a possible role in the modulation of interactions with human apolipoprotein A-I (ApoA-I), contributing to leptospiral virulence (86). Furthermore, LruA was shown to be essential for L. interrogans virulence in the hamster model (86). None of the Lru proteins have been evaluated as vaccine candidates and in order to do so, it will be necessary to exclude the regions that are responsible for leptospiral-related uveitis. LIC10024 was originally annotated as a membrane bound AGC, with an undefined cellular location. Another leptospiral protein (LA4008/LIC13201) with an AGC domain was shown to have host cell cAMP-elevating activity (87). The role of AGC proteins in leptospiral pathogenicity and the location of LIC10024 in the cell remain to be determined. Following in vitro analysis, LIC13411 was identified as a leptospiral adhesin that binds to VE-cadherin, an endothelial cell receptor (88). LIC13411 was also demonstrated to be present in the OM, supporting the findings of the current study. Unlike the βb-OMPs, the structural models of the OM lipoproteins could not be used to predict function or localization. The best matches to PDB structures included three eukaryotic proteins and a viral protein (Table 3). Most of the PDB structures identified were not bacterial surface-related proteins. This could be due to the absence of known conserved domains and low similarity to known protein structures. However, all eight lipoproteins were predicted to be OM lipoproteins and were highly conserved among Leptospira spp., including several immunogenic epitopes, suggesting they could be potential vaccine candidates.
Conclusion
We report the discovery of 26 new vaccine candidates using an innovative approach that represents the most extensive bioinformatics-based screening of vaccine targets in the field of leptospirosis and, to the best of our knowledge, is the first report using SV. The bioinformatics approach developed in this work can be applied to other diderm pathogens. The proteins identified in the current study are novel and have not yet been evaluated as vaccine candidates. We identified proteins that are likely to be functionally involved in diverse pathways, many related to pathogenesis, including iron and vitamin transport, OMP, and LPS assembly. The inhibition of these proteins by a host immune response will likely impair these essential pathways. Our group is currently evaluating a new experimental approach to confirm the subcellular location of these proteins in the leptospiral cell. Of the proteins identified in the present study, those that are surface-exposed will be evaluated as vaccine candidates in the hamster model of leptospirosis.
Materials and Methods
Genome Sequence Retrieval
The genome-derived proteome (chromosomes I and II) of L. interrogans serogroup Icterohaemorrhagiae serovar Copenhageni strain Fiocruz L1-130 was downloaded from the Leptospira Genome Project website (http://aeg.lbi.ic.unicamp.br/world/lic) in FASTA format, corresponding to GenBank accession numbers AE016823.1 and AE016824.1, respectively. High-quality draft, improved high-quality draft, or complete genomes for 20 additional Leptospira spp. (Table S4 in Supplementary Material) were obtained from GenBank.
Prediction of Primary and Secondary Structure Features
The amino acid sequences corresponding to all 3,773 CDS in both LIC chromosomes were used as the input sequences for 16 bioinformatics programs for the identification of βb integral transmembrane proteins and OM-associated lipoproteins. Subcellular localization was predicted using PSORTb v. 3.0.2 (89), CELLO v. 2.5 (90), and GNeg-mPLoc v. 2.0 (91). The presence of a SP was predicted using SignalP v. 4.1 (92), Signal-CF (93), and PrediSi (94). TMHs were predicted using MEMSAT3 (95), TMHMM v. 2.0 (96), HMMTOP v. 2.0 (97), and Phobius (98). Proteins with more than one TMH (cut-off <2 TMH) were excluded from further analysis. Proteins with a single TMH, especially when located at the N-terminus, were included as a predicted SP can be confused with a TMH. The βb-OMPs were predicted using HHomp (99), BOMP (100), MCMBB (101), and TMBETADISC-RBF (102). Leptospiral lipoproteins were predicted using LipoP v. 1.0 (38) and SpLip (37). Each program was used with the default settings and when available the option for Gram-negative bacteria was selected. Sequence data and results retrieval for each predictor was automated using Python scripts whenever possible.
Filtering Predicted Protein Features with Increased Confidence
Low agreement is expected between different bioinformatics tools when predicting OMPs (103). To reduce the impact of a prediction when naïve (unweighted) voting would result in ambiguities (e.g., two negative results and two positive results), we used an iterative weighted voting system. A Python script was used to integrate the results, increasing confidence in the consensus prediction of a protein feature identified by more than one predictor. The Consensus by Voting with Iterative Re-weighting based on Agreement (CoVIRA) algorithm, script, and several examples are available on a GitHub repository (https://github.com/biopro/covira) and the algorithm followed the logic:
where PtASj, Protein Agreement Score—the value per protein by a given predictor.
NPt—the total number of proteins evaluated.
Vi, Vote, always 1 or 0—one (1) when both predictors were in agreement, zero (0) for no agreement.
PdWi, predictor weight—the calculated weight of each predictor in the total number of votes per protein, varied by iteration.
NPd—total number of predictors used per protein feature.
