- 1Isermann Department of Chemical and Biological Engineering, Center for Biotechnology and Interdisciplinary Studies, Rensselaer Polytechnic Institute, Troy, NY, United States
- 2Department of Chemical Engineering, The Pennsylvania State University, University Park, PA, United States
The identification of mutations that enhance antibody affinity while maintaining high antibody specificity and stability is a time-consuming and laborious process. Here, we report an efficient methodology for systematically and rapidly enhancing the affinity of antibody variable domains while maximizing specificity and stability using novel synthetic antibody libraries. Our approach first uses computational and experimental alanine scanning mutagenesis to identify sites in the complementarity-determining regions (CDRs) that are permissive to mutagenesis while maintaining antigen binding. Next, we mutagenize the most permissive CDR positions using degenerate codons to encode wild-type residues and a small number of the most frequently occurring residues at each CDR position based on natural antibody diversity. This mutagenesis approach results in antibody libraries with variants that have a wide range of numbers of CDR mutations, including antibody domains with single mutations and others with tens of mutations. Finally, we sort the modest size libraries (~10 million variants) displayed on the surface of yeast to identify CDR mutations with the greatest increases in affinity. Importantly, we find that single-domain (VHH) antibodies specific for the α-synuclein protein (whose aggregation is associated with Parkinson’s disease) with the greatest gains in affinity (>5-fold) have several (four to six) CDR mutations. This finding highlights the importance of sampling combinations of CDR mutations during the first step of affinity maturation to maximize the efficiency of the process. Interestingly, we find that some natural diversity mutations simultaneously enhance all three key antibody properties (affinity, specificity, and stability) while other mutations enhance some of these properties (e.g., increased specificity) and display trade-offs in others (e.g., reduced affinity and/or stability). Computational modeling reveals that improvements in affinity are generally not due to direct interactions involving CDR mutations but rather due to indirect effects that enhance existing interactions and/or promote new interactions between the antigen and wild-type CDR residues. We expect that natural diversity mutagenesis will be useful for efficient affinity maturation of a wide range of antibody fragments and full-length antibodies.
Introduction
The widespread interest in using antibodies in diagnostic and therapeutic applications has led to considerable efforts in developing methods for optimizing their properties (1–6). Methods for improving antibody affinity are particularly important because lead antibodies identified using in vivo (immunization) and in vitro (e.g., phage display) methods typically do not have high enough affinity for therapeutic applications. Moreover, improvements in antibody affinity are generally expected to enhance the performance of diagnostic antibodies due to improved specificity at reduced antibody concentrations. Methods such as phage, yeast surface and ribosome display are commonly used for in vitro affinity maturation because of their many attractive properties (7–13). These properties include the ability to precisely control antigen presentation, conformation, and concentration as well as the ability to perform negative selections against various types of non-antigens to eliminate non-specific variants (14–17). These display methods have been used to achieve large enhancements in affinity for a wide variety of antibody fragments and full-length antibodies (9, 18–23).
Nevertheless, there are several outstanding challenges related to in vitro affinity maturation that need to be addressed. First, while it is possible to use saturation mutagenesis to evaluate every possible single mutation in antibody complementarity-determining regions (CDRs), single mutations typically do not result in large gains in affinity (1, 3, 24). Therefore, it is often necessary to generate sub-libraries to identify combinations of single mutations that result in large increases in affinity, which is a slow and laborious process. Second, it is not possible to test all combinations of single and multiple mutations in the CDRs of antibodies in a single library due to intractably large library sizes. For example, a library size of >1039 would be required to sample all possible combinations of single and multiple mutations at ~30 residues in the CDRs of typical variable domains. This means that only an extremely small subset of the possible single and multiple mutations can be tested using display methods, which is largely dictated by transformation efficiencies [~109–1010 for phage (25, 26) and ~107–108 for yeast (9, 27) using conventional transformation methods]. Therefore, it is important to develop smart library design methods that sample a relatively small number of residues at each CDR position that are most likely to generate antibodies with significant gains in affinity (28–41).
A third common challenge related to antibody affinity maturation is the identification of affinity-enhancing mutations that lead to reductions in antibody specificity (42–44). Highly interactive residues—such as arginine and aromatic residues—can be readily enriched in the CDRs during affinity maturation, which is concerning because they have increased risk for promoting non-specific interactions (43–47). While negative selections are useful for removing some non-specific variants, it is critical to use libraries with the highest possible fraction of specific variants to maximize the likelihood of isolating antibodies with not only increased affinity but also with high specificity. A related problem is that affinity-enhancing CDR mutations can lead to reductions in stability (48–51). Antibody affinity/stability trade-offs appear to be due to structural changes in the CDRs and frameworks that are necessary to increase affinity, and additional compensatory mutations are needed in some cases to maintain thermodynamic stability (48, 49, 51). Therefore, it is important to generate antibody libraries with the highest possible fraction of stable antibodies to minimize the frequency of isolating destabilized antibodies that require additional mutagenesis to restore stability.
To evaluate potential solutions to these challenges, we have sought to identify mutations that increase the affinity of a camelid single-domain antibody specific for the C-terminus of α-synuclein (52) (Figure 1). This variable (VHH) domain—originally referred to as NbSyn2 and herein referred to as N2—was previously isolated from an immune library. We selected this antibody domain for further optimization because its crystal structure is available in complex with antigen at high resolution (Figure 1), it is relatively simple to display on the surface of yeast for in vitro selections relative to more complex multidomain (scFv) and/or multichain (Fab or IgG) antibodies, it has intermediate affinity (KD of 58 ± 9 nM) that can be further increased, and it has relatively high stability (apparent melting temperature of 68 ± 0.3°C). We posit that efficient affinity maturation of antibody variable domains such as N2 can be accomplished in three steps: (i) identification of the most permissive sites in the CDRs that can be mutated without large (negative) impacts on affinity using alanine scanning mutagenesis; (ii) sampling of a small number of mutations at each permissive CDR site that correspond to either the wild-type residue or residues most commonly observed in natural antibodies at each CDR site; and (iii) screening of all possible combinations of single and multiple natural diversity antibody mutations in a single library. Here, we test this methodology by identifying the most permissive CDR sites in N2 and use these findings to generate a single library that is based on natural antibody diversity and includes both single and multiple (up to 14) CDR mutations. We demonstrate how this library design approach can be used along with yeast surface display to identify stable and specific variable domains with increased affinity.
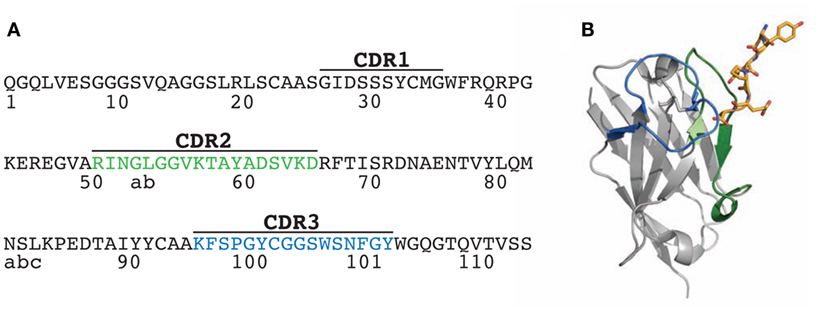
Figure 1. Sequence and structure of the N2 VHH antibody. (A) Amino acid sequence of wild-type N2 VHH antibody (originally referred to as NbSyn2). The framework and complementarity-determining region (CDR) sequences are defined according to Kabat. (B) Structure of N2 in complex with its antigen, a C-terminal α-synuclein peptide (residues 132-GYQDYEPEA-140; PDB 2X6M). Two of the key N2 CDRs involved in antigen binding are highlighted in green (CDR2) and blue (CDR3), while the antigen (α-synuclein peptide) is highlighted in yellow stick form.
Results
Alanine Scanning Mutagenesis Reveals Permissive CDR Sites
Toward our goal of developing systematic and robust affinity maturation methods, we first sought to identify permissive sites in the CDRs of N2 that weakly impact antibody affinity using both computational and experimental methods. Two of the CDRs (CDR2 and CDR3) are involved in mediating antigen binding (Figure 1). Our computational alanine scanning analysis of these CDRs identified two residues in CDR2 (N52 and K56) and two residues in CDR3 (Y100 and W100e) that are sensitive to mutation (Table S1 in Supplementary Material). We tested these observations using experimental alanine scanning mutagenesis at 18 sites in CDR2 and CDR3. Three sites in these CDRs (R50, P98, and C100a) were excluded from this analysis because they were either shown previously to be involved in mediating antigen binding (52) or suspected to be important for antibody structure and stability.
The alanine mutants were expressed in bacteria and purified using metal-affinity chromatography (purification yields of 0.7–2.6 mg/L). SDS-PAGE analysis revealed high purities (Figure S1 in Supplementary Material). The relative binding of each mutant was evaluated using fluorescence polarization at three VHH concentrations (44, 133, and 400 nM; Figure 2; Figure S2 in Supplementary Material). Consistent trends were observed at each VHH concentration. Eleven of the 18 mutants retained >50% of the wild-type binding activity, including three in CDR2 (L52b, G53, and V55) and eight in CDR3 (F96, S97, G99, G100b, G100c, S100d, S100f, and N100g). The other seven mutants that displayed greater reductions in binding included five CDR2 mutants (I51, N52, G52a, G54, and K56) and two CDR3 mutants (Y100 and W100e), which were not subjected to further mutagenesis. Four of the disruptive mutations (N52 and K56 in CDR2 and Y100 and W100e in CDR3) were identified in our computational alanine scanning mutagenesis (Table S1 in Supplementary Material). These and other previous results (39, 53, 54) highlight the value of alanine scanning mutagenesis to identify permissive CDR sites that can be mutated during antibody affinity maturation.
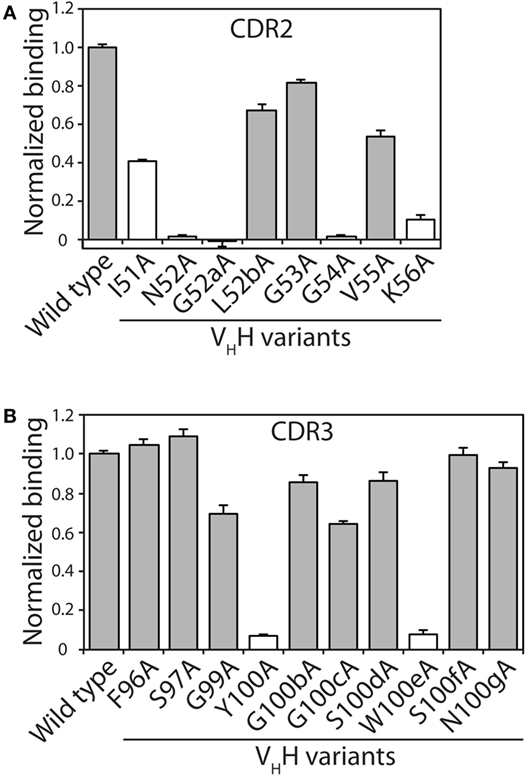
Figure 2. Identification of VHH complementarity-determining region (CDR) residues involved in antigen binding via alanine scanning mutagenesis. The relative antigen binding of the VHH variants (400 nM) with single alanine substitution mutations in (A) CDR2 and (B) CDR3 was evaluated using fluorescence polarization (2 nM TAMRA-labeled α-synuclein peptide). Raw polarization signals were background subtracted (background signals were obtained using samples with only TAMRA α-synuclein peptide), and normalized signals are reported (signal for mutant divided by that for wild type). Error bars represent the SD for three independent experiments. The VHH sequence is defined using Kabat numbering. Alanine mutants that have modest impacts on antigen binding (mutant binding is at least 50% of wild-type binding) are highlighted in gray fill, while those mutants with larger negative impacts on antigen binding are indicated in white fill.
