- Infection and Immunity Program, Monash Biomedicine Discovery Institute and Department of Biochemistry and Molecular Biology, Monash University, Clayton, VIC, Australia
The conformational dynamism of proteins is well established. Rather than having a single structure, proteins are more accurately described as a conformational ensemble that exists across a rugged energy landscape, where different conformational sub-states interconvert. The interaction between αβ T cell receptors (TCR) and cognate peptide-MHC (pMHC) is no exception, and is a dynamic process that involves substantial conformational change. This review focuses on technological advances that have begun to establish the role of conformational dynamics and dynamic allostery in TCR recognition of the pMHC and the early stages of signaling. We discuss how the marriage of molecular dynamics (MD) simulations with experimental techniques provides us with new ways to dissect and interpret the process of TCR ligation. Notably, application of simulation techniques lags behind other fields, but is predicted to make substantial contributions. Finally, we highlight integrated approaches that are being used to shed light on some of the key outstanding questions in the early events leading to TCR signaling.
Introduction
Self or foreign intracellular peptides are presented on the surface of antigen presenting cells (APC) by major histocompatibility complex (MHC) class I molecules. These peptide-bound MHC (pMHC) molecules undergo surveillance by CD8+ cytotoxic T lymphocytes (CTLs) via the αβ T cell receptors (TCR) that are expressed on their surface. TCR engagement of the pMHC leads to the formation of an immune synapse that is central to T cell activation (Figure 1A). The outcome of T cell engagement with the pMHC influences T cell fate, playing a role in the defense against infection and cancer, but on the flip side, allergic reactions, autoimmune disease, transplant rejection, and drug hypersensitivity. Despite the importance of T cell activation, we have a poor understanding of how the TCR-pMHC initiates an intracellular signal and this impedes our ability to manipulate the T cell response to target infection and cancer. What is clear however is that there is enormous complexity to the overall response and dissecting it requires the integration of a diverse suite of both experimental and computational tools and techniques.
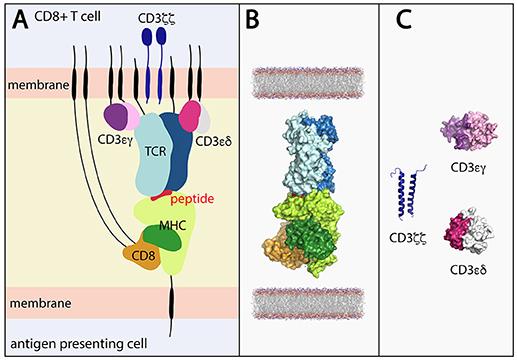
Figure 1. (A) Stylized view of the pMHC-TCR and core components required for T cell signaling. (B) Components of the pMHC-TCR complex for which structures have been determined in combination with one another. The complex depicts peptide-bound HLA-A*02 in complex with CD8 and the B7 TCR (derived by superimposing components of PDB ID's 1AKJ and 1BD2. (C) Portions of the CD3εγ (ectodomain; PDB ID 1SY6), CD3εδ (ectodomain; PDB 1XIW) and CD3ζζ (TM domains; PDB ID 2MAC) signaling components have been structurally determined, but not in complex with the TCR. Black lines represent regions of conformational flexibility. TCR α and β chain shown in dark and light blue, respectively. MHC class I heavy and light (β2-microglobulin) chain shown in light and dark green, respectively. Peptide shown in red. CD8αα shown in orange.
Due to the relatively small size of the TCR-pMHC, X-ray crystallography has led the way in the structural determination of the extracellular domains of pMHC and TCR alone or in complex with one another at near-atomic resolution. These studies detail the conformation of the peptide, its interactions with MHC as well as the TCR and structural changes the MHC and/or peptide undergoes upon TCR binding. It was long-anticipated that these accrued X-ray structures would also reveal how the information at the pMHC interface is communicated from the variable domains to the membrane proximal constant domains and via the CD3 subunits necessary for signal transduction. A clear mechanism however has not been revealed, exemplified by instances where single amino acid changes in a peptide produce near-identical structural snapshots but different T cell outcomes (1–3). This indicates additional factors, concealed by X-ray crystallographic snapshots, are at play.
There is emerging evidence that conformational dynamics and dynamic allostery influences T cell recognition and activation (4–6), yet until recently, this has been overlooked in our effort to understand the structural basis of TCR recognition of the pMHC. The importance of conformational dynamics at the immune synapse has been the subject of excellent recent reviews [see, for example (7–12)]. In this review, we instead focus on relevant methodologies (highlighted in Figure 2), and specifically recent advances in computational, structural and biophysical techniques, and how they can be integrated to provide powerful insights into the key early stages of the TCR-pMHC interaction. Finally, we highlight integrated approaches that are being used to shed light on some of the key outstanding questions in the early events leading to TCR signaling.
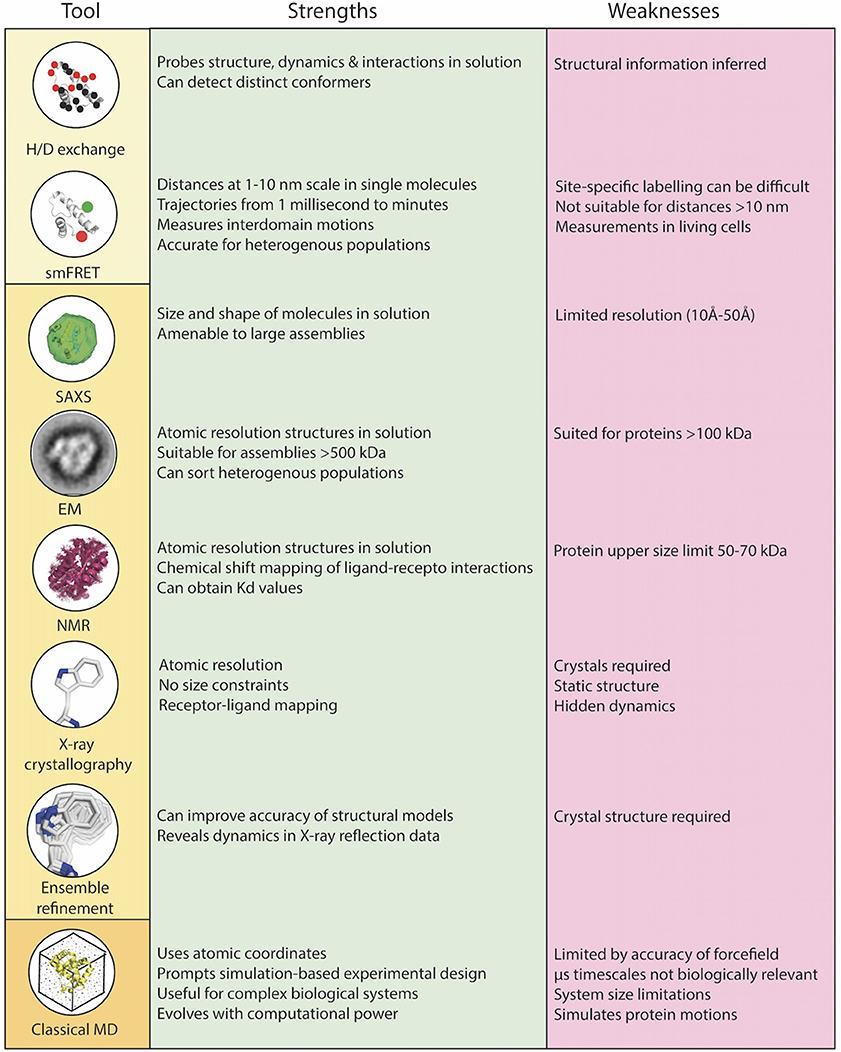
Figure 2. Combinations of biophysical, structural, and computational techniques are a necessity to overcome the limitations of each individual technique and to rigorously understand the role of dynamics in TCR-pMHC function at the core of the immunological synapse. Biophysical techniques (yellow box), structural techniques (light orange box), computational techniques (dark orange box).