In the first iteration, PdW was
From the second to the 1,000 iteration, the PdW for the next iteration was
where —the arithmetic mean per protein per predictor:
A predictor with low accuracy was expected to generate a low agreement score and as accuracy improved, so would the agreement score. Finally, the last vote, based on the final weight of each predictor, was performed to identify those βb-OMPs and OM lipoproteins with a high confidence prediction. A final vote value >0.5 (0–1 scale) for each feature of interest (SP, OM localization, etc.) was considered as a confident prediction, and these proteins were selected for further analysis. The voting algorithm was validated using a set of proteins with and without an SP. The presence of an SP was evaluated using SignalP, PreDiSi, and SignalCF. The results were analyzed by naïve voting, each predictor had an equal weight. Based on the same results, CoVIRA was executed to calculate a final score and prediction for each protein. The results were analyzed by a receiver operating characteristic (ROC) curve, and the CoVIRA prediction was improved compared to the naïve voting (see Data Sheet S2 in Supplementary Material).
Identification of Leptospira spp. Orthologs and Similar Mammalian Host Proteins
Orthologs to the selected proteins were identified in the leptospiral genome sequences (Table S1 in Supplementary Material) using the reciprocal best hit (RBH) method based on protein BLAST (BLASTp) searches. Protein sequences with >70% of similarity and >40% coverage that were also the best reciprocal hit were considered orthologs. A multiple sequence alignment was performed among orthologs using the online MUSCLE tool (MUltiple Sequence Comparison by Log-Expectation) (104). The βb-OMPs and OM lipoproteins with orthologs in other pathogenic Leptospira spp. were screened against the human, bovine, canine, equine, ovine, and swine genomes using the online Blastp server (taxIDs: Homo sapiens 9606, Bos taurus 9913, Canis familiaris 9615, Equus caballus 9796, Ovis aries 9940, and Sus scrofa domesticus 9825). Leptospiral proteins with >40% similarity to any host proteins were excluded from the final target list of potential vaccine candidates.
Structural Modeling of Predicted Surface-Exposed Leptospiral Proteins
The 3D structures of conserved βb-OMPs and OM lipoproteins were generated by protein threading. Prior to modeling, the SP sequence was manually removed from the final FASTA amino acid sequence. βb-spanning OM proteins and OM lipoprotein structures were predicted using the I-TASSER server (41, 42). I-TASSER predicted up to five alternative models and the model with the higher C-Score was refined using ModRefiner (105). Quality assessment of models was performed using ModFold4 (106), Qmean6 (107), and Procheck (108). ModFold and Qmean returned a score (0–1) that inferred the overall quality of the structure. ModFold also assigned a P-value and a degree of confidence (poor, low, medium, high, and cert) to the model. Procheck was used to analyze the stereochemistry of the refined model by evaluating the Ramachandran plot of each protein structure (109). The 3D structures were visualized using UCSF Chimera (110) and PyMol (111).
Sequence and Structural Functional Annotation
Functional annotation was performed using the InterProScan tool (112, 113). In addition, the UniProt Knowledgebase was screened using the locus tag (LIC number) to identify functional information and to access the annotation in other Leptospira spp. by KEGG database. In addition to the primary amino acid sequence analysis, a 3D structure-based functional annotation was performed using COFACTOR (114), to identify the PDB structures with the closest structure to the target protein model, and assigned GO terms for the protein model based on these PDB structures.
Epitope Prediction and OMP Structural Allocation
The presence of MHC-II linear epitopes in the amino acid sequence of the selected proteins was predicted using NetMHCII v. 2.2 (115, 116). Fourteen HLA-DRB alleles were used in the prediction of immunogenic epitopes for each protein, including the most frequent alleles in human populations. The location of SB immunogenic epitopes (threshold values of IC50 <50 nM) were determined in the 3D structures of the OMPs, those most likely located within the surface-exposed portion of the OMPs were selected. The orientation of the OMPs models in the OM was determined by an interpretation of the orientation of the closest PDB structure from the COFACTOR analysis. The predicted MHC-II epitopes were aligned to the corresponding region in the ortholog proteins from other pathogenic Leptospira spp. (Table 2) by multiple sequence alignment generated by MUSCLE, and sequence logos were generated for each epitope using WebLogo (117). Images of the structural models, highlighting the SREs were generated by PyMol.
Author Contributions
AG and AM designed the study and wrote the manuscript. FK wrote the Python scripts and automated software input whenever possible. AG, FK, JDS, and JCS performed the bioinformatics analysis. AG and JDS created the figures and tables. AG, FK, JDS, JCS, and LP analyzed data. All authors contributed to and revised the manuscript.
Conflict of Interest Statement
The authors declare that the research was conducted in the absence of any commercial or financial relationships that could be construed as a potential conflict of interest.
Acknowledgments
The authors would like to acknowledge Renata de Oliveira for the helpful review of the mathematics in this article.
Funding
This work was supported by grants 481133/2011-9 and 483052/2012-4 from the Brazilian National Council for Scientific and Technological Development (CNPq).
Supplementary Material
The Supplementary Material for this article can be found online at http://journal.frontiersin.org/article/10.3389/fimmu.2017.00463/full#supplementary-material.