Design of Antibody Libraries Using Natural Diversity Mutagenesis
We next sought to design a single antibody library with mutations in N2 at permissive sites in CDR2 and CDR3. We aimed to accomplish multiple objectives in our library design. First, we limited the library size to ~107 variants to enable 10-fold oversampling of the library using yeast surface display given that our typical yeast transformation efficiencies are ~108 transformants. Second, we aimed to generate a single library with all possible combinations of wild-type residues as well as single and multiple mutations at the 11 permissive sites in CDR2 and CDR3 as well as at three additional sites not tested during alanine mutagenesis (A49, A94, and K95). This limits the number of possible mutations at each CDR site to typically one to two mutations in addition to the wild-type residue. Third, we sought to sample mutations that most closely correspond to those observed in the CDRs of natural antibodies in a site-specific manner. To accomplish this, we used the AbYsis database to identify the most common amino acids in camelid VHH and human VH domains at each site in CDR2 and CDR3 (55). We used an average site-specific amino acid frequency for camelid and human domains at each CDR site given that there are many more sequences for human domains than for camelid domains. Fourth, we aimed to use inexpensive primer synthesis methods to generate the libraries encoded by standard degenerate codons. Therefore, we sought to identify degenerate codons at each CDR site that encoded the wild-type residue and ~1–5 additional residues that maximize the coverage (sum of individual site-specific amino acid frequencies) of the combined camelid and human natural diversity at each site (Figure 3).
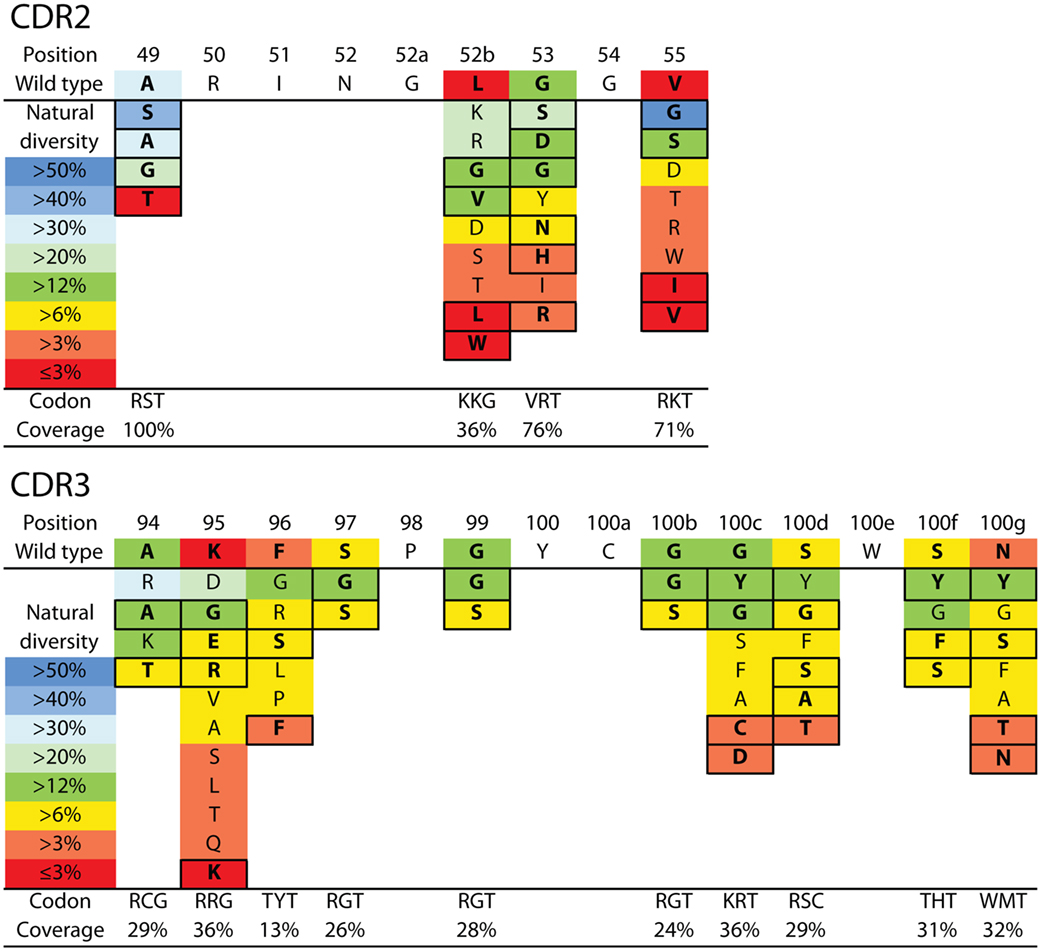
Figure 3. VHH library design for N2 affinity maturation using natural diversity mutagenesis. A single VHH library was designed that involved mutating four sites in CDR2 (top) and 10 sites in CDR3 (bottom). The CDR sites selected for mutagenesis were identified primarily using alanine scanning mutagenesis (11 CDR sites). Each mutated CDR site involved sampling the wild-type residue and one to five of the most common natural diversity mutations. Degenerate codons were selected at each CDR site that maximized the natural diversity coverage and minimized the total number of mutations. It was not possible to sample the wild-type residue and the most common natural diversity mutations at each CDR site due to the limitations of degenerate codons. The resulting library (9.4 × 106 variants) theoretically encodes all possible combinations of single and multiple CDR mutations (up to 14 mutations per VHH). The reported CDR site-specific natural diversity statistics are averaged values for human (VH) and camelid (VHH) variable domains, as reported in the abYsis database (55). Boxed amino acids correspond to the selected natural diversity mutations.
Based on these four key objectives, we designed the library shown in Figure 3 and generated it using the process outlined in Figure S3 in Supplementary Material. The library contains 9.4 × 106 unique variants and includes wild-type residues at each position as well as all possible combinations of single and multiple mutations at 14 sites in CDR2 and CDR3. We sequenced several (22) members of the initial library, and the results are summarized in Figure 4 and Figure S4 in Supplementary Material. All variants were found to be unique and contained mutations according to the proposed library design.
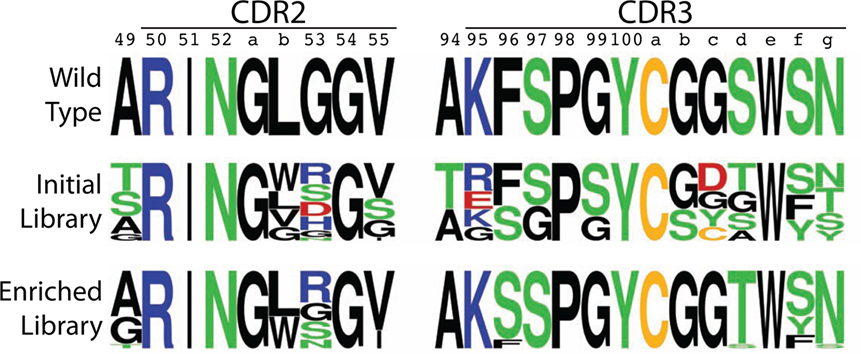
Figure 4. Amino acid logo summary of initial and enriched VHH libraries relative to the wild-type N2 VHH. The logo plots for the mutated portions of CDR2 and CDR3 were generated from sequencing results for 22 (initial library) and 17 (enriched library) VHH variants. The CDR sequences are defined using Kabat numbering, and the logos were generated using a web application (http://weblogo.berkeley.edu).
Sequence Analysis of VHH Libraries after Sorting for Enhanced Antigen Binding
The library of antibody variable domains was displayed on the surface of S. cerevisiae and screened for variants with increased affinity for the α-synuclein peptide. The sorting process involved five rounds of selection via magnetic-activated cell sorting (MACS) with progressively reduced concentrations of α-synuclein peptide (starting at 50 nM peptide and ending at 5 nM) and one additional round of selection via fluorescence-activated cell sorting (FACS) (20 nM peptide). The sorting process was continued until the antigen binding of the library was increased by at least fivefold relative to wild type, as judged by flow cytometry. Selections were performed in a buffer (PBS) that contained both BSA (1 mg/mL) and milk (1% w/v). We have found previously that antibody selections in complex environments (e.g., buffers supplemented with milk) lead to identification of antibodies with improved specificity (56).
The enriched VHH library was sequenced after sorts 5 and 6, and 17 unique variants were identified and further analyzed (based on sequencing 23 clones) with 1–6 mutations in CDR2 and CDR3. Sequence logos in Figure 4 summarize the general enrichment of amino acids in the CDRs, while the amino acid enrichment ratios are given in Figure S5 in Supplementary Material and the CDR sequences are given in Figure S6 in Supplementary Material. Most of the sites in CDR2 and CDR3 (11 out of 14) displayed either intermediate or strong preference for the wild-type residue (Figure 4). However, three sites (53 in CDR2, 96 and 100d in CDR3) either displayed similar preference for mutations as the wild-type residue (Arg, Gly, Ser, and Asn at position 53) or strong preference for a specific mutated residue (Ser at position 96 and Thr at position 100d). It is also notable that the four positions that were varied in CDR2 did not display strong preference for any single amino acid, while almost every residue in CDR3 (9 out of 10) displayed strong preference for a single residue. This result is unexpected based on alanine scanning mutagenesis, as the identified sites in CDR3 appeared to be as permissive (or even more permissive) to mutagenesis than those identified in CDR2.
Identification of Affinity-Matured Variable Domains with High Stability and Specificity
To evaluate the effectiveness of the affinity maturation process, we next expressed and purified the unique VHH variants that were identified in the enriched library. The variable domains expressed at levels (purification yields of 0.1–2.0 mg/L) that were generally similar to wild type (1.0 mg/L), and also displayed purities similar to wild type (Figure S7 in Supplementary Material). We first used fluorescence polarization to evaluate the affinities of the variable domains for the α-synuclein peptide (Figure 5A). The equilibrium dissociation constant for the wild-type N2 variable domain (KD of 57.6 ± 9.0 nM) was approximately threefold lower than the previously reported value (KD of 190 ± 30 nM) that was measured by isothermal calorimetry (52).