X-ray Crystallography: Pioneering Atomic Resolution Details
Typically, the structural flexibility of a TCR-pMHC system is solely interpreted from a single set of coordinates derived from X-ray diffraction data. In this case flexibility is merely inferred mainly by comparing structural differences between TCR-pMHC and their unbound constituents (13, 14) and the consideration of atomic temperature (B) factors. B-factors can be used to estimate atomic displacements that arise from static and dynamic disorder (alternative conformations in the crystal lattice, and atomic fluctuations in the crystal, respectively). By comparing identical molecules in different crystal lattices, the influence of crystal packing on protein structure can be analyzed (15–17). Indeed, crystal packing can select radically different conformations from a heterogeneous ensemble, giving clues to conformational dynamics (16). For example, structural variation of HLA-B*35:08-LPEP with SB27 TCR within two crystal forms suggested a “scanning” motion of the TCR on pMHC that was further supported and extended by molecular dynamics (MD) studies (3, 18).
Due to crystal packing and data collection at cryogenic temperatures, B-factors underrepresent the amplitude of conformational populations (19). Thus, due to the failure of current refinement algorithms to model structural heterogeneity, the analysis of single, static crystallographic models can reveal limited information on the dynamics of the system in solution (20–22). However, there are examples where flexibility insight has been successfully obtained and useful correlations made. For example, B-factor analysis of structures of HLA-B*35:01 and HLA-B*35:08, that differ by a single amino acid, but are bound to the same Epstein-Barr virus (EBV) peptide (HPVG) provided insights into the influence of MHC polymorphism on peptide mobility and the T cell response (23). Likewise, the structural comparison of a TCR in its unbound vs. pMHC-bound state revealed a conformational change in the A-B loop of the Cα domain that borders the CD3ε binding site (24) that was later verified to correlate with pMHC ligation (25).
In addition to concealing flexibility, the X-ray structures available are also incomplete and lack the core components necessary for signal transduction (Figures 1B,C). While the structures of TCR-pMHC, pMHC-CD8 (26–29), CD3 heterodimers (30–34), and CD8 homo/heterodimers (35, 36) have been determined, critically informative complexes such as CD8 and/or CD3 in complex with TCR-pMHC or even just TCR-CD3 are lacking due to the poor affinity of soluble CD3 and CD8 for the TCR and MHC, respectively (33, 37, 38). Also absent, due to technical challenges, are the stalk regions, membrane-spanning domains, and intracellular tails of the TCR, MHC, and CD8 and CD3 molecules, which play a role in complex assembly, the spatial organization of the components and signal transmission (39–46). Therefore, whilst X-ray crystallography can yield highly informative, high-resolution structures, its limitations necessitate the use of clever engineering and complementary techniques to make the next leap in terms of T cell signaling.
Molecular Dynamics Simulations: Producing Testable Hypotheses and Placing Experimental Findings into a Theoretical Framework
Although conventional X-ray crystallographic analysis provides little information regarding dynamics, the exquisite resolution as well as model completeness has provided a solid data foundation that has spurned an increasing amount of MD simulation studies. All-atom MD simulations probe the flexibility of the system by computing iterative solutions of Newton's equations of motion over time (47). The raw output of MD simulations—trajectories, describe the atomic positions at time-points during the simulation. Unfortunately however, MD is computationally demanding and limits the technique to examination of relatively short time spans (e.g., a microsecond), orders of magnitude shorter than more biologically relevant timescales over which many larger motions occur. The signal, for example, produced following T cell recognition of a pMHC is of the seconds to minutes timescale (48–52). Several approaches have emerged that allow this limitation to be mitigated somewhat and here we discuss briefly below the most popular and useful approaches. Replica exchange MD (REMD) can improve the sampling by simulating multiple copies of the same molecule at different temperatures, allowing an unbiased way of improving conformational sampling (53). REMD is computationally intensive and therefore currently limited to relatively small systems, for example to investigate how polymorphic amino acid differences between allotypes alter the conformational plasticity of the MHC class I binding pocket (54). Alternatively, steered MD (SMD) applies an external force to the protein to study its mechanical response, analogous to atomic force microscopy (55). This method is well suited to studying protein-protein interactions, and has been used to investigate TCR-pMHC dissociation (56–58). A novel use of SMD simulations based on agonist and non-agonist complex crystal structures was to develop a molecular model of TCR-pMHC “catch bond” formation (12). Catch bonds represent a net accumulation of molecular interactions under force, revealing an additional level of dynamic diversity built-in as a proofreading mechanism to link TCR recognition and subsequent activation. The related approaches of targeted MD (TMD) and umbrella sampling apply forces in order to promote new conformations, and are used to predict a pathway between two known conformations. Such “pulling” simulations, although not used to study dynamics per se, have been used to estimate the binding free energies between peptides and MHC (59). The atomic complexity of the system can be reduced significantly using coarse-grained (CG) methods in which groups of atoms are replaced by beads, allowing longer simulations at the cost of fine details (60). This approach is therefore useful for studying larger complexes such as TCR-pMHC in membrane (61) and TCR-pMHC-CD4 complexes (62). CG methods are complimentary to atomistic simulations and offer a feasible approach to tackling the combined challenges of large immune assemblies and long timescales associated with changes in membrane morphology. Accuracy of MD simulations are dependent upon available force fields—mathematical-physical descriptions of a system used to calculate the forces acting upon all atoms in order to solve Newton's equations of motion. Force fields, though improving constantly, have known imperfections (63–65), so currently it is preferable to seek experimental validation. Getting stuck in local energy minima is a particular limitation, but energy landscapes can be sampled more efficiently using advanced adaptive sampling techniques such as Markov state models (66), allowing the identification of metastable states.
In summary, MD simulation is an increasingly important member of the toolbox, since it is particularly well suited to producing experimentally testable hypotheses as well as placing existing experimental findings into a theoretical framework. Despite the advances in enhanced sampling methods and possible simulation of larger complexes, MD simulations of experimentally-determined TCR-pMHC structures have not been widely adopted.
Ensemble Refinement: Using MD Simulations to Extract Dynamics from the Crystalline State
Another way to explore protein dynamics from experimental diffraction data is by simultaneously performing short molecular dynamics simulations with structure refinement (20, 67, 68). This method, known as ensemble refinement, produces an ensemble of models of the same structure that provides extended biological insight, often whilst improving the refinement statistics [i.e., free R-factor (Rfree)]. Although the ensemble refinement method has long been established and is well-validated (69–72), it is not routinely incorporated as a tool to analyze the X-ray structures of pMHC class I systems. This prompted our re-analysis of 11 published systems to reveal the dynamics present in the X-ray data, revealing the benefits of incorporating ensemble refinement to the structural interpretation (73).
A pertinent example of how ensemble refinement can extend and enrich existing crystallographic interpretations relates to the induced fit vs. conformational selection model of TCR binding to pMHC. In the induced fit model, the TCR undergoes a conformational change upon binding the pMHC, whereas in the conformational selection model a conformation that is compatible with binding is selected from an ensemble of conformations (74). Borbulevych et al. (75) sought to understand the causes of the cross-recognition of self- and non-self-peptides by the A6 TCR, by comparing the structures of the self-peptide HuD and the non-self-peptide Tax, both when bound to MHC HLA-A*02 alone and in a MHC-TCR complex. While the bound conformations are very similar for both peptides, differences in the orientation of the p3 and p5 side chains necessitate that the HuD peptide must undergo a conformational change in order to bind to the TCR (Figure 3A), while the Tax peptide does not. Our ensemble results, however, show that the Tyr3 and Phe5 residues in the HuD peptide are flexible enough to convert between the two conformations (Figure 3B). This indicates that the differences found between the MHC and TCR-pMHC conformations may be due to intrinsic flexibility rather than any change elicited by binding itself, and suggests that differences between static pMHC and TCR-pMHC may be due to a combination of the inherent flexibilities of each system, and the complexation process. Static structures may bias the interpretation in favor of an induced fit mechanism, whereas analysis as a conformational ensemble can allow also for conformational selection. Furthermore, reliance on single crystallographic structures of pMHC, with or without TCR, entails pitfalls for understanding the rules of productive TCR ligation, particularly for static interpretations involving fine details such as interaction networks and side chain orientation. Since in the worst cases, it may fail to properly distinguish real results from noise, it supports a view that biological observations should be explained through the properties of ensembles rather than isolated structures, as these are less prone to observer bias.