Abbreviations
βb, β-barrel; βb-OMP, β-barrel transmembrane proteins; CDS, coding sequence; GO, Gene Ontology; Lig, Leptospiral immunoglobulin-like; LIC, Leptospira interrogans serovar Copenhageni strain Fiocruz L1-130; MHC, major histocompatibility complex; OM, outer membrane; OMP, outer membrane protein; POTRA, polypeptide translocation-associated domain; RMSD, root-mean-square deviation; RV, reverse vaccinology; SP, signal peptide; SV, structural vaccinology; TBDR, TonB-dependent receptor; TMH, transmembrane α-helix.
References
1. Levett PN. Systematics of leptospiraceae. Curr Top Microbiol Immunol (2015) 387:11–20. doi: 10.1007/978-3-662-45059-8_2
2. Bourhy P, Collet L, Brisse S, Picardeau M. Leptospira mayottensis sp. nov., a pathogenic species of the genus Leptospira isolated from humans. Int J Syst Evol Microbiol (2014) 64(Pt 12):4061–7. doi:10.1099/ijs.0.066597-0
3. Costa F, Hagan JE, Calcagno J, Kane M, Torgerson P, Martinez-Silveira MS, et al. Global morbidity and mortality of leptospirosis: a systematic review. PLoS Negl Trop Dis (2015) 9(9):e0003898. doi:10.1371/journal.pntd.0003898
4. Ellis WA. Animal leptospirosis. Curr Top Microbiol Immunol (2015) 387:99–137. doi:10.1007/978-3-662-45059-8_6
5. Adler B. Vaccines against leptospirosis. Curr Top Microbiol Immunol (2015) 387:251–72. doi:10.1007/978-3-662-45059-8_10
6. Haake DA, Levett PN. Leptospirosis in humans. Curr Top Microbiol Immunol (2015) 387:65–97. doi:10.1007/978-3-662-45059-8_5
7. Dellagostin OA, Grassmann AA, Hartwig DD, Felix SR, da Silva EF, McBride AJ. Recombinant vaccines against leptospirosis. Hum Vaccin (2011) 7(11):1215–24. doi:10.4161/hv.7.11.17944
8. Conrad NL, Cruz McBride FW, Souza JD, Silveira MM, Felix S, Mendonca KS, et al. LigB subunit vaccine confers sterile immunity against challenge in the hamster model of leptospirosis. PLoS Negl Trop Dis (2017) 11(3):e0005441. doi:10.1371/journal.pntd.0005441
9. Coutinho ML, Choy HA, Kelley MM, Matsunaga J, Babbitt JT, Lewis MS, et al. A LigA three-domain region protects hamsters from lethal infection by Leptospira interrogans. PLoS Negl Trop Dis (2011) 5(12):e1422. doi:10.1371/journal.pntd.0001422
10. Silva EF, Medeiros MA, McBride AJ, Matsunaga J, Esteves GS, Ramos JG, et al. The terminal portion of leptospiral immunoglobulin-like protein LigA confers protective immunity against lethal infection in the hamster model of leptospirosis. Vaccine (2007) 25(33):6277–86. doi:10.1016/j.vaccine.2007.05.053
11. Koizumi N, Watanabe H. Leptospiral immunoglobulin-like proteins elicit protective immunity. Vaccine (2004) 22(11–12):1545–52. doi:10.1016/j.vaccine.2003.10.007
12. Fouts DE, Matthias MA, Adhikarla H, Adler B, Amorim-Santos L, Berg DE, et al. What makes a bacterial species pathogenic? Comparative genomic analysis of the genus Leptospira. PLoS Negl Trop Dis (2016) 10(2):e0004403. doi:10.1371/journal.pntd.0004403
13. Seixas FK, Fernandes CH, Hartwig DD, Conceicao FR, Aleixo JA, Dellagostin OA. Evaluation of different ways of presenting LipL32 to the immune system with the aim of developing a recombinant vaccine against leptospirosis. Can J Microbiol (2007) 53(4):472–9. doi:10.1139/w06-138
14. Branger C, Chatrenet B, Gauvrit A, Aviat F, Aubert A, Bach JM, et al. Protection against Leptospira interrogans sensu lato challenge by DNA immunization with the gene encoding hemolysin-associated protein 1. Infect Immun (2005) 73(7):4062–9. doi:10.1128/IAI.73.7.4062-4069.2005
15. Branger C, Sonrier C, Chatrenet B, Klonjkowski B, Ruvoen-Clouet N, Aubert A, et al. Identification of the hemolysis-associated protein 1 as a cross-protective immunogen of Leptospira interrogans by adenovirus-mediated vaccination. Infect Immun (2001) 69(11):6831–8. doi:10.1128/IAI.69.11.6831-6838.2001
16. Sonrier C, Branger C, Michel V, Ruvoen-Clouet N, Ganiere JP, Andre-Fontaine G. Evidence of cross-protection within Leptospira interrogans in an experimental model. Vaccine (2000) 19(1):86–94. doi:10.1016/S0264-410X(00)00129-8
17. Grassmann AA, Felix SR, dos Santos CX, Amaral MG, Seixas Neto AC, Fagundes MQ, et al. Protection against lethal leptospirosis after vaccination with LipL32 coupled or coadministered with the B subunit of Escherichia coli heat-labile enterotoxin. Clin Vaccine Immunol (2012) 19(5):740–5. doi:10.1128/CVI.05720-11