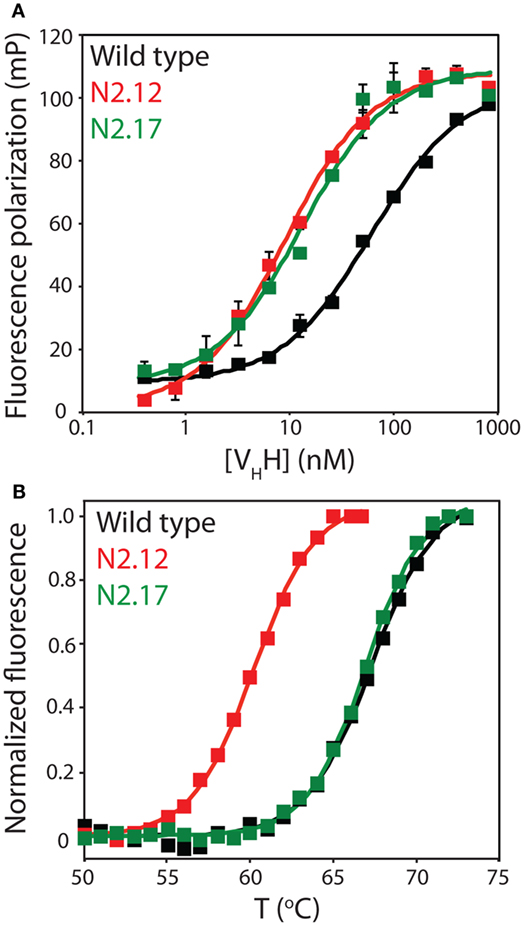
Figure 5. Evaluation of the affinity and stability of select VHH mutants that were enriched after library sorting for improved antigen binding. (A) Fluorescence polarization analysis of VHH binding to labeled antigen (2 nM TAMRA-labeled α-synuclein peptide). The analysis was performed in a PBS buffer supplemented with BSA (0.001%) and Tween 20 (0.001%). Three independent experiments were performed, and representative binding curves are shown for wild type (black), N2.12 (red), and N2.17 (green). Each point shown is the average of two repeats and the error bars are SD. Data were fit with a binding model that accounted for the fact that the VHH antibodies were not in excess of the antigen at some of the VHH concentrations (57). (B) Extrinsic fluorescence analysis of apparent VHH unfolding as a function of temperature. The fluorescence data were obtained using an extrinsic dye (Protein Thermal Shift dye, Life Technologies). Three independent experiments were performed, and representative melting curves are shown for wild type (black), N2.12 (red), and N2.17 (green). The data were background subtracted using background signals obtained without antibody. Next, the fluorescence data were subtracted by the relatively low signal at 50°C, and divided by the maximum fluorescence signal (after the maximum signal was subtracted by the signal at 50°C). Finally, the pre- and post-transition regions of the normalized fluorescence data were flattened using linear fits.
We chose to characterize two VHH domains in more detail (N2.12 and N2.17). Both variable domains displayed improved affinity (KD of 7.6 ± 0.4 nM for N2.12 and 13.2 ± 4.8 nM for N2.17 relative to 57.6 ± 9.0 nM for wild type; Figure 5A). Interestingly, the improved affinity of the N2.12 variant came at the cost of reduced stability (apparent Tm of 59.7 ± 0.3°C relative to 67.8 ± 0.3°C for wild type; Figure 5B). By contrast, the N2.17 variant displayed similar stability as wild type (66.9 ± 0.1°C for N2.17 relative to 67.8 ± 0.3°C for wild type; Figure 5B). This finding demonstrates that our affinity maturation method can be used to identify antibody variable domains such as N2.17 with increased affinity without significant reduction in stability despite the common observation of affinity/stability trade-offs (such as those observed for N2.12) during affinity maturation (51, 58).
We also evaluated the specificity of the N2.12 and N2.17 VHH domains to evaluate if gains in affinity were offset by reductions in specificity (Figure 6; Figure S8 in Supplementary Material). A simple test of non-specific interactions is to evaluate the propensities of antibodies to interact with well plates coated with different types of non-antigen proteins (milk proteins and a panel of six non-antigen proteins in Figure 6 and Figure S8 in Supplemental Material) at relatively high antibody concentrations (~1 μM). Interestingly, the N2.17 variant displays significantly lower non-specific interactions than wild type (p-values of 0.003 for milk proteins and 0.009 for six non-antigen proteins), while the N2.12 variant displays similar non-specific binding as wild type (p-values of 0.129 for milk proteins and 0.342 for non-antigen proteins). These results demonstrate that the affinity-matured VHH domains display similar or improved specificity relative to wild type.
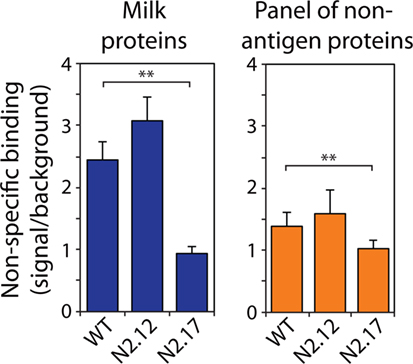
Figure 6. Analysis of non-specific binding for wild-type and affinity-matured VHH domains. Non-specific binding of VHH variants was evaluated using well plates coated with milk proteins (left) and a panel of six non-antigen proteins (right). The non-specific binding analysis was performed at an antibody concentration of 1,000 nM. The reported non-specific binding values are the signals for antibody binding to well plates coated with milk proteins or other non-antigen proteins divided by the background signal without primary antibody (VHH). The reported binding values (right) are the averages for six non-antigen proteins (ovalbumin, BSA, KLH, ribonuclease A, avidin, and lysozyme). The values are averages of three independent experiments, and the error bars are SD. A two-tailed Student’s t-test was used to determine statistical significance [p-values < 0.01 (**)].
We next analyzed the affinity and stability of the other 15 unique VHH variants that were isolated during the sorting process (Figure 7; Figures S9 and S10 in Supplementary Material). All but one of the variable domains (N2.5) displayed a statistically significant increase in affinity relative to wild type (p-values <0.01; Figure 7A). This suggests that our library design and selection strategies enable robust identification of variable domains with improved affinity. Interestingly, variants with the greatest improvements in affinity (at least threefold) contained at least three mutations and up to six mutations. This highlights the inherent limitations of attempting to identify variable domains with large increases in affinity using single mutations.
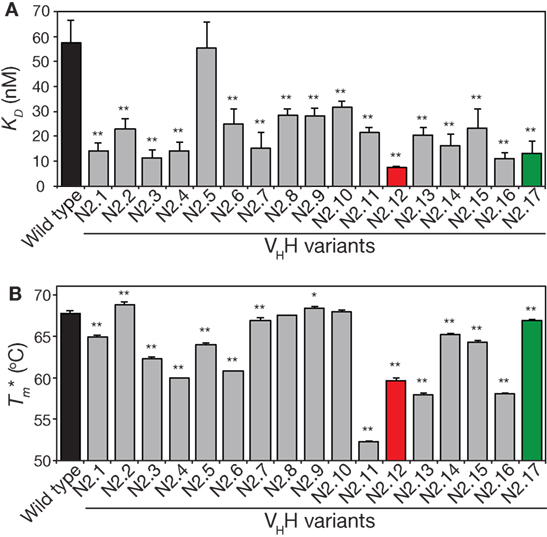
Figure 7. Analysis of affinity and stability for the panel of VHH mutants that were isolated after library sorting for improved antigen binding. (A) Equilibrium dissociation constant (KD) values were measured using fluorescence polarization. (B) Apparent melting temperatures were measured using extrinsic fluorescence measurements as a function of temperature. In (A,B), the measurements were performed as described in Figure 5, the values are averages for three independent experiments, and the error bars are SD. A two-tailed Student’s t-test was used to determine statistical significance [p-values < 0.05 (*) or 0.01 (**)].
The stability analysis of these variable domains also revealed interesting behaviors (Figure 7B). Most notably, the apparent stability of the VHH domains is much more variable than the affinity measurements. About one-third of variable domains (6 of 17) display similar stabilities as wild type (apparent melting temperature within 1°C of wild type). The variable domains with the largest reductions in apparent melting temperature (>7°C; N2.4, N2.11, N2.12, N2.13, and N2.16) had the highest number of mutations (5–6 mutations). A direct comparison of affinity versus stability for the VHH domains reveals a wide range of affinity/stability trade-offs (Figure 8).
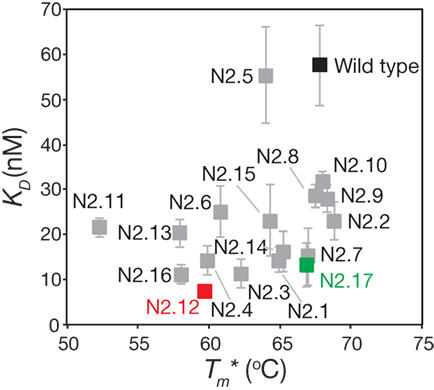
Figure 8. Comparison of the affinities and stabilities of the affinity-matured VHH variants. The equilibrium dissociation constants (KD) and apparent melting temperatures are from Figure 7. The values are averages of three independent experiments, and the error bars are SD (horizontal error bars for stability are smaller than the size of the data points).
Origins of Affinity/Stability and Affinity/Specificity Trade-offs for Affinity-Matured VHH Domains
To better understand the origins of the strong and weak trade-offs between affinity and both stability and specificity for the selected VHH domains, we performed reversion mutational analysis for two of the variable domains (N2.12 and N2.17) to evaluate the impact of the acquired mutations on affinity, stability, and specificity. Six single reversion mutants were created for N2.12, while four single reversion mutations were created for N2.17. The purities of the reversion mutants were similar to wild type (Figure S11 in Supplementary Material).
The affinity and stability measurements are summarized in Figure 9 and Figures S12 and S13 in Supplementary Material. In Figure 9, the affinity is reported as the equilibrium association constant (KA). Reversion mutations that reduced affinity and/or stability—which signifies that the original mutations increased affinity and/or stability—correspond to reduced KA or apparent melting temperature values. For the highest affinity variant identified in our studies (N2.12), one mutation (G49) is highly destabilizing and reversion to the wild-type residue (A49) results in a large increase in stability increases by 7.4°C; p-value of 2 × 10−5; Figure 9A) without a significant change in affinity (p-value of 0.67; Figure 9A). Surprisingly, this reversion mutant is the most desirable affinity-matured VHH domain that we obtained, as the large affinity enhancement (>7-fold) is achieved without compromising stability (p-value of 0.099 for comparison to wild type). This reversion mutational analysis also reveals that the affinity enhancement of N2.12 is largely due to four mutations (W52b, R53, S96, and T100d). The S96 mutation is particularly interesting because it contributes positively both to affinity and stability, as judged by the fact that the reversion mutation (F96) reduces both properties (p-values <0.03). By contrast, the R53 mutation increases affinity (p-value of 0.004) at the cost of stability (p-value of 0.001), and the W52b and T100d mutations increase affinity (p-values <0.005) without significantly impacting stability (p-values >0.1).