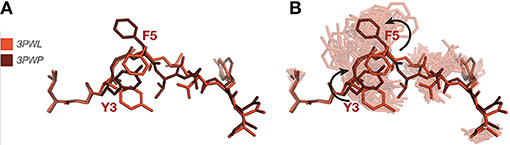
Figure 3. (A) Superposition of HuD-HLA peptide (red) (PDB ID 3PWL) and TCR-HuD-HLA peptide (brown) (PDB ID 3PWP) showing putative TCR binding-induced bond rotations. (B) Ensemble refinement show both these variants occur in the pMHC ensemble. MHC α-helices have been omitted.
While we tend to describe TCR binding to the pMHC as either undergoing induced fit or conformational selection, the likelihood is that the TCR binds through a combination of both models. Scott et al. (6), highlight using time resolved fluorescence measurements, MD and structural and thermodynamic data that the CDR3 loops of a TCR can have varying degrees of flexibility. In the case of the A6 TCR the CDR3β loop is highly flexible and can rapidly sample ligands with a range of conformations, whereas the CDR3α has a slower motion that restricts the repertoire of peptides that it can bind. Therefore, the two models are not necessarily mutually exclusive, but instead describe a continuum (74).
Computing requirements of ensemble refinement are typically much greater than for single structure analysis, likely explaining the relatively slow adoption of this technique. However, continual improvements in refinement software [notably Phenix, which allows straightforward and user-friendly ensemble refinement from a PC (76)] and hardware have now placed this technique easily within the grasp of most structural immunologists. For example, in a recent ensemble refinement analysis of pMHC structures, refinement could be completed in < 3 h for most systems, using a typical off-the-shelf desktop computer (73).
Nuclear Magnetic Resonance Spectroscopy (NMR): Atomistic Dynamics in Solution
NMR measures the absorbance and re-emission of electromagnetic radiation by nuclei in a magnetic field, and has provided significant information on protein structure and dynamics for the past 30 years. The use of NMR spectroscopy to study pMHC dynamics is complicated by the relatively large size of these systems (77), however several recent studies that have characterized TCR-pMHC binding have all found that significant conformational variation exists in the TCR, peptide, and MHC (78). In addition to noting conformational changes at the TCR-pMHC interface, conformational variation was also observed at remote sites, including within the membrane-proximal Cβ domain of the TCR, that implies an allosteric mechanism in TCR signaling (79), and the β2m (β2-microglobulin) binding site on the MHC that was sensitive to MHC polymorphism and the bound peptide (80). Notably, each of these NMR studies benefitted from mapping chemical shift perturbations onto available X-ray structures, but revealed flexible regions not otherwise observed from the X-ray structures alone. NMR has also been used to validate MD predictions that show long-range allosteric communication between the TCR binding sites for pMHC and CD3, a key step in early T-cell activation (81). These NMR studies reiterate the need to characterize the TCR-pMHC system as an allosteric ensemble in which ligand binding alters the energy landscape of the entire ensemble. In an allosteric ensemble conformational changes that concurrently occur at distal sites, but do not necessarily dominate the ensemble, can be mapped to reveal cooperativity between sites, or dynamically-driven allostery, revealing previously hidden and unforseen insights into signal transmission (82–84).
Fluorescence Spectroscopy: Probing Environmental Dynamics and Distances
The intrinsic fluorescence of aromatic (usually tryptophan) residues is sensitive to their environment, and can therefore be used to monitor dynamics. Fluorescence anisotropy, in particular, has become a powerful method with which to study pMHC-TCR dynamics, especially when coupled with other techniques (85–87). Dynamic insight has also been gained by Förster resonance energy transfer (FRET), in which energy transfered between a donor and an acceptor chromophore is used to measure distances between chromophore-labeled residues as a function of time, particularly powerful when combined with structural techniques (88–90). An elegant example of integrating experimental and computational approaches, from the protein folding field, combined small molecule (sm) FRET with advanced MD simulations and machine learning (91). Such a combined approach has not yet been reported for pMHC-TCR systems, but clearly holds much promise. Nevertheless, there are other examples pertaining to the value of the use of fluorescence to study pMHC-TCR systems. For example, site-directed fluorescence labeling, in which an extrinsic fluorescent probe is attached to a cysteine residue, has been used to note that the A-B loop within the TCR Cα domain undergoes a conformational change upon pMHC ligation (25). FRET has also been used to measure intermolecular associations in live cells. Yachi et al. (92) measured the molecular interaction between TCR-CD3ζ and CD8 on antigen presenting cells loaded with different peptides, revealing structurally similar peptides alter the kinetics of the CD8-TCR interaction and translate into differential T cell responses. Another study used a FRET sensor to map the spatiotemporal dynamics of protein clustering in live T cells, linking the molecular density of TCR clusters with TCR triggering (93). Clearly, our understanding of TCR-pMHC systems could benefit from the further integrated use of intramolecular and intermolecular FRET sensors, particularly when coupled with structural data.
Hydrogen/Deuterium (H/D) Exchange: Solvent Accessibility and Local Dynamics
H/D exchange involves a steady-state reaction in which deuterium atoms replace covalently bonded hydrogen atoms that are not participating in H-bonds. The rate of that exchange is usually measured by mass spectrometry, providing information on solvent accessibility and local dynamics. A study combining H/D exchange, fluorescence anisotropy, and structural analyses, showed that the flexibility of the peptide binding groove of the class I MHC protein HLA-A*02:01 varies significantly with different peptides (85). Further evidence for the role of conformational plasticity in peptide selection by MHC Class I was revealed by comparing H/D exchange of two allotypes in peptide-bound and free states (94). H/D exchange has also been used to probe the dynamics at the TCR-pMHC interface, with several studies highlighting that conformational flexibility is contingent upon the MHC allele (95), the bound peptide (85), and upon TCR ligation (96) and all of which have implications for T cell signaling.
Small Angle X-ray Scattering (SAXS): Low Resolution Structure in Solution
Despite inherent limitations in resolution that can be achieved, SAXS can be used to study the size, shape and assembly of proteins, without the size limitations of other techniques such as NMR (97). In a monodisperse solution, geometric parameters such as the maximum particle dimension (Dmax), radius of gyration (Rg), and the forward scattering intensity, I(0), can be calculated from SAXS data; these values can serve as a point of comparison with the dimensions provided by a crystal structure or when studying the same protein under various experimental conditions or in a liganded vs. unliganded state. For example, in conjunction with other techniques and by comparing the Rg of HLA-DR1 (MHC class II) bound to a wild-type peptide, or a weak- or tight-binding peptide variant Yin et al. (98, 99) correlated pMHC conformational differences with susceptibility to peptide exchange by the non-classical MHC class II molecule HLA-DM. In another pMHC class II system, SAXS was used to show the pathogen-derived proteins, Salp15 and gp120, caused binding-induced conformational changes in CD4 that subsequently influence CD4+ T cell activation during infection (100, 101).
SAXS can also be used to characterize polydisperse systems such as modular proteins with flexible linkers or proteins bearing disordered regions. The ensemble optimization method (EOM) (102) is one approach to describe this experimental SAXS data. It generates a pool of n independent models based upon the sequence and structural information of the target and then selects a subset of ensembles that best describe the experimental SAXS data. The distributions of the properties of the selected ensembles, including Rg (radius of gyration), Dmax (maximum particle dimension), Rflex (measure of flexibility) and Rσ (variance of the ensemble distribution with respect to the original pool), can then be compared to those of the pool of independent models to assess the flexibility of the system. To the best of our knowledge EOM has not yet been used to study TCR-pMHC systems, despite both MHCs and TCRs being multidomain proteins with flexible linkers. It is thus highly feasible the interdomain motions of these proteins are coupled to binding events and are linked to signal transduction. On that note, the flexible stalks of the TCR, MHC, CD8, and CD3 molecules also likely play a role.
Conclusions and Future Perspectives
Protein flexibility is inherent to protein structure and function, and TCR-pMHC systems are no exception. Despite this the systematic analysis of the flexibility of TCR-pMHC systems is lagging far behind that of other fields (103–105), particularly when it comes to integration of computational and experimental techniques.