18. Hartwig DD, Forster KM, Oliveira TL, Amaral M, McBride AJ, Dellagostin OA. A prime-boost strategy using the novel vaccine candidate, LemA, protects hamsters against leptospirosis. Clin Vaccine Immunol (2013) 20(5):747–52. doi:10.1128/CVI.00034-13
19. Hartwig DD, Seixas FK, Cerqueira GM, McBride AJ, Dellagostin OA. Characterization of the immunogenic and antigenic potential of putative lipoproteins from Leptospira interrogans. Curr Microbiol (2011) 62(4):1337–41. doi:10.1007/s00284-010-9865-1
20. Oliveira TL, Grassmann AA, Schuch RA, Seixas Neto AC, Mendonca M, Hartwig DD, et al. Evaluation of the Leptospira interrogans outer membrane protein OmpL37 as a vaccine candidate. PLoS One (2015) 10(11):e0142821. doi:10.1371/journal.pone.0142821
21. Grassmann AA, Souza JD, McBride AJ. A universal vaccine against leptospirosis: are we going in the right direction? Front Immunol (2017) 8:256. doi:10.3389/fimmu.2017.00256
22. Rappuoli R. Reverse vaccinology, a genome-based approach to vaccine development. Vaccine (2001) 19(17–19):2688–91. doi:10.1016/S0264-410X(00)00554-5
23. Serruto D, Bottomley MJ, Ram S, Giuliani MM, Rappuoli R. The new multicomponent vaccine against meningococcal serogroup B, 4CMenB: immunological, functional and structural characterization of the antigens. Vaccine (2012) 30(Suppl 2):B87–97. doi:10.1016/j.vaccine.2012.01.033
24. Murray GL, Lo M, Bulach DM, Srikram A, Seemann T, Quinsey NS, et al. Evaluation of 238 antigens of Leptospira borgpetersenii serovar Hardjo for protection against kidney colonisation. Vaccine (2013) 31(3):495–9. doi:10.1016/j.vaccine.2012.11.028
25. Dellagostin OA, Grassmann AA, Rizzi C, Schuch RA, Jorge S, Oliveira TL, et al. Reverse vaccinology: an approach for identifying leptospiral vaccine candidates. Int J Mol Sci (2017) 18(1):158. doi:10.3390/ijms18010158
26. Schulz GE. The structure of bacterial outer membrane proteins. Biochim Biophys Acta (2002) 1565(2):308–17. doi:10.1016/S0005-2736(02)00577-1
27. Wilson MM, Bernstein HD. Surface-exposed lipoproteins: an emerging secretion phenomenon in Gram-negative bacteria. Trends Microbiol (2016) 24(3):198–208. doi:10.1016/j.tim.2015.11.006
28. Dong C, Beis K, Nesper J, Brunkan-Lamontagne AL, Clarke BR, Whitfield C, et al. Wza the translocon for E. coli capsular polysaccharides defines a new class of membrane protein. Nature (2006) 444(7116):226–9. doi:10.1038/nature05267
29. Dormitzer PR, Grandi G, Rappuoli R. Structural vaccinology starts to deliver. Nat Rev Microbiol (2012) 10(12):807–13. doi:10.1038/nrmicro2893
30. Finco O, Rappuoli R. Designing vaccines for the twenty-first century society. Front Immunol (2014) 5:12. doi:10.3389/fimmu.2014.00012
31. Koehler Leman J, Ulmschneider MB, Gray JJ. Computational modeling of membrane proteins. Proteins (2015) 83(1):1–24. doi:10.1002/prot.24703
32. Baker M. Making membrane proteins for structures: a trillion tiny tweaks. Nat Methods (2010) 7(6):429–34. doi:10.1038/nmeth0610-429
33. Khor BY, Tye GJ, Lim TS, Choong YS. General overview on structure prediction of twilight-zone proteins. Theor Biol Med Model (2015) 12:15. doi:10.1186/s12976-015-0014-1
34. Delany I, Rappuoli R, Seib KL. Vaccines, reverse vaccinology, and bacterial pathogenesis. Cold Spring Harb Perspect Med (2013) 3(5):a012476. doi:10.1101/cshperspect.a012476
35. McBride AJ, Athanazio DA, Reis MG, Ko AI. Leptospirosis. Curr Opin Infect Dis (2005) 18(5):376–86. doi:10.1097/01.qco.0000178824.05715.2c
36. Casadio R, Martelli PL, Bartoli L, Fariselli P. Topology prediction of membrane proteins: how distantly related homologs come into play. In: Frishman D, editor. Structural Bioinformatics of Membrane Proteins. New York: Springer (2010). p. 61–82. doi:10.1007/978-3-7091-0045-5_4
37. Setubal JC, Reis M, Matsunaga J, Haake DA. Lipoprotein computational prediction in spirochaetal genomes. Microbiology (2006) 152(Pt 1):113–21. doi:10.1099/mic.0.28317-0
38. Juncker AS, Willenbrock H, Von Heijne G, Brunak S, Nielsen H, Krogh A. Prediction of lipoprotein signal peptides in Gram-negative bacteria. Protein Sci (2003) 12(8):1652–62. doi:10.1110/ps.0303703