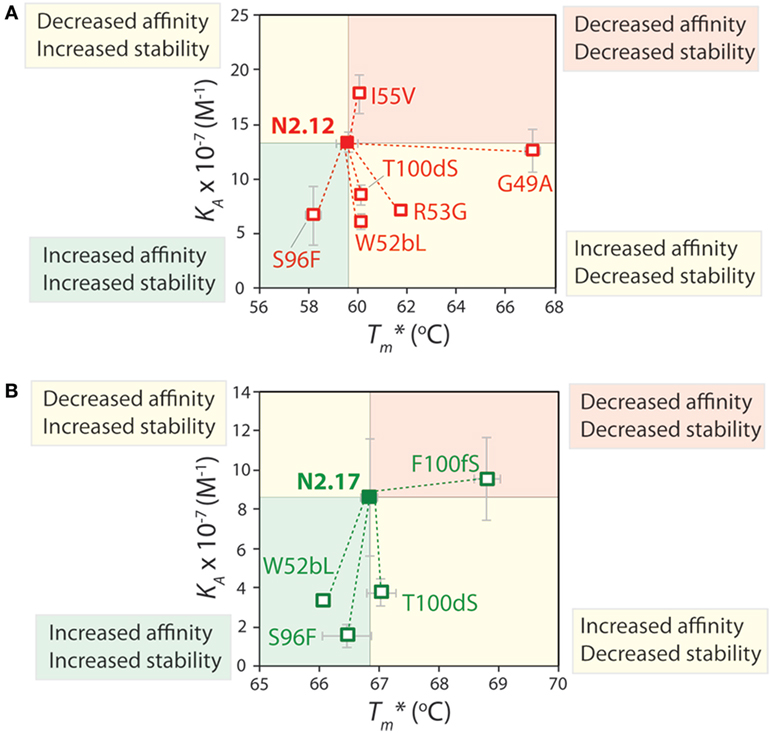
Figure 9. Mutational analysis of the contributions of specific VHH mutations to affinity and stability. Single reversion mutations were generated for two affinity-matured VHH variants [(A) N2.12 and (B) N2.17] to determine the contribution of each acquired mutation to affinity and stability. Values of the equilibrium association constant (KA) were measured using fluorescence polarization, and values of the apparent melting temperature were measured using extrinsic fluorescence measurements as a function of temperature. Reductions in either affinity or stability due to reversion mutations indicate that the original mutations acquired during affinity maturation contribute positively to either property. The values of KA and are averages from three independent experiments and the error bars are SD.
Reversion mutational analysis of the more stable VHH domain (N2.17) revealed key differences relative to the less stable N2.12 variant (Figure 9B). None of the four reversion mutations in N2.17 resulted in changes in apparent melting temperature >2°C. The most destabilizing N2.17 mutation was F100f, and the reversion mutation S100f increased stability to levels modestly higher than the wild-type N2 domain without a significant change in affinity relative to N2.17 (p-value of 0.74). The three key affinity mutations (W52b, S96, and T100d)—which were also observed in the less stable N2.12 domain—had little impact on stability (<1°C). These findings highlight that the affinity/stability trade-offs observed in our enriched library can be addressed either by screening a sufficient number of VHH variants or by performing reversion mutational analysis to identify destabilizing mutations that are not required for affinity.
The specificity of the reversion mutants was analyzed by evaluating their relative propensity to interact with milk proteins (Figure 10). A decrease in the normalized specificity of a reversion mutant indicates that the original mutation has a positive impact on antibody specificity. The N2.12 variable domain—which possesses similar specificity as the wild-type N2 domain—acquired five mutations that decreased specificity (p-values <0.01; Figure 10A). However, N2.12 also acquired a single mutation (W52b) that increased specificity (p-value of 9.4 × 10−6; Figure 10A) and which appears to offset the negative effects of the other five mutations. Interestingly, the improved specificity of N2.17 relative to wild type appears to be due to three mutations that enhance specificity (W52b, S96, and F100f; p-values <1.1 × 10−5; Figure 10B). This analysis highlights that affinity-enhancing mutations can contribute both positively and negatively to antibody specificity, and that significant improvements in specificity can be due to the cumulative effects of multiple mutations.
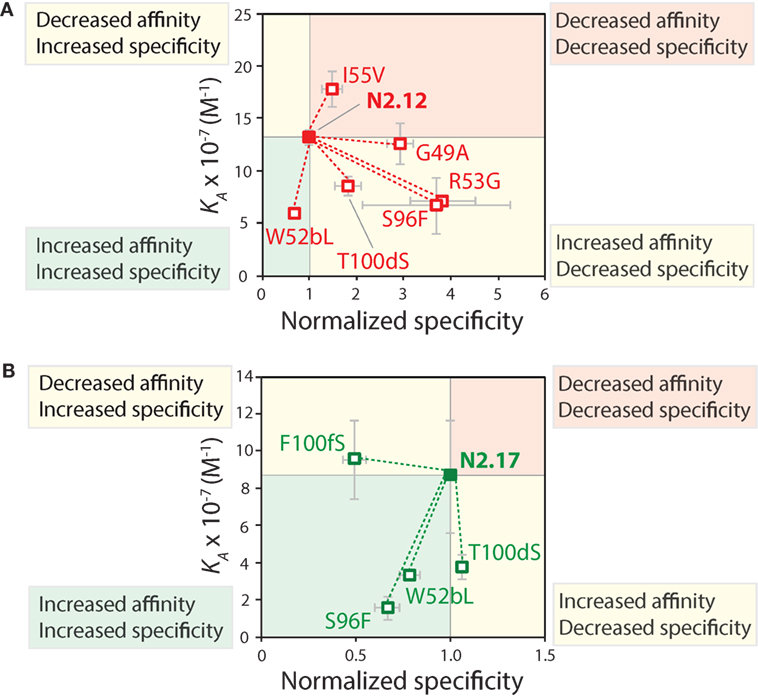
Figure 10. Mutational analysis of the contributions of specific VHH mutations to affinity and specificity. Single reversion mutations were generated for two affinity-matured VHH variants [(A) N2.12 and (B) N2.17] to determine the contribution of each acquired mutation to affinity and specificity. Values of the equilibrium association constant (KA) were measured as described in Figure 9. Normalized specificity was measured as the binding of the parent antibody to milk-blocked wells divided by that for the reversion mutant (parent/reversion mutant). Reductions in affinity or specificity for the reversion mutants indicates that the original mutations acquired during affinity maturation contribute positively to either property. The values of KA are averages from three independent experiments, and values for normalized specificity are averages of six replicates obtained from three independent experiments. The error bars are SD.
Computational Analysis of Natural Diversity Mutations That Enhance Affinity
To gain further understanding about how the selected mutations increased VHH affinity, we performed computational modeling of two of the mutant variable domains (N2.12 and N2.17). This was accomplished by introducing the corresponding mutations into the crystal structure of the wild-type N2 domain in complex with the α-synuclein peptide (PDB: 2X6M) and relaxing the structures via CHARMM force field energy minimization (59). The highest affinity domain we identified after library sorting (N2.12) contains six mutations that are located near but generally not in direct contact with the antigen (Figure 11A). The one exception is I55 in CDR2 (V55 in wild type), which forms a direct contact with E137 in the α-synuclein peptide via an interaction between the backbone amide in the antibody (I55) and carboxylate oxygen in the antigen (E137). However, this does not appear to explain the increased affinity of N2.12 because the mutation increases the interaction distance (2.6 Å) relative to wild type (1.7 Å). Instead, the increase in affinity for N2.12 appears to be due to indirect effects that involve enhancement of existing interactions as well as introduction of new interactions that involve wild-type CDR residues (Figure 11B). This includes an enhanced salt bridge between K56 (side chain nitrogen) in CDR2 and E139 (carboxylate oxygen) in the antigen. Moreover, a new electrostatic interaction is introduced between T57 (backbone carbonyl oxygen) in CDR2 and A140 (backbone amide nitrogen) in the antigen. The latter interaction appears to be mediated by a water bridge in both the crystal structure and energy minimized (relaxed) structure of the wild-type antibody-antigen complex (data not shown).
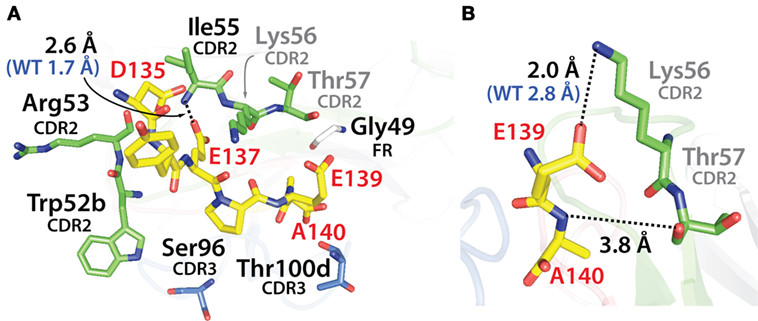
Figure 11. Analysis of the contributions of the acquired mutations in the N2.12 VHH antibody to enhanced affinity using computational models of the antibody–antigen complex. (A) Model of the N2.12 VHH in complex with the α-synuclein peptide. The six acquired CDR mutations are highlighted in black text, the wild-type residues are shown in gray, the nitrogen atoms are shown in blue, and the oxygen atoms are shown in red. Only one of the CDR mutations (Ile55) makes direct contact with the antigen, and the distance of this interaction is increased relative to wild type. (B) New or enhanced interactions between the N2.12 VHH and the α-synuclein peptide. Direct electrostatic interactions are shown with black dotted lines, and the distances are indicated in black for N2.12 relative to the original distances for wild type in blue (if there was a wild-type interaction). VHH residues are numbered according to Kabat.
Similar findings were obtained by examining the modeled structure of the more stable N2.17 variant in complex with the α-synuclein peptide (Figure 12A). None of the four mutations make direct contact with the antigen. Instead, the gains in VHH affinity appear to be due to indirect effects involving wild-type CDR residues (Figure 12B), as observed for N2.12 (Figure 11B). We observe enhanced hydrophobic packing between G100b, G100c, and T100d in CDR3 with A140 in the antigen (Figure 12B). In addition, there are new direct electrostatic interactions between T57 (CDR2) and A140 (antigen) as well as G100b (CDR3) and A140 (antigen). Finally, two electrostatic interactions are enhanced, namely R50 (CDR2) with A140 (antigen) and G100c (CDR3) with A140 (antigen). This enhancement is due to A140 in the α-synuclein peptide moving deeper into the binding pocket of the VHH domain, which is mediated by structural rearrangement of the CDRs. These results are consistent with the general understanding that affinity maturation of antibodies involves subtle changes to the antigen-binding site and beneficial mutations often mediate their effects indirectly via structural changes that optimize interactions involving wild-type CDR residues (60–63).
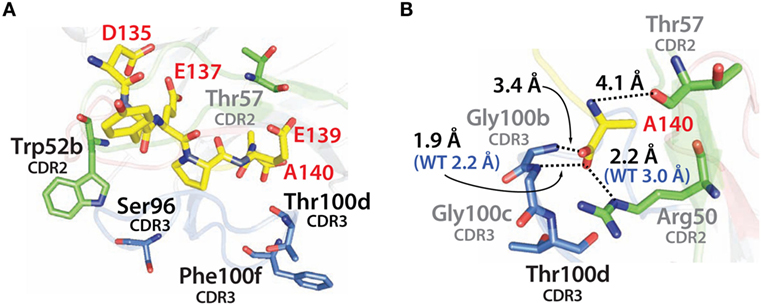
Figure 12. Analysis of the contributions of the acquired mutations in the N2.17 VHH antibody to enhanced affinity using computational models of the antibody–antigen complex. (A) Model of the N2.17 VHH in complex with the α-synuclein peptide. The four acquired CDR mutations are highlighted in black text. No CDR mutations make direct contact with the antigen. (B) New or enhanced interactions between the N2.17 VHH and the α-synuclein peptide. The labeling is the same as in Figure 11.