We propose that to advance our mechanistic understanding of how TCR-pMHC engagement initiates intracellular signaling, and the influence of the peptide on the signal, that there needs to be a shift in our approach, both in terms of the suite of techniques used to assess flexibility, and use of creative engineering to surpass limitations specific to the molecules in question and their applicability to a technique. Elegant examples that illustrate the strength of this marriage are now emerging. For example, Natarajan et al. (38) overcame the size barrier that limits the study of the soluble TCR by NMR through the use of perdeuteration, and concomitantly simplified the NMR spectra using partial subunit labeling. This NMR approach combined with mutagenesis, computational docking, and validation using cell-based assays has enhanced our understanding of how the extracellular engagement of the TCR-CD3 complex transmits a signal. Likewise, Birnbaum et al. (106) implemented clever strategies to circumvent size limitations and issues pertaining to sample heterogeneity to use electron microscopy to observe the molecular architecture of the membrane-associated TCR-CD3 complex bound to pMHC. Using this approach combined with SAXS they put forward a ligand-dependent dimerization mechanism for TCR signaling in which flexibility plays a key role.
We also propose the ensemble refinement technique be used routinely in the X-ray crystallographic analysis of TCR-pMHC systems. The routine extraction of this data, and validation/interpretation in conjunction with other experimental techniques, some of which are summarized here, will provide previously hidden insights into the scope of conformational changes permissible by peptides when bound to MHC that influence TCR binding and T cell activation and will also reveal insights into how TCR flexibility and dynamically-driven allostery play a role. This hitherto missing information will enable us to more fully consider how a signal is transduced from the pMHC interface via the CD3 subunits and to determine how flexibility at the interface correlates with the degree of T cell stimulation (79, 107). This may provide new insights into how the T cell response can be therapeutically manipulated to fight infections or cancer. For example, by considering the flexibility of an MHC-bound peptide in conjunction with other peptide characteristics (such as amino acid sequence, prominence, solvent exposure, and affinity for MHC) we may more accurately predict epitope immunogenicity, particularly for neoantigen-based vaccine design (108–112). The use of polypeptide vaccines bearing HLA-restricted CD8+ T cell epitopes is fast gaining traction for cancer immunotherapy (108, 113, 114). The aim is to vaccinate individuals with mutated tumor-associated epitopes (mimotopes) that are then presented by MHC and in doing so stimulate CD8+ T cells that prevent tumor growth. Often mimotopes with enhanced binding to MHC and/or altered TCR interactions elicit a more effective tumor-specific T cell response (115–119) and so their rational design, facilitated by accurately predicting peptide immunogenicity (108, 120, 121), would be highly beneficial.
Likewise, the rational design, or engineering, of the antigen-binding site of TCRs with the same specificity, but enhanced affinity and kinetics for tumor antigens (which are mostly self-derived), has practical implications for soluble TCR-based therapy (113, 122) and adoptive T cell immunotherapies for cancer (123, 124). Both approaches require the considered engineering of high affinity TCRs that maintain their specificity for target tumor antigens. Although this has been accomplished using techniques such as directed evolution (125–130) and structure-based design (58) these experimental approaches are, in tandem, informing the development of computational approaches to predict how to manipulate TCR binding properties (58, 128), and there are indications that the accuracy of these computational approaches is enhanced by incorporating MD simulations for the consideration of protein flexibility (129, 131). However, application of simulation techniques lags markedly behind other fields, so conceptual advances will require highly integrated experimental and computational approaches to fully understand, and exploit, the dynamics of the system.
Author Contributions
AB and NB wrote the manuscript and NB produced Figures 1,2.
Funding
NB is funded by an ARC Future Fellowship (110100223).
Conflict of Interest Statement
The authors declare that the research was conducted in the absence of any commercial or financial relationships that could be construed as a potential conflict of interest.
Acknowledgments
We thank James Fodor and Blake Riley for assistance with Figure 3.
References
1. Ding YH, Baker BM, Garboczi DN, Biddison WE, Wiley DC. Four A6-TCR/peptide/HLA-A2 structures that generate very different T cell signals are nearly identical. Immunity (1999) 11:45–56. doi: 10.1016/S1074-7613(00)80080-1
2. Tynan FE, Borg NA, Miles JJ, Beddoe T, El-Hassen D, Silins SL, et al. High resolution structures of highly bulged viral epitopes bound to major histocompatibility complex class I. Implications for T-cell receptor engagement and T-cell immunodominance. J Biol Chem. (2005) 280:23900–9. doi: 10.1074/jbc.M503060200
3. Reboul CF, Meyer GR, Porebski BT, Borg NA, Buckle AM. Epitope flexibility and dynamic footprint revealed by molecular dynamics of a pMHC-TCR complex. PLoS Comput Biol. (2012) 8:e1002404. doi: 10.1371/journal.pcbi.1002404
4. Borbulevych OY, Piepenbrink KH, Gloor BE, Scott DR, Sommese RF, Cole DK, et al. T cell receptor cross-reactivity directed by antigen-dependent tuning of peptide-MHC molecular flexibility. Immunity (2009) 31:885–96. doi: 10.1016/j.immuni.2009.11.003
5. Insaidoo FK, Borbulevych OY, Hossain M, Santhanagopolan SM, Baxter TK, Baker BM. Loss of T cell antigen recognition arising from changes in peptide and major histocompatibility complex protein flexibility: implications for vaccine design. J Biol Chem. (2011) 286:40163–73. doi: 10.1074/jbc.M111.283564
6. Scott DR, Borbulevych OY, Piepenbrink KH, Corcelli SA, Baker BM. Disparate degrees of hypervariable loop flexibility control T-cell receptor cross-reactivity, specificity, and binding mechanism. J Mol Biol. (2011) 414:385–400. doi: 10.1016/j.jmb.2011.10.006
7. Ayres CM, Corcelli SA, Baker BM. Peptide and peptide-dependent motions in mhc proteins: immunological implications and biophysical underpinnings. Front Immunol. (2017) 8:935. doi: 10.3389/fimmu.2017.00935
8. Van Hateren A, Bailey A, Elliott T. Recent advances in Major Histocompatibility Complex (MHC) class I antigen presentation: plastic MHC molecules and TAPBPR-mediated quality control. F1000Res. (2017) 6:158. doi: 10.12688/f1000research.10474.1
9. Wieczorek M, Abualrous ET, Sticht J, Alvaro-Benito M, Stolzenberg S, Noe F, et al. Major Histocompatibility Complex (MHC) Class I and MHC class II proteins: conformational plasticity in antigen presentation. Front Immunol. (2017) 8:292. doi: 10.3389/fimmu.2017.00292