39. Gupta CL, Akhtar S, Bajpai P. In silico protein modeling: possibilities and limitations. EXCLI J (2014) 13:513–5.
40. Moult J, Fidelis K, Kryshtafovych A, Schwede T, Tramontano A. Critical assessment of methods of protein structure prediction (CASP)—round x. Proteins (2014) 82(Suppl 2):1–6. doi:10.1002/prot.24452
41. Yang J, Zhang Y. Protein structure and function prediction using I-TASSER. Curr Protoc Bioinformatics (2015) 52:5.8.1–15. doi:10.1002/0471250953.bi0508s52
42. Zhang Y. I-TASSER server for protein 3D structure prediction. BMC Bioinformatics (2008) 9:40. doi:10.1186/1471-2105-9-40
43. Shaik MM, Lombardi C, Maragno Trindade D, Fenel D, Schoehn G, Di Guilmi AM, et al. A structural snapshot of type II pilus formation in Streptococcus pneumoniae. J Biol Chem (2015) 290(37):22581–92. doi:10.1074/jbc.M115.647834
44. Zuerner RL. Host response to Leptospira infection. Curr Top Microbiol Immunol (2015) 387:223–50. doi:10.1007/978-3-662-45059-8_9
45. Wang C, Peng B, Li H, Peng XX. TolC plays a crucial role in immune protection conferred by Edwardsiella tarda whole-cell vaccines. Sci Rep (2016) 6:29488. doi:10.1038/srep29488
46. Spreng S, Dietrich G, Goebel W, Gentschev I. Protection against murine listeriosis by oral vaccination with recombinant Salmonella expressing protective listerial epitopes within a surface-exposed loop of the TolC-protein. Vaccine (2003) 21(7–8):746–52. doi:10.1016/S0264-410X(02)00594-7
47. Yang TC, Ma XC, Liu F, Lin LR, Liu LL, Liu GL, et al. Screening of the Salmonella paratyphi A CMCC 50973 strain outer membrane proteins for the identification of potential vaccine targets. Mol Med Rep (2012) 5(1):78–83. doi:10.3892/mmr.2011.587
48. Higgins MK, Eswaran J, Edwards P, Schertler GF, Hughes C, Koronakis V. Structure of the ligand-blocked periplasmic entrance of the bacterial multidrug efflux protein TolC. J Mol Biol (2004) 342(3):697–702. doi:10.1016/j.jmb.2004.07.088
49. Eshghi A, Cullen PA, Cowen L, Zuerner RL, Cameron CE. Global proteome analysis of Leptospira interrogans. J Proteome Res (2009) 8(10):4564–78. doi:10.1021/pr9004597
50. Noinaj N, Guillier M, Barnard TJ, Buchanan SK. TonB-dependent transporters: regulation, structure, and function. Annu Rev Microbiol (2010) 64:43–60. doi:10.1146/annurev.micro.112408.134247
51. Nierman WC, Feldblyum TV, Laub MT, Paulsen IT, Nelson KE, Eisen JA, et al. Complete genome sequence of Caulobacter crescentus. Proc Natl Acad Sci U S A (2001) 98(7):4136–41. doi:10.1073/pnas.061029298
52. Nascimento AL, Verjovski-Almeida S, Van SMA, Monteiro-Vitorello CB, Camargo LE, Digiampietri LA, et al. Genome features of Leptospira interrogans serovar Copenhageni. Braz J Med Biol Res (2004) 37(4):459–77. doi:10.1590/S0100-879X2004000400003
53. Ferguson AD, Kodding J, Walker G, Bos C, Coulton JW, Diederichs K, et al. Active transport of an antibiotic rifamycin derivative by the outer-membrane protein FhuA. Structure (2001) 9(8):707–16. doi:10.1016/S0969-2126(01)00631-1
54. Haake DA, Matsunaga J. Leptospira: a spirochaete with a hybrid outer membrane. Mol Microbiol (2010) 77(4):805–14. doi:10.1111/j.1365-2958.2010.07262.x
55. Louvel H, Bommezzadri S, Zidane N, Boursaux-Eude C, Creno S, Magnier A, et al. Comparative and functional genomic analyses of iron transport and regulation in Leptospira spp. J Bacteriol (2006) 188(22):7893–904. doi:10.1128/JB.00711-06
56. Pinne M, Matsunaga J, Haake DA. Leptospiral outer membrane protein microarray, a novel approach to identification of host ligand-binding proteins. J Bacteriol (2012) 194(22):6074–87. doi:10.1128/JB.01119-12
57. Noinaj N, Buchanan SK, Cornelissen CN. The transferrin-iron import system from pathogenic Neisseria species. Mol Microbiol (2012) 86(2):246–57. doi:10.1111/mmi.12002
58. Greenwald J, Nader M, Celia H, Gruffaz C, Geoffroy V, Meyer JM, et al. FpvA bound to non-cognate pyoverdines: molecular basis of siderophore recognition by an iron transporter. Mol Microbiol (2009) 72(5):1246–59. doi:10.1111/j.1365-2958.2009.06721.x
59. Caimano MJ, Sivasankaran SK, Allard A, Hurley D, Hokamp K, Grassmann AA, et al. A model system for studying the transcriptomic and physiological changes associated with mammalian host-adaptation by Leptospira interrogans serovar Copenhageni. PLoS Pathog (2014) 10(3):e1004004. doi:10.1371/journal.ppat.1004004
60. Calmettes C, Ing C, Buckwalter CM, El Bakkouri M, Chieh-Lin Lai C, Pogoutse A, et al. The molecular mechanism of Zinc acquisition by the neisserial outer-membrane transporter ZnuD. Nat Commun (2015) 6:7996. doi:10.1038/ncomms8996