Discussion
This work identifies several key factors that impact the efficiency and robustness of antibody affinity maturation. First, we find that multiple mutations (>4) are necessary to achieve large (>5-fold) gains in affinity for the N2 VHH antibody. While there are obvious exceptions to our findings (1, 3, 5), they are generally consistent with previous findings that many single affinity-enhancing mutations cause relatively modest increases in affinity (24, 64–66). It is possible to identify and combine several single mutations that enhance affinity, but the collective effects of multiple mutations on antibody affinity are complex and often not additive (58, 62, 67, 68). Moreover, generating all possible combinations of single antibody mutations is a time-consuming process that involves multiple rounds of expression and affinity evaluation. It is also notable that the need for several mutations to achieve large increases in antibody affinity is likely at least part of the reason that it is particularly challenging to use computational methods for antibody affinity maturation (3, 24, 58, 67, 69). Accurate prediction of subtle structural changes caused by combinations of CDR mutations is notoriously difficult. Our natural diversity mutagenesis approach is attractive because it enables sampling of all possible combinations of single and multiple CDR mutations (~1–5 mutations per CDR site across 14 sites in this work) for rapid identification of antibody variants with large increases in affinity using a single antibody library.
There are multiple considerations related to our natural diversity mutagenesis approach that deserve further consideration. First, the primary problem during affinity maturation is obtaining mutations that increase affinity but reduce specificity. Our use of natural antibody diversity to guide library design—which has been reported previously in related ways by others (28–41, 70)—avoids overrepresentation of highly interactive residues that are likely to promote non-specific interactions. Many previous studies (including those from our own lab) have used NNN or NNK degenerate codons in antibody CDRs to identify affinity-enhancing mutations (58, 71–73). One of the limitations of this approach is that the frequency of sampling each amino acid is based on its corresponding codon frequency. In our experience, this is especially problematic for highly interactive residues such as arginine that have a large number of codons (up to six depending on the specific degenerate codon). By contrast, our library design infrequently sampled highly interactive residues, such as arginine (2 out of 14 CDR sites), tryptophan (1 out of 14 CDR sites), and phenylalanine (2 out of 14 CDR sites). In fact, one of the key affinity mutations in both N2.12 and N2.17 was F96S, which removed an aromatic residue and increased the hydrophilicity of CDR3.
It is also notable that our mutational approach was useful for identifying beneficial mutations in the highly variable CDR3 in addition to the less variable CDR2. Two of the key affinity mutations in both N2.12 and N2.17—F96S and S100dT—were in CDR3. The most common residues at many sites in CDR3 occur at relatively low frequency (13–21% for positions 95–100 g). Therefore, it was not obvious that sampling such a small number of natural diversity mutations (1–3 mutations per site for nine sites in CDR3) in such a highly diverse CDR would be sufficient to identify affinity-enhancing mutations. For example, the natural occurrence of the wild-type residue (Phe) at position 96 in CDR3 is 4% (combined human and camelid diversity), and we sampled only one mutation (Ser) at this site that is also relatively uncommon (9%) despite being more common than most other residues at this CDR3 site. Likewise, we sampled three mutations at position 100d in CDR3 (Gly, Ala, and Thr) that were all relatively uncommon (5–11%). Nevertheless, we identified a beneficial mutation (Thr) that occurs relatively infrequently (5%) at this site in CDR3. These results suggest that natural diversity mutations in CDR3—especially for affinity maturation—may be particularly useful for libraries aimed at isolating combinations of mutations that result in large increases in affinity without over enrichment in highly interactive residues that are likely to also mediate non-specific interactions.
Despite the strengths of our natural diversity mutagenesis approach, one obvious weakness is related to the use of inexpensive primer synthesis methods that rely on standard degenerate codons to generate libraries. This results in the limitation that some combinations of wild-type CDR residues and the most common natural diversity mutations are (i) not possible, (ii) require too many additional mutations to justify including them, and/or (iii) require inclusion of undesirable codons (e.g., those encoding cysteine or stop codons). While we allowed a cysteine mutation at one position (100c) to maximize natural diversity coverage, it is undesirable to include too many cysteine mutations due to complications associated with unpaired cysteines.
An example of the limitations of using degenerate codons to generate antibody libraries is related to position 52b in CDR2. The wild-type residue at position 52b is Leu, and the two most common residues at this position are Lys (29% based on combined camelid and human natural diversity) and Arg (22%). However, this requires sampling a minimum of six codons, which corresponds to a minimum of five residues and overrepresentation of arginine (two codons) to achieve natural diversity coverage of 56% (an average of ~9% per codon). Therefore, we sampled Gly, Val, and Trp in addition to the wild-type residue (Leu) at position 52b using four codons to achieve natural diversity coverage of 36% and similar average diversity per codon (9%). Likewise, the wild-type residue at position 96 in CDR3 is Phe. In order to sample Phe and the most common residue (Gly), this requires sampling a minimum of four codons that include Val (5%) and Cys (1.5%). Sampling these four residues would result in natural diversity coverage of 23% (an average of ~6% per codon). Instead, we sampled Ser in addition to Phe using two codons to achieve natural diversity coverage of 13% (an average of ~6% per codon). This approach allowed us to sample a similar amount of natural diversity per codon and eliminated the use of an undesirable codon (Cys). These examples highlight the limitations of using standard degenerate codons to achieve the highest possible coverage of natural diversity mutations. This limitation could be readily solved using more expensive trinucleotide synthesis methods.
Our results also demonstrate that affinity/stability trade-offs are common during antibody affinity maturation. We and others have previously found that CDR mutations that increase antibody affinity can be destabilizing (48, 49, 51). Indeed, several examples of natural antibodies have been reported that demonstrate how affinity-enhancing mutations can be destabilizing (48, 49). This destabilization is likely due to strain on the antibody framework that results from modifying the structure and chemistry of the antigen-binding site for increased affinity. Encouragingly, about one-third of our affinity-matured antibodies displayed little reduction in stability (<1°C) and we identified one of the highest affinity variants with similar stability as wild type after additional mutational analysis (N2.12 with A49; of 67.1 ± 0.3°C relative to 67.8 ± 0.3°C for wild type). Nevertheless, the fact that the highest affinity variants identified after library sorting were some of the most destabilized ones (e.g., N2.12 and N2.16) highlights the challenge of affinity/stability trade-offs during affinity maturation. One promising approach is to combine natural diversity mutations in the CDRs with those that naturally occur in the frameworks (74) to co-select for both affinity and stability mutations. We are currently in the process of evaluating this strategy to further improve the affinity maturation process for a wide range of single- and multidomain antibodies to isolate variants that possess high stability in addition to high affinity.
Another notable aspect of our findings relates to the impact of affinity-enhancing mutations on antibody specificity. Specificity is arguably the most difficult antibody property to maintain or enhance during affinity maturation (42–44). This is likely due to the natural tendency to accumulate highly interactive (solvent exposed) amino acids in antibody CDRs during affinity maturation that improve antigen binding but also promote non-specific interactions and reduced specificity. Indeed, we observed trade-offs between affinity and specificity for the N2.12 variant, as three of the four key affinity-enhancing mutations (R53, S96, and T100d) reduced specificity (Figure 10A). Interestingly, the N2.17 variant displayed reduced affinity/specificity trade-offs, as two (W52b and S96) of the three affinity-enhancing mutations also increased specificity (Figure 10B). The latter results are particularly notable because these same mutations (W52b and S96) also increased the stability of N2.17. It is also notable that the impacts of mutations on affinity and specificity were context dependent, as some mutations (e.g., S96) that increased affinity displayed opposite impacts on specificity (reduced specificity for N2.12 and increased specificity for N2.17). Despite these complexities, it will be important in the future to better define how CDR sequence and structure impacts antibody specificity because antibody specificity appears to be a key factor in differentiating approved antibody therapeutics from those in clinical trials (75).
Conclusion
Our systematic approach for using natural antibody diversity to design libraries with combinations of single and multiple mutations with limited diversity at each CDR site is effective for increasing the affinity of a camelid VHH domain while maintaining or enhancing stability and specificity. These encouraging results will need to be evaluated for other types of single- and multidomain antibodies to evaluate their generality. It will also be important to develop computational methods to improve library design by optimizing natural diversity coverage while minimizing the number of mutations. This is relatively straightforward to perform at any given CDR site but it is more challenging to globally optimize with increasing numbers of CDR sites. Nevertheless, efforts in optimizing antibody library design are key to avoid oversampling abnormal CDR sequences that are unlikely to lead to high antibody stability and specificity in addition to high affinity. We expect that methods such as the ones we have demonstrated in this work will be useful for rapidly and systematically optimizing antibodies for a wide range of diagnostic and therapeutic applications.
Experimental Methods
Cloning and Library Construction
The wild-type N2 gene was created using PCR-based gene synthesis (76). The amino acid sequence of the N2 VHH domain (Figure 1) was obtained from the PDB (2X6M). A hexahistidine tag was added to the C-terminus of the VHH domain for purification. The gene was flanked with N-terminal HindIII and C-terminal XhoI restriction sites. The digested PCR product was then ligated into a bacterial expression vector (pET-17b, Novagen) that contained an N-terminal pelB sequence for periplasmic secretion. Single point mutations of N2 were generated via site-directed mutagenesis using PfuUltra II (600850, Agilent Technologies).
The N2 natural diversity library was created using overlap extension PCR to introduce mutations in portions of CDR2 and CDR3 (Figure S3 in Supplementary Material). Mutagenesis was performed using degenerate codons at 14 sites in CDR2 and CDR3 (Figure 3). The first step in library generation was to perform three PCRs. These included amplification of DNA fragments encoding the N-terminus of VHH domain to framework 2, CDR2 to framework 3, and CDR3 to the C-terminus of VHH domain. The DNA fragments overlapped each other by ~20 bases, which enabled the three DNA fragments to be combined in a final amplification step using terminal primers. The terminal primers contained flanking NheI and SalI restriction sites as well as 45 bases of homology on each end with the yeast display plasmid (pCTCON2).
The N2 natural diversity library genes were ligated into the yeast display plasmid and transformed into S. cerevisiae (EBY100) via homologous recombination. This process was performed as described previously (9) with minor modifications to increase transformation efficiency. These modifications include using more yeast cells (500 mL of EBY100 was grown to OD600 of 1.2) for a single library transformation, more DNA (nine preparations of 4 µg PCR product and 1 µg digested vector), and electroporation at higher voltage (2,500 V). After the yeast cells were allowed to recover, the yeast library was grown in SDCAA (500 mL of 20 g/L dextrose, 6.7 g/L yeast nitrogen base, 5 g/L casamino acids, 14.7 g/L sodium citrate, and 4.3 g/L citric acid) for 48 h, and aliquotted for storage at −80°C. The library transformation resulted in 2 × 108 transformants. To assess the quality of the library, a small amount of the yeast library culture (1 mL) was miniprepped (Zymoprep II yeast miniprep kit, Zymo Research) and transformed into electroporation-competent bacterial cells (XL1-Blue, 200228, Agilent Technologies). Several (22) plasmids from the initial library were isolated and sequenced, and all were found to be unique.