10. Yanaka S, Sugase K. Exploration of the conformational dynamics of major histocompatibility complex molecules. Front Immunol. (2017) 8:632. doi: 10.3389/fimmu.2017.00632
11. Natarajan K, Jiang J, May NA, Mage MG, Boyd LF, Mcshan AC, et al. The role of molecular flexibility in antigen presentation and T cell receptor-mediated signaling. Front Immunol. (2018) 9:1657. doi: 10.3389/fimmu.2018.01657
12. Sibener LV, Fernandes RA, Kolawole EM, Carbone CB, Liu F, Mcaffee D, et al. Isolation of a structural mechanism for uncoupling T cell receptor signaling from peptide-MHC binding. Cell (2018) 174:672–687.e627. doi: 10.1016/j.cell.2018.06.017
13. Reiser JB, Grégoire C, Darnault C, Mosser T, Guimezanes A, Schmitt-Verhulst AM, et al. A T cell receptor CDR3beta loop undergoes conformational changes of unprecedented magnitude upon binding to a peptide/MHC class I complex. Immunity (2002) 16:345–54. doi: 10.1016/S1074-7613(02)00288-1
14. Tynan FE, Reid HH, Kjer-Nielsen L, Miles JJ, Wilce MC, Kostenko L, et al. A T cell receptor flattens a bulged antigenic peptide presented by a major histocompatibility complex class I molecule. Nat Immunol. (2007) 8:268–76. doi: 10.1038/ni1432
15. Ely KR, Herron JN, Harker M, Edmundson AB. Three-dimensional structure of a light chain dimer crystallized in water. Conformational flexibility of a molecule in two crystal forms. J Mol Biol. (1989) 210:601–15. doi: 10.1016/0022-2836(89)90135-6
16. Faber HR, Matthews BW. A mutant T4 lysozyme displays five different crystal conformations. Nature (1990) 348:263–6. doi: 10.1038/348263a0
17. Eigenbrot C, Randal M, Kossiakoff AA. Structural effects induced by mutagenesis affected by crystal packing factors: the structure of a 30-51 disulfide mutant of basic pancreatic trypsin inhibitor. Proteins (1992) 14:75–87. doi: 10.1002/prot.340140109
18. Tynan FE, Burrows SR, Buckle AM, Clements CS, Borg NA, Miles JJ, et al. T cell receptor recognition of a 'super-bulged' major histocompatibility complex class I-bound peptide. Nat Immunol. (2005) 6:1114–22. doi: 10.1038/ni1257
19. García AE, Krumhansl JA, Frauenfelder H. Variations on a theme by Debye and Waller: from simple crystals to proteins. Prot Struct Funct Bioinformat. (1997) 29:153–60. doi: 10.1002/(SICI)1097-0134(199710)29:2<153::AID-PROT3>3.0.CO;2-E
20. Furnham N, Blundell TL, Depristo MA, Terwilliger TC. Is one solution good enough? Nat Struct Mol Biol. (2006) 13:184–5. doi: 10.1038/nsmb0306-184
21. Woldeyes RA, Sivak DA, Fraser JS. E pluribus unum, no more: from one crystal, many conformations. Curr Opin Struct Biol. (2014) 28:56–62. doi: 10.1016/j.sbi.2014.07.005
22. Van Den Bedem H, Fraser JS. Integrative, dynamic structural biology at atomic resolution [mdash] it's about time. Nat Methods (2015) 12:307–18. doi: 10.1038/nmeth.3324
23. Miles JJ, Borg NA, Brennan RM, Tynan FE, Kjer-Nielsen L, Silins SL, et al. TCRα genes direct MHC restriction in the potent human T cell response to a class I-bound viral epitope. J Immunol. (2006) 177:6804–14. doi: 10.4049/jimmunol.177.10.6804
24. Kjer-Nielsen L, Clements CS, Purcell AW, Brooks AG, Whisstock JC, Burrows SR, et al. A structural basis for the selection of dominant alphabeta T cell receptors in antiviral immunity. Immunity (2003) 18:53–64. doi: 10.1016/S1074-7613(02)00513-7
25. Beddoe T, Chen Z, Clements CS, Ely LK, Bushell SR, Vivian JP, et al. Antigen ligation triggers a conformational change within the constant domain of the alphabeta T cell receptor. Immunity (2009) 30:777–88. doi: 10.1016/j.immuni.2009.03.018
26. Gao GF, Tormo J, Gerth UC, Wyer JR, Mcmichael AJ, Stuart DI, et al. Crystal structure of the complex between human CD8alpha(alpha) and HLA-A2. Nature (1997) 387:630–4. doi: 10.1038/42523
27. Kern PS, Teng MK, Smolyar A, Liu JH, Liu J, Hussey RE, et al. Structural basis of CD8 coreceptor function revealed by crystallographic analysis of a murine CD8alphaalpha ectodomain fragment in complex with H-2Kb. Immunity (1998) 9:519–30. doi: 10.1016/S1074-7613(00)80635-4
28. Wang R, Natarajan K, Margulies DH. Structural basis of the CD8 alpha beta/MHC class I interaction: focused recognition orients CD8 beta to a T cell proximal position. J Immunol. (2009) 183:2554–64. doi: 10.4049/jimmunol.0901276
29. Shi Y, Qi J, Iwamoto A, Gao GF. Plasticity of human CD8alphaalpha binding to peptide-HLA-A*2402. Mol Immunol. (2011) 48:2198–202. doi: 10.1016/j.molimm.2011.05.009
30. Sun ZJ, Kim KS, Wagner G, Reinherz EL. Mechanisms contributing to T cell receptor signaling and assembly revealed by the solution structure of an ectodomain fragment of the CD3 epsilon gamma heterodimer. Cell (2001) 105:913–23. doi: 10.1016/S0092-8674(01)00395-6
31. Arnett KL, Harrison SC, Wiley DC. Crystal structure of a human CD3-epsilon/delta dimer in complex with a UCHT1 single-chain antibody fragment. Proc Natl Acad Sci USA. (2004) 101:16268–73. doi: 10.1073/pnas.0407359101
32. Kjer-Nielsen L, Dunstone MA, Kostenko L, Ely LK, Beddoe T, Mifsud NA, et al. Crystal structure of the human T cell receptor CD3 epsilon gamma heterodimer complexed to the therapeutic mAb OKT3. Proc Natl Acad Sci USA. (2004) 101:7675–80. doi: 10.1073/pnas.0402295101
33. Sun ZY, Kim ST, Kim IC, Fahmy A, Reinherz EL, Wagner G. Solution structure of the CD3epsilondelta ectodomain and comparison with CD3epsilongamma as a basis for modeling T cell receptor topology and signaling. Proc Natl Acad Sci USA. (2004) 101:16867–72. doi: 10.1073/pnas.0407576101
34. Berry R, Headey SJ, Call MJ, Mccluskey J, Tregaskes CA, Kaufman J, et al. Structure of the chicken CD3epsilondelta/gamma heterodimer and its assembly with the alphabetaT cell receptor. J Biol Chem. (2014) 289:8240–51. doi: 10.1074/jbc.M113.544965
35. Chang HC, Tan K, Ouyang J, Parisini E, Liu JH, Le Y, et al. Structural and mutational analyses of a CD8alphabeta heterodimer and comparison with the CD8alphaalpha homodimer. Immunity (2005) 23:661–71. doi: 10.1016/j.immuni.2005.11.002
36. Liu Y, Li X, Qi J, Zhang N, Xia C. The structural basis of chicken, swine and bovine CD8alphaalpha dimers provides insight into the co-evolution with MHC I in endotherm species. Sci Rep. (2016) 6:24788. doi: 10.1038/srep24788
37. He Y, Rangarajan S, Kerzic M, Luo M, Chen Y, Wang Q, et al. Identification of the docking site for CD3 on the T cell receptor beta chain by solution NMR. J Biol Chem. (2015) 290:19796–805. doi: 10.1074/jbc.M115.663799
38. Natarajan A, Nadarajah V, Felsovalyi K, Wang W, Jeyachandran VR, Wasson RA, et al. Structural model of the extracellular assembly of the TCR-CD3 complex. Cell Rep. (2016) 14:2833–45. doi: 10.1016/j.celrep.2016.02.081
39. Blumberg RS, Alarcon B, Sancho J, Mcdermott FV, Lopez P, Breitmeyer J, et al. Assembly and function of the T cell antigen receptor. Requirement of either the lysine or arginine residues in the transmembrane region of the alpha chain. J Biol Chem (1990) 265:14036–43.