61. Shultis DD, Purdy MD, Banchs CN, Wiener MC. Crystallization and preliminary X-ray crystallographic analysis of the Escherichia coli outer membrane cobalamin transporter BtuB in complex with the carboxy-terminal domain of TonB. Acta Crystallogr Sect F Struct Biol Cryst Commun (2006) 62(Pt 7):638–41. doi:10.1107/S1744309106018240
62. Hu YH, Dang W, Sun L. A TonB-dependent outer membrane receptor of Pseudomonas fluorescens: virulence and vaccine potential. Arch Microbiol (2012) 194(9):795–802. doi:10.1007/s00203-012-0812-3
63. Kalbina I, Engstrand L, Andersson S, Strid A. Expression of Helicobacter pylori TonB protein in transgenic Arabidopsis thaliana: toward production of vaccine antigens in plants. Helicobacter (2010) 15(5):430–7. doi:10.1111/j.1523-5378.2010.00786.x
64. Cornelissen CN, Hollander A. TonB-dependent transporters expressed by Neisseria gonorrhoeae. Front Microbiol (2011) 2:117. doi:10.3389/fmicb.2011.00117
65. Hay ID, Rehman ZU, Rehm BH. Membrane topology of outer membrane protein AlgE, which is required for alginate production in Pseudomonas aeruginosa. Appl Environ Microbiol (2010) 76(6):1806–12. doi:10.1128/AEM.02945-09
66. Remminghorst U, Rehm BH. In vitro alginate polymerization and the functional role of Alg8 in alginate production by Pseudomonas aeruginosa. Appl Environ Microbiol (2006) 72(1):298–305. doi:10.1128/AEM.72.1.298-305.2006
67. Picardeau M, Bulach DM, Bouchier C, Zuerner RL, Zidane N, Wilson PJ, et al. Genome sequence of the saprophyte Leptospira biflexa provides insights into the evolution of Leptospira and the pathogenesis of leptospirosis. PLoS One (2008) 3(2):e1607. doi:10.1371/journal.pone.0001607
68. Noinaj N, Kuszak AJ, Gumbart JC, Lukacik P, Chang H, Easley NC, et al. Structural insight into the biogenesis of beta-barrel membrane proteins. Nature (2013) 501(7467):385–90. doi:10.1038/nature12521
69. Loosmore SM, Yang YP, Coleman DC, Shortreed JM, England DM, Klein MH. Outer membrane protein D15 is conserved among Haemophilus influenzae species and may represent a universal protective antigen against invasive disease. Infect Immun (1997) 65(9):3701–7.
70. Ruffolo CG, Adler B. Cloning, sequencing, expression, and protective capacity of the oma87 gene encoding the Pasteurella multocida 87-kilodalton outer membrane antigen. Infect Immun (1996) 64(8):3161–7.
72. Botos I, Majdalani N, Mayclin SJ, McCarthy JG, Lundquist K, Wojtowicz D, et al. Structural and functional characterization of the LPS transporter LptDE from Gram-negative pathogens. Structure (2016) 24(6):965–76. doi:10.1016/j.str.2016.03.026
73. van den Berg B, Bhamidimarri SP, Winterhalter M. Crystal structure of a COG4313 outer membrane channel. Sci Rep (2015) 5:11927. doi:10.1038/srep11927
74. Belchik SM, Schaeffer SM, Hasenoehrl S, Xun L. A beta-barrel outer membrane protein facilitates cellular uptake of polychlorophenols in Cupriavidus necator. Biodegradation (2010) 21(3):431–9. doi:10.1007/s10532-009-9313-8
75. LaBauve AE, Wargo MJ. Detection of host-derived sphingosine by Pseudomonas aeruginosa is important for survival in the murine lung. PLoS Pathog (2014) 10(1):e1003889. doi:10.1371/journal.ppat.1003889
76. Hearn EM, Patel DR, van den Berg B. Outer-membrane transport of aromatic hydrocarbons as a first step in biodegradation. Proc Natl Acad Sci U S A (2008) 105(25):8601–6. doi:10.1073/pnas.0801264105
77. Black PN. The fadL gene product of Escherichia coli is an outer membrane protein required for uptake of long-chain fatty acids and involved in sensitivity to bacteriophage T2. J Bacteriol (1988) 170(6):2850–4. doi:10.1128/jb.170.6.2850-2854.1988
78. Koster S, van Pee K, Yildiz O. Purification, refolding, and crystallization of the outer membrane protein OmpG from Escherichia coli. Methods Enzymol (2015) 557:149–66. doi:10.1016/bs.mie.2015.01.018
79. Zahner D, Gandhi AR, Stuchlik O, Reed M, Pohl J, Stephens DS. Pilus backbone protein PitB of Streptococcus pneumoniae contains stabilizing intramolecular isopeptide bonds. Biochem Biophys Res Commun (2011) 409(3):526–31. doi:10.1016/j.bbrc.2011.05.038
80. Lessa-Aquino C, Borges Rodrigues C, Pablo J, Sasaki R, Jasinskas A, Liang L, et al. Identification of seroreactive proteins of Leptospira interrogans serovar Copenhageni using a high-density protein microarray approach. PLoS Negl Trop Dis (2013) 7(10):e2499. doi:10.1371/journal.pntd.0002499