Yeast Surface Display and Library Screening
The yeast cultures were first grown at 30°C with agitation in SDCAA to an OD value of 1–2. To induce the expression of Aga2-VHH fusion proteins, the medium was switched to SGCAA (20 g/L galactose, 6.7 g/L yeast nitrogen base, 5 g/L casamino acids, 8.56 g/L NaH2PO4·H2O, and 6.76 g/L Na2HPO4·2H2O) and grown for 16 h at 30°C with agitation. The yeast medium was supplemented with ampicillin (100 µg/mL; BP1760-25, Thermo Fisher Scientific), kanamycin (100 µg/mL; BP906-5, Thermo Fisher Scientific), and penicillin-streptomycin (diluted to 1×; 15140122, Thermo Fisher Scientific).
The natural diversity library was sorted via five rounds of MACS and one round of FACS. For each sort, yeast were washed twice with PBS containing BSA (1 mg/mL; PBS-B) and resuspended in a solution containing the biotinylated α-synuclein peptide (biotin-GYQDYEPEA) and PBS-B supplemented with 1% milk (non-fat dry milk, PBS-BM). For the FACS sort, 1,000× diluted anti-c-myc chicken IgY antibody (A-21281, Life Technologies) was added to this mixture to detect VHH display. The yeast and α-synuclein peptide solution was mixed end-over-end at room temperature for 2–3 h. Next, the cells were washed once with PBS-B and sorted for antigen binding.
For MACS sorts, yeast cells were resuspended in PBS-B (5 mL) and mixed with Streptavidin MicroBeads (100 µL; 130-048-102, Miltenyi Biotec). After incubation on ice (10 min), the yeast cells were pelleted and resuspended in PBS-B and passed through a MACS separation column (130-042-401, Miltenyi Biotec). The column was connected to a MidiMACS separator magnet (130-042-302, Miltenyi Biotec) that was attached to a MACS MultiStand (130-042-303, Miltenyi Biotec). Next, the bound yeast cells were eluted by removing the column from the magnetic stand and flowing SDCAA (7 mL) through the column. The collected cells were then grown overnight in SDCAA (30°C) with agitation and subjected to additional rounds of sorting. For the FACS sort, yeast cells were resuspended in PBS-B (200 µL) with 100-fold diluted secondary reagents (Alexa Fluor 488-conjugated goat anti-chicken IgG, A-11039 and Alexa Fluor 647-conjugated streptavidin, S-32357; Life Technologies), and allowed to incubate on ice (5 min). The cells were washed once, analyzed, and sorted via flow cytometry (FACSAria, BD Biosciences). The enriched yeast cultures after sorts 5 and 6 were miniprepped and subcloned into a bacterial expression vector (pET-17b). Several (~10) plasmids from each sort were isolated and sequenced.
Bacterial Expression and Purification
VHH domains were expressed in bacteria [BL21(DE3)pLysS, 200132, Agilent Technologies] using auto-induction media (200 mL) supplemented with ampicillin (100 µg/mL) and chloramphenicol (35 µg/mL) (77). After 48 h of growth at 30°C, the cultures were pelleted and the supernatants were incubated overnight (4°C, 80 rpm) with 3 mL of Ni-NTA beads (30230, Qiagen). The beads were then washed with PBS (150 mL), eluted at pH 3 (PBS), and neutralized to pH 7.4. The protein samples were centrifuged at 21,000 × g (5 min) and filtered (0.22 µm filter, SLGV013SL, Millipore). Next, the VHH domains were refolded via buffer exchange (Zeba spin desalting columns, 89893, Thermo Fisher Scientific) into 6 M GuHCl (pH 7.4). The antibody domains were allowed to equilibrate overnight (4°C) before being buffer exchanged into PBS (pH 7.4). Finally, the VHH domains were concentrated (3 kDa spin filters; UFC800324, EMD Millipore) and filtered again (0.22 µm filters). The concentrations of the VHH domains were measured via UV absorbance measurements at 280 nm. The extinction coefficients of the VHH domains were 27,180–32,680 M−1cm−1, which were calculated based on their amino acid sequences. The purity of the VHH domains was evaluated using SDS-PAGE analysis (WG1203BOX, Life Technologies), and the gels were stained using Coomassie dye (24615, Thermo Fisher Scientific).
Antibody Affinity Analysis
The affinities of the N2 VHH and variants thereof were measured using fluorescence polarization. The VHH domains were prepared at a range of concentrations (0.8 nM–1.6 µM) and mixed (75 µL) with the α-synuclein peptide labeled with a tetramethylrhodamine (TAMRA) fluorophore (4 nM, 75 µL; Genemed Synthesis Inc.). The antibody–antigen mixtures were prepared in 96 well flat bottom black polystyrene plates (7605, ThermoFisher Scientific). The binding buffer was PBS supplemented with BSA [0.001% (w/v)] and Tween 20 [0.001% (v/v)]. Background wells were prepared that contained the same concentration of TAMRA-labeled α-synuclein peptide without antibody. The antibody–antigen mixtures were allowed to equilibrate at room temperature for 3 h. Fluorescence polarization was then measured (Infinite M1000 PRO, Tecan) at an excitation wavelength of 530 nm (5 nm bandwidth) and an emission wavelength of 582 nm (10 nm bandwidth).
The fluorescence polarization raw signals were background subtracted and two replicates were averaged for each antibody concentration. The average data were then fit to determine the KD value using a four-parameter model that accounts for the fact that the antibody is not in excess of antigen at some of the evaluated antibody concentrations:
where FP is the measured fluorescence polarization value, FPmin is the minimum fluorescence polarization value, FPmax is the maximum fluorescence polarization value, [Ab] is the total VHH concentration, [Ag] is the total antigen concentration, and KD is the equilibrium dissociation constant. The equation was fit using the Microsoft Excel solver tool to minimize differences—namely the sum of squared differences—between the data and the model. At least three independent experiments were performed for each VHH antibody.
Antibody Stability Analysis
The apparent stabilities of the VHH domains were determined using measurements of extrinsic fluorescence (Protein Thermal Shift dye, 4461146, Life Technologies) as a function of temperature. Protein Thermal Shift buffer (5 µL), VHH domains (12.5 µL of 0.08 µg/µL VHH), and Protein Thermal Shift dye (2.5 µL of 8× solution) were mixed in opaque 96-well PCR plates and sealed with foil (04729692001, Roche). The background samples were prepared with water (12.5 µL) instead of VHH domains. Thermal melts were performed using a LightCycler 480 real-time PCR instrument (Roche). The fluorescence (Ex: 558 nm, Em: 610 nm) was measured as the plate was heated from 37 to 95°C. Many (>60) acquisitions were collected per 1°C, and the heating rate was ~0.6°C/min.
The apparent melting temperatures of the VHH domains were determined by analyzing the first derivative of the fluorescence with respect to temperature. This involved fitting a second-order polynomial to the major peak and solving for the temperature at which the maximum occurred (or the minimum if the negative derivative is used). The reported melt curves were background subtracted using background signals obtained without antibody. Next, the fluorescence data were subtracted by the relatively low signal at 50°C and divided by the maximum fluorescence signal (after the maximum signal was subtracted by the signal at 50°C). Finally, the pre- and post-transition regions of the normalized fluorescence data were flattened using linear fits (58).
Antibody Specificity Analysis
The specificities of the VHH domains were evaluated using two methods. The first method evaluated the propensity of the purified antibodies to bind to well plates coated with milk proteins. Transparent 384 well plates (MaxiSorp, 464718, ThermoFisher Scientific) were coated with milk [100 µL of 10% (w/v) milk in PBS with 0.1% (v/v) Tween 20; PBST] for 8 h and then washed with PBS. The VHH domains were diluted to 1,000 nM in PBST, added to the well plates and allowed to incubate overnight at room temperature. The well plates were then washed with PBS and secondary reagents were added to detect bound antibodies. The second method evaluated the propensity of the purified antibodies to bind to six immobilized non-antigens [ovalbumin (A5503, Sigma), BSA (BP9706, Fisher Bioreagents), KLH (H8283, Sigma), ribonuclease A (R6513, Sigma), avidin (A9275, Sigma), and lysozyme (L6876, Sigma)]. Non-antigen proteins were diluted in PBS (75 µL, 0.2 mg/mL) and immobilized in separate wells at 37°C for 1 h in 384 well plates. The wells were subsequently washed with PBST. Variable domains (1,000 nM, 25 µL) in PBS with 1 g/L BSA and 0.1% (v/v) Tween 20 were added to the well plates and allowed to incubate at room temperature for 2 h.
Detection of bound VHH was performed similarly for both specificity tests. Secondary antibody (25 µL of 1,000× diluted anti-6X His tag antibody; ab18184, Abcam) in PBST was added, allowed to incubate for 1 h, and then washed with PBS. Next, the well plates were incubated with diluted horseradish peroxidase-conjugated goat anti-mouse IgG (25 µL of 1,000× dilution; 32430, Thermo Fisher Scientific) in PBST for 1 h and then were washed with PBS. The bound antibody was detected by adding substrate (25 µL of 1-Step Ultra TMB-ELISA, 34028, Thermo Fisher Scientific), quenching after 20–40 min (25 µL of 2 M H2SO4) and measuring the absorbance values at 450 nm (Tecan Safire2 plate reader). Normalized binding signals were calculated as signal divided by background, and the background values were absorbance measurements without primary (VHH) antibody.
Computational Modeling
The VHH-antigen crystal structure (PDB: 2X6M) was energy minimized using the CHARMM force field and the adopted basis Newton–Raphson routine (78). We applied the Newton–Raphson algorithm to a subspace of the coordinate vectors that were sampled by the displacement coordinates (during each iteration) with the objective of minimizing the energy of the complex. This enabled the rate of change of the gradient vectors to be computed and coupled with a subsequent eigenvector analysis to avoid saddle points (metastable energy states). At every Newton–Raphson iteration, the residual gradient vector was calculated and a steepest descent step was added to the Newton–Raphson step. This was done to incorporate a new direction into the basis set to avoid metastable states and find the shortest trajectory toward the atomic coordinates corresponding to the minimum potential energy of the complex.
Computational alanine scanning mutagenesis was performed in a similar manner as described previously (79). Python scripts were written for a new OptMAVEn module to compute the difference between binding energies of the N2 single alanine mutants (which were energy-minimized) and wild-type N2 (2X6M). Binding energy calculations were performed using the conformation-dependent binding energy function as used in the Robetta full-chain protein structure prediction server (80, 81).
Structural models of two affinity-matured VHH variants (N2.12 and N2.17) in complex with antigen were also generated. These structures were simulated alongside the energy-minimized wild-type complex. We created the N2.12 and N2.17 variants using the Mutator program of IPRO suite of programs (82). This approach uses the residue positions and mutations as input, and it performs backbone perturbation, rotamer repacking and energy minimization. A mixed-integer linear programming optimization step was performed to systematically identify the optimal rotamer combination of the new residues at the mutation sites and residues within 4.5 Å (83). This was done to prevent energetically unfavorable steric clashes upon mutation. We performed ensemble structure refinements to establish favorable Lennard-Jones interactions in addition to eliminate severe steric repulsions. The N2.12 and N2.17 variants were visualized in complex with antigen using PyMOL (version 1.8, Schrödinger). Shell scripts were written to identify direct and indirect polar contacts between the antigen (α-synuclein residues DYEPEA) and VHH variants. Only contacts within 5 Å were analyzed.