40. Call ME, Pyrdol J, Wiedmann M, Wucherpfennig KW. The organizing principle in the formation of the T cell receptor-CD3 complex. Cell (2002) 111:967–79. doi: 10.1016/S0092-8674(02)01194-7
41. Burrows SR, Chen Z, Archbold JK, Tynan FE, Beddoe T, Kjer-Nielsen L, et al. Hard wiring of T cell receptor specificity for the major histocompatibility complex is underpinned by TCR adaptability. Proc Natl Acad Sci USA. (2010) 107:10608–13. doi: 10.1073/pnas.1004926107
42. Yin Y, Wang XX, Mariuzza RA. Crystal structure of a complete ternary complex of T-cell receptor, peptide-MHC, and CD4. Proc Natl Acad Sci USA. (2012) 109:5405–10. doi: 10.1073/pnas.1118801109
43. Li Y, Yin Y, Mariuzza RA. Structural and biophysical insights into the role of CD4 and CD8 in T cell activation. Front Immunol. (2013) 4:206. doi: 10.3389/fimmu.2013.00206
44. Gras S, Chadderton J, Del Campo CM, Farenc C, Wiede F, Josephs TM, et al. Reversed T cell receptor docking on a major histocompatibility class I complex limits involvement in the immune response. Immunity (2016) 45:749–60. doi: 10.1016/j.immuni.2016.09.007
45. Merkle PS, Irving M, Hongjian S, Ferber M, Jorgensen TJD, Scholten K, et al. The T-cell receptor can bind to the peptide-bound major histocompatibility complex and uncomplexed beta2-microglobulin through distinct binding sites. Biochemistry (2017) 56:3945–61. doi: 10.1021/acs.biochem.7b00385
46. Sullivan LC, Walpole NG, Farenc C, Pietra G, Sum MJW, Clements CS, et al. A conserved energetic footprint underpins recognition of human leukocyte antigen-E by two distinct alphabeta T cell receptors. J Biol Chem. (2017) 292:21149–58. doi: 10.1074/jbc.M117.807719
47. Kass I, Buckle AM, Borg NA. Understanding the structural dynamics of TCR-pMHC complex interactions. Trends Immunol. (2014) 35:604–12. doi: 10.1016/j.it.2014.10.005
48. Boniface JJ, Reich Z, Lyons DS, Davis MM. Thermodynamics of T cell receptor binding to peptide-MHC: evidence for a general mechanism of molecular scanning. Proc Natl Acad Sci USA. (1999) 96:11446–51. doi: 10.1073/pnas.96.20.11446
49. Huse M, Klein LO, Girvin AT, Faraj JM, Li Q-J, Kuhns MS, et al. Spatial and temporal dynamics of T cell receptor signaling with a photoactivatable agonist. Immunity (2007) 27:76–88. doi: 10.1016/j.immuni.2007.05.017
50. Dustin ML, Groves JT. Receptor signaling clusters in the immune synapse. Annu Rev Biophys. (2012) 41:543–56. doi: 10.1146/annurev-biophys-042910-155238
51. Brodovitch A, Bongrand P, Pierres A. T lymphocytes sense antigens within seconds and make a decision within one minute. J Immunol. (2013) 191:2064–71. doi: 10.4049/jimmunol.1300523
52. Guedj C, Abraham N, Jullie D, Randriamampita C. T cell adhesion triggers an early signaling pole distal to the immune synapse. J Cell Sci. (2016) 129:2526–37. doi: 10.1242/jcs.182311
53. Zhou R. Replica exchange molecular dynamics method for protein folding simulation. Methods Mol Biol. (2007) 350:205–23. doi: 10.1385/1-59745-189-4:205
54. Ostermeir K, Springer S, Zacharias M. Coupling between side chain interactions and binding pocket flexibility in HLA-B*44:02 molecules investigated by molecular dynamics simulations. Mol Immunol. (2015) 63:312–9. doi: 10.1016/j.molimm.2014.07.021
55. Izrailev S, Stepaniants S, Isralewitz B, Kosztin D, Lu H, Molnar F., et al. Steered molecular dynamics. In: Denflhard P, Hermans J, Leimkuhler B, Mark A, Skeel R, Reich S, editiors. Computational Molecular Dynamics: Challenges, Methods, Ideas. Berlin: Springer-Verlag (1998). p. 39–65.
56. Cuendet MA, Michielin O. Protein-protein interaction investigated by steered molecular dynamics: the TCR-pMHC complex. Biophys J. (2008) 95:3575–90. doi: 10.1529/biophysj.108.131383
57. Cuendet MA, Zoete V, Michielin O. How T cell receptors interact with peptide-MHCs: a multiple steered molecular dynamics study. Proteins (2011) 79:3007–24. doi: 10.1002/prot.23104
58. Zoete V, Irving M, Ferber M, Cuendet MA, Michielin O. Structure-based, rational design of T cell receptors. Front Immunol. (2013) 4:268. doi: 10.3389/fimmu.2013.00268
59. Huang M, Huang W, Wen F, Larson RG. Efficient estimation of binding free energies between peptides and an MHC class II molecule using coarse-grained molecular dynamics simulations with a weighted histogram analysis method. J Comput Chem. (2017) 38:2007–19. doi: 10.1002/jcc.24845
60. Marrink SJ, Risselada HJ, Yefimov S, Tieleman DP, De Vries AH. The MARTINI force field: coarse grained model for biomolecular simulations. J Phys Chem B (2007) 111:7812–24. doi: 10.1021/jp071097f
61. Chen J, Xie ZR, Wu Y. Study of protein structural deformations under external mechanical perturbations by a coarse-grained simulation method. Biomech Model Mechanobiol. (2016) 15:317–29. doi: 10.1007/s10237-015-0690-0
62. Wan S, Flower DR, Coveney PV. Toward an atomistic understanding of the immune synapse: large-scale molecular dynamics simulation of a membrane-embedded TCR-pMHC-CD4 complex. Mol Immunol. (2008) 45:1221–30. doi: 10.1016/j.molimm.2007.09.022
63. Lange OF, Van Der Spoel D, De Groot BL. Scrutinizing molecular mechanics force fields on the submicrosecond timescale with NMR data. Biophys J. (2010) 99:647–55. doi: 10.1016/j.bpj.2010.04.062
64. Piana S, Lindorff-Larsen K, Shaw DE. How robust are protein folding simulations with respect to force field parameterization? Biophys J. (2011) 100:L47–L49. doi: 10.1016/j.bpj.2011.03.051
65. Lindorff-Larsen K, Maragakis P, Piana S, Eastwood MP, Dror RO, Shaw DE. Systematic validation of protein force fields against experimental data. PloS ONE (2012) 7:e32131. doi: 10.1371/journal.pone.0032131
66. Pande VS, Beauchamp K, Bowman GR. Everything you wanted to know about Markov State Models but were afraid to ask. Methods (2010) 52:99–105. doi: 10.1016/j.ymeth.2010.06.002
67. Levin EJ, Kondrashov DA, Wesenberg GE, Phillips GNJr. Ensemble refinement of protein crystal structures: validation and application. Structure (2007) 15:1040–52. doi: 10.1016/j.str.2007.06.019
68. Burnley BT, Afonine PV, Adams PD, Gros P. Modelling dynamics in protein crystal structures by ensemble refinement. Elife (2012) 1:e00311. doi: 10.7554/eLife.00311
69. Forneris F, Burnley BT, Gros P. Ensemble refinement shows conformational flexibility in crystal structures of human complement factor D. Acta Crystallogr Sect D (2014) 70:733–43. doi: 10.1107/S1399004713032549
70. Weerth RS, Michalska K, Bingman CA, Yennamalli RM, Li H, Jedrzejczak R, et al. Structure of a cupin protein Plu4264 from photorhabdus luminescens subsp. laumondii TTO1 at 1.35 A resolution. Proteins (2015) 83:383–8. doi: 10.1002/prot.24705
71. Cao H, Tan K, Wang F, Bigelow L, Yennamalli RM, Jedrzejczak R, et al. Structural dynamics of a methionine gamma-lyase for calicheamicin biosynthesis: rotation of the conserved tyrosine stacking with pyridoxal phosphate. Struct Dyn. (2016) 3:034702. doi: 10.1063/1.4948539
72. Correy GJ, Carr PD, Meirelles T, Mabbitt PD, Fraser NJ, Weik M, et al. Mapping the accessible conformational landscape of an insect carboxylesterase using conformational ensemble analysis and kinetic crystallography. Structure (2016) 24:977–87. doi: 10.1016/j.str.2016.04.009
73. Fodor J, Riley BT, Borg NA, Buckle AM. Previously hidden dynamics at the TCR-Peptide-MHC interface revealed. J Immunol. (2018) 200:4134–45. doi: 10.4049/jimmunol.1800315
74. Csermely P, Palotai R, Nussinov R. Induced fit, conformational selection and independent dynamic segments: an extended view of binding events. Trends Biochem Sci. (2010) 35:539–46. doi: 10.1016/j.tibs.2010.04.009
75. Borbulevych OY, Piepenbrink KH, Baker BM. Conformational melding permits a conserved binding geometry in TCR recognition of foreign and self molecular mimics. J Immunol. (2011) 186:2950–8. doi: 10.4049/jimmunol.1003150
76. Adams PD, Afonine PV, Bunkóczi G, Chen VB, Davis IW, Echols N, et al. PHENIX: a comprehensive Python-based system for macromolecular structure solution. Acta Crystallogr. Sect D (2010) 66:213–21. doi: 10.1107/S0907444909052925
77. Insaidoo FK, Zajicek J, Baker BM. A general and efficient approach for NMR studies of peptide dynamics in class I MHC peptide binding grooves. Biochemistry (2009) 48:9708–10. doi: 10.1021/bi9008787