81. Ruback E, Lobo LA, Franca TC, Pascutti PG. Structural analysis of Pla protein from the biological warfare agent Yersinia pestis: docking and molecular dynamics of interactions with the mammalian plasminogen system. J Biomol Struct Dyn (2013) 31(5):477–84. doi:10.1080/07391102.2012.703072
82. Suomalainen M, Haiko J, Ramu P, Lobo L, Kukkonen M, Westerlund-Wikstrom B, et al. Using every trick in the book: the Pla surface protease of Yersinia pestis. Adv Exp Med Biol (2007) 603:268–78. doi:10.1007/978-0-387-72124-8_24
83. Hritonenko V, Stathopoulos C. Omptin proteins: an expanding family of outer membrane proteases in Gram-negative enterobacteriaceae. Mol Membr Biol (2007) 24(5–6):395–406. doi:10.1080/09687680701443822
84. Verma A, Matsunaga J, Artiushin S, Pinne M, Houwers DJ, Haake DA, et al. Antibodies to a novel leptospiral protein, LruC, in the eye fluids and sera of horses with Leptospira-associated uveitis. Clin Vaccine Immunol (2012) 19(3):452–6. doi:10.1128/CVI.05524-11
85. Verma A, Artiushin S, Matsunaga J, Haake DA, Timoney JF. LruA and LruB, novel lipoproteins of pathogenic leptospira interrogans associated with equine recurrent uveitis. Infect Immun (2005) 73(11):7259–66. doi:10.1128/IAI.73.11.7259-7266.2005
86. Zhang K, Murray GL, Seemann T, Srikram A, Bartpho T, Sermswan RW, et al. Leptospiral LruA is required for virulence and modulates an interaction with mammalian apolipoprotein AI. Infect Immun (2013) 81(10):3872–9. doi:10.1128/IAI.01195-12
87. Lehmann JS, Fouts DE, Haft DH, Cannella AP, Ricaldi JN, Brinkac L, et al. Pathogenomic inference of virulence-associated genes in Leptospira interrogans. PLoS Negl Trop Dis (2013) 7(10):e2468. doi:10.1371/journal.pntd.0002468
88. Evangelista KV, Hahn B, Wunder EA Jr, Ko AI, Haake DA, Coburn J. Identification of cell-binding adhesins of Leptospira interrogans. PLoS Negl Trop Dis (2014) 8(10):e3215. doi:10.1371/journal.pntd.0003215
89. Yu NY, Wagner JR, Laird MR, Melli G, Rey S, Lo R, et al. PSORTb 3.0: improved protein subcellular localization prediction with refined localization subcategories and predictive capabilities for all prokaryotes. Bioinformatics (2010) 26(13):1608–15. doi:10.1093/bioinformatics/btq249
90. Yu CS, Chen YC, Lu CH, Hwang JK. Prediction of protein subcellular localization. Proteins (2006) 64(3):643–51. doi:10.1002/prot.21018
91. Shen HB, Chou KC. Gneg-mPLoc: a top-down strategy to enhance the quality of predicting subcellular localization of Gram-negative bacterial proteins. J Theor Biol (2010) 264(2):326–33. doi:10.1016/j.jtbi.2010.01.018
92. Petersen TN, Brunak S, von Heijne G, Nielsen H. SignalP 4.0: discriminating signal peptides from transmembrane regions. Nat Methods (2011) 8(10):785–6. doi:10.1038/nmeth.1701
93. Chou KC, Shen HB. Signal-CF: a subsite-coupled and window-fusing approach for predicting signal peptides. Biochem Biophys Res Commun (2007) 357(3):633–40. doi:10.1016/j.bbrc.2007.03.162
94. Hiller K, Grote A, Scheer M, Munch R, Jahn D. PrediSi: prediction of signal peptides and their cleavage positions. Nucleic Acids Res (2004) 32(Web Server issue):W375–9. doi:10.1093/nar/gkh378
95. Jones DT. Improving the accuracy of transmembrane protein topology prediction using evolutionary information. Bioinformatics (2007) 23(5):538–44. doi:10.1093/bioinformatics/btl677
96. Krogh A, Larsson B, von Heijne G, Sonnhammer EL. Predicting transmembrane protein topology with a hidden Markov model: application to complete genomes. J Mol Biol (2001) 305(3):567–80. doi:10.1006/jmbi.2000.4315
97. Tusnady GE, Simon I. The HMMTOP transmembrane topology prediction server. Bioinformatics (2001) 17(9):849–50. doi:10.1093/bioinformatics/17.9.849
98. Kall L, Krogh A, Sonnhammer EL. A combined transmembrane topology and signal peptide prediction method. J Mol Biol (2004) 338(5):1027–36. doi:10.1016/j.jmb.2004.03.016
99. Remmert M, Linke D, Lupas AN, Soding J. HHomp—prediction and classification of outer membrane proteins. Nucleic Acids Res (2009) 37(Web Server issue):W446–51. doi:10.1093/nar/gkp325
100. Berven FS, Flikka K, Jensen HB, Eidhammer I. BOMP: a program to predict integral beta-barrel outer membrane proteins encoded within genomes of Gram-negative bacteria. Nucleic Acids Res (2004) 32(Web Server issue):W394–9. doi:10.1093/nar/gkh351
101. Bagos PG, Liakopoulos TD, Hamodrakas SJ. Finding beta-barrel outer membrane proteins with a Markov chain model. WSEAS Trans Biol Biomed (2004) 2(1):186–9.