Author Contributions
KT, PT, RC, TL, and CM designed the research; KT and SS performed experiments; RC and TL performed computational analysis; SL performed bioinformatics analysis; and KT, RC, CM, and PT wrote the paper.
Conflict of Interest Statement
PT has received consulting fees and/or honorariums for presentations of this and/or related research findings at MedImmune, Eli Lilly, Bristol-Myers Squibb, Janssen, Merck, Genentech, Amgen, Pfizer, Adimab, Abbvie, Abbott, DuPont, Schrödinger, and Novo Nordisk. All other authors declare that the research was conducted in the absence of any commercial or financial relationships that could be construed as a potential conflict of interest.
Acknowledgments
We thank Dane Wittrup for providing the pCTCON2 yeast display vector, EBY100 yeast strain and for their helpful discussions; and Eric Shusta, David Colby, Jennifer Cochran, Eric Boder, and Ben Hackel for their helpful advise in performing yeast surface display. We thank Catherine Royer for the use of the Infinite M1000 PRO plate reader. We also thank members of the Tessier lab for their helpful suggestions. This work was supported by the National Institutes of Health (R01GM104130 to PT), National Science Foundation (CBET 1159943 and 1605266 to PT, Graduate Research Fellowship to KT), and Rensselaer Polytechnic Institute (the Richard Baruch M.D. Chair to PT).
Supplementary Material
The Supplementary Material for this article can be found online at http://journal.frontiersin.org/article/10.3389/fimmu.2017.00986/full#supplementary-material.
References
1. Tiller KE, Tessier PM. Advances in antibody design. Annu Rev Biomed Eng (2015) 17:191–216. doi:10.1146/annurev-bioeng-071114-040733
2. Zolot RS, Basu S, Million RP. Antibody-drug conjugates. Nat Rev Drug Discov (2013) 12:259–60. doi:10.1038/nrd3980
3. Kuroda D, Shirai H, Jacobson MP, Nakamura H. Computer-aided antibody design. Protein Eng Des Sel (2012) 25:507–21. doi:10.1093/protein/gzs024
4. Desjarlais JR, Lazar GA. Modulation of antibody effector function. Exp Cell Res (2011) 317:1278–85. doi:10.1016/j.yexcr.2011.03.018
5. Maynard J, Georgiou G. Antibody engineering. Annu Rev Biomed Eng (2000) 2:339–76. doi:10.1146/annurev.bioeng.2.1.339
6. Perchiacca JM, Tessier PM. Engineering aggregation-resistant antibodies. Annu Rev Chem Biomol Eng (2012) 3:263–86. doi:10.1146/annurev-chembioeng-062011-081052
7. McCafferty J, Griffiths AD, Winter G, Chiswell DJ. Phage antibodies: filamentous phage displaying antibody variable domains. Nature (1990) 348:552–4. doi:10.1038/348552a0
8. Sidhu SS. Phage display in pharmaceutical biotechnology. Curr Opin Biotechnol (2000) 11:610–6. doi:10.1016/S0958-1669(00)00152-X
9. Chao G, Lau WL, Hackel BJ, Sazinsky SL, Lippow SM, Wittrup KD. Isolating and engineering human antibodies using yeast surface display. Nat Protoc (2006) 1:755–68. doi:10.1038/nprot.2006.94
10. Boder ET, Wittrup KD. Yeast surface display for screening combinatorial polypeptide libraries. Nat Biotechnol (1997) 15:553–7. doi:10.1038/nbt0697-553
11. Irving RA, Coia G, Roberts A, Nuttall SD, Hudson PJ. Ribosome display and affinity maturation: from antibodies to single V-domains and steps towards cancer therapeutics. J Immunol Methods (2001) 248:31–45. doi:10.1016/S0022-1759(00)00341-0
12. Hanes J, Jermutus L, Weber-Bornhauser S, Bosshard HR, Pluckthun A. Ribosome display efficiently selects and evolves high-affinity antibodies in vitro from immune libraries. Proc Natl Acad Sci U S A (1998) 95:14130–5. doi:10.1073/pnas.95.24.14130
13. Hanes J, Schaffitzel C, Knappik A, Pluckthun A. Picomolar affinity antibodies from a fully synthetic naive library selected and evolved by ribosome display. Nat Biotechnol (2000) 18:1287–92. doi:10.1038/82407
14. Ackerman M, Levary D, Tobon G, Hackel B, Orcutt KD, Wittrup KD. Highly avid magnetic bead capture: an efficient selection method for de novo protein engineering utilizing yeast surface display. Biotechnol Prog (2009) 25:774–83. doi:10.1002/btpr.174
15. Xu Y, Roach W, Sun T, Jain T, Prinz B, Yu TY, et al. Addressing polyspecificity of antibodies selected from an in vitro yeast presentation system: a FACS-based, high-throughput selection and analytical tool. Protein Eng Des Sel (2013) 26:663–70. doi:10.1093/protein/gzt047
16. Mann JK, Park S. Epitope-specific binder design by yeast surface display. Methods Mol Biol (2015) 1319:143–54. doi:10.1007/978-1-4939-2748-7_7
17. Sheehan J, Marasco WA. Phage and yeast display. Microbiol Spectr (2015) 3:AID–0028–2014. doi:10.1128/microbiolspec.AID-0028-2014
18. Zhao A, Tohidkia MR, Siegel DL, Coukos G, Omidi Y. Phage antibody display libraries: a powerful antibody discovery platform for immunotherapy. Crit Rev Biotechnol (2016) 36:276–89. doi:10.3109/07388551.2014.958978
19. Kretzschmar T, von Ruden T. Antibody discovery: phage display. Curr Opin Biotechnol (2002) 13:598–602. doi:10.1016/S0958-1669(02)00380-4
20. Dufner P, Jermutus L, Minter RR. Harnessing phage and ribosome display for antibody optimisation. Trends Biotechnol (2006) 24:523–9. doi:10.1016/j.tibtech.2006.09.004
21. Groves MA, Osbourn JK. Applications of ribosome display to antibody drug discovery. Expert Opin Biol Ther (2005) 5:125–35. doi:10.1517/14712598.5.1.125
22. Pepper LR, Cho YK, Boder ET, Shusta EV. A decade of yeast surface display technology: where are we now? Comb Chem High Throughput Screen (2008) 11:127–34. doi:10.2174/138620708783744516
23. Rhiel L, Krah S, Gunther R, Becker S, Kolmar H, Hock B. REAL-select: full-length antibody display and library screening by surface capture on yeast cells. PLoS One (2014) 9:e114887. doi:10.1371/journal.pone.0114887
24. Lippow SM, Wittrup KD, Tidor B. Computational design of antibody-affinity improvement beyond in vivo maturation. Nat Biotechnol (2007) 25:1171–6. doi:10.1038/nbt1336
25. Sidhu SS, Lowman HB, Cunningham BC, Wells JA. Phage display for selection of novel binding peptides. Methods Enzymol (2000) 328:333–63. doi:10.1016/S0076-6879(00)28406-1
26. Winter G, Griffiths AD, Hawkins RE, Hoogenboom HR. Making antibodies by phage display technology. Annu Rev Immunol (1994) 12:433–55. doi:10.1146/annurev.iy.12.040194.002245
27. Rakestraw JA, Baskaran AR, Wittrup KD. A flow cytometric assay for screening improved heterologous protein secretion in yeast. Biotechnol Prog (2006) 22:1200–8. doi:10.1021/bp0600233
28. Lee CV, Liang WC, Dennis MS, Eigenbrot C, Sidhu SS, Fuh G. High-affinity human antibodies from phage-displayed synthetic Fab libraries with a single framework scaffold. J Mol Biol (2004) 340:1073–93. doi:10.1016/j.jmb.2004.05.051
29. Fellouse FA, Esaki K, Birtalan S, Raptis D, Cancasci VJ, Koide A, et al. High-throughput generation of synthetic antibodies from highly functional minimalist phage-displayed libraries. J Mol Biol (2007) 373:924–40. doi:10.1016/j.jmb.2007.08.005
30. Birtalan S, Fisher RD, Sidhu SS. The functional capacity of the natural amino acids for molecular recognition. Mol Biosyst (2010) 6:1186–94. doi:10.1039/b927393j
31. Birtalan S, Zhang Y, Fellouse FA, Shao L, Schaefer G, Sidhu SS. The intrinsic contributions of tyrosine, serine, glycine and arginine to the affinity and specificity of antibodies. J Mol Biol (2008) 377:1518–28. doi:10.1016/j.jmb.2008.01.093
32. Baek DS, Kim YS. Construction of a large synthetic human Fab antibody library on yeast cell surface by optimized yeast mating. J Microbiol Biotechnol (2014) 24:408–20. doi:10.4014/jmb.1401.01002
33. Nelson B, Sidhu SS. Synthetic antibody libraries. Methods Mol Biol (2012) 899:27–41. doi:10.1007/978-1-61779-921-1_2
34. Fellouse FA, Li B, Compaan DM, Peden AA, Hymowitz SG, Sidhu SS. Molecular recognition by a binary code. J Mol Biol (2005) 348:1153–62. doi:10.1016/j.jmb.2005.03.041
35. Knappik A, Ge L, Honegger A, Pack P, Fischer M, Wellnhofer G, et al. Fully synthetic human combinatorial antibody libraries (HuCAL) based on modular consensus frameworks and CDRs randomized with trinucleotides. J Mol Biol (2000) 296:57–86. doi:10.1006/jmbi.1999.3444
36. Fellouse FA, Wiesmann C, Sidhu SS. Synthetic antibodies from a four-amino-acid code: a dominant role for tyrosine in antigen recognition. Proc Natl Acad Sci U S A (2004) 101:12467–72. doi:10.1073/pnas.0401786101
37. Rajan S, Sidhu SS. Simplified synthetic antibody libraries. Methods Enzymol (2012) 502:3–23. doi:10.1016/B978-0-12-416039-2.00001-X
38. Chen G, Sidhu SS. Design and generation of synthetic antibody libraries for phage display. Methods Mol Biol (2014) 1131:113–31. doi:10.1007/978-1-62703-992-5_8
39. Robin G, Sato Y, Desplancq D, Rochel N, Weiss E, Martineau P. Restricted diversity of antigen binding residues of antibodies revealed by computational alanine scanning of 227 antibody-antigen complexes. J Mol Biol (2014) 426:3729–43. doi:10.1016/j.jmb.2014.08.013
40. Shim H. Synthetic approach to the generation of antibody diversity. BMB Rep (2015) 48:489–94. doi:10.5483/BMBRep.2015.48.9.120
41. Zhai W, Glanville J, Fuhrmann M, Mei L, Ni I, Sundar PD, et al. Synthetic antibodies designed on natural sequence landscapes. J Mol Biol (2011) 412:55–71. doi:10.1016/j.jmb.2011.07.018
42. Kehoe JW, Whitaker B, Bethea D, Lacy ER, Boakye K, Santulli-Marotto S, et al. Isolation and optimization for affinity and biophysical characteristics of anti-CCL17 antibodies from the VH1-69 germline gene. Protein Eng Des Sel (2014) 27:199–206. doi:10.1093/protein/gzu012
43. Dobson CL, Devine PW, Phillips JJ, Higazi DR, Lloyd C, Popovic B, et al. Engineering the surface properties of a human monoclonal antibody prevents self-association and rapid clearance in vivo. Sci Rep (2016) 6:38644. doi:10.1038/srep38644