78. Hawse WF, De S, Greenwood AI, Nicholson LK, Zajicek J, Kovrigin EL, et al. TCR scanning of peptide/MHC through complementary matching of receptor and ligand molecular flexibility. J Immunol. (2014) 192:2885–91. doi: 10.4049/jimmunol.1302953
79. Natarajan K, Mcshan AC, Jiang J, Kumirov VK, Wang R, Zhao H, et al. An allosteric site in the T-cell receptor Cbeta domain plays a critical signalling role. Nat Commun. (2017) 8:15260. doi: 10.1038/ncomms15260
80. Beerbaum M, Ballaschk M, Erdmann N, Schnick C, Diehl A, Uchanska-Ziegler B, et al. NMR spectroscopy reveals unexpected structural variation at the protein-protein interface in MHC class I molecules. J Biomol NMR (2013) 57:167–78. doi: 10.1007/s10858-013-9777-z
81. Rangarajan S, He Y, Chen Y, Kerzic MC, Ma B, Gowthaman R, et al. Peptide-MHC (pMHC) binding to a human antiviral T cell receptor induces long-range allosteric communication between pMHC- and CD3-binding sites. J Biol Chem. (2018) 293:15991–6005. doi: 10.1074/jbc.RA118.003832
82. Cooper A, Dryden DT. Allostery without conformational change. A plausible model. Eur Biophys J. (1984) 11:103–9. doi: 10.1007/BF00276625
83. Smock RG, Gierasch LM. Sending signals dynamically. Science (2009) 324:198–203. doi: 10.1126/science.1169377
84. Motlagh HN, Wrabl JO, Li J, Hilser VJ. The ensemble nature of allostery. Nature (2014) 508:331–9. doi: 10.1038/nature13001
85. Hawse WF, Gloor BE, Ayres CM, Kho K, Nuter E, Baker BM. Peptide modulation of class I major histocompatibility complex protein molecular flexibility and the implications for immune recognition. J Biol Chem. (2013) 288:24372–81. doi: 10.1074/jbc.M113.490664
86. Kozono H, Matsushita Y, Ogawa N, Kozono Y, Miyabe T, Sekiguchi H, et al. Single-Molecule Motions of MHC class II rely on bound peptides. Biophys J. (2015) 108:350–9. doi: 10.1016/j.bpj.2014.12.004
87. Pohlmann T, Bockmann RA, Grubmuller H, Uchanska-Ziegler B, Ziegler A, Alexiev U. Differential peptide dynamics is linked to major histocompatibility complex polymorphism. J Biol Chem. (2004) 279:28197–201. doi: 10.1074/jbc.C400128200
88. Gakamsky DM, Lewitzki E, Grell E, Saulquin X, Malissen B, Montero-Julian F, et al. Kinetic evidence for a ligand-binding-induced conformational transition in the T cell receptor. Proc Natl Acad Sci USA. (2007) 104:16639–44. doi: 10.1073/pnas.0707061104
89. Huppa JB, Axmann M, Mortelmaier MA, Lillemeier BF, Newell EW, Brameshuber M, et al. TCR-peptide-MHC interactions in situ show accelerated kinetics and increased affinity. Nature (2010) 463:963–7. doi: 10.1038/nature08746
90. Schutz GJ, Huppa JB. Forster resonance energy transfer to study TCR-pMHC interactions in the immunological synapse. Methods Mol Biol. (2017) 1584:207–29. doi: 10.1007/978-1-4939-6881-7_14
91. Matsunaga Y, Sugita Y. Linking time-series of single-molecule experiments with molecular dynamics simulations by machine learning. Elife (2018) 7:e32668. doi: 10.7554/eLife.32668
92. Yachi PP, Ampudia J, Zal T, Gascoigne NR. Altered peptide ligands induce delayed CD8-T cell receptor interaction–a role for CD8 in distinguishing antigen quality. Immunity (2006) 25:203–11. doi: 10.1016/j.immuni.2006.05.015
93. Ma Y, Pandzic E, Nicovich PR, Yamamoto Y, Kwiatek J, Pageon SV, et al. An intermolecular FRET sensor detects the dynamics of T cell receptor clustering. Nat Commun. (2017) 8:15100. doi: 10.1038/ncomms15100
94. Van Hateren A, Anderson M, Bailey A, Werner JM, Skipp P, Elliott T. Direct evidence for conformational dynamics in major histocompatibility complex class I molecules. J Biol Chem. (2017) 292:20255–69. doi: 10.1074/jbc.M117.809624
95. Fabian H, Huser H, Narzi D, Misselwitz R, Loll B, Ziegler A, et al. HLA-B27 subtypes differentially associated with disease exhibit conformational differences in solution. J Mol Biol. (2008) 376:798–810. doi: 10.1016/j.jmb.2007.12.009
96. Hawse WF, Champion MM, Joyce MV, Hellman LM, Hossain M, Ryan V, et al. Cutting edge: evidence for a dynamically driven T cell signaling mechanism. J Immunol. (2012) 188:5819–23. doi: 10.4049/jimmunol.1200952
97. Putnam CD, Hammel M, Hura GL, Tainer JA. X-ray solution scattering (SAXS) combined with crystallography and computation: defining accurate macromolecular structures, conformations and assemblies in solution. Q Rev Biophys. (2007) 40:191–285. doi: 10.1017/S0033583507004635
98. Zarutskie JA, Sato AK, Rushe MM, Chan IC, Lomakin A, Benedek GB, et al. A conformational change in the human major histocompatibility complex protein HLA-DR1 induced by peptide binding. Biochemistry (1999) 38:5878–87. doi: 10.1021/bi983048m
99. Yin L, Trenh P, Guce A, Wieczorek M, Lange S, Sticht J, et al. Susceptibility to HLA-DM protein is determined by a dynamic conformation of major histocompatibility complex class II molecule bound with peptide. J Biolog Chem. (2014) 289:23449–64. doi: 10.1074/jbc.M114.585539
100. Ashish JIJ, Garg R, Boone CD, Anguita J, Krueger JK. Conformational rearrangement within the soluble domains of the CD4 receptor is ligand-specific. J Biol Chem. (2008) 283:2761–72. doi: 10.1074/jbc.M708325200
101. Zimmermann K, Liechti T, Haas A, Rehr M, Trkola A, Gunthard HF, et al. The orientation of HIV-1 gp120 binding to the CD4 receptor differentially modulates CD4+ T cell activation. J Immunol. (2015) 194:637–49. doi: 10.4049/jimmunol.1401863
102. Tria G, Mertens HD, Kachala M, Svergun DI. Advanced ensemble modelling of flexible macromolecules using X-ray solution scattering. IUCrJ. (2015) 2:207–17. doi: 10.1107/S205225251500202X
103. Schaeffer RD, Fersht A, Daggett V. Combining experiment and simulation in protein folding: closing the gap for small model systems. Curr Opin Struct Biol. (2008) 18:4–9. doi: 10.1016/j.sbi.2007.11.007
104. Shaw DE, Maragakis P, Lindorff-Larsen K, Piana S, Dror RO, Eastwood MP, et al. Atomic-level characterization of the structural dynamics of proteins. Science (2010) 330:341–6. doi: 10.1126/science.1187409
105. Best RB. Atomistic molecular simulations of protein folding. Curr Opin Struct Biol. (2012) 22:52–61. doi: 10.1016/j.sbi.2011.12.001
106. Birnbaum ME, Berry R, Hsiao YS, Chen Z, Shingu-Vazquez MA, Yu X, et al. Molecular architecture of the alphabeta T cell receptor-CD3 complex. Proc Natl Acad Sci USA. (2014) 111:17576–81. doi: 10.1073/pnas.1420936111
107. Park S, Krshnan L, Call MJ, Call ME, Im W. Structural conservation and effects of alterations in T cell receptor transmembrane interfaces. Biophys J. (2018) 114:1030–5. doi: 10.1016/j.bpj.2018.01.004
108. Balachandran VP, Luksza M, Zhao JN, Makarov V, Moral JA, Remark R, et al. Identification of unique neoantigen qualities in long-term survivors of pancreatic cancer. Nature (2017) 551:512–6. doi: 10.1038/nature24462
109. Lauss M, Donia M, Harbst K, Andersen R, Mitra S, Rosengren F, et al. Mutational and putative neoantigen load predict clinical benefit of adoptive T cell therapy in melanoma. Nat Commun. (2017) 8:1738. doi: 10.1038/s41467-017-01460-0
110. Wirth TC, Kuhnel F. Neoantigen targeting-dawn of a new era in cancer immunotherapy? Front Immunol. (2017) 8:1848. doi: 10.3389/fimmu.2017.01848
111. Aurisicchio L, Pallocca M, Ciliberto G, Palombo F. The perfect personalized cancer therapy: cancer vaccines against neoantigens. J Exp Clin Cancer Res. (2018) 37:86. doi: 10.1186/s13046-018-0751-1
112. Nogueira C, Kaufmann JK, Lam H, Flechtner JB. Improving cancer immunotherapies through empirical neoantigen selection. Trends Cancer (2018) 4:97–100. doi: 10.1016/j.trecan.2017.12.003
113. Boudousquie C, Bossi G, Hurst JM, Rygiel KA, Jakobsen BK, Hassan NJ. Polyfunctional response by ImmTAC (IMCgp100) redirected CD8(+) and CD4(+) T cells. Immunology (2017) 152:425–38. doi: 10.1111/imm.12779
114. Kuai R, Ochyl LJ, Bahjat KS, Schwendeman A, Moon JJ. Designer vaccine nanodiscs for personalized cancer immunotherapy. Nat Mater. (2017) 16:489–96. doi: 10.1038/nmat4822
115. Parkhurst MR, Salgaller ML, Southwood S, Robbins PF, Sette A, Rosenberg SA, et al. Improved induction of melanoma-reactive CTL with peptides from the melanoma antigen gp100 modified at HLA-A*0201-binding residues. J Immunol. (1996) 157:2539–48.