102. Ou YY, Gromiha MM, Chen SA, Suwa M. TMBETADISC-RBF: discrimination of beta-barrel membrane proteins using RBF networks and PSSM profiles. Comput Biol Chem (2008) 32(3):227–31. doi:10.1016/j.compbiolchem.2008.03.002
103. Diaz-Mejia JJ, Babu M, Emili A. Computational and experimental approaches to chart the Escherichia coli cell-envelope-associated proteome and interactome. FEMS Microbiol Rev (2009) 33(1):66–97. doi:10.1111/j.1574-6976.2008.00141.x
104. Edgar RC. MUSCLE: multiple sequence alignment with high accuracy and high throughput. Nucleic Acids Res (2004) 32(5):1792–7. doi:10.1093/nar/gkh340
105. Xu D, Zhang Y. Improving the physical realism and structural accuracy of protein models by a two-step atomic-level energy minimization. Biophys J (2011) 101(10):2525–34. doi:10.1016/j.bpj.2011.10.024
106. McGuffin LJ, Buenavista MT, Roche DB. The ModFOLD4 server for the quality assessment of 3D protein models. Nucleic Acids Res (2013) 41(Web Server issue):W368–72. doi:10.1093/nar/gkt294
107. Benkert P, Kunzli M, Schwede T. QMEAN server for protein model quality estimation. Nucleic Acids Res (2009) 37(Web Server issue):W510–4. doi:10.1093/nar/gkp322
108. Morris AL, MacArthur MW, Hutchinson EG, Thornton JM. Stereochemical quality of protein structure coordinates. Proteins (1992) 12(4):345–64. doi:10.1002/prot.340120407
109. Laskowski RA, MacArthur MW, Moss DS, Thornton JM. PROCHECK—a program to check the stereochemical quality of protein structures. J Appl Crystal (1993) 26(2):283–91. doi:10.1107/S0021889892009944
110. Pettersen EF, Goddard TD, Huang CC, Couch GS, Greenblatt DM, Meng EC, et al. UCSF Chimera—a visualization system for exploratory research and analysis. J Comput Chem (2004) 25(13):1605–12. doi:10.1002/jcc.20084
112. Mitchell A, Chang HY, Daugherty L, Fraser M, Hunter S, Lopez R, et al. The InterPro protein families database: the classification resource after 15 years. Nucleic Acids Res (2015) 43(Database issue):D213–21. doi:10.1093/nar/gku1243
113. Jones P, Binns D, Chang HY, Fraser M, Li W, McAnulla C, et al. InterProScan 5: genome-scale protein function classification. Bioinformatics (2014) 30(9):1236–40. doi:10.1093/bioinformatics/btu031
114. Roy A, Yang J, Zhang Y. COFACTOR: an accurate comparative algorithm for structure-based protein function annotation. Nucleic Acids Res (2012) 40(Web Server issue):W471–7. doi:10.1093/nar/gks372
115. Nielsen M, Lund O. NN-align. An artificial neural network-based alignment algorithm for MHC class II peptide binding prediction. BMC Bioinformatics (2009) 10:296. doi:10.1186/1471-2105-10-296
116. Nielsen M, Lund O, Buus S, Lundegaard C. MHC class II epitope predictive algorithms. Immunology (2010) 130(3):319–28. doi:10.1111/j.1365-2567.2010.03268.x
Keywords: Leptospira interrogans, outer membrane protein, epitope prediction, bioinformatics, transport proteins, structural modeling, genome mining, diderm bacteria
Citation: Grassmann AA, Kremer FS, Santos JC, Souza JD, Pinto LS and McBride AJA (2017) Discovery of Novel Leptospirosis Vaccine Candidates Using Reverse and Structural Vaccinology. Front. Immunol. 8:463. doi: 10.3389/fimmu.2017.00463
Received: 30 October 2016; Accepted: 04 April 2017;
Published: 27 April 2017
Edited by:
Monica E. Embers, Tulane University, USAReviewed by:
Catherine Ayn Brissette, University of North Dakota, USAMaria Gomes-Solecki, University of Tennessee Health Science Center, USA
Copyright: © 2017 Grassmann, Kremer, Santos, Souza, Pinto and McBride. This is an open-access article distributed under the terms of the Creative Commons Attribution License (CC BY). The use, distribution or reproduction in other forums is permitted, provided the original author(s) or licensor are credited and that the original publication in this journal is cited, in accordance with accepted academic practice. No use, distribution or reproduction is permitted which does not comply with these terms.
*Correspondence: Alan John Alexander McBride, YWxhbi5tY2JyaWRlQHVmcGVsLmVkdS5icg==