44. Wu SJ, Luo J, O’Neil KT, Kang J, Lacy ER, Canziani G, et al. Structure-based engineering of a monoclonal antibody for improved solubility. Protein Eng Des Sel (2010) 23:643–51. doi:10.1093/protein/gzq037
45. Bethea D, Wu SJ, Luo J, Hyun L, Lacy ER, Teplyakov A, et al. Mechanisms of self-association of a human monoclonal antibody CNTO607. Protein Eng Des Sel (2012) 25:531–7. doi:10.1093/protein/gzs047
46. Perchiacca JM, Ladiwala AR, Bhattacharya M, Tessier PM. Aggregation-resistant domain antibodies engineered with charged mutations near the edges of the complementarity-determining regions. Protein Eng Des Sel (2012) 25:591–601. doi:10.1093/protein/gzs042
47. Perchiacca JM, Lee CC, Tessier PM. Optimal charged mutations in the complementarity-determining regions that prevent domain antibody aggregation are dependent on the antibody scaffold. Protein Eng Des Sel (2014) 27:29–39. doi:10.1093/protein/gzt058
48. Sun SB, Sen S, Kim NJ, Magliery TJ, Schultz PG, Wang F. Mutational analysis of 48G7 reveals that somatic hypermutation affects both antibody stability and binding affinity. J Am Chem Soc (2013) 135:9980–3. doi:10.1021/ja402927u
49. Wang F, Sen S, Zhang Y, Ahmad I, Zhu X, Wilson IA, et al. Somatic hypermutation maintains antibody thermodynamic stability during affinity maturation. Proc Natl Acad Sci U S A (2013) 110:4261–6. doi:10.1073/pnas.1301810110
50. Dimitrov JD, Kaveri SV, Lacroix-Desmazes S. Thermodynamic stability contributes to immunoglobulin specificity. Trends Biochem Sci (2014) 39:221–6. doi:10.1016/j.tibs.2014.02.010
51. Julian MC, Li L, Garde S, Wilen R, Tessier PM. Efficient affinity maturation of antibody variable domains requires co-selection of compensatory mutations to maintain thermodynamic stability. Sci Rep (2017) 7:45259. doi:10.1038/srep45259
52. De Genst EJ, Guilliams T, Wellens J, O’Day EM, Waudby CA, Meehan S, et al. Structure and properties of a complex of alpha-synuclein and a single-domain camelid antibody. J Mol Biol (2010) 402:326–43. doi:10.1016/j.jmb.2010.07.001
53. Kawa S, Onda M, Ho M, Kreitman RJ, Bera TK, Pastan I. The improvement of an anti-CD22 immunotoxin: conversion to single-chain and disulfide stabilized form and affinity maturation by alanine scan. MAbs (2011) 3:479–86. doi:10.4161/mabs.3.5.17228
54. Chi SW, Maeng CY, Kim SJ, Oh MS, Ryu CJ, Kim SJ, et al. Broadly neutralizing anti-hepatitis B virus antibody reveals a complementarity determining region H3 lid-opening mechanism. Proc Natl Acad Sci U S A (2007) 104:9230–5. doi:10.1073/pnas.0701279104
55. Swindells MB, Porter CT, Couch M, Hurst J, Abhinandan KR, Nielsen JH, et al. abYsis: integrated antibody sequence and structure-management, analysis, and prediction. J Mol Biol (2017) 429:356–64. doi:10.1016/j.jmb.2016.08.019
56. Tiller KE, Li L, Kumar S, Julian MC, Garde S, Tessier PM. Arginine mutations in antibody complementarity-determining regions display context-dependent affinity/specificity trade-offs. J Biol Chem. (2017). doi:10.1074/jbc.M117.783837
57. Swillens S. Interpretation of binding curves obtained with high receptor concentrations: practical aid for computer analysis. Mol Pharmacol (1995) 47:1197–203.
58. Julian MC, Lee CC, Tiller KE, Rabia LA, Day EK, Schick AJ III, et al. Co-evolution of affinity and stability of grafted amyloid-motif domain antibodies. Protein Eng Des Sel (2015) 28:339–50. doi:10.1093/protein/gzv050
59. Brooks BR, Brooks CL III, Mackerell AD Jr, Nilsson L, Petrella RJ, Roux B, et al. CHARMM: the biomolecular simulation program. J Comput Chem (2009) 30:1545–614. doi:10.1002/jcc.21287
60. Zahnd C, Spinelli S, Luginbuhl B, Amstutz P, Cambillau C, Pluckthun A. Directed in vitro evolution and crystallographic analysis of a peptide-binding single chain antibody fragment (scFv) with low picomolar affinity. J Biol Chem (2004) 279:18870–7. doi:10.1074/jbc.M309169200
61. Cauerhff A, Goldbaum FA, Braden BC. Structural mechanism for affinity maturation of an anti-lysozyme antibody. Proc Natl Acad Sci U S A (2004) 101:3539–44. doi:10.1073/pnas.0400060101
62. Midelfort KS, Wittrup KD. Context-dependent mutations predominate in an engineered high-affinity single chain antibody fragment. Protein Sci (2006) 15:324–34. doi:10.1110/ps.051842406
63. Boder ET, Midelfort KS, Wittrup KD. Directed evolution of antibody fragments with monovalent femtomolar antigen-binding affinity. Proc Natl Acad Sci U S A (2000) 97:10701–5. doi:10.1073/pnas.170297297
64. Marvin JS, Lowman HB. Redesigning an antibody fragment for faster association with its antigen. Biochemistry (2003) 42:7077–83. doi:10.1021/bi026947q
65. Wells JA. Additivity of mutational effects in proteins. Biochemistry (1990) 29:8509–17. doi:10.1021/bi00489a001
66. Daugherty PS, Chen G, Iverson BL, Georgiou G. Quantitative analysis of the effect of the mutation frequency on the affinity maturation of single chain Fv antibodies. Proc Natl Acad Sci U S A (2000) 97:2029–34. doi:10.1073/pnas.030527597
67. Clark LA, Boriack-Sjodin PA, Eldredge J, Fitch C, Friedman B, Hanf KJ, et al. Affinity enhancement of an in vivo matured therapeutic antibody using structure-based computational design. Protein Sci (2006) 15:949–60. doi:10.1110/ps.052030506
68. Mateu MG, Andreu D, Carreno C, Roig X, Cairo JJ, Camarero JA, et al. Non-additive effects of multiple amino acid substitutions on antigen-antibody recognition. Eur J Immunol (1992) 22:1385–9. doi:10.1002/eji.1830220609
69. Kiyoshi M, Caaveiro JM, Miura E, Nagatoishi S, Nakakido M, Soga S, et al. Affinity improvement of a therapeutic antibody by structure-based computational design: generation of electrostatic interactions in the transition state stabilizes the antibody-antigen complex. PLoS One (2014) 9:e87099. doi:10.1371/journal.pone.0087099
70. Soderlind E, Strandberg L, Jirholt P, Kobayashi N, Alexeiva V, Aberg AM, et al. Recombining germline-derived CDR sequences for creating diverse single-framework antibody libraries. Nat Biotechnol (2000) 18:852–6. doi:10.1038/78458
71. Miersch S, Sidhu SS. Synthetic antibodies: concepts, potential and practical considerations. Methods (2012) 57:486–98. doi:10.1016/j.ymeth.2012.06.012
72. Sidhu SS, Li B, Chen Y, Fellouse FA, Eigenbrot C, Fuh G. Phage-displayed antibody libraries of synthetic heavy chain complementarity determining regions. J Mol Biol (2004) 338:299–310. doi:10.1016/j.jmb.2004.02.050
73. Li B, Russell SJ, Compaan DM, Totpal K, Marsters SA, Ashkenazi A, et al. Activation of the proapoptotic death receptor DR5 by oligomeric peptide and antibody agonists. J Mol Biol (2006) 361:522–36. doi:10.1016/j.jmb.2006.06.042
74. Lombana TN, Dillon M, Bevers J III, Spiess C. Optimizing antibody expression by using the naturally occurring framework diversity in a live bacterial antibody display system. Sci Rep (2015) 5:17488. doi:10.1038/srep17488
75. Jain T, Sun T, Durand S, Hall A, Houston NR, Nett JH, et al. Biophysical properties of the clinical-stage antibody landscape. Proc Natl Acad Sci U S A (2017) 114:944–9. doi:10.1073/pnas.1616408114
76. Hoover DM, Lubkowski J. DNAWorks: an automated method for designing oligonucleotides for PCR-based gene synthesis. Nucleic Acids Res (2002) 30:e43. doi:10.1093/nar/30.10.e43
77. Studier FW. Protein production by auto-induction in high density shaking cultures. Protein Expr Purif (2005) 41:207–34. doi:10.1016/j.pep.2005.01.016
78. Brooks BR, Bruccoleri RE, Olafson BD, States DJ, Swaminathan S, Karplus M. CHARMM: a program for macromolecular energy, minimization, and dynamics calculations. J Comput Chem (1983) 4:187–217. doi:10.1002/jcc.540040211
79. Kortemme T, Baker D. A simple physical model for binding energy hot spots in protein-protein complexes. Proc Natl Acad Sci U S A (2002) 99:14116–21. doi:10.1073/pnas.202485799
80. Kim DE, Chivian D, Baker D. Protein structure prediction and analysis using the Robetta server. Nucleic Acids Res (2004) 32:W526–31. doi:10.1093/nar/gkh468
81. Kortemme T, Kim DE, Baker D. Computational alanine scanning of protein-protein interfaces. Sci STKE (2004) 2004:l2. doi:10.1126/stke.2192004pl2
82. Pantazes RJ, Grisewood MJ, Li T, Gifford NP, Maranas CD. The iterative protein redesign and optimization (IPRO) suite of programs. J Comput Chem (2015) 36:251–63. doi:10.1002/jcc.23796
Keywords: complementarity-determining region, stability, specificity, library, directed evolution, yeast surface display, protein design
Citation: Tiller KE, Chowdhury R, Li T, Ludwig SD, Sen S, Maranas CD and Tessier PM (2017) Facile Affinity Maturation of Antibody Variable Domains Using Natural Diversity Mutagenesis. Front. Immunol. 8:986. doi: 10.3389/fimmu.2017.00986
Received: 07 June 2017; Accepted: 02 August 2017;
Published: 04 September 2017
Edited by:
Kevin A. Henry, National Research Council Canada, CanadaReviewed by:
Jennifer Maynard, University of Texas at Austin, United StatesXin Ge, University of California, Riverside, United States
Yongku Cho, University of Connecticut, United States
Copyright: © 2017 Tiller, Chowdhury, Li, Ludwig, Sen, Maranas and Tessier. This is an open-access article distributed under the terms of the Creative Commons Attribution License (CC BY). The use, distribution or reproduction in other forums is permitted, provided the original author(s) or licensor are credited and that the original publication in this journal is cited, in accordance with accepted academic practice. No use, distribution or reproduction is permitted which does not comply with these terms.
*Correspondence: Peter M. Tessier, cHRlc3NpZXJAdW1pY2guZWR1
†Present address: Tong Li, BASF Corporation, San Diego, CA, United States