116. Zaremba S, Barzaga E, Zhu M, Soares N, Tsang KY, Schlom J. Identification of an enhancer agonist cytotoxic T lymphocyte peptide from human carcinoembryonic antigen. Cancer Res. (1997) 57:4570–7.
117. Salazar E, Zaremba S, Arlen PM, Tsang KY, Schlom J. Agonist peptide from a cytotoxic t-lymphocyte epitope of human carcinoembryonic antigen stimulates production of tc1-type cytokines and increases tyrosine phosphorylation more efficiently than cognate peptide. Int J Cancer (2000) 85:829–38. doi: 10.1002/(SICI)1097-0215(20000315)85:6<829::AID-IJC16>3.0.CO;2-K
118. Borbulevych OY, Baxter TK, Yu Z, Restifo NP, Baker BM. Increased immunogenicity of an anchor-modified tumor-associated antigen is due to the enhanced stability of the peptide/MHC complex: implications for vaccine design. J Immunol. (2005) 174:4812–20. doi: 10.4049/jimmunol.174.8.4812
119. Mcmahan RH, Mcwilliams JA, Jordan KR, Dow SW, Wilson DB, Slansky JE. Relating TCR-peptide-MHC affinity to immunogenicity for the design of tumor vaccines. J Clin Invest. (2006) 116:2543–51. doi: 10.1172/JCI26936
120. Rapin N, Lund O, Bernaschi M, Castiglione F. Computational immunology meets bioinformatics: the use of prediction tools for molecular binding in the simulation of the immune system. PLoS ONE (2010) 5:e9862. doi: 10.1371/journal.pone.0009862
121. Matsushita H, Vesely MD, Koboldt DC, Rickert CG, Uppaluri R, Magrini VJ, et al. Cancer exome analysis reveals a T-cell-dependent mechanism of cancer immunoediting. Nature (2012) 482:400–4. doi: 10.1038/nature10755
122. Mccormack E, Adams KJ, Hassan NJ, Kotian A, Lissin NM, Sami M, et al. Bi-specific TCR-anti CD3 redirected T-cell targeting of NY-ESO-1- and LAGE-1-positive tumors. Cancer Immunol Immunother. (2013) 62:773–85. doi: 10.1007/s00262-012-1384-4
123. Robbins PF, Morgan RA, Feldman SA, Yang JC, Sherry RM, Dudley ME, et al. Tumor regression in patients with metastatic synovial cell sarcoma and melanoma using genetically engineered lymphocytes reactive with NY-ESO-1. J Clin Oncol. (2011) 29:917–24. doi: 10.1200/JCO.2010.32.2537
124. Rapoport AP, Stadtmauer EA, Binder-Scholl GK, Goloubeva O, Vogl DT, Lacey SF, et al. NY-ESO-1-specific TCR-engineered T cells mediate sustained antigen-specific antitumor effects in myeloma. Nat Med. (2015) 21:914–21. doi: 10.1038/nm.3910
125. Holler PD, Holman PO, Shusta EV, O'herrin S, Wittrup KD, Kranz DM. In vitro evolution of a T cell receptor with high affinity for peptide/MHC. Proc Natl Acad Sci USA. (2000) 97:5387–92. doi: 10.1073/pnas.080078297
126. Li Y, Moysey R, Molloy PE, Vuidepot AL, Mahon T, Baston E, et al. Directed evolution of human T-cell receptors with picomolar affinities by phage display. Nat Biotechnol. (2005) 23:349–54. doi: 10.1038/nbt1070
127. Dunn SM, Rizkallah PJ, Baston E, Mahon T, Cameron B, Moysey R, et al. Directed evolution of human T cell receptor CDR2 residues by phage display dramatically enhances affinity for cognate peptide-MHC without increasing apparent cross-reactivity. Protein Sci. (2006) 15:710–21. doi: 10.1110/ps.051936406
128. Pierce BG, Hellman LM, Hossain M, Singh NK, Vander Kooi CW, Weng Z, et al. Computational design of the affinity and specificity of a therapeutic T cell receptor. PLoS Comput Biol. (2014) 10:e1003478. doi: 10.1371/journal.pcbi.1003478
129. Smith SN, Wang Y, Baylon JL, Singh NK, Baker BM, Tajkhorshid E, et al. Changing the peptide specificity of a human T-cell receptor by directed evolution. Nat Commun. (2014) 5:5223. doi: 10.1038/ncomms6223
130. Harris DT, Wang N, Riley TP, Anderson SD, Singh NK, Procko E, et al. Deep mutational scans as a guide to engineering high affinity T cell receptor interactions with peptide-bound major histocompatibility complex. J Biol Chem. (2016) 291:24566–78. doi: 10.1074/jbc.M116.748681
Keywords: TCR, MHC, conformational dynamics, T cell activation, immune synapse, TCR recognition
Citation: Buckle AM and Borg NA (2018) Integrating Experiment and Theory to Understand TCR-pMHC Dynamics. Front. Immunol. 9:2898. doi: 10.3389/fimmu.2018.02898
Received: 19 September 2018; Accepted: 26 November 2018;
Published: 07 December 2018.
Edited by:
Jorge Bernardino De La Serna, United Kingdom Research and Innovation, United KingdomReviewed by:
Rainer A. Böckmann, Friedrich-Alexander-Universität Erlangen-Nürnberg, GermanyBrian M. Baker, University of Notre Dame, United States
Copyright © 2018 Buckle and Borg. This is an open-access article distributed under the terms of the Creative Commons Attribution License (CC BY). The use, distribution or reproduction in other forums is permitted, provided the original author(s) and the copyright owner(s) are credited and that the original publication in this journal is cited, in accordance with accepted academic practice. No use, distribution or reproduction is permitted which does not comply with these terms.
*Correspondence: Ashley M. Buckle, YXNobGV5LmJ1Y2tsZUBtb25hc2guZWR1
Natalie A. Borg, bmF0YWxpZS5ib3JnQG1vbmFzaC5lZHU=